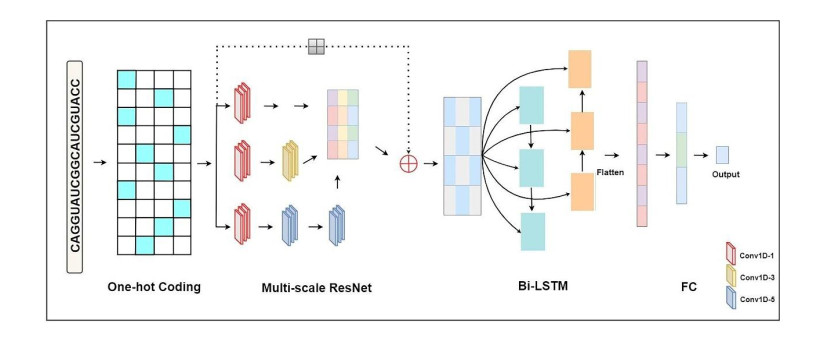
Phasic small interfering RNAs are plant secondary small interference RNAs that typically generated by the convergence of miRNAs and polyadenylated mRNAs. A growing number of studies have shown that miRNA-initiated phasiRNA plays crucial roles in regulating plant growth and stress responses. Experimental verification of miRNA-initiated phasiRNA loci may take considerable time, energy and labor. Therefore, computational methods capable of processing high throughput data have been proposed one by one. In this work, we proposed a predictor (DIGITAL) for identifying miRNA-initiated phasiRNAs in plant, which combined a multi-scale residual network with a bi-directional long-short term memory network. The negative dataset was constructed based on positive data, through replacing 60% of nucleotides randomly in each positive sample. Our predictor achieved the accuracy of 98.48% and 94.02% respectively on two independent test datasets with different sequence length. These independent testing results indicate the effectiveness of our model. Furthermore, DIGITAL is of robustness and generalization ability, and thus can be easily extended and applied for miRNA target recognition of other species. We provide the source code of DIGITAL, which is freely available at https://github.com/yuanyuanbu/DIGITAL.
Citation: Yuanyuan Bu, Jia Zheng, Cangzhi Jia. An efficient deep learning based predictor for identifying miRNA-triggered phasiRNA loci in plant[J]. Mathematical Biosciences and Engineering, 2023, 20(4): 6853-6865. doi: 10.3934/mbe.2023295
[1] | Keruo Jiang, Zhen Huang, Xinyan Zhou, Chudong Tong, Minjie Zhu, Heshan Wang . Deep belief improved bidirectional LSTM for multivariate time series forecasting. Mathematical Biosciences and Engineering, 2023, 20(9): 16596-16627. doi: 10.3934/mbe.2023739 |
[2] | Yufeng Qian . Exploration of machine algorithms based on deep learning model and feature extraction. Mathematical Biosciences and Engineering, 2021, 18(6): 7602-7618. doi: 10.3934/mbe.2021376 |
[3] | Long Wen, Liang Gao, Yan Dong, Zheng Zhu . A negative correlation ensemble transfer learning method for fault diagnosis based on convolutional neural network. Mathematical Biosciences and Engineering, 2019, 16(5): 3311-3330. doi: 10.3934/mbe.2019165 |
[4] | Jianhua Jia, Lulu Qin, Rufeng Lei . DGA-5mC: A 5-methylcytosine site prediction model based on an improved DenseNet and bidirectional GRU method. Mathematical Biosciences and Engineering, 2023, 20(6): 9759-9780. doi: 10.3934/mbe.2023428 |
[5] | Jianhua Jia, Mingwei Sun, Genqiang Wu, Wangren Qiu . DeepDN_iGlu: prediction of lysine glutarylation sites based on attention residual learning method and DenseNet. Mathematical Biosciences and Engineering, 2023, 20(2): 2815-2830. doi: 10.3934/mbe.2023132 |
[6] | Yutao Wang, Qian Shao, Shuying Luo, Randi Fu . Development of a nomograph integrating radiomics and deep features based on MRI to predict the prognosis of high grade Gliomas. Mathematical Biosciences and Engineering, 2021, 18(6): 8084-8095. doi: 10.3934/mbe.2021401 |
[7] | Shuai Cao, Biao Song . Visual attentional-driven deep learning method for flower recognition. Mathematical Biosciences and Engineering, 2021, 18(3): 1981-1991. doi: 10.3934/mbe.2021103 |
[8] | Honglei Wang, Wenliang Zeng, Xiaoling Huang, Zhaoyang Liu, Yanjing Sun, Lin Zhang . MTTLm6A: A multi-task transfer learning approach for base-resolution mRNA m6A site prediction based on an improved transformer. Mathematical Biosciences and Engineering, 2024, 21(1): 272-299. doi: 10.3934/mbe.2024013 |
[9] | Pingping Sun, Yongbing Chen, Bo Liu, Yanxin Gao, Ye Han, Fei He, Jinchao Ji . DeepMRMP: A new predictor for multiple types of RNA modification sites using deep learning. Mathematical Biosciences and Engineering, 2019, 16(6): 6231-6241. doi: 10.3934/mbe.2019310 |
[10] | H. Swapnarekha, Janmenjoy Nayak, H. S. Behera, Pandit Byomakesha Dash, Danilo Pelusi . An optimistic firefly algorithm-based deep learning approach for sentiment analysis of COVID-19 tweets. Mathematical Biosciences and Engineering, 2023, 20(2): 2382-2407. doi: 10.3934/mbe.2023112 |
Phasic small interfering RNAs are plant secondary small interference RNAs that typically generated by the convergence of miRNAs and polyadenylated mRNAs. A growing number of studies have shown that miRNA-initiated phasiRNA plays crucial roles in regulating plant growth and stress responses. Experimental verification of miRNA-initiated phasiRNA loci may take considerable time, energy and labor. Therefore, computational methods capable of processing high throughput data have been proposed one by one. In this work, we proposed a predictor (DIGITAL) for identifying miRNA-initiated phasiRNAs in plant, which combined a multi-scale residual network with a bi-directional long-short term memory network. The negative dataset was constructed based on positive data, through replacing 60% of nucleotides randomly in each positive sample. Our predictor achieved the accuracy of 98.48% and 94.02% respectively on two independent test datasets with different sequence length. These independent testing results indicate the effectiveness of our model. Furthermore, DIGITAL is of robustness and generalization ability, and thus can be easily extended and applied for miRNA target recognition of other species. We provide the source code of DIGITAL, which is freely available at https://github.com/yuanyuanbu/DIGITAL.
