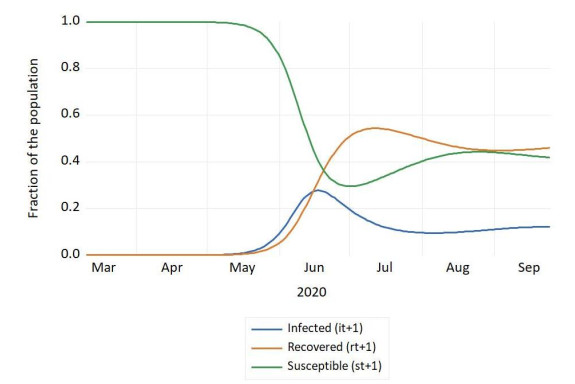
Citation: Rudy R. Negenborn, Peter-Jules van Overloop, Tamás Keviczky, Bart De Schutter. Distributed model predictive control of irrigation canals[J]. Networks and Heterogeneous Media, 2009, 4(2): 359-380. doi: 10.3934/nhm.2009.4.359
[1] | Ugo Avila-Ponce de León, Ángel G. C. Pérez, Eric Avila-Vales . A data driven analysis and forecast of an SEIARD epidemic model for COVID-19 in Mexico. Big Data and Information Analytics, 2020, 5(1): 14-28. doi: 10.3934/bdia.2020002 |
[2] | Antonio N. Bojanic . Accounting for the Trump factor in modeling the COVID-19 epidemic: the case of Louisiana. Big Data and Information Analytics, 2021, 6(0): 74-85. doi: 10.3934/bdia.2021006 |
[3] | Shuqi Wang, Wen Tang, Liyan Xiong, Mengyu Fang, Bingsong Zhang, Chi-Yang Chiu, Ruzong Fan . Mathematical modeling of transmission dynamics of COVID-19. Big Data and Information Analytics, 2021, 6(0): 12-25. doi: 10.3934/bdia.2021002 |
[4] | Nickson Golooba, Woldegebriel Assefa Woldegerima, Huaiping Zhu . Deep neural networks with application in predicting the spread of avian influenza through disease-informed neural networks. Big Data and Information Analytics, 2025, 9(0): 1-28. doi: 10.3934/bdia.2025001 |
[5] | Wenxue Huang, Yuanyi Pan, Lihong Zheng . Proportional association based roi model. Big Data and Information Analytics, 2017, 2(2): 119-125. doi: 10.3934/bdia.2017004 |
[6] | Ricky Fok, Agnieszka Lasek, Jiye Li, Aijun An . Modeling daily guest count prediction. Big Data and Information Analytics, 2016, 1(4): 299-308. doi: 10.3934/bdia.2016012 |
[7] | Bill Huajian Yang, Jenny Yang, Haoji Yang . Modeling portfolio loss by interval distributions. Big Data and Information Analytics, 2020, 5(1): 1-13. doi: 10.3934/bdia.2020001 |
[8] | S. Chen, Z. Wang, M. Kelly . Aggregate loss model with Poisson-Tweedie frequency. Big Data and Information Analytics, 2021, 6(0): 56-73. doi: 10.3934/bdia.2021005 |
[9] | Dongyang Yang, Wei Xu . Statistical modeling on human microbiome sequencing data. Big Data and Information Analytics, 2019, 4(1): 1-12. doi: 10.3934/bdia.2019001 |
[10] | Zhouchen Lin . A Review on Low-Rank Models in Data Analysis. Big Data and Information Analytics, 2016, 1(2): 139-161. doi: 10.3934/bdia.2016001 |
In Bolivia, the first reported cases of COVID-19 appeared on March 10 in the cities Santa Cruz and Oruro. Since then and until September 24,132,618 people have been infected throughout the country. Though reliable data is always an issue with epidemics of this magnitude1, certain aspects are becoming clearer. At the time of writing, 7765 people have died and 92,101 have recovered; the virus has spread throughout the country, but the departments of Santa Cruz, La Paz, and Cochabamba, account for around 68% of infected cases; 80% of recovered; and 77% of the dead2. There is no reliable information on whether the virus affects certain groups more than others—i.e., men and women; old and young; urban and rural; healthy and sick—but anecdotal evidence seems to suggest that, as in other countries, the virus is particularly prevalent in older enclaves and in people with underlying health conditions. The government of Bolivia began applying mitigation measures, including a strict quarantine order, on March 22, earlier than most other countries in Latin America; it closed its borders to international travel on March 21 and severely restricted domestic travel as well. On June 1 it began relaxing some of these measures, and at the time of writing was considering implementing them once again after an uptick in new infections and deaths.
