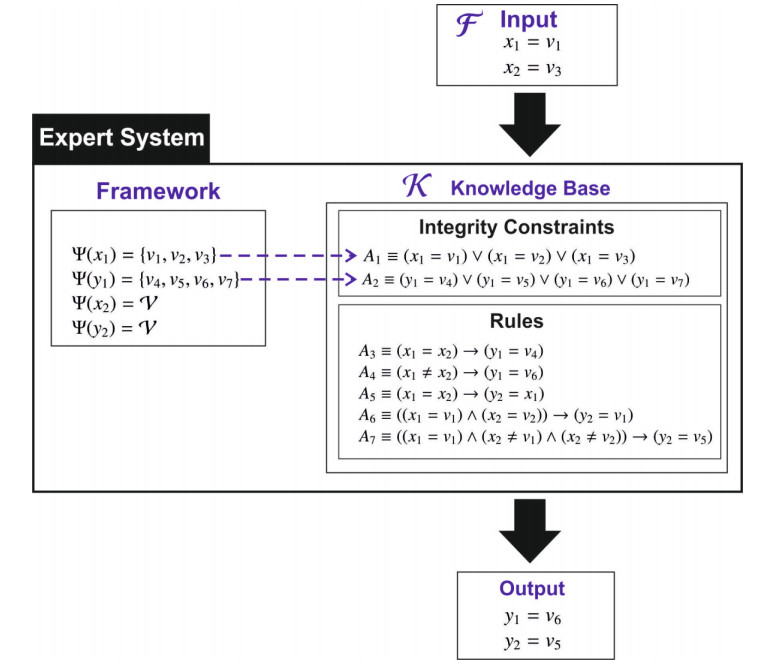
The collaboration of private companies in the fulfillment of the Sustainable Development Goals (SDGs) is key to address global challenges of climate change, social inequality and environmental degradation. This collaboration can also boost their own organizational performance. However, the research on the relationship between SDG commitment and organizational performance remains inconclusive. The diversity of findings could stem from cross-cultural differences in corporate environments. The aim of this study, therefore, was to analyze the interaction between SDG commitment and organizational performance and to examine how this interaction is influenced by cultural factors. Using simultaneous equation modeling on a sample of 3,420 companies from 30 countries for the period 2015 to 2020, our results show that engagement with SDGs has an impact on organizational performance levels which is further enhanced by the catalytic effect of certain cultural factors.
Citation: Ana Bellostas, Cristina Del Río, Karen González-Álvarez, Francisco J López-Arceiz. Cultural context, organizational performance and Sustainable Development Goals: A pending task[J]. Green Finance, 2023, 5(2): 211-239. doi: 10.3934/GF.2023009
[1] | Muhammad Ihsan, Muhammad Saeed, Atiqe Ur Rahman, Mazin Abed Mohammed, Karrar Hameed Abdulkaree, Abed Saif Alghawli, Mohammed AA Al-qaness . An innovative decision-making framework for supplier selection based on a hybrid interval-valued neutrosophic soft expert set. AIMS Mathematics, 2023, 8(9): 22127-22161. doi: 10.3934/math.20231128 |
[2] | Muhammad Ihsan, Muhammad Saeed, Atiqe Ur Rahman, Hüseyin Kamacı, Nehad Ali Shah, Wajaree Weera . An MADM-based fuzzy parameterized framework for solar panels evaluation in a fuzzy hypersoft expert set environment. AIMS Mathematics, 2023, 8(2): 3403-3427. doi: 10.3934/math.2023175 |
[3] | Muhammad Ihsan, Muhammad Saeed, Alhanouf Alburaikan, Hamiden Abd El-Wahed Khalifa . Product evaluation through multi-criteria decision making based on fuzzy parameterized Pythagorean fuzzy hypersoft expert set. AIMS Mathematics, 2022, 7(6): 11024-11052. doi: 10.3934/math.2022616 |
[4] | Murugan Palanikumar, Nasreen Kausar, Harish Garg, Aiyared Iampan, Seifedine Kadry, Mohamed Sharaf . Medical robotic engineering selection based on square root neutrosophic normal interval-valued sets and their aggregated operators. AIMS Mathematics, 2023, 8(8): 17402-17432. doi: 10.3934/math.2023889 |
[5] | Nurshazneem Roslan, Saratha Sathasivam, Farah Liyana Azizan . Conditional random k satisfiability modeling for k = 1, 2 (CRAN2SAT) with non-monotonic Smish activation function in discrete Hopfield neural network. AIMS Mathematics, 2024, 9(2): 3911-3956. doi: 10.3934/math.2024193 |
[6] | Nurul Atiqah Romli, Nur Fariha Syaqina Zulkepli, Mohd Shareduwan Mohd Kasihmuddin, Nur Ezlin Zamri, Nur 'Afifah Rusdi, Gaeithry Manoharam, Mohd. Asyraf Mansor, Siti Zulaikha Mohd Jamaludin, Amierah Abdul Malik . Unsupervised logic mining with a binary clonal selection algorithm in multi-unit discrete Hopfield neural networks via weighted systematic 2 satisfiability. AIMS Mathematics, 2024, 9(8): 22321-22365. doi: 10.3934/math.20241087 |
[7] | Xue Jiang, Yihe Gong . Algorithms for computing Gröbner bases of ideal interpolation. AIMS Mathematics, 2024, 9(7): 19459-19472. doi: 10.3934/math.2024948 |
[8] | Ahmet S. Cevik, Eylem G. Karpuz, Hamed H. Alsulami, Esra K. Cetinalp . A Gröbner-Shirshov basis over a special type of braid monoids. AIMS Mathematics, 2020, 5(5): 4357-4370. doi: 10.3934/math.2020278 |
[9] | Aneeza Imtiaz, Umer Shuaib . On conjunctive complex fuzzification of Lagrange's theorem of ξ−CFSG. AIMS Mathematics, 2023, 8(8): 18881-18897. doi: 10.3934/math.2023961 |
[10] | Sohail Ahmad, Ponam Basharat, Saleem Abdullah, Thongchai Botmart, Anuwat Jirawattanapanit . MABAC under non-linear diophantine fuzzy numbers: A new approach for emergency decision support systems. AIMS Mathematics, 2022, 7(10): 17699-17736. doi: 10.3934/math.2022975 |
The collaboration of private companies in the fulfillment of the Sustainable Development Goals (SDGs) is key to address global challenges of climate change, social inequality and environmental degradation. This collaboration can also boost their own organizational performance. However, the research on the relationship between SDG commitment and organizational performance remains inconclusive. The diversity of findings could stem from cross-cultural differences in corporate environments. The aim of this study, therefore, was to analyze the interaction between SDG commitment and organizational performance and to examine how this interaction is influenced by cultural factors. Using simultaneous equation modeling on a sample of 3,420 companies from 30 countries for the period 2015 to 2020, our results show that engagement with SDGs has an impact on organizational performance levels which is further enhanced by the catalytic effect of certain cultural factors.
Expert systems are computational programs designed to emulate the decision-making process of human experts within a specific field. One effective method for representing knowledge in such a system is through propositional logic, where knowledge inference is intrinsically linked to the concept of Tautological consequence. Utilizing a mathematical result [1] based on prior work [2,3,4,5,6], this issue can be converted into an algebraic problem involving the calculation of certain Gröbner bases [7,8]. Consequently, expert systems predicated on propositional logic can be readily implemented using a computer algebra system like CoCoA [9]. This approach has facilitated the development of various expert systems in recent years [10,11,12,13,14,15,16,17,18,19].
The 'Concept-Attribute-Value' paradigm provides an alternative method for representing knowledge in Expert Systems. This approach is often more natural and efficient, offering several advantages over propositional logic. When the possible values of attributes can only take a limited set of values, knowledge inference under this paradigm can be translated into algebraic terms [20]. This translation facilitates the implementation of an Expert System based on the 'Concept-Attribute-Value' paradigm within Computer Algebra Systems. Despite the differences between the algebraic approach based on Boolean propositional logic and the 'Concept-Attribute-Value' paradigm, they are in some ways equivalent. Specifically, any Expert System that can be implemented in one model can also be implemented in the other, provided that attributes can assume only a finite set of values [21].
However, there are instances where it is not feasible to impose this limitation, necessitating the consideration of scenarios where an attribute, x, can assume one value from an infinite (or very large finite) set. In such cases, we may encounter formulae like (x≠2 or x≠0→y=0). In these circumstances, the expert system cannot be represented using propositional logic, as x can take on an infinite number of possible values. Up until now, no method had been found to implement it on a Computer Algebra System. However, we have made a breakthrough and will present our newly discovered method in this paper.
In this paper, we address these specific constraints and introduce an innovative algebraic methodology for the development of expert systems. This methodology takes into account variables or attributes that are capable of assuming a value from an infinite set. As we will demonstrate, this model offers a fresh perspective on previous results, to the point where they can be viewed as specific instances of our proposed solution.
The structure of this paper is outlined as follows: In Section, we conduct a comparative analysis of our models with related ones. Section 3 introduces the representation formalism used to implement expert systems, wherein an attribute can take on a value from an infinite set. In Section 4, we provide proofs related to the proposed algebraic model for implementing this type of expert system. Section 5 provides a deeper understanding of our proposed algebraic model. This model is illustrated using a real-world example: A railway interlocking system that can identify hazardous situations in a railway station (see Section 6 for details). Finally, in Section 7, we summarize our conclusions and discuss the potential implications of our findings.
Our approach is centered on harnessing the capabilities of Computer Algebra Systems for the swift and effective development of expert systems. These systems represent knowledge, input and output through polynomials on multiple variables. By employing a representation paradigm based on propositional logic or 'Concept-Attribute-Value', we can translate knowledge using these polynomials. These algebraic models frame the problem of determining the system's output as an algebraic problem, typically the ideal membership problem, which can be resolved using Gröbner bases. In instances where we utilize propositional logic that accommodates uncertainty, we can deploy expert systems that handle these situations, making it particularly suitable in fields like medicine.
However, all these algebraic models are constrained by the assumption that the values of the variables, or attributes, necessary for knowledge representation must be confined to a potentially finite set (in the case of propositional logic, all variables are Boolean, with possible values {True, False}).
The novelty of our paper lies in its provision of an algebraic model that accommodates variables not restricted to a potentially finite set of values, allowing for an infinite range of potential variable values. In such cases, our model, unlike its predecessors, can implement these systems with Computer Algebra Systems using the proposed algebraic model. In essence, our approach extends previous models.
