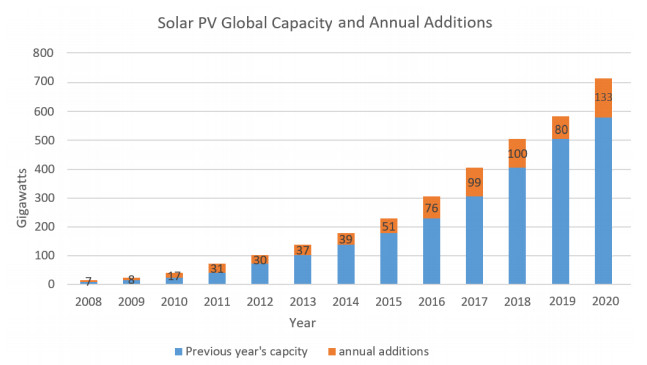
As distributed generation (DG) becomes increasingly integrated into the distribution grid, the structure of the distribution network is becoming more complex. To enhance the safety and cost-effectiveness of distribution systems, distribution network reconfiguration is gaining significant importance. Achieving optimal distribution network reconfiguration entails two key considerations: A feasible topology and economic efficiency. This paper addresses these challenges by introducing a novel approach that combines the potential island detection in undirected-graphs and the application of a whale optimization algorithm (WOA) for network reconfiguration optimization. To begin, we identified island categories based on the type of switchable-branches connected to these islands, allowing for the construction of potential island groups. Subsequently, unfeasible topologies were eliminated based on the conditions under which islands form within these potential island groups. Feasible topologies were then used to construct a model for network reconfiguration optimization. The optimal distribution network topology is determined using the WOA. In the final phase, the proposed method's effectiveness was demonstrated through a case study on the IEEE-33 node distribution network under scenarios with and without DG integration. The results showed that the proposed method exhibited better performance than traditional approaches in distribution network reconfiguration.
Citation: Zijian Hu, Hong Zhu, Chen Deng. Distribution network reconfiguration optimization method based on undirected-graph isolation group detection and the whale optimization algorithm[J]. AIMS Energy, 2024, 12(2): 484-504. doi: 10.3934/energy.2024023
[1] | Hayder S. Al-Madhhachi, Ahmed M. Ajeena, Nihad A. Al-Bughaebi . Dynamic simulation and energy analysis of forced circulation solar thermal system in two various climate cities in Iraq. AIMS Energy, 2021, 9(1): 138-149. doi: 10.3934/energy.2021008 |
[2] | Laveet Kumar, Jahanzaib Soomro, Hafeez Khoharo, Mamdouh El Haj Assad . A comprehensive review of solar thermal desalination technologies for freshwater production. AIMS Energy, 2023, 11(2): 293-318. doi: 10.3934/energy.2023016 |
[3] | Joanna McFarlane, Jason Richard Bell, David K. Felde, Robert A. Joseph III, A. Lou Qualls, Samuel Paul Weaver . Performance and Thermal Stability of a Polyaromatic Hydrocarbon in a Simulated Concentrating Solar Power Loop. AIMS Energy, 2014, 2(1): 41-70. doi: 10.3934/energy.2014.1.41 |
[4] | Akthem Mohi Al-Abdali, Handri Ammari . Thermal energy storage using phase-change material in evacuated-tubes solar collector. AIMS Energy, 2022, 10(3): 486-505. doi: 10.3934/energy.2022024 |
[5] | Chao-Jen Li, Peiwen Li, Kai Wang, Edgar Emir Molina . Survey of Properties of Key Single and Mixture Halide Salts for Potential Application as High Temperature Heat Transfer Fluids for Concentrated Solar Thermal Power Systems. AIMS Energy, 2014, 2(2): 133-157. doi: 10.3934/energy.2014.2.133 |
[6] | Daniel Chuquin-Vasco, Cristina Calderón-Tapia, Nelson Chuquin-Vasco, María Núñez-Moreno, Diana Aguirre-Ruiz, Vanesa G. Lo-Iacono-Ferreira . Mathematical modeling of a binary ORC operated with solar collectors. Case study—Ecuador. AIMS Energy, 2023, 11(6): 1153-1178. doi: 10.3934/energy.2023053 |
[7] | Thiago M. Vieira, Ézio C. Santana, Luiz F. S. Souza, Renan O. Silva, Tarso V. Ferreira, Douglas B. Riffel . A novel experimental procedure for lock-in thermography on solar cells. AIMS Energy, 2023, 11(3): 503-521. doi: 10.3934/energy.2023026 |
[8] | Xian-long Meng, Cun-liang Liu, Xiao-hui Bai, De-hai Kong, Kun Du . Improvement of the performance of parabolic trough solar concentrator using freeform optics and CPV/T design. AIMS Energy, 2021, 9(2): 286-304. doi: 10.3934/energy.2021015 |
[9] | Van Duong Nguyen, Duy Duc Ha, Thi Hoai Thu Nguyen, Duc Huy Nguyen . Generation expansion planning toward Net Zero target considering co-firing green fuel, CCUS installation and early decommissioning in thermal power plants: A case study of Vietnam. AIMS Energy, 2025, 13(3): 540-568. doi: 10.3934/energy.2025021 |
[10] | Nassir D. Mokhlif, Muhammad Asmail Eleiwi, Tadahmun A. Yassen . Experimental evaluation of a solar water heater integrated with a corrugated absorber plate and insulated flat reflectors. AIMS Energy, 2023, 11(3): 522-539. doi: 10.3934/energy.2023027 |
As distributed generation (DG) becomes increasingly integrated into the distribution grid, the structure of the distribution network is becoming more complex. To enhance the safety and cost-effectiveness of distribution systems, distribution network reconfiguration is gaining significant importance. Achieving optimal distribution network reconfiguration entails two key considerations: A feasible topology and economic efficiency. This paper addresses these challenges by introducing a novel approach that combines the potential island detection in undirected-graphs and the application of a whale optimization algorithm (WOA) for network reconfiguration optimization. To begin, we identified island categories based on the type of switchable-branches connected to these islands, allowing for the construction of potential island groups. Subsequently, unfeasible topologies were eliminated based on the conditions under which islands form within these potential island groups. Feasible topologies were then used to construct a model for network reconfiguration optimization. The optimal distribution network topology is determined using the WOA. In the final phase, the proposed method's effectiveness was demonstrated through a case study on the IEEE-33 node distribution network under scenarios with and without DG integration. The results showed that the proposed method exhibited better performance than traditional approaches in distribution network reconfiguration.