1 This problem is compounded in developing countries like Bolivia
2 Bolivia is divided into 9 Departments: La Paz, Cochabamba, Santa Cruz, Oruro, Chuquisaca, Pando, Tarija, Beni, and Potosí
Although not explicitly analyzed here, it is important to note that the healthcare system in the country has been completely overwhelmed by the epidemic, both in terms of providing adequate and opportune testing that might help in containing the virus; and in providing basic healthcare services to people infected by the virus. Unfortunately, as is often the case in countries like Bolivia, people who are in greatest need of the system—the uninsured, the poor, and more generally, anyone living outside the principal urban centers—are suffering the most and are paying the consequences of the mismanagement, underfunding, and corruption that have afflicted the public health system in the country for generations.
An additional complicating factor in the spread of the virus is the political situation. The present government—headed by Jeanine Añez and in power since November 2019—is a transitional administration tasked with organizing democratic elections in 2020. Given its transitional nature and the story of Bolivian politics, the current government is inherently fragile and limited in its ability to impose and enforce proper mitigation measures.
We analyze the projected spread of COVID-19 at the national and departmental levels utilizing the Susceptible-Infective-Recovered (SIR) model3. Though several assumptions are made, particularly in terms of transmission and recovery rates and the number of contacts between infected and susceptible individuals, we find that our predicted model fits the data well. Further, the model allows us to make predictions regarding the impact of public interventions—including the establishment of quarantines and the introduction of effective antiviral treatments—on the trajectory of the virus.
3 In the SIR model, ‘Recovered’ includes those individuals that recover naturally or through medication from the disease and those that die (i.e., are removed) because of the disease. We did not choose a more detailed model due to lack of reliable data.
A summary of findings is this: on its current path and at the national level, a maximum fraction of infected individuals occurs around June 17, when approximately 28% of the population (3.2 million) is infected by the virus; when the epidemic begins to subside, about 54 % of the population (6.3 million) is in the recovered group, indicating that more than half of the population will have been infected by COVID-19 during some period of the epidemic. There is an uptick in the number of susceptible individuals after July 1, highlighting that the relaxing of mitigation measures might have happened too soon.
The rest of the paper is organized as follows. Section 2 presents a short review of the literature; section 3 analyzes the data and the methodology; section 4 reports the principal results, and section 5 concludes.
Predictive mathematical models for epidemics are fundamental to understand the trajectory of an epidemic and to plan effective control strategies to contain it. Developed by Kermack and McKendrick [1], the SIR model for human-to-human transmission of a disease is a commonly used methodology that describes the flow of individuals through three mutually exclusive stages of infection: Susceptible, infected, and recovered.
There have been many extensions to the SIR model and its utilization to describe the COVID-19 epidemic. Lin, et al. [2] for instance, utilize an SEIR (susceptible, exposed, infectious, removed) model considering risk perception and a cumulative number of cases; Anastassopoulou, et al. [3] analyze a discrete-time SIR model that includes dead individuals; Casella [4] develops a control-oriented SIR model that stresses the effects of delays and compares the outcomes of different containment policies; Giordano, et al. [5] compare simulation results with real data from Italy utilizing a novel SIDARTHE (susceptible, infected, diagnosed, ailing, recognized, threatened, healed, and extinct) model; Wu, et al. [6] use transmission dynamics to estimate the clinical severity of COVID-19; and Tam, et al. [7], utilizing a SIR model but adding the number of quarantined people, analyze the projected spread of COVID-19 in Louisiana without including the effects of the stay-at-home order. Some authors have also considered stochastic transmission models, including Hellewell, et al. [8] and Kucharski, et al. [9].