We believe our strategy can be applied to various expert systems where uncertainty is not a factor. It proves especially effective for decision trees that culminate in a finite set of outputs rather than intermediate steps and results with fluctuating levels of certainty.
However, our model does present some limitations:
● Our model does not account for uncertainty in knowledge. We plan to extend our algebraic model to consider uncertainty in future work.
● Our strategy has some limitations when variables take on a value from an infinite set. We have only considered relations between variables with equality (xi=xj) and inequality (xi≠xj). The ability to represent order relations such as xi>xj is currently beyond our scope.
Similar to preceding algebraic models, our model relies on the computation of Gröbner bases, thus presenting the same degree of complexity, and the inference engine operates within comparable time frames. However, in instances where all variables are Boolean, we can employ computer algebra systems like Polybori [22], which are tailored for Boolean polynomials, leading to significantly more efficient systems.
Despite these limitations, our strategy provides a framework that can be readily applied in scenarios where systems do not incorporate uncertainty. In this paper, we have demonstrated a practical application of our strategy in addressing interlocking problems (see Section 6).
Like previous algebraic models, our approach implements an expert system through a Computer Algebra System. While our approach is of theoretical interest, it shares a practical limitation with previous models. Since Computer Algebra Systems have not been certified for use in safety-critical implementations, systems developed with our framework cannot be integrated with safety instrumented system computers to achieve the targeted Safety Integrity Level (SIL). However, the results may prove beneficial for simulations that do not require certification credit.
In this section, we will explore the fundamental concepts and principles that underpin the algebraic methodologies employed in the development of Expert Systems within Computer Algebra Systems. Expert Systems are computational programs characterized by three core components:
Input. The input of the expert system is the collection of facts observed in the environment. The set is denoted by the symbol F.
Knowledge−Base. The knowledge-base encapsulates the information stored within the system. This information, used in conjunction with the input of the expert system, is used to inference the output of the system. The knowledge, which mirrors that of an expert, is expressed as a finite set of formulae K.
Output. The output of the classification expert system constitutes the knowledge inferred from the input and the knowledge base within the expert system.
Each of the three components within an expert system necessitates the representation of knowledge. Analogous to Propositional Logic, we posit that knowledge in an expert system is depicted through a finite set of variables x1…xN. However, diverging from propositional logic, we do not confine variables to Boolean values; in fact, variables may assume a value from an infinite set of values. In the following definition, we will formally establish the conceptual framework of an expert system
Definition 3.1 (Conceptual Framework). A conceptual ground is (X,V,Ψ) where X is a finite set of possible 'variables', V is a (non-necessary) finite set of possible 'values' and Ψ is a function X⟶P(V) where P(V) represents the power set of V. Ψ(x) represents the possible values that the variable x may take.
The aforementioned definition encapsulates the expert system predicated on Propositional Logic. In the context of Propositional Logic, as per Definition 3.1, we have X=x1…xN, V={True,False} and each variable xi assumes the potential values {True,False}, that is to say, Ψ(xi)={True,False}.
The information of the environment is represented by means of states. A state is an instantiation of the conceptual framework: every variable xi takes a value from the set of its potential values Ψ(xi). Formally:
Definition 3.2 (State). A state S is defined as a function S:X⟶V. We designate S as the set encompassing all states.
Given a state, s∈S, we can state relations between the variables X by means of formulae (in the same way, as formulae in propositional logic relates boolean variables).
Definition 3.3 (Formula). A formula is defined as follows:
● Positive Atomic Formula.
– x=v, where x∈X is a variable and v∈Ψ(x) is a possible value of x.
– x=y, where x,y∈X are variables.
● Negative Atomic Formula.
– x≠v, where x∈X is a variable and v∈Ψ(x) is a possible value of x.
– x≠y, where x,y∈X are variables.
● Disjunctive of atomic formulae:
A1∨...∨Ar |
where A1,...,Ar are positive or negative atomic formulae.
We designate C as the set encompassing all formulae.
Definition 3.4. Given an atomic formula A, we will denote the atomic formula ¬A as the following formula:
Case A≡(x=v) where x is a variable and v∈Ψ(x).
¬A≡(x≠v) |
Case A≡(x≠v) where x is a variable and v∈Ψ(x).
¬A≡(x=v) |
Case A≡(x=y) where x and y are variables.
¬A≡(x≠y) |
Case A≡(x≠y) where x and y are variables.
¬A≡(x=y) |
Notation 3.1. Rules serve as the conventional method for representing knowledge within an expert system and are incorporated into the preceding definition. Similar to Propositional Logic, we employ the notation of rules
(A1∧...∧Ar)⟶(B1∨...∨Bs) |
(where A1,...,Ar,B1,...Bs are atomic formulae) to denote the formula:
¬A1∨...∨¬Ar∨B1∨...∨Bs |
We can now formally define the components of an expert system: Input, Knowledge-base and Output:
Definition 3.5. We formally define the three components of an expert system as:
● Input. This is a finite set of positive atomic formulae, denoted as F⊂C
● Knowledge-base. This is a set of formulae denoted as K⊂C. The knowledge base comprises two types of formulae:
Integrity Constraints. For each variable xi that can only assume a finite set of potential values, i.e., Ψ(xi)={v1…vm}, the following formula represents the intrinsic integrity constraint:
(x1=v1)∨(x1=v2)∨…∨(x1=vm) |
Rules. These are formulae expressed via rules (provided by a human expert)
● Output. This is a finite set of atomic formulae.
Figure 1 depicts the components of the expert system. As may be seen, integrity constraints are intuitively and implicitly derived from the inherent nature of variables and they are immediately obtained by the function Ψ of the conceptual framework (see Definition 3.1). For instance, a boolean variable, x, is associated with the integrity constraint x=False∨x=True because Ψ(x)={True, False}, which signifies that x must assume one of two possible values: True or False. Similarly, if x denotes the current colour of a semaphore, we would have the integrity constraint x=red∨x=orange∨x=green, indicating that the semaphore's colour can be either red, orange, or green, which involves that Ψ(x)={red, orange, green}. In Figure 1, the variable x1, with Ψ(x1)={v1,v2,v3}, is associated with the integrity constraint A1≡(x1=v1)∨(x1=v2)∨(x1=v3).
On the other hand, rules are formulas explicitly provided by a human expert. The acquisition of these rules is not a straightforward task. It necessitates a process that involves multiple interviews with experts, not only to elucidate these rules from them but also to validate the completeness and accuracy of the system. Although we provide a theorem that can be used to verify if the system is at least consistent (see Theorem 4.2), we do not delve into the process of elucidating these rules. Instead, our focus will be on the design of an inference engine based on an algebraic representation through polynomials, which allows for the automatic deduction of the system's output (as we will demonstrate in Section 4.2). In this way, the system's output is correct, as we will demonstrate, in the sense that it is deduced from the facts and rules.
Next, we will establish the semantics of formulae:
Definition 3.3 (Holds). Let A∈C be a formula. Let S∈S be a state
We say that the formula A holds in the state S if and only if:
Case A≡x=v where x∈X and v∈Ψ(x).
A holds in S⇔S(x)=v.
Case A≡x≠v where x∈X and v∈Ψ(x).
A holds in S⇔S(x)≠v.
Case A≡x=y where x,y∈X.
A holds in S⇔S(x)=S(y).
Case A≡x≠y where x,y∈X.
A holds in S⇔S(x)≠S(y).
Case A≡B1∨...∨Br where B1,...,Br are atomic formulae.
A holds in S⇔ ∃i∈{1,...,r} such that Bi holds in S.
Similar to Propositional logic, we need to establish the concepts of consistency and inference.
Definition 3.7 (Consistency). The set of formulae {A1,...,An} is consistent if and only if ∃S∈S such that ∀i∈{1,...,n}Ai holds in S.
Definition 3.8 (Derivable Formula). The formula B∈C is derivable from the formulae A1,...,An if and only if ∀S∈S in which all the formulae A1, ..., An hold, the formula B also holds in S.
Analogous to Propositional Logic, the subsequent proposition is valid:
Proposition 3.1. Let B=B1∨...∨Br be a formula where B1,...,Br are atomic formulae. The formula B is derivable from A1,...,An if and only if
∀i∈{1,...,r} the set of formulae {A1,...,An,¬Bi} is not consistent. |
Let x be a variable which may take a finite set of values, represented as Ψ(x)={v1,...,vr}. The potential values of this variable can be expressed by the formula: (xi=v1)∨(xi=v2)∨...∨(xi=vr). Such formulae are integral to the knowledge base of the expert system, K.
Example 3.1. To better illustrate the concepts we have discussed, let us consider a small example of the expert system depicted in Figure 1. We will define the set of variables as X={x1,x2,y1,y2}, and the potential values as V={vi|i∈N}.
● We will define the conceptual framework of the expert system (see Definition 3.1)
– The variable x1 can take any value of the set {v1,v2,v3}. That is to say, we have that Ψ(x1)={v1,v2,v3}.
– The variable x2 can take any value. That is to say, we have that Ψ(x2)=V.
– The variable y1 can take any value of the set {v4,v5,v6,v7}. That is to say, we have that Ψ(y1)={v4,v5,v6,v7}.
– The variable y2 can take any value. That is to say, we have that Ψ(y2)=V.
● Next, we will consider the knowledge-base in the system (see Definition 3.5).
Integrity Constrains. Here, we will examine formulae derived from the potential set of values. We are dealing with only two variables, x1 and y1, each with a finite set of potential values. As a result, we can establish that:
– The variable x1 may take the values {v1,v2,v3}.
A1≡(x1=v1)∨(x1=v2)∨(x1=v3).
– The variable y1 may take the values {v4,v5,v6,v7}.
A2≡(y1=v4)∨(y1=v5)∨(y1=v6)∨(y1=v7).
Rules. We will consider that the expert system in this example considers the following rules:
A3≡(x1=x2)→(y1=v4)
A4≡(x1≠x2)→(y1=v6)
A5≡(x1=x2)→(y2=x1)
A6≡((x1=v1)∧(x2=v2))→(y2=v1)
A7≡((x1=v1)∧(x2≠v1)∧(x2≠v2))→(y2=v5)
The knowledge-base of the expert system is K={A1,...,A7}.
