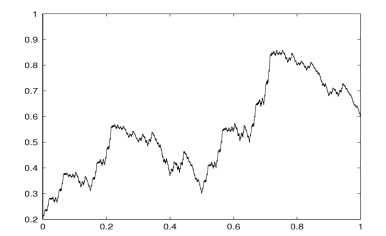
Fractal interpolation function (FIF) is a fixed point of the Read–Bajraktarević operator defined on a suitable function space and is constructed via an iterated function system (IFS). In this paper, we considered the generalized affine FIF generated through the IFS defined by the functions Wn(x,y)=(an(x)+en,αn(x)y+ψn(x)), n=1,…,N. We studied the shift of the fractal interpolation curve, by computing the error estimate in response to a small perturbation on αn(x). In addition, we gave a sufficient condition on the perturbed IFS so that it satisfies the continuity condition. As an application, we computed an upper bound of the maximum range of the perturbed FIF.
Citation: Najmeddine Attia, Neji Saidi, Rim Amami, Rimah Amami. On the stability of Fractal interpolation functions with variable parameters[J]. AIMS Mathematics, 2024, 9(2): 2908-2924. doi: 10.3934/math.2024143
[1] | Najmeddine Attia, Taoufik Moulahi, Rim Amami, Neji Saidi . Note on fractal interpolation function with variable parameters. AIMS Mathematics, 2024, 9(2): 2584-2601. doi: 10.3934/math.2024127 |
[2] | Najmeddine Attia, Rim Amami . On linear transformation of generalized affine fractal interpolation function. AIMS Mathematics, 2024, 9(7): 16848-16862. doi: 10.3934/math.2024817 |
[3] | Xuezai Pan, Minggang Wang . The uniformly continuous theorem of fractal interpolation surface function and its proof. AIMS Mathematics, 2024, 9(5): 10858-10868. doi: 10.3934/math.2024529 |
[4] | Baoxing Zhang, Yunkun Zhang, Yuanyuan Xie . Generating irregular fractals based on iterated function systems. AIMS Mathematics, 2024, 9(5): 13346-13357. doi: 10.3934/math.2024651 |
[5] | Hasib Khan, Jehad Alzabut, Anwar Shah, Sina Etemad, Shahram Rezapour, Choonkil Park . A study on the fractal-fractional tobacco smoking model. AIMS Mathematics, 2022, 7(8): 13887-13909. doi: 10.3934/math.2022767 |
[6] | Tunçar Şahan, Yunus Atalan . Novel escape criteria for complex-valued hyperbolic functions through a fixed point iteration method. AIMS Mathematics, 2025, 10(1): 1529-1554. doi: 10.3934/math.2025071 |
[7] | Cemil Tunç, Alireza Khalili Golmankhaneh . On stability of a class of second alpha-order fractal differential equations. AIMS Mathematics, 2020, 5(3): 2126-2142. doi: 10.3934/math.2020141 |
[8] | Martin Do Pham . Fractal approximation of chaos game representations using recurrent iterated function systems. AIMS Mathematics, 2019, 5(6): 1824-1840. doi: 10.3934/math.2019.6.1824 |
[9] | Yuki Takahashi . On iterated function systems with inverses. AIMS Mathematics, 2025, 10(4): 9034-9041. doi: 10.3934/math.2025415 |
[10] | Jin Xie, Xiaoyan Liu, Lei Zhu, Yuqing Ma, Ke Zhang . The C3 parametric eighth-degree interpolation spline function. AIMS Mathematics, 2023, 8(6): 14623-14632. doi: 10.3934/math.2023748 |
Fractal interpolation function (FIF) is a fixed point of the Read–Bajraktarević operator defined on a suitable function space and is constructed via an iterated function system (IFS). In this paper, we considered the generalized affine FIF generated through the IFS defined by the functions Wn(x,y)=(an(x)+en,αn(x)y+ψn(x)), n=1,…,N. We studied the shift of the fractal interpolation curve, by computing the error estimate in response to a small perturbation on αn(x). In addition, we gave a sufficient condition on the perturbed IFS so that it satisfies the continuity condition. As an application, we computed an upper bound of the maximum range of the perturbed FIF.