For the specific case of Bolivia, a handful of working papers, reports, and policy briefs have been developed on the theme of COVID-19. Utilizing an SEIR4 model and with national data only, Birbuet and López [10] present an analysis of the spread of the virus before and after the establishment of a quarantine. A report from the MRC Centre for Global Infectious Disease Analysis5 focuses on back-calculating an ‘inferred number of COVID-19 infections’ to estimate the number of people that have been infected and to make short-term projections for future healthcare needs; two additional documents—a report from the World Bank6 and a policy brief from United Nations7—focus on the impact of COVID 19 on the economic, social, and political performance of countries in Latin America and the Caribbean, but neither analyzes the evolution of the virus in each country.
4 We are not comfortable utilizing a latency number to predict the number of exposed individuals; we feel that an SIR model is more accurate in describing the spread of COVID-19 in Bolivia. If we had included a latent state in addition to the susceptible, infected, and recovered states, we would have to utilize two additional parameters – i.e., time it takes for an infected person to become infectious; and the average time during which an infected person is able to transmit the disease – which may not add significantly to the basic findings since, as it is the case with all parameters, much is still uncertain about COVID-19
5 Imperial College, London. Available at: https://mrc-ide.github.io/global-lmic-reports/BOL/
6 Available at: https://openknowledge.worldbank.org/handle/10986/33555
7 Available at: https://www.un.org/sites/un2.un.org/files/sg_policy_brief_covid_lac.pdf
As the preceding review makes clear, there is a gap concerning a formal analysis of COVID-19 in Bolivia, particularly at the departmental level. The contribution of this paper rests on filling this gap by presenting a mathematical model that describes the evolution of the epidemic from its inception point in early March of 2020 to its eventual decline, including how the trajectory of the virus might be altered if mitigation policies are put into place to control the spread of the virus.
The primary source of data for the number of infections, dead, and recovered is the government of Bolivia. It has set up an official website—Bolivia Segura8—where all statistical data is placed daily. The website also serves to inform the public on all matters related to the epidemic. A second webpage built by a group of university students—COVID Bolivia9—processes the data collected in Bolivia Segura and cross-references it with other private sources of data to control for the reliability of government-produced statistics. The data utilized here comes from Bolivia Segura but it is continually cross-referenced with data from COVID Bolivia10.
8 https://www.boliviasegura.gob.bo/
9 https://www.covid-bolivia.com/
10 At the onset of the epidemic, there were small discrepancies between these sources of data, but as time elapsed these discrepancies have almost disappeared.
The SIR dynamical system consists of three ordinary difference equations describing the evolution of the population in each state over time11:
(1) |
(2) |
(3) |
11 The SIR model presented is based on the description by Tassier [11]
where s(t), i(t), and r(t) represent fractions of the total population, per time, in the susceptible, infected, and recovered states (or categories), respectively. The parameters are defined as follows:
● α denotes the percent of contacts resulting in an infection; also known as the transmission rate
● γ represents the number of non-infected people contacted by each infected person
● k represents the fraction of the population that recovers from the disease each period; also known as the recovery rate
● and n and m denote the birth and death rates, respectively
If we let β = αγ, Eqs (1)–(3) can be restated as:
(4) |
(5) |
(6) |
The SIR models (4)–(6) are a bilinear system with three difference equations. The system is positive: All the state variables take non-negative values for t ≥ 0 if initialized at time 0 with non-negative values. Additionally, it assumes a constant population, hence n = m. The system is compartmental, and therefore the sum of the states (total population) is constant. Because the variables denote population fractions, we assume
(7) |
Note that we are above the epidemic threshold whenever it+1 > it; from Eq (5), this inequality is equivalent to βst > k – m, which implies that whenever this inequality holds we will be above the epidemic threshold and the number of infected individuals in the population will increase. Alternatively, if , the level of infected individuals increases; if , the level of infected individuals decreases.
Since at the beginning of the epidemic st ≈ 1, . The latter fraction is known as the Reproduction Number (R0) and it denotes the average number of people who will contract a contagious disease from one person with that disease. It specifically applies to a population of people who were previously free of infection and have not been vaccinated, hence this number is particularly relevant at the beginning of an epidemic.
