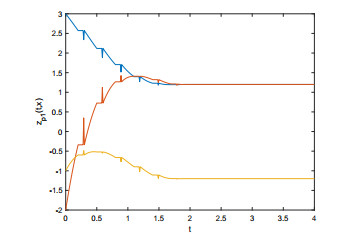
We propose a new mathematical framework for the addition of stochastic attachment to biofilm models, via the use of random ordinary differential equations. We focus our approach on a spatially explicit model of cellulolytic biofilm growth and formation that comprises a PDE-ODE coupled system to describe the biomass and carbon respectively. The model equations are discretized in space using a standard finite volume method. We introduce discrete attachment events into the discretized model via an impulse function with a standard stochastic process as input. We solve our model with an implicit ODE solver. We provide basic simulations to investigate the qualitative features of our model. We then perform a grid refinement study to investigate the spatial convergence of our model. We investigate model behaviour while varying key attachment parameters. Lastly, we use our attachment model to provide evidence for a stable travelling wave solution to the original PDE-ODE coupled system.
Citation: Jack M. Hughes, Hermann J. Eberl, Stefanie Sonner. A mathematical model of discrete attachment to a cellulolytic biofilm using random DEs[J]. Mathematical Biosciences and Engineering, 2022, 19(7): 6582-6619. doi: 10.3934/mbe.2022310
[1] | Prasina Alexander, Fatemeh Parastesh, Ibrahim Ismael Hamarash, Anitha Karthikeyan, Sajad Jafari, Shaobo He . Effect of the electromagnetic induction on a modified memristive neural map model. Mathematical Biosciences and Engineering, 2023, 20(10): 17849-17865. doi: 10.3934/mbe.2023793 |
[2] | P. E. Greenwood, L. M. Ward . Rapidly forming, slowly evolving, spatial patterns from quasi-cycle Mexican Hat coupling. Mathematical Biosciences and Engineering, 2019, 16(6): 6769-6793. doi: 10.3934/mbe.2019338 |
[3] | Pan Wang, Xuechen Li, Qianqian Zheng . Synchronization of inertial complex-valued memristor-based neural networks with time-varying delays. Mathematical Biosciences and Engineering, 2024, 21(2): 3319-3334. doi: 10.3934/mbe.2024147 |
[4] | Mahtab Mehrabbeik, Fatemeh Parastesh, Janarthanan Ramadoss, Karthikeyan Rajagopal, Hamidreza Namazi, Sajad Jafari . Synchronization and chimera states in the network of electrochemically coupled memristive Rulkov neuron maps. Mathematical Biosciences and Engineering, 2021, 18(6): 9394-9409. doi: 10.3934/mbe.2021462 |
[5] | Shuai Zhang, Yongqing Yang, Xin Sui, Yanna Zhang . Synchronization of fractional-order memristive recurrent neural networks via aperiodically intermittent control. Mathematical Biosciences and Engineering, 2022, 19(11): 11717-11734. doi: 10.3934/mbe.2022545 |
[6] | Guowei Wang, Yan Fu . Spatiotemporal patterns and collective dynamics of bi-layer coupled Izhikevich neural networks with multi-area channels. Mathematical Biosciences and Engineering, 2023, 20(2): 3944-3969. doi: 10.3934/mbe.2023184 |
[7] | Karim El Laithy, Martin Bogdan . Synaptic energy drives the information processing mechanisms in spiking neural networks. Mathematical Biosciences and Engineering, 2014, 11(2): 233-256. doi: 10.3934/mbe.2014.11.233 |
[8] | Hai Lin, Jingcheng Wang . Pinning control of complex networks with time-varying inner and outer coupling. Mathematical Biosciences and Engineering, 2021, 18(4): 3435-3447. doi: 10.3934/mbe.2021172 |
[9] | Wenjing Wang, Jingjing Dong, Dong Xu, Zhilian Yan, Jianping Zhou . Synchronization control of time-delay neural networks via event-triggered non-fragile cost-guaranteed control. Mathematical Biosciences and Engineering, 2023, 20(1): 52-75. doi: 10.3934/mbe.2023004 |
[10] | Biwen Li, Xuan Cheng . Synchronization analysis of coupled fractional-order neural networks with time-varying delays. Mathematical Biosciences and Engineering, 2023, 20(8): 14846-14865. doi: 10.3934/mbe.2023665 |
We propose a new mathematical framework for the addition of stochastic attachment to biofilm models, via the use of random ordinary differential equations. We focus our approach on a spatially explicit model of cellulolytic biofilm growth and formation that comprises a PDE-ODE coupled system to describe the biomass and carbon respectively. The model equations are discretized in space using a standard finite volume method. We introduce discrete attachment events into the discretized model via an impulse function with a standard stochastic process as input. We solve our model with an implicit ODE solver. We provide basic simulations to investigate the qualitative features of our model. We then perform a grid refinement study to investigate the spatial convergence of our model. We investigate model behaviour while varying key attachment parameters. Lastly, we use our attachment model to provide evidence for a stable travelling wave solution to the original PDE-ODE coupled system.
The memristor is the fourth basic circuit element, and it was firstly proposed by Chua in 1971 [1]. The first memristor device was realized in 2008 [2], and since then it has received much attention from research area, such as neural networks, signal processing and artificial intelligence. As a particular type of neural networks, memristive neural networks (MNNs) have become an interesting topic for researchers [3,4,5,6]. MNNs can be regarded as a switch system since the memristive connection weights switch according to the system states. Compared with the traditional network model, MNNs have more equilibrium points and can better modify the dynamics of neurons in human brains. Lately, the dynamical analysis of MNNs has attracted great attention [7,8,9].
As we know, reaction-diffusion influences are important and common in chemistry, biology and neuroscience. Since the electrons in circuits move in a nonuniform electric field, the state of the electrons dose not only depend on time but also on the spatial information. Due to this reason, MNNs with reaction-diffusion terms can better modify the real-world systems than the traditional MNNs. Recently, the research on dynamics of reaction-diffusion MNNs has made great progress, such as stability [10], passivity [11,12], and asymptotical synchronization [13,14]. However, most of these results only focus on the cooperative interactions between neurons, while the competitive relationships have not been considered yet.
In the real world, competition and cooperation relationships coexist in many dynamic systems. For instance, in biological systems, interactions between cells may be cooperative or competitive; in social networks, relationships between people may be hostile or friendly. Hence, it is meaningful and necessary to consider the model of coopetition networks, in which the competition and cooperation relationships coexist [15,16,17,18,19,20,21]. Due to the existence of competition relationships, the traditional synchronization cannot be realized, and instead we will consider bipartite synchronization. In Reference [15], the idea of bipartite consensus in coopetition networks was first proposed, it requires that all nodes' states will reach the consensus in modulus but may not in sign. Recently, bipartite synchronization of coopetition networks has attracted the attention of many scholars [18,19,20,21]. In Reference [19], by designing an adaptive control strategy, the bipartite synchronization of coupled coopetition MNNs is investigated. In Reference [20], the model of inertial MNNs with antagonistic interactions is considered, and the criteria for bipartite synchronization are derived in both the leaderless and leader-following case. However, the above works only focus on the asymptotic or exponential synchronization, and how to improve the convergence speed in bipartite synchronization still remains an open topic.
Synchronization is an important phenomenon in engineering and biological systems. As we know, the convergence speed is an important issue in synchronization. To overcome the constraints of long convergence time, the issue of finite-time synchronization (FTS) is proposed [24]. Different from asymptotic synchronization [22,23], FTS guarantees the system will reach synchronization in a finite time. However, the convergence time of FTS is related to the initial conditions [24,25,26,27], which may be unmeasurable in practice. To solve this problem, the idea of fixed-time synchronization (FxT) is introduced [28]. Although the FxTS control removes the limitation of initial dependence, its settling time is related to the control parameters [28,29,30,31]. Hence, the settling time of traditional FTS and FxTS control still depend on the initial conditions and system parameters, which is a great limitation for some practical applications. To solve this problem, prespecified-time synchronization (PTS) control is proposed [32,33,34,35], in which the settling time is independent of both initial value and control parameters. As we know, the investigation of prespecified-time control is still very limited, especially for the coupled networks with competitive interactions.
