Special Issue: Computational Methods for Process Mining in Healthcare
Guest Editors
Prof. Wil van der Aalst
Process and Data Science chair (PADS), RWTH Aachen University, Ahornstraße 55, 52074 Aachen, Germany
Email: wvdaalst@pads.rwth-aachen.de
Prof. Davide Aloini
Department of Energy, Systems, Territory and Construction Engineering, University of Pisa, Largo Lucio Lazzarino 1, 56122, Pisa, Italy.
Email: davide.aloini@unipi.it
Elisabetta Benevento
Department of Energy, Systems, Territory and Construction Engineering, University of Pisa, Largo Lucio Lazzarino 1, 56122, Pisa, Italy.
Email: elisabetta.benevento@ing.unipi.it
Marco Pegoraro
Process and Data Science chair (PADS), RWTH Aachen University, Ahornstraße 55, 52074 Aachen, Germany
Email: pegoraro@pads.rwth-aachen.de
Manuscript Topics
Process mining [1] is a branch of data science that enables data-driven analytics based on discrete event data. Through recordings of past process executions collected in event logs, process mining allows for the automatic discovery of process models and for the measurements of potential deviations between normative models and real-life execution of processes. With the steep increase in demand of domain-specific, multi-perspective data science and analytics in industry, commercial tools based on process mining are enjoying financial success: while in 2018 the process mining market was assessed to amount to between 160 and 183 million USD [2, 3], some projections estimate that this figure will grow tenfold by 2023 [3]. While process mining techniques have been developed in the context of business processes, recent years have also seen a proliferation of process mining techniques in a number of new fields of application. One of such fields is healthcare. A wide array of process mining approaches for analyzing both clinical and administrative data from the healthcare domain have now been introduced, and the application of process science techniques to the healthcare and medical domains is now an established and rapidly-growing branch of data science [4–8]. In light of all the above, this Special Issue invites the community of process mining researchers to submit novel scientific results on the topic of Computational Methods for Process Mining in Healthcare. Papers submitted should introduce new frameworks for clinical and organizational healthcare event data analysis, or obtain novel significant insights on the context of a case study in the healthcare domain.
Specific topics of interest include (but are not limited to):
● Process Model Discovery: Development of novel techniques for discovering process models from healthcare event data, including innovative formalisms and perspectives. The presented approaches should contribute to solving known challenges in process discovery (noise, missing data, concurrency) in the healthcare context.
● Conformance Checking and Diagnostics: Development of methods to measure deviations between a normative process model, designed on the basis of domain knowledge provided by healthcare professionals, and real-life data recorded by information systems of hospitals and healthcare facilities. Includes proposals of techniques for anomalies detection within the control flow of a healthcare process, as well as diagnostic methodologies in the context of processes.
● Process Model Enhancement: Proposals for extensions of existing formalisms for process models with additional information extracted from the process, in order to enrich the semantics of a process model.
● Predictive Monitoring and Pattern Detection: Adoption of techniques from machine learning, data mining and statistics, adapted for deployment on a process management setting, which enable the prediction of specific process features. Includes supervised (predictive monitoring) and unsupervised (clustering, pattern recognition/detection) approaches for clinical and medical applications, such as disease trajectory estimation.
● Decision Mining, Recommendation and Operational Support: Proposals for methodologies that, deployed within healthcare organizations and facilities, provide real-time support for managing decisions within the workflow of a healthcare process.
● Data Quality and Management: Description of novel techniques for anomaly detection in healthcare event data, as well as techniques for filtering, repairing, correcting, normalizing, managing and storing event data related to a healthcare process.
● Responsible Data Science Applications: Development of process mining and analysis techniques that incorporate the Fairness, Accuracy, Confidentiality and Transparency principles (FACT) within the domain of healthcare.
● Case Study: A report on insights and findings from the application of existing process-oriented data science on healthcare data. The datasets subject of the study may consist of clinical data, logistic data, administrative data, or a combination thereof.
● Comparative Data Analysis: A report on an analytical comparison between a number of logs of events recorded in different organizations or institutions (hospitals, laboratories, healthcare facilities and organizations) in the context of analogous processes. The comparative study might be qualitative and/or quantitative, and should result in insights on the difference between the executions of similar processes in different organizations and countries.
● Review: A meta-analysis of recent scientific results within the topic of process sciences applied to the healthcare domain, providing a qualitative and/or quantitative discussion on contemporary advancements in process and data science techniques in the domains of healthcare and medicine.
References
1. van der Aalst, Wil M.P., “Process Mining: Data Science in Action”. Heidelberg: Springer International Publishing, 2016.
2. https://www.gartner.com/en/documents/3939836/market-guide-for-process-mining
3. https://www.researchandmarkets.com/reports/4576970/process-analytics-market-byprocess-mining-type
4. Mans, Ronny S., Wil M.P. Van der Aalst, and Rob J.B. Vanwersch. “Process mining in healthcare: evaluating and exploiting operational healthcare processes”. Heidelberg: Springer International Publishing, 2015.
5. Rojas, Eric, Jorge Munoz-Gama, Marcos Sepúlveda, and Daniel Capurro. “Process mining in healthcare: A literature review.” Journal of biomedical informatics 61 (2016): 224-236.
6. Ghasemi, Mahdi, and Daniel Amyot. “Process mining in healthcare: a systematised literature review.” International Journal of Electronic Healthcare 9.1 (2016): 60-88.
7. Erdogan, Tugba Gurgen, and Ayca Tarhan. “Systematic mapping of process mining studies in healthcare.” IEEE Access 6 (2018): 24543-24567.
8. Fernandez-Llatas, Carlos. "Interactive Process Mining in Healthcare." Heidelberg: Springer International Publishing, 2020.
Keywords: Process mining, Process science, Healthcare, Clinical data
Instructions for authors
http://www.aimspress.com/mbe/news/solo-detail/instructionsforauthors
Please submit your manuscript to online submission system
https://aimspress.jams.pub/
Paper Submission
All manuscripts will be peer-reviewed before their acceptance for publication. The deadline for manuscript submission is 30 December 2022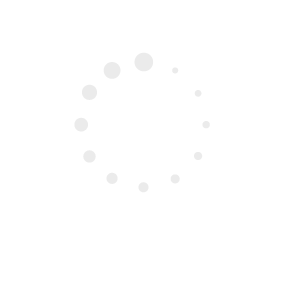