Finally, note that, from Eq (5), if , the epidemic never materializes. Rearranging, the epidemic will disappear if . Thus, herd immunity is achieved if of the population are recovered and immune.
The parameters for the baseline SIR model are set at α = 0.03; γ = 10; k = 0.10; and n = m = 0.02512. The resulting basic reproduction number is R0 = 4, a clear indication that an epidemic is set to take off. Figure 1 shows the model evolution at the national level for the period March 10 to September 24, 2020.
12 The values for α, γ, and k are estimates based on available information. The transmission rate (α) remains highly uncertain and the value utilized here represents a lower bound in two comparable studies: one by Munayco, et al. [12] on Peru and the other one by Birbuet and López [10] on Bolivia. The recovery rate (k) also represents a lower bound value based on the number of daily and accumulated number of recovered individuals with respect to daily and accumulated infected individuals in Bolivia. The birth rate (n) was obtained from the World Bank (https://data.worldbank.org/indicator/SP.DYN.CBRT.IN?locations=BO).
As illustrated in Figure 1, with the baseline parameters the epidemic peaks on June 17, when approximately 28% of the population (3.2 million) becomes infected. Subsequent to the peak, the share of infected individuals decreases until around August 4, when 9% of the population are infected, but it gradually increases after that, breaking the 12% mark on September 16. On July 12, 54% of the population (6.3 million) are part of the recovered population, implying that more than half of the people in the country become infected with COVID-19 at some point during the epidemic. Beginning on July 13, the share of recovered individuals starts decreasing gradually but stabilizes at around 45% after September 10. The share of susceptible individuals also experiences an uneven evolution: after reaching a low of 29% on July 1, it begins to increase on July 2 reaching a level of 44% in August 28 when it once again retakes its downward trend. These fluctuations in the fractions of recovered and susceptible individuals highlight that the loosening of mitigation measures—which began on June 1—might have caused some recovered individuals to become susceptible again, denting the progress made in terms of preventing susceptible individuals from coming into contact with people carrying the disease13. Either way, the duration of the epidemic is likely to be extended by fluctuations in the number of susceptible individuals14. On present trends, about 58% of the population () will have to be immunized to achieve herd immunity.
13 It is also possible that fluctuations in the number of recovered individuals is the result of inexact testing; that is, infected individuals that eventually tested negative for the virus might have not recovered.
14 An alternative explanation for the fluctuation in susceptible individuals is that, with new births, the number of people in state S will always rise once the reproduction number falls below 1. Then, as S increases, the reproduction number rises and the number of infections increase as well. The result being a new peak of infections and a continuation of the disease dynamics in a cyclical pattern. Absent a permanent cure (i.e., the introduction of an effective vaccine), the process generates a steady state (endemic) level of infections over time.
Figure 2 illustrates the impact of loosening and tightening quarantine restrictions on the share of infected individuals at the national level.
If quarantine restrictions were tightened and the number of contacts between infected and susceptible individuals decreases by a single person (from a baseline value γ = 10 to γ = 9), a lower fraction of the population becomes infected (from a baseline peak of 28% on June 17 to a lower high peak of 24% on July 5); likewise, if quarantine restrictions are loosened and the number of contacts increases by 1 (from γ = 10 to γ = 11), a larger percentage of the population becomes infected (32% on June 4), highlighting that quarantine restrictions can help control the spread of the virus and reduce the level of infections, though at the cost of extending the duration of the epidemic. A similar exercise can be done with the recovery rate. If an effective treatment is found and k increases—say, from k = 0.10 to k = 0.15—so that individuals recover more quickly, the peak level of infections decreases to approximately 14% of the population (1.58 million) and the number of total infections decreases15. The behavioral pattern of the epidemic becomes more apparent if changes to γ and k are more significant than those reported here.
15 The graphs showing how the trajectory of the virus changes with varying values of k are available upon request.
Figure 3 illustrates the evolution of COVID-19 in Santa Cruz and La Paz, two of the departments most affected by it. The parameters are equal to the baseline parameters utilized at the national level with the only difference being the number of contacts γ; the value of γ is a function of the level of unrest in each department.