● Let us consider that the input of our expert system is:
F={(x1=v1),(x2=v3)} |
● Consider a potential state S (refer to Definition 3.2) of the system where every formula in K∪F is satisfied:
S(x1)=v1;S(x2)=v3 |
S(y1)=v6;S(y2)=v5 |
It can be observed that all the formulas in K∪F hold (see Definition 3.6). For instance, the formula (refer to Notation 3.1)
A7≡((x1=v1)∧(x2≠v1)∧(x2≠v2))→(y2=v5) |
is equivalently written as:
A7≡(x1≠v1)∨(x2=v1)∨(x2=v2)∨(y2=v5) |
Given that S(y2)=v5, the formula A7 holds in the state S as per Definition 3.6.
Similarly, the remaining formulas in K∪F hold.
● As can be easily deduced, the expert system outputs the formulae:
y1=v6y2=v5 |
We intuitively deduce them as follows:
– Given x1≠x2 (since x1=v1 and x2=v3), by applying rule A4, we conclude that y1=v6.
– Given x1=v1, and, since x2=v3, x2≠v1 and x2≠v2, by applying rule A7, we conclude that y2=v5.
Formally, we deduce y1=v6 and y2=v5 because for every state where all formulae in K∪F hold, the formulae y1=v6 and y2=v5 also hold (refer to Definition 3.8). In other words, for every state S such that S(x1)=v1 and S(x2)=v2 and the formulae in knowledge-base hold, it follows that S(y1)=v6 and S(y2)=v5.
According to Proposition 3.1, the formula y1=v6 is derived from input and knowledge-base as evidenced by the inconsistency of K∪F∪{y1≠v6}. Similarly, y2=v5 is inferred due to the inconsistency of K∪F∪{y2≠v5}. In other words, there is no state S such that the formulae K∪F hold and S(y1)≠v6; and there is no state S such that the formulae K∪F hold and S(y2)≠v5 (refer to Definition 3.7).
In this section, we will introduce an algebraic model that encapsulates the representation paradigm delineated in the preceding section. Utilizing this model, the following issues will be transposed into algebraic terms:
● The challenge of determining if there exists no possible state within a given knowledge base (refer to Theorem 4.2).
● The challenge of determining if a formula can be derived from the input and the knowledge-base (see Corollary 1).
Let us consider a set of variables X={x1,...,xm} and a set of formulae {A1,...,An}. Initially, we define a bijection, ϕ, that maps the possible values V to the field Q.
ϕ:V⟶Q |
Each potential negative atomic formula (i.e., the formulae of the form xi≠vj or xi≠xj) is associated with an auxiliary variable wi. We assume that there are w1,...,wk auxiliary variables for representing the set of formulae {A1,...,An}.
Next, we define the polynomial ring:
A=Q[x1,...,xm,w1,...,wk,z] |
Subsequently, we translate formulae into polynomials.
Definition 4.1 (Polynomial associated to a formula). For a formula A∈C, the polynomial pA∈A associated to the formula A is defined as follows:
Case A≡(xi=v) where xi∈X and v∈Ψ(xi).
pA=xi−ϕ(v).
Case A≡(xi=xj) where xi,xj∈X.
pA=xi−xj.
Case A≡(xi≠v) where xi∈X and v∈Ψ(xi).
pA=xi+w−ϕ(v) where wA is the variable associated to the formula xi≠v.
Case A≡(xi≠xj) where xi,xj∈X.
pA=xi−xj+wA where wA is the variable associated to the formula xi≠xj.
Case A≡B1∨...∨Br where B1,...,Br are atomic formulae.
pA=qB1⋅...⋅qBr
As we will explore later, each polynomial pA represents an equation pA=0, which describes the semantics of the formula A. For instance, the formula A≡(xi=v) is associated with the polynomial pA=xi−ϕ(v), because xi−ϕ(v)=0 if and only if xi=ϕ(v). This corresponds to the semantics of the formula A≡xi=v. It can be observed that a negative atomic formula involves the use of an auxiliary variable wi. These variables wi must assume values different from 0. Consequently, the formula A≡(xi=xj) is associated with the polynomial pA=xi−xj+wA, because for wA≠0, pA=0 if and only if xi≠xj.
Nevertheless, it is possible to circumvent the use of this auxiliary variable when the negative atomic formula takes the form x≠v1 where x is a variable that can only assume a finite set of values Ψ(x)={v1,...,vr}. In this scenario, the formula is equivalent to (and therefore can be replaced by) the formula:
(x=v2)∨...∨(x=vr) |
This equivalence is particularly interesting when x assumes a small number of possible values. Specifically, in the case where x can only assume two possible values {v1,v2} the negative atomic formula x≠v1 is equivalent to the atomic formula x=v2.
This becomes especially noteworthy when considering a rule of the form:
(A1∧A2∧...∧Ar)→(B1∨...∨Bs) |
where A1,...Ar,B1,...,Bs are atomic formulae. As mentioned above, this rule is expressed as the formula ¬A1∨...∨¬Ar∨B1∨...∨Bs which corresponds to the polynomial:
p¬A1⋅...⋅p¬Ar⋅pB1...⋅pBs |
Therefore, in the scenario where A1,...,Ar are negative atomic formulae and B1,...,Bs are positive atomic formula, the rule does not necessitate any auxiliary variable to represent this polynomial. In Example 1, we have that the rule A4≡(x1≠x2)→(y1=v6) is represented by pA4=(x1−x2)(y1−ϕ(v6)). In the same way, since x1 can only take a finite set of values (we have that Ψ(x1)={v1,v2,v3}), we can state that the rule A7≡((x1=v1)∧(x2≠v1)∧(x2≠v2))→(y2=v5) is equivalent to the rule:
((x1≠v2)∧(x1≠v3)∧(x2≠v1)∧(x2≠v2))→(y2=v5) |
whose polynomial associated is:
(x1−ϕ(v2))(x1−ϕ(v3))(x2−ϕ(v1))(x2−ϕ(v2))(y2−ϕ(v5)) |
Example 4.1. Consider the expert system described in Example 1. We define the bijection ϕ:V→C as follows:
ϕ(vi)=i |
Next, we computes the polynomials associated with the formulae in K (see Definition 4.1):
● A1≡(x1=v1)∨(x1=v2)∨(x1=v3)
pA1=(x1−1)(x1−2)(x1−3) |
● A2≡(y1=v4)∨(y1=v5)∨(y3=v6)∨(y3=v7)
pA2=(y1−4)(y1−5)(y1−6)(y1−7) |
● A3≡(x1=x2)→(y1=v4)
pA3=(x1−x2+w1)(y1−4) |
● A4≡(x1≠x2)→(y1=v6)
pA4=(x1−x2)(y1−6) |
● A5≡(x1=x2)→(y2=x1)
pA5=(x1−x2+w1)(y2−x1) |
● A6≡((x1=v1)∧(x2=v2))→(y2=v1)
pA6=(x1−2)(x1−3)(x2+w2−2)(y2−1) |
● A7≡((x1=v1)∧(x2≠v1)∧(x2≠v2))→(y2=v5)
pA7=(x1−2)(x1−3)(x2−1)(x2−2)(y2−5) |
where the auxiliary variables w1 and w2 are respectively associated to the formulae (x1≠x2) and (x2≠v2).
In this section we will in we will present some findings that recast the problem of verifying consistency and deduction in an expert system into algebraic terms (refer to Theorem 4.2 and Corollary 1). In the upcoming proposition, we will establish an initial relation between a formula and its corresponding polynomial.
Proposition 4.1. Let A(x1,...,xm) be a formula. Let S∈S be a state. Let pA(x1,...,xm,w1,...,wk)∈A be the polynomial associated to the formula A. We have that A(x1,...,xm) holds in S if and only if the following holds:
∃w∗1,...,w∗k∈Q−{0} such that pA(ϕ(S(x1)),...,ϕ(S(xm)),w∗1,...,w∗k)=0 |
Proof. First, we will prove it when A is an atomic formula.
Case A≡(xi=v) where v∈V.
We have that pA(xi)=xi−ϕ(v).
A(xi) holds in S⇔S(xi)=v⇔ϕ(S(xi))=ϕ(v)⇔ϕ(S(xi))−ϕ(v)=0⇔ pA(ϕ(S(xi)))=0
Case A≡(xi≠v) where v∈V.
We have that pA(xi,wj)=xi+wj−ϕ(v) where wj is the auxiliary variable associated to A.
A(xi) holds in S⇔S(xi)≠v⇔ϕ(S(xi))≠ϕ(v)⇔
⇔∃w∗j∈Q−{0} such that ϕ(S(xi))−ϕ(v)+w∗j=0⇔
⇔∃w∗j∈Q−{0} such that pA(ϕ(S(xi)),w∗j)=0
Case A≡(xi=xj).
We have that pA(xi,xj)=xi−xj.
A(xi,xj) holds in S⇔S(xi)=S(xj)⇔ϕ(S(xi))=ϕ(S(xj))⇔
⇔pA(ϕ(S(xi)),ϕ(S(xj)))=0
Case A≡(xi≠xj).
We have that pA(xi,xj,ws)=xi−xj+ws where ws is the auxiliary variable associated to A.
A(xi,xj) holds in S⇔S(xi)≠S(xj)⇔ϕ(S(xi))≠ϕ(S(xj))⇔
⇔∃w∗s∈Q−{0} such that ϕ(S(xi))−ϕ(S(xj))+w∗s=0⇔
⇔∃w∗s∈Q−{0} such that pA(ϕ(S(xi)),ϕ(S(xj)),w∗s)=0
Now, we will prove for the case that A is a disjunction of atomic formulae. That is to say, A≡B1∨...∨Br where B1,...,Br are atomic formulae. Therefore, we have that: pA(x1,...,xm,w1,...,wk)=pB1(x1,...,xm,w1,...,wk)⋅...⋅pBr(x1,...,xm,w1,...,wk).
