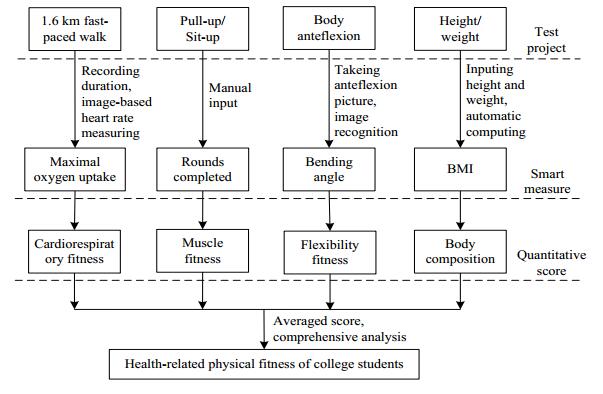
Citation: Xuewei Wang, Xiaohu Zhao, Fei Li, Qiang Lin, Zhenghui Hu. Sample entropy and surrogate data analysis for Alzheimer’s disease[J]. Mathematical Biosciences and Engineering, 2019, 16(6): 6892-6906. doi: 10.3934/mbe.2019345
[1] | Katrin Gossen, Marius Dotter, Bennet Brockhagen, Jan Lukas Storck, Andrea Ehrmann . Long-term investigation of unsealed DSSCs with glycerol-based electrolytes of different compositions. AIMS Materials Science, 2022, 9(2): 283-296. doi: 10.3934/matersci.2022017 |
[2] | Ha Thanh Tung, Ho Kim Dan, Dang Huu Phuc . Effect of the calcination temperature of the FTO/PbS cathode on the performance of a quantum dot-sensitized solar cell. AIMS Materials Science, 2023, 10(3): 426-436. doi: 10.3934/matersci.2023023 |
[3] | Noah M. Johnson, Yuriy Y. Smolin, Chris Shindler, Daniel Hagaman, Masoud Soroush, Kenneth K. S. Lau, Hai-Feng Ji . Photochromic dye-sensitized solar cells. AIMS Materials Science, 2015, 2(4): 503-509. doi: 10.3934/matersci.2015.4.503 |
[4] | Silvia Colodrero . Conjugated polymers as functional hole selective layers in efficient metal halide perovskite solar cells. AIMS Materials Science, 2017, 4(4): 956-969. doi: 10.3934/matersci.2017.4.956 |
[5] | Stavroula Sfaelou, Panagiotis Lianos . Photoactivated Fuel Cells (PhotoFuelCells). An alternative source of renewable energy with environmental benefits. AIMS Materials Science, 2016, 3(1): 270-288. doi: 10.3934/matersci.2016.1.270 |
[6] | Etsana Kiros Ashebir, Berhe Tadese Abay, Taame Abraha Berhe . Sustainable A2BⅠBⅢX6 based lead free perovskite solar cells: The challenges and research roadmap for power conversion efficiency improvement. AIMS Materials Science, 2024, 11(4): 712-759. doi: 10.3934/matersci.2024036 |
[7] | Avner Neubauer, Shira Yochelis, Gur Mittelman, Ido Eisenberg, Yossi Paltiel . Simple down conversion nano-crystal coatings for enhancing Silicon-solar cells efficiency. AIMS Materials Science, 2016, 3(3): 1256-1265. doi: 10.3934/matersci.2016.3.1256 |
[8] | Takayuki Aoyama, Mari Aoki, Isao Sumita, Atsushi Ogura . Effects of particle size of aluminum powder in silver/aluminum paste on n-type solar cells. AIMS Materials Science, 2018, 5(4): 614-623. doi: 10.3934/matersci.2018.4.614 |
[9] | Roger Chang, Kemakorn Ithisuphalap, Ilona Kretzschmar . Impact of particle shape on electron transport and lifetime in zinc oxide nanorod-based dye-sensitized solar cells. AIMS Materials Science, 2016, 3(1): 51-65. doi: 10.3934/matersci.2016.1.51 |
[10] | Aruna P. Wanninayake, Shengyi Li, Benjamin C. Church, Nidal Abu-Zahra . Electrical and optical properties of hybrid polymer solar cells incorporating Au and CuO nanoparticles. AIMS Materials Science, 2016, 3(1): 35-50. doi: 10.3934/matersci.2016.1.35 |
Physical fitness is interpreted as a measure of the ability to perform physical activity that integrates the majority of bodily functions, and its components are divided into those related to health and those related to athletic skill [1]. Common people are mainly concerned about physical fitness, which is needed to promote health conditions, prevent diseases, and improve daily life and work efficiency. This fitness is physical-related physical fitness [2,3]. Physical-related physical fitness is composed of five basic elements: cardiorespiratory fitness, muscle fitness, muscular endurance fitness, flexibility fitness, and body composition [4,5]. Chinese college students generally have many physical problems, such as muscle weakness and being overweight [6]. Some declining physical indexes of college students are highly consistent with the five basic elements of health-related physical fitness. For example, improving cardiorespiratory fitness is conducive to enhancing the pulmonary function of college students. Improving muscle fitness and muscular endurance and flexibility fitness is beneficial to enhancing endurance, strength, speed, and other physical indexes of college students. Optimization of body composition is beneficial to reducing the weight of obese college students. Thus, improving the health-related physical fitness of college students can effectively improve their physical conditions.
Most countries have formulated relevant physical test methods for students [7,8,9]. The traditional test method of the health-related physical fitness of college students, which is accomplished with human assistance, is the most common test method at present. To improve the level and statistical efficiency of the testing of the health-related physical fitness of college students, researchers have developed several electronic test devices [10,11,12,13,14]. These devices are generally designed for tests and records of specific items and include the electronic long-distance running tester, electronic pull-up tester, electronic sit-and-reach tester, and electronic sit-up tester. These devices generally consist of detection and record devices. Sensors in detection devices are responsible for measuring items and transmitting data to a recording device through wired or wireless communication means. These instruments generally have large volume, high cost, diversified categories, complicated installation and arrangement, and inconvenient applications. Therefore, developing a simple and flexible testing technology for the health-related physical fitness of college students is necessary to reduce detection cost and simplify detection operations.
Currently, mobile Internet and intelligent mobile terminals are developed quickly and widely used in various aspects of production and daily living, thereby changing the lifestyles of people [15,16]. These technologies provide new ideas for the intelligent testing of health-related physical fitness. Computer-supported systems or tools have been increasingly emerging in intelligent mobile terminals [17]; most of them target college students and youth. Such systems and applications include Fitness Tour [18], StepSteam [19], and tools proposed by Buttussi et al. [20] and Cai et al. [21]. Fitness Tour is an Android-based mobile application in the healthcare domain that aims to promote physical activity by randomly generating exercise tours. StepSteam is a computer-supported system for improving the attitudes of students toward fitness and increasing their sense of social support for fitness. Buttussi et al. designed a mobile phone application that dynamically presents virtual monsters, gold, and other items corresponding to such factors as users' heart rate, age, and exercise phase to encourage users to speed up or slow down. Cai et al. presented the foundation of a design framework used to integrate game elements to an application with location-based services for smartphones.