Public demonstrations against mitigation measures have been particularly prevalent in La Paz16. Additionally, demands for national elections, which the current government has postponed until October 18 due to concerns for greater propagation of the virus, have also been more visible and widespread in La Paz. As both types of public manifestations require large gatherings of people, we assume that the number of contacts with infected individuals is likely higher in this department than in relatively more peaceful Santa Cruz. If γ = 11 in La Paz and γ = 8 in Santa Cruz, we observe that in La Paz the virus is likely to infect a larger share of the population but will last a shorter period of time. Conversely, in relatively more peaceful Santa Cruz, a smaller fraction of the population is infected, with the epidemic lasting longer.
16 Social unrest has also occurred in other departments, but La Paz, as the administrative capital of the country, is where public demonstrations have been fiercest.
Figure 4 illustrates the evolution of the recovered state utilizing actual data (i.e., the share of recovered individuals in the population) and the predicted values of the SIR model (i.e., the share of recovered individuals as reflected in Eq (6)) for the departments of Santa Cruz and La Paz. The parameters are those utilized for describing the evolution of the virus in Figure 3.
As is evident, the pattern of behavior for the predicted value of the recovered share of the population mimics the evolution of the actual share of recovered individuals in both departments. The correlation coefficient between both variables in Santa Cruz is +0.95 and +0.39 in La Paz, and though there seems to be lag between the variables—especially in La Paz, where the predicted increase in recovered cases lags by almost 3 months the actual increase in cases—the model does seem to describe the actual evolution of the share of recovered individuals relatively well17.
17 Similar comparisons were made between predicted and actual values for the susceptible and infected states and the model accurately describes the actual evolution of the data in all departments. These graphical comparisons are available upon request.
Finally, it has been noted that the virus has spread throughout the country. Summary statistics for all departments are shown in Table 1.
Department | Infections started on: | Infections predicted to peak on: | Share of Infections (%) | Share of Recovered (%) | Share of Dead (%) |
Santa Cruz | March 10 | July 27 | 31.95 | 41.59 | 50.02 |
La Paz | March 19 | June 9 | 25.77 | 26.88 | 12.80 |
Cochabamba | March 13 | June 1 | 10.17 | 11.58 | 14.11 |
Oruro | March 10 | June 2 | 4.28 | 2.94 | 3.80 |
Potosi | March 26 | June 22 | 6.04 | 2.45 | 3.08 |
Pando | March 28 | July 16 | 1.78 | 0.54 | 2.11 |
Tarija | March 30 | June 23 | 9.57 | 7.46 | 3.76 |
Chuquisaca | March 27 | June 20 | 5.22 | 4.56 | 6.17 |
Beni | April 20 | August 12 | 5.36 | 2.50 | 4.55 |
Notes: 1. Date for peak on infections based on baseline parameters and levels of unrest. 2. Share of infections as a percentage of total infections. 3. Share of recovered as a percentage of total recovered. 4. Share of dead as a percentage of total dead. 5. Infections, recovered, and dead figures as of September 24, 2020. |
As Table 1 illustrates, though the brunt of the epidemic is being felt in Santa Cruz, La Paz and Cochabamba, it has become a national epidemic. There has been a constant increase in the number of dead in all departments, and this increase has become particularly visible in Chuquisaca and Beni. Additionally, the share of infections is increasing everywhere, being especially evident in the departments of Potosí and Tarija. As the preceding analysis makes clear, the epidemic in Bolivia is nowhere near being contained, and mitigation measures—particularly the mandatory quarantine in place until June 1—were lifted too soon.
The first two cases of COVID-19 appeared in Bolivia on March 10 of 2020. Since then, the virus has spread throughout the country and has overwhelmed an already weak public health system. The SIR model presented here accurately describes the trajectory of the virus, both at the national and departmental level, and also demonstrates that this trajectory could readily be altered by mitigation measures, particularly mandatory quarantines, that aim to reduce the number of contacts between infected and susceptible individuals. The model also shows that in the absence of effective mitigation measures the epidemic could become more widespread and last longer.
