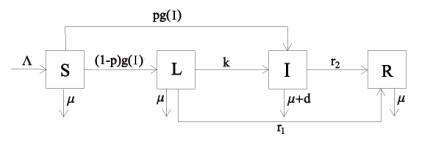
Traditional breast ultrasound relies too much on the operation skills of diagnostic doctors, and the repeatability in different doctors was low. This study aimed to evaluate the assistant diagnostic value of S-Detect artificial intelligence (AI) system in differentiating benign from malignant breast masses.
The ultrasound images of 40 patients who underwent ultrasound examination in our hospital were collected. The conventional ultrasound images, elastic images, and S-Detect mode of breast lesions were analyzed. The breast imaging reporting and data system recommended by the American Society of Radiology (BI-RADS) classification for each breast mass was evaluated both by the doctor and AI. The receiver operator characteristics (ROC) curves were drawn to compare the diagnostic efficiency.
Among the 40 lesions, 16 were benign, and 24 were malignant. The S-Detect AI system had a high diagnostic efficiency for malignant mass, with sensitivity, specificity, and accuracy of 95.8%, 93.8%, and 89.6%. The accuracy of AI was higher than the elastic image and then than the conventional gray-scale image. With the assistance of the S-Detect AI system, the accuracy of BI-RADS classification was improved significantly.
The S-Detect AI system will enhance breast cancer diagnostic accuracy and improve ultrasound examination quality.
Citation: Qun Xia, Yangmei Cheng, Jinhua Hu, Juxia Huang, Yi Yu, Hongjuan Xie, Jun Wang. Differential diagnosis of breast cancer assisted by S-Detect artificial intelligence system[J]. Mathematical Biosciences and Engineering, 2021, 18(4): 3680-3689. doi: 10.3934/mbe.2021184
[1] | Yoichi Enatsu, Yukihiko Nakata . Stability and bifurcation analysis of epidemic models with saturated incidence rates: An application to a nonmonotone incidence rate. Mathematical Biosciences and Engineering, 2014, 11(4): 785-805. doi: 10.3934/mbe.2014.11.785 |
[2] | Guo Lin, Shuxia Pan, Xiang-Ping Yan . Spreading speeds of epidemic models with nonlocal delays. Mathematical Biosciences and Engineering, 2019, 16(6): 7562-7588. doi: 10.3934/mbe.2019380 |
[3] | Thomas Torku, Abdul Khaliq, Fathalla Rihan . SEINN: A deep learning algorithm for the stochastic epidemic model. Mathematical Biosciences and Engineering, 2023, 20(9): 16330-16361. doi: 10.3934/mbe.2023729 |
[4] | Yanmei Wang, Guirong Liu . Dynamics analysis of a stochastic SIRS epidemic model with nonlinear incidence rate and transfer from infectious to susceptible. Mathematical Biosciences and Engineering, 2019, 16(5): 6047-6070. doi: 10.3934/mbe.2019303 |
[5] | Yanni Xiao, Tingting Zhao, Sanyi Tang . Dynamics of an infectious diseases with media/psychology induced non-smooth incidence. Mathematical Biosciences and Engineering, 2013, 10(2): 445-461. doi: 10.3934/mbe.2013.10.445 |
[6] | Alain Rapaport, Jérôme Harmand . Biological control of the chemostat with nonmonotonic response and different removal rates. Mathematical Biosciences and Engineering, 2008, 5(3): 539-547. doi: 10.3934/mbe.2008.5.539 |
[7] | Xinyu Bai, Shaojuan Ma . Stochastic dynamical behavior of COVID-19 model based on secondary vaccination. Mathematical Biosciences and Engineering, 2023, 20(2): 2980-2997. doi: 10.3934/mbe.2023141 |
[8] | Tingting Xue, Long Zhang, Xiaolin Fan . Dynamic modeling and analysis of Hepatitis B epidemic with general incidence. Mathematical Biosciences and Engineering, 2023, 20(6): 10883-10908. doi: 10.3934/mbe.2023483 |
[9] | Xunyang Wang, Canyun Huang, Yixin Hao, Qihong Shi . A stochastic mathematical model of two different infectious epidemic under vertical transmission. Mathematical Biosciences and Engineering, 2022, 19(3): 2179-2192. doi: 10.3934/mbe.2022101 |
[10] | Arturo J. Nic-May, Eric J. Avila-Vales . Global dynamics of a two-strain flu model with a single vaccination and general incidence rate. Mathematical Biosciences and Engineering, 2020, 17(6): 7862-7891. doi: 10.3934/mbe.2020400 |
Traditional breast ultrasound relies too much on the operation skills of diagnostic doctors, and the repeatability in different doctors was low. This study aimed to evaluate the assistant diagnostic value of S-Detect artificial intelligence (AI) system in differentiating benign from malignant breast masses.
The ultrasound images of 40 patients who underwent ultrasound examination in our hospital were collected. The conventional ultrasound images, elastic images, and S-Detect mode of breast lesions were analyzed. The breast imaging reporting and data system recommended by the American Society of Radiology (BI-RADS) classification for each breast mass was evaluated both by the doctor and AI. The receiver operator characteristics (ROC) curves were drawn to compare the diagnostic efficiency.
Among the 40 lesions, 16 were benign, and 24 were malignant. The S-Detect AI system had a high diagnostic efficiency for malignant mass, with sensitivity, specificity, and accuracy of 95.8%, 93.8%, and 89.6%. The accuracy of AI was higher than the elastic image and then than the conventional gray-scale image. With the assistance of the S-Detect AI system, the accuracy of BI-RADS classification was improved significantly.
The S-Detect AI system will enhance breast cancer diagnostic accuracy and improve ultrasound examination quality.
The incidence of a disease is the number of individuals who become infected per unit of time and plays an important role in the study of mathematical epidemiology. Generally, incidence rate depends on both the susceptible and infective classes. In many epidemic models, the bilinear incidence rate is frequently used[1]. However, in recent years, researchers have taken into account oscillations in incidence rates and proposed many nonlinear incidence rates. Let S(t) be the number of susceptible individuals, I(t) be the number of infective individuals, and R(t) be the number of removed individuals at time t, respectively. After studying the cholera epidemic spread in Bari in 1973, Capasso and Serio [2] introduced the saturated incidence g(I)S into epidemic models, where g(I) is decreasing when I is large enough, i.e.
g(I)=kI1+αI | (1.1) |
This incidence rate seems more reasonable than the bilinear incidence rate because it includes the behavioral change and crowding effect of the infective individuals and prevents the unboundedness of the contact rate by choosing suitable parameters.
To incorporate the effect of the behavioral changes of the susceptible individuals, Liu et al.[3] proposed the general incidence rate
g(I)=kIp1+αIq | (1.2) |
where p and q are positive constants and α is a nonnegative constant. The special cases when p,q and k take different values have been used by many authors. For example, Ruan and Wang [4] studied the case when p=2,q=2 i.e. g(I)=kI21+αI2 and got some complicated dynamics phenomenons, such as saddle-node bifurcation, Hopf bifurcation, Bogdanov-Takens bifurcation and the existence of none, one and two limit cycles. Derrick and van den Driessche[5], Hethcote [6], Alexander and Moghadas[7], etc. also used the general incidence rate.
If the function g(I) is nonmonotone, that is, g(I) is increasing when I is small and decreasing when I is large, it can be used to interpret the 'psychological' effect: for a very large number of infective individuals the infection force may decrease as the number of infective individuals increases, because in the presence of large number of infectives the population may tend to reduce the number of contacts per unit time[8]. To model this phenomenon, Xiao and Ruan[8] proposed a incidence rate
g(I)=kI1+αI2 | (1.3) |
where kI measures the infection force of the disease and 1/(1+αI2) describes the psychological or inhibitory effect from the behavioral change of the susceptible individuals when the number of infective individuals is very large. This is important because the number of effective contacts between infective individuals and susceptible individuals decreases at high infective levels due to the crowding of infective individuals or due to the protection measures by the susceptible individuals. Notice that when α=0, the nonmonotone incidence rate (1.3) becomes the bilinear incidence rate[8]. They used this incidence rate in an SIR model and got the result that this model does not exhibit complicated dynamics as other epidemic models with other types of incidence rates reported in. In this paper, we use this incidence rate in an SLIR model.
In fact, epidemic models are inevitably affected by environmental white noise which is an important component in realism, because it can provide an additional degree of realism in compared to their deterministic counterparts. Therefore, many stochastic models for the epidemic populations have been developed. In addition, both from a biological and from a mathematical perspective, there are different possible approaches to include random effects in the model. Here, we mainly mention three approaches. The first one is through time Markov chain model to consider environment noise in HIV epidemic (see, e.g., [9,10,11,12]). The second is with parameters perturbation. There is an intensive literature on this area, such as [13,14,15,16,17,18,19]. The last important issue to model stochastic epidemic system is to robust the positive equilibria of deterministic model. In this situation, it is mainly to investigate whether the stochastic system preserves the asymptotic stability properties of the positive equilibria of deterministic models, see [20,21,22]. Recently, Yang et.al[19] discusses the stochastic SIR and SEIR epidemic models with saturated incidence rate βS/(1+αI). In this paper, we introduce randomness in the SLIR model with the second approaches as [13] and [15].
The rest of the paper is organized as follows: in Section 2, the deterministic SLIR mathematical model is formulated, boundedness of solutions and existence of a positively invariant and attracting set are shown. In Section 3, the basic reproductive number, the conditions to the existence of possible equilibria of the system and their stability are established. In section 4, we obtain the analytic results of dynamics of the SDE model. Finally, a brief discussion is given in Section 5.
In this section, we formulate an SLIR model with incidence rate (1.3). Figure 1 shows the model diagram. The total population at time t, denoted by N(t), is divided into four classes: susceptible (S), latent (L), infectious (I) and treatment (R).
All recruitment is into the susceptible class, and occurs at a constant rate Λ. We assume that an individual may be infected only through contacts with infectious individuals. The natural death rate is μ. The infectious class has an additional death rate due to the disease with rate constant d. Thus individuals leave class L for class I at rate k. Latent and Infectious individuals are treated with rate constant r1 and r2, entering the treatment class, respectively. A fraction p of newly infected individuals moves to the latent class (L), and the remaining fraction 1−p, moves to the active class (I). The incidence rate is g(I)=βI1+αI2. It is assumed that individuals in the latent class do not transmit infection. Combining all the aforementioned assumptions, the model is given by the following system of differential equations:
dSdt=Λ−βSI1+αI2−μS,dLdt=(1−p)βSI1+αI2−(μ+k+r1)L,dIdt=pβSI1+αI2+kL−(μ+d+r2)I,dRdt=r1L+r2I−μR. | (2.1) |
By adding all Eq (2.1), the dynamics of the total population N(t) is given by:
dN/dt=Λ−μN−dI. | (2.2) |
Since dN/dt<0 for N>Λ/μ, then, without loss of generality, we can consider only solutions of (2.1) in the following positively subset of R4:
Ωε={(S,L,I,R)|S,L,I,R≥0,S+L+I+R≤Λμ}. |
With respect to model system (2.1), we have the following result:
Proposition 2.1. The compact set Ωε is a positively invariant and absorbing set that attracts all solutions of Eq (2.1) in R4.