The above test methods for health-related physical fitness based on intelligent mobile terminals are mainly used in game application programs to increase the interest of youths in health testing and exercise training. These systems often lack scientificity and stringency and are inapplicable to the official and formal testing of the health-related physical fitness of college students. In this study, image pattern recognition and artificial intelligence technology are combined with evaluation standards of health-related physical fitness to explore an intelligent scientific test method for health-related physical fitness.
An intelligent comprehensive test system for the health-related physical fitness of college students based on intelligent mobile terminals (smartphones) is designed. Users can determine their health-related physical fitness levels by using their smartphones and performing standard test operations. A quantitative test result is relayed to users by the comprehensive analysis of an intelligent software in their smartphones. Thus, individuals can have an intuitive knowledge of their health-related physical fitness level. The system also provides exercise suggestions and methods to improve health-related physical fitness according to specific indexes of users. The test process of the proposed method is shown in Figure 1.
The proposed method divides the health-related physical fitness of college students into four modules (muscle fitness and muscular endurance fitness are integrated.), namely, cardiorespiratory fitness, muscle/muscular endurance fitness, flexibility fitness, and body composition. In the following subsections, the test methods of the different modules are elaborated.
The 1.6 km fast-paced walk, a common cardiorespiratory fitness test item [22], is chosen as the cardiorespiratory fitness test item of college students in this system. Maximum oxygen uptake (VO2max) is chosen as the major test index [23], which can be calculated according to the following equations: VO2max = 132.853 − (0.1695 × body weight) − (0.3877 × age) + (6.3150 × gender) − (3.2649 × time length) − (0.1565 × heart rate) [24]. The unit of body weight is kg, and the unit of age is years. The gender values are male = 1 and female = 0. The time length unit is min, and the heart unit is bpm. The unit of VO2max is ml/kg/min. Time length is the time needed to finish the 1.6 km fast-paced walk, which can be automatically timed and recorded into the system by phones. Heart rate is the heart rate tested at the first time after arriving at the destination of the 1.6 km fast-paced walk. This system measures heart rate by image processing of smartphones and without the assistance of other tools. Every heartbeat of the human body will make a waveform flow of blood in the blood capillary of a fingertip. At hyperemia of the blood capillary, the oxygen content in blood increases, and blood appears bright red. After the oxygen in blood is consumed, the blood becomes dark red. Thus, fingertip color changes are recorded by smartphones to detect the change period of colors. Then, the heartbeat period, that is, the heart rate, can be calculated [25,26]. In this system, a user places a fingertip at the camera of a smartphone and turns the flash lamp on to enhance the imaging effect. Image sensors in the phone camera are used to take pictures of blood color changes in the fingertip of the user. Heart rate is calculated after the detected image signals are denoised. The process of finger image analysis is shown in Figure 2.
The interfaces of heart detection and time-length record functions in this system are shown in Figures 3(a) and 3(b).
In this system, pull-up and sit-up are chosen as the test items for the muscle/muscular endurance fitness of male and female students, respectively. The number of pull-ups or sit-ups is used as the test index. The higher the number, the better the muscle/muscular endurance fitness. Users can accomplish some detection by manual counting and inputting data into smartphones.
This system uses the body anteflexion (sit-and-reach or stand-trunk-bending) as the test program for the flexibility fitness measurement of college students. Using smartphones, it directly measures the bending angle of users through image recognition to observe the flexibility fitness level. The specific process is as follows. Sitting or stand-trunk-bending images of users are captured by others or through a mirror from the side by phone cameras. The images are analyzed and processed by the system. Images are processed by calibration, marginal extraction, and feature point selection. The bending angle of users is calculated. The image acquisition and analysis processes are shown in Figure 4 The study participant in the figure is one of the researchers of this work, and he agreed to publish the images.
The following procedure is used for bending image recognition.
(1) Image preprocessing. The image is converted to grayscale.
(2) The edge of the human body is detected by an improved edge detector. The bilateral filter is used to filter an image to preserve its edge information, and then, a 3*3 template is used to calculate the gradient amplitude and direction of the image.
(3) Three feature points needed by the algorithm are extracted, and their coordinates are obtained from the image.
(4) The angle of the points is computed, and the result is outputted.
The body mass index (BMI) is used as the test index of the body composition of college students in the system. BMI, the proportion of weight to square of height (weight/square of height), is an important parameter that represents the composition of the human body [27]. Therefore, the BMI of users can be calculated by smartphones after the weight and height are entered into the system, and the body composition level is consequently reflected.
Different algorithms are designed in the system to score the results of detection items. With reference to national standards, relevant data and measurement experiment, the calculation formulas of the quantitative scores of the different modules are listed in Table 1.
Modules | Male | Female |
Cardiorespiratory fitness (V is the maximum oxygen uptake) | {0,V⩽385.8824(V - 38),38<V<55100,V⩾55 | {0,V⩽295.5556(V - 29),29<V<47100,V⩾47 |
Muscle/Muscular endurance fitness(N is number of accomplishment) | {0,N⩽29.0909(N−2),2<N<13100,N⩾13 | {0,N⩽162.6316(N−16),16<N<54100,N⩾54 |
Flexibility fitness(β is the bending angle) | {0,β⩾682.7778(68−β),32<β<68100,β⩽32 | {0,β⩾612.8571(61−β),26<β<61100,β⩽26 |
Body composition(B is the body mass index) | {0,B≤14∥B≥2814.2857(B−14),14<B<2114.2857(28−B),21<B<28 | {0,B≤14∥B≥2814.2857(B−14),14<B<2114.2857(28−B),21<B<28 |
The four modules are scored from 0 to 100, where 0 is the lowest, and 100 is the highest. Results are used as the user scores in the corresponding modules. The total points of the health-related physical fitness of users are the mean of scores of the four modules. Based on the abovementioned method, the system will detect the health-related physical fitness level of users. Furthermore, specific exercise suggestions and methods are given according to the scores of each module.
To verify the validity of the proposed method, a total of 116 undergraduates from Guangdong Polytechnic Normal University in China are chosen for the test. The correlation between the traditional artificial test results and results of the proposed method is compared. The experiments are carried out under the consent of Guangdong Polytechnic Normal University and in accordance with national regulations, and informed consent is obtained from all subjects. These undergraduates come from three majors, namely, automation (42), education (38), and music performance (36), with 59 males and 57 females aged between 18 and 22 (average 20.3 years old) included. A general description of the test respondents is shown in Table 2.