Fractal interpolation provides a framework for interpolating sets of data that may exhibit some self-similarity at different scales. While classical interpolation techniques, such as polynomial interpolation and spline interpolation generate smooth interpolants, fractal interpolation is able to model irregular sets of data. The concept of the fractal interpolation functions (FIFs) was first introduced by Barnsley [1,2,3]. Since then, this theory has become a powerful and useful tool in applied science and engineering, especially when dealing with real-world signals such as financial series, time series, climate data and bioelectric recordings.
The iterated function systems (IFSs in short) are useful to construct some fractal sets [4,5,6,7,8]). Specifically, IFS employs contractive functions over a complete metric space (X,d), where the existence and uniqueness of the fixed point are guaranteed by Banach's theorem. This is done using the Hutchinson operator, which also is a contraction mapping over H(X), where H(X) is the space of all compact subsets of X (see, for instance, [4,9,10,11,12,13,14] for some extension of Hutchinson's framework). Recently, the existence of FIFs through different well-known results given by the fixed point theory have been studied by many researchers [15,16,17,18,19,20]. Moreover, the wide range of FIFs constructed and studied proved many important properties including calculus, dimensionality, stability, smoothness and disturbance error [17,20,21,22,23,24,25,26].
Let N≥2. In this paper, we consider the generalized affine FIF defined by
{Ln(x)=anx+enFn(x,y)=αn(x)y+ψn(x),n∈J:={1,…N}, |
where, for each n, αn is a Lipschitz function, ψn is a continuous function and an and en are real numbers. When the functions {αn}n are constants (they are called vertical scaling factors), this system is extensively studied by many authors [21,22,23,27,28,29,30,31]. In this case, the set of vertical scaling factors has a decisive influence on the properties and shape of the corresponding FIF. In particular, the smoothness of FIFs can be described through the vertical scaling factor [31,32] and, therefore by choosing the appropriate vertical scale factor, they can fit the real rough curve precisely. Moreover, all aforementioned works are dealing with different choices of functions ψn and highlighted some choice of these functions. In the section 2 we recall some preliminaries concerning IFS and FIF, and in Section 3, we examine the change of the fractal interpolation curve in response to a minor perturbation on αn(x). We compute a sufficient condition so that the new (perturbed) IFS satisfies the continuity condition. Additionally, we discuss how these changes influence FIFs by computing the error estimate. In Section 4, we consider the case when Fn(x,y) are defined as follows
Fn(x,y)=αn(x)y+f(Ln(x))−αn(x)b(x), |
where the functions αn:I⟶R are Lipschitz functions and b,f are continuous functions on [x0,xN] such that b(x0)=f(x0) and b(xN)=f(xN). The FIF interpolates the function f at the nodes of the partition : x0<x1<x2⋯<xN [33,34,35]. We will compute in this case an appropriate upper bound of the maximum range of the perturbed FIF.
Let (X,d) be a complete metric space. A mapping g:X⟶X is called a contraction if there exists c∈[0,1) such that d(g(x1),g(x2))≤cd(x1,x2) for all x1,x2∈X. We define on H(X), the space of compact subsets of X, the Hausdorff metric dH as
dH(K1,K2)=max{d(K1,K2),d(K2,K1)},K1,K2∈H(X), |
where d(K1,K2)=supx∈K1infy∈K2d(x,y), then (H(X),dH) is complete space [5] and compact whenever X is compact. Now, we consider I={X, wn n∈J} to be an IFS, where wn:X⟶X is a continuous mapping for each n∈J. We define also the Hutchinson operator W:H(X)⟶H(X) as
W(A)=N⋃n=1wn(A),∀ A∈H(X). |
It is well know that any IFS admits at least one attractor, that is, a set G∈H(X) such that W(G)=G [2]. In addition [2,5], the Hutchinson operator is a contraction mapping on (H(X),dH) whenever I is hyperbolic; that is, for each n∈J, wn is a contraction.