This project aims to study solar thermal energy applications in Nordic Countries. An analysis of the current solar plants Danish market is conducted, aiming at extrapolating the results to other Nordic Countries (Finland and Norway). Background research took place in order to understand the current situation of solar thermal energy around the world, its purpose and deficiencies and its current use in Denmark.
Climate change is one of the most urgent problems of this generation. Based on scientific evidence, human-induced global warming reached 1 ℃ above pre-industrial levels already, and it is increasing at 0.2 ℃ per decade [1].
An active approach to this problem has already been taken by many countries, trying to both reduce their emissions and control the production of greenhouse gasses [2]. One of the keys to stopping climate change and all its consequences is to produce energy in a more sustainable way [3]. After the oil crisis in the early 70s the focus was placed on the cost of energy, but during the past decades the reality of environmental degradation and its risk has changed the way of looking at electricity generation [4]. Renewable energy sources, such as wind, solar, hydro, geothermal, and biomass produce electricity and heating by using natural resources and in some cases, they can even be produced for heating, ventilation, and air-conditioning [5], solar water heating [6], and lighting [7]. These renewable energy technologies have been changing in promising ways in the last decades, dominating the global market for new power generation capacity. In many markets, solar PV and wind are increasingly the cheapest sources of electricity [8]. Moreover, most renewable power sources will become the dominating electricity and heating generating way within the next decade [9]. Nowadays, renewable power generation is growing faster than power demand. In 2019 a huge milestone was reached, when renewable electricity generation increase was higher than that of electricity demand, while fossil-fuel electricity decreased. This was the first time in years when there was a reduction of fossil-fuel based generation while the overall electricity generation increased [9].
The European Commission has set a long-term strategy to lead European countries in global climate action with the main goal of "achieving net-zero greenhouse gas emissions by 2050 through a socially-fair transition in a cost-efficient manner" [1]. In 2016, the Paris Agreement was signed by 181 parties, requiring strong global action to reduce greenhouse emissions in order to stop the global temperature increase. It is with this aim that countries have started developing their policies and strategies around renewable energy [1].
Only three renewable energy sources can be used to produce enough heat energy to power the whole world's demand: solar, geothermal and biomass [10]. Out of these three, solar energy is the one that presents the highest global potential, since geothermal energy is limited to a few locations and biomass is not naturally present in nature. This has created a lot of interest in solar energy and the study of how to make the most out of it.
Solar energy technologies have a long history. From 1860 until the First World War an assortment of technologies was developed to create steam using the sun's heat in order to run engines and irrigation pumps. In 1954, solar PV cells were invented at Bell Labs, United States [11]. The years immediately following the oil-shock in the seventies saw much interest in the commercialization of solar energy [12]. Later on, the sharp decline in oil prices and a lack of policy support stopped this increasing interest in the solar industry. It is since early 2000 when solar energy markets have gained momentum, showing phenomenal growth recently. Both technological improvements resulting in cost reductions and government policies in favour of renewable energies have made the future of solar energy promising [10].
There are two main types of solar panels: PV solar panels and solar thermal collectors. Whilst the first ones convert sunlight into electricity, the latest use the same solar energy to generate heat, converting it into the thermal energy of a fluid.
Solar PVs have become the world's fastest-growing energy sector, with new markets. Demand for solar energy is expanding and it has become the most competitive option for electricity generation in a number of markets (for residential and commercial applications). In Figure 1, the solar PV global capacity from 2008 to 2018 can be found. It can be seen that each year the PV capacity is higher than the previous one, proving the expanding trend of this energy source.
The annual global market for PV surpassed the 100 GW level in 2018 for the first time. Large demands were found in new markets and in Europe due to ongoing price reductions. By the end of 2018, a total of 32 countries presented a cumulative capacity of 1 GB. The top five national markets, which are China, India, United States, Japan and Australia, accounted for about three-quarters of newly installed capacity in 2018. The next five markets were Germany, Mexico, the Republic of Korea, Turkey and the Netherlands [13].
