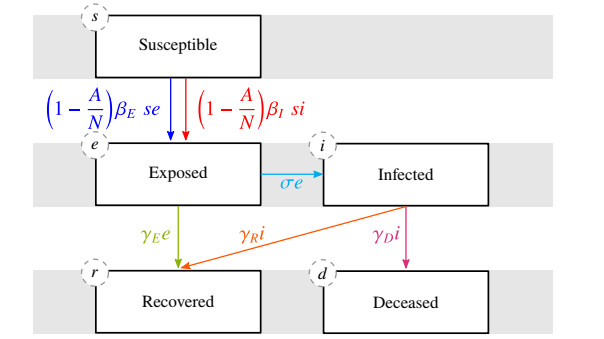
Estimating the volume of food plays an important role in diet monitoring. However, it is difficult to perform this estimation automatically and accurately. A new method based on the multi-layer superpixel technique is proposed in this paper to avoid tedious human-computer interaction and improve estimation accuracy. Our method includes the following steps: 1) obtain a pair of food images along with the depth information using a stereo camera; 2) reconstruct the plate plane from the disparity map; 3) warp the input image and the disparity map to form a new direction of view parallel to the plate plane; 4) cut the warped image into a series of slices according to the depth information and estimate the occluded part of the food; and 5) rescale superpixels for each slice and estimate the food volume by accumulating all available slices in the segmented food region. Through a combination of image data and disparity map, the influences of noise and visual error in existing interactive food volume estimation methods are reduced, and the estimation accuracy is improved. Our experiments show that our method is effective, accurate and convenient, providing a new tool for promoting a balanced diet and maintaining health.
Citation: Xin Zheng, Chenhan Liu, Yifei Gong, Qian Yin, Wenyan Jia, Mingui Sun. Food volume estimation by multi-layer superpixel[J]. Mathematical Biosciences and Engineering, 2023, 20(4): 6294-6311. doi: 10.3934/mbe.2023271
[1] | Ahmed Alshehri, Saif Ullah . A numerical study of COVID-19 epidemic model with vaccination and diffusion. Mathematical Biosciences and Engineering, 2023, 20(3): 4643-4672. doi: 10.3934/mbe.2023215 |
[2] | Ziqiang Cheng, Jin Wang . Modeling epidemic flow with fluid dynamics. Mathematical Biosciences and Engineering, 2022, 19(8): 8334-8360. doi: 10.3934/mbe.2022388 |
[3] | S. H. Sathish Indika, Norou Diawara, Hueiwang Anna Jeng, Bridget D. Giles, Dilini S. K. Gamage . Modeling the spread of COVID-19 in spatio-temporal context. Mathematical Biosciences and Engineering, 2023, 20(6): 10552-10569. doi: 10.3934/mbe.2023466 |
[4] | Roman Zúñiga Macías, Humberto Gutiérrez-Pulido, Edgar Alejandro Guerrero Arroyo, Abel Palafox González . Geographical network model for COVID-19 spread among dynamic epidemic regions. Mathematical Biosciences and Engineering, 2022, 19(4): 4237-4259. doi: 10.3934/mbe.2022196 |
[5] | Michael James Horry, Subrata Chakraborty, Biswajeet Pradhan, Maryam Fallahpoor, Hossein Chegeni, Manoranjan Paul . Factors determining generalization in deep learning models for scoring COVID-CT images. Mathematical Biosciences and Engineering, 2021, 18(6): 9264-9293. doi: 10.3934/mbe.2021456 |
[6] | Javad Hassannataj Joloudari, Faezeh Azizi, Issa Nodehi, Mohammad Ali Nematollahi, Fateme Kamrannejhad, Edris Hassannatajjeloudari, Roohallah Alizadehsani, Sheikh Mohammed Shariful Islam . Developing a Deep Neural Network model for COVID-19 diagnosis based on CT scan images. Mathematical Biosciences and Engineering, 2023, 20(9): 16236-16258. doi: 10.3934/mbe.2023725 |
[7] | Sarafa A. Iyaniwura, Musa Rabiu, Jummy F. David, Jude D. Kong . Assessing the impact of adherence to Non-pharmaceutical interventions and indirect transmission on the dynamics of COVID-19: a mathematical modelling study. Mathematical Biosciences and Engineering, 2021, 18(6): 8905-8932. doi: 10.3934/mbe.2021439 |
[8] | Yukun Tan, Durward Cator III, Martial Ndeffo-Mbah, Ulisses Braga-Neto . A stochastic metapopulation state-space approach to modeling and estimating COVID-19 spread. Mathematical Biosciences and Engineering, 2021, 18(6): 7685-7710. doi: 10.3934/mbe.2021381 |
[9] | Sarita Bugalia, Vijay Pal Bajiya, Jai Prakash Tripathi, Ming-Tao Li, Gui-Quan Sun . Mathematical modeling of COVID-19 transmission: the roles of intervention strategies and lockdown. Mathematical Biosciences and Engineering, 2020, 17(5): 5961-5986. doi: 10.3934/mbe.2020318 |
[10] | Xiangtao Chen, Yuting Bai, Peng Wang, Jiawei Luo . Data augmentation based semi-supervised method to improve COVID-19 CT classification. Mathematical Biosciences and Engineering, 2023, 20(4): 6838-6852. doi: 10.3934/mbe.2023294 |
Estimating the volume of food plays an important role in diet monitoring. However, it is difficult to perform this estimation automatically and accurately. A new method based on the multi-layer superpixel technique is proposed in this paper to avoid tedious human-computer interaction and improve estimation accuracy. Our method includes the following steps: 1) obtain a pair of food images along with the depth information using a stereo camera; 2) reconstruct the plate plane from the disparity map; 3) warp the input image and the disparity map to form a new direction of view parallel to the plate plane; 4) cut the warped image into a series of slices according to the depth information and estimate the occluded part of the food; and 5) rescale superpixels for each slice and estimate the food volume by accumulating all available slices in the segmented food region. Through a combination of image data and disparity map, the influences of noise and visual error in existing interactive food volume estimation methods are reduced, and the estimation accuracy is improved. Our experiments show that our method is effective, accurate and convenient, providing a new tool for promoting a balanced diet and maintaining health.
After the emergence of the coronavirus disease 2019 (COVID-19), many policy decisions directly affecting personal gatherings, business operations and healthcare utilization have been predicated on forecasted case counts and hospitalization statistics. Many such predictions use compartmental models based on ordinary differential equations (ODEs), which capture temporal variation for a population. Compartmental models derived from the susceptible-infected-removed (SIR) model have been used extensively. These models often include additional compartments and stratifications based on age, comorbidity, sex etc., to account for complex disease dynamics [1,2]. These approaches are based on an aggregated population for a given geographical domain and are well suited for individual population centers and cities or for studying global trends in broader regions. Agent- and network-based models [3,4] are also popular particularly for studying localized virus spread by employing microscale data concerning disease transmission and population structure, which are difficult to acquire for large geographical domains. However, when the geographical domain of interest is a province/state/region or a country, spatial variations in disease dynamics become critical for accurate predictions [5,6]. Critically compartmental models based on partial differential equations (PDEs) [7,8,9,10] capture the continuous spread of a virus both in space and time, providing a more complete description of disease dynamics over a large geographical domain. Their outputs indicate highly contagious zones and the evolution of disease dynamics among other clinically relevant information which can inform the decision makers about preventative measures and hospital preparedness.
The numerical solution of the problem involves spatial and temporal discretizations that lead to a nonlinear system of equations, typically with millions of unknowns. A parallel iterative solver with an appropriate preconditioner can be used to achieve faster solutions for the linearized system derived from the nonlinear system. Domain decomposition (DD) methods refer to approaches for solving linear (or nonlinear) systems arising from PDEs that rely on dividing the domain into smaller sub problems and concurrently iterating to find a converged solution. They are generally used as preconditioners to Krylov subspace based solvers because of the inherent parallelism and ability to adapt to complex problems providing faster convergence. The development of DD has parallelly evolved into two branches of overlapping and non-overlapping decompositions [11,12,13,14,15]. We utilize an overlapping Schwarz DD framework to develop efficient preconditioners for the nonlinear system. Newton-Krylov methods linearize the system by using Newton's iteration and then employ a preconditioned Krylov solver for the linear system obtained at each step [16,17]. Other nonlinear preconditioning techniques available in the literature [18,19,20] solve nonlinear problems in subdomains with modified preconditioners. These are mainly suitable when localized nonlinearity cannot be effectively handled by global linearization. In this paper we use Picard iterations for the nonlinear system followed by a DD-based iterative solver at each step for simplicity. We find our linearization and preconditioning strategy satisfactory for the current five-compartment model problem to demonstrate the importance and suitability of DD methods to compartmental models of COVID-19.
Now, we note below the compelling reasons for applying a DD-based solver for COVID modelling.
● Like their ODE-based counterparts, PDE-based compartmental models can be very fine grained, accounting for accurate dynamics among populations (see C). This can be further extended to consider different age groups, socio-economic status, vaccination status, and co-morbidity (e.g., [1]). We note that corresponding spatial and temporal data for these stratifications could be obtained from private healthcare databases (e.g., [21]). These highly complex coupled models can be solved efficiently by using an iterative solver equipped with parallel preconditioners offered by DD methods.
● The application of the above mentioned five-compartment model with finer spatial and temporal discretizations covering large geographical areas (including many public health units) involves system sizes above millions as well as the associated computational cost.
● The inclusion of uncertainty in the model (as in [3,22]) resulting from considering parameters as random variables or random fields leads to stochastic PDEs which can be solved by using sampling (Monte Carlo or quadrature) methods or sampling-free stochastic Galerkin methods [23]. Slow convergence of Monte Carlo or large stochastic dimensions can increase the number of deterministic sample evaluations which in turn increases the computational cost for sampling approaches. Even though DD-based solvers can be utilized for each deterministic evaluation, parallel overhead may reduce its efficiency. Sampling-free stochastic Galerkin methods demand solutions of a large linear/nonlinear system of equations for the stochastic PDEs. Depending on the stochastic discretization (number of input random variables/order of output expansion), the size of this linear system may grow exponentially which requires scalable parallel solvers built on DD-based methods (e.g., [24]).
● In the Bayesian inference framework, inverse problems for the estimation of model parameters requires the forward model to be evaluated numerous times for the computation of the likelihood function [7]. For a high resolution model with many compartments in which a single forward evaluation takes hours, this computation could take months to complete. With the highly scalable and efficient solver developed here, this computational cost can be reduced to days or even hours.
● For a time-dependent nonlinear system of PDEs such as a compartmental model of COVID-19, different solution strategies can be adopted, such as: (i) discretize in time, linearize and adopt a linear preconditioner for the iterative solver for the non-symmetric system, (ii) discretize in time and apply a nonlinear preconditioner [18,19,20] and (iii) apply a parallel in time method [25]. The first strategy is adopted in this paper where in an efficient preconditioner for the GMRES iterative solver is described. In contrast to the conjugate gradient method used for symmetric and positive definite systems, the convergence criteria of the GMRES algorithm is difficult to establish by using the spectral information on the coefficient matrix. It is thus important to identify a problem-specific preconditioner that expedites the convergence and improves scalabilities of the iterative solver.