Proof. Define a Lyapunov function as W(t)=S(t)+L(t)+I(t)+R(t), then we have:
dW(t)dt=Λ−μW−dI≤Λ−μW. | (2.3) |
Hence, that dWdt≤0 for W>Λμ. Ωε is a positively invariant set. On the other hand, solving the differential inequality Eq (2.3) yields:
0<W(t)<Λμ+W(0)e−μt. |
W(0) is the initial condition of W(t). Thus, as t→∞, one has that 0≤W(t)≤Λμ.
To analysis the system (2.1), we notice that the variable R does not participate in the first three equations. Thus we can consider only the equations for S, L and I, i.e. the following system:
{dSdt=Λ−βSI1+αI2−μS,dLdt=(1−p)βSI1+αI2−(μ+k+r1)L,dIdt=pβSI1+αI2+kL−(μ+d+r2)I. | (2.4) |
In this section, the model is analyzed in order to obtain the basic reproduction number, conditions for the existence and uniqueness of non-trivial equilibria and asymptotic stability of equilibria.
In this subsection, we define the basic reproduction number R0 of system (2.4). R0 is the average number of secondary infections that occur when one infective is introduced into a completely susceptible population[23]. For many deterministic epidemiology models, an infection can get started in a fully susceptible population if and only if R0>1. Thus the basic reproductive number R0 is often considered as the threshold quantity that determines when an infection can invade and persist in a new host population[1].
The disease-free equilibrium of system (2.4) is X0=(S0,0,0) with S0=Λ/μ. In order to compute the basic reproduction number, it is important to distinguish new infections from all other class transitions in the population. The infected classes are L and I. Following Van den Driessche and Watmough[24], we can rewrite system (2.4) as
˙x=f(x)=F(x)−V(x)=F(x)−(V−(x)−V+(x)). |
where x=(L,I,S), F is the rate of appearance of new infections in each class, V+ is the rate of transfer into each class by all other means and V− is the rate of transfer out of each class. Hence,
F(x)=((1−p)βSI1+αI2,pβSI1+αI2,0)T |
and
V(x)=((μ+k+r1)L−kL+(μ+d+r2)IμS+βSI1+αI2−Λ). |
The jacobian matrices of F and V at the disease-free equilibrium X0=(0,0,Λ/μ) can be partitioned as
DF(X0)=(F000) and DV(X0)=(V0J1J2). |
where F and V correspond to the derivatives of F and V with respect to the infected classes:
F=(0(1−p)βS00pβS0) and V=(μ+k+r10−kμ+d+r2). |
The basic reproduction number is defined, following Van den Driessche and Watmough [24], as the spectral radius of the next generation matrix, FV−1:
R0=βΛμk(1−p)+(μ+k+r1)p(μ+k+r1)(μ+d+r2). |
We have the following result about the global stability of the disease free equilibrium:
Theorem 3.1. When R0>1, the disease free equilibrium X0 is unstable.When R0≤1, the disease free equilibrium X0 is globally asymptotically stable in Ωε; this implies the global asymptotic stability of the disease free equilibrium on the nonnegative orthant R3. This means that the disease naturally dies out.
Proof. The Jacobian matrix of system (2.4) at X0 is
J(X0)=(−μ0−βS00−(μ+k+r1)(1−p)βS00k−(μ+d+r2)+pβS0) |
and the characteristic equation is
(λ+μ)[λ2+(μ+k+r1+μ+d+r2−pβS0)λ+(μ+k+r1)(μ+d+r2)(1−R0)]=(λ+μ)f(λ)=0. | (3.1) |
From (3.1), we get the discriminant of f(λ) is
Δ1=(μ+k+r1+μ+d+r2−pβS0)2−4(μ+k+r1)(μ+d+r2)(1−R0)=(μ+k+r1−μ−d−r2+pβS0)2+4k(1−p)βS0>0. |
Therefore, (3.1) has three real roots.
If R0<1, we have
βS0<(μ+k+r1)(μ+d+r2)k(1−p)+(μ+k+r1)p, |
then
μ+k+r1+μ+d+r2−pβS0>μ+k+r1+μ+d+r2−p(μ+k+r1)(μ+d+r2)k(1−p)+(μ+k+r1)p=μ+k+r1+k(1−p)(μ+d+r2)k(1−p)+(μ+k+r1)p>0. |
(3.1) has three negative real roots and hence X0 is locally stable.
If R0>1, (3.1) has at least one positive real root and hence X0 is unstable.
When R0≤1, we can define the following Lyapunov-LaSalle function
V(t)=kk(1−p)+(μ+k+r1)pL+μ+k+r1k(1−p)+(μ+k+r1)pI. |
Its time derivative along the trajectories of system (2.4) satisfies
˙V=kk(1−p)+(μ+k+r1)p˙L+μ+k+r1k(1−p)+(μ+k+r1)p˙I=βSI1+αI2−(μ+k+r1)(μ+d+r2)k(1−p)+(μ+k+r1)pI=βI(11+αI2S−S0R0)≤βI(S−S0R0) |
Thus R0≤1 implies that ˙V≤0. By LaSalle's invariance principle, the largest invariant set in Ωε contained in {(S,L,I,R)∈Ωε,˙V=0} is reduced to the disease-free equilibrium X0. This proves the global asymptotic stability of the disease-free equilibrium on Ωε [25]. Since Ωε is absorbing, this proves the global asymptotic stability on the nonnegative octant for R0≤1. It should be stressed that we need to consider a positively invariant compact set to establish the stability of X0 since V is not positive definite. Generally, the LaSalle's invariance principle only proves the attractivity of the equilibrium. Considering Ωε permits to conclude for the stability[25,26,27]. This achieves the proof.
To find the positive equilibrium, let
Λ−βSI1+αI2−μS=0, |
(1−p)βSI1+αI2−(μ+k+r1)L=0, |
pβSI1+αI2+kL−(μ+d+r2)I=0, |
which yields
μαI2+βI+μ(1−R0)=0 |
We can see that
(ⅰ) there is one positive equilibrium if R0>1;
(ⅱ) there is no positive equilibrium if R0≤1.
Then we have the following result:
Theorem 3.2. When R0>1, there exist an unique endemic equilibrium X∗=(S∗,L∗,I∗) for the system (2.4) where S∗,L∗, and I∗ are defined as in (3.2) which is in the nonnegative octant R3+.
S∗=1μ[Λ−(μ+k+r1)(μ+d+r2)k(1−p)+(μ+k+r1)pI∗],L∗=(μ+d+r2)(1−p)k(1−p)+(μ+k+r1)pI∗,I∗=−β+√β2+4μ2α(R0−1)2μα. | (3.2) |
In this section, we denote μ+k+r1=A,μ+d+r2=B for writing convenience.
Theorem 3.3. If R0>1, the unique endemic equilibrium X∗ of system (2.4) is locally asymptotically stable.
Proof. The Jacobian matrix of system (2.4) at endemic equilibrium X∗=(S∗,L∗,I∗) is
J(X∗)=(−μ−g(I∗)0−g′(I∗)S∗(1−p)g(I∗)−A(1−p)g′(I∗)S∗pg(I∗)k−B+pg′(I∗)S∗)). | (3.3) |
where
g(I∗)=βI∗1+αI∗2, g′(I∗)=β(1−αI∗2)(1+αI∗2)2=g(I∗)I∗−2βαI∗2(1+αI∗2)2<g(I∗)I∗. |
The characteristic equation of J(X∗) is
λ3+aλ2+bλ+c=0. |
where
a=μ+g(I∗)+A+B−pg′(I∗)S∗>μ+g(I∗)+A+B−pg(I∗)S∗I∗=μ+g(I∗)+A+B−B+kL∗I∗>0,b=(μ+g(I∗))A+(μ+g(I∗)+A)(B−pg′(I∗)S∗)+pg(I∗)g′(I∗)S∗−k(1−p)g′(I∗)S∗,c=(μ+g(I∗))A(B−pg′(I∗)S∗)+pg(I∗)g′(I∗)S∗A−μk(1−p)g′(I∗)S∗+k(1−p)g(I∗)g′(I∗)S∗=μAB−μApg′(I∗)S∗+ABg(I∗)−μk(1−p)g′(I∗)S∗+k(1−p)g(I∗)g′(I∗)S∗>μAB−μA(B−kL∗I∗)+ABg(I∗)−μkAL∗I∗+k(1−p)g(I∗)g′(I∗)S∗=ABg(I∗)+k(1−p)g(I∗)g′(I∗)S∗>0 |
ab−c=[μ+g(I∗)+A+B−pg′(I∗)S∗][(μ+g(I∗))A+(μ+g(I∗)(B−pg′(I∗)S∗)+pg(I∗)g′(I∗)S∗+A(B−pg′(I∗)S∗)−k(1−p)g′(I∗)S∗]−(μ+g(I∗))A(B−pg′(I∗)S∗)−k(1−p)g(I∗)g′(I∗)S∗−pg(I∗)g′(I∗)S∗(A+μk(1−p)g′(I∗)S∗≥(μ+g(I∗)+A)(μ+g(I∗))A+(μ+g(I∗)+A)(μ+g(I∗))(B−pg′(I∗)S∗)+(μ+g(I∗))pg(I∗)g′(I∗)S∗+(μ+g(I∗))(B−pg′(I∗)S∗)2+μk(1−p)g′(I∗)S∗+pg(I∗)g′(I∗)S∗(B−pg′(I∗)S∗)−k(1−p)g(I∗)g′(I∗)S∗≥(μ+g(I∗)+A)(μ+g(I∗))A+(μ+g(I∗))2(B−pg′(I∗)S∗)+Aμ(B−pg′(I∗)S∗)+(μ+g(I∗))pg(I∗)g′(I∗)S∗+(μ+g(I∗))(B−pg′(I∗)S∗)2+μk(1−p)g′(I∗)S∗+pg(I∗)g′(I∗)S∗(B−pg′(I∗)S∗)>0. |
According to direct calculation we have a,c>0 and ab>c when R0>1. Therefore the endemic equilibrium X∗ is locally asymptotically stable in Ωε by Routh-Hurwitz criterion.
In this section, we briefly outline a general mathematical framework developed in [28] for proving global stability, which will be used to prove Theorem 3.10. Consider the autonomous dynamical system
x′=f(x) | (3.4) |
where f:D→Rn open set and f∈C1(D). Let ˉx be an equilibrium of (3.3), i.e. f(ˉx)=0. We recall that ˉx is said to be globally stable in D if it is locally stable and all trajectories in D converge to ˉx.
Assume that the following hypothesis hold:
(H1) D is simply connected;
(H2) There exists a compact absorbing set Γ⊂D;
(H3) Eq (3.4) has a unique equilibrium ˉx in D.