This paper is intended to explore the prespecified-time bipartite synchronization (PTBS) of CRDMNNs with both cooperative and competitive relationships. The main contributions of this work are as follows:
1) Different from most previous results that only focus on cooperative interactions, this paper considers the model of CRDMNNs with both cooperative and competitive relationships between neurons.
2) By designing a novel control protocol, criteria for leader-following PTBS and leaderless PTBS of CRDMNNs are derived. It is shown that under the proposed protocol, all nodes' states will reach synchronization in modulus (but might not in sign) within a prescribed time.
3) Different from the traditional FTS and FxTS methods, the prespecified-time control method in this work can lead to a predetermined settling time, which is independent of both initial values and system parameters.
Notations. In this paper, sgn(⋅) denotes the signum function. The symbol T denotes vector transposition. C1 denotes the set of continuous functions. λmax(A) is the maximal eigenvalue of matrix A. ⊗ denotes the Kronecker product. Ω={x=(x1,⋯,xϑ)T|hmk≤|xk|≤hMk,k=1,⋯,ϑ} is a bounded compact set with smooth boundary ∂Ω.
A signed graph is represented by G={P,J,G}, where P={p1,p2,⋯,pN} is the node set, J⊂P×P={(pi,pj)|pi,pj∈P} denotes the edge set, G=[Gij]∈RN×N is the adjacent matrix satisfying that Gij≠0 if (pi,pj)∈J, and Gij=0 otherwise. It is assumed that Gii=0. If the relationship between nodes pi and pj is competitive (cooperative), then Gij<0 (Gij>0). Due to the existence of negative edges, the elements in the Laplacian matrix L of a signed graph are given as
lik={∑j∈Ni|Gij|,k=i,Gik,k≠i. |
Definition 1. For a signed graph, it is called structurally balanced if the node set P can be divided into two subgroups P1 and P2, where P1∪P2=P and P1∩P2=∅, such that Gij≥0 for ∀pi,pj∈Pl(l∈{1,2}) and Gij≤0 for ∀pi∈Pl,pj∈Pk(k≠l,k,l∈{1,2}) [15].
Lemma 1. Suppose L is the Laplacian matrix of a signed graph G. If G is structurally balanced, then there is a gauge transformation matrix S=diag(s1,⋯,sN) with si∈{−1,1}, such that SLS has allnonnegative entries [15].
The model of CRDMNNs with competitive interactions is:
∂zp(t,x)∂t=DΔzp(t,x)−Czp(t,x)+A(zp(t,x))f(zp(t,x))+σ∑q∈Np|Gpq|Γ(sgn(Gpq)zq(t,x)−zp(t,x))+up(t,x),p=1,⋯,n | (2.1) |
where zp(t,x)=(zp1(t,x),⋯,zpn(t,x))T∈Rn denotes the state of the pth neural network at time t and space x∈Ω⊆Rz. f(zp(t,x))=(f1(zp1(t,x)),⋯,fn(zpn(t,x)))T∈Rn denotes the activation function. Δ=∑ϑk=1(∂2∂x2k) is the Laplace diffusion operator. D=diag(d1,⋯,dn)>0. C=diag(c1,c2,⋯,cn)>0. A(zp(t,x))=[akj(zpj(t,x))]n×n stands for the memristive connection weight matrix. σ is a positive number denoting the coupling strength, and Np is the neighbor set of node p. Γ=diag(γ1,γ2,⋯,γn)∈Rn×n is the inner coupling matrix. up(t,x) is the control input to be designed. G=(Gpq)N×N is the symmetric weighted adjacency matrix of the signed graph, representing the competitive-cooperation relationship between neurons. The memristive connection weights akj(zpj(t,x)) can be described as
akj(zpj(t,x))={ˊakj,|zpj(t,x)|≤Tj,ˊakj,|zpj(t,x)|>Tj, | (2.2) |
where Tj>0 is the switching jump, and ˊakj,ˊakj are real numbers. Define
a_kj=min{ˊakj,ˊakj},ˉakj=max{ˊakj,ˊakj},a+kj=max{|ˊakj|,|ˊakj|}. |
The initial condition and Dirichlet boundary condition of zp(t,x) are
{zp(t0,x)=ψp(x),x∈Ω,zp(t,x)=0,(t,x)∈[t0,+∞)×∂Ω. | (2.3) |
Remark 1. In this article, the reaction-diffusion term is contained in the MNNs model, and it is closer to the real networks because spatial location is considered. So, our results are more general than previous results in [19,20].
Next, we make the following assumptions.
Assumption 1. The cooperative-competitive network G is structurally balanced and connected.
Assumption 2. The activation function fp(⋅) is bounded, and there existconstants σp and Υ such that
|fp(x)−sqfp(y)|≤σp|x−sqy|,∀x,y∈R,|fp(x)|≤Υ,∀x∈R. |
In addition, define F=diag(σ1,⋯,σn)∈Rn×n.
Lemma 2. If η(x)=η(x1,⋯,xϑ):Ω→R satisfies that η(x)∈C1(Ω) and η(x)|∂Ω=0, then [12]
∫Ωϑ∑k=1(η(x)∂2η(x)∂x2k)dx≤−ϑ∑k=1π2(hMk−hmk)2∫Ωη2(x)dx. |
Based on the feature of Laplacian matrix L of the signed graph, we have:
∑q∈Np|Gpq|Γ(sgn(Gpq)zq(t,x)−zp(t,x))=∑q∈NpGpqΓzq(t,x)−∑q∈Np|Gpq|Γzq(t,x)=N∑q=1lpqΓzq(t,x). | (2.4) |
Hence, CRDMNN (2.1) can be written as
∂zp(t,x)∂t=DΔzp(t,x)−Czp(t,x)+A(zp(t,x))f(zp(t,x))+σN∑q=1lpqΓzq(t,x)+up(t,x). | (2.5) |
Definition 2. The CRDMNNs System (2.5) is said to reach leaderless PTBS, if there exists a time T, which is a prespecified constant for any initial values, such that
limt→T−‖ |
Remark 2. In this work, we use z_{q}(t, x)-z_{p}(t, x) to represent the cooperation relationship between nodes p and q , and we use z_{q}(t, x)+z_{p}(t, x) to depict the competitive relationship between nodes p and q .