Sex | Age (years) | Height (cm) | Weight (kg) |
M (n = 59) | 20.5 ± 0.7 | 172.3 ± 6.4 | 61.8 ± 10.0 |
F (n = 57) | 20.0 ± 0.5 | 160.2 ± 5.2 | 50.4 ± 6.6 |
In terms of the proposed method, the muscle/muscular endurance fitness module and body composition module manually input test data into the intelligent terminals and hence do not involve the intelligent test technology. Therefore, the data of these modules are deemed consistent with those of the traditional method without the need for experimental comparison and verification. In this study, only the cardiorespiratory fitness and muscle/muscular endurance fitness modules are verified by experiments. In the experiment of the cardiorespiratory fitness module, the test results of 1000 (M)/800 (F) m run are compared with test results of the proposed method. In the flexibility fitness experiment, results of the traditional sit-and-reach test are compared with those of the proposed method. All experiments have reasonable intervals in the middle process and will not influence the physical or body performances of the respondents as well as the mutual influences of the different test items.
The cardiorespiratory fitness scores of the 116 college students, which are obtained from the traditional 1000 (M)/800 (F) m run and the 1.6 km fast-paced walk in the proposed method, are listed in Table 3.
Sex | Score of traditional method | Score of proposed method |
M (n = 59) | 69.6 ± 9.5 | 70.9 ± 9.7 |
F (n = 57) | 77.1 ± 9.9 | 77.7 ± 9.8 |
Test data of 10 males and 10 females are chosen randomly (Table 4).
No. | Sex | Age | 1000/800 m | Score of Traditional method | VO2max | Score of proposed method | Difference of scores |
1 | M | 19 | 4'11'' | 68 | 50.2 | 72 | 4 |
2 | M | 21 | 4'04'' | 70 | 50.6 | 74 | 4 |
3 | M | 20 | 3'38'' | 80 | 52.4 | 85 | 5 |
4 | M | 20 | 4'23'' | 62 | 48.9 | 64 | 2 |
5 | M | 22 | 4'03'' | 70 | 49.4 | 67 | 3 |
6 | M | 18 | 4'18'' | 66 | 49.6 | 68 | 2 |
7 | M | 21 | 3'40'' | 80 | 51.4 | 79 | 1 |
8 | M | 19 | 4'33'' | 50 | 47.0 | 53 | 3 |
9 | M | 19 | 3'43'' | 78 | 51.9 | 82 | 5 |
10 | M | 20 | 4'12'' | 68 | 48.9 | 64 | 4 |
11 | F | 18 | 3'18'' | 100 | 47.2 | 100 | 0 |
12 | F | 19 | 4'11'' | 68 | 42.0 | 72 | 4 |
13 | F | 20 | 3'32'' | 85 | 44.5 | 86 | 1 |
14 | F | 20 | 3'40'' | 80 | 42.9 | 77 | 3 |
15 | F | 19 | 4'01'' | 72 | 42.1 | 73 | 1 |
16 | F | 21 | 3'55'' | 74 | 42.7 | 76 | 2 |
17 | F | 22 | 3'44'' | 80 | 43.9 | 83 | 3 |
18 | F | 19 | 4'16'' | 66 | 40.7 | 65 | 1 |
19 | F | 18 | 3'59'' | 74 | 41.6 | 70 | 4 |
20 | F | 19 | 4'03'' | 72 | 42.5 | 75 | 3 |
The flexibility fitness scores of the 116 college students that are measured by the traditional method and the proposed method in the experiment are listed in Table 5.
Sex | Score of traditional method | Score of proposed method |
M (n = 59) | 74.6 ± 11.9 | 74.8 ± 11.5 |
F (n = 57) | 73.3 ± 11.5 | 75.0 ± 11.2 |
Test data of 10 males and 10 females are chosen randomly (Table 6).
No. | Sex | Age | Sit-and-reach tester | Score of Traditional method | Angle of body bending | Score of proposed method | Difference of scores |
1 | M | 20 | 6.9 | 64 | 43.2 | 69 | 5 |
2 | M | 18 | 16.2 | 76 | 39.6 | 79 | 3 |
3 | M | 21 | 16.3 | 74 | 39.2 | 80 | 6 |
4 | M | 20 | 7.3 | 64 | 46.0 | 61 | 3 |
5 | M | 19 | 11.1 | 70 | 43.5 | 68 | 2 |
6 | M | 22 | 21.2 | 85 | 38.8 | 81 | 4 |
7 | M | 19 | 6.3 | 62 | 44.6 | 65 | 3 |
8 | M | 21 | 27.3 | 100 | 31.2 | 100 | 0 |
9 | M | 19 | 20.5 | 85 | 39.6 | 79 | 6 |
10 | M | 20 | 9.9 | 68 | 44.6 | 65 | 2 |
11 | F | 21 | 9.4 | 64 | 40.0 | 60 | 4 |
12 | F | 19 | 16.7 | 76 | 32.3 | 82 | 6 |
13 | F | 20 | 8.9 | 64 | 37.9 | 66 | 2 |
14 | F | 19 | 20.7 | 85 | 29.8 | 89 | 4 |
15 | F | 20 | 18.5 | 78 | 31.5 | 85 | 7 |
16 | F | 18 | 6.9 | 60 | 38.9 | 63 | 3 |
17 | F | 22 | 23.2 | 90 | 30.2 | 88 | 2 |
18 | F | 20 | 8.5 | 62 | 37.5 | 67 | 5 |
19 | F | 19 | 8.9 | 64 | 36.8 | 69 | 5 |
20 | F | 20 | 22.1 | 85 | 32.3 | 82 | 3 |
In the cardiorespiratory fitness experiment, the quiet heart rate test, 3 min stepping test, 1.6 km fast-paced walk, and 12 m run are the test items that are appropriate for common college students [28,29]. Each of these test items has its own advantages and disadvantages. The quiet heart rate test is easy to operate, but it cannot completely and accurately reflect the cardiorespiratory fitness level. The 3 min stepping test has low requirements on fields, but it has high requirements on action standards of test objects. According to studies and practices, it is inapplicable to be used extensively to college students in China [30]. The 12 min run is a method of indirectly deducing the maximum oxygen uptake, and it has been used extensively in many countries. The time length of 12 min is a challenge to college students, and some students cannot accomplish it well. Therefore, the test results cannot meet the needs of our study. In this work, the 1.6 km fast-paced walk is chosen as the test item, and the maximum oxygen uptake is calculated according to the test results to evaluate the cardiorespiratory fitness level.