Plant virus diseases have brought great losses to agriculture. RNA interference (RNAi) attracts more and more attention as one important mechanism of plant resistance to viruses [1]. There are mainly three types of key proteins in RNAi: Dicer-like (DCL), RNA-dependent RNA polymerase (PDR) and Argonaute (AGO) [2,3,4]. The main process is that: (1) DCL cuts double strand RNA (dsRNA) into primary small interference RNA (siRNA); (2) PDR reconstitutes siRNA into dsRNA, and then cuts the newly synthesized dsRNA into more secondary siRNA; (3) AGO is combined with siRNA to form RNA silencing complex (RISC) [5]. RNAi can cut the RISC, target and ultimately degrade virus or RNA nucleic acid sequence through complementary base pairs. SiRNAs, in the size range of 21–24 nucleotides, mediate RNAi and play the most important mechanism in the whole process of RNAi [6]. The main activity of siRNAs is the negative regulation of specific mRNAs or gene expression through target degradation, translational repression, or directing chromatin modification [7,8].
Phasic small interfering RNAs (phasiRNAs) are plant secondary siRNAs that typically produced by miRNAs targeting polyadenylated mRNAs [9]. A growing number of studies have shown that miRNA-initiated phasiRNAs play crucial roles in regulating plant growth and stress responses [10,11,12]. Substantial analyses in genome and small RNA (sRNA) sequence enhanced the annotations of sRNAs, notably phasiRNAs as well as their targets [13]; therefore relevant databases have been established in succession. Recently, Liu et al. [14] established a database named TarDB that contained 62,888 cross-species conserved miRNA targets, 4304 degradome PARE-seq supported miRNA targets and 3182 miRNA triggered phasiRNA loci.
Given the importance of phasiRNA in plant-pathogen interactions, we proposed an efficient deep learning based predictor, named DIGITAL, for identifying miRNA-triggered phasiRNA loci. We collected experimental verified duplex mRNA and phasiRNAs from TarDB database, and generated the negative dataset by randomly substituting a certain number of nucleotides in positive samples. The key architecture of DIGITAL consists of a multi-scale residual network (multi-scale ResNet) and a bi-directional long-short term memory (bi-LSTM) network. Consequently, when tested on two independent test sets of 21-nt and 24-nt phasiRNAs, DIGITAL reached the accuracy of 98.45% and 94.02%, respectively, which proves its good robustness and generalization ability.
Figure 1 illustrates the overall design of DIGITAL. The input layer transforms each nucleic acid into a four-dimensional binary vector by one-hot encoding, which means A, C, G and T are represented as (1 0 0 0), (0 1 0 0), (0 0 1 0) and (0 0 0 1), respectively. To get the feature vectors with the same dimension, we use the way of supplementing 0. Then a deep residual block formed by multi-scale CNN layers is employed to extract local relevant features in input vectors; besides, the bi-directional long-short term memory (bi-LSTM) network is implemented to explore long-range global contextual information. Finally, the resultant latent information is integrated through a flattened layer, and a following fully connected layer with softmax is adopted for label classification.
We collected the siRNA sequence information from the TarDB database. [14] This database contains three categories of relatively high-confidence plant miRNA targets: (i) cross-species conserved miRNA targets; (ii) degradome/PARE (Parallel Analysis of RNA Ends) sequencing supported miRNA targets; (iii) miRNA-triggered phasiRNA loci. However, only the miRNA-triggered phasiRNAs were used to construct our prediction model, because they have been identified by previous well-documented criteria [15,16,17,18].
The TarDB platform deposits both 21-nt and 24-nt phasiRNA in various plants. We obtained 6389 miRNA-phasiRNA target duplex in which miRNA triggered 21-nt phasiRNA, as well as 526 miRNA-phasiRNA target duplex in which miRNA triggered 24-nt phasiRNA in 43 plant species. After removing the repetitive miRNA-target pair, there are 5,408 duplex data left for miRNA-initiated 21-nt phasiRNAs, altogether with 443 duplex data for miRNA-initiated 24-nt phasiRNA, as positive samples.
The approach to constructing corresponding negative dataset is similar to the method proposed by Mhaned Oubounyt et al. [19], based on the fact that positive and negative sets with less intersection are easier to distinguish [20]. In detail, each positive sequence is divided into multiple 1bp long fragments, and 60% of the fragments are selected and replaced randomly, with the remaining 40% conserved. In this approach, each negative sequence is generated from a positive sequence, and they are equal in length. Also, the number of negative data generated by this process is equivalent to that of positive data.