The System (2.5) can be rewritten as the following matrix form:
\begin{align} \frac{\partial z(t,x)}{\partial t} = &\check{D}\Delta z(t,x)-\check{C}z(t,x)+\check{A}(z(t,x))f(z(t,x))\\ &+\sigma(L\otimes\Gamma)z(t,x)+u(t,x). \end{align} | (2.6) |
where
\begin{align} &z(t,x) = (z^{T}_{1}(t,x),\cdots,z^{T}_{N}(t,x))^{T},\check{D} = I_{N}\otimes D,\\ &u(t,x) = (u^{T}_{1}(t,x),\cdots,u^{T}_{N}(t,x))^{T},\; \check{C} = I_{N}\otimes C,\\ &\check{A}(z(t,x)) = \mathrm{diag}(A(z_{1}(t,x)),\cdots,A(z_{N}(t,x))),\\ &f(z(t,x)) = (f^{T}(z_{1}(t,x)),\cdots,f^{T}(z_{N}(t,x)))^{T} \end{align} |
To achieve the main results, two classes of matrices are given. Define
\begin{eqnarray*} \label{M} M = \left[\begin{array}{ccccccc} s_{1} & -s_{2} & 0 & 0 & \cdots & 0 & 0\\ 0 & s_{2} & -s_{3} & 0 & \cdots & 0 & 0\\ 0 & 0 & s_{3} & -s_{4} & \cdots & 0 & 0\\ \vdots & \vdots & \vdots & \vdots & \vdots & \vdots & \vdots\\ 0 & 0 & 0 & 0 & \ldots & s_{N-1} & -s_{N}\\ \end{array} \right]_{(N-1)\times N} \end{eqnarray*} |
\begin{eqnarray*} \label{J} J = \left[\begin{array}{ccccccc} s_{1} & s_{1} & s_{1} & \cdots & s_{1} & s_{1}\\ 0 & s_{2} & s_{2} & \cdots & s_{2} & s_{2}\\ 0 & 0 & s_{3} & s_{3} & \cdots & s_{3}\\ \vdots & \vdots & \vdots & \vdots & \vdots & \vdots\\ 0 & 0 & 0 & \ldots & 0 & s_{N-1}\\ 0 & 0 & 0 & \ldots & 0 & 0\\ \end{array} \right]_{N\times(N-1)} \end{eqnarray*} |
Definition 3. For the CRDMNN System (2.6), define:
\begin{align} &d(z) = \|{\bf{M}}z(t,x)\|^{2} = \int_{\Omega}z^{T}(t,x){\bf{M}}^{T}{\bf{M}}z(t,x)dx, \end{align} |
where {\bf{M}} = M\otimes I_{n} . Then, the networks' states can reach leaderless PTBS, if there exists a time T , which is a prescribed constant for any initial values, such that
\begin{align} &\lim\limits_{t\rightarrow T^{-}}d(z) = 0,\; d(z)\equiv0, \forall t > T. \end{align} |
Lemma 3. For the Laplacian matrix L\in\mathbb{R}^{N\times N} , there is a matrix H\in\mathbb{R}^{(N-1)\times(N-1)} given by H = MLJ , so that the following condition is satisfied:
\begin{align} &HM = ML,\; H_{ij} = \sum^{j}_{k = 1}s_{i}s_{k}l_{ik}-s_{i+1}s_{k}l_{i+1,k} \end{align} |
For convenience, define
\begin{align} &\bar{\bf{A}} = I_{N}\otimes\bar{A},\; \bar{\bf{A}}_{1} = I_{N-1}\otimes\bar{A},\; {\bf{H}} = H\otimes\Gamma, \\ &\hat{C}_{1} = I_{N-1}\otimes C, \hat{D}_{1} = I_{N-1}\otimes D, \; {\bf{F}} = I_{N-1}\otimes F, \\ &{{\bf{D^{*}}}} = \sum\limits_{k = 1}^{\vartheta}\frac{\pi^{2}}{(h^{M}_{k}-h^{m}_{k})^{2}}\hat{D}_{1} \end{align} |
To achieve leaderless PTBS, the following controller for System (2.5) is introduced:
\begin{align} u_{p}(t,x) = &-Ks_{p}\sum^{N-1}_{j = p}sgn(s_{j}z_{j}(t,x)-s_{j+1}z_{j+1}(t,x))\\ &-\xi_{p}z_{p}(t,x)-\psi\frac{r}{T}\mu(t)z_{p}(t,x) \end{align} | (3.1) |
where K = \mathrm{diag}(k_{1}, k_{2}, \cdots, k_{n}) > 0 , \xi_{p} > 0 and \psi > 0 are control gains to be designed. r is a positive constant, and T > 0 is a prespecified time chosen by users. Let t_{b} = t_{0}+T , and \mu(t) is a function defined as:
\begin{equation} \begin{aligned} \mu(t) = \left\{\begin{array}{c} \frac{T}{t_{b}-t}, \; \; t\in[t_{0},t_{b}), \\ \; \; \; \; 1, \; \; \; \; t\in[t_{b},+\infty). \end{array}\right. \end{aligned} \end{equation} | (3.2) |
The derivative of \mu(t) can be calculated:
\begin{equation} \begin{aligned} \dot{\mu}(t) = \left\{\begin{array}{c} \frac{1}{T}\mu^{2}(t), \; \; t\in[t_{0},t_{b}), \\ \; \; \; \; 0, \; \; \; \; \; \; \; \; t\in[t_{b},+\infty). \end{array}\right. \end{aligned} \end{equation} | (3.3) |
With the control protocol (3.1), the theorem can be achieved.
Theorem 1. Under Assumptions 1 and 2, if the control gains constants k_{j}, \xi_{p} satisfy the following conditions
\begin{align} &k_{j}\geq2\sum\limits_{s = 1}^{n}(\bar{a}_{js}-\underline{a}_{js})\Upsilon,\; \; j = 1,2,\cdots,n,\\ &-\tilde{\xi}+\lambda_{\max}(-{\bf{D^{*}}}-\hat{C}_{1}+\bar{\bf{A}}_{1}\bar{\bf{A}}^{T}_{1}+{\bf{F}}^{2}+\sigma{\bf{H}})\leq-\frac{\phi}{2} \end{align} |
where \tilde{\xi} = \min_{p}\{\xi_{p}\} , and \phi > 0 is a positive constant, then the leaderless bipartite prespecified-time synchronization for CRDMNN (2.6) can be achieved with controller (3.1).
Proof. Before proceeding, let y(t, x) = {\bf{M}}z(t, x) , and then we get y_{i}(t, x) = s_{i}z_{i}(t, x)-s_{i+1}z_{i+1}(t, x), i = 1, \cdots, N-1 . Consider the Lyapunov functional
\begin{align} V(t) = &\frac{1}{2}\int_{\Omega}z^{T}(t,x){\bf{M}}^{T}{\bf{M}}z(t,x)dx. \end{align} | (3.4) |
Computing the derivative along (2.6), it follows that
\begin{align} \frac{dV(t)}{dt} = &\int_{\Omega}z^{T}(t,x){\bf{M}}^{T}{\bf{M}}\dot{z}(t,x)dx\\ = &\int_{\Omega}z^{T}(t,x){\bf{M}}^{T}{\bf{M}}\Big(\check{D}\Delta z(t,x)-\check{C}z(t,x)\\ &+\check{A}(z(t,x))f(z(t,x))+\sigma(L\otimes\Gamma)z(t,x)+u(t,x)\Big)dx. \end{align} | (3.5) |
From Lemma 2, we get
\begin{align} &\int_{\Omega}z^{T}(t,x){\bf{M}}^{T}{\bf{M}}\check{D}\Delta z(t,x)dx\\ = &\int_{\Omega}z^{T}(t,x){\bf{M}}^{T}\hat{D}_{1}{\bf{M}}\Delta z(t,x)dx\\ = &\int_{\Omega}\sum\limits_{i = 1}^{N-1}y^{T}_{i}(t,x)D\Delta y_{i}(t,x)dx\\ \leq&-\sum\limits_{k = 1}^{\vartheta}\frac{\pi^{2}}{(h^{M}_{k}-h^{m}_{k})^{2}}\int_{\Omega}\sum\limits_{i = 1}^{N-1}y^{T}_{i}(t,x)Dy_{i}(t,x)dx\\ = &-\sum\limits_{k = 1}^{\vartheta}\frac{\pi^{2}}{(h^{M}_{k}-h^{m}_{k})^{2}}\int_{\Omega}z^{T}(t,x){\bf{M}}^{T}\hat{D}_{1}{\bf{M}}z(t,x)dx\\ = &\int_{\Omega}z^{T}(t,x){\bf{M}}^{T}{\bf{D^{*}}}{\bf{M}}z(t,x)dx. \end{align} | (3.6) |
Note that
\begin{align} &{\bf{M}}\hat{A}(z(t,x))f(z(t,x))\\ = &{\bf{M}}{\bf{\bar{A}}}f(z(t,x))+{\bf{M}}(\check{A}(z(t,x))-{\bf{\bar{A}}})f(z(t,x)). \end{align} | (3.7) |
Based on Assumption 2, we have
\begin{align} &z^{T}(t,x){\bf{M}}^{T}{\bf{M}}\Big(\check{A}(z(t,x))-\bar{\bf{A}}\Big)f(z(t,x))\\ = &\sum\limits_{i = 1}^{N-1}y^{T}_{i}(t,x)\Big(s_{i}(A(z_{i}(t,x))-\bar{A})f(z_{i}(t,x))\\ &-s_{i+1}(A(z_{i+1}(t,x))-\bar{A})f(z_{i+1}(t,x))\Big)\\ = &\sum\limits_{i = 1}^{N-1}\sum\limits_{j = 1}^{n}y_{ij}(t,x)\sum\limits_{s = 1}^{n}[(a_{js}(z_{is})-\bar{a}_{js})s_{i}f_{s}(z_{is}(t,x))\\ &-(a_{js}(z_{i+1,s})-\bar{a}_{js})s_{i+1}f_{s}(z_{i+1,s}(t,x))]\\ \leq&\sum\limits_{i = 1}^{N-1}\sum\limits_{j = 1}^{n}2\sum\limits_{s = 1}^{n}(\bar{a}_{js}-\underline{a}_{js})\Upsilon|y_{ij}(t,x)|. \end{align} | (3.8) |