The 20 samples in Table 4 show that the cardiorespiratory fitness scores of the college students in the proposed system are close to those in the traditional method. The average difference is small (3.3 in males and 2.2 in females). To further verify the correlation between the data of the proposed and traditional methods and determine whether the data difference between the two methods has statistical significance, the test data are analyzed by SPSS. Bivariate correlation analysis is firstly conducted, and the Pearson's correlation coefficient is obtained. Two groups of data are matched for a T-test of the samples. The confidence level (%) is set at 95%, and the two-tailed significance is calculated. Results are shown in Table 7.
Sex | Pearson's correlation coefficient r | Significance (two-tailed) p |
M | 0.944 | 0.145 |
F | 0.965 | 0.489 |
Table 7 demonstrates that values of two r are relatively large, indicating a strong correlation between the two groups. The p values are higher than 0.05, implying no statistical significance of the difference between the two groups. Thus, the cardiorespiratory fitness test module in the proposed method is scientific and accurate.
Currently, flexibility fitness is mainly tested by mechanical sit-and-reach testers. The maximum stretching distance of fingers is used as the evaluation index. This test method, which replaces the joint motion angle by the stretching distance of fingers, has a considerable shortcoming because different arm lengths will influence the stretching distances of fingers, thereby increasing measurement error and reducing detection validity. Based on the correlation of body anteflexion with upper limbs, lower limbs, body, and angle, several researchers have identified certain unreasonable factors of the sit-and-reach and stand-trunk-bending test methods [31,32,33]. If the moving angles of body joints can be measured, the effects of limb length on measurement validity can be overcome. The image recognition technology used in the proposed method directly detects the bending angle of the human body. This innovation of flexibility fitness testing presents a certain advancement.
Table 6 shows that the flexibility fitness scores of the proposed method are close to those of the traditional method. The mean difference is small (3.4 for males and 4.1 for females). Test results of the traditional sit-and-reach tester are sensitive to the limb length of the test objects, and the test results are not completely accurate. However, as the standard physical fitness test item of students in many countries, it is still authoritative. In this work, correlation and difference between the data of the two methods are tested by SPSS. First, bivariate correlation is analyzed, and Pearson's coefficient is calculated. The two groups of data are matched for a T-test of the samples. The confidence level (%) is set at 95% and two-tailed significance is calculated. Results are listed in Table 8.
Sex | Pearson's correlation coefficient r | Significance(two-tailed) p |
M | 0.944 | 0.941 |
F | 0.938 | 0.098 |
Table 8 illustrates that the r values are relatively large, indicating a strong correlation between the data of the two methods. The difference between the two groups of data is not statistically significant, as the two p values are higher than 0.05. Therefore, the flexibility fitness module in the proposed system is scientific and accurate.
The current situations regarding the evaluation standards and testing technologies of health-related physical fitness are analyzed. The significance of mobile Internet in health-related physical fitness is discussed. A test method of the health-related physical fitness of college students that is based on mobile Internet is designed. In this method, the camera of a mobile phone is used to capture the finger and bending images of users, and the calculation function of the mobile phone is used to obtain the measurements of the users' heart rate, flexibility, and other parameters. Then, we can determine the cardiorespiratory fitness, muscle fitness, muscular endurance fitness, flexibility fitness, and body composition of the users. Experimental results demonstrate that the proposed method can accurately test the health-related physical fitness of college students. The test data have a strong correlation with authoritative traditional data, and no statistically significant difference between the two methods is observed. The proposed system can change the current situations characterized by heavy dependence on complicated professional instruments and current data management depending on artificial records and inputs. It also effectively improves the management efficiency of the physical fitness testing of college students. Moreover, this method can encourage college students to participate in health-related physical fitness testing and help them determine their health-related physical fitness level at any time by using smartphones. Eventually, this technology can stimulate the enthusiasm of college students toward sports exercises and improve their physical health.
This work is supported in part by the National Natural Science Foundation of China (61802073), the Key Scientific and Technological Planning Project of Guangzhou, China (201704020113), and the Scientific and Technological Project of Guangdong Province, China (2017A040405059).