In addition, the miRNA dataset that initiates 21-nt phasiRNAs is further divided into three subsets, including the training dataset (60% of the original dataset), the validation dataset (20% of the original dataset) and the independent test dataset (20% of the original dataset, denoted as dataset test_21), where the training set is used to train the classifier, the validation set is used to optimize hyper-parameters and the independent test set is used to evaluate the performance of DIGITAL. The miRNA dataset that initiates 24-nt phasiRNAs is also used as an independent test set to evaluate the performance of DIGITAL, denoted as dataset test_24. The statistics of each dataset are shown in Table 1.
Dataset | Positive | Negative |
Training | 3244 | 3244 |
Validation | 1082 | 1082 |
Test_21 | 1082 | 1082 |
Test_24 | 443 | 443 |
Fundamental structures in DIGITAL are a multi-scale ResNet network and a bi-LSTM architecture, which have been used by some researches [21,22,23]. Compared with the traditional CNN, the residual network improves the interaction of information, and avoids the gradient disappearance and degradation problems caused by network depth. So we used multi-scale ResNet network with identity mapping. At the same time, in order to extract long-term global context information, we combined multi-scale ResNet network and BiLSTM. Details are as follows.
The multi-scale ResNet network includes three channels of 1-dimension CNN with 64 convolution filters. Among them, the first channel contains one convolution layer, and the size of the convolution kernel is fixed to 1; the second channel employs two convolution layers, with kernels in size 1 and 3, respectively; the third channel uses three convolution layers, and the sizes of the corresponding convolution kernel are set as 1, 5 and 5, respectively. The bi-LSTM with a self-attention network consists of 121 hidden units, followed by a fully-connected layer with 16 units. The Adam optimizer with a batch size of 110 simultaneously trains all layers in our model, and the learning rate scheduler in Keras is employed to regulate the learning rate. Early stopping is applied based on validation loss. To provide insight into the training process of DIGITAL, the average validation loss and accuracy change during training are shown in Supplementary Figure S1.
We evaluate DIGITAL based on four most common metrics, containing sensitivity (Sn), specificity (Sp), accuracy (Acc), and Matthew's correlation coefficient (MCC). The formulas are listed as below:
{Sp=TNTN+FPSn=TPFN+TPAcc=TP+TNTP+TN+FN+FPMcc=TP×TN−FP×FN√(TP+FN)(FP+TN)(TP+FP)(FN+TN) | (1) |
where TP, TN, FP and FN represent the number of true positives, true negatives, false positives and false negatives, respectively. In addition, the area under the receiver operating characteristic curve (AUC) is also used to examine the performance of DIGITAL.
In this study, we proposed a deep learning model, named DIGITAL, based on multi-scale ResNet network and bi-LSTM to predict miRNA-triggered phasiRNA loci. During training, Bayesian optimization was used to search the most appropriate parameters for identifying miRNA-triggered phasiRNA sites. DIGITAL reaches the satisfying Acc of 98.45% and 94.02% on independent datasets test_21 and test_24, respectively. In addition, six traditional classification algorithms were also constructed and compared with DIGITAL. In empirical studies based on independent tests, DIGITAL outperforms six traditional classification algorithms, and this fact demonstrates the effectiveness of our model. In addition, the robustness and generalization ability of DIGITAL suggest it can be easily extended and applied for recognizing miRNA targets of other species.
Bayesian optimization is a very effective global optimization algorithm widely used in multitudinous prediction tasks in bioinformatics [24,25,26,27]. In this work, to further improve the performance of DIGITAL, we also applied this method to optimize key hyper-parameters in the training process. As works in previous [28,29], the difference between the experimental value and the predictive value on the validation set is defined as the fitness function evaluation of the hyper-parameter optimization during the training process. The unit number in Bi-LSTM [30,31,32] and the fully-connected layer, as well as the batch size, all varies in the range of (16,128). Corresponding results for each combination are listed in Supplementary Table S1, and the best results with the Acc of 98.71%, MCC of 96.13%, and AUC of 99.78% are achieved at the combination of (121, 16,110).
In addition, we also choose the parameters by empirical methods [33,34], where the unit number of Bi-LSTM is set as 64, the unit number of the fully-connected layer is set as 32, and the batch size is set as 100. Prediction performance of this combination is shown Figure 2 as DIGITAL_E. As shown in Figure 2, the model based on Bayesian optimization achieved superior results on the validation dataset. Thus, the final model for phasiRNA identification is designed as 121 units in Bi-LSTM, 16 units in the fully-connected layer, and the batch size is designed as 110. DIGITAL denotes a Bayesian optimization and DIGITAL_E denotes an empirical parameter.
In this section, the independent datasets test_21 and test_24 are applied to further evaluate the robustness and generalization ability of DIGITAL. As shown in Table 1, DIGITAL obtains the Acc of 98.48%, Sn of 98.95%, Sp of 98.02% and MCC of 96.95% on independent dataset test_21, and achieves the Acc of 94.02%, Sn of 95.04%, Sp of 93.00% and MCC of 88.05% on independent dataset test_24. In order to display the prediction results more intuitively, we plot the ROC curves and calculate the AUC values, as shown in Figure 3. Our model achieves satisfactory AUC of 99.88% on the independent dataset test_21 and AUC of 98.41% on the independent dataset test_24. The similar prediction performance demonstrates that DIGITAL has good robustness and generalization ability. Besides, these two groups of results also demonstrate that the length of the sequence has a great influence on the prediction performance. With the increasing amount of data in the future, it is necessary to establish special predictors aiming at different sequence lengths.