In this case, we have that:
A holds in S⇔∃i∈{1,...,r} such that Bi holds in S⇔
∃i∈{1,...,r} ∃w∗1,...,x∗k∈Q−{0}pBi(ϕ(S(x1)),...,ϕ(S(xm)),w∗1,…w∗k)=0⇔∃w∗1,...,x∗k∈Q−{0} pB1(ϕ(S(x1)),...,ϕ(S(xm)),w∗1,…w∗k)⋅...⋅pBr(ϕ(S(x1)),...,ϕ(S(xm)),w∗1,…w∗k)=0⇔ pA(ϕ(S(x1)),...,ϕ(S(x1)))=0
Lemma 4.1. Let A1,...,An∈C be formulae. {A1,...,Ar} is a consistent set of formulae if and only if ∃x∗1,...,x∗m,w∗1,...,w∗k,z∗∈Q such that
∀i∈{1,...,n}pAi(x∗1,...,x∗m,w∗1,...,w∗k)=0 |
1+z∗⋅w∗1⋅...⋅w∗k=0 |
Proof. ⇒) Let {A1,...,An} be a consistent set of formulae.
Let S be a state in which all the formulae A1,...,An hold.
Let x∗i=ϕ(S(xi))∈Q where i∈{1,...,m}.
According to Proposition 4.1, we have that ∃w∗1,...,w∗k∈Q−{0} such that:
∀i∈{1,...,r}pAi(x∗1,...,x∗m,w∗1,...,w∗k)=0 |
Since ∀i∈{1,...,k}w∗i≠0, we have that ∃z∗∈Q such that
1+z∗⋅w∗1⋅...⋅w∗k=0 |
⇐)Let x∗1,...,x∗m,w∗1,...,w∗k,z∗∈Q such that:
● 1+z∗⋅w∗1⋅...⋅w∗k=0.
● ∀i∈{1,...,n}pAi(x∗1,...,x∗m,w∗1,...,w∗k)=0
Let S be the state such that ∀i∈{1,...,m}S(xi)=ϕ−1(x∗i).
Since 1+z∗⋅w∗1⋅...⋅w∗k=0, we have that ∀i∈{1,...,k}w∗i≠0. That is to say w∗1,...,w∗k∈Q−{0}. Since ∀i∈{1,...,n}pAi(x∗1,...,x∗m,w∗1,...,w∗k)=0, by Proposition 4.1, ∀i∈{1,...,n}Ai holds in S.
Consequently, we have that {A1,...,An} is consistent.
Theorem 4.2. Let A1,...,An∈C be formulae.
{A1,...,An} is consistent ⇔1∉⟨pA1,...,pAn,1+z⋅w1⋅...⋅wk⟩ |
Proof.
Let I=⟨pA1,...,pAn,1+z⋅w1⋅...⋅wk⟩
⇒) Suppose that {A1,...,An} is consistent. By Lemma 4.1, we have that ∃x∗1,…x∗m,w∗1,…w∗k,z∗∈Q such that
∀ipAi(ϕ(S(x1))…ϕ(S(xm)),w∗1…w∗k)=0 |
1+z∗⋅w∗1⋅w∗k=0 |
We will establish this proof by employing a reductio ad absurdum argument. Let's assume that 1∈I. Under this assumption, we would have
1=α1pA1+...+αnpAn+αn+1(1+z⋅w1⋅...⋅wk) |
Therefore, we have that
1=1(ϕ(S(x1))…ϕ(S(xm)),w∗1…w∗k)= |
=α1pA1+...+αnpAn+αn+1(1+z⋅w1⋅...⋅wk)(ϕ(S(x1))…ϕ(S(xm)),w∗1…w∗k)=0 |
This leads to a contradiction. Therefore, we must conclude that 1∉I.
⇐) We will consider that {A1,...,An} is inconsistent. We will consider that all the polynomials pA1…pAn,1+z⋅w1⋅...⋅wk lie in C[x1…xn,w1…wk,z]⊂Q[x1…xn,w1…wk,z]. Since {A1,...,An} is inconsistent, we have that ∄x∗1,…x∗m,w∗1,…w∗k,z∗∈Q such that
∀ipAi(ϕ(S(x1))…ϕ(S(xm)),w∗1…w∗k)=0 |
1+z∗⋅w∗1⋅w∗k=0 |
Since pAi is the product of simple factors, it is immediate to state that: ∄x∗1,…x∗m,w∗1,…w∗k,z∗∈C such that
∀ipAi(ϕ(S(x1))…ϕ(S(xm)),w∗1…w∗k)=0 |
1+z∗⋅w∗1⋅w∗k=0 |
Consequently, we ascertain that V(I)=∅ and, by applying the weak Hilbert's Nullstellensatz, we infer that I=⟨1⟩. This leads us to the conclusion that 1∈I.
Corollary 4.1. A formula B is derivable from {A1…An} if and only if
1∈⟨p¬B,pA1,…pAn,1+z⋅w1⋅...⋅wk⟩ |
Proof. This is an immediate consequence of Proposition 3.1 and Theorem 4.2.
In Figure 2 we illustrate how we can implement the expert systems developed in Section 3 by means of the mathematical results obtained previously.
Formulae in the input and the knowledge base are represented by means of polynomials according to Definition 4.1, resulting in the ideal F and K:
Ideal F. This is the ideal generated by the polynomials representing the formulae in the input F.
Ideal K. This is the ideal generated by the polynomials representing the formulae in the knowledge-base K.
In accordance with Corollary 4.1, the expert system infers the formula B if and only if
1∈K+F+⟨p¬B,1+z⋅w1⋅...⋅wk⟩ |
where w1…wk are the auxiliary variables utilized to represent the formulae in K, F and the formula ¬B.
Consequently, we need to consider two additional polynomials (see Figure 2):
● The polynomial 1+z⋅w1⋅...⋅wk associated to the auxiliary variables w's needed to represent negative atomic formulae.
● The polynomial pB associated to the atomic formula B we wish to determine if the system outputs.
According to this corollary, we can determine if the system outputs B by calculating the reduced Gröbner basis of the ideal generated by all previous polynomials (that is to say, the reduced Gröbner basis of the ideal K+F+⟨p¬B,1+z⋅w1⋅...⋅wk⟩) and examining whether it equals $ [1] .Ifitdoes,expertsystemoutputs B $.
Example 4.2. Let us consider the expert system described in Example 1 and Example 2, and depicted in Figure 2. By applying Theorem 4.2, we will verify that the output of the expert system is (y1=v6) and y2=v5 when the input of the expert system is F={(x1=v1),(x2=v3)}.
We will stop, as an example, at the polynomials associated with some formulae (see Definition 4.1) in the input, F, and the knowledge-base, K:
● The atomic formula in the input x1=v1 corresponds to the polynomial:
x1−1 |
● The integrity constraint A1≡(x1=v1)∨(x1=v2)∨(x1=v3) corresponds to the polynomial:
pA1=(x1−1)(x1−2)(x1−3) |
● The rule A4≡(x1≠x2)→(y1=v6) corresponds to the polynomial:
pA4=(x1−x2)(y1−6) |
Note that the formula A4 can be also written (see Notation 3.1) as A4≡(x1=x2)∨(y1=v6)
● The formula A3≡(x1=x2)→(y1=v4) corresponds to the polynomial:
pA3=(x1−x2+w1)(y1−4) |
Note that the formula A3 can be also written (see Notation 3.1) as A3≡(x1≠x2)→(y1=v4) and we need an auxiliary variable, w1, for the atomic formula x1≠x2.
In this way, we have that:
● The ideal associated to the input is
F=⟨x1−1,x2−3⟩ |
● The ideal K associated with the knowledge-base of the expert system is:
K=⟨pA1,pA2,pA3,pA4,pA5,pA6,pA7⟩ |
Besides, we need two additional polynomials:
● The polynomial associated to ¬B. If the output formula B is y1=v6, we have that p¬B is:
y1+w3−6 |
where w3 is an auxiliary variable used for the negative atomic formula ¬B≡y1≠v6
● The polynomial associated to all the auxiliary variables w's used:
1+z⋅w1⋅w2⋅w3 |
To verify whether the system can infer y1=v6, we need to check if the reduced Gröbner basis of the ideal generated by the previous polynomials equals [1]:
K+F+⟨y1+w3−6,1+z⋅w1⋅w2⋅w3⟩ |
In this section, we strive to clarify the underlying logic of our approach. The inference engine is fundamentally based on an algebraic approach. Both rules and facts are interconnected through algebraic equations that a state compatible with formulae must satisfy. Each polynomial p used to infer the output of the system is associated with the algebraic equation p=0. Finding a state S compatible with all the formulae is equivalent to solve a this set of algebraic equations. In Figure 3 we illustrate the set of equations generated by the expert system in Example 1, which are related to the polynomials used in Example 3. For example:
● The formula A1≡(x1=v1)∨(x1=v2)∨(x1=v3) corresponds to the polynomial:
pA1=(x1−1)(x1−2)(x1−3) |
Note that pA1=(x1−1)(x1−2)(x1−3)=0 if and only if either x1=1 or x1=2 or x1=3, which aligns with the semantics of the formula A1.
● The formula A4≡(x1≠x2)→(y1=v6) corresponds to the polynomial:
pA4=(x1−x2)(y1−6) |
Note that pA4=(x1−x2)(y1−6)=0 if and only if either x1=x2 or y1=6. In other words, if (x1≠x2) then y1 must be 6, which aligns with the semantics of the formula A4≡(x1≠x2)→(y1=v6).
● The formula A3≡(x1=x2)→(y1=v4) corresponds to the polynomial:
pA3=(x1−x2+w1)(y1−4) |
where w1 must be a value different from 0.
Given that w1≠0, note that pA3=(x1−x2+w1)(y1−4)=0 if and only if either x1≠x2 (since w1≠0) or y1=4. In other words, if x1=x2 then y1 must be 4, which aligns with the semantics of the formula A3≡(x1=x2)→(y1=v4).
In Example 4.2, we have the input F={x1=v1,x2=v3}, represented by the polynomials:
● The fact x1=v1 corresponds to the polynomial:
x1−1 |
Note that x1−1=0 if and only if x1=1, which aligns with the semantics of the fact x1=v1.
● The fact x2=v3 corresponds to the polynomial:
x2−3 |
Note that x2−3=0 if and only if x2=3, which aligns with the semantics of the fact x2=v3.
To verify if the system outputs y1=v6, we need to represent the formula y1≠v6. This is represented by the polynomial:
y1+w3−6 |
where w3≠0. Note that y1+w3−6=0 if and only if y1≠6 (since w3≠0), which aligns with the semantics of the formula y1=v6.