The authors declare no conflict of interest in this paper.
[1] | J. E. Cooper, On the publication of the diagnostic and statistical manual of mental disorders: Fourth edition (dsm-iv), Br. J. Psychiat., 166 (1995), 4–8. |
[2] | M. Basso, J. Yang, L. Warren, et al., Volumetry of amygdala and hippocampus and memory performance in alzheimer's disease, Psychiat. Res., 146 (2006), 251–261. |
[3] | C. I. Wright, B. C. Dickerson, E. Feczko, et al., A functional magnetic resonance imaging study of amygdala responses to human faces in aging and mild alzheimers disease, Biol. Psychiat., 62 (2007), 1388–1395. |
[4] | A. Drzezga, Diagnosis of alzheimer's disease with [18f] pet in mild and asymptomatic stages, Behav. Neurol., 21 (2009), 101–115. |
[5] | P. H. Tsai, C. Lin, J. Tsao, et al., Empirical mode decomposition based detrended sample entropy in electroencephalography for alzheimer's disease, J. Neurosci. Methods, 210 (2012), 230–237. |
[6] | H. Zhang, C. L. Wong and P. Shi, Estimation of cardiac electrical propagation from medical image sequence, 2006 9th International Conference on Medical Image Computing and Computer-assisted Intervention (Copenhagen), Springer, (2006), 528–535. |
[7] | K. D. Tzimourta, N. Giannakeas, A. T. Tzallas, et al., Eeg window length evaluation for the detection of alzheimer's disease over different brain regions, Brain Sci., 9 (2019), Article 81. |
[8] | C. Coronel, H. Garn, M. Waser, et al., Quantitative eeg markers of entropy and auto mutual information in relation to mmse scores of probable alzheimer's disease patients, Entropy, 19 (2017), 1099–4300. |
[9] | J. Poza, C. Gmez, M. Garca, et al., Spatio-Temporal fluctuations of neural dynamics in mild cognitive impairment and alzheimer's disease, Curr. Alzheimer Res., 14 (2017), 924–936. |
[10] | N. Emanuel, B. Felix, A. Harald, et al., Regularized linear discriminant analysis of eeg features in dementia patients, Front. Aging Neurosci., 8 (2016), Article 273. |
[11] | M. Dottori, L. Sedeo, M. C. Martorell, et al., Towards affordable biomarkers of frontotemporal dementia: a classification study via networks information sharing, Sci. Rep., 7 (2017), Article 3822. |
[12] | N. N. Kulkarni and V. K. Bairagi, Extracting salient features for eeg-based diagnosis of alzheimer's disease using support vector machine classifier, IETE J. Res., 63 (2016), 11–22. |
[13] | T. H. Falk, F. J. Fraga, L. Trambaiolli, et al., Eeg amplitude modulation analysis for semi-automated diagnosis of alzheimers disease, EURASIP J. Adv. Signal Process., 2014 (2014), Arti-cle 192. |
[14] | S. Nobukawa, T. Yamanishi, H. Nishimura, et al., Atypical temporal-scale-specific fractal changes in alzheimers disease eeg and their relevance to cognitive decline, Cogn. Neurodynamics, 13 (2019), 1–11. |
[15] | Y. Chen, L. Cai, R. Wang, et al., Dcca cross-correlation coefficients reveals the change of both synchronization and oscillation in eeg of alzheimer disease patients, Physica A, 490 (2017), 171–184. |
[16] | H. Zhang, Z. Gao, L. Xu, et al., A meshfree representation for cardiac medical image computing, IEEE J. Transl. Eng. Health. Med., 6 (2018), 1800212. |
[17] | H. Zhang, H. Ye and W. Huang, A meshfree method for simulating myocardial electrical activity, Comput. Math. Methods. Med., 2012 (2012), 1–16. |
[18] | A. I. Triggiani, V. Bevilacqua, A. Brunetti, et al.,Classification of healthy subjects and alzheimer's disease patients with dementia from cortical sources of resting state eeg rhythms: a study using artificial neural networks, Front. Neurosci., 10 (2016), Article 604. |
[19] | W. He, J. Zhu and H. Yang, Contrastive analysis of correlation dimension of eeg signals between normal and pathological groups, 2008 World Automation Congress (Hawaii), IEEE, 2008. |
[20] | Z. Hu and P. Shi, Regularity and complexity of human electroencephalogram dynamics: ap-plications to diagnosis of alzheimers disease, 2006 18th International Conference on Pattern Recognition, IEEE CS, 2006. |
[21] | L. Tylov, J. Kukal, V. Hubata-Vacek, et al., Unbiased estimation of permutation entropy in eeg analysis for alzheimer's disease classification, Biomed. Signal Process. Control, 39 (2018), 424–430. |
[22] | J. S. Richman and J. R. Moorman, Physiological time-series analysis using approximate entropy and sample entropy, AM. J. Physiol-Heart C., 278 (2000), H2039–H2049. |
[23] | C. Gomez C, F. Vaquerizo-Villar, J. Poza, et al., Bispectral analysis of spontaneous eeg activity from patients with moderate dementia due to alzheimer's disease, 2017 39th Annual International Conference of the IEEE Engineering in Medicine and Biology Society, IEEE, (2017), 422–425. |
[24] | D. Labate, F. L. Foresta, G. Morabito, et al., Entropic measures of eeg complexity in alzheimer's disease through a multivariate multiscale approach, IEEE Sens. J., 13 (2013), 3284–3292. |
[25] | P. Espino, S. Simons and D. Absolo, Fuzzy Entropy analysis of the electroencephalogram in patients with alzheimer's disease: Is the method superior to Sample Entropy?, Entropy, 20 (2018). |
[26] | C. Ying and T. D. Pham, Sample entropy and regularity dimension in complexity analysis of cortical surface structure in early alzheimer's disease and aging, J. Neurosci. Methods, 215 (2013), 210–217. |
[27] | E. V. S. Luiz and L. M. Otavio, Evaluation of physiologic complexity in time series using gener-alized sample entropy and surrogate data analysis, Chaos, 22 (2012), 479–487. |