In addition, we also implemented 5-fold and 10-fold cross-validation tests to further evaluate the generalization capability, respectively, and listed the average results in the Supplementary Table S2. We observed that COPPER achieved the average Acc of 98.14% and 98.30% on 5-fold and 10-fold cross-validation, respectively. The k-fold (k = 5, 10) results are basically consistent with those results on validation dataset.
In addition to deep learning classification algorithm, we also applied six other commonly used traditional machine learning methods to develop predictive models, consisting of support vector machines (SVM), Naive Bayes (NB), k-nearest neighbors (KNN), XGBoost, logistic regression (LR), and random forest (RF). For each classification algorithm, we implemented parameter selection to achieve the best prediction results. Prediction performances before and after parameter selection on the validation dataset are shown in Supplementary Figure S2. It is surprising that except KNN, the other models do not show significant change before and after parameter selection. For this reason, we tested the six models using default parameters on our two independent datasets and compared them with DIGITAL. As shown in Table 2, DIGITAL reveals better predictive performance relative to the other predictors in terms of MCC, Acc, Sn and Sp, except for Sp on which random forest reaches the best performance. Specifically, the MCC of DIGITAL is 1% higher than the second best method SVM on test_21 dataset, and 16.9% higher than the second best method XGBoost on test_24 dataset. The improved MCC suggests that the Sn and Sp are balanced and relatively similar.
Method | Dataset | Sn(%) | Sp(%) | Acc(%) | MCC | AUC |
DIGITAL | test_21 | 98.95 | 98.02 | 98.45 | 0.969 | 0.999 |
test_24 | 95.04 | 93.00 | 94.02 | 0.881 | 0.984 | |
SVM | test_21 | 96.08 | 99.81 | 97.92 | 0.959 | 0.979 |
test_24 | 43.57 | 99.09 | 71.33 | 0.513 | 0.713 | |
KNN | test_21 | 97.72 | 12.57 | 55.78 | 0.197 | 0.551 |
test_24 | 83.97 | 73.81 | 78.89 | 0.581 | 0.789 | |
NB | test_21 | 88.89 | 99.81 | 94.27 | 0.891 | 0.944 |
test_24 | 1.58 | 98.65 | 50.11 | 0.009 | 0.501 | |
XGBoost | test_21 | 97.63 | 98.87 | 98.24 | 0.965 | 0.983 |
test_24 | 73.14 | 96.36 | 84.65 | 0.712 | 0.847 | |
LR | test_21 | 95.26 | 94.28 | 94.78 | 0.896 | 0.948 |
test_24 | 11.29 | 92.10 | 51.69 | 0.058 | 0.517 | |
RF | test_21 | 95.81 | 1.0 | 97.87 | 0.958 | 0.979 |
test_24 | 4.51 | 1.0 | 52.26 | 0.152 | 0.523 |
As shown in Table 2, for all the seven classification algorithms, prediction results on dataset test_24 are inferior to those on dataset test_21. This may be due to these models are established based on miRNA-initiated 21-nt phasiRNAs. In the future, we shall pay efforts to overcome the influence of sequence length on the model.
In this section, we constructed the classification model based on word2vec embedding method. We adopted the grammar of 1, window size of context 4 and dimensions of embedding vector of 4 because the dimension of one-hot is also 4. When training the embedding matrix, we chose our training set as the corpus. The comparison of one-hot and word2vec is shown in Figure 4. It can be seen that the model based on one-hot encoding reached the best performance on validation for all of five indicators, and gave relatively low Sps and high values of other for indicators on both test-21 and test-24 datasets. Therefore, we provided the code of two models at https://github.com/yuanyuanbu/DIGITAL.
The hybrid network of DIGITAL is composed of multi-scale ResNet and bi-LSTM these two parts. To analyze the role of each part, we built two based models based on only multi-scale ResNet and bi-LSTM, respectively. The prediction results are listed in Table 3 of measurement by five evaluation indictors. It can be observed that DIGITAL obviously outperformed other two models on for indicators of Sn, Acc, MCC and AUC, especially with the improvement of more than 5% for Sn, but the model based on multi-scale ResNet achieved the high Sp of 99.34% and the model based on only bi-LSTM achieved the high Sp of 98.88%. The reason why the integration of multi-scale ResNet and bi-LSTM can improve Sn significantly is worth studying in the future.
Model | Sn(%) | Sp(%) | Acc(%) | MCC | AUC |
DIGITAL | 98.86 | 97.31 | 98.06 | 0.961 | 0.998 |
Only bi-LSTM | 91.57 | 99.34 | 95.37 | 0.910 | 0.994 |
Only multi-scale ResNet | 93.86 | 98.88 | 96.35 | 0.928 | 0.969 |
In order to intuitively display the process of deep learning to distinguish samples, we employed the popular visualization algorithm termed t-distributed stochastic neighbor embedding (t-SNE) which has been used in bioinformatics. [35,36] As illustrated in Figure 5A and 5B, these two kinds of points are mixed up in confusion by using one-hot encoding and after Multi-scale ResNet. In contrast, most of the points in the two kinds have been separated after bi-LSTM, except that the boundary is not obvious (Figure 5C). Through the last Dense layer, the two types of points are almost completely separated, and the boundary is clear. Taken together, it can be concluded the DIGITAL framework can effectively learn the effective information from the one-hot encoding mapped from the RNA sequences.
This work was supported by the Fundamental Research Funds for the Central Universities 3132022204.
The authors declare no competing interests.