Similarly, we get
\begin{align} &z^{T}(t,x){\bf{M}}^{T}{\bf{M}}\bar{\bf{A}}f(z(t,x))\\ = &z^{T}(t,x){\bf{M}}^{T}\bar{\bf{A}}_{1}{\bf{M}}f(z(t,x))\\ = &\sum\limits_{i = 1}^{N-1}(s_{i}z_{i}(t,x)-s_{i+1}z_{i+1}(t,x))^{T}\bar{A}(s_{i}f(z_{i}(t,x))-s_{i+1}f(z_{i+1}(t,x)))\\ \leq&\sum\limits_{i = 1}^{N-1}y^{T}_{i}(t,x)\bar{A}\bar{A}^{T}y_{i}(t,x)+\sum\limits_{i = 1}^{N-1}y^{T}_{i}(t,x)F^{2}y_{i}(t,x)\\ = &z^{T}(t,x){\bf{M}}^{T}\bar{\bf{A}}_{1}\bar{\bf{A}}^{T}_{1}{\bf{M}}z(t,x)+z^{T}(t,x){\bf{M}}^{T}{\bf{F}}^{2}{\bf{M}}z(t,x). \end{align} | (3.9) |
By Lemma 3, we get
\begin{align} &z^{T}(t,x){\bf{M}}^{T}{\bf{M}}(L\otimes\Gamma)z(t,x)\\ = &z^{T}(t,x){\bf{M}}^{T}(M\otimes I_{n})(L\otimes\Gamma)z(t,x)\\ = &z^{T}(t,x){\bf{M}}^{T}(ML\otimes\Gamma)z(t,x)\\ = &z^{T}(t,x){\bf{M}}^{T}(HM\otimes\Gamma)z(t,x)\\ = &z^{T}(t,x){\bf{M}}^{T}(H\otimes\Gamma)(M\otimes I_{n})z(t,x)\\ = &z^{T}(t,x){\bf{M}}^{T}{\bf{H}}{\bf{M}}z(t,x), \end{align} | (3.10) |
and
\begin{align} &z^{T}(t,x){\bf{M}}^{T}{\bf{M}}u(t,x)\\ = &-\sum\limits_{i = 1}^{N-1}\sum\limits_{j = 1}^{n}k_{j}y_{ij}(t,x)sgn(y_{ij}(t,x))-\tilde{\xi}z^{T}(t,x){\bf{M}}^{T}{\bf{M}}z(t,x)\\ &-\psi\frac{r}{T}\mu(t)z^{T}(t,x){\bf{M}}^{T}{\bf{M}}z(t,x)\\ = &-\sum\limits_{i = 1}^{N-1}\sum\limits_{j = 1}^{n}k_{j}|y_{ij}(t,x)|-(\tilde{\xi}+\psi\frac{r}{T}\mu(t))z^{T}(t,x){\bf{M}}^{T}{\bf{M}}z(t,x). \end{align} | (3.11) |
From the condition in Theorem 1, we derive that
\begin{align} \dot{V}(t)\leq&\int_{\Omega}z^{T}(t,x){\bf{M}}^{T}(-\tilde{\xi}-{\bf{D^{*}}}-\hat{C}_{1}+\bar{\bf{A}}_{1}\bar{\bf{A}}^{T}_{1}+{\bf{F}}^{2}\\ &+\sigma{\bf{H}}){\bf{M}}z(t,x)dx-2\psi\frac{r}{T}\mu(t)V(t)\\ \leq&-\phi V(t)-2\psi\frac{r}{T}\mu(t)V(t). \end{align} | (3.12) |
According to the definition of \mu(t) , defining \Omega(t) = \mu^{r}(t) yields
\begin{align} \frac{r}{T}\mu(t) = \frac{\dot{\Omega}(t)}{\Omega(t)}, \; t\in[t_{0},t_{b}). \end{align} | (3.13) |
Multiplying \Omega^{2\psi}(t) on both sides of (3.12) yields
\begin{align} \frac{d[\Omega^{2\psi}(t)V(t)]}{dt}\leq-\phi[\Omega^{2\psi}(t)V(t)]. \end{align} | (3.14) |
Solving the above inequality yields
\begin{align} \Omega^{2\psi}(t)V(t)\leq e^{-\phi(t-t_{0})}\Omega^{2\psi}(t_{0})V(t_{0}). \end{align} | (3.15) |
Then, we have
\begin{align} V(t)\leq e^{-\phi(t-t_{0})}\mu(t)^{-2r\psi}\mu(t_{0})^{2r\psi}V(t_{0}). \end{align} | (3.16) |
Note that
\begin{align} \mu(t_{0})^{2r\psi} = 1, \lim\limits_{t\rightarrow t^{-}_{b}}\mu(t)^{-2r\psi} = 0. \end{align} | (3.17) |
which yields
\begin{align} \lim\limits_{t\rightarrow t^{-}_{b}}V(t) = 0. \end{align} | (3.18) |
Hence, \lim_{t\rightarrow t^{-}_{b}}\|y(t, x)\| = 0 , and the systems can reach synchronization in prespecified time T .
Next, we prove that the synchronization can be maintained on [t_{b}, +\infty) .
\begin{align} \dot{V}(t)\leq-(\phi+2\psi\frac{r}{T})V(t) = -\hat{\theta}V(t), \; t\in[t_{b},+\infty). \end{align} | (3.19) |
where \hat{\theta} = \phi+2\psi\frac{r}{T} > 0 .
Then, we can get
\begin{align} 0\leq V(t)\leq V(t_{b}) = 0,\; t\in[t_{b},+\infty). \end{align} | (3.20) |
So, we obtain that V(t)\equiv0 over [t_{b}, +\infty) . Hence, \|y(t, x)\|\equiv0 on [t_{b}, +\infty) . Based on Definition 3, leaderless PTBS for Systems (2.6) can be realized with controller (3.1). The proof is complete.
Remark 3. Different from [33,34,35], the competitive relationship between network nodes is considered in this paper, which is described by negative edges in the signed graph. It can be seen that the prescribed-time synchronization in the above papers is a special case of the bipartite prescribed-time synchronization in this work. On the other hand, compared with the asymptotic bipartite synchronization criteria in [19,20], our approach ensures that the CRDMNNs can reach bipartite synchronization within prespecified time t_{b} , which is independent of initial values and control parameters.
Remark 4. Since there are switching parameters in the memristor system, the first term of controller (3.1) is designed to remove the influence of the memristor parameters. Then, the terms -\xi_{p}z_{p}(t, x) and -\psi\frac{r}{T}\mu(t)z_{p}(t, x) are devoted to realizing the convergence in a prespecified time. The function \mu(t) plays an important role to ensure the prespecified-time synchronization. It can be seen from (3.17) that \mu(t_{0})^{2r\psi} = 1, \lim_{t\rightarrow t^{-}_{b}}\mu(t)^{-2r\psi} = 0 . In practical applications, there may exist a leader in the community. In this case, leader-following synchronization is a meaningful issue to be considered.
Next, we aim to investigate leader-following PTBS of CRDMNNs. Considering the following system with coupling delays:
\begin{align} \frac{\partial z_{p}(t,x)}{\partial t} = &D\Delta z_{p}(t,x)-Cz_{p}(t,x)+A(z_{p}(t,x))f(z_{p}(t,x))\\ &+\sigma\sum\limits_{q\in\mathcal{N}_{p}}|G_{pq}|\Gamma\Big(sgn(G_{pq})z_{q}(t-\tau,x)-z_{p}(t-\tau,x)\Big)\\ &+u_{p}(t,x). \end{align} | (3.21) |
The reference target system is:
\begin{align} \frac{\partial z_{0}(t,x)}{\partial t} = &D\Delta z_{0}(t,x)-Cz_{0}(t,x)+A(z_{0}(t,x))f(z_{0}(t,x)). \end{align} | (3.22) |
where z_{0}(t, x) = (z_{01}(t, x), z_{02}(t, x), \cdots, z_{0n}(t, x))^{T}\in\mathbb{R}^{n} is the leader node.
Definition 4. For the CRDMNN System (3.21), it is said that the network can reach leader-following PTBS, if there exists a prespecified constant T , which is independent of any initial values or control parameters, such that
\begin{align} &\lim\limits_{t\rightarrow T^{-}}\|z_{p}(t,x)-s_{p}z_{0}(t,x)\| = 0,\\ &\|z_{p}(t,x)-s_{p}z_{0}(t,x)\|\equiv0, \forall t > T, \end{align} |
where s_{p} = 1 if p\in \mathbb{P}_{1} , and s_{p} = -1 if p\in \mathbb{P}_{2} .