[28] | F. Onorati, L. T. Mainardi, F. Sirca, et al., Nonlinear analysis of pupillary dynamics, Biomed. Eng., 61 (2016), 95–106. |
[29] | J. Theiler, E. Stephen, A. Longtin, et al., Testing for nonlinearity in time series: The method of surrogate data, Physica D, 58 (1992), 77–94. |
[30] | P. Shang, Y. Wu and Y. Li, Modified generalized multiscale sample entropy and surrogate data analysis for financial time series, Nonlinear Dyn., 92 (2018), 1335–1350. |
[31] | P. Shang, M. Xu and J.Huang, Modified generalized sample entropy and surrogate data analysis for stock markets, Commun. Nonlinear Sci., 35 (2016), 17–24. |
[32] | M. Xu, D. Shang and P.Shang, Generalized sample entropy analysis for traffic signals based on similarity measure, Physica A, 474 (2017), 1–7. |
[33] | T. Schreiber and A. Schmitz,Surrogate time series, Physica D, 142 (1999), 346–382. |
[34] | C. P. Pan, B. Zheng, Y. Wu, et al., Detrended fluctuation analysis of human brain electroen-cephalogram, Phys. Lett. A, 329 (2004), 130–135. |
[35] | G. Liu, Y. Zhang, Z. Hu, et al.,Complexity analysis of electroencephalogram dynamics in patients with parkinson's disease, Parkinsons Dis., 2017 (2017), 1–9. |
[36] | D. E. Lake, J. S. Richman, M. P. Griffin, et al., Sample entropy analysis of neonatal heart rate variability, Am. J. Physiol. Regul. Integr. Comp. Physiol., 283 (2002), Article R789. |
[37] | X. J. Tang, S. Zhuo, Y. Zhuo, et al., Entropy measures of erp recordings for dual tasks in man, Acta. Biophysica. Sinica., 21 (2005), 371–376. |
[38] | J. Theiler and P. E. Rapp, Re-examination of the evidence for low-dimensional, nonlinear structure in the human electroencephalogram, Electroencephalogr. Clin. Neurophysiol. Suppl. , 98 (1996), 213–222. |
[39] | L. Gemma, I. Dmytro, P. Aleksandra, et al., Surrogate data for hypothesis testing of physical systems, Phys. Rep., 748 (2018), 1–72. |
[40] | W. Chantarangsi, W. Liu, F. Bretz, et al., Normal probability plots with confidence, Biom. J., 57 (2015), 52–63. |
[41] | D. Absolo, J. Escudero, R. Hornero, et al., Approximate entropy and auto mutual information analysis of the electroencephalogram in Alzheimers disease patients, Med. Biol. Eng. Comput., 46 (2008), 1019–1028. |
[42] | D. Abasolo, R. Hornero, P. Espino, et al., Entropy analysis of the eeg background activity in alzheimer's disease patients, Physiol. Meas., 27 (2006), 241–253. |
[43] | P. Alberto, G. R. Tomaso, E. Tobaldini, et al., Progressive decrease of heart period variability entropy-based complexity during graded head-up tilt, J. Appl. Physiol., 103 (2007), 1143–1149. |
1. | Andrea Ehrmann, Tomasz Błachowicz, Solarstrom aus Früchtetee, 2020, 51, 0031-9252, 196, 10.1002/piuz.202001578 | |
2. | Fahimeh Hooriabad Saboor, Tuan Anh Nguyen, 2021, 9780128215487, 435, 10.1016/B978-0-12-821548-7.00016-6 | |
3. | Chuang Han, Yue-Hua Li, Ming-Yu Qi, Fan Zhang, Zi-Rong Tang, Yi-Jun Xu, Surface/Interface Engineering of Carbon‐Based Materials for Constructing Multidimensional Functional Hybrids, 2020, 4, 2367-198X, 1900577, 10.1002/solr.201900577 | |
4. | Sophia Kohn, Daria Wehlage, Irén Juhász Junger, Andrea Ehrmann, Electrospinning a Dye-Sensitized Solar Cell, 2019, 9, 2073-4344, 975, 10.3390/catal9120975 | |
5. | Jan Lukas Storck, Marius Dotter, Bennet Brockhagen, Timo Grothe, Evaluation of Novel Glycerol/PEO Gel Polymer Electrolytes for Non-Toxic Dye-Sensitized Solar Cells with Natural Dyes Regarding Long-Term Stability and Reproducibility, 2020, 10, 2073-4352, 1158, 10.3390/cryst10121158 | |
6. | Lina Jatautė, Valentina Krylova, Nijolė Dukštienė, Martynas Lelis, Simona Tučkutė, Ag-In-Se films on flexible architectural textiles as efficient material for optoelectronics applications: A preliminary study, 2021, 721, 00406090, 138566, 10.1016/j.tsf.2021.138566 | |
7. | Jan Storck, Marius Dotter, Sonia Adabra, Michelle Surjawidjaja, Bennet Brockhagen, Timo Grothe, Long-Term Stability Improvement of Non-Toxic Dye-Sensitized Solar Cells via Poly(ethylene oxide) Gel Electrolytes for Future Textile-Based Solar Cells, 2020, 12, 2073-4360, 3035, 10.3390/polym12123035 | |
8. | Tina Harifi, 2021, 9780128207772, 125, 10.1016/B978-0-12-820777-2.00008-X | |
9. | José F. Serrano-Claumarchirant, Isaac Brotons-Alcázar, Mario Culebras, Maria J. Sanchis, Andrés Cantarero, Rafael Muñoz-Espí, Clara M. Gómez, Electrochemical Synthesis of an Organic Thermoelectric Power Generator, 2020, 12, 1944-8244, 46348, 10.1021/acsami.0c12076 | |
10. | Achala Satharasinghe, Theodore Hughes-Riley, Tilak Dias, A Review of Solar Energy Harvesting Electronic Textiles, 2020, 20, 1424-8220, 5938, 10.3390/s20205938 | |
11. | Al Mamun, Marah Trabelsi, Michaela Klöcker, Lilia Sabantina, Christina Großerhode, Tomasz Blachowicz, Georg Grötsch, Carsten Cornelißen, Almuth Streitenberger, Andrea Ehrmann, Electrospun Nanofiber Mats with Embedded Non-Sintered TiO2 for Dye-Sensitized Solar Cells (DSSCs), 2019, 7, 2079-6439, 60, 10.3390/fib7070060 | |
12. | Steffen Müller, David Wieschollek, Irén Juhász Junger, Eva Schwenzfeier-Hellkamp, Andrea Ehrmann, Back electrodes of dye-sensitized solar cells on textile fabrics, 2019, 198, 00304026, 163243, 10.1016/j.ijleo.2019.163243 | |
13. | Effect of Caffeine Copigmentation of Anthocyanin Dyes on DSSC Efficiency, 2019, 12, 1996-1944, 2692, 10.3390/ma12172692 | |