To this end, the main contributions of the paper are as follows.
● Development of a parallel scalable solver for the solution of complex compartmental models of COVID-19 for large geographical areas.
● Scalability studies of the one-level restricted additive Schwarz (RAS) and two-grid Schwarz preconditioner variants in terms of the iteration count and solution time.
● Development of an efficient solver through the use of a two-grid RAS preconditioner for the nonlinear coupled PDEs. The adapted solver with an algebraic multigrid preconditioning for the coarse problem reduces the execution time and improves scalabilities. The comprehensive numerical experiments demonstrate the superior performance of this two-grid RAS solver against the other variant of the two-grid RAS preconditioner. While the architecture of the preconditioner is inspired by [26,27,28,29,30], the specific choice of the DD preconditioner with the algebraic multigrid for the coarse solver is novel, which improves scalabilities with respect to existing two-grid Schwarz preconditioners for large-scale systems. The multilevel Schwarz method has been proposed in the literature [31,32,33]; it uses the hierarchy of coarse spaces with additive or multiplicative corrections in order to construct the preconditioners at each level. The proposed methodology uses just a two-grid Schwarz method in which the coarse solver leverages the algebraic multigrid as the preconditioner, thereby permitting multilevel error reduction. While the existing literature focuses on the elliptic PDEs leading to the symmetric and positive-definite system matrices, we tackle nonlinear PDEs which translate to solving linearized systems with unsymmetric system matrices.
● Numerical illustration of a susceptible-exposed-infected-recovered-deceased (SEIRD) model with spatio-temporally varying infection rate parameters that capture the realistic trends of infection through the region.
● Application of the two-grid RAS-based solver to a large geographical domain of Southern Ontario with over 92 million unknowns demonstrating the efficiency of the solver in a realistic setting.
● Verification studies for one and two-dimensional compartmental models of COVID-19 through the use of the method of manufactured solutions (MMS).
We note that this article presents a sub-component needed for the accurate and precise predictive COVID-19 models through the development of a scalable solver for these models. These models may contain large numbers of compartments and associated stratifications (as described in Appendix C: 22-compartment model) which increases the problem size posing challenges for sequential solvers. This study illustrates the computational benefits of the proposed solver for such comprehensive compartmental models (i.e., drastically reducing time-to-solution in a manner that scales well). This computational efficiency is critical for future work, which will leverage data (from Ontario or elsewhere) and more sophisticated calibration methods (Bayesian inference) to solve the inverse problem of estimating the model parameters in a more systematic manner. Without the increase in speed afforded by these methods, it would be prohibitively expensive to perform such calibration exercises for a system of this size (or other geographic regions with similarly fine spatial mesh resolution).
The paper is organized as follows. Section 2 introduces the PDE-based compartmental model and formulation of weak form to which the solver is applied. Sections 3 and 4 briefly cover the basics of the overlapping Schwarz method, coarse corrections and a new two-grid preconditioner. Section 5 applies the different preconditioners and assesses their relative effectiveness. The selected approach is then applied to a realistic model of Southern Ontario. The weak form of the equations are given in Appendix A, the model validation is shown in Appendix B and details on a more complex 22-compartment PDE-based model are provided in Appendix C.
A compartmental model consisting of SEIRD states is considered. The susceptible compartment is the population density of individuals who are vulnerable to infection, but have not yet been exposed. This state acts as the feeding state for infection to spread. The current model does not consider the possibility of reinfection after recovery (as we will only consider a single wave of infection); thus the susceptible compartment decreases monotonically until a steady state is reached. The exposed and infected compartments consist of people who are the carriers of the virus and who may infect others. We distinguish between these two compartments by defining exposed as cases that are asymptomatic or cases that end in recovery without being detected, whereas infected accounts for cases that are positively identified. The spread of infected people is thus considered to be lower due to quarantine/isolation measures after detection. The deceased compartment accounts for people who die of acute COVID-19, and the recovered compartment accounts for all other possible outcomes associated with COVID-19 infection where the individual survives.
The movement of a population is generally based on assumptions about the behavior of the host and their interaction with the surrounding environment [34]. A randomly mixing population on the microscale can be modeled as a diffusion process on the macroscale (see [35], Section 11.1). The current model assumes a heterogeneous population-dependent diffusion term for the movement of population in space. Along with diffusion in space, at each time step, the population changes states according to the interaction between the respective compartments. All model states are functions of space x and time t. However, we generally omit these in our notations for brevity. The spatio-temporal evolution of the densities of the susceptible s(x,t), exposed e(x,t), infected i(x,t), recovered r(x,t), and deceased d(x,t) compartments are described by the following coupled nonlinear PDEs [7,9,10].
∂ts=∇⋅(NˉνS∇s)+αN−(1−AN)βIsi−(1−AN)βEse−μs | (2.1) |
∂te=∇⋅(NˉνE∇e)+(1−AN)βIsi+(1−AN)βEse−σe−γEe−μe | (2.2) |
∂ti=∇⋅(NˉνI∇i)+σe−γRi−γDi−μi | (2.3) |
∂tr=∇⋅(NˉνR∇r)+γEe+γRi−μr | (2.4) |
∂td=γDi, | (2.5) |
where N is the total population density defined as
N(x,t)=s(x,t)+e(x,t)+i(x,t)+r(x,t)+d(x,t), | (2.6) |
and ˉνS,ˉνE,ˉνI and ˉνR are the scalar diffusion coefficients dictating the spread of infection in space. The terms containing α and μ are the population vital dynamics respectively, representing the birth rate and death rates (excluding deaths caused by COVID-19). Given the time scale, both are considered zero for the present study. The remaining model parameters are described below in relation to their appearance in Figure 1, which provides a visual representation of the dynamics described by Eqs (2.1)–(2.5). It can be noticed that these equations are analogous to a diffusion-reaction model [36,37]. The diffusion coefficients ˉνS,ˉνE,ˉνI and ˉνR control the spatial distribution of infection.
Note that the five-compartment model [7,9,10] in Figure 1 is a simplified version of the 22-compartment model shown in C. As an initial investigation, this five-compartment model is used to demonstrate the usefullness of the DD-based solvers.
In the equations above, (i) susceptible individuals flow from s to e after being exposed to the disease by coming into contact with individuals in either the e or i compartments according to the rate parameters βE, βI; (ii) individuals in the exposed compartment either move to the infected compartment i if they are detected according to the rate parameter σ, or if they go undetected, they remain in the e compartment for the duration of their infectious period before proceeding to the recovered compartment at the rate γE; (iii) individuals in the i compartment will proceed to either the recovered r or deceased compartment d according to the rate parameters γR or γD. The susceptible and exposed compartments contain a term (1−A/N), called the Allee effect. For a given value of A, this term enforces higher transmission rates in locations of higher population density and conversely lower transmission rates for regions with lower population density.
In general, precise values of these parameters are not known but can be obtained by calibrating the model using data and prior clinical knowledge. When the domain is isolated (as is the case here), the total population over the whole domain remains constant in time, but the density may vary through space and time. The nonlinear coupled PDEs are discretized by using the finite element method, converting them to a system of linearized algebraic equations as explained in the next section.
The system of Eqs (2.1)–(2.5) can be written concisely as follows [10],
∂tu−∇⋅(ν(u)∇u)=B(u)u, | (2.7) |
where,
u=[seird],ν(u)=N(x,t)[ˉνS00000ˉνE00000ˉνI00000ˉνR000000], | (2.8) |
B(u)=[α−μ−(1−AN)βEe−(1−AN)βIiαααα(1−AN)βIi(1−AN)βEs−μ−σ−γE0000σ−γR−γD−μ000γEγR−μ000γD00]. | (2.9) |
Previous studies involving compartmental models used the backward Euler/implicit method to model the spread of COVID-19 due to their superior stability properties [7,10,38]. Implicit-explicit (IMEX) time integration schemes have been adopted previously for compartmental models [8], treating the nonlinear diffusion term implicitly and remaining nonlinear terms explicitly. IMEX schemes for reaction-diffusion-type problems are prone to erroneous results and can increase the number of iterations for iterative solvers if not chosen carefully [39,40]. We note that a finely stratified model with 22 compartments (or more) [1] (as in Appendix C) will involve complex dynamics with a range of time scales. Such systems will contain numerous nonlinear terms (often leading to stiff systems) which can accentuate the errors stemming from explicit treatment of these nonlinear terms [38], consequently affecting the stability of the IMEX scheme. A discrete time version of this PDE system for the state vector u can be written by using a backward Euler/implicit method:
un+1−Δt(∇⋅(ν(un+1)∇un+1))=un+Δt(B(un+1)un+1), | (2.10) |
where un+1 is the solution at time step n+1, as computed from the solution un(x)≈u(x,tn) for the previous time step. When n=0, u0(x) is the initial condition to the problem, the weak form of Eq (2.10) becomes:
(un+1,v)+Δt(ν(un+1)∇un+1,∇v)−Δt(B(un+1)un+1,v)−Δt∫ΓNν(un+1)∇un+1⋅ˆndΓ=(un,v), | (2.11) |
where (u,v)=∫Ωuvdx, Ω is the spatial domain, ΓN denotes the boundary where the Neumann boundary condition is specified and ˆn denotes the unit normal vector to the boundary. A homogeneous Neumann boundary for the domain enforces a no-flux condition which prevents the migration of a population across the boundary of the domain. This means complete isolation of the region, which is representative of restrictions on international/interprovincial travel.
This nonlinear system must be solved by using either Picard iterations or Newton's method inside the time discretizations. For simplicity, we chose to apply Picard iteration directly to Eq (2.11). Consider the Picard iteration at the time step n+1 with the current iteration number k+1 as follows (note that the boundary integral in Eq (2.11) vanishes due to homogenous conditions):
(un+1,k+1,v)+Δt(ν(un+1,k)⏟∇un+1,k+1,∇v)−Δt(B(un+1,k)⏟un+1,k+1,v)=(un,v). | (2.12) |
The initial guess for each iteration at un+1,k=0 is chosen as the solution at the previous time step un. In the Picard iteration, the nonlinear coefficient terms are treated explicitly by using the solution from the previous iteration, resulting in a linear approximation at each iteration. Note that the underbraced coefficient terms in Eq (2.12) depend only on the previous iteration k. Thus the solution to the nonlinear coupled PDE system is calculated at each Picard iteration inside each time step through the use of Eq (2.12).