The Global Stability Problem arising in [28] is to find conditions under which the global stability of ¯x with respect to D is implied by its local stability. In [28], the main idea of this geometric approach to the global stability problem is as follows: Assume that (3.4) satisfies a condition in D, which precludes the existence of periodic solutions and suppose that this condition is robust, in the sense that it is also satisfied by ordinary differential equations that are C1-close to (3.4); Then every nonwandering point of (3.4) is an equilibrium, as otherwise, by the C1 closing lemma of Pugh [29,30], we can perturb (3.4) near such a nonequilibrium nonwandering point to get a periodic solution. As a special case, every omega limit point of (3.4) is an equilibrium. This implies that ¯x attracts points in D. As a consequence, its global stability is implied by the local stability. For this purpose, we introduce the notion of robustness of a Bendixson criterion under local C1 perturbations of f.
Definition 3.4. A point x0∈D is wandering for (3.4) if there exists a neighborhood U of x0 and t∗>0 such that U∩x(t,U) is empty for all t>t∗.
Thus, for example, any equilibrium, alpha limit point, or omega limit point is nonwandering.
Definition 3.5. A function g∈C1(D→Rn) is called a C1 local ϵ-perturbation of f at x0∈D if there exists an open neighborhood U of x0 in D such that the support supp(f−g)⊂U and |f−g|C1<ϵ, where
|f−g|C1=sup{|f(x)−g(x)|+|∂f∂x(x)−∂g∂x(x)|:x∈D}. |
and |⋅| denotes a vector norm on Rn and the operator norm that it induces for linear mappings from Rn to Rn.
Definition 3.6. A Bendixson Criterion for (3.4) is a condition satisfied by f, which precludes the existence of nonconstant periodic solutions to (3.4).
Definition 3.7. A Bendixson criterion is said to be robust under C1 local perturbations of f at x0 if, for each sufficiently small ϵ>0 and neighborhood U of x0, it is also satisfied by each C1 local ϵ-perturbations g such that supp(f−g)⊂U.
The following is the local version of the global-stability principle proved by [28].
Theorem 3.8. Suppose that assumptions (H2) and (H3) hold and that (3.4) satisfies a Bendixson criterion that is robust under C1 local perturbations of f at all nonequilibrium nonwandering points for (3.4), then ˉx is globally asymptotically stable with respect to D, provided it is stable.
The following is to introduce the quantity ˉq2 given in [28]. Assume that (3.4) has a compact absorbing set K⊂D. Then every solution x(t,x0) of (3.4) exists for any t>0. The quantity ˉq2 is well defined:
ˉq2=lim supt→∞supx0∈K1t∫t0ρ(B(x(s,x0)))ds, | (3.5) |
where
B=PfP−1+P∂f[2]∂xP−1, | (3.6) |
and x↦P(x) is a (n2)×(n2) matrix-valued function, which is C1 in D and Af=(DP)(f) or, equivalently, Pf is the matrix obtained by replacing each entry aij in P by its directional derivative in the direction of f, ∂f∂x[2] is the second additive compound matrix of the Jacobian matrix ∂f∂x of f. If |⋅| is a vector norm on R(n2), then ρ(B) is the Lozinskˇi measure with respect to |⋅|, which is defined by
ρ(B)=limh→0+|I+hB|−1h. |
Under assumptions (H1) and (H2), [28] proved that ˉq2<0 is a Bendixson criterion for (3.3) and it is robust under C1 local perturbations of f at all nonequilibrium nonwandering points for (3.3) by means of the local version of C1 closing lemma of Pugh [29,30]. Then we have the following theorem
Theorem 3.9. Under assumptions (H1), (H2), and (H3), the unique equilibrium ˉx is globally asymptotically stable in D if ˉq2<0.
The criterion ˉq2<0 provides the flexibility of a choice of (n2)×(n2) arbitrary function in addition to the choice of vector norms |⋅| in deriving suitable conditions. It has been remarked in [28] that, under the assumptions of Theorem 3.9, the classical result of Lyapunov, the classical Bendixson-Dulac condition, and the criterion in [31] are obtained as special cases [28]. In addition, it has also been stated in [28] that, under the assumptions of Theorem 3.9 the condition ˉq2<0 also implies the local stability of the equilibrium ˉx, because, assuming the contrary, ˉx is both the alpha and the omega limit point of a homoclinic orbit that is ruled out by the condition ˉq2<0.
Global stability of endemic equilibrium.
We will focus on investigating the globally asymptotical stability of the unique endemic equilibrium X∗(S∗,L∗,I∗). The main theorem of the method requires the use of Lozinskˇi Logarithmic norm.
Theorem 3.10. Assume that R0>1. Then the unique endemic equilibrium X∗ is globally asymptotically stable in R+3.
Proof. We set P as the following diagonal matrix:
P(S,L,I)=diag(1,LI,SI). |
Then P is C1 and nonsingular in ∘Ωε. Let f denote the vector field of (2.4). Then
PfP−1=diag(0,L′L−I′I,S′S−I′I), |
the second compound matrix J[2] of the Jacobian matrix of system (2.4) can be calculated as follows
J[2]=(−A−μ−g(I)(1−p)g′(I)Sg′(I)Sk−μ−g(I)−B+pg′(I)S0−pg(I)(1−p)g(I)−A−B+pg′(I)S). |
Then the matrix B=PfP−1+PJ[2]P−1 in (3.6) can be written in matrix form
B=(B11B12B21B22). |
where
B11=−A−μ−g(I),B12=(IL⋅(1−p)g′(I)S,g′(I)I),B21=(k⋅LI,−pg(I)⋅SI)T,B22=(−μ−g(I)−B+pg′(I)S+L′L−I′I0(1−p)g(I)SL−A−B+pg′(I)S) |
Let (u,v,w) denote the vectors in R3, we select a norm in R3 as |(u,v,w)|=max{|u|,|v|+|w|} and let ˜μ denote the Lozinskˇi measure with respect to this norm. Following the method in [32], we have the estimate ˜μ(B)≤sup{g1,g2}, where
g1=˜μ1(B11)+|B12|, and g2=|B21|+˜μ(B22) |
|B12| and |B21| are matrix norms with respect to the l1 vector norm and ˜μ1 denotes the Lozinskˇi measure with respect to the l1 norm. So
˜μ1(B11)=−A−μ−g(I),|B12|=max{IL⋅(1−p)g′(I)S,g′(I)I},|B21|=max{k⋅LI,pg(I)⋅SI}=kLI |
To calculate ˜μ(B22) we add the absolute value of the off-diagonal elements to the diagonal one in each column of B22, and then take the maximum of two sums, see [33]. We thus obtain,
˜μ(B22)=−B+pg′(I)S−I′I+max{−μ−g(I)+L′L,−A+S′S+(1−p)g(I)SL}=−B+pg′(I)S−I′I+L′L+S′S≤−(μ+d+r2)+pg(I)SI−I′I+L′L+S′S=L′L+S′S−kLI. |
Therefore
g1=−A−μ−g(I)+max{IL⋅(1−p)g′(I)S,g′(I)I}, | (3.7) |
g2≤kLI+L′L+S′S−kLI=L′L+S′S. | (3.8) |
From (2.4) we have
−A−μ−g(I)+IL⋅(1−p)g′(I)S≤−A−μ−g(I)+IL⋅(1−p)g(I)IS=−A−μ−g(I)+L′L+A=L′L−μ−g(I),−A−μ−g(I)+g′(I)I≤−A−μ−g(I)+g(I)=−A−μ. | (3.9) |
The uniform persistence constant c can be adjusted so that there exists T>0 independent of (S(0),L(0),I(0))∈K, the compact absorbing set, such that
I(t)>c, and L(t)>c for t>T. | (3.10) |
Substituting (3.9) into (3.7) and using (3.10), we obtain, for t>T,
g1≤L′L−μ, | (3.11) |
Therefore ˜μ(B)≤L′L+S′S for t>T by (3.8) and (3.11). Along each solution (S(t),L(t),I(t)) to (2.4) such that (S(0),L(0),I(0))∈K and for t>T, we have
1t∫t0˜μ(B)ds≤1t∫T0˜μ(B)ds+1tlogL(t)L(T)+1tlogS(t)S(T), |
which implies ˉq2≤0 from (3.5), proving Theorem 3.10.
In this section we proposed a nonmonotone and nonlinear incidence rate of the form βIS/(1+αI2), which is increasing when I is small and decreasing when I is large. It can be used to interpret the 'psychological' effect: the number of effective contacts between infective individuals and susceptible individuals decreases at high infective levels due to the quarantine of infective individuals or the protection measures by the susceptible individuals.
Recall that the parameter α describes the psychological effect of the general public toward the infectives. Though the basic reproduction number R0 does not depend on α explicitly, numerical simulations indicate that when the disease is endemic, the steady state value I∗ of the infectives decreases as α increases (see Figure 2). From the steady state expression (3.2) we can see that I∗ approaches zero as α tends to infinity.
In this section we consider the stochastic model associated with the deterministic model in (2.1). We introduce randomness into the model (2.1) by replacing the parameters μ by μ→μ+σidBi(t)(i=1,2,3,4) with the second approaches as [13] and [15], where Bi(t)(i=1,2,3,4) are independent standard Brownian motions with Bi(0)=0 and σ2i(i=1,2,3,4) denote the intensities of the white noise. Then equation (2.1) becomes
{dS=[Λ−βSI1+αI2−μS]dt+σ1SdB1(t),dL=[(1−p)βSI1+αI2−(μ+k+r1)L]dt+σ2LdB2(t),dI=[pβSI1+αI2+kL−(μ+d+r2)I]dt+σ3IdB3(t),dR=(r1L+r2I−μR)dt+σ4RdB4(t). | (4.1) |
Throughout this section, unless otherwise specified, let (Ω,F,P) be a complete probability space with a filtration {Ft}t≥0. The Brown motions are defined on the complete probability space (Ω,F,P). Denote
Rn+={x∈Rn:xi>0, for all 1≤i≤n}. |
In general, consider n-dimensional stochastic differential equation[35]:
dx(t)=ˉf(x(t),t)dt+ˉg(x(t),t)dB(t), on t≥t0 | (4.2) |
with initial value x(t0)=x0∈Rn. B(t) denotes n-dimensional standard Brownian motions defined on the above probability space. Define the differential operator L associated with (4.2) by
L=∂∂t+n∑i=1ˉfi(x,t)∂∂xi+12n∑i,j=1[ˉgT(x,t)ˉg(x,t)]ij∂2∂xi∂xj. |
If L acts on a function V∈C2,1(Sh×ˉR+;ˉR+), then
LV(x,t)=Vt(x,t)+Vx(x,t)ˉf(x,t)+12trace[ˉgT(x,t)Vxx(x,t)ˉg(x,t)], |
where
Vt=∂V∂t,Vx=(∂V∂x1,⋯,∂V∂xn), and Vxx=(∂2V∂xi∂xj)n×n. |
By Itô's formula,
dV(x(t),t)=LV(x(t),t)dt+Vx(x(t),t)ˉg(x(t),t)dB(t). |
In this subsection we first show the solution of system (4.1) is global and positive. In order for a stochastic differential equation to have a unique global (i.e. no explosion in a finite time) solution for any given initial value, the coefficients of the equation are generally required to satisfy the linear growth condition and local Lipschitz condition [34,35]. However, the coefficients of system (4.1) do not satisfy the linear growth condition (as the incidence is nonlinear), so the solution of system (4.1) may explode at a finite time [34,35]. In this section, using Lyapunov analysis method (mentioned in [36]), we show the solution of system (4.1) is positive and global.