Solar thermal collectors are practically behaving as heat exchangers, absorbing solar radiation and turning it into internal energy (heat) of a fluid, usually either air, water or other fluid. This heat carried by the fluid can be used for domestic purposes, such as water heating. Otherwise the heat can be saved on a thermal storage tank for later use. The global capacity of solar water heating from 2008 to 2018 can be seen in Figure 2.
By the end of 2018, residential, commercial, and industrial clients from 130 different countries had implemented solar thermal heating. Heating and cooling systems provided around 369 TWh of heat annually, which is equivalent to the energy content of 233 million barrels of oil. The cumulative global operating capacity at the end of the year reached an estimated 480 GW [13].
The rapid electrification worldwide and the massive usage of heat pumps resulted in a decline in sales of solar hot water systems. On top of that, weak demand since in several countries the national support scheme for solar heat projects was not there anymore—as it was for several years—contributed that the collector capacity went down worldwide.
A number of factors such as latitude, diurnal variation, climate and geographic variation are highly responsible for determining the solar intensity in a specific site. For instance, high winds could be proven beneficial for the panels' efficiency [14]. Lately, the floating PVs have gained some space in industrial large-scale applications [15]. The sunniest place on Earth receives about 2,500 kWh/m2. On the other hand, the Nordic Region annual density varies between 1.100 kWh/m2 (in the south of the region) and 700 kWh/m2 (in the North). A sunny day in summer will give about 8 kWh/m2 and a cloudy winter day only gives about 0.002 kWh/m2 [16]. These numbers make one question if Nordic conditions are not apt for solar production. If we look at the case of Finland, it is located between parallels of latitude 60° and 70°. If we take into account that only about 19 million people in the world live above the latitude of 60°, the Finnish population represents about 28% of that [17]. This shows how Finland is one of the most Nordic countries, making it hard to believe solar energy could be used there.
The influence of climate change on solar energy production is mostly based on changes in irradiation, for example, the cloud cover lever, and temperature. For solar energy in the Nordic Region, the ground reflection effect during winter is of high significance due to reflection from the snow [18].
In the last years, an increase in the use of solar energy has been found in the Nordic Region, especially in Denmark. It has become a leading country on solar thermal systems, having the biggest large-scale solar district heating application. By the end of 2018, there was a total of 118 active plants with a total capacity of 970 MW, which makes up 63% of the capacity worldwide [17]. By 2019, the milestone of surpassing 1 GW of solar district heating in Denmark was achieved.
The installed capacity in large-scale solar systems in Denmark between 2010 and 2018 can be seen in Figure 3. The record is in 2016, with 348 MW installed capacity.
The Danish government started using investment subsides during the 1990s to support combined heat power plants, energy efficiency measures and renewable energy generation. Most of these grants are no longer available for new district heating investments. Nonetheless, decentralised combined heat and power operating in the electricity market will receive a premium tariff over the market price. Since the end of 2018, this is only available for combined heat and power plants using renewable energy. An indirect incentive to the solar district heating development in Denmark is the requirement for all district heating utilities to collect a certain amount of energy savings every year. If a plant cannot fulfil these required savings, it has to purchase credits from another utility, which has a surplus of energy savings [17].
Prior agreements between the energy distributors and the Danish Energy Agency allowed some district heating networks to count the first year of solar heat production as energy savings, therefore creating a surplus of valuable credits for the utility allowing to pay back part of the investment. By the end of 2016, this agreement expired and a new one was not released [17]. This caused a general refrain to invest in new solar thermal systems, which is reflected in Figure 3 as a sharp market decline in 2017 and 2018. In 2019, a new agreement was reached in order to help the market recover.
Additionally, a ban on the installation of oil and gas boilers in new buildings was implemented in 2013, and since 2016 oil-fried boilers, installation is prohibited in existing buildings, making more favourable the conditions for solar thermal technologies [20].
Regarding the large-scale solar thermal system, Denmark has a mature commercial market with distinct characteristics. Almost all systems supply heat to district heating networks, with no significant industrial applications. Most combined heat and power plants in Denmark sell electricity at a market price in the Nordic power market. Denmark has very favourable conditions for solar thermal integration into district heating networks, since the country has a long-standing tradition of it. A total of 63% of Danish households are connected to district heating networks [17]. District heating is regulated by the Heat Supply Act regulation. The idea behind it is that the end-users can benefit from this system. This allows the installations to have a long-term investment horizon, which favours solar thermal technologies.
Denmark is also known for having a strong solar thermal industry. Arcon-Sunmark A/S, the main manufacturer of plate collectors in Denmark, has installed over 80% of the large-scale heating plants in Europe [17].
Four solar thermal plants from Denmark are studied in this project: Silkeborg, Vollerup and Gråsten. The technical details of each plant are given in the following subsections. The goal of this project is to conduct in-depth research on the Danish approach to solar thermal energy. To do so, the main country solar thermal plants were studied.
Silkeborg is a Danish town with a population of 46,179. It is located in the middle of the Jutland, slightly west of the geographical centre of Denmark. In 2018, Silkeborg Supply (a municipality-owned utility supplying heat in the area) constructed a solar thermal plant at Sejling Hede, near Silkeborg [21]. The plant has a minimum estimated lifetime of 25 years and it consists of 12,436 solar collectors, making it the biggest solar thermal plant in the world. Its cost was approximately 250 million DKK and it reduces CO2 emissions by 15.000 tons per year. Solar collectors have been the most cost-effective technology in Silkeborg, supplying 22.000 households with renewable heating and helping the town reach its goal of being 100% carbon neutral by 2030 [22].