For the current model, a fully coupled approach involves solving the system of five PDEs in Eq (2.12) together as a vector of compartments. However, this approach does not significantly improve the efficiency of the solver due to the weak coupling observed among the compartments after applying the Picard iteration. Note that the introduction of additional compartments, model stratifications, the inclusion of uncertainty etc., can affect the system properties. In such cases, it may be necessary to adopt a fully coupled solution approach and use Newton's method. The detailed equations involving the formulation for each compartment is shown in Appendix A. We use triangular elements with linear interppolation functions for discretization. The linear system assembled from the weak form can be solved by using Krylov subspace solvers with appropriate preconditioners inside each Picard iteration. The Picard iteration is assumed to be converged when the error, ϵ reaches a specified tolerance defined as:
ϵ=∥un+1,k+1h−un+1,kh∥2∥unh∥2, | (2.13) |
where uh represents the discretized solution vector. The next section introduces DD concepts and the associated preconditioners to be applied to the above model.
The DD method is a divide and conquer algorithm that provides fast solutions to computational models involving PDEs [11]. Independent treatment of each part of a complex domain provides a naturally parallel formulation for multiphysics and heterogeneous domains. Generally, these methods are applied as preconditioners to Krylov solvers at the discrete level.
The two main branches of DD methods, namely overlapping and non-overlapping, are fundamentally distinguished based on whether or not the adjacent subdomains overlap one another. Consider the domain Ω, illustrated in Figure 2 as the union of two overlapping subdomains where Ω=Ω1∪Ω2 with the boundary ∂Ω. The subdomain boundaries are ∂Ω1 and ∂Ω2 and the artificial boundary created inside each subdomains are Γ1 and Γ2. The overlapping DD method considers subdomains on which the solution is sought independently by using artificial boundary values from an adjacent subdomain. The overlapped regions are then updated by combining the solutions from each subdomain and the process is repeated to reach convergence of the solution. The method can be sequential or parallel. We present here briefly the iterative version of an overlapping method for a decomposition into two subdomains.
Consider the Poisson problem of finding the solution u over the domain Ω with homogeneous boundaries. This problem can be split into independent sub-problems in each subdomain as below [12,13,14,15]:
−Δun+1i=finΩiun+1i=0on∂Ωi∩∂Ωun+1i=un3−ionΓ3−i. | (3.1) |
In the parallel case, finding the solution at the iteration n+1 on the artificial boundary of the ith subdomain ui=1,2 involves the previous solution iteration from the adjacent subdomains which allows the solutions to develop independently. A sequential version on the other hand alternately solves the subdomains i=1,2 by using the updated solution at the current step. The implementation of overlapping methods is more straightforward than that for their non-overlapping counterparts, wherein it is necessary to solve the combined interface problem before tackling the interior nodes of each subdomain. Also, the definition of interfacing points can be quite involved especially in higher dimensions where cross points can appear. Overlapping methods only require consecutive solutions of the original problem in smaller subdomains and the exchange of artificial boundary solutions to neighbouring subdomains. While Schwarz methods can be used as solvers or as preconditioners for the accelerated convergence of Krylov solvers, they are seldom used as solvers due to their slow convergence compared to Krylov solvers. Preconditioners are operators applied to coefficient matrices transforming them to have favourable properties of convergence for iterative solvers [41,42]. This transformation, in general alters the spectral properties and conditions the coefficient matrix. We briefly discuss two main types of preconditioners used in the literature in a discrete framework [12].
The additive Schwarz method (ASM) relies on local solutions in subdomains which are then assembled globally as follows [12]:
M−1ASM=N∑i=1RTi(RiARTi)−1Ri, | (3.2) |
where A represents the linearized coefficient matrix assembled inside each Picard iteration for each time step, Ri represents the restriction operator transferring the solution vector on global mesh to the local subdomain level and RTi is the extension matrix reversing the operation. The ASM exchanges information between subdomains without taking into account the redundancies in the overlap. For this reason, this can only be used as a preconditioner, since its iterative counterpart does not converge to the true solution. It can be noted that the preconditioner formed is symmetric. The RAS preconditioner is defined as follows [12]:
M−1RAS=N∑i=1RTiDi(RiARTi)−1Ri, | (3.3) |
where Di denotes the Boolean square matrices called partition of unity matrices such that Id=∑Ni=1RTiDiRi. The partition of unity matrices scale the residuals so that consistent contributions from each subdomain are only added. These preconditioners are not assembled explicitly, as a series of steps replicating their action are applied, i.e., matrix-vector computations and local linear solves. The global residual computed is distributed to the local subdomain and a local Dirichlet problem is solved. This solution is combined by using the partition of unity matrices for all subdomains. Both RAS and ASM solve for the increment/correction to the solution for all subdomains before combining them appropriately. ASM and RAS differ only in the way these corrections are combined. We use the RAS-based preconditioners since it provides faster convergence than its counterpart.
One-level methods only communicate and exchange information with adjacent subdomains. This strategy is effective in reducing the high frequency component of error. When the number of subdomains is large, one-level methods converge slowly due to significant low-frequency components of error. The efficient global exchange of information among subdomains can be used to reduce the low-frequency component of error which enhances scalability. This is achieved by using two-grid methods with coarse corrections [12].
A coarse space correction is constructed to remove the low-frequency component of the error due to small eigenvalues in the one-level preconditioned matrix. Intuitively, these components represent constant functions for a Poisson problem or rigid body modes for elasticity [12]. For a complex problem, where simple representation of the coarse space components is not available, a simple solution is to use a coarser triangulation from which the fine grid is constructed by refinement leading to a grid-based coarse space. In what follows, we will explain how the coarse correction matrix is built for a grid-based coarse space.
Figure 3 shows a square domain with overlapping subdomains and an underlying finite element mesh. We construct a rectangular matrix Z of size n×nc which is an interpolation operator from a coarse to fine grid, where n is the total number of degrees of freedom and nc is the number of coarse degrees of freedom. A coarse matrix Ac=R0ART0 is constructed using the Galerkin projection where R0=ZT or by directly assembling the given PDE in the coarse grid. An additive coarse correction constructed from this coarse grid is applied to the one-level preconditioner in Eq (3.3) as follows [12]:
M−12=M−1RAS+Q, | (3.4) |
where Q=RT0A−1cR0 is the global coarse correction. Other variants such as deflated and hybrid forms of corrections can also be applied to obtain favourable properties [30]. Multiplicative corrections update the residual in between various levels providing a better convergence rate than additive corrections [14].
This section describes the two-grid Schwarz preconditioners constructed by combining the above-described one-level methods and coarse space corrections. We utilize the multilevel parallel implementational architechture from PETSc [43] (more implementational details in 4.1) to construct a two-grid preconditioner with multiplicative corrections. A three-step correction is applied with a one-level RAS preconditioner as a pre-smoother, post-smoother and coarse grid correction in between them. A combination of these three corrections applied at different levels (fine-coarse-fine) can be used to construct the two-grid preconditioner as below.
Consider three preconditioners P1,P2,P3 applied to the system as a pre-smoother, coarse correction, and post-smoother respectively as follows [30],
ui+1/3=ui+P1(f−Aui) | (4.1) |
ui+2/3=ui+1/3+P2(f−Aui+1/3) | (4.2) |
ui+1=ui+2/3+P3(f−Aui+2/3). | (4.3) |
Substituting Eq (4.1) into Eq (4.2) and further Eq (4.2) into Eq (4.3) gives us,
ui+2/3=ui+(P1+P2−P2AP1)⏟P4(f−Aui) | (4.4) |
ui+1=ui+(P4+P3−P3AP4)⏟P5(f−Aui), | (4.5) |
where P5 is our desired preconditioner expanded as,
P5=P1+P2+P3−P2AP1−P3AP1−P3AP2+P3AP3AP1. | (4.6) |
Using P1=P3=M−1RAS and P2=Q, the final two-grid preconditioner can be written as,
M−12=M−1RASP+QPQ−1M−1RAS+Q−M−1RASPAM−1RAS, | (4.7) |
where P=(I−AQ) is the projection matrix. The residual is recalculated at each level by using an updated solution which results in multiplicative corrections. The restriction and interpolation operators are R0 and RT0 respectively as defined earlier.
For the two-grid preconditioner above, it is evident that the coarse problem has the same structure as the original problem; hence it can be constructed just by using an interpolation operator from the original system matrix. It also couples all of the subdomains enabling global error propagation. However, for very large meshes, the system size grows rendering the solution of the coarse problem computationally expensive using a direct solver. In these cases, it is useful to adopt a preconditioner to iteratively solve the coarse problem A−1c in the coarse correction Q effectively to reduce the execution time and memory requirement. To this end, we adopt two choices: (i) a one-level RAS preconditioner as in Eq (3.3); (ii) an algebraic multigrid (AMG) V-cycle preconditioner [44]. Through numerical experiments, we demonstrate that the AMG preconditioner is more effective than the one-level RAS preconditioner. Next, we briefly explain the multigrid method relevant to the problem.
Multigrid methods offer hierarchy of grids (geometric or algebraic) through which errors are iteratively reduced [44,45,46,47]. The error in the iterative solution is decomposed into geometrically oscillatory and non-oscillatory parts. By utilizing a simple and cheap solver/smoother, highly oscillatory components of the errors are removed through a smoothing/relaxation step. The remaining non-oscillatory errors in the fine grid are corrected by using a coarse grid. The advantage lies in the fact that geometrically smooth errors in the fine grid become oscillatory in the coarse grid, and are then removed easily. After solving for the error in the coarse grid they are interpolated back to the fine grid to correct the solution [45]. Following the same steps repeatedly in cycles on these grids reduces the errors to required precision. Algebraic multigrids work on the same principle but do not depend on geometric information on the grid for error reduction [44]. This is useful for complex domains with unstructured meshes where the construction of the coarse grid is difficult. In an AMG, the coarse level is constructed by analyzing each entry in the coefficient matrix and selecting a specific subset of elements with the greatest contribution to the solution compared to their neighbours [48,49]. Using such a multi-level preconditioner for coarse grid solvers improves the scalability of the two-grid RAS solver of the original system.
The linearized system at each Picard iteration is solved by using the GMRES iterative solver and associated preconditioners. Next we point out the different variants of the one-level and two-grid preconditioners and their notations used in this work.
● One-level RAS: one-level RAS preconditioner as in Eq (3.3).
● Two-grid RAS: two-grid RAS preconditioner with a coarse problem solved by the direct solver (LU factorization).
● Two-grid RAS - V2: two-grid RAS preconditioner with a coarse problem solved by using a GMRES iterative solver equipped with a RAS preconditioner.
● Two-grid RAS - V3: two-grid RAS preconditioner with a coarse problem solved by using a GMRES with an AMG preconditioner.
Note that the application of a two-grid preconditioner for the coarse solver in the two-grid preconditioners is avoided due to the complexity of constructing coarser levels and associated interpolation operators among them. The time taken for the construction of preconditioners in this case may not be able to offset the reduction in solution time achieved. In contrast, the two-grid RAS - V3 efficiently handles this by avoiding the use of grids in the construction of the coarse level preconditioner as is made evident by numerical experiments later.