Theorem 4.1. There is a unique solution (S(t),L(t),I(t),R(t)) of system (4.1) on t≥0 for any intial value (S(0),L(0),I(0),R(0))∈R4+, and the solution will remain in R4+ with probability 1, namely, (S(t),L(t),I(t),R(t))∈R4+ for all t>0 almost surely.
Proof. Since the coefficients of system (4.1) satisfy the local Lipschitz condition, then for any initial value (S(0),L(0),I(0),R(0))∈R4+, there is a unique local solution (S(t),L(t),I(t),R(t)) on [0,τe), where τe is the explosion time. To show this solution is global, we only need to prove that τe=∞ a.s. To this end, let n0>0 be sufficiently large for every component of S(0),L(0),I(0),R(0) lying within the interval [1n0,n0]×[1n0,n0]×[1n0,n0]×[1n0,n0]. For each integer n>n0, define the following stopping time
τn=inf{t∈[0,τe):min{S(t),L(t),I(t),R(t)}≤1n or max{S(t),L(t),I(t),R(t)}≥n} |
where throughout this paper, we set inf∅=∞(∅ represents the empty set). Obviously, τn is increasing as n→∞. Let τ∞=lim supn→∞τn, then τ∞≤τe a.s. In the following, we need to verify τ∞=∞ a.s. If this assertion is violated, there is a constant K>0 and an ε∈(0,1) sucn that P{τ∞≤K}>ε. As a consequence, there exists an integer n1≥n0 such that
P{τn≤K}≥ε, n≥n1. |
Define a C2-function V:R4+→R+ by
V(S,L,I,R)=(S−1−logS)+(L−1−logL)+(I−1−logI)+(R−1−logR). |
Applying the Itô's formula, we obtain
dV(S,L,I,R)=LVdt+σ1(S−1)dB1+σ2(L−1)dB2+σ3(I−1)dB3+σ4(R−1)dB4. |
where
LV=(1−1S)(Λ−βSI1+αI2−μS)+(1−1L)((1−p)βSI1+αI2−(μ+k+r1)L)+(1−1I)×(pβSI1+αI2+kL−(μ+d+r2)I)+(1−1R)(r1L+r2I−μR)+σ21+σ22+σ23+σ242=Λ+4μ+k+r1+d+r2−μ(S+L+I+R)−dI−ΛS+βI1+αI2−kLI−(1−p)βSIL(1+αI2)−pβS1+αI2−r1LR−r2IR+σ21+σ22+σ23+σ242≤Λ+4μ+k+r1+d+r2+βI1+αI2+σ21+σ22+σ23+σ242:=M. |
For ∀I>0,I1+αI2≤12√α. Hence there exists a suitable constant M>0 independent of S,L,I,T and t such that LV≤M. The remainder of the proof is similar to Theorem 3.1 of [36] and hence is omitted. This completes the proof.
Obviously, X0=(Λμ,0,0,0) is the solution of system (2.1), which is called the disease-free equilibrium. If R0<1, then X0 is globally asymptotically stable, which means the disease will vanish after some period of time. Therefore, it is interesting to study the disease-free equilibrium for controlling infectious disease. But, there is no disease-free equilibrium in system (4.1). It is natural to ask how we can consider the disease will extinct. In this subsection we mainly estimate the average of oscillation around X0 in time to exhibit whether the disease will die out.
Theorem 4.2. Let (S(t),L(t),I(t),R(t)) be the solution of system (4.1) with initial value (S(0),L(0),I(0),R(0))∈R4+. If R0=βΛμk(1−p)+(μ+k+r1)p(μ+k+r1)(μ+d+r2)≤1, and
((2μ+k+r1)22μ(μ+k+r1)+r2k(2μ+d+r2)22(2r2k2+μr21)(μ+d+r2)+μr2k+12r2k2+μr21)σ21<μ+μ2r2k2(2r2k2+μr21), |
σ22<μ+r1, σ23<dμ, σ24<μ, |
then
lim supt→∞1tE∫t0{[μ+μ2r2k2(2r2k2+μr21)−((2μ+k+r1)22μ(μ+k+r1)+r2k(2μ+d+r2)22(2r2k2+μr21)(μ+d+r2)+μr2k+12r2k2+μr21)σ21]⋅ (S−Λμ)2+μ+r1−σ222L2+μr2k(d−μσ23)2(2r2k2+μr21)I2+μ2k(μ−σ24)4(2r2k2+μr21)R2}dr≤(2+μ2r2k+μr212r2k2+μr21)Λ2σ21μ2. |
Proof. Define C2-function V1,V2:R+→R+ and V3,V4,V5:R2+→R+, respectively by
V1(S)=(S−Λμ)22, V2(R)=R22, V3(L,I)=L+μ+r1+kkI, |
V4(S,L)=(S−Λμ+L)22, V5(S,I)=(S−Λμ+I)22. |
Along the trajectories of system (4.1), we have
LV1=(S−Λμ)(Λ−βSI1+αI2−μS)+σ21S22=−μ(S−Λμ)2−β(S−Λμ)2I1+αI2−βΛ(S−Λμ)Iμ(1+αI2)+σ21S22LV2=r1LR+r2IR−μR2+σ24R22≤r22I2μ−(μ−σ24)R22+r21L2μ,LV3=(1−p)βSI1+αI2+μ+k+r1kpβSI1+αI2−μ+k+r1k(μ+d+r2)I=[k(1−p)+(μ+k+r1)p]β(S−Λμ)Ik(1+αI2)+(μ+k+r1)(μ+d+r2)Ik(R01+αI2−1),LV4≤−μ(S−Λμ)2−pβΛ(S−Λμ)Iμ(1+αI2)+(2μ+k+r1)22(μ+k+r1)(S−Λμ)2−(μ+k+r1)L22+σ21S22+σ22L22, |
LV5≤−μ(S−Λμ)2−(1−p)β(S−Λμ)2I1+αI2−(1−p)βΛ(S−Λμ)Iμ(1+αI2)+kL(S−Λμ)−(2μ+d+r2)(S−Λμ)I+kLI−(μ+d+r2)I2+σ21S22+σ23I22 |
Hence
LV1+Λk[k(1−p)+(μ+k+r1)p]μLV3≤−μ(S−Λμ)2−β(S−Λμ)2I1+αI2+σ21S22≤−(μ−σ21)(S−Λμ)2+σ21Λ2μ2 |
LV4+pΛk[k(1−p)+(μ+k+r1)p]μLV3≤−(μ−(2μ+k+r1)22(μ+k+r1))(S−Λμ)2+σ21S22+σ22L22≤((2μ+k+r1)22(μ+k+r1)−μ+σ21)(S−Λμ)2+σ21Λ2μ2+σ22L22−(μ+k+r1)L22 | (4.3) |
LV5+(1−p)Λk[k(1−p)+(μ+k+r1)p]μLV3+μ2r2LV2≤−μ(S−Λμ)2+kL(S−Λμ)−(2μ+d+r2)(S−Λμ)I+kLI−(μ+d+r2)I2+σ21S22+σ23I22+r22I2+r212r2L2−μ(μ−σ24)4r2R2≤−μ(S−Λμ)2+k2μL2+μ2(S−Λμ)2+(2μ+d+r2)22(μ+d+r2)(S−Λμ)2−d+r22I2+σ21S22+σ23I22+r22I2+r212r2L2−μ(μ−σ24)4r2R2≤((2μ+d+r2)22(μ+d+r2)−μ2)(S−Λμ)2+(k2μ+r212r2)L2+−d2I2+σ21S22+σ23I22−μ(μ−σ24)4r2R2 | (4.4) |
From (4.3) and (4.4) we have
LV4+pΛk[k(1−p)+(μ+k+r1)p]μLV3+μr2k2r2k2+μr21[LV5+(1−p)Λk[k(1−p)+(μ+k+r1)p]μLV3+μ2r2LV2]≤−(μ−(2μ+k+r1)22(μ+k+r1))(S−Λμ)2+σ21S22+σ22L22−μ2r2k2(2r2k2+μr21)μ2(S−Λμ)2+μ2r2k2r2k2+μr21(k2μ+r212r2)L2+μ2r2k2(2r2k2+μr21)(2μ+d+r2)22(μ+d+r2)(S−Λμ)2−μ2r2k2(2r2k2+μr21)d2I2+μ2r2k2(2r2k2+μr21)σ21S22+μ2r2k2(2r2k2+μr21)σ23I22−μ2k(μ−σ24)4(2r2k2+μr21)R2≤((2μ+k+r1)22(μ+k+r1)−μ−μ2r2k2(2r2k2+μr21)+μr2k(2μ+d+r2)22(2r2k2+μr21)(μ+d+r2))(S−Λμ)2+(μ2r2k2r2k2+μr21+1)σ21S22+σ22L22−μ+r12L2−dμr2k2(2r2k2+μr21)I2+μ2r2kσ232(2r2k2+μr21)I2−μ2k(μ−σ24)4(2r2k2+μr21)R2 |
Considering positive definite C^2 function V:R_+^4\rightarrow R_+ such that
\begin{equation*} \begin{split} V: = &\Big(\frac{(2\mu+k+r_1)^2}{2\mu(\mu+k+r_1)}+\frac{r_2k(2\mu+d+r_2)^2}{2(2r_2k^2+\mu r_1^2)(\mu+d+r_2)}\Big)\Big(V_1+\frac{\Lambda k}{k(1-p)+(\mu+k+r_1)p}V_3\Big)+V_4+\\& \frac{p\Lambda k}{k(1-p)+(\mu+k+r_1)p}V_3+\frac{\mu r_2k}{2r_2k^2+\mu r_1^2}\Big(V_5+\frac{(1-p)\Lambda k}{[k(1-p)+(\mu+k+r_1)p]\mu}V_3+\frac{\mu}{2r_2}V_2\Big). \end{split} \end{equation*} |
By computation,
\begin{equation} \begin{split} \mathcal{L}V\leq &-\Bigg[\mu+\frac{\mu^2 r_2k}{2(2r_2k^2+\mu r_1^2)}-\bigg(\frac{(2\mu+k+r_1)^2}{2\mu(\mu+k+r_1)}+\frac{ r_2k(2\mu+d+r_2)^2}{2(2r_2k^2+\mu r_1^2)(\mu+d+r_2)}+\frac{\mu r_2k+1}{2r_2k^2+\mu r_1^2}\bigg)\sigma_1^2\Bigg]\Big(S-\frac{\Lambda}{\mu}\Big)^2\\&-\frac{\mu+r_1-\sigma_2^2}{2}L^2-\frac{\mu r_2k(d-\mu \sigma_3^2)}{2(2r_2k^2+\mu r_1^2)}I^2-\frac{\mu^2k(\mu-\sigma_4^2)}{4(2r_2k^2+\mu r_1^2)}R^2+\Big(2+\frac{\mu^2r_2k+\mu r_1^2}{2r_2k^2+\mu r_1^2}\Big)\frac{\Lambda^2\sigma_1^2}{\mu^2} \end{split} \end{equation} | (4.5) |
Taking expectation above, (4.5) yields
\begin{equation*} \begin{split} EV(t)-&EV(0) = \int_0^tE\mathcal{L}V(r)dr \leq -\Bigg[\mu+\frac{\mu^2 r_2k}{2(2r_2k^2+\mu r_1^2)}-\bigg(\frac{(2\mu+k+r_1)^2}{2\mu(\mu+k+r_1)}+\frac{\mu r_2k+1}{2r_2k^2+\mu r_1^2}\\&+\frac{ r_2k(2\mu+d+r_2)^2}{2(2r_2k^2+\mu r_1^2)(\mu+d+r_2)}\bigg)\sigma_1^2\Bigg]\int_0^t\bigg(S(r)-\frac{\Lambda}{\mu}\bigg)^2dr-\frac{\mu+r_1-\sigma_2^2}{2}\int_0^tL^2(r)dr\\& -\frac{\mu r_2k(d-\mu \sigma_3^2)}{2(2r_2k^2+\mu r_1^2)}\int_0^tI^2 (r)dr-\frac{\mu^2k(\mu-\sigma_4^2)}{4(2r_2k^2+\mu r_1^2)} \int_0^tR^2(r)dr+ \bigg(2+\frac{\mu^2r_2k+\mu r_1^2}{2r_2k^2+\mu r_1^2}\bigg)\frac{\Lambda^2\sigma_1^2}{\mu^2}t \end{split} \end{equation*} |
Hence
\begin{equation*} \begin{split} \limsup\limits_{t\rightarrow \infty}&\frac{1}{t}E\int_0^t \Bigg{\{} \bigg[\mu+\frac{\mu^2 r_2k}{2(2r_2k^2+\mu r_1^2)}-\Big(\frac{(2\mu+k+r_1)^2}{2\mu(\mu+k+r_1)}+\frac{ r_2k(2\mu+d+r_2)^2}{2(2r_2k^2+\mu r_1^2)(\mu+d+r_2)}+\frac{\mu r_2k+1}{2r_2k^2+\mu r_1^2}\Big)\sigma_1^2\bigg]\\&\ \ \ \cdot\Big(S-\frac{\Lambda}{\mu}\Big)^2+\frac{\mu+r_1-\sigma_2^2}{2}L^2+\frac{\mu r_2k(d-\mu \sigma_3^2)}{2(2r_2k^2+\mu r_1^2)}I^2 +\frac{\mu^2k(\mu-\sigma_4^2)}{4(2r_2k^2+\mu r_1^2)}R^2\Bigg{\}}dr \\& \leq \Big(2+\frac{\mu^2r_2k+\mu r_1^2}{2r_2k^2+\mu r_1^2}\Big)\frac{\Lambda^2\sigma_1^2}{\mu^2}.\end{split} \end{equation*} |
This complete the proof.