Additionally, we have another polynomial associated with the variables wi:
1+z⋅w1⋅w2⋅w3 |
Note that 1+z⋅w1⋅w2⋅w3=0 if and only if w1≠0 and w2≠0 and w3≠0. Consequently, this equation associated with this polynomial ensures that the variables w must be different from 0.
In this way, we have the following set of equations:
● The set of equations related to K:
(x1−1)(x1−2)(x1−3)=0(y1−4)(y1−5)(y1−6)(y1−7)=0(x1−x2+w1)(y1−4)=0(x1−x2)(y1−6)=0(x1−x2+w1)(y2−x1)=0(x1−2)(x1−3)(x2+w2−2)(y2−1)=0(x1−2)(x1−3)(x2−1)(x2−2)(y2−5)=0 |
● The set of equations related to F:
x1−1=0x2−3=0 |
● The equation related to the polynomial associated with the set of variables w's:
1+z⋅w1⋅w2⋅w3=0 |
● The equation related to the output:
y1+w3−6=0 |
Through algebraic methods (by verifying that the reduced Gröbner basis is [1]), we conclude that it is unfeasible to find values for the variables x1,x2,y1,y2 that would satisfy all the preceding equations. Thus, if we discovered values for the variables x1,x2,y1,y2 that satisfied the equations of K∪F∪{1+z⋅w1⋅w2⋅w3=0} (in other words, the formulae of the knowledge base and the input hold), then the equation y1+w3−6=0 would not be satisfied (in other words, the formula associated to the output does not hold). Considering that w3≠0 (as the equation 1+z⋅w1⋅w2⋅w3=0 is satisfied), y1 must be 6 for the equation y1+w3−6=0 to not be satisfied. In other words, we infer that y1 must be equal to v6. In summary, our analysis of the previous equations leads us to deduce that y1=v6 is a consequence of the input and the knowledge base.
In this section, we will explore the potential of designing an interlocking problem for a railway system using our algebraic approach. An interlocking system is a safety-critical mechanism engineered to prevent train collisions. Various approaches have been proposed by researchers have to address the problem of determining if two trains may collide [23,24,25,26,27,28,29]. In this section we will easily design an interlocking system by means of the paradigm described here: An interlocking system as an expert system whose variables assume an infinite set of potential values. This approach allows for a straightforward design process.
Given a railway station, our goal is to design an expert system that can determine whether a given situation poses a danger. We will illustrate the concepts of our paper using the railway station depicted in Figure 4. However, it is important to note that these principles can be generalized to any railway station.
We will be examining the railway station depicted in Figure 4. As can be observed, it comprises:
● 11 sections,
● Two turnouts: one turnout connecting S2 to sections S3 or S4 (since the switch is on direct track position, trains move from S2 to S3), and another connecting S6 to sections S5 or S11 (in this case, since the switch is on diverted track position, trains move from S6 to S11)
● 10 semaphores depicted by cycles (black representing red colour, white representing green colour)
● Three trains, T1, T2 and T3, placed respectively in sections S1, S10 and S8.
As may be seen, the situation depicted in Figure 4 is dangerous: the trains situated in sections S10 and S8 could collide in section S7: Train in S10 moves from S10 to S11, then to S6 and finally from S6 to S7; train in S8 moves from S8 to S7.
We will consider a railway station that has N sections, denoted as S1…SN, and trains in the station are identified by a natural number. We will consider that V=N.
The set of variables, X consists of two types:
A variable ei,j for each two pair of connected sections Si and Sj. For any two sections Si and Sj that are connected at the edge, we will define the variable ei,j∈{0,1}, i.e. Ψ(ei,j{0,1}. The variable ei,j=1 indicates that it is possible for a train to pass from section Si to section Sj and ei,j=0 indicates that it is not possible to pass from section si to section sj.
In the railway station depicted in Figure 4, we would have the following variables:
e1,2,e2,9,e9,10,e10,11,e11,6,e2,3,e3,4,e4,5,e5,6,e6,7,e7,8,
e2,1,e9,2,e10,9,e11,10,e6,11,e3,2,e4,3,e5,4,e6,5,e7,6,e8,7
A variable xi for each section Si in the railway station. We define Ψ(xi)=V=N. The value xi=tj signifies that train tj∈N can reach the section Si. In no trains can reach section Si, then we set xi=0. If a situation is dangerous, there would be two different trains tj and tk that could reach the same section Sj. As a result, we would have the formulae xi=tj and xi=tk≠tj, which are inconsistent formulae. In the railway station depicted in Figure 4, we would have the following variables:
x1,x2,x3,x4,x5,x6,x7,x8,x9,x10,x11 |
We will consider the following formulae in the knowledge-base, K:
Integrity Constraints. These are formulae related to the possible values, {0,1}, that variables ei,j may assume. Specifically, for every pair of sections i and j we have that (ei,j=0)∨(ei,j=1). Consequently, we have that: e1,2=0∨e1,2=1
e2,9=0∨e2,9=1
e9,10=0∨e9,10=1
e10,11=0∨e10,11=1
e11,6=0∨e11,6=1
e2,3=0∨e2,3=1
e3,4=0∨e3,4=1
e4,5=0∨e4,5=1
e5,6=0∨e5,6=1
e6,7=0∨e6,7=1
e7,8=0∨e7,8=1
e2,1=0∨e2,1=1
e9,2=0∨e9,2=1
e10,9=0∨e10,9=1
e11,10=0∨e11,10=1
e6,11=0∨e6,11=1
e3,2=0∨e3,2=1
e4,3=0∨e4,3=1
e5,4=0∨e5,4=1
e6,5=0∨e6,5=1
e7,6=0∨e7,6=1
e8,7=0∨e8,7=1
Rules. These are formulae related to the possible movements of trains. Given two sections Si and Sj which may be connected, we will consider the following rule*:
*This rule implies that if the train Tk may reach section Si (i.e., xi=k≠0), and it is possible to pass from section Si to section Sj, then the same train Tk can reach section Sj (i.e., xj=k=xi).
(ei,j=1)∧(xi≠0)→(xj=xi)
Consequently, we have the following rules:
(e1,2=1)∧(x1≠0)→(x2=x1)
(e2,9=1)∧(x2≠0)→(x9=x2)
(e9,10=1)∧(x9≠0)→(x10=x9)
(e10,11=1)∧(x10≠0)→(x11=x10)
(e11,6=1)∧(x11≠0)→(x6=x11)
(e2,3=1)∧(x2≠0)→(x3=x2)
(e3,4=1)∧(x3≠0)→(x4=x3)
(e4,5=1)∧(x4≠0)→(x5=x4)
(e5,6=1)∧(x5≠0)→(x6=x5)
(e6,7=1)∧(x6≠0)→(x7=x6)
(e7,8=1)∧(x7≠0)→(x8=x7)
(e2,1=1)∧(x2≠0)→(x1=x2)
(e9,2=1)∧(x9≠0)→(x2=x9)
(e10,9=1)∧(x10≠0)→(x9=x10)
(e11,10=1)∧(x11≠0)→(x10=x11)
(e6,11=1)∧(x6≠0)→(x11=x6)
(e3,2=1)∧(x3≠0)→(x2=x3)
(e4,3=1)∧(x4≠0)→(x3=x4)
(e5,4=1)∧(x5≠0)→(x4=x5)
(e6,5=1)∧(x6≠0)→(x5=x6)
(e7,6=1)∧(x7≠0)→(x6=x7)
(e8,7=1)∧(x8≠0)→(x7=x8)
The input is intrinsically linked to the status of the turnouts and semaphores within the railway station, as well as the positioning of the trains. As illustrated in Figure 4, the input is as follows:
Variables eij. For each pair of connected sections Si, Sj, we have either an atomic formula (ei,j=1) or (ei,j=0) representing whether it is possible to transition from section Si to section Sj. The colour of the semaphores and the position of turnout switches. For example, e1,2=1 in Figure 4 because the semaphore between section S1 to S2 is green. The turnout switch connecting sections S2, S3 and S9 being on direct results in e2,3=1 and e2,9=0: We have the following: e1,2=1;e9,10=1;e10,11=1;e11,6=1;e2,3=1;e3,4=1;e5,6=1;e6,7=1;e7,8=1;
e2,1=1;e11,10=1;e6,11=1;e3,2=1;e5,4=1;e8,7=1;
e2,9=0;e4,5=0;e9,2=0;e10,9=0;e4,3=0;e6,5=0;e7,6=0;
Variables xi. For each train Tj located in section Si, we consider the positive atomic formula xi=j. Given that there are three trains positioned on S1, S10 and S8 in Figure 4, we have that:
x1=1
x10=2
x8=3
The primary objective of the system is to ensure the safety of the railway. If the railway is deemed unsafe, it two trains, represented as Tj and Tk, could potentially arrive at the same section Si. In such a case, we would have that xi=tj and xi=tk, and since tj≠tk, it would lead to a contradiction in the set of formulae derived from the input and the knowledge base (as we can infer that xi=k≠j=xi). Therefore, the output mainly involves checking the consistency of the set of formulae generated by the input and the knowledge base. A consistent set indicates a safe situation, while an inconsistent set signifies danger.
According to Section 4, we have that the polynomial ring is:
A=Q[e1,2,e2,9,e9,10,e10,11,e11,6,e2,3,e3,4,e4,5,e5,6,e6,7,e7,8,e2,1,e9,2,e10,9,e11,10,e6,11,e3,2,e4,3,e5,4,e6,5,e7,6,e8,7x1,x2,x3,x4,x5,x6,x7,x8,x9,x10,x11] |
In CoCoA syntax, we would have:
use QQ[e1_2, e2_9, e9_10, e10_11, e11_6, e2_3, e3_4, e4_5, e5_6, e6_7, e7_8, e2_1, e9_2, e10_9, e11_10, e6_11, e3_2, e4_3, e5_4, e6_5, e7_6, e8_7, x[1..11]]; |
We will convert the rules and the integrity constraints of the system's knowledge base, denoted as K, into polynomials. As per 9:
● The integrity constraint (ei,j=0)∨(ei,j=1) is represented by the polynomial
ei,j⋅(eij−1) |
● The rule (ei,j=1)∧(xi≠0)→(xj=xi) is represented by the polynomial:
ei,j⋅xi⋅(xj−xi) |
We define the ideal K as the ideal generated by the polynomials that represent these formulae in K.