Iter | Target | Bi-LSTM | Dense | Batch_size |
1 | 0.9815 | 43 | 75 | 23 |
2 | 0.9815 | 28 | 105 | 61 |
3 | 0.9797 | 45 | 73 | 41 |
4 | 0.9852 | 58 | 125 | 64 |
5 | 0.9838 | 127 | 67 | 26 |
6 | 0.9797 | 74 | 128 | 60 |
7 | 0.9871 | 121 | 16 | 110 |
8 | 0.9834 | 113 | 73 | 123 |
9 | 0.9866 | 98 | 42 | 61 |
10 | 0.9838 | 97 | 79 | 106 |
11 | 0.9783 | 38 | 66 | 98 |
12 | 0.9810 | 30 | 107 | 116 |
13 | 0.9806 | 126 | 86 | 18 |
14 | 0.9834 | 42 | 19 | 81 |
15 | 0.9806 | 70 | 41 | 55 |
16 | 0.9834 | 99 | 111 | 35 |
17 | 0.9801 | 98 | 41 | 60 |
18 | 0.9838 | 106 | 99 | 89 |
19 | 0.9815 | 56 | 97 | 39 |
20 | 0.9866 | 112 | 104 | 95 |
21 | 0.9861 | 76 | 119 | 52 |
22 | 0.9847 | 81 | 89 | 112 |
23 | 0.9797 | 110 | 24 | 61 |
24 | 0.9820 | 44 | 89 | 106 |
25 | 0.9810 | 69 | 65 | 85 |
26 | 0.9857 | 82 | 19 | 109 |
27 | 0.9838 | 79 | 87 | 73 |
28 | 0.9834 | 61 | 43 | 38 |
29 | 0.9783 | 97 | 80 | 106 |
30 | 0.9857 | 98 | 21 | 59 |
Sn(%) | Sp(%) | Acc(%) | MCC | AUC | |
5-fold | 98.44 | 97.83 | 98.14 | 0.963 | 0.997 |
10-fold | 98.61 | 97.99 | 98.30 | 96.61 | 99.78 |
[1] |
B. He, J. Huang, H. Chen, PVsiRNAPred: Prediction of plant exclusive virus-derived small interfering RNAs by deep convolutional neural network, J Bioinform. Comput. Biol., 17 (2019), 1950039. https://doi.org/10.1142/S0219720019500392 doi: 10.1142/S0219720019500392
![]() |
[2] |
D. Baulcombe, RNA silencing in plants, Nature, 431 (2004), 356-363. https://doi.org/10.1038/nature02874 doi: 10.1038/nature02874
![]() |
[3] |
E. J. Chapman, J. C. Carrington, Specialization and evolution of endogenous small RNA pathways, Nat. Rev. Genet., 8 (2007), 884-896. https://doi.org/10.1038/nrg2179 doi: 10.1038/nrg2179
![]() |
[4] |
M. Niu, Y. Lin, Q. Zou, sgRNACNN: Identifying sgRNA on-target activity in four crops using ensembles of convolutional neural networks, Plant. Mol. Biol., 105 (2021), 483-495. https://doi.org/10.1007/s11103-020-01102-y doi: 10.1007/s11103-020-01102-y
![]() |
[5] |
S. M. Hammond, E. Bernstein, D. Beach, G. J. Hannon, An RNA-directed nuclease mediates post-transcriptional gene silencing in Drosophila cells, Nature, 404 (2000), 293-296. https://doi.org/10.1038/35005107 doi: 10.1038/35005107
![]() |
[6] |
S.-W. Ding, R. Lu, Virus-derived siRNAs and piRNAs in immunity and pathogenesis, Curr. Opin. Virol., 1 (2011), 533-544. https://doi.org/10.1016/j.coviro.2011.10.028 doi: 10.1016/j.coviro.2011.10.028
![]() |
[7] |
X. Chen, Small RNAs and their roles in plant development, Annu. Rev. Cell. Dev. Biol., 25 (2009), 21-44. https://doi.org/10.1146/annurev.cellbio.042308.113417 doi: 10.1146/annurev.cellbio.042308.113417
![]() |
[8] |
C. Cao, J. Wang, D. Kwok, F. Cui, Z. Zhang, D. Zhao, et al., WebTWAS: A resource for disease candidate susceptibility genes identified by transcriptome-wide association study, Nucleic Acids Res., 50 (2021), D1123-D1130. https://doi.org/10.1093/nar/gkab957 doi: 10.1093/nar/gkab957
![]() |
[9] |
X. Song, P. Li, J. Zhai, M. Zhou, L. Ma, B. Liu, et al., Roles of DCL4 and DCL3b in rice phased small RNA biogenesis, Plant J., 69 (2012), 462-474. https://doi.org/10.1111/j.1365-313X.2011.04805.x doi: 10.1111/j.1365-313X.2011.04805.x
![]() |
[10] |
Y. Liu, C. Teng, R. Xia, B. C. Meyers, PhasiRNAs in Plants: Their biogenesis, genic sources, and roles in stress responses, development, and reproduction, Plant Cell, 32 (2020), 3059-3080. https://doi.org/10.1105/tpc.20.00335 doi: 10.1105/tpc.20.00335
![]() |
[11] |
Q. Fei, R. Xia, B. C. Meyers, Phased, secondary, small interfering RNAs in posttranscriptional regulatory networks, Plant Cell, 25 (2013), 2400-2415. https://doi.org/10.1105/tpc.113.114652 doi: 10.1105/tpc.113.114652
![]() |
[12] |