14. | Tomasz Blachowicz, Andrea Ehrmann, Recent Developments of Solar Cells from PbS Colloidal Quantum Dots, 2020, 10, 2076-3417, 1743, 10.3390/app10051743 | |
15. | Mohammad Mamunur Rashid, Barbara Simončič, Brigita Tomšič, Recent advances in TiO2-functionalized textile surfaces, 2021, 22, 24680230, 100890, 10.1016/j.surfin.2020.100890 | |
16. | Katrin Gossen, Marius Dotter, Bennet Brockhagen, Jan Lukas Storck, Andrea Ehrmann, Long-term investigation of unsealed DSSCs with glycerol-based electrolytes of different compositions, 2022, 9, 2372-0484, 283, 10.3934/matersci.2022017 | |
17. | Marius Dotter, Jan Lukas Storck, Michelle Surjawidjaja, Sonia Adabra, Timo Grothe, Investigation of the Long-Term Stability of Different Polymers and Their Blends with PEO to Produce Gel Polymer Electrolytes for Non-Toxic Dye-Sensitized Solar Cells, 2021, 11, 2076-3417, 5834, 10.3390/app11135834 | |
18. | Seyedeh Nooshin Banitaba, Andrea Ehrmann, Application of Electrospun Nanofibers for Fabrication of Versatile and Highly Efficient Electrochemical Devices: A Review, 2021, 13, 2073-4360, 1741, 10.3390/polym13111741 | |
19. | Shayan Seyedin, Tian Carey, Adrees Arbab, Ladan Eskandarian, Sivasambu Bohm, Jong Min Kim, Felice Torrisi, Fibre electronics: towards scaled-up manufacturing of integrated e-textile systems, 2021, 13, 2040-3364, 12818, 10.1039/D1NR02061G | |
20. | Amjid Rafique, Isabel Ferreira, Ghulam Abbas, Ana Catarina Baptista, Recent Advances and Challenges Toward Application of Fibers and Textiles in Integrated Photovoltaic Energy Storage Devices, 2023, 15, 2311-6706, 10.1007/s40820-022-01008-y | |
21. | Mariya Aleksandrova, Ivailo Pandiev, Ajaya Kumar Singh, Implementation of 3ω Method for Studying the Thermal Conductivity of Perovskite Thin Films, 2022, 12, 2073-4352, 1326, 10.3390/cryst12101326 | |
22. | Thennakoon Mudiyanselage Wijendra J Bandara, Jayamaha Mudalige Chandi Hansadi, Federico Bella, A review of textile dye-sensitized solar cells for wearable electronics, 2022, 28, 0947-7047, 2563, 10.1007/s11581-022-04582-8 | |
23. | Yong Zhang, Huimin Wang, Haojie Lu, Shuo Li, Yingying Zhang, Electronic fibers and textiles: Recent progress and perspective, 2021, 24, 25890042, 102716, 10.1016/j.isci.2021.102716 | |
24. | Sujin Cha, Eugene Lee, Gilsoo Cho, Fabrication of Poly(3,4-ethylenedioxythiophene):Poly(styrenesulfonate)/Poly(vinylidene fluoride) Nanofiber-Web-Based Transparent Conducting Electrodes for Dye-Sensitized Photovoltaic Textiles, 2021, 13, 1944-8244, 28855, 10.1021/acsami.1c06081 | |
25. | Marius Dotter, Lion Lukas Placke, Jan Lukas Storck, Uwe Güth, Characterization of PAN-TiO2 Nanofiber Mats and their Application as Front Electrodes for Dye-sensitized Solar Cells, 2022, 65, 2350-3696, 298, 10.14502/tekstilec.65.2022081 | |
26. | Fabian Schoden, Marius Dotter, Dörthe Knefelkamp, Tomasz Blachowicz, Eva Schwenzfeier Hellkamp, Review of State of the Art Recycling Methods in the Context of Dye Sensitized Solar Cells, 2021, 14, 1996-1073, 3741, 10.3390/en14133741 | |
27. | Nitasha Chaudhari, Sanjay Darvekar, Paresh Nasikkar, Atul Kulkarni, Chandrakant Tagad, Recent developments on green synthesised nanomaterials and their application in dye-sensitised solar cells, 2022, 43, 0143-0750, 7133, 10.1080/01430750.2022.2063185 | |
28. | Tomasz Blachowicz, Dariusz Wójcik, Maciej Surma, Mirosław Magnuski, Guido Ehrmann, Andrea Ehrmann, Textile Fabrics as Electromagnetic Shielding Materials—A Review of Preparation and Performance, 2023, 11, 2079-6439, 29, 10.3390/fib11030029 | |
29. | Iftikhar Ali, Md Rashedul Islam, Junyi Yin, Stephen J. Eichhorn, Jun Chen, Nazmul Karim, Shaila Afroj, Advances in Smart Photovoltaic Textiles, 2024, 18, 1936-0851, 3871, 10.1021/acsnano.3c10033 | |
30. | 2024, 9783527350551, 201, 10.1002/9783527838240.ch10 | |
31. | Jonathan Plentz, Uwe Brückner, Gabriele Schmidl, Annett Gawlik, Klaus Richter, Gudrun Andrä, Solar Fabric Based on Amorphous Silicon Thin Film Solar Cells on Flexible Textiles, 2025, 18, 1996-1073, 1448, 10.3390/en18061448 | |
32. | Endah Purnomosari, Valentinus Galih Vidia Putra, Isom Hilmi, Dye-sensitized solar cells incorporating PVA/TiO 2 nanofibers, PVA/LiOH gel electrolyte, and natural dye for sustainable energy harvesting , 2025, 0040-5000, 1, 10.1080/00405000.2025.2521565 |
Modules | Male | Female |
Cardiorespiratory fitness (V is the maximum oxygen uptake) | {0,V⩽385.8824(V - 38),38<V<55100,V⩾55 | {0,V⩽295.5556(V - 29),29<V<47100,V⩾47 |
Muscle/Muscular endurance fitness(N is number of accomplishment) | {0,N⩽29.0909(N−2),2<N<13100,N⩾13 | {0,N⩽162.6316(N−16),16<N<54100,N⩾54 |
Flexibility fitness(β is the bending angle) | {0,β⩾682.7778(68−β),32<β<68100,β⩽32 | {0,β⩾612.8571(61−β),26<β<61100,β⩽26 |
Body composition(B is the body mass index) | {0,B≤14∥B≥2814.2857(B−14),14<B<2114.2857(28−B),21<B<28 | {0,B≤14∥B≥2814.2857(B−14),14<B<2114.2857(28−B),21<B<28 |
Sex | Age (years) | Height (cm) | Weight (kg) |
M (n = 59) | 20.5 ± 0.7 | 172.3 ± 6.4 | 61.8 ± 10.0 |
F (n = 57) | 20.0 ± 0.5 | 160.2 ± 5.2 | 50.4 ± 6.6 |
Sex | Score of traditional method | Score of proposed method |
M (n = 59) | 69.6 ± 9.5 | 70.9 ± 9.7 |
F (n = 57) | 77.1 ± 9.9 | 77.7 ± 9.8 |
No. | Sex | Age | 1000/800 m | Score of Traditional method | VO2max | Score of proposed method | Difference of scores |
1 | M | 19 | 4'11'' | 68 | 50.2 | 72 | 4 |