All numerical experiments were carried out in FreeFEM [50] integrated with PETSc [43]. The codes are downloadable from https://bitbucket.org/sudhipv/mbe_covid. The correspondence among the code and relevant equations is detailed on the README.md file in the repository. The two-grid variants of preconditioners differ only in the coarse solver. The preconditioner architecture is implemented by using a single V-cycle multigrid framework from PETSc. The two-grid RAS-V3 makes use of HYPRE [51] which is available through PETSc which runs BoomerAMG [52] as preconditioner for the coarse solver. This multilevel coarse solver adopts a V-cycle with Jacobi smoother and Gaussian elimination for coarse correction [43,44]. This was specifically chosen to address the convergence bottleneck for high-resolution models. A minimum overlap is used for RAS algorithms in all cases as depicted in Figure 3. All preconditioners are used within the GMRES solver with the right preconditioning since it calculates the true residual norm in contrast to the preconditioned residual norm as in the left preconditioned systems. This permits a direct comparison of residuals among different methods [41,43]. The stopping criteria for the Krylov solver (GMRES) follow PETSc implementations [43] which are based on (a) the absolute residual norm, atol (set as 10−50), (b) the decrement of the residual l2 norm relative to the l2 norm of the right-hand side, rtol (set as 10−5), and (c) the relative increment in residual, dtol (set as 105). The convergence and divergence in any iteration j is established respectively as follows:
∥rj∥2<max(rtol×∥b∥2,atol) | (4.8) |
∥rj∥2>max(dtol×∥b∥2) | (4.9) |
where rj denotes the residual at the jth iteration of the GMRES solver and the right-hand vector b. Apart from this, the maximum number of outer and inner (coarse) Krylov iterations for two-grid solvers were restricted to 200 and 100 respectively. In cases where an inner GMRES solver is used, the outer solver is modified to use the Flexible GMRES (FGMRES) algorithm [53] which permits changes in the preconditioning at every step. A detailed comparison of preconditioned Krylov methods for nonsymmetric systems relevant to this study can be found in [54].
In high performance computing (HPC) terminology, a process (or task) refers to an instance of the data parallel program that is delegated to a specific core. In our implementation, the computational domain is divided into several overlapping subdomains by using DD. The DD algorithm lends itself to a data parallel program which utilizes Message Passing Interface (MPI) libraries to exploit distributed memory machines (linux clusters) maintained by Digital Research Alliance of Canada [55] such as Beluga [56] or Niagara [57]. In these machines, each computational node consists of 40 Intel Skylake cores running at 2.4 GHz connected through an EDR infiniband network. In our parallel implementation, the MPI initializes the same number of tasks/processes as the number of cores giving exactly one task per core called a pure distributed-memory program [58]. Although no hybrid parallelism is used in the current investigation, it can be implemented by delegating subdomain (local) solves to accelerators such as graphics processing units.
This section applies the preconditioners mentioned in the previous section to solve the linearized system in Eq (2.12) at each Picard iteration for all time steps. With five compartments, the total number of unknowns becomes five times the number of degrees of freedom for the finite element mesh. The coarse grid is nested inside the fine grid, with a splitting ratio of two for a fine-to-coarse grid. One-level and two-grid preconditioners are compared by using their average number of Picard and GMRES iterations and total time-to-solution for a square domain. The numerical and parallel scalabilities for each case were studied to select the most suitable preconditioner. This preconditioner has been applied to a large geographical domain of Southern Ontario to demonstrate the scalibility of the solver in a realistic setting. The parameter values used in each of the numerical experiments are shown in 1. We denote the units of a population as 'people', time in 'days' and length in 'km'. The initial ratio of exposed to infected population was assumed to be 1:1 (50 % detection was assumed). The time step for all experiments was fixed at 0.1 days based on converged solutions with fine spatial discretizations. We have explicitly demonstrated temporal and spatial convergence properties of the one- dimensional model in Section B.2. We used the order of accuracy criteria (see Eqs (B.1)–(B.6)) to test the convergence and the theoretical order of accuracy (for both spatial and temporal scales) was retrieved from the numerical models in Tables B2 and B3. Considering the two-dimensional square domain model, the spatial and temporal grid convergence studies were also conducted but not shown for brevity. Using the MMS, we have plotted the sum of relative error for all compartments (see Eq (B.7)) over time in Figure B2e. Under the condition of small diffusion, a comparison of time traces of compartments for PDE-based compartmental model against an ODE-based SEIRD model is plotted in Figure B3. For the domain of Southern Ontario, spatial and temporal grid convergence studies were also conducted but the results are not shown for brevity.
Parameter | Square domain (Section 5.1) | Southern Ontario (Section 5.2) | Units |
A | 500 | 8.9×10−3 | peoplekm2 |
βI,βE | 3.78×10−4 | Eq (5.3) | km2people×days |
νS,νE,νR | 3.94×10−6 | 4.5×10−7 | km4people×days |
νI | 10−8 | 10−9 | km4people×days |
γR | 1/24 | 1/11 | 1days |
γD | 1/160 | 1/750 | 1days |
σ | 1/7 | 1/5 | 1days |
γE | 1/6 | 1/15 | 1days |
For numerical investigation, a unit square domain with a uniform total population density of N=2000peoplekm2 was selected. A Gaussian function models the initial infected population at the center of domain as:
i(x,y)=0.1Nexp(−10[(x−0.5)2+(y−0.5)2]), | (5.1) |
where N represents the total population density. The densities of compartments e, r and d were initially set to zero. The susceptible density s is calculated by subtracting the known densities from total density. The domain is discretized in space by using triangular elements and backward Euler discretization is used for temporal discretization. The error tolerance for the Picard iteration was set to 10−8. The time traces of all compartments averaged over the entire domain and the contour plot for infected density at 10 days is shown in Figure 4.
Table 2 shows the time taken and iteration counts for various cases as obtained by using a fine mesh of size 4000×4000 solved using 600 processes. We report the time-to-solution for 10 time steps for comparison. In the decoupled approach, since all compartments are solved separately inside Picard iterations, the Krylov solver iteration counts are calculated as the sum of all five compartments. The Picard iteration counts and the Krylov solver iteration counts are reported as the averaged value over all time steps. The Picard iteration counts do not change from one level and two-grid versions of the RAS preconditioners. However, the number of Krylov iterations reduces drastically for two-grid methods. As the system size grows, the convergence rate decreases for one-level methods which can only be compensated with a coarse solver enabling global error propagation. Comparing two-grid methods, the time taken to solve 10 steps is lowest for RAS-V3, followed closely by RAS-V2. The direct factorization of the two-grid RAS consumes more time than its counterparts which increases the execution time. It is interesting to note the shorter time for one-level RAS than two-grid RAS which demonstrates the importance of optimizing the coarse solver. We also note that the reported parameters for two-grid solvers do not change in time significantly; and that the selected duration represents the average behaviour. The numerical studies conducted using time steps of 0.05 days, 0.1 days, and 0.2 days (not shown in manuscript) indicated that the Picard iteration counts decrease with smaller time steps but the iteration counts of the preconditioned GMRES solver remain unchanged [59]. This demonstrates that the preconditioner is insensitive to time steps in the current model.
Variants | Average Number of Krylov iterations | Average Picard iteration count | Time taken for 10 steps (s) |
One-level RAS | 3399 | 4 | 868 |
Two-grid RAS | 21 | 4 | 1308 |
Two-grid RAS - V2 | 26 | 4 | 530 |
Two-grid RAS - V3 | 21 | 4 | 434 |
After preliminary analysis, we selected the two-grid variants RAS-V2 and RAS-V3 to perform further scalability studies using the same square domain and the same model parameters. The strong parallel and strong numerical scalabilities are measured by the execution time and the Krylov solver iteration counts respectively to solve a fixed problem with an increasing number of subdomains. A higher level of parallelization decreases the execution time demonstrating strong parallel scalability. Constant iteration counts with increasing processes shows the numerical scalability. However, a stagnation point is reached when the interprocessor communication time overwhelms the floating point operation time and it is no longer suitable to increase the number of processes. For the fixed fine mesh, the number of processes/subdomains are increased from 80 to 600. We have plotted the preconditioner setup time and system solve time which are fully parallelizable. The simulation involves 10 time steps. Figure 5a shows the reduction in time for variants RAS-V2 and RAS-V3 with increasing processes. For a large number of subdomains/processes, both RAS-V2 and RAS-V3 have minimal difference in the time-to-solution. Even though iteration counts are constant with increasing processes for both RAS-V2 and RAS-V3, RAS-V2 takes higher iterations for convergence (see Figure 5b).
Weak parallel and weak numerical scalabilities are related to a nearly constant execution time and constant Krylov iteration counts respectively for a constant problem size per subdomain with increasing the number of processes. Hence the total problem size is increased along with number of processes. Figure 5c and 5d show the parallel and numerical scalabilities of both two-grid preconditioners. The system size is shown on the right y axis of Figure 5c. As the problem size increases, the execution time for RAS-V2 increases significantly, but the execution time for RAS-V3 remains nearly constant in Figure 5c. From the perspective of numerical scalability, note that outer iteration counts are the same for RAS-V3 and RAS-V2 for all processes, with the exception of a slight increase observed for RAS-V2 (see Figure 5d).
The degraded performance of the RAS-V2 can be attributed to the coarse solver. It is found that the coarse solver for the RAS-V2 preconditioner requires more than 100 iterations to reach a specified tolerance for some compartments (e.g., susceptible, exposed) while RAS-V3 converges in less than 10 iterations. This increased coarse solver iterations at each outer Krylov iteration drastically increases the execution time for RAS-V2. This performance degradation of RAS-V2 becomes severe with an increase in the size of coarse grid. On the other hand, RAS-V3 shows excellent scalability having constant iteration counts at fine and coarse levels. This is due to the multiple levels of error reduction in the coarse solver, as obtained by using the AMG preconditioner, leading to better convergence behaviour.
Next, we demonstrate the performance of the RAS-V3 preconditioner, including the speedup and the efficiency of the solver. The speedup is defined as the ratio of the sequential solve time to the parallel solve time and the efficiency is the ratio of speedup and the number of processes [13,14]. For the fixed problem size of 80 million, a minimum of 80 cores are needed to solve the system. Figure 6 shows the preconditioner setup and solve times associated with the two-grid RAS-V3 preconditioner with the same problem size as shown in Figure 5. Figure 7 demonstrates the speedup achieved while increasing the number of processes from 80 to 600 with an efficiency of 95.5. In Figure 6b, note that the execution time remains nearly constant as the number of process is increased to 640 for the fixed problem size/core of 0.5 million which demonstrates the weak scalability of the algorithm. These results clearly highlight the scalability of the RAS-V3 preconditioner against problem size and processes (subdomains).