S. Belanger, S. Pokhrel, K. Czymmek, B. C. Meyers, Premeiotic, 24-nucleotide reproductive phasiRNAs are abundant in anthers of wheat and barley but not rice and maize, Plant Physiol., 184 (2020), 1407-1423. https://doi.org/10.1104/pp.20.00816 doi: 10.1104/pp.20.00816
![]() |
[13] |
C. Chen, J. Li, J. Feng, B. Liu, L. Feng, X. Yu, et al., sRNAanno-a database repository of uniformly annotated small RNAs in plants, Hortic Res., 8 (2021), 45. https://doi.org/10.1038/s41438-021-00480-8 doi: 10.1038/s41438-021-00480-8
![]() |
[14] |
J. Liu, X. Liu, S. Zhang, S. Liang, W. Luan, X. Ma, TarDB: An online database for plant miRNA targets and miRNA-triggered phased siRNAs, BMC Genomics, 22 (2021), 348. https://doi.org/10.1186/s12864-021-07680-5 doi: 10.1186/s12864-021-07680-5
![]() |
[15] |
H. M. Chen, L. T. Chen, K. Patel, Y. H. Li, D. C. Baulcombe, S. H. Wu, 22-Nucleotide RNAs trigger secondary siRNA biogenesis in plants, Proc. Natl. Acad. Sci. U. S. A., 107 (2010), 15269-15274. https://doi.org/10.1073/pnas.1001738107 doi: 10.1073/pnas.1001738107
![]() |
[16] |
R. Xia, J. Xu, S. Arikit, B. C. Meyers, Extensive families of miRNAs and PHAS Loci in Norway spruce demonstrate the origins of complex phasiRNA networks in seed plants, Mol. Biol. Evol., 32 (2015), 2905-2918. https://doi.org/10.1093/molbev/msv164 doi: 10.1093/molbev/msv164
![]() |
[17] |
J. Zhai, D. H. Jeong, E. De Paoli, S. Park, B. D. Rosen, Y. Li, et al., MicroRNAs as master regulators of the plant NB-LRR defense gene family via the production of phased, trans-acting siRNAs, Genes Dev., 25 (2011), 2540-2553. https://doi.org/10.1101/gad.177527.111 doi: 10.1101/gad.177527.111
![]() |
[18] |
E. de Paoli, A. Dorantes-Acosta, J. Zhai, M. Accerbi, D. H. Jeong, S. Park, et al., Distinct extremely abundant siRNAs associated with cosuppression in petunia, RNA, 15 (2009), 1965-1970. https://doi.org/10.1261/rna.1706109 doi: 10.1261/rna.1706109
![]() |
[19] |
M. Oubounyt, Z. Louadi, H. Tayara, K. T. Chong, DeePromoter: Robust promoter predictor using deep learning, Front. Genet., 10 (2019), 286. https://doi.org/10.3389/fgene.2019.00286 doi: 10.3389/fgene.2019.00286
![]() |
[20] | Y. Qian, Y. Zhang, B. Guo, S. Ye, Y. Wu, J. Zhang, An improved promoter recognition model using convolutional neural network, in 2018 IEEE 42nd Annual Computer Software and Applications Conference (COMPSAC), (2018), 471-476. https://doi.org/10.1109/COMPSAC.2018.00072 |
[21] |
Y. Yang, Z. Hou, Z. Ma, X. Li, K. C. Wong, iCircRBP-DHN: Identification of circRNA-RBP interaction sites using deep hierarchical network, Brief. Bioinform., 22 (2021). https://doi.org/10.1093/bib/bbaa274 doi: 10.1093/bib/bbaa274
![]() |
[22] |
D. Wang, C. Zhang, B. Wang, B. Li, Q. Wang, D. Liu, et al., Optimized CRISPR guide RNA design for two high-fidelity Cas9 variants by deep learning, Nat. Commun., 10 (2019), 4284. https://doi.org/10.1038/s41467-019-12281-8 doi: 10.1038/s41467-019-12281-8
![]() |
[23] |
Neeraj, V. Singhal, J. Mathew, R. K. Behera, Detection of alcoholism using EEG signals and a CNN-LSTM-ATTN network, Comput. Biol. Med., 138 (2021), 104940. https://doi.org/10.1016/j.compbiomed.2021.104940 doi: 10.1016/j.compbiomed.2021.104940
![]() |
[24] |
Q. Liu, J. Chen, Y. Wang, S. Li, C. Jia, J. Song, et al., DeepTorrent: A deep learning-based approach for predicting DNA N4-methylcytosine sites, Brief. Bioinform., 22 (2020). https://doi.org/10.1093/bib/bbaa124 doi: 10.1093/bib/bbaa124
![]() |
[25] |
Y. Zhu, F. Li, D. Xiang, T. Akutsu, J. Song, C. Jia, Computational identification of eukaryotic promoters based on cascaded deep capsule neural networks, Briefi. Bioinform., 22 (2020). https://doi.org/10.1093/bib/bbaa299 doi: 10.1093/bib/bbaa299
![]() |
[26] |