K: = Ideal(
e1_2 * (e1_2 - 1), e2_9 * (e2_9 - 1),
e9_10 * (e9_10 - 1), e10_11 * (e10_11 - 1),
e11_6 * (e11_6 - 1), e2_3 * (e2_3 - 1),
e3_4 * (e3_4 - 1), e4_5 * (e4_5 - 1),
e5_6 * (e5_6 - 1), e6_7 * (e6_7 - 1),
e7_8 * (e7_8 - 1), e2_1 * (e2_1 - 1),
e9_2 * (e9_2 - 1), e10_9 * (e10_9 - 1),
e11_10 * (e11_10 - 1), e6_11 * (e6_11 - 1),
e3_2 * (e3_2 - 1), e4_3 * (e4_3 - 1),
e5_4 * (e5_4 - 1), e6_5 * (e6_5 - 1),
e7_6 * (e7_6 - 1), e8_7 * (e8_7- 1),
e1_2 * x[1] * (x[2] - x[1]), e2_9 * x[2] * (x[9] - x[2]),
e9_10 * x[9] * (x[10] - x[9]), e10_11 * x[10] * (x[11] - x[10]),
e11_6 * x[11] * (x[6] - x[11]), e2_3 * x[2] * (x[3] - x[2]),
e3_4 * x[3] * (x[4] - x[3]), e4_5 * x[4] * (x[5] - x[4]),
e5_6 * x[5] * (x[6] - x[5]), e6_7 * x[6] * (x[7] - x[6]),
e7_8 * x[7] * (x[8] - x[7]), e2_1 * x[2] * (x[1] - x[2]),
e9_2 * x[9] * (x[2] - x[9]), e10_9 * x[10] * (x[9] - x[10]),
e11_10 * x[11] * (x[10] - x[11]), e6_11 * x[6] * (x[11] - x[6]),
e3_2 * x[3] * (x[2] - x[3]), e4_3 * x[4] * (x[3] - x[4]),
e5_4 * x[5] * (x[4] - x[5]), e6_5 * x[6] * (x[5] - x[6]),
e7_6 * x[7] * (x[6] - x[7]), e8_7 * x[8] * (x[7] - x[8]));
For the situation depicted in Figure 4, the input is as follows:
F: = Ideal(e1_2 - 1, e9_10 - 1, e10_11 - 1, e11_6 - 1, e2_3 - 1, e3_4 - 1, e5_6 - 1, e6_7 - 1, e7_8 - 1, e2_1 - 1, e11_10 - 1, e6_11 - 1, e3_2 - 1, e5_4 - 1, e8_7 - 1, e2_9, e4_5, e9_2, e10_9, e4_3, e6_5, e7_6, x[1]-1, x[10]-2, x[8]-3); |
According to Theorem 4.2, we can determine if the set of formulae K∪F is inconsistent (indicating a dangerous situation), by checking if the reduced Gröbner basis of the ideal F+K is [1]. In CoCoA syntax, this is represented as:
ReducedGBasis(F+K) = [1];
Since CoCoA outputs true. Consequently, the situation is dangerous.
In this paper, we introduce an innovative algebraic methodology for the development of expert systems that can accommodate attributes capable of assuming a value from an infinite set. Prior algebraic approaches were reliant on representation formalisms based on either Propositional Logic or the Concept-Attribute-Value paradigm. Both of these formalisms necessitate that attributes assume a finite set of values. Despite the differences between these two approaches, they exhibit a certain degree of equivalence: Any expert system that can be algebraically implemented using one model can also be implemented using the other.
However, in scenarios where an attribute can assume a value from an infinite (or very large finite) set, the expert system cannot be represented using propositional logic. Until now, no method had been identified to implement such a system on a Computer Algebra System. This paper breaks new ground by presenting a model that not only addresses this gap but also provides a fresh perspective on previous results. In fact, these prior results can be viewed as specific instances within the broader framework of our proposed solution.
Our methodology can be employed in expert systems across various applications where uncertainty is not a factor. It is particularly suited for decision trees that culminate in a finite number of outputs, as opposed to intermediate steps and results with variable levels of certainty [12] (in fields such as medical diagnostics, quality improvement and business decision-making, among others). Indeed, our approach does present some limitations when variables assume a value from an infinite set since we have only considered relations between variables with equality (xi=xj) and inequality (xi≠xj). The representation of order relations such as xi>xj is currently not supported. Despite these limitations, our methodology offers a framework that can be applied in situations where systems do not utilize uncertainty. In this paper, we have demonstrated a practical application of our approach in determining interlocking problems.
The authors declare that they have not used Artificial Intelligence (AI) tools in the creation of this article.
Professor José Luis Galán-García is a Guest Editor for AIMS Mathematics and was not involved in the editorial review or the decision to publish this article. All authors declare that there are no competing interests.
[1] | Ahmad N, Buniamin S (2021) The relationship between SDG engagement and corporate financial performance: Evidence from public listed companies in Malaysia. Global Bus Manage Res 13: 730–741. |
[2] |
Anzola-Román P, Garcia-Marco T, Zouaghi F (2023) The Influence of CSR Orientation on Innovative Performance: Is the Effect Conditioned to the Implementation of Organizational Practices?. J Bus Ethics, 1–18. https://doi.org/10.1007/s10551-023-05406-z doi: 10.1007/s10551-023-05406-z
![]() |
[3] |
Atan R, Alam MM, Said J, et al. (2018) The impacts of environmental, social, and governance factors on firm performance: Panel study of Malaysian companies. Manage Environ Quality 29: 182–194. https://doi.org/10.1108/MEQ-03-2017-0033 doi: 10.1108/MEQ-03-2017-0033
![]() |
[4] |
Berrone P, Fosfuri A, Gelabert L, et al. (2013) Necessity as the mother of 'green' inventions: Institutional pressures and environmental innovations. Strat Manage J 34: 891–909. https://doi.org/10.1002/smj.2041 doi: 10.1002/smj.2041
![]() |
[5] | Bertelsmann S (2018) Culture and Conflict in Global Perspective, The Cultural Dimensions of Global Conflicts 1945–2007, Bertelsmann Stiftung (ed.), Verlag Bertelsmann Stiftung. |
[6] |
Biermann F, Kanie N, Kim RE (2017) Global governance by goal-setting: The novel approach of the UN Sustainable Development Goals. Curr Opin Env Sust 26: 26–31. https://doi.org/10.1016/j.cosust.2017.01.010 doi: 10.1016/j.cosust.2017.01.010
![]() |
[7] |
Blodgett JG, Lu LC, Rose GM, et al. (2001) Ethical sensitivity to stakeholder interests: A cross-cultural comparison. J Acad Mark Sci 29: 190–202. https://doi.org/10.1177/03079459994551 doi: 10.1177/03079459994551
![]() |
[8] |
Bradley SW, Wiklund J, Shepherd DA (2010) Swinging a double-edged sword: The effect of slack on entrepreneurial management and growth. J Bus Venturing 26: 537–554. https://doi.org/10.1016/j.jbusvent.2010.03.002 doi: 10.1016/j.jbusvent.2010.03.002
![]() |
[9] |
Buallay A (2019) Between cost and value: Investigating the effects of sustainability reporting on a firm's performance. J Appl Account Res 20: 481–496.https://doi.org/10.1108/JAAR-12-2017-0137 doi: 10.1108/JAAR-12-2017-0137
![]() |
[10] |
Buhr N, Freedman M (2001) Culture, institutional factors and differences in environmental disclosure between Canada and the United States. Crit Perspect Accoun 12: 293–322. https://doi.org/10.1006/cpac.2000.0435 doi: 10.1006/cpac.2000.0435
![]() |
[11] |
Calabrese A, Costa R, Gastaldi M, et al. (2021) Implications for Sustainable Development Goals: A framework to assess company disclosure in sustainability reporting. J Clean Prod 319: 128624. https://doi.org/10.1016/j.jclepro.2021.128624 doi: 10.1016/j.jclepro.2021.128624
![]() |
[12] |
Campagnolo L, Eboli F, Farnia L, et al. (2018) Supporting the UN SDGs transition: Methodology for sustainability assessment and current worldwide ranking. Economics 12: 1–31. https://doi.org/10.5018/economics-ejournal.ja.2018-10 doi: 10.5018/economics-ejournal.ja.2018-10
![]() |
[13] |
Chen S, Bouvain P (2009) Is corporate responsibility converging? A comparison of corporate responsibility reporting in the USA, UK, Australia, and Germany. J Bus Ethics 87: 299–317. https://doi.org/10.1007/s10551-008-9794-0 doi: 10.1007/s10551-008-9794-0
![]() |
[14] |
Chu Z, Xu J, Lia F, et al. (2018) Institutional theory and environmental pressures: The moderating effect of market uncertainty on innovation and firm performance. IEEE T Eng Manage 65: 392–403. https://doi.org/10.1109/TEM.2018.2794453 doi: 10.1109/TEM.2018.2794453
![]() |
[15] |
Delmas MA, Toffel MW (2008) Organizational responses to environmental demands: Opening the black box. Strat Manage J 29: 1027–1055. https://doi.org/10.1002/smj.701 doi: 10.1002/smj.701
![]() |
[16] |
Diaz-Sarachaga JM (2021) Monetizing impacts of Spanish companies toward the Sustainable Development Goals. Corp Soc Resp Env Ma 28: 1313–1323. http://doi.org/10.1002/csr.2149 doi: 10.1002/csr.2149
![]() |
[17] |
DiMaggio PJ, Powell WW (1983) The iron cage revisited: Institutional isomorphism and collective rationality in organizational fields. Am Sociol Rev 48: 147–160. https://doi.org/10.2307/2095101 doi: 10.2307/2095101
![]() |
[18] | Doupnik TS, Salter SB (1995) External environment, culture, and accounting practice: A preliminary test of a general model of international accounting development. Int J Account 30: 189–207. |
[19] |
Durugbo C, Amankwah‐Amoah J (2019) Global sustainability under uncertainty: How do multinationals craft regulatory policies? Corp Soc Resp Env Ma 26: 1500–1516. https://doi.org/10.1002/csr.1764 doi: 10.1002/csr.1764
![]() |
[20] | EIKON-Refinitiv (2019). Environmental, Social and Governance (ESG) Scores. Thomson Reuters. |
[21] |
Elalfy A, Weber O, Geobey S (2021) The Sustainable Development Goals (SDGs): A rising tide lifts all boats? Global reporting implications in a post SDGs world. J Appl Account Res 22: 557–575. http://doi.org/10.1108/JAAR-06-2020-0116 doi: 10.1108/JAAR-06-2020-0116