In this section we apply the two-grid RAS - V3 preconditioner to the region of Southern Ontario. This region, consisting of 27 Public Health Units (PHUs), is densely populated, accounting for 95% of the population of the province of Ontario, while only occupying approximately 13% of area [60]. The public data on COVID-19 statistics from these PHUs can help to infer the status of COVID-19 infections in time and space. We utilized the open source software QGIS [61] to define the geographical boundaries and generate a mesh file [62]. The meshed domain was then subdivided into different subdomains for the domain decomposition solver. The mesh of Southern Ontario subdivided into 200 subdomains is shown in Figure 8 (overlapping parts not shown for clarity). Note that these subdomains do not correspond to the aforementioned PHUs. The initial conditions were obtained from publicly reported testing data on September 1, 2020. We generated the densities of each compartment as a sum of 27 Gaussian pulses, centered at the main city of each PHU (following a similar approach as [7]):
s(x,0)=27∑i=1Aiexp[(x−xi)2+(y−yi)22B2i], | (5.2) |
where each (xi,yi) denotes the coordinates of the cities, and Bi represents the radius around (xi,yi) which captures 95 % of the population of the ith PHU. The value of Ai is a constant which ensures that the integral of the two dimensional function over the entire domain equals to the total number of people in the respective compartment for that PHU.
The parameters reported for the Southern Ontario case involved a two-step manual tuning. First, an ODE-based SEIRD model was initialized with the values used for the square domain. The time-invariant rate parameters γE=1/15 days, σ=1/5 days, γR=1/11 days and γD=1/750 days (summarized in 1) were then obtained by manually adjusting these parameters until satisfactory agreement was achieved between the models output and the reported statistics for active cases and deaths for the six-month period from September 1, 2020 to February 28, 2021 (representing the second wave of infection in the province [63]). Second, using the PDE model with negligible diffusion (νs,νe,νi,νr), the parameters βE and βI were tuned to match the integrated model output with the aggregated case counts for all PHUs in Southern Ontario. Then, the diffusion coefficients were made to be non-zero to allow for the modelling of the localized spatial interaction/spread of the different compartments. It was assumed that the diffusion among susceptible, exposed and recovered persons would be similar. However, the diffusion of infectious individuals was expected to be lower due to self-isolation restrictions. An iterative process was used wherein the diffusion coefficients were adjusted to encourage sufficient mixing of the population within the city without causing significant inter-city population movement. Then the infection rate parameters βE and βI and the Allee parameter A were adjusted by trial and error until the results shown in Figure 9 were achieved.
We have defined the infection rate parameters βi(x,t) and βe(x,t) as sigmoid functions in time to mimic the sudden reduction in disease transmission following the provincial lockdown after the 2020 holiday season as shown in Figure 9a. Furthermore, we subdivided the domain of Southern Ontario into western, central and eastern regions as in Figure 9b. The central region contains many PHUs with large populations in close proximity to one another. This region is separated from the larger population centers in the eastern and western regions of the province by large areas of low population density. In order to capture this spatiotemporal variation of the infection rate, we assume:
βi(x,t)=βe(x,t)=(0.101−0.051+exp(130−t))⏟β(t)β(x), | (5.3) |
where,
β(x)=βcentral+βeastern−βcentral(1+exp−5(x−xeastern))+βwestern−βcentral(1+exp10(x−xwestern)), | (5.4) |
with xeastern and xwestern denoting the longitudinal boundaries separating the eastern from the central region and the central from the western region of the domain, respectively. Similarly βeastern, βcentral and βwestern are the infection rates in the corresponding regions. In Eq (5.4), we model the spatial variation for βi(x,t) and βe(x,t) along the longitude x, but they are invariant along the latitude y.
Figure 10 shows the averaged infected population counts for different regions against the reported field data. The contour plots in Figures 11 and 12 show the interaction of the populations of adjacent PHUs in the central region (containing the densely populated Greater Toronto and Hamilton areas) as the case counts increase. We can observe similar growth in the number of infections in the more isolated regions of Windsor in the western region as well as Ottawa in the eastern region. The use of a heterogeneous population density-dependent diffusion coefficients permit the mixing of the population at a relatively local scale within individual PHUs and with adjacent PHUs in high density regions. This does not permit a realistic account of the spread of the disease among the population centers that are separated by regions of low density. In this case, more sophisticated methods such as that in [22], which uses kinetic transport equations to model commuters and diffusion to model non-commuters, or [8] which defines an anisotropic diffusion coefficient according to various geographical features (such as highway networks, rivers and mountains) would be required. A more rigorous framework would model the effects of human mobility patterns (e.g., [6,64]) to reflect the spatial spread. Note that the spatial variability in COVID-19 incidence rates can be related to various social and economic parameters (e.g., [5] and [64]), which can be captured in the model by including much finer stratifications of age, socio-economic status etc. as described in [1]. Although these stratifications are not included in the current model, an extensive 22-compartment model is proposed for future studies (see C).
To demonstrate the scalability of the solver for a realistic case involving Southern Ontario, we varied the problem size from 47.69 to 186.57 million. Figure 13a demonstrates the reduction in time to solution for a problem size of 186.57 million with an increasing number of processes. Using 800 processes as the reference we can calculate the speedup and efficiency. Sub-linear speedup was observed as we increased the number of processes to 1600 with an efficiency of 92.1 which then decreased to 74.7 using 3200 processes. The decreased efficiency using 3200 processes is due to the parallel overhead stemming from the communication cost, as incurred by using a large number of processes. For weak scalability in Figure 13b, the problem size was increased from 47.69 to 186.57 million with a corresponding increase in the number of processes from 800 to 3200. The moderate increase in the execution time again can be attributed to the increased communication cost.
Extrapolating the results in Figure 13a to a single core, the simulation time for three months with a problem size of 186.57 million can be approximated by using a time step of 0.1 days as follows, t1=133010×8001×3×300.1×13600×24=1108.3days. However, with 3200 processes this time could be reduced to, t3200=445.110×3×300.1×13600×24=0.46365days=11.128hours. Hence, the speedup can be computed as 1108.3/0.46365 = 2390.3 with an efficiency of 2390.3/3200 = 74.7. These values demonstrate the savings in computational cost by utilizing DD-based solvers instead of a sequential solver.
Here, we have only employed deterministic modelling with constant parameters showing the excellent scalability of DD-based preconditioners. However, model parameters like the infection rate, the diffusion coefficient, recovery rates etc., as well as initial conditions of the system are not precisely known. Moreover, error in modelling and noise in the data could also be taken into account by using a stochastic error term. It is thus important to consider a stochastic model to calibrate and reliably predict the infections with uncertainty bounds. The increased dimensionality and increase in time taken for likelihood evaluations with the sampling approach could be effectively reduced by using the sampling-free approaches and DD-based solvers [65,66,67]. Details on future works that extend this five-compartment model to the 22-compartment model and apply the state of the art Bayesian inference algorithms for reliable predictions are provided in [1].
A PDE-based compartmental model for COVID-19 is essential for continuous space-time trace of infections. For high resolution meshes, finely stratified compartmental models can drastically increase the computational requisite needed to accurately capture the disease dynamics. In this investigation, a DD-based parallel scalable iterative solver was developed to enhance the computational efficacy of these complex models. A two-grid RAS preconditioner equipped with an algebraic multigrid preconditioner for the coarse solver provides excellent scalability. A five-compartment SEIRD model of COVID-19 for a large geographical domain of Southern Ontario has been used to demonstrate the scalability of the solver in a realistic setting. The solver is capable of simulating the infections for a period of up to three months for a problem size of 186.57 million within 12 hours when using 3200 processes, thereby saving orders of magnitude computational time as compared to conventional sequential solvers.
The authors declare they have not used Artificial Intelligence (AI) tools in the creation of this article.
Sudhi Sharma acknowledges the support of the Ontario Trillium Scholarship for International Doctoral Students. Brandon Robinson acknowledges the support of the Alexander Graham Bell Canada Graduate Scholarships-Doctoral Program from the Natural Sciences and Engineering Research Council of Canada (NSERC) and the Ontario Graduate Scholarship. Abhijit Sarkar acknowledges the support of a Discovery Grant from NSERC.
The authors declare that there is no conflict of interest.
The bilinear form of Eq (2.12) is explicitly written for each of the compartments by using a decoupled approach below. This approximation of handling each PDE seperately works best for weakly coupled systems. This approach has advantages for a stochastic extension of a sampling-free method for uncertainty quantification [24] whereby each scalar PDE is transformed into a coupled set of deterministic PDEs. More elaborate studies on this aspect is a subject of future research. Note that we have ignored α and μ terms in the formulations below since their values are considered to be zero for the current study, although their inclusion is straightforward. We define the different indices as follows:
1) n+1: current time step,
2) n: previous time step,
3) k+1: current Picard iteration number and
4) k: previous Picard iteration number.
The weak form for the SEIRD compartmental model can be written as follows:
Susceptible
(sn+1,k+1,ϕS)+Δt((1−ANn+1,k)βIsn+1,k+1in+1,k,ϕS)+Δt((1−ANn+1,k)βEsn+1,k+1en+1,k,ϕS)+Δt(Nn+1,kˉνS∇sn+1,k+1,∇ϕS)=(sn,ϕS) | (A.1) |
Exposed
(en+1,k+1,ϕE)−Δt((1−ANn+1,k)βEsn+1,k+1en+1,k+1,ϕE)+Δt((σ+γE)en+1,k+1,ϕE)+Δt(Nn+1,kˉνE∇en+1,k+1,∇ϕE)=(en,ϕE)+Δt((1−ANn+1,k)βIsn+1,k+1in+1,k,ϕE) | (A.2) |
Infected
(in+1,k+1,ϕI)+Δt((γD+γR)in+1,k+1,ϕI)+Δt(Nn+1,kˉνI∇in+1,k+1,∇ϕI)=(in,ϕI)+Δt(σen+1,k+1,ϕI) | (A.3) |
Recovered
(rn+1,k+1,ϕR)+Δt(Nn+1,kˉνR∇rn+1,k+1,∇ϕR)=(rn,ϕR)+Δt(γRin+1,k+1,ϕR)+Δt(γEen+1,k+1,ϕR) | (A.4) |
Deceased
(dn+1,k+1,ϕD)=(dn,ϕD)+Δt(γDin+1,k+1,ϕD), | (A.5) |
where ϕS,ϕE,ϕI,ϕR and ϕD are the test functions for each compartments. Note that once the solution is obtained for a particular (e.g., susceptible) compartment at the iteration k+1, its updated value can be used in the calculations of the other compartments. This has been used to improve the convergence rate of the algorithm [7].
The SEIRD compartmental model can be verified by using the process of the method of manufactured solutions (MMS). Convergence of the finite element solutions with increasing discretizations in space and time are studied. This process is applied to both one-dimensional and two-dimensional models. Validation of the model is conducted by comparing the spatially averaged PDE solution to an ODE model which provides a time trace of aggregated infection over a region.
MMS is a process of generating analytical solutions to mathematical models of a system to verify the numerical solutions [68,69]. This approach can help to detect errors in numerical implementations and solution accuracy. Mathematical models of many physical processes do not have exact analytical solutions; and hence, numerical methods are used to obtain solutions. The MMS can verify the numerical solution through the manufactured solution. A compatible forcing function to generate the manufactured solution is then found by solving the model backwards. This forcing function is used to generate the numerical solutions and their accuracy can be verified through the manufactured solutions.