D. Salimi, A. Moeini, Incorporating K-mers highly correlated to epigenetic modifications for Bayesian inference of gene interactions, Curr. Bioinform., 16 (2021), 484-492. https://doi.org/10.2174/1574893615999200728193621 doi: 10.2174/1574893615999200728193621
![]() |
[27] |
S. Ye, Y. Liang, B. Zhang, Bayesian functional mixed-effects models with grouped smoothness for analyzing time-course gene expression data, Curr. Bioinform., 16 (2021), 2-12. https://doi.org/10.2174/1574893615999200520082636 doi: 10.2174/1574893615999200520082636
![]() |
[28] |
D. Chai, C. Jia, J. Zheng, Q. Zou, F. Li, Staem5: A novel computational approachfor accurate prediction of m5C site, Mol. Ther. Nucl. Acids., 26 (2021), 1027-1034. https://doi.org/10.1016/j.omtn.2021.10.012 doi: 10.1016/j.omtn.2021.10.012
![]() |
[29] |
H. Abbasimehr, R. Paki, Prediction of COVID-19 confirmed cases combining deep learning methods and Bayesian optimization, Chaos Solitons Fractals, 142 (2021), 110511. https://doi.org/10.1016/j.chaos.2020.110511 doi: 10.1016/j.chaos.2020.110511
![]() |
[30] |
J. Chen, Q. Zou, J. Li, DeepM6ASeq-EL: Prediction of human N6-Methyladenosine (m6A) sites with LSTM and ensemble learning, Front.. Comput. Sci., 16 (2022), 162302. https://doi.org/10.1007/s11704-020-0180-0 doi: 10.1007/s11704-020-0180-0
![]() |
[31] |
A. K. Sharma, R. Srivastava, Protein secondary structure prediction using character Bi-gram embedding and Bi-LSTM, Curr. Bioinform., 16 (2021), 333-338. https://doi.org/10.2174/1574893615999200601122840 doi: 10.2174/1574893615999200601122840
![]() |
[32] |
A. Rafiei, A. Rezaee, F. Hajati, S. Gheisari, M. Golzan, SSP: Early prediction of sepsis using fully connected LSTM-CNN model, Comput. Biol. Med., 128 (2021), 104110. https://doi.org/10.1016/j.compbiomed.2020.104110 doi: 10.1016/j.compbiomed.2020.104110
![]() |
[33] |
H. Lv, F. Y. Dao, Z. X. Guan, H. Yang, Y. W. Li, H. Lin, Deep-Kcr: Accurate detection of lysine crotonylation sites using deep learning method, Brief. Bioinform., 22 (2021), 255. https://doi.org/10.1093/bib/bbaa255 doi: 10.1093/bib/bbaa255
![]() |
[34] |
S. Gholamizoj, B. Ma, SPEQ: Quality assessment of peptide tandem mass spectra with deep learning, Bioinformatics, 38 (2022), 1568-1574. https://doi.org/10.1093/bioinformatics/btab874 doi: 10.1093/bioinformatics/btab874
![]() |
[35] | D. D. S. Lima, L. J. A. Amichi, A. A. Constantino, M. A. Fernandez, F. A. V. Seixas, NCYPred: A bidirectional LSTM network with attention for Y RNA and short non-coding RNA classification, IEEE-ACM Trans. Comput. Biol. Bioinform. (2021), 1-1. https://doi.org/10.1109/TCBB.2021.3131136 |
[36] | M. L. Chen, A. Doddi, J. Royer, L. Freschi, M. Schito, M. Ezewudo, et al., Deep learning predicts tuberculosis drug resistance status from genome sequencing data, BioRxiv, (2018), 275628. https://doi.org/10.1101/275628 |
1. | Shree Prakash Pandey, 2024, 9781394209934, 283, 10.1002/9781394209965.ch12 | |
2. | Shree P. Pandey, Priyanka Pandey, Prashant K. Srivastava, 2025, Chapter 3, 978-1-0716-4397-6, 73, 10.1007/978-1-0716-4398-3_3 |
Dataset | Positive | Negative |
Training | 3244 | 3244 |
Validation | 1082 | 1082 |
Test_21 | 1082 | 1082 |
Test_24 | 443 | 443 |
Method | Dataset | Sn(%) | Sp(%) | Acc(%) | MCC | AUC |
DIGITAL | test_21 | 98.95 | 98.02 | 98.45 | 0.969 | 0.999 |
test_24 | 95.04 | 93.00 | 94.02 | 0.881 | 0.984 | |
SVM | test_21 | 96.08 | 99.81 | 97.92 | 0.959 | 0.979 |
test_24 | 43.57 | 99.09 | 71.33 | 0.513 | 0.713 | |
KNN | test_21 | 97.72 | 12.57 | 55.78 | 0.197 | 0.551 |
test_24 | 83.97 | 73.81 | 78.89 | 0.581 | 0.789 | |
NB | test_21 | 88.89 | 99.81 | 94.27 | 0.891 | 0.944 |
test_24 | 1.58 | 98.65 | 50.11 | 0.009 | 0.501 | |
XGBoost | test_21 | 97.63 | 98.87 | 98.24 | 0.965 | 0.983 |
test_24 | 73.14 | 96.36 | 84.65 | 0.712 | 0.847 | |