This solar plant supplies around 20% of Silkeborg's district heating annual demand via the district heating network, counting the cold winter months. This equals the yearly district heating demands for 4,400 one-family houses. Up to 100% of the demand has been supplied by the plant on sunny days, with 2.7 litres of water per hour circulating through the system. Extra heat can be stored in tanks for later use [23].
In April 2020, 12,842 MWh were produced in the plant, representing 38.5% of the town's heat consumption. The best day was the 23rd of April when 88.5% of the heat demand was met by the solar plant. The heat production for the first four months of 2020 has been around 25% higher than expected [24].
Vollerup is Sønderborg's easternmost district. Sønderborg is a city and municipality in Southern Jutland, on both sides of Alssund. The majority of the city is located on the island of Als.
The solar thermal park in Vollerup includes solar panels that provide more than 50% of the heat demand for the local area of almost 1,000 households. The fluid in the solar heating installation is water mixed with eco-friendly glycol. If there is an excess of heating, it can be stored in the storage tank, which has a capacity of 4,000 m3. In addition to the thermal solar collectors, there is an array of PV solar cells that also supply the facility's pumps' and instruments' needs [25].
Gråsten is a town on the southeast coast of the Jutland Peninsula, Denmark. It belongs to the Sønderborg municipality in the Region of Southern Denmark. In 2012, a solar thermal plant was built close to Gråsten by Arcon Solar. It consists of 1,519 solar modules with an estimated maximum heat production of 9,700 MWhth. The reduction of CO2 per year caused by the solar heating is 2,800 tons [26].
The other Nordic Countries have yet not taken such a strong approach to solar thermal applications as Denmark has. Some installations can be found, but they are mostly for industrial or private use. The biggest solar thermal projects in Norway and Finland are described in this section.
In 2011, a heating park, Akershus EnergiPark, was built outside Lillestrøm. Lillestrøm is a town in the municipality of Lillestrom in Viken country. The city is located in the Oslo region, in the southeast of Norway. Akershus EnergiPark is based on a close collaboration between Akerhus Energi, business and research and educational institutions in the region.
The main source of heat production of the park is forest wood chips. In addition, it also uses biogas that comes in a 5km long pipeline from the Bøhler landfill and heat pumps. It also includes a solar heating plant that alone supplies 4 Gigawatt-hour thermal (GWhth) annually. The plant is equipped with an accumulator tank of 1,200 m3 for energy storage [27].
The plant provides 150 GWhth annually, which corresponds to the heating demand of 15,000 households, reducing CO2 emissions by over 30,000 T per year [28].
The biggest solar thermal installation in Finland is found in Hämeelinna. Hämeelinna is a city of around 68,200 inhabitants, located in the heart of Häme, in the south of Finland. In 2017, Saco-Solar installed a solar thermal system for the swimming hall in the city. The heat from the panels meets part of the building needs (such as space heating and hot water). If there is any surplus energy, for example in the days when the building is closed to the public, it is distributed to the local district heating network. A total of 124 KW can be obtained from the renewable source in this installation [29].
The goal of this project was to study solar thermal energy in the northern countries and compare its usage in Denmark with Finland and Norway. Three different Danish plants are going to be studied: Silkeborg, Vollerup and Gråsten, chosen mostly due to the abundance of information. For each of these plants, different types of data were collected and analysed [30]. An analysis of the current solar thermal market in Denmark was conducted in order to understand how the country came into the use of this renewable energy source [31] ending up with an educated guess about the future of solar thermal energy in the Nordic Countries. Different national policies in the Nordics were taken into account, since their policies have a huge impact on the use of solar thermal in each country [32].
Two different data sets were used for the project: satellite images and numerical data. The data studied focused on different solar parks - considered in terms of research design as secondary data – defined as "written sources that interpret or record primary data" [33], as they were obtained from free to access governmental sources. The collection of this data was done through the program Google Earth Pro. The online option of "Wayback imagery" was also used to properly validate the data [34]. It is with these tools that images of the solar plants were searched. Then, using the "historical images" option, time is reversed to see how the plant was constructed, paying special interest in large extensions of the plants throughout time. Once the different phases of construction were identified, the "polygon" option is used to draw polygons through the plants' area, allowing one to obtain the total area (in square-meters) of each plant before and after the extensions. This same process was repeated for each of the selected solar plants.
The second type of data collection consisted of numerical data representing the solar heat production of each plant. These were considered secondary data since they were not directly taken by the researcher of this project, but they were extracted by a validated source [35]. It should be noted that not enough data on the Vollerup solar plant were available to conduct a proper analysis, so only the other two plants were studied. Daily and weekly data were retrieved from 2017, 2018 and 2019. Additionally to the data analysis, a short market study of Denmark solar thermal energy status was conducted. To do so, background research was done and presented in section 1.4., from which some conclusions and further discussions were also extracted. Finally, an extrapolation of all the previous analyses will be made to try to predict the future of solar thermal applications in Denmark and other Nordic Countries.