Typically, three different acceptance criteria for the test can be used, namely the percentage error, consistency, and order of accuracy. Consistency ensures that the discretization error decreases to zero as the grid size tends to zero [68]. However, it is not feasible to test this aspect since reducing the grid size to zero is computationally impractical. The order of accuracy criterion checks for consistency and calculates the order in which the error decreases. The observed order of accuracy, ps for spatial discretization and pt for temporal refinement are calculated as follows [68]:
Egrid1,s≈CsΔhp | (B.1) |
Egrid2,s≈Cs(Δh/rs)p | (B.2) |
Egrid1,t≈CtΔtp | (B.3) |
Egrid2,t≈Ct(Δt/rt)p | (B.4) |
ps≈log(Egrid1,sEgrid2,s)log(rs) | (B.5) |
pt≈log(Egrid1,tEgrid2,t)log(rt) | (B.6) |
where Egrid1,s,Egrid2,s,Egrid1,t and Egrid2,t are the error in discretization for grid 1 and grid 2 in spatial and temporal scales respectively, Δh is the grid spacing, Δt is the time step, rs and rt are the refinement ratios which dictate the amount of refinement in spatial and temporal discretizations, respectively, and Cs and Ct are the constants independent of Δh and Δt. The theoretical order of accuracy for a triangular element with linear interpolation functions can be estimated to be two [70]. Similarly, the temporal order of accuracy for the backward Euler implicit scheme can be computed as one [71]. We test our numerical solutions to retain the theoretical order of accuracy as obtained from the interpolation functions and temporal discretization scheme. The relative error ϵα for any given compartment α=s,e,i,r,d is calculated as:
ϵα=∥u−uh∥2∥u∥2 | (B.7) |
where u,uh are the true solution and its finite element counterpart for a compartment respectively. Hence, the total relative error can be computed as the sum of all individual compartments.
The order of accuracy in spatial and temporal dimensions for a simple one-dimensional SEIRD model is examined in this section. The manufactured solutions for each compartment is expressed as follows:
s=Bsin(10x+0.2t)+AS | (B.8) |
e=Bsin(10x+0.2t)+AE | (B.9) |
i=Bsin(10x+0.2t)+AI | (B.10) |
r=Bsin(10x+0.2t)+AR | (B.11) |
d=Bsin(10x+0.2t)+AD, | (B.12) |
where B=25, AS=500, AE=300, AI=200, AR=100 and AD=80 are the constant parameter values.
Parameter | Value | Units |
A | 0 | people |
βI,βE | 0.01 | 1people×days |
νS,νR | 4.5×10−5 | 1people×days |
νE | 10−3 | 1people×days |
νI | 10−10 | 1people×days |
γR | 1/24 | 1days |
γD | 1/160 | 1days |
σ | 1/8 | 1days |
γE | 1/6 | 1days |
The model parameters used are given in Table B1. Note that the domain is normalized by dividing with the original length as ˆX=x/L which is also reflected in the units. The initial conditions and Dirichlet boundary conditions on both ends are derived from the manufactured solution. Since the model involves both spatial and temporal discretizations, it is important to remove the error caused by one discretization on the other. Thus we chose a sufficiently small time step of Δt=10−5 days to remove any error propagating from the temporal scale while computing the order of the accuracy in the spatial scale, ps as shown in 4. Similarly we fixed the characteristic element length Δh=2×10−4 and calculated the error at 5 days to estimate the order of accuracy in the temporal scale, pt as in Table B3. Note from Tables B2 and B3 that the estimated accuracies of spatial and temporal discretizations were close to 2 and 1 as expected from theoretical considerations. Figures B1 demonstrates that the numerical solutions and manufactured solutions match closely both in space and time with Δh=0.0005 and Δt=0.1 days.
Δh (Characteristic element length) | Error at 0.002 days | Order of Accuracy (ps) |
0.05 | 0.01289 | |
0.02 | 0.00208 | 1.9920 |
0.01 | 0.00052 | 1.9985 |
0.002 | 2.0809×10−5 | 1.9998 |
0.001 | 5.2025×10−6 | 1.9999 |
0.0005 | 1.3008×10−6 | 1.9998 |
0.0002 | 2.0842×10−7 | 1.9985 |
Δt (Time step) | Error at 5 days | Order of Accuracy (pt) |
0.1 | 0.00374 | |
0.01 | 0.00037 | 0.9995 |
0.005 | 0.00019 | 0.9994 |
0.001 | 3.7553×10−5 | 0.9982 |
0.0005 | 1.8847×10−5 | 0.9946 |
The sinusoidal functions similar to those in the one-dimensional case are used as the manufactured solutions for the two-dimensional case as follows:
s=Bsin(10xy+0.2t)+AS | (B.13) |
e=Bsin(10xy+0.2t)+AE | (B.14) |
i=Bsin(10xy+0.2t)+AI | (B.15) |
r=Bsin(10xy+0.2t)+AR | (B.16) |
d=Bsin(10xy+0.2t)+AD, | (B.17) |
where B=25, AS=500, AE=300, AI=200, AR=100 and AD=80. Other model parameters are given in 1 for the square domain, with the exception of the value of A=100. We use Neumann boundary conditions that vary in time, as derived from manufactured solution as follows:
gN,left=−10Bycos(0.2t+10xy) | (B.18) |
gN,right=10Bycos(0.2t+10xy) | (B.19) |
gN,top=10Bxcos(0.2t+10xy) | (B.20) |
gN,bottom=−10Bxcos(0.2t+10xy) | (B.21) |
A square domain with a mesh size containing 13,470 vertices and a time step of Δt=0.01 days was chosen to verify the solutions. A fully implicit approach of time discretization was implemented. The tolerance of the Picard iteration (see Eq (2.13)) was chosen to be 10−10. The GMRES solver with a one-level RAS preconditioner was used for the linearized system solve. The integrated solution over the entire domain and the solution at a point against time are shown in Figure B2. The sum of relative error for all compartments (see Eq (B.7)) reduces over time in an oscillatory fashion.
The PDE-based SEIRD model captures the variation of infection in space through the diffusion term. By decreasing the diffusion to a very low value and integrating the solution over the entire domain, the PDE model can be reduced to an equivalent ODE system. Thus, from Eq (2.1) to Eq (2.5) an ODE compartmental model describing the same dynamics as the PDE model can be expressed as follows:
dsdt=−(1−A)βIsi−(1−A)βEse | (B.22) |
dedt=(1−A)βIsi+(1−A)βEse−σe−γEe | (B.23) |
didt=σe−(γR+γD)i | (B.24) |
drdt=γEe+γRi | (B.25) |
dddt=γDi, | (B.26) |
where s, e, i, r and d denote the susceptible, exposed, infected, recovered and deceased proportion of the population, where the sum of all compartments s+e+i+r+d=1. The model parameters can be observed from the square domain case in Figure 1, with the exception of the diffusion coefficients are now 10−20. As the initial condition, 10 of the total population was chosen to be in the infected compartment. The initial value of the exposed, recovered and deceased compartments were set to be zero, and the susceptible compartment was calculated as 0.9. For the PDE model, all compartment densities were initially assumed to be uniform over the entire domain. For the square domain, we considered the population sizes of 10 and 100 respectively for numerical investigation. The tolerance of the Picard iterations was set to 10−10 and the integration time step of 0.1 days was used for a total duration of 210 days. The PDE model was integrated over the entire domain and normalized by the total population for comparison with the ODE model. The results in Figure B3 demonstrates that the solutions of PDE and ODE models match closely when the diffusion coefficients are very small.
This 22-compartment PDE-based SEIRD model becomes computationally expensive which inspired the development of the scalable solvers reported in this paper. Next we describe the extension of a 22-compartment ODE-based SEIRD model to the PDE-based system to consider the geo-spatial spread of the disease dynamics. If properly calibrated by field data, such a comprehensive model can more realistically represent the disease dynamics in order to assist clinical and public health decision-makers. To this end, we chose to modify the 22-compartment ODE-based model proposed by Robinson et al. [1], to a system of PDEs, representing the model states as population densities in space and time. In the system of equations below, we use capital letters to denote model compartments (see Table C4) to maintain consistency with the format presented in [1], and we use Greek letters to denote model parameters (see Table C5).
The following 10 compartments involves a diffusion term, modeling spatio-temporal population movement : S, V, E, F, A, B, C, P, R1, R2. Publicly available data may not permit construction and calibration of stratified SEIRD models based on age, co-morbidity, sex, socio-economic status, etc. [1]. However, private health care databases (e.g., COVID-19 database from ICES [21]) can permit such detailed stratified SEIRD-based modeling.