Let N∗ be the set of positive integers and I=[x0,xN] be a real compact interval. We consider the set of data Δ={(xn,yn)∈I×R n∈J0:={0,1,…,N}}, where N∈N∗, x0<x1<⋯<xN, yi∈[a,b], with −∞<a<b<∞. Now we consider, for n∈J, the set In=[xn−1,xn] and we define the contractive homeomorphism Ln:I⟶In such that
Ln(x0)=xn−1,Ln(xN)=xn|Ln(x)−Ln(x′)|≤l|x−x′|, ∀ x,x′∈I, | (2.1) |
for some l∈[0,1). We define also N continuous mappings Fn:K:=I×[a,b]⟶R, such that
Fn(x0,y0)=yn−1,Fn(xN,yN)=yn | (2.2) |
|Fn(x,y)−Fn(x,y′)|≤|αn||y−y′|,∀x∈I,y,y′∈[a,b], | (2.3) |
where αn∈(−1,1),n∈J. Now, we define the mapping Wn:K⟶In×R, as
Wn(x,y)=(Ln(x),Fn(x,y)),∀(x,y)∈K,n∈J. |
Under the conditions (2.1), (2.2) and (2.3), the IFS {K,Wn:n∈J} has a unique attractor G. Furthermore, G is the graph of continuous function f that passes through the points {(xn,yn)}Nn=1 [2]. On the other hand, we define the complete metric space (G,ρ), where
G={g:I⟶R, such that g is continuous, g(x0)=x0 and g(xN)=xN} |
and
ρ(g,h)=‖g−h‖∞:=max{|g(x)−h(x)|:x∈I},∀g,h∈G. |
Now, we define the Read-Bajraktarevic operator T, defined on (G,ρ) by
T(g(x))=Fn(L−1n(x),g(L−1n(x))),x∈In,n∈J, |
then, using (2.3), we obtain ‖T(f)−T(g)‖≤α‖f−g‖∞, where α:=maxn|αn|. Hence, T is a contraction mapping and possesses a unique fixed point f on G. From this, the FIF is the unique function satisfying the following functional relation
f(x)=Fn(L−1n(x),f(L−1n(x))),∀x∈In,n∈J. | (2.4) |
The most widely studied FIFs are defined by the following system
{Ln(x)=anx+enFn(x,y)=αny+ψn(x),n∈J, | (2.5) |
where the real constants an and en are determined by condition (2.1), ψn are continuous functions such that conditions (2.2) and (2.3) hold and −1<αn<1 are free parameters called vertical scaling factors. Recently, many authors managed to construct more general FIFs that do not have to exhibit the strict self similarity (see, for instance, [15]). In this work, we consider the IFS with variable parameters [32] defined by :
Wn(x,y)=(anx+en,αn(x)y+ψn(x),),∀(x,y)∈K,n∈J, |
where αn:I⟶R are Lipschitz functions such that ‖αn‖∞:=sup{αn(x);x∈I}<1. In this case, the FIF will be called generalized affine FIF and denoted by fαΔ,N where α:=(α1,α2,…,αN) (or simply by f if there is no ambiguity). Moreover, consider that generalized affine FIF provides a wide variety of systems for different approximations problems. In Figure 1, we plot the FIF with constant parameters : α1(x)=0.4, α2(x)=0.3, α3(x)=0.5 and α4(x)=0.2. Meanwhile in Figure 2, we plot the FIF with variable parameters : α1(x)=0.4sin(5x)+0.2, α2(x)=0.3cos(10x), α3(x)=0.5exp(−2x)+0.3 and α4(x)=0.2exp(x)sin(x)+0.1. As we can see, the self-similarity in Figure 2 is weaker than that of the affine fractal interpolation curve shown in Figure 1 [32].