The objective of this venture is to conduct in-depth inquire about the Danish approach to solar thermal plants. To do so, most nation solar-powered thermal facilities were examined. To ensure internal validity, which is "the degree to which we can appropriately conclude that the changes in X caused the changes in Y" [36], a triangulation of the data is conducted whilst comparing data from different sources and, at the same time, checking the results with the experience of experts on the area. Nevertheless, since the samples taken are also those with higher influence on the outcome, an inductive statement extracted from this paper should be correct for all cases. The reliability of the project is assured with a good explanation of every step taken in the making of it, allowing others to repeat the analysis and assuring they will obtain the same results. In this project, actual data from the solar plants could provide an insight complementing the satellite data. The results from this report will show the potential of solar thermal energy for Nordic Countries, using Denmark as the main example.
There is a specific way solar plants are developed in Denmark in comparison to the other Nordic countries. In Denmark, all the studied plants are built in two phases: first, some panels are implemented and if the result is as predicted, the plant is then extended to its optimal size. In the other Scandinavian countries, a one-stage construction phase is followed for the plants.
If we look at the Silkeborg Plant, the first phase consisted of around 172,000 m2 (two polygons of 45,929 m2 and 125,611 m2). This is then expanded with a 20,360 m2 big area and a small part of 9,077 m2. The total final area of the plant is then around 334,000 m2. This means that the extension of the plant consists of adding a 99.86% of its first size. In other words, the plant size was almost doubled in size. The plant construction phases are shown in Figure 4.
The building process of the Vollerup solar thermal plant follows the same pattern. First, a total of around 12,700 m2 are built (consisting of 4 polygons of 2,128 m2 the top one, 3.167 m2 the left one, 3,606 m2 the right one and 3,816 the bottom one). Later on, two extra polygons of area 1,274 m2 and 1,752 m2 were added, making the total plant area around 15,700 m2. The added area was a 23.62% of the original installation. The plant construction phases and the polygons used to measure it are shown in Figure 5.
The Gråsten solar thermal-plant had an original size of around 39.350 m2, divided into 3 areas of 3,524 m2, 15,082 m2 and 20,750 m2 from left to right. An extra polygon of 24,717 m2 was added later on, making the total plant area about 67,000 m2. The added area is a 70.3% of the original one. The polygons used to measure the plant and its phases of construction are shown in Figure 6.
If the different areas are compared, Silkeborg is 5 times larger than Gråsten and 23 times larger than Vollerup. It is also this plant the one that had the higher percentage of added area. A summary of each plant area can be seen in Table 1. It is noticed that all the plants were constructed until their optimal size using a second construction phase. It should also be pointed out that all the solar plants studied are large-scale plants.
Silkeborg | Vollerup | Gråsten | |
Initial area [m2] | 171,540 | 12,700 | 39,350 |
Added area [m2] | 17, 1305 | 3,000 | 27,650 |
Total area [m2] | 342,845 | 15,700 | 67,000 |
% of added area | 99.8 | 23.6 | 70.3 |
On the other hand, if the area of Akershus (Norway) and Hämeenlinna (Finland) installations are analysed, it can be seen that only one construction phase was used.
As for the Akershus, the total measured area is 28,550 m2, consisting of two polygons of 8,725 m2 and 19,825 m2, as shown in Figure 7.
The Hämeenlinna total area is 515 m2 and it is situated on the roof of a building (the swimming pool hall), as shown in Figure 8. This is a small installation since its only purpose is to supply heat to one building.
Both the Norwegian and Finnish plans have not been taken to their maximum production level, with only one construction phase. These installations are considered small-scale, consisting of a small area if compared with the Danish plants. This is due to the approach to solar energy made by the countries, decided to entrust small-scale applications and industrial utilities instead of large-scale plants. For a higher increase of solar energy use in these countries, the policies prioritising small installations should be revised.
The results of the study of solar heat production of two Danish solar thermal plants (Silkeborg and Gråsten) are now presented. The monthly solar heat production from 2017 until 2019 is shown in Figure 9A, C for Silkeborg and Gråsten respectively. If both graphs are observed it can be seen that they follow a similar sinusoidal pattern, with high heat production during the summer months and low during the winter months. This is a pattern that is repeated every year, indifferently from the plant position or value of solar heat production.
A closer inspection of the last year can be seen in Figure 9B, D. The daily solar heat production is represented for each plant (Silkeborg and Gråsten). It is observed that, whilst the followed pattern is quite similar, the amount of solar heat production in Silkeborg is quite higher than that of Gråsten. This is also related to the size of the solar plants, since the Silkeborg plant is 4 times larger than the one in Gråsten. Silkeborg highest solar heat production is around 4,500 MWh whilst Gråsten's is around 700 MWh.
Once the solar thermal plants have been studied, it is important to know the context in which these are found. A quick study of the Danish market is done. As shown in section 1.4., the Danish solar thermal market has seen a huge increase in the last years, placing it amongst the top countries using this energy source. The main reasons behind this market expansion are summarised below.