The principal conclusions are these: Nationally and at present trends, the virus peaks in mid-June when around 28% of the population becomes infected; as the epidemic begins to subside in July, more than half of the population belongs to the ‘recovered’ group, implying that more than 50% of Bolivians will have become infected with COVID-19 at some point during the epidemic. Beginning in July, there has been a fluctuation in the share of susceptible and recovered individuals, highlighting that the epidemic is nowhere near being contained. The pattern of behavior at the departmental level is similar than at the national level. The date when each department peaks in terms of infection shares varies, as does the timing when the epidemic begins to subside, but in all cases the evidence and the model show that the epidemic is far from over.
A key policy recommendation is that a mandatory quarantine throughout the country should once again be established to contain the latest uptick in infections. Though difficult because of the inherent weakness of the public health sector and the fragility of the current government, trying to isolate those infected with the disease and preventing contact with susceptible individuals seems to be the only current alternative to containing the epidemic. An additional recommendation concerns the state of government entities in charge of managing public health crises. The epidemic has laid bare the limitations of the public sector to deal with crises like the one unfolding now. Of critical importance when the Bolivian government finally addresses the fragility of these institutions is their capacity to collect—and make public—periodic, reliable data that allows the design of proper policy responses, and, from the perspective of this work, the development of more thorough epidemiological models that describe the spread of a disease.
The authors would like to thank an anonymous referee for helpful comments and suggestions. Any errors that remain are ours.
All authors declare no conflicts of interest in this paper.
1. | Christine Leyns, Elliot McClenaghan, Patricia Rodriguez, Patrick Nguipdop-Djomo, Carla Ascarrunz, Daniel Eid Rodriguez, Punam Mangtani, Javier Guitian, Hybrid, vaccine-induced and natural immunity against SARS-CoV-2 in traditional food markets in Bolivia (2020−2022): A cross-sectional analysis of a serological survey, 2025, 54, 0264410X, 127104, 10.1016/j.vaccine.2025.127104 |
Department | Infections started on: | Infections predicted to peak on: | Share of Infections (%) | Share of Recovered (%) | Share of Dead (%) |
Santa Cruz | March 10 | July 27 | 31.95 | 41.59 | 50.02 |
La Paz | March 19 | June 9 | 25.77 | 26.88 | 12.80 |
Cochabamba | March 13 | June 1 | 10.17 | 11.58 | 14.11 |
Oruro | March 10 | June 2 | 4.28 | 2.94 | 3.80 |
Potosi | March 26 | June 22 | 6.04 | 2.45 | 3.08 |
Pando | March 28 | July 16 | 1.78 | 0.54 | 2.11 |
Tarija | March 30 | June 23 | 9.57 | 7.46 | 3.76 |
Chuquisaca | March 27 | June 20 | 5.22 | 4.56 | 6.17 |
Beni | April 20 | August 12 | 5.36 | 2.50 | 4.55 |
Notes: 1. Date for peak on infections based on baseline parameters and levels of unrest. 2. Share of infections as a percentage of total infections. 3. Share of recovered as a percentage of total recovered. 4. Share of dead as a percentage of total dead. 5. Infections, recovered, and dead figures as of September 24, 2020. |
Department | Infections started on: | Infections predicted to peak on: | Share of Infections (%) | Share of Recovered (%) | Share of Dead (%) |
Santa Cruz | March 10 | July 27 | 31.95 | 41.59 | 50.02 |
La Paz | March 19 | June 9 | 25.77 | 26.88 | 12.80 |
Cochabamba | March 13 | June 1 | 10.17 | 11.58 | 14.11 |
Oruro | March 10 | June 2 | 4.28 | 2.94 | 3.80 |
Potosi | March 26 | June 22 | 6.04 | 2.45 | 3.08 |
Pando | March 28 | July 16 | 1.78 | 0.54 | 2.11 |
Tarija | March 30 | June 23 | 9.57 | 7.46 | 3.76 |
Chuquisaca | March 27 | June 20 | 5.22 | 4.56 | 6.17 |
Beni | April 20 | August 12 | 5.36 | 2.50 | 4.55 |
Notes: 1. Date for peak on infections based on baseline parameters and levels of unrest. 2. Share of infections as a percentage of total infections. 3. Share of recovered as a percentage of total recovered. 4. Share of dead as a percentage of total dead. 5. Infections, recovered, and dead figures as of September 24, 2020. |