Define synchronization error \delta_{p}(t, x) = z_{p}(t, x)-s_{p}z_{0}(t, x) , and since \sum^{N}_{q = 1}l_{pq}s_{q} = 0 , we have
\begin{align} &\sigma\sum\limits_{q\in \mathcal{N}_{p}}|G_{pq}|\Gamma\Big(sgn(G_{pq})z_{q}(t-\tau,x)-z_{p}(t-\tau,x)\Big)\\ = &\sigma\sum^{N}_{q = 1}l_{pq}\Gamma\Big(z_{q}(t-\tau,x)-s_{q}z_{0}(t-\tau,x)+s_{q}z_{0}(t-\tau,x)\Big)\\ = &\sigma\sum\limits_{q\in \mathcal{N}_{p}}|G_{pq}|\Gamma\Big(sgn(G_{pq})\delta_{q}(t-\tau,x)-\delta_{p}(t-\tau,x)\Big) \end{align} | (3.23) |
Then, the error system can be obtained:
\begin{align} \frac{\partial \delta_{p}(t,x)}{\partial t} = &D\Delta \delta_{p}(t,x)-C\delta_{p}(t,x)+A(z_{p}(t,x))f(z_{p}(t,x))\\ &-s_{p}A(z_{0}(t,x))f(z_{0}(t,x))\\ &+\sigma\sum^{N}_{q = 1}l_{pq}\Gamma \delta_{q}(t-\tau,x)+u_{p}(t,x). \end{align} | (3.24) |
Define the norm
\begin{align} \|\delta_{p}(t,x)\|_{2} = \Big(\int_{\Omega}\delta^{T}_{p}(t,x)\delta_{p}(t,x)dx\Big)^{\frac{1}{2}} \end{align} |
The prespecified-time controller u_{p}(t, x) is designed as
\begin{align} u_{p} = \left\{ \begin{aligned} &-Ksgn(\delta_{p}(t,x))-\xi_{p}\delta_{p}(t,x)-2\psi\frac{r}{T}\mu(t)\delta_{p}(t,x)\notag\\ &-\phi\frac{\delta_{p}(t,x)}{\|\delta_{p}(t,x)\|_{2}^{2}}\int^{t}_{t-\tau}\delta^{T}_{p}(\theta,x)\delta_{p}(\theta,x)d\theta\notag\\ &-2\psi\frac{r}{T}\mu(t)\frac{\delta_{p}(t,x)}{\|\delta_{p}(t,x)\|_{2}^{2}}\int^{t}_{t-\tau}\delta^{T}_{p}(\theta,x)\delta_{p}(\theta,x)d\theta,\notag\\ &\mathrm{if}\; \delta_{p}(t,x)\neq0,\notag\\ &0,\; \; \; \mathrm{otherwise}, \end{aligned} \right. \end{align} | (3.25) |
where K = \mathrm{diag}(k_{1}, k_{2}, \cdots, k_{n}) with k_{i} > 0 , \xi_{p}, \phi, \psi > 0 are the control gains. sgn(\delta_{p}(t, x)) = (sgn(\delta_{p1}(t, x)), \cdots, sgn(\delta_{pn}(t, x)))^{T}\in \mathbb{R}^{n} . T , r and \mu(t) are defined the same as in controller (3.1).
Theorem 2. Under Assumptions 1 and 2, suppose the following conditions hold:
\begin{align} &k_{i} > 2\sum\limits_{s = 1}^{n}a^{+}_{is}\Upsilon,\; \; i = 1,2,\cdots,n,\\ &-\tilde{\xi}-c_{\min}-\sum\limits_{k = 1}^{\vartheta}\frac{\pi^{2}d_{\min}}{(h^{M}_{k}-h^{m}_{k})^{2}}+\sigma^{2}\lambda_{\max}[(L\otimes\Gamma)^{2}]\leq-\frac{\phi}{2} \end{align} |
where \phi > 0 is given in controller (3.25), \tilde{\xi} = \min_{p}\{\xi_{p}\} , c_{\min} = \min\{c_{1}, c_{2}, \cdots, c_{n}\} , and d_{\min} = \min\{d_{1}, d_{2}, \cdots, d_{n}\} . Then, leader-following PTBS for Systems (3.21) and (3.22) can be achieved under controller (3.25).
Proof. Design the Lyapunov functional
\begin{align} \label{} V(t) = &\frac{1}{2}\sum\limits_{p = 1}^{N}\int_{\Omega}\delta^{T}_{p}(t,x)\delta_{p}(t,x)dx\\ &+\int_{\Omega}\int^{t}_{t-\tau}\delta^{T}(\theta,x)\delta(\theta,x)d\theta dx. \end{align} |
Computing the derivative along (3.24), we get
\begin{align} \frac{dV(t)}{dt} = &\sum\limits_{p = 1}^{N}\int_{\Omega}\delta^{T}_{p}(t,x)\dot{\delta}_{p}(t,x)dx+\int_{\Omega}\delta^{T}(t,x)\delta(t,x)dx\\ &-\int_{\Omega}\delta^{T}(t-\tau,x)\delta(t-\tau,x)dx\\ = &\sum\limits_{p = 1}^{N}\int_{\Omega}\delta^{T}_{p}(t,x)\Big(-C\delta_{p}(t,x)+D\Delta \delta_{p}(t,x)\\ &+A(z_{p}(t,x))f(z_{p}(t,x))-s_{p}A(z_{0}(t,x))f(z_{0}(t,x))\\ &+\sigma\sum^{N}_{q = 1}l_{pq}\Gamma \delta_{q}(t-\tau,x)+u_{p}(t,x)\Big)dx\\ &+\int_{\Omega}\delta^{T}(t,x)\delta(t,x)dx-\int_{\Omega}\delta^{T}(t-\tau,x)\delta(t-\tau,x)dx. \end{align} | (3.26) |
Based on Lemma 2, we get
\begin{align} &\int_{\Omega}\delta^{T}_{p}(t,x)D\Delta \delta_{p}(t,x)dx\\ \leq&-\sum\limits_{k = 1}^{\vartheta}\frac{\pi^{2}}{(h^{M}_{k}-h^{m}_{k})^{2}}\int_{\Omega}\delta^{T}_{p}(t,x)D\delta_{p}(t,x)dx\\ \leq&-\sum\limits_{k = 1}^{\vartheta}\frac{\pi^{2}d_{\min}}{(h^{M}_{k}-h^{m}_{k})^{2}}\int_{\Omega}\delta^{T}_{p}(t,x)\delta_{p}(t,x)dx. \end{align} | (3.27) |
According to Assumption 2, we have
\begin{align} &\sum\limits_{p = 1}^{N}\delta^{T}_{p}(t,x)\Big(A(z_{p})f(z_{p}(t,x))-s_{p}A(z_{0})f(z_{0}(t,x))\Big)\\ = &\sum\limits_{p = 1}^{N}\sum\limits_{i = 1}^{n}\delta_{pi}(t,x)\sum\limits_{s = 1}^{n}\Big(a_{is}(z_{ps})f_{s}(z_{ps}(t,x))-s_{p}a_{is}(z_{0s})f_{s}(z_{0s}(t,x))\Big)\\ \leq&\sum\limits_{p = 1}^{N}\sum\limits_{i = 1}^{n}2\sum\limits_{s = 1}^{n}a^{+}_{is}\Upsilon|\delta_{pi}(t,x)|. \end{align} | (3.28) |
For the coupling terms, it can be yielded that
\begin{align} &\sigma\sum\limits_{p = 1}^{N}\delta^{T}_{p}(t,x)\sum\limits_{q = 1}^{N}l_{pq}\Gamma \delta_{q}(t-\tau,x)\\ = &\sigma \delta^{T}(t,x)(L\otimes\Gamma)\delta(t-\tau,x)\\ \leq&\delta^{T}(t-\tau,x)\delta(t-\tau,x)+\sigma^{2}\delta^{T}(t,x)(L\otimes\Gamma)^{2}\delta(t,x)\\ \leq&\delta^{T}(t-\tau,x)\delta(t-\tau,x)+\sigma^{2}\lambda_{\max}[(L\otimes\Gamma)^{2}]\delta^{T}(t,x)\delta(t,x) \end{align} | (3.29) |
For the control term (3.25), we get