Denmark presents a high market penetration of district heating networks, specifically for the heat of households. In most Scandinavian countries, the share of district heating systems is above 60%. Only Finland has a share of lower than 40% [37]. Moreover, there is high compatibility of solar thermal heat with these district heating loads. Some examples of this compatibility would be the high heat demand and density of the networks and the opportunity of obtaining cheap land for collectors near the supply points. Another important aspect that encourages the use of thermal energy in Denmark is the high taxes on fossil fuels, making coal and natural gas plants less profitable. The situation regarding the cost of natural gas becomes even more complicated for all Europe under the Russo-Ukrainian War and the overall availability and costs for all European countries including, of course, the Nordics [38]. Since this conflict goes if not back to the dissolution of the Soviet Union, definitely back to 2014—where hundreds maybe thousands of enmities took (and still continue to take) place in the borders of the two countries, it is therefore, imperative to focus on other alternatives for district heating apart from natural gas. Furthermore, the presence of market-based subsidies for large-scale thermal systems encourages the use of this energy source. These incentives are focused on district heating facilities instead of small-scale applications or industrial utilities—such as solar air-conditioning [39]—an effective strategy to promote large-scale plants. Finally, the country non-profit orientation regulations regarding heat supply allow a long-term investment horizon and facilitate the availability of easily accessible bank loans for the utilities.
These conditions seem to outweigh the lacking solar resources, making a country like Denmark with a low irradiance levels, competent in the solar thermal market world. Whilst the other countries have a higher rate of solar radiation, Denmark unique policies and market strategy for this energy source allow it to be competent.
Solar thermal energy is an energy source that has gained a lot of importance in the last few years, but there are still improvements to be done. It is believed that its use will grow even more in the following years, since solar costs—including solar thermal applications—have dropped more than 80% over the past decade [40]. Denmark is a pioneer in the large-scale use of solar thermal plants, expanding its utilities until its optimal dimension in order to get as much solar energy as possible. Nowadays, a clear increase in the cumulative amount of annual solar heat production of Danish solar plants is noted, indicating an increase in solar radiation in the Nordic Region over the last years. These climate transformations that we are living in nowadays are a key component in the future development of solar energy in the Nordic Countries. Solar energy may not have been considered a profitable source in the past in these countries, but with climate change, this should be reconsidered [41].
The main difference between Denmark and the other Nordic Countries analysed is the marketplace of solar thermal heat. Whilst Denmark has decided to include it in their district heating, the other countries are using it only still for small-scale installations and industrial purposes. This is mainly due to each country's legislative framework and its approach to renewable energies. Denmark has decided to approach the heat market in a smart way that allows the profitable existence of large-scale solar plants. The other Nordic Countries may change their approach to this energy source in the following years, since it has been proven that it is a profitable renewable energy even for a Nordic country such as Denmark.
In this project, the solar thermal energy in the Nordic countries was studied, mainly though focused on Denmark. In the last years, solar energy has been gaining popularity all around the world as one of the two most dominating renewable energy sources with several innovative applications, and the Nordic Countries are not falling behind in this trend. Whilst it is true that the weather conditions in these countries are not the most favourable for solar energy, Climate Change is giving the Nordic Region a chance. Denmark is one of the pioneers of solar thermal energy. Even if it does not have the highest solar radiation, it is due to its smart approach to this energy source that the country has a big impact on the solar thermal market. Their main use is in large-scale plants connected to district heating distribution, which is possible thanks to the country regulations and favourable agreements. This makes them able to use this energy to its maximum potential whilst other countries are still struggling to integrate it into their energy system.
Other Nordic Countries are yet not as developed in this energy source as Denmark is. In this project, the case of Norway and Finland have been studied. The main difference that has been found between these countries and Denmark is their solar thermal plants' size. Whilst Denmark is betting for large-scale plants with a two-phase system to bring them to their optimal point, the other countries are still using small-scale facilities. They focus mostly on private and self-consuming (running under a net metering contract) installations, not having integrated this energy source into their energy system yet. It has been shown in this project that the amount of solar heat production of Danish plants and, therefore, the solar radiation in this country has had a noticeable increment in the last years. It is also believed that for other Nordic Countries to have a profitable use of solar thermal energy, a change in their approach to it should be made. Taking Denmark as an example, expanding the size of their solar thermal installations should be considered, using solar thermal energy not only for private use but also as one of their main heat energy sources. Policies as those used in Denmark could be used to boost the solar market, offering agreements favourable to the large-scale use of the energy source.
All authors declare no conflicts of interest in this paper.