Susceptible:∂S∂t=−λS−γVS+γTV+γR(R1+R2)+∇⋅(νS∇S) | (C.1) |
Vaccinated:∂V∂t=−(1−rV)λV+γVS−γTV+∇⋅(νV∇V) | (C.2) |
Exposed:∂E∂t=(1−δS)λS+(1−δV)(1−rV)λV−γEE+∇⋅(νE∇E) | (C.3) |
Exposed, isolating:∂Q∂t=δSλS+δV(1−rV)λV−γEQ | (C.4) |
Infectious, presymtomatic:∂A∂t=γEE−γPA+∇⋅(νA∇A) | (C.5) |
Infectious, pre-symptomatic, isolating:∂W∂t=γEQ−γPW | (C.6) |
Infectious, asymptomatic:∂F∂t=σAγPA−γAF−γDAF+∇⋅(νF∇F) | (C.7) |
Infectious, mild-to-moderate symptoms:∂B∂t=(1−σA)(1−σS)γPA−γMB−γDMB+∇⋅(νB∇B) | (C.8) |
Infectious, severe symptoms:∂C∂t=(1−σA)σSγpA−γS1C+∇⋅(νC∇C) | (C.9) |
Infectious, asymptomatic, isolating:∂X∂t=σAγPW−γAX−γDAX | (C.10) |
Infectious, mild-to-moderate symptoms, isolating:∂Y∂t=(1−σA)(1−σS)γPW−γMY−γDMY | (C.11) |
Infectious, severe symptoms, isolating:∂Z∂t=(1−σA)σSγPW−γS1Z | (C.12) |
Infectious, isolating after testing positive:∂G∂t=γDA(F+X)+γDM(B+Y)−γIG | (C.13) |
Inadequate access to health care resources:∂N∂t=(1−σH)γS1(C+Z)−γS2N | (C.14) |
Hospital:∂H∂t=σH(1−σC)γS1(C+Z)−πHH | (C.15) |
Pre-ICU:∂H1∂t=σHσCγS1(C+Z)−πAH1 | (C.16) |
ICU:∂I∂t=πAH1−πBI | (C.17) |
Post-ICU:∂H2∂t=(1−κI)πBI−πCH2 | (C.18) |
Recovered:∂R1∂t=(1−ϕC)((1−ϕM)(γIG+γA(F+X)+γM(B+Y))+(1−ϕS)((1−κN)γS2N+(1−κH)πHH+πCH2))+(1−ϕP)(1−κP)γCP−γRR1+∇⋅(νR1∇R1) | (C.19) |
Recovered with long-term health complications:∂R2∂t=(1−ϕC)(ϕM(γIG+γA(F+X)+γM(B+Y))+ϕS((1−κN)γS2N+(1−κH)πHH+πCH2))+ϕP(1−κP)γCP−γRR2−γLR2+∇⋅(νR2∇R2) | (C.20) |
Post-acute COVID-19:∂P∂t=ϕC(γIG+γA(F+X)+γM(B+Y)+(1−κN)γS2N+(1−κH)πHH+πCH2)−γCP+∇⋅(νP∇P) | (C.21) |
Death:∂D∂t=κHπHH+κIπBI+κNγS2N+γLR2+κPγCP | (C.22) |
Symbol | Definition |
S | Susceptible |
V | Vaccinated |
E | Exposed |
Q | Exposed, isolating |
A | Infectious, pre-symptomatic |
W | Infectious, pre-symptomatic, isolating |
F | Infectious, asymptomatic |
B | Infectious, mild-to-moderate symptomatic (i.e., symptoms not requiring hospitalization) |
C | Infectious, severe symptomatic (i.e., symptoms requiring hospitalization) |
X | Infectious, asymptomatic, isolating |
Y | Infectious, mild-to-moderate symptomatic, isolating |
Z | Infectious, severe symptomatic, isolating |
G | Infectious, mild-to-moderate symptomatic, isolating but not previously in isolation |
N | No access to hospital care |
H | Hospitalized, never to be admitted to the intensive care unit (ICU) |
H1 | Hospitalized, to be admitted to the ICU |
I | Hospitalized, in the ICU |
H2 | Hospitalized, after being discharged from the ICU |
R1 | Recovered, without long-term health complications |
R2 | Recovered, with long-term health complications |
P | Post-acute COVID-19 |
D | Death |
Symbol | Definition |
rV | Vaccine effectiveness (1 indicates 100% immunity, 0 indicates no immunity) |
δS | Probability that a susceptible individual exposed to the virus will self-isolate (without prior testing) |
δV | Probability that a vaccinated individual exposed to the virus will self-isolate (without prior testing) |
γA | 1/the average duration of the infectious period for asymptomatic individuals |
γC | 1/the average duration of sub-acute COVID-19 |
γDA | Rate of detection among asymptomatic cases |
γDM | Rate of detection among mild-to-moderate cases |
γE | 1/the average incubation period |
γI | 1/the average duration of self-isolation |
γL | Rate of deaths due to long-term health complications |
γM | 1/the average duration of the infectious period for individuals with mild-to-moderate symptoms |
γP | 1/the average duration of the pre-symptomatic infectious period |
γR | 1/the average effective duration of temporary immunity from having recovered from the virus |
γS1 | 1/the average duration of severe symptoms before seeking hospitalization |
γS2 | 1/the average remaining duration of symptomatic period for individuals with severe symptoms |
γT | 1/the average effective duration of temporary immunity from vaccination |
γV | Rate of vaccination |
σA | Probability that an infectious individual is asymptomatic |
σC | Probability that a hospitalized case will be admitted to the ICU |
σH | Probability that an individual has access to hospital care |
σS | Probability that a case displaying symptoms will require hospitalization |
πA | 1/the average time in hospital prior to ICU |
πB | 1/the average time in ICU |
πC | 1/the average time in hospital following ICU |
πH | 1/the average duration of hospitalization (non-ICU track) |
ϕA | Probability of acute COVID |
ϕM | Probability of long-term complications for asymptomatic, mild-to-moderate cases |
ϕS | Probability of long-term complications for severe cases |
ϕP | Probability of long-term complications for post-acute COVID-19 cases |
κH | Probability of death among hospital cases |
κI | Probability of death among ICU cases |
κN | Probability of death among cases without access to hospital care |
κP | Probability of death among post-acute COVID-19 cases |
[1] | World Health Organisation, Obesity and overweight, 2018. Available from: http://www.who.int/mediacentre/factsheets/fs311/en/. |
[2] | G. Ni, J. Zhang, F. Zheng, The current situation and trend of obesity epidemic in China, Food Nutr. China, 19 (2013), 70–74. |
[3] | World Health Organisation, What are the health consequences of being overweight?, 2013. Available from: https://www.who.int/features/qa/49/en/. |
[4] |
F. Lo, Y. Sun, J. Qiu, B. Lo, Image-based food classification and volume estimation for dietary assessment: A review, IEEE J. Biomed. Health Inform., 24 (2020), 1926–1939. https://doi.org/10.1109/JBHI.2020.2987943 doi: 10.1109/JBHI.2020.2987943
![]() |
[5] |
W. Tay, B. Kaur, R. Quek, Current developments in digital quantitative volume estimation for the optimisation of dietary assessment, Nutrients, 12 (2020), 1167. https://doi.org/10.3390/nu12041167 doi: 10.3390/nu12041167
![]() |
[6] |
I. Nyalala, C. Okinda, K. Chen, T. Korohou, L. Nyalala, C. Qi, Weight and volume estimation of poultry and products based on computer vision systems: A review, Poult. Sci., 100 (2021). https://doi.org/10.1016/j.psj.2021.101072 doi: 10.1016/j.psj.2021.101072
![]() |
[7] |
V. B. Raju, E. Sazonov, A Systematic Review of Sensor-Based Methodologies for Food Portion Size Estimation, IEEE Sens. J., 21 (2021), 12882–12899. https://doi.org/10.1109/JSEN.2020.3041023 doi: 10.1109/JSEN.2020.3041023
![]() |
[8] |
M. Sun, Q. Liu, K. Schmidt, J. Yang, N. Yao, J. Fernstrom, et al., Determination of food portion size by image processing, Proc. Annu. Int. Conf. IEEE Eng. Med. Biol. Soc., (2008), 871–874. https://doi.org/10.1109/EMBS10205.2008 doi: 10.1109/EMBS10205.2008
![]() |
[9] |
Y. Yang, W. Jia, T. Bucher, H. Zhang, M. Sun, Image-based food portion size estimation using a smartphone without a fiducial marker, Public Health Nutrition, 22 (2018), 1180–1192. https://doi.org/10.1017/S136898001800054X doi: 10.1017/S136898001800054X
![]() |
[10] |
F. Zhu, M. Bosch, I. Woo, S. Kim, C. Boushey, D. Ebert, et al., The use of mobile devices in aiding dietary assessment and evaluation, IEEE J. Sel. Top. Sign. Proces., 4 (2010), 756–766. https://doi.org/10.1109/JSTSP.2010.2051471 doi: 10.1109/JSTSP.2010.2051471
![]() |
[11] |
H. C. Chen, Y. Yue, Z. Li, J. Fernstrom, Y. Bai, C. Li, et al., Accuracy of food portion size estimation from digital pictures acquired by a chest-worn camera, Public Health Nutr., 17 (2014), 1671–1681. https://doi.org/10.1017/S1368980013003236 doi: 10.1017/S1368980013003236
![]() |
[12] |
H. Chen, W. Jia, Y. Yue, Z. Li, Y. Sun, J. Fernstrom, et al., Model-based measurement of food portion size for image-based dietary assessment using 3D/2D registration, Meas. Sci. Technol., 24 (2013). https://doi.org/10.1088/0957-0233/24/10/105701 doi: 10.1088/0957-0233/24/10/105701
![]() |
[13] |
C. Xu, Y. He, N. Khanna, C. Boushey, E. Delp, Model-based food volume estimation using 3D pose, IEEE Int. Conf. Image Process., (2013), 2534–2538. https://doi.org/10.1109/ICIP.2013.6738522 doi: 10.1109/ICIP.2013.6738522
![]() |
[14] |
J. Dehais, M. Anthimopoulos, S. Shevchik, S. Mougiakakou, Two-view 3D reconstruction for food volume estimation, IEEE Trans Multimedia, 19 (2017), 1090–1099. https://doi.org/10.1109/TMM.2016.2642792 doi: 10.1109/TMM.2016.2642792
![]() |
[15] |
M. Puri, Z. Zhu, Q. Yu, A. Divakaran, H. Sawhney, Recognition and volume estimation of food intake using a mobile device, Workshop Appl. Comput. Vis., (2009), 1–8. https://doi.org/10.1109/WACV.2009.5403087 doi: 10.1109/WACV.2009.5403087
![]() |
[16] |
M. Rahman, Q. Li, M. Pickering, M. Frater, D. Kerr, C. Bouchey, et al., Food volume estimation in a mobile phone based dietary assessment system, Int. Conf. Signal Image Technol. Internet Based Syst., (2012), 988–995. https://doi.org/10.1109/SITIS.2012.146 doi: 10.1109/SITIS.2012.146
![]() |
[17] |
T. Suzuki, K. Futatsuishi, K. Yokoyama, N. Amaki, Point cloud processing method for food volume estimation based on dish space, Proc. Annu. Int. Conf. IEEE Eng. Med. Biol. Soc., (2020), 5665–5668. https://doi.org/10.1109/EMBC44109.2020.9175807 doi: 10.1109/EMBC44109.2020.9175807
![]() |
[18] | H. Yin, 3D reconstruction from infrared stereo image pairs, Masters Abstr. Inte., 2013. |
[19] |
L. Zhou, C. Zhang, F. Liu, Z. Qiu, Y. He, Application of deep learning in food: A review. Compr. Rev. Food Sci. Food Saf., 18 (2019), 1793–1811. https://doi.org/10.1111/1541-4337.12492 doi: 10.1111/1541-4337.12492
![]() |
[20] |
F. Lo, Y. Sun, J. Qiu, B. Lo, Food volume estimation based on deep learning view synthesis from a single depth map, Nutr., 10 (2018). https://doi.org/10.3390/nu10122005 doi: 10.3390/nu10122005
![]() |
[21] |
F. Boemer, E. Ratner, A. Lendasse, Parameter-free image segmentation with SLIC, Neurocomputing, 277 (2018), 228–236. https://doi.org/10.1016/j.neucom.2017.05.096 doi: 10.1016/j.neucom.2017.05.096
![]() |
[22] |
J. Hou, C. Sha, L. Chi, Q. Xia, N. Qi, Merging dominant sets and DBSCAN for robust clustering and image segmentation, IEEE Int. Conf. Image Process., (2014), 4422–4426. https://doi.org/10.1109/ICIP.2014.7025897 doi: 10.1109/ICIP.2014.7025897