\begin{align} &\sum\limits_{p = 1}^{N}\delta^{T}_{p}(t,x)u_{p}(t,x)\\ = &\sum\limits_{p = 1}^{N}\delta^{T}_{p}(t,x)\Big(-Ksgn(\delta_{p}(t,x))-\xi_{p}\delta_{p}(t,x)\\ &-\psi\frac{r}{T}\mu(t)\delta_{p}(t,x)-\frac{\phi \delta_{p}(t,x)}{\|\delta_{p}(t,x)\|_{2}^{2}}\Big|\int^{t}_{t-\tau}\delta^{T}_{p}(\theta,x)\delta_{p}(\theta,x)d\theta\Big|\\ &-2\psi\frac{r}{T}\mu(t)\frac{\delta_{p}(t,x)}{\|\delta_{p}(t,x)\|_{2}^{2}}\Big|\int^{t}_{t-\tau}\delta^{T}_{p}(\theta,x))\delta_{p}(\theta,x)d\theta\Big|\Big)\\ = &-\sum\limits_{p = 1}^{N}\sum\limits_{i = 1}^{n}k_{i}|\delta_{pi}(t,x)|-\sum\limits_{p = 1}^{N}\xi_{p}\delta^{T}_{p}(t,x)\delta_{p}(t,x)\\ &-\sum\limits_{p = 1}^{N}\phi\int^{t}_{t-\tau}\delta^{T}_{p}(\theta,x)\delta_{p}(\theta,x)d\theta-2\psi\frac{r}{T}\mu(t)\sum\limits_{p = 1}^{N}\frac{1}{2}\delta^{T}_{p}(t,x)\delta_{p}(t,x)\\ &-2\psi\frac{r}{T}\mu(t)\sum\limits_{p = 1}^{N}\int^{t}_{t-\tau}\delta^{T}_{p}(\theta,x)\delta_{p}(\theta,x)d\theta. \end{align} | (3.30) |
By combining the above inequalities, one has
\begin{align} \frac{dV(t)}{dt} = &\int_{\Omega}\delta^{T}(t,x)\Big(-\tilde{\xi}-c_{\min}-\sum\limits_{k = 1}^{\vartheta}\frac{\pi^{2}d_{\min}}{(h^{M}_{k}-h^{m}_{k})^{2}}\\ &+\lambda_{\max}[(L\otimes\Gamma)^{2}]\Big)\delta(t,x)dx\\ &-\phi\sum\limits_{p = 1}^{N}\int_{\Omega}\int^{t}_{t-\tau}\delta^{T}_{p}(\theta,x))\delta_{p}(\theta,x)d\theta dx\\ &-2\psi\frac{r}{T}\mu(t)\cdot\frac{1}{2}\sum\limits_{p = 1}^{N}\int_{\Omega}\delta^{T}_{p}(t,x)\delta_{p}(t,x)dx\\ &-2\psi\frac{r}{T}\mu(t)\sum\limits_{p = 1}^{N}\int_{\Omega}\int^{t}_{t-\tau}\delta^{T}_{p}(\theta,x)\delta_{p}(\theta,x)d\theta dx\\ \leq&-\phi V(t)-2\psi\frac{r}{T}\mu(t)V(t). \end{align} | (3.31) |
Following the discussion in Theorem 1, we derive \lim_{t\rightarrow t^{-}_{b}}\|z_{p}(t, x)-s_{p}z_{0}(t, x)\|_{2} = 0 and \|z_{p}(t, x)-s_{p}z_{0}(t, x)\|_{2}\equiv0 on [t_{b}, +\infty) . Thus, leader-following PTBS for Systems (3.21) and (3.22) can be realized with controller (3.25).
Remark 5. Different from [12,13,14], the competitive relationship and coupling delay are considered in this paper, which can better modify the real network model. Due to the existence of antagonistic interactions and coupling delays, the traditional synchronization methods in [12,13,14] cannot be directly used to realize PTBS. Moreover, the convergence speed is required to be prescribed instead of asymptotic in this work, and thus the Theorem in our work greatly generalizes the previous results on bipartite synchronization.
Remark 6. In Reference [27], by applying nonsmooth analysis and some novel inequality techniques in the complex field, several nonseparation method-based fixed-time synchronization criteria are derived. In Reference [30], the FXTS of dynamic systems are reconsidered in this article based on special functions from the view of improving the estimate accuracy for settling time and reducing the chattering caused by the sign function. In this paper, the convergence time is prescribed in advance according to actual requirements, and thus our criteria are less conservative and more flexible.
To verify our theorem, two simulation examples are proposed in this section. We consider a network with 3 nodes, and since the structural balance condition is satisfied, the node set V can be separated into V_{1} = \{1, 2\} and V_{2} = \{3\} . Then, one has s_{1} = s_{2} = 1 and s_{3} = -1 . We first consider Theorem 1.
Example 1. Choose the CRDMNNs with 2 neurons as below:
\begin{align} \frac{\partial z_{p}(t,x)}{\partial t} = &D\Delta z_{p}(t,x)-Cz_{p}(t,x)+A(z_{p}(t,x))f(z_{p}(t,x))\\ &+\sigma\sum\limits_{q\in\mathcal{N}_{p}}|G_{pq}|\Gamma\Big(sgn(G_{pq})z_{q}(t,x)-z_{p}(t,x)\Big)+u_{p}(t,x) \end{align} | (4.1) |
where z_{p}(t, x) = (z_{p1}(t, x), z_{p2}(t, x))^{T} , D = \mathrm{diag}(1, 1), C = \mathrm{diag}(2, 2) , and \Omega = \{x|-1\leq x\leq1\} . The memristive matrix A(z_{p}(t, x)) switches between \check{A} and \hat{A} , where
\begin{equation} \begin{aligned} \hat{A} = \left[\begin{array}{cc} 0.4 & 0.1 \\ 0.1 & 0.1 \\ \end{array} \right],\; \; \check{A} = \left[\begin{array}{cc} 0.1 & -0.1 \\ -0.1 & -0.1 \\ \end{array} \right] \end{aligned} \end{equation} | (4.2) |
The activation functions are chosen as
\begin{align} f_{p}(x) = 0.5(|x+1|-|x-1|). \end{align} | (4.3) |
It can be checked that F = 0.5I_{2} and \Upsilon = 0.5 . Hence, the conditions in Assumption 2 are satisfied. Choose \Gamma = I_{2} and \sigma = 1 . The Laplacian matrix is
\begin{eqnarray*} \label{} L = \left[\begin{array}{ccc} 2 & -1 & -1 \\ 0 & 2 & -1 \\ 1 & -1 & 1 \\ \end{array} \right]. \end{eqnarray*} |
The controller is
\begin{align} u_{p}(t,x) = &-Ks_{p}\sum^{N-1}_{j = p}sgn(s_{j}z_{j}(t,x)-s_{j+1}z_{j+1}(t,x))\\ &-\xi_{p}z_{p}(t,x)-\psi\frac{r}{T}\mu(t)z_{p}(t,x). \end{align} | (4.4) |
Choose feedback matrix K = 0.6I_{2} , \xi_{p} = 0.5, \psi = 1.5, r = 2 , \phi = 1 . Let t_{0} = 0 , and set the prespecified time t_{b} = 2 . It can be checked that
\begin{align} &k_{j}\geq2\sum\limits_{s = 1}^{2}(\bar{a}_{js}-\underline{a}_{js})\Upsilon,\; \; j = 1,2\\ &-\tilde{\xi}+\lambda_{\max}(-{\bf{D^{*}}}-\hat{C}_{1}+\bar{\bf{A}}_{1}\bar{\bf{A}}^{T}_{1}+\bf{F}^{2}+\sigma\bf{H})\leq-\frac{\phi}{2} \end{align} |
Thus, the conditions in Theorem 1 are satisfied. Choosing initial values in [-3, 3] , Figures 1 and 2 depict the evolutions of states z_{p1}(t, x) and z_{p2}(t, x) . It can be seen that system (4.1) can reach leaderless PTBS in prespecified time t_{b} under control law (4.4).