LR | test_21 | 95.26 | 94.28 | 94.78 | 0.896 | 0.948 |
test_24 | 11.29 | 92.10 | 51.69 | 0.058 | 0.517 | |
RF | test_21 | 95.81 | 1.0 | 97.87 | 0.958 | 0.979 |
test_24 | 4.51 | 1.0 | 52.26 | 0.152 | 0.523 |
Model | Sn(%) | Sp(%) | Acc(%) | MCC | AUC |
DIGITAL | 98.86 | 97.31 | 98.06 | 0.961 | 0.998 |
Only bi-LSTM | 91.57 | 99.34 | 95.37 | 0.910 | 0.994 |
Only multi-scale ResNet | 93.86 | 98.88 | 96.35 | 0.928 | 0.969 |
Iter | Target | Bi-LSTM | Dense | Batch_size |
1 | 0.9815 | 43 | 75 | 23 |
2 | 0.9815 | 28 | 105 | 61 |
3 | 0.9797 | 45 | 73 | 41 |
4 | 0.9852 | 58 | 125 | 64 |
5 | 0.9838 | 127 | 67 | 26 |
6 | 0.9797 | 74 | 128 | 60 |
7 | 0.9871 | 121 | 16 | 110 |
8 | 0.9834 | 113 | 73 | 123 |
9 | 0.9866 | 98 | 42 | 61 |
10 | 0.9838 | 97 | 79 | 106 |
11 | 0.9783 | 38 | 66 | 98 |
12 | 0.9810 | 30 | 107 | 116 |
13 | 0.9806 | 126 | 86 | 18 |
14 | 0.9834 | 42 | 19 | 81 |
15 | 0.9806 | 70 | 41 | 55 |
16 | 0.9834 | 99 | 111 | 35 |
17 | 0.9801 | 98 | 41 | 60 |
18 | 0.9838 | 106 | 99 | 89 |
19 | 0.9815 | 56 | 97 | 39 |
20 | 0.9866 | 112 | 104 | 95 |
21 | 0.9861 | 76 | 119 | 52 |
22 | 0.9847 | 81 | 89 | 112 |
23 | 0.9797 | 110 | 24 | 61 |
24 | 0.9820 | 44 | 89 | 106 |
25 | 0.9810 | 69 | 65 | 85 |
26 | 0.9857 | 82 | 19 | 109 |
27 | 0.9838 | 79 | 87 | 73 |
28 | 0.9834 | 61 | 43 | 38 |
29 | 0.9783 | 97 | 80 | 106 |
30 | 0.9857 | 98 | 21 | 59 |
Sn(%) | Sp(%) | Acc(%) | MCC | AUC | |
5-fold | 98.44 | 97.83 | 98.14 | 0.963 | 0.997 |
10-fold | 98.61 | 97.99 | 98.30 | 96.61 | 99.78 |
Dataset | Positive | Negative |
Training | 3244 | 3244 |
Validation | 1082 | 1082 |
Test_21 | 1082 | 1082 |
Test_24 | 443 | 443 |
Method | Dataset | Sn(%) | Sp(%) | Acc(%) | MCC | AUC |
DIGITAL | test_21 | 98.95 | 98.02 | 98.45 | 0.969 | 0.999 |
test_24 | 95.04 | 93.00 | 94.02 | 0.881 | 0.984 | |
SVM | test_21 | 96.08 | 99.81 | 97.92 | 0.959 | 0.979 |
test_24 | 43.57 | 99.09 | 71.33 | 0.513 | 0.713 | |
KNN | test_21 | 97.72 | 12.57 | 55.78 | 0.197 | 0.551 |
test_24 | 83.97 | 73.81 | 78.89 | 0.581 | 0.789 | |
NB | test_21 | 88.89 | 99.81 | 94.27 | 0.891 | 0.944 |
test_24 | 1.58 | 98.65 | 50.11 | 0.009 | 0.501 | |
XGBoost | test_21 | 97.63 | 98.87 | 98.24 | 0.965 | 0.983 |
test_24 | 73.14 | 96.36 | 84.65 | 0.712 | 0.847 | |
LR | test_21 | 95.26 | 94.28 | 94.78 | 0.896 | 0.948 |
test_24 | 11.29 | 92.10 | 51.69 | 0.058 | 0.517 | |
RF | test_21 | 95.81 | 1.0 | 97.87 | 0.958 | 0.979 |
test_24 | 4.51 | 1.0 | 52.26 | 0.152 | 0.523 |
Model | Sn(%) | Sp(%) | Acc(%) | MCC | AUC |
DIGITAL | 98.86 | 97.31 | 98.06 | 0.961 | 0.998 |
Only bi-LSTM | 91.57 | 99.34 | 95.37 | 0.910 | 0.994 |
Only multi-scale ResNet | 93.86 | 98.88 | 96.35 | 0.928 | 0.969 |
Iter | Target | Bi-LSTM | Dense | Batch_size |
1 | 0.9815 | 43 | 75 | 23 |
2 | 0.9815 | 28 | 105 | 61 |
3 | 0.9797 | 45 | 73 | 41 |
4 | 0.9852 | 58 | 125 | 64 |
5 | 0.9838 | 127 | 67 | 26 |
6 | 0.9797 | 74 | 128 | 60 |
7 | 0.9871 | 121 | 16 | 110 |
8 | 0.9834 | 113 | 73 | 123 |
9 | 0.9866 | 98 | 42 | 61 |
10 | 0.9838 | 97 | 79 | 106 |
11 | 0.9783 | 38 | 66 | 98 |
12 | 0.9810 | 30 | 107 | 116 |
13 | 0.9806 | 126 | 86 | 18 |
14 | 0.9834 | 42 | 19 | 81 |
15 | 0.9806 | 70 | 41 | 55 |
16 | 0.9834 | 99 | 111 | 35 |
17 | 0.9801 | 98 | 41 | 60 |
18 | 0.9838 | 106 | 99 | 89 |
19 | 0.9815 | 56 | 97 | 39 |
20 | 0.9866 | 112 | 104 | 95 |
21 | 0.9861 | 76 | 119 | 52 |
22 | 0.9847 | 81 | 89 | 112 |
23 | 0.9797 | 110 | 24 | 61 |
24 | 0.9820 | 44 | 89 | 106 |
25 | 0.9810 | 69 | 65 | 85 |
26 | 0.9857 | 82 | 19 | 109 |
27 | 0.9838 | 79 | 87 | 73 |
28 | 0.9834 | 61 | 43 | 38 |
29 | 0.9783 | 97 | 80 | 106 |
30 | 0.9857 | 98 | 21 | 59 |
Sn(%) | Sp(%) | Acc(%) | MCC | AUC | |
5-fold | 98.44 | 97.83 | 98.14 | 0.963 | 0.997 |
10-fold | 98.61 | 97.99 | 98.30 | 96.61 | 99.78 |