2 | M | 21 | 4'04'' | 70 | 50.6 | 74 | 4 |
3 | M | 20 | 3'38'' | 80 | 52.4 | 85 | 5 |
4 | M | 20 | 4'23'' | 62 | 48.9 | 64 | 2 |
5 | M | 22 | 4'03'' | 70 | 49.4 | 67 | 3 |
6 | M | 18 | 4'18'' | 66 | 49.6 | 68 | 2 |
7 | M | 21 | 3'40'' | 80 | 51.4 | 79 | 1 |
8 | M | 19 | 4'33'' | 50 | 47.0 | 53 | 3 |
9 | M | 19 | 3'43'' | 78 | 51.9 | 82 | 5 |
10 | M | 20 | 4'12'' | 68 | 48.9 | 64 | 4 |
11 | F | 18 | 3'18'' | 100 | 47.2 | 100 | 0 |
12 | F | 19 | 4'11'' | 68 | 42.0 | 72 | 4 |
13 | F | 20 | 3'32'' | 85 | 44.5 | 86 | 1 |
14 | F | 20 | 3'40'' | 80 | 42.9 | 77 | 3 |
15 | F | 19 | 4'01'' | 72 | 42.1 | 73 | 1 |
16 | F | 21 | 3'55'' | 74 | 42.7 | 76 | 2 |
17 | F | 22 | 3'44'' | 80 | 43.9 | 83 | 3 |
18 | F | 19 | 4'16'' | 66 | 40.7 | 65 | 1 |
19 | F | 18 | 3'59'' | 74 | 41.6 | 70 | 4 |
20 | F | 19 | 4'03'' | 72 | 42.5 | 75 | 3 |
Sex | Score of traditional method | Score of proposed method |
M (n = 59) | 74.6 ± 11.9 | 74.8 ± 11.5 |
F (n = 57) | 73.3 ± 11.5 | 75.0 ± 11.2 |
No. | Sex | Age | Sit-and-reach tester | Score of Traditional method | Angle of body bending | Score of proposed method | Difference of scores |
1 | M | 20 | 6.9 | 64 | 43.2 | 69 | 5 |
2 | M | 18 | 16.2 | 76 | 39.6 | 79 | 3 |
3 | M | 21 | 16.3 | 74 | 39.2 | 80 | 6 |
4 | M | 20 | 7.3 | 64 | 46.0 | 61 | 3 |
5 | M | 19 | 11.1 | 70 | 43.5 | 68 | 2 |
6 | M | 22 | 21.2 | 85 | 38.8 | 81 | 4 |
7 | M | 19 | 6.3 | 62 | 44.6 | 65 | 3 |
8 | M | 21 | 27.3 | 100 | 31.2 | 100 | 0 |
9 | M | 19 | 20.5 | 85 | 39.6 | 79 | 6 |
10 | M | 20 | 9.9 | 68 | 44.6 | 65 | 2 |
11 | F | 21 | 9.4 | 64 | 40.0 | 60 | 4 |
12 | F | 19 | 16.7 | 76 | 32.3 | 82 | 6 |
13 | F | 20 | 8.9 | 64 | 37.9 | 66 | 2 |
14 | F | 19 | 20.7 | 85 | 29.8 | 89 | 4 |
15 | F | 20 | 18.5 | 78 | 31.5 | 85 | 7 |
16 | F | 18 | 6.9 | 60 | 38.9 | 63 | 3 |
17 | F | 22 | 23.2 | 90 | 30.2 | 88 | 2 |
18 | F | 20 | 8.5 | 62 | 37.5 | 67 | 5 |
19 | F | 19 | 8.9 | 64 | 36.8 | 69 | 5 |
20 | F | 20 | 22.1 | 85 | 32.3 | 82 | 3 |
Sex | Pearson's correlation coefficient r | Significance (two-tailed) p |
M | 0.944 | 0.145 |
F | 0.965 | 0.489 |
Sex | Pearson's correlation coefficient r | Significance(two-tailed) p |
M | 0.944 | 0.941 |
F | 0.938 | 0.098 |
Modules | Male | Female |
Cardiorespiratory fitness (V is the maximum oxygen uptake) | {0,V⩽385.8824(V - 38),38<V<55100,V⩾55 | {0,V⩽295.5556(V - 29),29<V<47100,V⩾47 |
Muscle/Muscular endurance fitness(N is number of accomplishment) | {0,N⩽29.0909(N−2),2<N<13100,N⩾13 | {0,N⩽162.6316(N−16),16<N<54100,N⩾54 |
Flexibility fitness(β is the bending angle) | {0,β⩾682.7778(68−β),32<β<68100,β⩽32 | {0,β⩾612.8571(61−β),26<β<61100,β⩽26 |
Body composition(B is the body mass index) | {0,B≤14∥B≥2814.2857(B−14),14<B<2114.2857(28−B),21<B<28 | {0,B≤14∥B≥2814.2857(B−14),14<B<2114.2857(28−B),21<B<28 |
Sex | Age (years) | Height (cm) | Weight (kg) |
M (n = 59) | 20.5 ± 0.7 | 172.3 ± 6.4 | 61.8 ± 10.0 |
F (n = 57) | 20.0 ± 0.5 | 160.2 ± 5.2 | 50.4 ± 6.6 |
Sex | Score of traditional method | Score of proposed method |
M (n = 59) | 69.6 ± 9.5 | 70.9 ± 9.7 |
F (n = 57) | 77.1 ± 9.9 | 77.7 ± 9.8 |
No. | Sex | Age | 1000/800 m | Score of Traditional method | VO2max | Score of proposed method | Difference of scores |
1 | M | 19 | 4'11'' | 68 | 50.2 | 72 | 4 |
2 | M | 21 | 4'04'' | 70 | 50.6 | 74 | 4 |
3 | M | 20 | 3'38'' | 80 | 52.4 | 85 | 5 |
4 | M | 20 | 4'23'' | 62 | 48.9 | 64 | 2 |
5 | M | 22 | 4'03'' | 70 | 49.4 | 67 | 3 |
6 | M | 18 | 4'18'' | 66 | 49.6 | 68 | 2 |
7 | M | 21 | 3'40'' | 80 | 51.4 | 79 | 1 |
8 | M | 19 | 4'33'' | 50 | 47.0 | 53 | 3 |
9 | M | 19 | 3'43'' | 78 | 51.9 | 82 | 5 |
10 | M | 20 | 4'12'' | 68 | 48.9 | 64 | 4 |
11 | F | 18 | 3'18'' | 100 | 47.2 | 100 | 0 |
12 | F | 19 | 4'11'' | 68 | 42.0 | 72 | 4 |
13 | F | 20 | 3'32'' | 85 | 44.5 | 86 | 1 |
14 | F | 20 | 3'40'' | 80 | 42.9 | 77 | 3 |
15 | F | 19 | 4'01'' | 72 | 42.1 | 73 | 1 |
16 | F | 21 | 3'55'' | 74 | 42.7 | 76 | 2 |
17 | F | 22 | 3'44'' | 80 | 43.9 | 83 | 3 |
18 | F | 19 | 4'16'' | 66 | 40.7 | 65 | 1 |
19 | F | 18 | 3'59'' | 74 | 41.6 | 70 | 4 |
20 | F | 19 | 4'03'' | 72 | 42.5 | 75 | 3 |
Sex | Score of traditional method | Score of proposed method |
M (n = 59) | 74.6 ± 11.9 | 74.8 ± 11.5 |
F (n = 57) | 73.3 ± 11.5 | 75.0 ± 11.2 |
No. | Sex | Age | Sit-and-reach tester | Score of Traditional method | Angle of body bending | Score of proposed method | Difference of scores |
1 | M | 20 | 6.9 | 64 | 43.2 | 69 | 5 |
2 | M | 18 | 16.2 | 76 | 39.6 | 79 | 3 |
3 | M | 21 | 16.3 | 74 | 39.2 | 80 | 6 |
4 | M | 20 | 7.3 | 64 | 46.0 | 61 | 3 |
5 | M | 19 | 11.1 | 70 | 43.5 | 68 | 2 |
6 | M | 22 | 21.2 | 85 | 38.8 | 81 | 4 |
7 | M | 19 | 6.3 | 62 | 44.6 | 65 | 3 |
8 | M | 21 | 27.3 | 100 | 31.2 | 100 | 0 |
9 | M | 19 | 20.5 | 85 | 39.6 | 79 | 6 |
10 | M | 20 | 9.9 | 68 | 44.6 | 65 | 2 |
11 | F | 21 | 9.4 | 64 | 40.0 | 60 | 4 |
12 | F | 19 | 16.7 | 76 | 32.3 | 82 | 6 |
13 | F | 20 | 8.9 | 64 | 37.9 | 66 | 2 |
14 | F | 19 | 20.7 | 85 | 29.8 | 89 | 4 |
15 | F | 20 | 18.5 | 78 | 31.5 | 85 | 7 |
16 | F | 18 | 6.9 | 60 | 38.9 | 63 | 3 |
17 | F | 22 | 23.2 | 90 | 30.2 | 88 | 2 |
18 | F | 20 | 8.5 | 62 | 37.5 | 67 | 5 |
19 | F | 19 | 8.9 | 64 | 36.8 | 69 | 5 |
20 | F | 20 | 22.1 | 85 | 32.3 | 82 | 3 |
Sex | Pearson's correlation coefficient r | Significance (two-tailed) p |
M | 0.944 | 0.145 |
F | 0.965 | 0.489 |
Sex | Pearson's correlation coefficient r | Significance(two-tailed) p |
M | 0.944 | 0.941 |
F | 0.938 | 0.098 |