In this section we consider the FIFs generated through the following IFS
{Ln(x)=anx+enFn(x,y)=αn(x)y+ψn(x),n∈J, | (3.1) |
where an and en are determined by condition (2.1) and the functions ψn are continuous functions such that conditions (2.2) and (2.3) hold. Assume that αn:I⟶R are Lipschitz functions, with Lipschitz constant Cn, such that α:=maxn‖αn‖∞<1. Now we define, for x∈I,
{Ln1n2…nk(x):=Ln1∘Ln2∘⋯∘Lnk(x)Ln1n2…nk(I):=Ln1∘Ln2∘⋯∘Lnk(I), |
where nj∈J, k≥1, j∈{1,…,k}. Finally, let σj denote the j-fold composition of σ with itself such that
Lσj(n1n2…nk)(x)=Lnj+1…nk(x),1≤j≤k−1, |
while Lσk(n1n2…nk)(x)=x. Using a successive iteration and induction (see [36]), we have, for all nj∈J, j=1,…,k,
Ln1n2…nk(x)=(k∏j=1anj)x+k∑r=1(r−1∏j=1anj)enr, | (3.2) |
with the convention ∏0j=1Sj(x)=1 for any family of functions {Sj}j. In this section we consider the following perturbation of the IFS defined in (3.1),
{Ln(x)=anx+en˜Fn(x,y)=βn(x)y+ψn(x)+λn(x), | (3.3) |
where βn and λn are continuous functions such that ‖βn‖∞<1. We define also
δn(x):=βn(x)−αn(x)andγn:=λn(xN)−λn(x0). |
We will say that the IFS satisfies the continuous condition if, for 1≤n<N, we have
˜Fn(xN,yN)=˜Fn+1(x0,y0). |
We assume, for all 1≤n<N, that
λn+1(x0)=(n∑j=1δj(xN))yN−(n+1∑j=2δj(xN))y0+(n∑j=1γj)+λ1(x0). | (3.4) |
Notice that by using (3.2) and the fact that |an|<1 for n∈J that
x=limk→∞Ln1n2…nk(x)=∞∑r=1(r−1∏j=1anj)enr, |
for all x∈I. Our main result in this section is the following.
Theorem 1. Let f and ˜f be the FIFs generated by the IFSs (3.1) and (3.3), respectively.
1. Under (3.4) the IFS defined by the system (3.3) satisfies the continuous condition.
2. For a given x∈I, let {nj},nj∈J be sequence such that x=∑∞r=1(∏r−1j=1anj)enr, then
˜f(x)−f(x)=∞∑r=1[r−1∏j=1βnj(Lj[x])−r−1∏j=1αnj(Lj[x])]ψnr(Lr[x])+∞∑r=1[r−1∏j=1βnj(Lj[x])]λnr(Lr[x]), |
where Lr[x]=∑∞l=1(∏l−1j=1anr+j)enr+l.
Proof. 1. The continuous condition implies, for each n=1,…,N−1, that ˜Fn(xN,yN)=˜Fn+1(x0,y0), then
βn+1(x0)y0+ψn+1(x0)+λn+1(x0)=βn(xN)yN+ψn(xN)+λn(xN). |
Thus, we obtain δn+1(x0)y0+λn+1(x0)=δn(xN)yN+λn(xN) and then
λn+1(x0)=δn(xN)yN−δn+1(x0)y0+λn(xN)=δn(xN)yN−δn+1(x0)y0+γn+λn(x0)=(δn(xN)+δn−1(xN))yN−(δn+1(x0)+δn(x0))y0+γn+γn−1+λn−1(x0)⋮=(n∑j=1δj(xN))yN−(n+1∑j=2δj(x0))y0+(n∑j=1γj)+λ1(x0). |
2. Using (3.2), we have
f(Ln1n2⋯nk(x))=[k∏j=1αnj(Lσj(n1n2…nk)(x))]f(x)+k∑r=1[r−1∏j=1αnj(Lσj(n1n2…nk)(x))]ψnr(Lσr(n1n2…nk)(x)) |
and
˜f(Ln1n2⋯nk(x))=[k∏j=1βnj(Lσj(n1n2…nk)(x))]˜f(x)+k∑r=1[r−1∏j=1βnj(Lσj(n1n2…nk)(x))]⋅[ψnr(Lσr(n1n2…nk)(x))+λnr(Lσr(n1n2…nk)(x))]. |
Now remark that ⋂∞k=1Ln1n2…nk(I) consists of a single point in I for any sequence {nk} of integers such that 1≤nk≤N. Moreover, for any fixed x∈I, there exists a sequence {nk} satisfying
{x}=∞⋂k=1Ln1n2…nk(I)=limk→∞Ln1n2…nk(I). |
This implies by using (3.2) that
x=limk→∞Ln1n2…nk(x′)=∞∑r=1(r−1∏j=1anj)enr, |
where x′ is any point belonging to I. Therefore, there exists x′∈I such that
f(x)=limk→∞k∑r=1[r−1∏j=1αnj(Lσj(n1n2…nk)(x′))]ψnr(Lσr(n1n2…nk)(x′)).=∞∑r=1[r−1∏j=1αnj(Lj[x])]ψnr(Lr[x]). |
Similarly, we have
˜f(x)=∞∑r=1[r−1∏j=1βnj(Lj[x])](ψnr(Lr[x])+λnr(Lr[x])), |
then we obtain the desired result.