In this section, we will show there is a unique distribution for system (4.1) instead of asymptotically stable equilibrium(see [37]). We only consider the first three equation of system (4.1) because the variable R does not participate in the first three equations. Before giving the main theorem, we first give a lemma (see [38]).
Let X(t) be a regular temporally homogeneous Markov process in E_l\subset R^l described by the stochastic differential equation:
dX(t) = b(X)t+\sum\limits_{r = 1}^{k}\sigma_r(X)dB_r(t), |
and the diffusion matrix is defined as follows
A(x) = (a_{i, j}(x)), \ \ a_{i, j}(x) = \sum\limits_{r = 1}^{k}\sigma_r^i(x)\sigma_r^j(x). |
Lemma 4.3. (see [38]) We assume that there exists a bounded domain U\subset E_l with regular boundary, having the following properties:
● In the domain U and some neighborhood thereof, the smallest eigenvalue of the diffusion matrix A(x) is bounded away from zero.
● If x\in E_l\backslash U , the mean time \tau at which a path issuing from x reaches the set U is finite, and \sup_{x\in K}E_x\tau < \infty for every compact subsect K\subset E_l .
then, the Markov process X(t) has a stationary distribution \mu(\cdot) with density in E_l such that for any Borel set \mathcal{B}\subset E_l ,
\lim\limits_{t\rightarrow\infty}P(t, x, \mathcal{B}) = \breve{\mu}(\mathcal{B}), |
and
P_x\Big{\{}\lim\limits_{T\rightarrow \infty}\frac{1}{T}\int_0^Tf(x(t))dt = \int_{E_l}f(x)\breve{\mu}(dx)\Big{\}} = 1, |
for all x\in E_l and f(x) being a function integrable with respect to the measure \breve{\mu} .
Theorem 4.4. Let (S(t), L(t), I(t)) be the solution of system (4.1) with initial value (S(0), L(0), I(0))\in R_+^3 . If R_0 = \frac{\beta \Lambda}{\mu}\frac{k(1-p)+(\mu+k+r_1)p}{(\mu+k+r_1)(\mu+d+r_2)}\leq 1 , and the following conditions are satisfied:
(i)
\begin{equation*} \begin{split}&\rho_1 = \Big(\mu+\frac{(2\mu+d+r_2+r_1)\mu}{\beta S^*}-\frac{(2\mu+r_1)^2}{2(\mu+r_1)}-\frac{(2\mu+d+r_2+r_1+\beta S^*)\sigma_1^2}{\beta S^*}-\frac{(2\mu+d+r_2)^2}{4\alpha k^2(\mu+d+r_2)}\Big)\vee\\& \Big(\mu+\frac{(2\mu+d+r_2+r_1)p}{\sqrt{\alpha}I^*}-\frac{(2\mu+r_1)^2}{2(\mu+r_1)}-\frac{(2\mu+d+r_2)^2}{2 (\mu+d+r_2)}-\frac{((2\mu+d+r_2+r_1)p+\sqrt{\alpha}I^*)\sigma_1^2}{\sqrt{\alpha}I^*}\Big) \gt 0\\ &\rho_2 = \frac{\mu+r_1}{2}-\sigma_2^2 \gt 0, \ \ \rho_3 = \frac{\mu+d+r_2}{2}-\sigma_3^2 \gt 0;\end{split} \end{equation*} |
(ii) \delta < \min \{\rho_1 S^{*2}, \rho_2L^{*2}, \rho_3I^{*2}\} , where S^*, L^*, I^* are difined as in (3.2) and
\delta = \Big[\frac{(2\mu+d+r_2+r_1)p+\sqrt{\alpha}I^*}{\sqrt{\alpha}I^*}\vee \frac{2\mu+d+r_2+r_1+\beta S^*}{\beta S^*}\Big]\sigma_1^2S^{*2}+\sigma_2^2L^{*2}+\sigma_3^2I^{*2} |
then we have
\lim\limits_{t\rightarrow\infty}\frac{1}{t}E\int_0^t[\rho_1(S(r)-S^*)^2+\rho_2(L(r)-L^*)^2+\rho_3(I(r)-I^*)^2]dr\leq\delta. |
Proof. As R_0 > 1 , there is a unique endemic equilibrium X^* = (S^*, L^*, I^*) such that
\begin{equation} \begin{split} \Lambda = \frac{\beta S^*I^*}{1+\alpha I^{*2}}+\mu S^*, \ \frac{(1-p)\beta S^*I^*}{1+\alpha I^{*2}} = (\mu+k+r_1)L^*, \ \frac{p\beta S^*I^*}{1+\alpha I^{*2}} = (\mu+d+r_2)I^*-kL^*.\end{split} \end{equation} | (4.6) |
Define C^2 functions as follows:
V_1(S, L, I) = \frac{S-S^*+L-L^*+I-I^*}{2}, \ \ V_2(I) = I-I^*-I^*\log\frac{I}{I^*}, \ \ \ V_3(S) = \frac{(S-S^*)^2}{2}. |
By computation, we have
\begin{equation*} \begin{split} \mathcal{L}V_1 = &(S-S^*+L-L^*+I-I^*)(-\mu(S-S^*)-(\mu+r_1)(L-L^*)-(\mu+d+r_2)(I-I^*))+\frac{\sigma_1^2S^2}{2}+\\&\frac{\sigma_2^2L^2}{2}+\frac{\sigma_3^2I^2}{2}\\ = &-\mu(S-S^*)^2-(\mu+r_1)(L-L^*)^2-(\mu+d+r_2)(I-I^*)^2-(2\mu+r_1)(S-S^*)(L-L^*)-\\&(2\mu+d+r_2)(S-S^*)(I-I^*)-(2\mu+d+r_2+r_1)(L-L^*)(I-I^*) +\frac{\sigma_1^2S^2}{2}+\frac{\sigma_2^2L^2}{2}+\frac{\sigma_3^2I^2}{2}\\ \mathcal{L}V_2 = &(1-\frac{I^*}{I})(\frac{p\beta SI}{1+\alpha I^2}+kL-(\mu+d+r_2)I)\\ = &p\beta(I-I^*)(\frac{S}{1+\alpha I^2}-\frac{S^*}{1+\alpha I^{*2}})+\frac{k(I-I^*)(L-L^*)}{I^*}-\frac{kL(I-I^*)^2}{II^*}\\ \leq &\frac{p\beta }{1+\alpha I^{*2}}(S-S^*)(I-I^*)+\frac{k(I-I^*)(L-L^*)}{I^*}\\ \mathcal{L}V_3 = &(S-S^*)(\Lambda-\frac{\beta SI}{1+\alpha I^2}-\mu S)+\frac{\sigma_1^2S^2}{2}\\ = &-\mu(S-S^*)^2-\frac{\beta S^*(\alpha II^*-1)}{(1+\alpha I^{*2})(1+\alpha I^2)}(S-S^*)(I-I^*)+\frac{\sigma_1^2S^2}{2} \end{split} \end{equation*} |
We discuss the follow prove in two cases: (S-S^*)(I-I^*)\geq0 or (S-S^*)(I-I^*) < 0 .