[1] |
Guan W, Chen C, Lan T, et al. (2021) Research on distribution network fault recovery reconfiguration considering distributed generations. IOP Conf Ser: Earth Environ Sci 769: 042011. https://doi.org/10.1088/1755-1315/769/4/042011 doi: 10.1088/1755-1315/769/4/042011
![]() |
[2] |
Mahdavi M, Schmitt K, Ramos RAV, et al. (2022) Role of hydrocarbons and renewable energies in Iran's energy matrix focusing on bioenergy: Review. IET Renewable Power Gener 16: 3384–3405. https://doi.org/10.1049/rpg2.12540 doi: 10.1049/rpg2.12540
![]() |
[3] |
Mahdavi M, Vera D (2023) Importance of renewable energy sources and agricultural biomass in providing primary energy demand for Morocco. Int J Hydrogen Energy 48: 34575–34598. https://doi.org/10.1016/j.ijhydene.2023.05.246 doi: 10.1016/j.ijhydene.2023.05.246
![]() |
[4] |
Mahdavi M, Schmitt KEK, Jurado F (2023) Robust distribution network reconfiguration in the presence of distributed generation under uncertainty in demand and load variations. IEEE Trans Power Delivery 38: 3480–3495. https://doi.org/10.1109/TPWRD.2023.3277816 doi: 10.1109/TPWRD.2023.3277816
![]() |
[5] |
Li Y, Feng B, Li GQ, et al. (2018) Optimal distributed generation planning in active distribution networks considering integration of energy storage. Appl Energy 210: 1073–1081. https://doi.org/10.1016/j.apenergy.2017.08.008 doi: 10.1016/j.apenergy.2017.08.008
![]() |
[6] |
Li Y, Feng B, Wang B, et al. (2022) Joint planning of distributed generations and energy storage in active distribution networks: A Bi-Level programming approach. Energy 245: 123226. https://doi.org/10.1016/j.energy.2022.123226 doi: 10.1016/j.energy.2022.123226
![]() |
[7] |
Mahdavi M, Alhelou HH, Siano P, et al. (2022) Robust mixed-integer programing model for reconfiguration of distribution feeders under uncertain and variable loads considering capacitor banks, voltage regulators, and protective relays. IEEE Trans Industrial Inf 18: 7790–7803. https://doi.org/10.1109/tii.2022.3141412 doi: 10.1109/tii.2022.3141412
![]() |
[8] |
Kim HW, Ahn SJ, Yun SY, et al. (2023) Loop-based encoding and decoding algorithms for distribution network reconfiguration. IEEE Trans Power Delivery 38: 2573–2584. https://doi.org/10.1109/TPWRD.2023.3247826 doi: 10.1109/TPWRD.2023.3247826
![]() |
[9] |
Shaheen AM, Elsayed AM, El-Sehiemy RA, et al. (2021) Equilibrium optimization algorithm for network reconfiguration and distributed generation allocation in power systems. Appl Soft Computing 98: 106867. https://doi.org/10.1016/j.asoc.2020.106867 doi: 10.1016/j.asoc.2020.106867
![]() |
[10] |
Helmi AM, Carli R, Dotoli M, et al. (2021) Efficient and sustainable reconfiguration of distribution networks via metaheuristic optimization. IEEE Trans Automation Sci Eng 19: 82–98. https://doi.org/10.1109/TASE.2021.3072862 doi: 10.1109/TASE.2021.3072862
![]() |
[11] |
Mahdavi M, Alhelou HH, Hatziargyriou ND, et al. (2021) An efficient mathematical model for distribution system reconfiguration using AMPL. IEEE Access 9: 79961–79993. https://doi.org/10.1109/ACCESS.2021.3083688 doi: 10.1109/ACCESS.2021.3083688
![]() |
[12] |
Shaheen AM, Elsayed AM, Ginidi AR, et al. (2021) Effective automation of distribution systems with joint integration of DGs/SVCs considering reconfiguration capability by jellyfish search algorithm. IEEE Access 9: 92053–92069. https://doi.org/10.1109/ACCESS.2021.3092337 doi: 10.1109/ACCESS.2021.3092337
![]() |
[13] |
Mansouri SA, Ahmarinejad A, Nematbakhsh E, et al. (2022) A sustainable framework for multi-microgrids energy management in automated distribution network by considering smart homes and high penetration of renewable energy resources. Energy 245: 123228. https://doi.org/10.1016/j.energy.2022.123228 doi: 10.1016/j.energy.2022.123228
![]() |
[14] |
Abou El-Ela AA, El-Seheimy RA, Shaheen AM, et al. (2021) PV and battery energy storage integration in distribution networks using equilibrium algorithm. J Energy Storage 42: 103041. https://doi.org/10.1016/j.est.2021.103041 doi: 10.1016/j.est.2021.103041
![]() |
[15] |
Ali MH, Kamel S, Hassan MH, et al. (2022) An improved wild horse optimization algorithm for reliability based optimal DG planning of radial distribution networks. Energy Rep 8: 582–604. https://doi.org/10.1016/j.egyr.2021.12.023 doi: 10.1016/j.egyr.2021.12.023
![]() |
[16] | Gautam M, Bhusal N, Benidris M (2022) Deep Q-Learning-based distribution network reconfiguration for reliability improvement. 2022 IEEE/PES Transmission and Distribution Conference and Exposition (T & D), New Orleans, LA, USA, 1–5, https://doi.org/10.1109/TD43745.2022.9817000 |
[17] |
Eid A, Kamel S, Abualigah L (2021) Marine predators algorithm for optimal allocation of active and reactive power resources in distribution networks. Neural Computing Appl 33: 14327–14355. https://doi.org/10.1007/s00521-021-06078-4 doi: 10.1007/s00521-021-06078-4
![]() |
[18] |