Example 1. We consider the Weierstrass function (see Figure 3) that can be seen as a classical fractal function since it is continuous everywhere, yet differentiable nowhere. Therefore, while its graph is connected, it looks jagged when viewed on arbitrarily small scales. There are many works on fractal dimensions of their graphs, including box dimension, Hausdorff dimension, and other types of dimensions [37,38]. Let l≥2 be an integer, 1/l<λ<1 and ϕ:R⟶R is a Z-periodic real analytic function. We define
fϕλ,l(x)=∞∑k=0λkϕ(lkx),x∈R. | (3.5) |
In fact, such a function is real analytic, or the Hausdorff dimension of its graph is equal to 2+logl(λ) [39].
In this example, we consider the classical Weierstrass function f; that is, when ϕ(x)=cos(2πx). Let I=[0,1], N=l=3 and choose in definition (3.5) the special case λ=1/2, then we obtain f(0)=f(1)=2. Now, consider
Wn(x,y)=(x+n−13,αn(x)y+ϕ(x+n−13)),(x,y)∈I×R, |
where αn(x)=12+(−1)1+⌊n/2⌋sin(2πx)4, for n∈{1,2,3}, where ⌊n/2⌋ means the integer part of x. In this case, we have that the Weierstrass function f is the FIF defined by {Wn}3n=1 [40]. Now, consider the following perturbed system defined by
{Ln(x)=x+n−13˜F(x,y)=βn(x)y+ϕ(x+n−13)+λn(x),(x,y)∈I×R, | (3.6) |
where βn(x)=(−1)1+⌊n/2⌋sin(2πx)4 for n∈{1,2,3}. In this case, we have δn(x):=δn=−12. Choose λ1(x)=x and then, by (3.4), we have
λn+1(x0)=2(n∑j=1δj)−2(n+1∑j=2δj)+(n∑j=1γj)=n∑j=1γj. |
Hence, if we take λ2(x)=λ3(x)=x+1, the system defined in (3.6) satisfies the continuous condition.
In the following, we consider a special case of Theorem 1. For this, we define the following perturbation of the IFS defined in (2.5):
{Ln(x)=anx+en˜Fn(x,y)=(αn+δn)y+ψn(x)+λn, | (3.7) |
where δn and λn are constants such that |αn+δn|<1. In this situation, we have γn=0 and we obtain the following result.
Theorem 2. Let f and ˜f be the functions generated by the IFSs (2.5) and (3.7), respectively. For a given x∈I, let {nj},nj∈J be sequence such that x=∑∞r=1(∏r−1j=1anj)enr.
1. Assume for all 1≤n<N that
λn+1=(n∑j=1δj)yN−(n+1∑j=2δj)y0+λ1, |
then the IFS defined by the system (3.7) satisfies the continuous condition.
2. For a given x∈I, let {nj},nj∈J be sequence such that x=∑∞r=1(∏r−1j=1anj)enr, then
|˜f(x)−f(x)|≤∞∑r=1|(s+t)r−1−tr−1|ψnr(∞∑l=1(l−1∏j=1anr+j)enr+l)+∞∑r=1(r−1∏j=1βnj)λnr, |
where t:=maxn|αn| and s=maxn|βn−αn|.