1). If (S-S^*)(I-I^*)\geq 0 , define
V(S, L, I) = V_1+\frac{(2\mu+d+r_2+r_1)I^*}{k}V_2+\frac{2\mu+d+r_2+r_1}{\beta S^*}V_3 |
By computation, we have
\begin{equation*} \begin{split} \mathcal{L}V\leq&-\mu(S-S^*)^2-(\mu+r_1)(L-L^*)^2-(\mu+d+r_2)(I-I^*)^2-(2\mu+r_1)(S-S^*)(L-L^*)+\\&\frac{(2\mu+d+r_2+r_1)p\beta I^*}{k(1+\alpha I^{*2})}(S-S^*)(I-I^*)-\frac{(2\mu+d+r_2+r_1)\mu}{\beta S^*}(S-S^*)^2 +\frac{\sigma_1^2S^2}{2}+\frac{\sigma_2^2L^2}{2}+\\&\frac{\sigma_3^2I^2}{2}+\frac{2\mu+d+r_2+r_1}{\beta S^*}\frac{\sigma_1^2S^2}{2}\\ \leq&-\Big(\mu+\frac{(2\mu+d+r_2+r_1)\mu}{\beta S^*}-\frac{(2\mu+r_1)^2}{2(\mu+r_1)}-\frac{(2\mu+d+r_2)^2}{4\alpha k^2(\mu+d+r_2)}\Big)(S-S^*)^2-\frac{\mu+r_1}{2}(L-L^*)^2-\\&\frac{\mu+d+r_2}{2}(I-I^*)^2+\frac{2\mu+d+r_2+r_1+\beta S^*}{\beta S^*}\frac{\sigma_1^2S^2}{2}+ \frac{\sigma_2^2L^2}{2}+\frac{\sigma_3^2I^2}{2} \end{split} \end{equation*} |
2). If (S-S^*)(I-I^*) < 0 , define
{V(S, L, I)} = V_1+\frac{(2\mu+d+r_2+r_1)I^*}{k}V_2+\frac{(2\mu+d+r_2+r_1)p}{\sqrt{\alpha}I^*}V_3 |
By computation, we have
\begin{equation*} \begin{split} \mathcal{L}{V}\leq&-\mu(S-S^*)^2-(\mu+r_1)(L-L^*)^2-(\mu+d+r_2)(I-I^*)^2-(2\mu+r_1)(S-S^*)(L-L^*)-\\&(2\mu+d+r_2)(S-S^*)(I-I^*)-\frac{(2\mu+d+r_2+r_1)\mu p}{\sqrt{\alpha}I^*}(S-S^*)^2 +\frac{\sigma_1^2S^2}{2}+\frac{\sigma_2^2L^2}{2}+\frac{\sigma_3^2I^2}{2}+\\&\frac{(2\mu+d+r_2+r_1)\mu p}{\sqrt{\alpha}I^*}\frac{\sigma_1^2S^2}{2}\\ \leq&-\Big(\mu+\frac{(2\mu+d+r_2+r_1)p}{\sqrt{\alpha}I^*}-\frac{(2\mu+r_1)^2}{2(\mu+r_1)}-\frac{(2\mu+d+r_2)^2}{2 (\mu+d+r_2)}\Big)(S-S^*)^2-\frac{\mu+r_1}{2}(L-L^*)^2-\\&\frac{\mu+d+r_2}{2}(I-I^*)^2+\frac{(2\mu+d+r_2+r_1)p+\sqrt{\alpha}I^*}{\sqrt{\alpha}I^*}\frac{\sigma_1^2S^2}{2}+ \frac{\sigma_2^2L^2}{2}+\frac{\sigma_3^2I^2}{2} \end{split} \end{equation*} |
Over all we have
\begin{equation} \begin{split} \mathcal{L}V\leq-\rho_1(S-S^*)^2-\rho_2(L-L^*)^2-\rho_3(I-I^*)^2+\delta \end{split} \end{equation} | (4.7) |
where
\begin{equation*} \begin{split}\rho_1 = &\Big(\mu+\frac{(2\mu+d+r_2+r_1)\mu}{\beta S^*}-\frac{(2\mu+r_1)^2}{2(\mu+r_1)}-\frac{(2\mu+d+r_2+r_1+\beta S^*)\sigma_1^2}{\beta S^*}-\frac{(2\mu+d+r_2)^2}{4\alpha k^2(\mu+d+r_2)}\Big)\vee \\&\Big(\mu+\frac{(2\mu+d+r_2+r_1)p}{\sqrt{\alpha}I^*}-\frac{(2\mu+r_1)^2}{2(\mu+r_1)}-\frac{(2\mu+d+r_2)^2}{2 (\mu+d+r_2)}-\frac{((2\mu+d+r_2+r_1)p+\sqrt{\alpha}I^*)\sigma_1^2}{\sqrt{\alpha}I^*}\Big)\\ \rho_2 = &\frac{\mu+r_1}{2}-\sigma_2^2, \ \ \ \ \ \rho_3 = \frac{\mu+d+r_2}{2}-\sigma_3^2, \\ \delta = &\Big[\frac{(2\mu+d+r_2+r_1)p+\sqrt{\alpha}I^*}{\sqrt{\alpha}I^*}\vee \frac{2\mu+d+r_2+r_1+\beta S^*}{\beta S^*}\Big]\sigma_1^2S^{*2}+\sigma_2^2L^{*2}+\sigma_3^2I^{*2} \end{split} \end{equation*} |
If \delta < \min \{\rho_1 S^{*2}, \rho_2L^{*2}, \rho_3I^{*2}\} , then the ellipsoid
\rho_1(S-S^*)^2+\rho_2(L-L^*)^2+\rho_3(I-I^*)^2 = \delta |
lies entirely R_+^3 . We can take U as any neighborhood of the ellipsoid such that \overline{U}\in R_+^3 , where \overline{U} is the closure of U . Thus, we have LV < 0 which implies the second condition in Lemma 4.3 is satisfied. Besides, there is Q > 0 such that
\sum\limits_{i, j = 1}^{n}\Big(\sum\limits_{k = 1}^na_{ik}(x)a_{jk}(x)\Big)\xi_i\xi_j = \sigma_1^2x_1^2\xi_1^2+\sigma_2^2x_2^2\xi_2^2+\sigma_3^2x_3^2\xi_3^2 \geq Q|\xi|^2, \ \text{for all} x\in \overline{U}, \xi\in R^3 |
Applying Rayleigh's principle (see [39], p. 349), the first condition in Lemma 4.3 is satisfied. Therefore, the stochastic system (4.1) has a unique stationary distribution \mu(\cdot) and it is ergodic.
In this section, we investigate the exponential decay of the global solution of system (4.1) as the intensity of white noise is great. It can be shown below, even if the endemic equilibrium exists in the system (2.1), the stochastic effect may make washout more likely in system (4.1).
Theorem 4.5. Let (S(t), L(t), I(t), R(t)) be the solution of system (4.1) with any initial value (S(0), L(0), I(0), R(0))\in R_+^4 . If \frac{\Lambda \beta (\mu+k+r_1)}{\mu k} < (\frac{\sigma_3^2}{2}+\mu+d+r_2)\wedge (\frac{\sigma_2^2}{2}) then,
\begin{array}{l} \mathop {\lim \sup }\limits_{t \to \infty } \frac{1}{t}\log [L + \frac{{\mu + k + {r_1}}}{k}I] \le \frac{{\Lambda \beta k}}{{\mu (\mu + k + {r_1})}} - {(\frac{k}{{\mu + k + {r_1}}})^2}[(\frac{{\sigma _3^2}}{2} + \mu + d + {r_2}) \wedge (\frac{{\sigma _2^2}}{2})]\\ \mathop {\lim \sup }\limits_{t \to \infty } \frac{1}{t}\log R(t) \le \{ ({r_1} \vee {r_2}) \cdot \{ [ - (\mu + \frac{{\sigma _4^2}}{2})] \vee \zeta \} \} \\ \mathop {\lim \sup }\limits_{t \to \infty } \frac{1}{t}\int_0^t S (u)du = \frac{\Lambda }{\mu },\;\;\;a.e.\;\;\;S(t){ \to ^\omega }\nu ,\;\;\;as\;\;t \to \infty , \end{array} |
where \rightarrow ^\omega means the convergence in distribution and \nu is a probability measure in R_+^1 such that \int_0^\infty x\nu(dx) = \frac{\Lambda}{\mu} . In particularly, \nu has density (A\sigma_1^2 x^2p(x))^{-1} , where A is a normal constant,
p(x) = \mathit{\text{exp}}\Big(-\frac{2\Lambda}{\sigma_1^2}\Big)x^{\frac{2\mu}{\sigma_1^2}}\mathit{\text{exp}}\Big(\frac{2\Lambda}{\sigma_1^2 x}\Big), \ \ x \gt 0. |
Proof. By comparison theorem, we see that S(t)\leq X(t) , where X(t) is the global solution of the following stochastic system with initial value X(0) = S(0) :
\begin{equation} dX = (\Lambda-\mu X)dt+\sigma_1XdB_1(t) \end{equation} | (4.8) |
Obviously, (4.8) is a diffusion process lying in R_+^1 .
Firstly, we show (4.8) is stable in distribution and ergodic. Let Y(t) = X(t)-\frac{\Lambda}{\mu} , then Y(t) satisfies
\begin{equation} dY = -\mu Ydt+\sigma_1(Y+\frac{\Lambda}{\mu})dB_1(t). \end{equation} | (4.9) |
Theorem 2.1(a) in[40] with C = 1 implies that the diffusion process Y(t) is stable in distribution as t\rightarrow \infty , so does X(t) .
To prove the ergodicity of X(t) , we difine
p(x) = \text{exp}\Big(-2\int_0^x \frac{\Lambda-\mu y}{\sigma_1^2 y^2}dy\Big) = \text{exp}\Big(-\frac{2\Lambda}{\sigma_1^2}\Big)x^{\frac{2\mu}{\sigma_1^2}}\text{exp}\Big(\frac{2\Lambda}{\sigma_1^2 x}\Big) |
and it is noted that for each integer n\geq 1 , there exist positive constants C_1(n), C_2(n) and M(n) such that
\begin{equation} p(x)\geq C_1(n)x^{\frac{2\mu}{\sigma_1^2}-n}, \ \ as \ \ 0 \lt x \lt \frac{1}{M(n)}, \ \ \ p(x)\geq C_2(n)x^{\frac{2\mu}{\sigma_1^2}}, \ \ as\ \ x \gt \frac{1}{M(n)}. \end{equation} | (4.10) |
Therefore, together with (4.10) we see
\int_1^\infty p(x)dx = \infty, \ \ \ \int_0^1 p(x)dx = \infty, \ \ \int_0^\infty \frac{dx}{\sigma_1^2 p(x)x^2} \lt \infty. |
So X(t) is ergodic (Theorem 1.16 in [41]), and with respect to the Lebesgue measure its invariant measure \nu has density (A\sigma_1^2 p(x)x^2)^{-1} , where A is a normal constant.