![]() |
[22] |
Erin O, Bamigboye O, Arumona J (2020) Risk governance and financial performance: An empirical analysis. Bus Theory Practice 21: 758–768. https://doi.org/10.3846/btp.2020.10850 doi: 10.3846/btp.2020.10850
![]() |
[23] |
Erin OA, Bamigboye OA, Oyewo B (2022) Sustainable development goals (SDG) reporting: an analysis of disclosure. J Account Emerg Econ 12: 761–789. https://doi.org/10.1108/JAEE-02-2020-0037 doi: 10.1108/JAEE-02-2020-0037
![]() |
[24] | Eurostat (2023) https://ec.europa.eu/eurostat/statistics explained/index.php?title = Archive: Quality_of_life_in_Europe_-_facts_and_views_-_leisure_and_social_relations |
[25] | Faisal F, Tower G, Rusmin R (2015) Legitimising corporate sustainability reporting throughout the world. Aust Account Bus Financ J 6: 19–34. |
[26] |
Feng Y, Akram R, Hieu VM, et al. (2021) The impact of corporate social responsibility on the sustainable financial performance of Italian firms: Mediating role of firm reputation. Econ Research-Ekonomska Istraživanja, 1–19. https://doi.org/10.1080/1331677X.2021.2017318 doi: 10.1080/1331677X.2021.2017318
![]() |
[27] |
Florini A, Pauli M (2018) Collaborative governance for the Sustainable Development Goals. Asia Pacific Policy Stud 5: 583–598. https://doi.org/10.1002/app5.252 doi: 10.1002/app5.252
![]() |
[28] | Freeman RE (1984) Strategic management: A stakeholder approach, Boston: Pitman Publishing. |
[29] | Frey M, Sabbatino A (2018) The role of the private sector in global sustainable development: The UN 2030 agenda, In: Grigore, G., Stancu, A., and McQueen, D. (eds), Corporate responsibility and digital communities: An international perspective towards sustainability, Cham: Palgrave Macmillan. https://doi.org/10.1007/978-3-319-63480-7_10 |
[30] |
García-Meca E, Martínez-Ferrero J (2021) Is SDG reporting substantial or symbolic? An examination of controversial and environmentally sensitive industries. J Clean Prod 298: 126781. https://doi.org/10.1016/j.jclepro.2021.126781 doi: 10.1016/j.jclepro.2021.126781
![]() |
[31] |
Gaziulusoy AI, Brezet H (2015) Design for system innovations and transitions: A conceptual framework integrating insights from sustainability science and theories of system innovations and transitions. J Clean Prod 108: 558–568. https://doi.org/10.1016/j.jclepro.2015.06.066 doi: 10.1016/j.jclepro.2015.06.066
![]() |
[32] | Global UN. (2012). https://www.un.org/millenniumgoals/pdf/Think%20Pieces/2_culture.pdf |
[33] |
Gneiting U, Mhlanga R (2021) The partner myth: Analysing the limitations of private sector contributions to the Sustainable Development Goals. Dev Pract 31: 920–926. https://doi.org/10.1080/09614524.2021.1938512 doi: 10.1080/09614524.2021.1938512
![]() |
[34] |
Hák T, Janoušková S, Moldan B (2016) Sustainable Development Goals: A need for relevant indicators. Col Indic 60: 565–573. https://doi.org/10.1016/j.ecolind.2015.08.003 doi: 10.1016/j.ecolind.2015.08.003
![]() |
[35] |
Hannan MP, Freeman JH (1977) The population ecology of organizations. Am J Soc 82: 929–964. https://doi.org/10.1086/226424 doi: 10.1086/226424
![]() |
[36] | Hawley A (1968) Human ecology, In: Sills, D.L. (ed.), International encyclopedia of the social sciences, New York: Macmillan. |
[37] | Hofstede G (2001) Culture's consequences: Comparing values, behaviors, institutions and organizations across nations, 2 Eds., United States: Sage. |
[38] |
Hofstede G (2011) Dimensionalizing cultures: The Hofstede model in context. Online Readings in Psychology and Culture 2. https://doi.org/10.9707/2307-0919.1014 doi: 10.9707/2307-0919.1014
![]() |
[39] | Hofstede G (2022) Hofstede insights. Available from: https://www.hofstede-insights.com/ [Accessed November 24th, 2022]. |
[40] |
Jacob A (2017) Mind the gap: Analyzing the impact of data gap in Millennium Development Goals'(MDGs) indicators on the progress toward MDGs. World Dev 93: 260–278. http://dx.doi.org/10.1016/j.worlddev.2016.12.016 doi: 10.1016/j.worlddev.2016.12.016
![]() |
[41] |
Jensen JC, Berg N (2012) Determinants of traditional sustainability reporting versus integrated reporting. An Institutionalist Approach. Bus Strat Environ 21: 299–316. https://doi.org/10.1002/bse.740 doi: 10.1002/bse.740
![]() |
[42] |
Khaled R, Ali H, Mohamed EK (2021) The Sustainable Development Goals and corporate sustainability performance: Mapping, extent and determinants. J Clean Prod 311: 127599. http://dx.doi.org/10.1016/j.jclepro.2021.127599 doi: 10.1016/j.jclepro.2021.127599
![]() |
[43] |
Kücükgül E, Cerin P, Liu Y (2022) Enhancing the value of corporate sustainability: An approach for aligning multiple SDGs guides on reporting. J Clean Prod 333: 130005. https://doi.org/10.1016/j.jclepro.2021.130005 doi: 10.1016/j.jclepro.2021.130005
![]() |
[44] |
Lassala C, Orero-Blat M, Ribeiro-Navarrete S (2021) The financial performance of listed companies in pursuit of the Sustainable Development Goals (SDG). Econ Research-Ekonomska Istraživanja 34: 427–449. http://doi.org/10.1080/1331677X.2021.1877167 doi: 10.1080/1331677X.2021.1877167
![]() |
[45] | Lee KH, Herold DM (2018) Cultural relevance in Environmental and Sustainability Management Accounting (EMA) in the Asia-Pacific region: A Link between cultural values and accounting values towards EMA values, In: Lee, K.H., and Schaltegger, S. (eds), Accounting for sustainability: Asia Pacific perspectives, Cham: Springer, 11–37. https://doi.org/10.1007/978-3-319-70899-7_2 |
[46] |
Li B, Wu K (2017) Environmental management system adoption and the operational performance of firm in the textile and apparel industry of China. Sustainability 9: 1–11. http://doi.org/10.3390/su9060992 doi: 10.3390/su9060992
![]() |
[47] |
Lior N, Radovanović M, Filipović S (2018) Comparing sustainable development measurement based on different priorities: Sustainable Development Goals, economics, and human well-being—Southeast Europe case. Sustainability Sci 13: 973–1000. http://dx.doi.org/10.1007/s11625-018-0557-2 doi: 10.1007/s11625-018-0557-2
![]() |
[48] |
López-Arceiz FJ, Bellostas AJ, Rivera MP (2017) Accessibility and transparency: Impact on social economy. Online Infor Rev 41: 35–52. https://doi.org/10.1108/OIR-09-2015-0296 doi: 10.1108/OIR-09-2015-0296
![]() |
[49] |
López-Arceiz FJ, Bellostas AJ, Rivera MP (2018) Twenty years of research on the relationship between economic and social performance: A meta-analysis approach. Soc Ind Res 140: 453–484. https://doi.org/10.1007/s11205-017-1791-1 doi: 10.1007/s11205-017-1791-1
![]() |
[50] |
López‐Arceiz FJ, Del Río C, Bellostas AJ (2020) Sustainability performance indicators: Definition, interaction, and influence of contextual characteristics. Corp Soc Resp Env Ma 27: 2615–2630. http://dx.doi.org/10.1002/csr.1986 doi: 10.1002/csr.1986
![]() |
[51] |
Ma JH, Choi SB, Ahn YH (2017) The impact of eco-friendly management on product quality, financial performance and environmental performance. J Distrib Sci 15: 17–28. https://doi.org/10.15722/jds.15.5.201705.17 doi: 10.15722/jds.15.5.201705.17
![]() |
[52] |
McArthur JW, Rasmussen K (2019) Classifying Sustainable Development Goal trajectories: A country-level methodology for identifying which issues and people are getting left behind. World Dev 123: 104608. https://doi.org/10.1016/j.worlddev.2019.06.031 doi: 10.1016/j.worlddev.2019.06.031
![]() |
[53] | Meyer JW (1979) The impact of the centralization of educational funding and control on state and local organizational governance. Stanford, CA: Institute for Research on Educational Finance and Governance, Stanford University, Program Report No. 79-B20. |
[54] | Meyer JW, Rowan B (1991) Institutionalized organizations: Formal structure as myth and ceremony, In: Dimaggio, P and Powell, W. (Eds), The new institutionalism in organizational analysis, Chicago: The University of Chicago Press. |
[55] |
Morioka SN, Bolis I, Carvalho MD (2018) From an ideal dream towards reality analysis: Proposing Sustainable Value Exchange Matrix (SVEM) from systematic literature review on sustainable business models and face validation. J Clean Prod 178: 76–88. http://doi.org/10.1016/j.jclepro.2017.12.078 doi: 10.1016/j.jclepro.2017.12.078
![]() |
[56] |
Muhmad SN, Muhamad R (2021) Sustainable business practices and financial performance during pre-and post-SDG adoption periods: A systematic review. J Sust Financ Invest 11: 291–309. https://doi.org/10.1080/20430795.2020.1727724 doi: 10.1080/20430795.2020.1727724
![]() |
[57] |
Muñoz-Torres MJ, Fernandez-Izquierdo MA, Rivera-Lirio JM, et al. (2019) Can environmental, social, and governance rating agencies favor business models that promote a more sustainable development? Corp Soc Resp Env Ma 26: 439–452. https://doi.org/10.1002/csr.1695 doi: 10.1002/csr.1695
![]() |
[58] |
Naciti V (2019) Corporate governance and board of directors: The effect of a board composition on firm sustainability performance. J Clean Prod 237: 117727. https://doi.org/10.1016/j.jclepro.2019.117727 doi: 10.1016/j.jclepro.2019.117727
![]() |
[59] |