Next, we consider the leader-following PTBS in Theorem 2.
Example 2. Consider the following 2-node CRDMNN with coupling delays:
\begin{align} \frac{\partial z_{p}(t,x)}{\partial t} = &D\Delta z_{p}(t,x)-Cz_{p}(t,x)+A(z_{p}(t,x))f(z_{p}(t,x))\\ &+\sigma\sum\limits_{q\in\mathcal{N}_{p}}|G_{pq}|\Gamma\Big(sgn(G_{pq})z_{q}(t-\tau,x)\\ &-z_{p}(t-\tau,x)\Big)+u_{p}(t,x) \end{align} | (4.5) |
where \tau = 0.5 . Other parameters are the same as in Example 1. The desired reference target is
\begin{align} \frac{\partial z_{0}(t,x)}{\partial t} = &D\Delta z_{0}(t,x)-Cz_{0}(t,x)+A(z_{0}(t,x))f(z_{0}(t,x)). \end{align} | (4.6) |
Let \varepsilon_{p}(t, x) = z_{p}(t, x)-s_{p}z_{0}(t, x) , and the error system is
\begin{align} \frac{\partial \varepsilon_{p}(t,x)}{\partial t} = &D\Delta \varepsilon_{p}(t,x)-C\varepsilon_{p}(t,x)+A(z_{p}(t,x))f(z_{p}(t,x))\\ &-s_{p}A(z_{0}(t,x))f(z_{0}(t,x))\\ &+\sigma\sum^{N}_{q = 1}l_{pq}\Gamma \varepsilon_{q}(t-\tau,x)+u_{p}(t,x). \end{align} | (4.7) |
The prespecified-time controller u_{p}(t, x) is chosen as (3.25). Choose k_{i} = 2, \xi_{p} = 2 . Other parameters are the same as in Example 1. It can be checked that
\begin{align} &k_{i} > 2\sum\limits_{s = 1}^{2}a^{+}_{is}\Upsilon,\\ &-\tilde{\xi}-c_{\min}-\sum\limits_{k = 1}^{\vartheta}\frac{\pi^{2}d_{\min}}{(h^{M}_{k}-h^{m}_{k})^{2}}+\sigma^{2}\lambda_{\max}[(L\otimes\Gamma)^{2}]\leq-\frac{\phi}{2} \end{align} |
Thus, the condition in Theorem 2 holds, and the leader-following PTBS is achieved. Take initial random conditions in the interval [-3, 3] , and Figure 3 describes the synchronization error \varepsilon_{p}(t, x) between system (4.5) and (4.6) with controller (3.25). From the simulations, leader-following synchronization can be achieved in prescribed time t_{b} = 2 .
This paper investigates the prespecified-time bipartite synchronization (PTBS) of CRDMNNs with both cooperative and competitive interactions. Two types of PTBS are considered in our work: leaderless PTBS and leader-following PTBS. In addition, the coupling delays are considered in the leader-following case. By designing suitable Lyapunov functionals and novel control protocols, two criteria are derived for leaderless PTBS and leader-following PTBS based on a structural balance condition. Compared with FTS and FxTS, the settling time in our theorem can be predetermined according to the task, which is independent of the initial values and control parameters.
Our future study will focus on 1) PTBS control of neural networks under time-scale and 2) PTBS control of quaternion-valued neural networks.
This work is supported by the Natural Science Foundation of Jiangsu Province (Grant No. BK20210635).
The authors have no conflict of interest.
[1] |
D. López, H. Vlamakis, R. Kolter, Biofilms, CSH Perspect. Biol., 13 (2010), 1–11. https://doi.org/10.1101/cshperspect.a000398 doi: 10.1101/cshperspect.a000398
![]() |
[2] |
L. Hall-Stoodley, J. W. Costerton, P. Stoodley, Bacterial biofilms: Form the natural environment to infectious diseases, Nat. Rev. Microbiol., 2 (2004), 95–108. https://doi.org/10.1038/nrmicro821 doi: 10.1038/nrmicro821
![]() |
[3] |
R. M. Donlan, Biofilms: Microbial life on surfaces, Emerg. Infect. Dis., 8 (2002), 881–890. https://doi.org/10.3201/eid0809.020063 doi: 10.3201/eid0809.020063
![]() |
[4] | O. Wanner, H. Eberl, E. Morgenroth, D. R. Noguera, C. Picioreanu, B. Rittmann, et al., Math. model. biofilms, IWA Publishing, 2006. |
[5] |
M. C. Van Loosdrecht, J. J. Heijnen, H. Eberl, J. Kreft, C. Picioreanu, Mathematical modelling of biofilm structures, Antonie van Leeuwenhoek, 81 (2002), 245–256. https://doi.org/10.1023/a:1020527020464 doi: 10.1023/a:1020527020464
![]() |
[6] |
Y. H. An, R. J. Friedman, Concise review of mechanisms of bacterial adhesion to biomaterial surfaces, J. Biomed. Mater. Res., 43 (1998), 338–348. https://doi.org/10.1002/(sici)1097-4636(199823)43:3<338::aid-jbm16>3.0.co;2-b doi: 10.1002/(sici)1097-4636(199823)43:3<338::aid-jbm16>3.0.co;2-b
![]() |
[7] |
A. Dumitrache, G. Wolfaardt, G. Allen, S. N. Liss, L. R. Lynd, Form and function of Clostridium thermocellum biofilms, Appl. Environ. Microbiol., 79 (2013), 231–239. https://doi.org/10.1128/AEM.02563-12 doi: 10.1128/AEM.02563-12
![]() |
[8] |
Z. W. Wang, S. H. Lee, J. G. Elkins, J. L. Morrell-Falvey, Spatial and temporal dynamics of cellulose degradation and biofilm formation by Caldicellulosiruptor obsidianis and Clostridium thermocellum, AMB Express, 1 (2011), 1–10. https://doi.org/10.1186/2191-0855-1-30 doi: 10.1186/2191-0855-1-30
![]() |
[9] |
Y. Rohanizadegan, S. Sonner, H. J. Eberl, Discrete attachment to a cellulolytic biofilm modeled by an Itô stochastic differential equation, Math. Biosci. Eng., 17 (2020), 2236–2271. https://doi.org/10.3934/mbe.2020119 doi: 10.3934/mbe.2020119
![]() |
[10] | B. K. Øksendal, Stochastic differential equations: an introduction with applications, Springer, 2013. |
[11] |
A. Carroll, C. Somerville, Cellulosic biofuels, Annu. Rev. Plant. Biol., 60 (2009), 165–182. https://doi.org/10.1146/annurev.arplant.043008.092125 doi: 10.1146/annurev.arplant.043008.092125
![]() |
[12] | M. H. Langholtz, B. J. Stokes, L. M. Eaton, 2016 billion-ton report: Advancing domestic resources for a thirving bioeconomy, Oak Ridge National Lab., 2016. |
[13] | J. G. Linger, A. Darzins, Consolidated Bioprocessing, Springer, 2013. |
[14] |
B. G. Schuster, M. S. Chinn, Consolidated bioprocessing of lignocellulosic feedstocks for ethanol fuel production, Bioenergy Res., 6 (2012), 416–435. https://doi.org/10.1007/s12155-012-9278-z doi: 10.1007/s12155-012-9278-z
![]() |
[15] |
V. Mbaneme-Smith, M. S. Chinn, Consolidated bioprocessing for biofuel production: Recent advances, Energy Emission Control Technol., 3 (2015), 23–44. https://doi.org/10.2147/EECT.S63000 doi: 10.2147/EECT.S63000
![]() |
[16] |
H. J. Eberl, E. M. Jalbert, A. Dumitrache, G. M. Wolfaardt, A spatially explicit model of inverse colony formation of cellulolytic biofilms, Biochem. Eng. J., 122 (2017), 141–151. https://doi.org/10.1016/j.bej.2017.03.007 doi: 10.1016/j.bej.2017.03.007
![]() |
[17] | L. N. Trefethen, Finite difference and spectral methods for ordinary and partial differential equations, Cornell University Department of Computer Science and Center for Applied Mathematics, 1996. |
[18] |
B. D'Acunto, V. Luongo, M. R. Mattei, Free boundary approach to modelling multispecies biofilms, Ric. Mat., 70 (2020), 267–284. https://doi.org/10.1007/s11587-020-00523-7 doi: 10.1007/s11587-020-00523-7
![]() |
[19] |