Now, we show that f(t): = EX^p(t) is uniformly bounded for some p > 1 determined later. Applying It \hat{\text{o}} 's formula to X^p , we have
dX^p = \Big(p\Lambda X^{p-1}-p\mu X^p+\frac{\sigma_1^2p(p-1)X^p}{2}\Big)dt+p\sigma_1 X^p dB_1(t). |
Taking expectation of equation above, and using the fact a^{\frac{1}{p}}b^{\frac{p-1}{p}}\leq \frac{a}{p}+\frac{b(p-1)}{p}, a, b > 0 ,
f'(t)\leq \frac{\Lambda ^p}{\varepsilon^{p-1}}+\frac{p\varepsilon}{p-1}f(t)-p\Big[\mu-\frac{\sigma_1^2(p-1)}{2}\Big]f(t)\leq\frac{\Lambda ^p}{\varepsilon^{p-1}}+p\Big[\frac{\varepsilon}{p-1}-(\mu-\frac{\sigma_1^2(p-1)}{2})\Big]f(t). |
Choosing \varepsilon > 0 sufficiently small and p > 1 closely enough to 1 such that
\mu-\frac{\sigma_1^2(p-1)}{2} \lt 0, \ \ \ \frac{\varepsilon}{p-1}-(\mu-\frac{\sigma_1^2(p-1)}{2}) \lt 0. |
Hence, \sup_{t\geq 0}EX^p(t) = \sup_{t\geq 0}f(t) < \infty , implying that \int_0^\infty x^p\nu(dx) < \infty . Together with its ergodicity we have
\begin{equation} p_x\Big\{\lim\limits_{T\rightarrow \infty}\frac{1}{T}\int_0^T X(t)dt = \int_0^\infty x\nu(dx)\Big\} = 1. \end{equation} | (4.11) |
for all x\in R_+^1 . On the other hand, Jensen's inequality yields
E\Big[\frac{1}{T}\int_0^T X(t)dt \Big]^p\leq E\frac{1}{T}\int_0^TX^p(t)dt\leq \sup\limits_{t\geq 0}EX^p(t) \lt \infty, |
therefore, \{ \frac{1}{T}\int_0^T X(t)dt, t\geq 0\} is uniformly integrable. Together with (4.11), we have
\begin{equation} E\frac{1}{T}\int_0^TX^p(t)dt\rightarrow \int_0^\infty x\nu (dx). \end{equation} | (4.12) |
Taking expectation of (4.8), we have
\frac{EX(t)}{t} = \Lambda-\frac{\mu}{t}E\int_0^tX(s)ds. |
Let t\rightarrow \infty , taking (4.12) into account, then we have \int_0^\infty x\nu(dx) = \frac{\Lambda}{\mu} .
We shall eventually show that S(t) is stable in distribution. To do this, as in [15], we introduce a new stochastic process S_\varepsilon (t) which is defined by initial condition S_\varepsilon(0) = S(0) and the stochastic differential equation
dS_\varepsilon = [\Lambda-(\mu+\varepsilon)S_\varepsilon]dt+\sigma_1S_\varepsilon d B_1(t). |
We prove that
\begin{equation} \lim\limits_{t\rightarrow\infty}(S(t)-S_\varepsilon (t))\geq 0, \ \ \ a.e. \end{equation} | (4.13) |
Therefore consider
d(S-S_\varepsilon) = \Big[\Big(\varepsilon-\frac{\beta I}{1+\alpha I^2}\Big)S-(\mu+\varepsilon)(S-S_\varepsilon)\Big]dt+\sigma_1(S-S_\varepsilon)dB_1(t). |
The solution is given by
\begin{equation*} \begin{split}S(t)-S_\varepsilon (t) = &\text{exp}\Big{\{} -\Big(\mu+\varepsilon+\frac{\sigma_1^2}{2}\Big)t+\sigma_1B_1(t)\Big{\}}\int_0^t\text{exp}\Big{\{}\Big(\mu+\varepsilon+\frac{\sigma_1^2}{2}\Big)- \sigma_1B_1(s)\Big{\}}\cdot\Big(\varepsilon-\frac{\beta I(s)}{1+\alpha I^2(s)}\Big)S(s)ds.\end{split} \end{equation*} |
For almost \omega\in\Omega, \exists T = T(\omega) such that \varepsilon > \frac{\beta I(t)}{1+\alpha I^2(t)}, \ \ \forall t > T .
Hence as the proof of Theorem 5.2 in [15] for almost \omega\in \Omega , for any t > T ,
\begin{equation*} \begin{split}S(t)-S_\varepsilon (t)\geq& \text{exp}\Big{\{}-\Big(\mu+\varepsilon+\frac{\sigma_1^2}{2}\Big)t+\sigma_1B_1(t)\Big{\}}\int_0^T\text{exp}\Big{\{}\Big(\mu+\varepsilon+\frac{\sigma_1^2}{2}\Big)- \sigma_1B_1(s)\Big{\}} \cdot\Big(\varepsilon-\frac{\beta I(s)}{1+\alpha I^2(s)}\Big)S(s)ds. \end{split} \end{equation*} |
Therefore, \liminf\limits_{t\rightarrow\infty}(S(t)-S_\varepsilon(t)\geq 0, \ a.e. Next, it is noted that
d(X-S_\varepsilon) = [\varepsilon S_\varepsilon-\mu(X-S_\varepsilon)]dt+\sigma_1(X-S_\varepsilon)dB_1(t). |
Taking the expectation of above equation, we see
E|X(t)-S_\varepsilon(t)| = \int_0^t[\varepsilon S_\varepsilon(u)-\mu(X(u)-S_\varepsilon(u))]du\leq \int_0^t[\varepsilon X(u)-\mu|X(u)-S_\varepsilon(u)|]du |
where the last inequality is using the fact that S_\varepsilon(t)\leq X(t) . Hence we have
E|X(t)-S_\varepsilon(t)|\leq \frac{\varepsilon \sup\limits_{u\geq 0}EX_u}{\mu}(1-\text{exp}(-\mu t)). |
This implies that
\begin{equation} \liminf\limits_{\varepsilon\rightarrow 0}\lim\limits_{t\rightarrow \infty}E|X(t)-S_\varepsilon(t)| = 0. \end{equation} | (4.14) |
Combining (4.13), (4.14) and the fact that S(t)\leq X(t) , we have
\lim\limits_{t\rightarrow \infty}(X(t)-S(T)) = 0, \ \ \ \text{in probability}. |
It has been shown that X(t) converges weakly to distribution \nu , so does S(t) as t\rightarrow\infty .
Secondly, using It \hat{\text{o}} 's formula to \log[L+\frac{\mu+k+r_1}{k}I] and the fact that S(t)\leq X(t) show
\begin{equation*} \begin{split}d\log\Big[L+\frac{\mu+k+r_1}{k}I\Big] = &\frac{(k(1-p)+(\mu+k+r_1)p)\beta SI}{k(1+\alpha I^2)(L+\frac{\mu+k+r_1}{k}I)}-\frac{1}{(L+\frac{\mu+k+r_1}{k}I)^2}\Big[\Big(\frac{\sigma_3^2}{2}+\mu+d+r_2\Big)\Big(\frac{\mu+k+r_1}{k}\Big)^2I^2\\&+\frac{\sigma_2^2L^2}{2}\Big]dt + \frac{\sigma_2L}{L+\frac{\mu+k+r_1}{k}I}dB_2(t)+\frac{\sigma_3(\mu+k+r_1)I}{k(L+\frac{\mu+k+r_1}{k}I)}dB_3(t)\\ \leq &\frac{(k(1-p)+(\mu+k+r_1)p)\beta kX}{\mu+k+r_1}-\Big(\frac{k}{\mu+k+r_1}\Big)^2\Big[(\frac{\sigma_3^2}{2}+\mu+d+r_2)\wedge (\frac{\sigma_2^2}{2})\Big]dt+ \\& \frac{\sigma_2L}{L+\frac{\mu+k+r_1}{k}I}dB_2(t)+ \frac{\sigma_3(\mu+k+r_1)I}{k(L+\frac{\mu+k+r_1}{k}I)}dB_3(t)\\ \end{split} \end{equation*} |
Integrating the above inequality from 0 to t , together with (4.11) and the fact that \lim_{t\rightarrow \infty}\frac{|B_i(t)|}{t} = 0, i = 2, 3 [35], yields
\begin{equation} \limsup\limits_{t\rightarrow\infty}\frac{1}{t}\log\Big[L+\frac{\mu+k+r_1}{k}I\Big]\leq \frac{\Lambda \beta k}{\mu(\mu+k+r_1)}-\Big(\frac{k}{\mu+k+r_1}\Big)^2\Big[(\frac{\sigma_3^2}{2}+\mu+d+r_2)\wedge (\frac{\sigma_2^2}{2})\Big] = :\zeta \end{equation} | (4.15) |
To help with the proof we introduce another diffusion process \widetilde{R}(t) which is defined by the initial condition \widetilde{R}(0) = R(0) and the stochastic differential equation
d\widetilde{R} = -\mu\widetilde{R}(t)dt+\sigma_4 \widetilde{R}dB_4(t). |
Then consider
d(R-\widetilde{R}) = (r_1 L+r_2I-\mu (R-\widetilde{R}))dt+\sigma_4(R-\widetilde{R})dB_4(t). |
The solution is given by
R(t)-\widetilde{R}(t) = \text{exp}\Big{\{}-\Big(\mu+\frac{\sigma_4^2}{2}\Big)t+\sigma_4B_4(t)\Big{\}}\int_0^t\text{exp}\Big{\{}\Big(\mu+\frac{\sigma_4^2}{2}\Big)s- \sigma_4B_4(s)\Big{\}}(r_1L(s)+r_2I(s))ds. |
By (4.15) and the fact that \lim\limits_{t\rightarrow \infty}\frac{|B_4(t)|}{t} = 0, it has been shown that, for any \tilde{\varepsilon} > 0 and almost \omega\in \Omega, \exists T = T(\omega) such that
\begin{equation*} \begin{split}r_1L(t)+r_2I(t)\leq& (r_1 \vee r_2)(L(t)+I(t))\leq (r_1 \vee r_2) L(t)+\frac{\mu+k+r_1}{k}I(t) \leq (r_1 \vee r_2)\text{exp}((\zeta+\tilde{\varepsilon})t), \ \ \forall t\geq T, \end{split} \end{equation*} |
where \zeta is defined in (4.15). Hence for all \omega\in \Omega , if t > T(\omega) , then
\begin{equation*} \begin{split} |R(t)-\widetilde{R}(t)|\leq & \text{exp}\Big{\{}-\Big(\mu+\frac{\sigma_4^2}{2}\Big)t+\sigma_4B_4(t)\Big{\}}\int_0^T\text{exp}\Big{\{}\Big(\mu+\frac{\sigma_4^2}{2}\Big)s- \sigma_4B_4(s)\Big{\}}(r_1L(s)+r_2I(s))ds+ \\&(r_1 \vee r_2)\text{exp}\Big{\{}-\Big(\mu+\frac{\sigma_4^2}{2}\Big)t+\sigma_4B_4(t)+ \sigma_4\max\limits_{s\leq t}|B_4(t)| \Big{\}}\int_T^t\text{exp}\Big{\{}\Big(\mu+\frac{\sigma_4^2}{2}+\zeta+\tilde{\varepsilon}\Big)s\Big{\}}ds \end{split} \end{equation*} |
Therefore,
\limsup\limits_{t\rightarrow \infty}\frac{1}{t}\log|R(t)-\widetilde{R}(t)|\leq (r_1 \vee r_2)\cdot \Big{\{}\Big[-\Big(\mu+\frac{\sigma_4^2}{2}\Big)\Big]\vee[\zeta+\tilde{\varepsilon}]\Big{\}}, \ \ a.e. |
Let \tilde{\varepsilon}\rightarrow 0 , we get \limsup\limits_{t\rightarrow \infty }\frac{1}{t}\log|R(t)-\widetilde{R}(t)|\leq (r_1 \vee r_2)\cdot \{[-(\mu+\frac{\sigma_4^2}{2})]\vee \zeta\}, a.e. On the other hand,
\widetilde{R}(t) = R(0)\text{exp} \Big{\{}-\Big(\mu+\frac{\sigma_4^2}{2}\Big)t+\sigma_4B_4(t)\Big{\}} |
and hence, \limsup\limits_{t\rightarrow \infty }\frac{1}{t}\log\widetilde{R}(t) = -(\mu+\frac{\sigma_4^2}{2}) , Therefore
\mathop {\lim \sup }\limits_{t \to \infty } \frac{1}{t}\log R(t) \le \{ ({r_1} \vee {r_2}) \cdot \{ [ - (\mu + \frac{{\sigma _4^2}}{2})] \vee \zeta \} \} . |
Based on the model proposed in Xiao and Ruan[8], we proposed an SLIR model with a nonmonotone and nonlinear incidence rate of the form \beta IS/(1 + \alpha I^2) which is increasing when I is small and decreasing when I is large. It can be used to interpret the 'psychological' effect: the number of effective contacts between infective individuals and susceptible individuals decreases at high infective levels due to the quarantine of infective individuals or the protection measures by the susceptible individuals.