![]() |
[23] |
P. Torr, A. Zisserman, MLESAC: A New Robust Estimator with Application to Estimating Image Geometry, Comput. Vis. Image Und., 78 (2000), 138–156, https://doi.org/10.1006/cviu.1999.0832 doi: 10.1006/cviu.1999.0832
![]() |
1. | Philippe Bisaillon, Brandon Robinson, Mohammad Khalil, Chris L. Pettit, Dominique Poirel, Abhijit Sarkar, Robust Bayesian state and parameter estimation framework for stochastic dynamical systems with combined time-varying and time-invariant parameters, 2024, 575, 0022460X, 118106, 10.1016/j.jsv.2023.118106 | |
2. | Vo Anh Khoa, Pham Minh Quan, Ja’Niyah Allen, Kbenesh W. Blayneh, Efficient relaxation scheme for the SIR and related compartmental models, 2025, 84, 18777503, 102478, 10.1016/j.jocs.2024.102478 |
Parameter | Square domain (Section 5.1) | Southern Ontario (Section 5.2) | Units |
A | 500 | 8.9×10−3 | peoplekm2 |
βI,βE | 3.78×10−4 | Eq (5.3) | km2people×days |
νS,νE,νR | 3.94×10−6 | 4.5×10−7 | km4people×days |
νI | 10−8 | 10−9 | km4people×days |
γR | 1/24 | 1/11 | 1days |
γD | 1/160 | 1/750 | 1days |
σ | 1/7 | 1/5 | 1days |
γE | 1/6 | 1/15 | 1days |
Variants | Average Number of Krylov iterations | Average Picard iteration count | Time taken for 10 steps (s) |
One-level RAS | 3399 | 4 | 868 |
Two-grid RAS | 21 | 4 | 1308 |
Two-grid RAS - V2 | 26 | 4 | 530 |
Two-grid RAS - V3 | 21 | 4 | 434 |
Parameter | Value | Units |
A | 0 | people |
βI,βE | 0.01 | 1people×days |
νS,νR | 4.5×10−5 | 1people×days |
νE | 10−3 | 1people×days |
νI | 10−10 | 1people×days |
γR | 1/24 | 1days |
γD | 1/160 | 1days |
σ | 1/8 | 1days |
γE | 1/6 | 1days |
Δh (Characteristic element length) | Error at 0.002 days | Order of Accuracy (ps) |
0.05 | 0.01289 | |
0.02 | 0.00208 | 1.9920 |
0.01 | 0.00052 | 1.9985 |
0.002 | 2.0809×10−5 | 1.9998 |
0.001 | 5.2025×10−6 | 1.9999 |
0.0005 | 1.3008×10−6 | 1.9998 |
0.0002 | 2.0842×10−7 | 1.9985 |
Δt (Time step) | Error at 5 days | Order of Accuracy (pt) |
0.1 | 0.00374 | |
0.01 | 0.00037 | 0.9995 |
0.005 | 0.00019 | 0.9994 |
0.001 | 3.7553×10−5 | 0.9982 |
0.0005 | 1.8847×10−5 | 0.9946 |
Symbol | Definition |
S | Susceptible |
V | Vaccinated |
E | Exposed |
Q | Exposed, isolating |
A | Infectious, pre-symptomatic |
W | Infectious, pre-symptomatic, isolating |
F | Infectious, asymptomatic |
B | Infectious, mild-to-moderate symptomatic (i.e., symptoms not requiring hospitalization) |
C | Infectious, severe symptomatic (i.e., symptoms requiring hospitalization) |
X | Infectious, asymptomatic, isolating |
Y | Infectious, mild-to-moderate symptomatic, isolating |
Z | Infectious, severe symptomatic, isolating |
G | Infectious, mild-to-moderate symptomatic, isolating but not previously in isolation |
N | No access to hospital care |
H | Hospitalized, never to be admitted to the intensive care unit (ICU) |
H1 | Hospitalized, to be admitted to the ICU |
I | Hospitalized, in the ICU |
H2 | Hospitalized, after being discharged from the ICU |
R1 | Recovered, without long-term health complications |
R2 | Recovered, with long-term health complications |
P | Post-acute COVID-19 |
D | Death |
Symbol | Definition |
rV | Vaccine effectiveness (1 indicates 100% immunity, 0 indicates no immunity) |
δS | Probability that a susceptible individual exposed to the virus will self-isolate (without prior testing) |
δV | Probability that a vaccinated individual exposed to the virus will self-isolate (without prior testing) |
γA | 1/the average duration of the infectious period for asymptomatic individuals |
γC | 1/the average duration of sub-acute COVID-19 |
γDA | Rate of detection among asymptomatic cases |
γDM | Rate of detection among mild-to-moderate cases |
γE | 1/the average incubation period |
γI | 1/the average duration of self-isolation |
γL | Rate of deaths due to long-term health complications |
γM | 1/the average duration of the infectious period for individuals with mild-to-moderate symptoms |
γP | 1/the average duration of the pre-symptomatic infectious period |
γR | 1/the average effective duration of temporary immunity from having recovered from the virus |
γS1 | 1/the average duration of severe symptoms before seeking hospitalization |
γS2 | 1/the average remaining duration of symptomatic period for individuals with severe symptoms |
γT | 1/the average effective duration of temporary immunity from vaccination |
γV | Rate of vaccination |
σA | Probability that an infectious individual is asymptomatic |
σC | Probability that a hospitalized case will be admitted to the ICU |
σH | Probability that an individual has access to hospital care |
σS | Probability that a case displaying symptoms will require hospitalization |
πA | 1/the average time in hospital prior to ICU |
πB | 1/the average time in ICU |
πC | 1/the average time in hospital following ICU |
πH | 1/the average duration of hospitalization (non-ICU track) |
ϕA | Probability of acute COVID |
ϕM | Probability of long-term complications for asymptomatic, mild-to-moderate cases |
ϕS | Probability of long-term complications for severe cases |
ϕP | Probability of long-term complications for post-acute COVID-19 cases |
κH | Probability of death among hospital cases |
κI | Probability of death among ICU cases |
κN | Probability of death among cases without access to hospital care |
κP | Probability of death among post-acute COVID-19 cases |
Parameter | Square domain (Section 5.1) | Southern Ontario (Section 5.2) | Units |
A | 500 | 8.9×10−3 | peoplekm2 |
βI,βE | 3.78×10−4 | Eq (5.3) | km2people×days |
νS,νE,νR | 3.94×10−6 | 4.5×10−7 | km4people×days |
νI | 10−8 | 10−9 | km4people×days |
γR | 1/24 | 1/11 | 1days |
γD | 1/160 | 1/750 | 1days |
σ | 1/7 | 1/5 | 1days |
γE | 1/6 | 1/15 | 1days |
Variants | Average Number of Krylov iterations | Average Picard iteration count | Time taken for 10 steps (s) |
One-level RAS | 3399 | 4 | 868 |
Two-grid RAS | 21 | 4 | 1308 |
Two-grid RAS - V2 | 26 | 4 | 530 |
Two-grid RAS - V3 | 21 | 4 | 434 |
Parameter | Value | Units |
A | 0 | people |
βI,βE | 0.01 | 1people×days |
νS,νR | 4.5×10−5 | 1people×days |
νE | 10−3 | 1people×days |
νI | 10−10 | 1people×days |
γR | 1/24 | 1days |
γD | 1/160 | 1days |
σ | 1/8 | 1days |
γE | 1/6 | 1days |
Δh (Characteristic element length) | Error at 0.002 days | Order of Accuracy (ps) |
0.05 | 0.01289 | |
0.02 | 0.00208 | 1.9920 |
0.01 | 0.00052 | 1.9985 |
0.002 | 2.0809×10−5 | 1.9998 |
0.001 | 5.2025×10−6 | 1.9999 |
0.0005 | 1.3008×10−6 | 1.9998 |
0.0002 | 2.0842×10−7 | 1.9985 |
Δt (Time step) | Error at 5 days | Order of Accuracy (pt) |
0.1 | 0.00374 | |
0.01 | 0.00037 | 0.9995 |
0.005 | 0.00019 | 0.9994 |
0.001 | 3.7553×10−5 | 0.9982 |
0.0005 | 1.8847×10−5 | 0.9946 |
Symbol | Definition |
S | Susceptible |
V | Vaccinated |
E | Exposed |
Q | Exposed, isolating |
A | Infectious, pre-symptomatic |
W | Infectious, pre-symptomatic, isolating |
F | Infectious, asymptomatic |
B | Infectious, mild-to-moderate symptomatic (i.e., symptoms not requiring hospitalization) |
C | Infectious, severe symptomatic (i.e., symptoms requiring hospitalization) |
X | Infectious, asymptomatic, isolating |
Y | Infectious, mild-to-moderate symptomatic, isolating |
Z | Infectious, severe symptomatic, isolating |
G | Infectious, mild-to-moderate symptomatic, isolating but not previously in isolation |
N | No access to hospital care |
H | Hospitalized, never to be admitted to the intensive care unit (ICU) |
H1 | Hospitalized, to be admitted to the ICU |
I | Hospitalized, in the ICU |
H2 | Hospitalized, after being discharged from the ICU |
R1 | Recovered, without long-term health complications |
R2 | Recovered, with long-term health complications |
P | Post-acute COVID-19 |
D | Death |
Symbol | Definition |
rV | Vaccine effectiveness (1 indicates 100% immunity, 0 indicates no immunity) |
δS | Probability that a susceptible individual exposed to the virus will self-isolate (without prior testing) |
δV | Probability that a vaccinated individual exposed to the virus will self-isolate (without prior testing) |
γA | 1/the average duration of the infectious period for asymptomatic individuals |
γC | 1/the average duration of sub-acute COVID-19 |
γDA | Rate of detection among asymptomatic cases |
γDM | Rate of detection among mild-to-moderate cases |
γE | 1/the average incubation period |
γI | 1/the average duration of self-isolation |
γL | Rate of deaths due to long-term health complications |
γM | 1/the average duration of the infectious period for individuals with mild-to-moderate symptoms |
γP | 1/the average duration of the pre-symptomatic infectious period |
γR | 1/the average effective duration of temporary immunity from having recovered from the virus |
γS1 | 1/the average duration of severe symptoms before seeking hospitalization |
γS2 | 1/the average remaining duration of symptomatic period for individuals with severe symptoms |
γT | 1/the average effective duration of temporary immunity from vaccination |
γV | Rate of vaccination |
σA | Probability that an infectious individual is asymptomatic |
σC | Probability that a hospitalized case will be admitted to the ICU |
σH | Probability that an individual has access to hospital care |
σS | Probability that a case displaying symptoms will require hospitalization |
πA | 1/the average time in hospital prior to ICU |
πB | 1/the average time in ICU |
πC | 1/the average time in hospital following ICU |
πH | 1/the average duration of hospitalization (non-ICU track) |
ϕA | Probability of acute COVID |
ϕM | Probability of long-term complications for asymptomatic, mild-to-moderate cases |
ϕS | Probability of long-term complications for severe cases |
ϕP | Probability of long-term complications for post-acute COVID-19 cases |
κH | Probability of death among hospital cases |
κI | Probability of death among ICU cases |
κN | Probability of death among cases without access to hospital care |
κP | Probability of death among post-acute COVID-19 cases |