A. Mašić, H. J. Eberl, Persistence in a single species CSTR model with suspended flocs and wall attached biofilms, Bull. Math. Biol., 74 (2012), 1001–1026. https://doi.org/10.1007/s11538-011-9707-8 doi: 10.1007/s11538-011-9707-8
![]() |
[20] |
H. J. Gaebler, H. J. Eberl, A simple model of biofilm growth in a porous medium that accounts for detachment and attachment of suspended biomass and their contribution to substrate degradation, European J. Appl. Math., 29 (2018), 1110-1140. https://doi.org/10.1017/S0956792518000189 doi: 10.1017/S0956792518000189
![]() |
[21] |
H. J. Gaebler, H. J. Eberl, Thermodynamic inhibition in chemostat models: With an application to bioreduction of uranium, Bull. Math. Biol., 82 (2020), 1–25. https://doi.org/10.1007/s11538-020-00758-3 doi: 10.1007/s11538-020-00758-3
![]() |
[22] |
H. J. Eberl, D. F. Parker, M. C. Van Loosdrecht, A new deterministic spatio-temporal continuum model for biofilm development, J. Theor. Med., 3 (2001), 161–175. https://doi.org/https://doi.org/10.1080/10273660108833072 doi: 10.1080/10273660108833072
![]() |
[23] | H. J. Eberl, L. Demaret, A finite difference scheme for a degenerated diffusion equation arising in microbial ecology, Electron. J. Differential Equations, 15 (2007), 77–95. |
[24] | J. Monod, The growth of bacterial cultures, Annu. Rev. Microbiol., 3 (1949), 371–394. |
[25] |
A. Dumitrache, H. J. Eberl, D. G. Allen, G. M. Wolfaardt, Mathematical modeling to validate on-line CO_2 measurements as a metric for cellulolytic biofilm activity in continuous-flow bioreactors, Biochem. Eng. J., 101 (2015), 55–67. https://doi.org/10.1016/j.bej.2015.04.022 doi: 10.1016/j.bej.2015.04.022
![]() |
[26] |
M. Ghasemi, H. J. Eberl, Time adaptive numerical solution of a highly degenerate diffusion-reaction biofilm model based on regularisation, J. Sci. Comput., 74 (2018), 1060–1090. https://doi.org/10.1007/s10915-017-0483-y doi: 10.1007/s10915-017-0483-y
![]() |
[27] | X. Han, P. E. Kloeden, Random ordinary differential equations and their numerical solution, 1^st edition, Springer, 2017. |
[28] | M. Lefebvre, Applied Stochastic Processes, Springer, 2007. |
[29] |
A. Dumitrache, G. M. Wolfaardt, D. G. Allen, D. G. Liss, L. R. Lynd, Tracking the cellulolytic activity of Clostridium thermocellum biofilms, Biotechnol. Biofuels, 6 (2013), 1–15. https://doi.org/10.1186/1754-6834-6-175 doi: 10.1186/1754-6834-6-175
![]() |
[30] |
M. A. Efendiev, S. V. Zelik, H. J. Eberl, Existence and longtime behavior of a biofilm model, Commun. Pure Appl. Anal., 8 (2009), 509–531. https://doi.org/10.3934/cpaa.2009.8.509 doi: 10.3934/cpaa.2009.8.509
![]() |
[31] | W. Walter Ordinary differential equations, 1^st edition, Springer, 1998. |
[32] | Y. Asai, E. Hermmann, P. E. Kloeden, Stiff integration of stiff random ordinary differential equations, Stoch. Anal. Appl., 31 (2013), 293–313. |
[33] | Y. Asai, P. E. Kloeden, Numerical schemes for random ODEs via stochastic differential equations, Commun. Appl. Anal., 17 (2013), 511–528. |
[34] |
L. Shampine, S. Thompson, J. Kierzenka, G. Byrne, Non-negative solutions of ODEs, Appl. Math. Comput., 170 (2005), 556–569. https://doi.org/10.1016/j.amc.2004.12.011 doi: 10.1016/j.amc.2004.12.011
![]() |
[35] | L. N. Trefethen, D. Bau, Numerical Linear Algebra, SIAM Society for Industrial and Applied Mathematics, 2000. |
[36] | J. Hughes, A mathematical model of discrete attachment to a cellulolytic biofilm using random DEs, M. Sc. Thesis, University of Guelph, 2021. https://hdl.handle.net/10214/26321 |
[37] |
J. Bezanson, A. Edelman, S. Karpinski, V. B. Shah, Julia: A fresh approach to numerical computing, SIAM Rev., 59 (2017), 65–98. https://doi.org/10.1137/141000671 doi: 10.1137/141000671
![]() |
[38] | R. V. Hogg, E. A. Tanis, D. L. Zimmermanm Probability and statistical inference, 9th edition, Pearson, 2020. |
[39] | W. Burger, M. Burge, Principles of digital image processing: core algorithms, Springer-Verlag, 2009. |
[40] | E. Jalbert, Comparison of a semi-implicit and a fully-implicit time integration method for a highly degenerate diffusion-reaction equations coupled with an ordinary differential equations, M. Sc. Thesis, University of Guelph, 2016. http://hdl.handle.net/10214/9448 |
[41] | K. Mitra, J. M. Hughes, S. Sonner, H. J. Eberl, J. D. Dockery, Travelling waves in a PDE-ODE coupled system with nonlinear diffusion, preprint, arXiv: 2202.07748. |
[42] | K. Eichinger, M. V. Gnann, C. Kuehn, Multiscale analysis for traveling-pulse solutions to the stochastic FitzHugh-Nagumo equations, preprint, arXiv: 2002.07234. |
[43] |
C. H. S. Hamster, H. J. Hupkes, Stability of traveling waves on exponentially long timescales in stochastic reaction-diffusion equations, SIAM J. Appl. Dyn. Syst., 19 (2020), 2469–2499. https://doi.org/10.1137/20M1323539 doi: 10.1137/20M1323539
![]() |
[44] |
C. H. S. Hamster, H. J. Hupkes, A general framework for stochastic traveling waves and patterns, with application to neural field equations, SIAM J. Appl. Dyn. Syst., 15 (2016), 195–234. https://doi.org/10.1137/15M102856X doi: 10.1137/15M102856X
![]() |
1. | Peng Liu, Ting Liu, Junwei Sun, Zhigang Zeng, Predefined-Time Synchronization of Multiple Fuzzy Recurrent Neural Networks via a New Scaling Function, 2024, 32, 1063-6706, 1527, 10.1109/TFUZZ.2023.3327682 | |
2. | Kai Wu, Ming Tang, Han Ren, Liang Zhao, Quantized pinning bipartite synchronization of fractional-order coupled reaction–diffusion neural networks with time-varying delays, 2023, 174, 09600779, 113907, 10.1016/j.chaos.2023.113907 | |
3. | Pan Wang, Xuechen Li, Qianqian Zheng, Synchronization of inertial complex-valued memristor-based neural networks with time-varying delays, 2024, 21, 1551-0018, 3319, 10.3934/mbe.2024147 | |
4. | Mengjiao Wang, Mingyu An, Shaobo He, Xinan Zhang, Herbert Ho-Ching Iu, Zhijun Li, Two-dimensional memristive hyperchaotic maps with different coupling frames and its hardware implementation, 2023, 33, 1054-1500, 10.1063/5.0154516 | |
5. | Ting Yuan, Huizhen Qu, Dong Pan, Random periodic oscillations and global mean-square exponential stability of discrete-space and discrete-time stochastic competitive neural networks with Dirichlet boundary condition, 2023, 45, 10641246, 3729, 10.3233/JIFS-230821 | |
6. | Lisha Yan, Xia Huang, Zhen Wang, Min Xiao, Bipartite synchronization of coopetitive MNNs subject to communication delays via dynamic switching event-triggered control, 2025, 0924-090X, 10.1007/s11071-025-10966-y | |
7. | Yanli Huang, Wenjing Duan, Tse Chiu Wong, 2024, Robust General Decay Synchronization of State and Spatial Diffusion Coupled Reaction-diffusion Memristive Neural Networks, 979-8-3315-0475-5, 616, 10.1109/CSIS-IAC63491.2024.10919282 |