We have provided a complete description of the asymptotic behaviour of the solutions of the deterministic model (2.1) and (2.4). Interestingly, this model does not exhibit complicated dynamics as other epidemic models with other types of incidence rates reported in [1,3,4,5,6,8]. In terms of the basic reproduction number R_0 = \frac{\beta \Lambda}{\mu}\frac{k(1-p)+(\mu+k+r_1)p}{(\mu+k+r_1)(\mu+d+r_2)} , our main results indicate that when R_0 < 1 , the disease-free equilibrium is globally attractive. When R_0 > 1 , the endemic equilibrium exists and is globally stable.
A stochastic differential equation (SDE) is formulated for describing the disease in this case. We prove the existence and uniqueness of the solution of this SDE. We proved the positivity of the solutions. Then, we investigate the stability of the model. We illustrated the dynamical behavior of the SLIR model according to R_0 < 1 . We proved that the infective tends asymptotically to zero exponentially almost surely as R_0 < 1 . We also proved that the SLIR model has the ergodic property as the fluctuation is small, where the positive solution converges weakly to the unique stationary distribution.
This work is supported by the National Natural Science Foundation of China No:11601542 and 11771407, the Postdoctoral Research Grant in Henan Province No:001803011.
The authors declare no conflict of interest.
[1] |
R. Singh, S. V. S. Deo, E. Dhamija, S. Mathur, S. Thulkar, To Evaluate the Accuracy of Axillary Staging Using Ultrasound and Ultrasound-Guided Fine-Needle Aspiration Cytology (USG-FNAC) in Early Breast Cancer Patients-a Prospective Study, Indian J. Surg. Oncol., 11 (2020), 726-734. doi: 10.1007/s13193-020-01222-3
![]() |
[2] |
I. González-Huebra, A. Elizalde, A. García-Baizán, M. Calvo, A. Ezponda, F. Martínez-Regueira, et al., Is it worth to perform preoperative MRI for breast cancer after mammography, tomosynthesis and ultrasound?, Magn. Reson. Imag., 57 (2019), 317-322. doi: 10.1016/j.mri.2018.12.005
![]() |
[3] |
Y. Huang, F. Li, J. Han, C. Peng, Q. Li, L. Cao, et al., Shear wave elastography of breast lesions: quantitative analysis of elastic heterogeneity improves diagnostic performance, Ultrasound Med. Biol., 45 (2019), 1909-1917. doi: 10.1016/j.ultrasmedbio.2019.04.019
![]() |
[4] |
M. Di Segni, V. de Soccio, V. Cantisani, G. Bonito, A. Rubini, G. Di Segni, et al., Automated classification of focal breast lesions according to S-detect: validation and role as a clinical and teaching tool, J. Ultrasound, 21 (2018), 105-118. doi: 10.1007/s40477-018-0297-2
![]() |
[5] |
T. V. Bartolotta, A. A. M. Orlando, L. Spatafora, M. Dimarco, C. Gagliardo, A. Taibbi, S-Detect characterization of focal breast lesions according to the US BI RADS lexicon: a pictorial essay, J. Ultrasound, 23 (2020), 207-215. doi: 10.1007/s40477-020-00447-w
![]() |
[6] | T. V. Bartolotta, A. A. M. Orlando, M. L. Di Vittorio, F. Amato, M. Dimarco, D. Matranga, et al., S-Detect characterization of focal solid breast lesions: a prospective analysis of inter-reader agreement for US BI-RADS descriptors, J. Ultrasound, 5 (2020), 1-8. |
[7] |
R. M. Jales, M. T. Dória, K. P. Serra, M. M. Miranda, C. A. Menossi, K. Schumacher, et al., Power Doppler Ultrasonography and Shear Wave Elastography as Complementary Imaging Methods for Suspected Local Breast Cancer Recurrence, J. Ultrasound Med., 37 (2018), 1493-1501. doi: 10.1002/jum.14493
![]() |
[8] |
R. Guo, G. Lu, B. Qin, B. Fei, Ultrasound Imaging Technologies for Breast Cancer Detection and Management: A Review, Ultrasound Med. Biol., 44 (2018), 37-70. doi: 10.1016/j.ultrasmedbio.2017.09.012
![]() |
[9] |
J. Geisel, M. Raghu, R. Hooley, The role of ultrasound in breast cancer screening: the case for and against ultrasound, Semin Ultrasound CT MR, 39 (2018), 25-34. doi: 10.1053/j.sult.2017.09.006
![]() |
[10] |
J. Y. Wu, Z. Z. Zhao, W. Y. Zhang, M. Liang, B. Ou, H. Y. Yang, et al., Computer-aided diagnosis of solid breast lesions with ultrasound: factors associated wth false-negative and false-positive results, J. Ultrasound Med., 38 (2019), 3193-3202. doi: 10.1002/jum.15020
![]() |
[11] |
A. S. Tagliafico, M. Piana, D. Schenone, R. Lai, A. M. Massone, N. Houssami, Overview of radiomics in breast cancer diagnosis and prognostication, Breast, 49 (2020), 74-80. doi: 10.1016/j.breast.2019.10.018
![]() |
[12] |
K. Kim, M. K. Song, E. K. Kim, J. H. Yoon, Clinical application of S-Detect to breast masses on ultrasonography: a study evaluating the diagnostic performance and agreement with a dedicated breast radiologist, Ultrasonography, 36 (2017), 3-9. doi: 10.14366/usg.16012
![]() |
[13] |
E. Cho, E. K. Kim, M. K. Song, J. H. Yoon, Application of computer-aided diagnosis on breast ultrasonography: evaluation of diagnostic performances and agreement of radiologists according to different levels of experience, J. Ultrasound Med., 37 (2018), 209-216. doi: 10.1002/jum.14332
![]() |
[14] |
J. H. Choi, B. J. Kang, J. E. Baek, H. S. Lee, S. H. Kim, Application of computer-aided diagnosis in breast ultrasound interpretation: improvements in diagnostic performance according to reader experience, Ultrasonography, 37 (2018): 217-225. doi: 10.14366/usg.17046
![]() |
[15] |
W. H. Yuan, A. F. Li, Y. H. Chou, H. C. Hsu, Y. Y. Chen, Clinical and ultrasonographic features of male breast tumors: A retrospective analysis, PLoS One, 13 (2018), e0194651. doi: 10.1371/journal.pone.0194651
![]() |
[16] |
S. H. Yeo, G. R. Kim, S. H. Lee, W. K. Moon, Comparison of ultrasound elastography and color doppler ultrasonography for distinguishing small triple-negative nreast cancer from fibroadenoma, J. Ultrasound Med., 37 (2018), 2135-2146. doi: 10.1002/jum.14564
![]() |
[17] |
A. Vourtsis, Three-dimensional automated breast ultrasound: Technical aspects and first results, Diagn. Interv. Imaging, 100 (2019), 579-592. doi: 10.1016/j.diii.2019.03.012
![]() |
[18] |
D. A. Spak, J. S. Plaxco, L. Santiago, M. J. Dryden, B. E. Dogan, BI-RADS(®) fifth edition: A summary of changes, Diagn. Interv. Imaging, 98 (2017), 179-190. doi: 10.1016/j.diii.2017.01.001
![]() |
[19] |
X. X. Qu, Y. Song, Y. H. Zhang, H. M. Qing, Value of ultrasonic elastography and conventional ultrasonography in the differential diagnosis of non-mass-like breast lesions, Ultrasound Med. Biol., 45 (2019), 1358-1366. doi: 10.1016/j.ultrasmedbio.2019.01.020
![]() |
[20] |
B. L. Niell, P. E. Freer, R. J. Weinfurtner, E. K. Arleo, J. S. Drukteinis, Screening for breast cancer, Radiol. Clin. North Am., 55 (2017), 1145-1162. doi: 10.1016/j.rcl.2017.06.004
![]() |
[21] |
F. Valdora, N. Houssami, F. Rossi, M. Calabrese, A. S. Tagliafico, Rapid review: radiomics and breast cancer, Breast Cancer Res. Treat., 169 (2018), 217-229. doi: 10.1007/s10549-018-4675-4
![]() |
[22] |
F. Taskin, Y. Durum, A. Soyder, A. Unsal, Review and management of breast lesions detected with breast tomosynthesis but not visible on mammography and ultrasonography, Acta. Radiol., 58 (2017), 1442-1447. doi: 10.1177/0284185117710681
![]() |
1. | Jayanta Kumar Ghosh, Prahlad Majumdar, Uttam Ghosh, Qualitative analysis and optimal control of an SIR model with logistic growth, non-monotonic incidence and saturated treatment, 2021, 16, 0973-5348, 13, 10.1051/mmnp/2021004 | |
2. | Puspa Eosina, Aniati Murni Arymurthy, Adila Alfa Krisnadhi, A Non-Uniform Continuous Cellular Automata for Analyzing and Predicting the Spreading Patterns of COVID-19, 2022, 6, 2504-2289, 46, 10.3390/bdcc6020046 | |
3. | Zhicheng Zheng, Wei Shen, Yang Li, Yaochen Qin, Lu Wang, Spatial equity of park green space using KD2SFCA and web map API: A case study of zhengzhou, China, 2020, 123, 01436228, 102310, 10.1016/j.apgeog.2020.102310 | |
4. | Eric Innocenti, Marielle Delhom, Corinne Idda, Pierre-Regis Gonsolin, Dominique Urbani, 2021, Agent-Based Modelling of the spread of COVID-19 in Corsica, 978-1-7281-9048-8, 1, 10.1109/SSCI50451.2021.9660184 | |
5. | Abhi Chakraborty, K.M. Ariful Kabir, Enhancing vaccination strategies for epidemic control through effective lockdown measures, 2024, 10, 24058440, e32308, 10.1016/j.heliyon.2024.e32308 | |
6. | Wei Wei, Hongjun Gao, Qiyong Cao, Synchronization of differential equations driven by linear multiplicative fractional Brownian motion, 2024, 14, 2158-3226, 10.1063/5.0186441 | |
7. | Pritam Saha, Kalyan Kumar Pal, Uttam Ghosh, Pankaj Kumar Tiwari, Dynamic analysis of deterministic and stochastic SEIR models incorporating the Ornstein–Uhlenbeck process, 2025, 35, 1054-1500, 10.1063/5.0243656 |