Castiblanco-Pérez CM, Toro-Rodríguez DE, Montoya OD, et al. (2021) Optimal placement and sizing of D-STATCOM in radial and meshed distribution networks using a discrete-continuous version of the genetic algorithm. Electronics 10: 1452. https://doi.org/10.3390/electronics10121452 doi: 10.3390/electronics10121452
![]() |
[19] |
Mahdavi M, Javadi MS, Wang F, et al. (2022) An efficient model for accurate evaluation of consumption pattern in distribution system reconfiguration. IEEE Trans Industry Appl 58: 3102–3111. https://doi.org/10.1109/tia.2022.3148061 doi: 10.1109/tia.2022.3148061
![]() |
[20] |
Mahdavi M, Schmitt K, Jurado F, et al. (2023) An efficient framework for optimal allocation of renewable energy sources in reconfigurable distribution systems with variable loads. IEEE Trans Industry Appl 60: 2431–2442. https://doi.org/10.1109/tia.2023.3341876 doi: 10.1109/tia.2023.3341876
![]() |
[21] |
Mahdavi M, Schmitt K, Chamana M, et al. (2023) Effective strategies for distribution systems reconfiguration considering loads voltage dependence. IEEE Trans Industry Appl 60: 2621–2632. https://doi.org/10.1109/tia.2023.3341890 doi: 10.1109/tia.2023.3341890
![]() |
[22] |
Mahdavi M, Schmitt K, Chamana M, et al. (2023) Distribution systems reconfiguration considering dependency of loads on grid voltage and temperature. IEEE Trans Power Delivery 39: 882–897. https://doi.org/10.1109/tpwrd.2023.3340344 doi: 10.1109/tpwrd.2023.3340344
![]() |
[23] |
Zhang J, Wang P, Zhang N (2021) Distribution network admittance matrix estimation with linear regression. IEEE Trans Power Syst 36: 4896–4899. https://doi.org/10.1109/TPWRS.2021.3090250 doi: 10.1109/TPWRS.2021.3090250
![]() |
[24] |
Sarkar D, Konwar P, De A, et al. (2020) A graph theory application for fast and efficient search of optimal radialized distribution network topology. J King Saud University Eng Sci 32: 255–264. https://doi.org/10.1016/j.jksues.2019.02.003 doi: 10.1016/j.jksues.2019.02.003
![]() |
[25] |
Chen Y, Deng J, Zhang X, et al. (2020) Distribution network topology identification method based on matching loop power. J Physics: Conf Ser 1633: 012093. https://doi.org/10.1088/1742-6596/1633/1/012093 doi: 10.1088/1742-6596/1633/1/012093
![]() |
[26] |
Shaheen AM, Elsayed AM, El-Sehiemy RA, et al. (2021) Equilibrium optimization algorithm for network reconfiguration and distributed generation allocation in power systems. Appl Soft Computing 98: 106867. https://doi.org/10.1016/j.asoc.2020.106867 doi: 10.1016/j.asoc.2020.106867
![]() |
[27] |
Jiang LH, Wang WY, Wang PJ, et al. (2014) Distribution network reconfiguration optimization method for reducing the voltage sag impact on important loads. Appl Mech Mater 492: 478–481. https://doi.org/10.4028/www.scientific.net/AMM.492.478 doi: 10.4028/www.scientific.net/AMM.492.478
![]() |
[28] |
Ma X, Wang P, Wang Y, et al. (2022) Penalty and barrier-based numerical optimization for efficiency and power density of interleaved buck/boost converter. IEEE Trans Power Electron 37: 12095–12107. https://doi.org/10.1109/TPEL.2022.3173008 doi: 10.1109/TPEL.2022.3173008
![]() |
[29] |
Zhang Y, Qian T, Tang W (2022) Buildings-to-distribution-network integration considering power transformer loading capability and distribution network reconfiguration. Energy 244: 123104. https://doi.org/10.1016/j.energy.2022.123104 doi: 10.1016/j.energy.2022.123104
![]() |
1. | Ioan Sarbu, Matei Mirza, Daniel Muntean, Integration of Renewable Energy Sources into Low-Temperature District Heating Systems: A Review, 2022, 15, 1996-1073, 6523, 10.3390/en15186523 | |
2. | Ioan Sarbu, Alexandru Dorca, Matei Mirza, Exhaustive overview of advances in integrating renewable energy sources into district heating systems, 2024, 116, 22106707, 105897, 10.1016/j.scs.2024.105897 | |
3. | Kristian Emil Schrøder Hansen, George Xydis, Long-term heat storage opportunities of renewable energy for district heating networks, 2024, 1751-4223, 1, 10.1680/jener.23.00023 | |
4. | Qianyun Wen, Axel Lindfors, Yang Liu, How should you heat your home in the green energy transition? A scenario-based multi-criteria decision-making approach, 2023, 421, 09596526, 138398, 10.1016/j.jclepro.2023.138398 | |
5. | Georgios Martinopoulos, Domestic hot water solar thermal systems as an energy-security alternative in the EU: A parametric analysis, 2024, 69, 22131388, 103921, 10.1016/j.seta.2024.103921 | |
6. | Arvin Sohrabi, Shuli Liu, Erdem Cuce, Yongliang Shen, Sheher Yar Khan, Mahesh Kumar, Utilization of low-grade thermal energy for residential applications: A review of the existing and potential technologies, 2025, 219, 13640321, 115832, 10.1016/j.rser.2025.115832 |
Silkeborg | Vollerup | Gråsten | |
Initial area [m2] | 171,540 | 12,700 | 39,350 |
Added area [m2] | 17, 1305 | 3,000 | 27,650 |
Total area [m2] | 342,845 | 15,700 | 67,000 |
% of added area | 99.8 | 23.6 | 70.3 |