Naomi P, Akbar I (2021) Beyond sustainability: empirical evidence from oecd countries on the connection among natural resources, ESG performances, and economic development. Econ Soc 14: 89–106. https://doi.org/10.14254/2071-789X.2021/14-4/5 doi: 10.14254/2071-789X.2021/14-4/5
![]() |
[60] |
Nicolò G, Zanellato G, Tiron-Tudor A, et al. (2022) Revealing the corporate contribution to Sustainable Development Goals through integrated reporting: A worldwide perspective. Soc Resp J. https://doi.org/10.1108/SRJ-09-2021-0373 doi: 10.1108/SRJ-09-2021-0373
![]() |
[61] |
Nurunnabi M (2015) The impact of cultural factors on the implementation of global accounting standards (IFRS) in a developing country. Adv Account 31: 136–149. http://dx.doi.org/10.1016/j.adiac.2015.03.015 doi: 10.1016/j.adiac.2015.03.015
![]() |
[62] |
Ordonez‐Ponce E (2023) The role of local cultural factors in the achievement of the sustainable development goals. Sust Dev 31: 1122–1134. https://doi.org/10.1002/sd.2445 doi: 10.1002/sd.2445
![]() |
[63] | Organisation for Economic Cooperation and Development (2017). Measuring distance to the SDG targets: An assessment of where OECD countries stand OECD. Paris: OECD. |
[64] |
Ortas E, Moneva JM (2011) Sustainability stock exchange indexes and investor expectations: Multivariate evidence from DJSI-Stoxx. Spanish J Financ Account 40: 395–416. https://doi.org/10.1080/02102412.2011.10779706 doi: 10.1080/02102412.2011.10779706
![]() |
[65] |
Pizzi S, Del Baldo M, Caputo F, et al. (2022) Voluntary disclosure of Sustainable Development Goals in mandatory non‐financial reports: The moderating role of cultural dimension. J Int Financ Manage Account 33: 83–106. https://doi.org/10.1111/jifm.12139 doi: 10.1111/jifm.12139
![]() |
[66] |
Pizzi S, Caputo A, Corvino A, et al. (2020) Management research and the UN Sustainable Development Goals (SDGs): A bibliometric investigation and systematic review. J Clean Prod 276: 124033. https://doi.org/10.1016/j.jclepro.2020.124033 doi: 10.1016/j.jclepro.2020.124033
![]() |
[67] |
Pizzi S, Rosati F, Venturelli A (2021) The determinants of business contribution to the 2030 Agenda: Introducing the SDG reporting score. Bus Strat Environ 30: 404–421. http://doi.org/10.1002/bse.2628 doi: 10.1002/bse.2628
![]() |
[68] | Powell WW, DiMaggio PJ (1984) The new institutionalism in organizational analysis, Chicago: The University of Chicago Press. |
[69] | Prexl A, Signitzer B (2008) When a new CEO is ahead: Leadership change processes in the light of change communication, personal public relations, and reputation management. Austria: Veröffentlicht. |
[70] |
Ramos DL, Chen S, Rabeeu A, et al. (2022) Does SDG coverage influence firm performance? Sustainability 14: 4870. https://doi.org/10.3390/su14094870 doi: 10.3390/su14094870
![]() |
[71] |
Richard PJ, Devinney TM, Yip GS, et al. (2009) Measuring organizational performance: Towards methodological best practice. J Manage 35: 718–804. https://doi.org/10.1177/0149206308330560 doi: 10.1177/0149206308330560
![]() |
[72] | RobecoSAM (2022) The sustainability yearbook 2021. Switzerland: S & P Global. |
[73] |
Rosati F, Faria L (2019a) Addressing the SDGs in sustainability reports: The relationship with institutional factors. J Clean Prod 215: 1312–1326. https://doi.org/10.1016/j.jclepro.2018.12.107 doi: 10.1016/j.jclepro.2018.12.107
![]() |
[74] |
Rosati F, Faria L (2019b) Business contribution to the Sustainable Development Agenda: organizational factors related to early adoption of SDG reporting. Corp Soc Resp Envir Ma 26: 588–597. https://doi.org/10.1002/csr.1705 doi: 10.1002/csr.1705
![]() |
[75] | Salem RB, Ayadi SD (2022) The impact of acculturation process and the institutional isomorphism on IFRS adoption. Euro Med J Bus, 1–23. |
[76] |
Santos MJ, Bastos CS (2020) The adoption of Sustainable Development Goals by large Portuguese companies. Soc Resp J 17: 1079–1099. https://doi.org/10.1108/SRJ-07-2018-0184 doi: 10.1108/SRJ-07-2018-0184
![]() |
[77] |
Scheyvens R, Banks G, Hughes E (2016) The private sector and the SDGs: The need to move beyond 'business as usual'. Sust Dev 24: 371–382. https://doi.org/10.1002/sd.1623 doi: 10.1002/sd.1623
![]() |
[78] |
Schramade W (2017) Investing in the UN Sustainable Development Goals: Opportunities for companies and investors. J Appl Corp Financ 29: 87–99. https://doi.org/10.1111/jacf.12236 doi: 10.1111/jacf.12236
![]() |
[79] | Scott WR (2013) Institutions and organizations: Ideas, interests, and identities, USA: Sage. |
[80] |
Sierra Garcia L, Bollas-Araya HM, Garcia Benau MA (2022) Sustainable development goals and assurance of non-financial information reporting in Spain. Sust Account Manage Policy J 13: 878–898. https://doi.org/10.1108/SAMPJ-04-2021-0131 doi: 10.1108/SAMPJ-04-2021-0131
![]() |
[81] |
Signorini P, Wiesemes R, Murphy R (2009) Developing alternative frameworks for exploring intercultural learning: a critique of Hofstede's cultural difference model. Teach Higher Edu 14: 253–264. https://doi.org/10.1080/13562510902898825 doi: 10.1080/13562510902898825
![]() |
[82] |
Stevens C, Kanie N (2016) The transformative potential of the Sustainable Development Goals (SDGs). Int Environ Agree 16: 393–396. https://doi.org/10.1007/s10784-016-9324-y doi: 10.1007/s10784-016-9324-y
![]() |
[83] |
Suchman MC (1995) Managing legitimacy: Strategic and institutional approaches. Acad Manage Rev 20: 571–610. https://doi.org/10.5465/amr.1995.9508080331 doi: 10.5465/amr.1995.9508080331
![]() |
[84] | United Nations (2015) Transforming our world: The 2030 Agenda for sustainable development. USA: United Nations. |
[85] | United Nations (2021) SDG Compass. Available from: https://sdgcompass.org/. |
[86] | United Nations Global Compact (2015) A global compact for sustainable development business and the SDGs: Acting responsibly and finding opportunities. Available from: https://www.unglobalcompact.org/library/2291. |
[87] | United Nations Global Compact (2020) SDG Ambition. Activating ambitous action to achieve the SDGs. USA: United Nations. |
[88] |
Van den Heiligenberg HA, Heimeriks GJ, Hekkert MP, et al. (2022) Pathways and harbours for the translocal diffusion of sustainability innovations in Europe. Environ Innov Soc Tr 42: 374–394. https://doi.org/10.1016/j.eist.2022.01.011 doi: 10.1016/j.eist.2022.01.011
![]() |
[89] | Van Tulder R (2018) Business and the sustainable development goals: A framework for effective corporate involvement, Rotterdam: Erasmus University Rotterdam. |
[90] | Van Zanten JA, Huij J (2022) Corporate sustainability performance: Introducing an SDG score and testing its validity relative to ESG ratings. Available at SSRN 4186680. |
[91] |
Van Zanten JA, Van Tulder R (2018) Multinational enterprises and the Sustainable Development Goals: An institutional approach to corporate engagement. J Int Bus Policy 1: 208–233. http://doi.org/10.1057/s42214-018-0008-x doi: 10.1057/s42214-018-0008-x
![]() |
[92] |
Vazquez-Brust D, Piao RS, de Melo MF, et al. (2020). The governance of collaboration for sustainable development: Exploring the "black box". Clean Prod 256: 120260. https://doi.org/10.1016/j.jclepro.2020.120260 doi: 10.1016/j.jclepro.2020.120260
![]() |
[93] |
Vildåsen SS (2018) Corporate sustainability in practice: An exploratory study of the sustainable development goals (SDGs). Bus Strat Dev 1: 256–264. https://doi.org/10.1002/bsd2.35 doi: 10.1002/bsd2.35
![]() |
[94] |
Vormedal IH, Ruud A (2009). Sustainability reporting in Norway - an assessment of performance in the context of legal demands and socio-political drivers. Bus Strat Environ 18: 207–222. https://doi.org/10.1002/bse.560 doi: 10.1002/bse.560
![]() |
[95] |
Wang Z, Reimsbach D, Braam G (2018) Political embeddedness and the diffusion of corporate social responsibility practices in China: A trade-off between financial and CSR performance? J Clean Prod 198: 1185–1197. https://doi.org/10.1016/j.jclepro.2018.07.116 doi: 10.1016/j.jclepro.2018.07.116
![]() |
[96] | World Business Council for Sustainable Development (2021) SDG Essentials for Business. World Business Council for Sustainable Development, Geneva. |
[97] |
Zabala-Aguayo F, Ślusarczyk B (2020) Risks of banking services' digitalization: The practice of diversification and Sustainable Development Goals. Sustainability 12: 4040. http://doi.org/10.3390/su12104040 doi: 10.3390/su12104040
![]() |
[98] |
Zampone G, García‐Sánchez IM, Sannino G (2023) Imitation is the sincerest form of institutionalization: Understanding the effects of imitation and competitive pressures on the reporting of sustainable development goals in an international context. Bus Strat Environ. https://doi.org/10.1002/bse.3357 doi: 10.1002/bse.3357
![]() |
[99] |
Zhang KQ, Chen HH (2017) Environmental performance and financing decisions impact on sustainable financial development of Chinese environmental protection enterprises. Sustainability 9: 1–14. http://doi.org/10.3390/su9122260 doi: 10.3390/su9122260
![]() |
![]() |
![]() |
1. | Antonio Hernando, José Luis Galán–García, Yolanda Padilla–Domínguez, María Ángeles Galán–García, Gabriel Aguilera–Venegas, A library in CoCoA for implementing railway interlocking systems, 2025, 466, 03770427, 116594, 10.1016/j.cam.2025.116594 |