Proof. The first assertion is a direct application of Theorem 1, and we will only prove the second one. Using Theorem 1, we obtain
˜f(x)−f(x)=∞∑r=1[r−1∏j=1βnj−r−1∏j=1αnj]ψnr(∞∑l=1(l−1∏j=1anr+j)enr+l)+∞∑r=1(r−1∏j=1βnj)λnr. | (3.8) |
Note that (3.8) can be found in [41, Theorem 3]. Moreover, using [32, Lemma 3.2], we have
|˜f(x)−f(x)|≤∞∑r=1|(s+t)r−1−tr−1|ψnr(∞∑l=1(l−1∏j=1anr+j)enr+l)+∞∑r=1(r−1∏j=1βnj)λnr, |
where t:=maxn|αn| and s=maxn|βn−αn|.
Remark 1. Consider the IFS defined by (3.7) such that α:=α1=⋯=αN. Choose δ=1−α2 so that we have α+δ<1. Therefore, by a direct computation using (2.4), we obtain for all x∈I
|˜f(x)−f(x)|≤maxn∈J|˜Fn(L−1n(x),˜f∘L−1n(x))−Fn(L−1n(x),f∘L−1n(x))|=maxn∈J|(α+δ)˜f(L−1n(x))+λn−αf(L−1n(x))|≤α‖˜f−f‖∞+δ‖˜f−f‖∞+δ‖f‖∞+λ∞, |
where λ∞:=maxn∈Jλn. It follows that
‖˜f−f‖∞≤δ‖f‖∞+λ∞1−α−δ≤21−α(‖f‖∞+λ∞). |
Now, applying Theorem 2, we get
|˜f(x)−f(x)|≤∞∑r=1(1+α2)r−1maxn‖ψn‖∞+∞∑r=1(1+α2)r−1λ∞≤21−α(maxn‖ψn‖∞+λ∞). |
Example 2. In this example, we consider a special case of Theorem 2 by choosing all the parameters λn as equal for n∈J. This is done by choosing y0=yN=0. For this, let us consider the sets of data points:
Δ:={(0,0),(1/3,1),(2/3,−1),(1,0)}, |
and we consider the IFS defined by (2.5) where
Ln(x)=x3+n−1N,ψ1(x)=x,ψ2(x)=1−2x,ψ3(x)=x−1andα1=α2=α3=−0.5. |
Now, we consider the following perturbed system
{Ln(x)=x3+n−1N˜Fn(x,y)=(αn+δn)y+ψn(x)+λn. | (3.9) |
We choose λ1=0 and we collect the different values of δn in the Table 1. Moreover, different perturbed FIF are plotted in Figure 4. As we may see, we obtain smooth or non-smooth FIF depending on the choice of δn. One can describe the self-similar structures of the graph of FIF by computing the box dimension D (also known as the Minkowski–Bouligand dimension or the box-counting dimension), which is a mathematical object used to describe the complexity of certain figures and proved to be an appropriate and effective method for fractal dimension estimate. More precisely,
D=limϵ→0logNϵlog(1/ϵ), | (3.10) |
where Nϵ is the minimum number of ϵ×ϵ squares needed to cover the graph of f.
δ | λ | box dimension | |
Initial system | δn=0 | λn=0 | 1.369 |
First perturbation | δn=1.25 | λn=0 | 1.738 |
Second perturbation | δ1=0.65 δ2=0.6 δ3=0.85 |
λn=0 | 1 |
Third perturbation | δ1=1 δ2=0.65 δ3=−0.3 |
λn=0 | 1.338 |
As an application of Theorem 2, the following result concerning the Weierstrass function is considered in Example 1.
Corollary 1. Let f be the Weierstrass function defined in (3.5) with ϕ(0)=0, then there exists an FIF ˜f satisfying
‖˜f−f‖∞≤2‖ϕ‖∞1−λ. |
Proof. Let I=[0,1] and the interpolating points x0=0<x1<⋯<xN=1 such that xn−xn−1=1/N (N=l). We consider the following system defined as
{Ln(x)=xN+n−1NFn(x,y)=αy+ϕ(x+n−1N), |
where α=λ. It is well known that the function f is an FIF [40]. Indeed, consider for n∈J the function
Wn(x,y)=(x+n−1N,αy+ϕ(x+n−1N)),(x,y)∈[0,1]×R. |
It follows that
f(Ln(x))=f(x+n−1N)=ϕ(x+n−1N)+α∞∑k=0αkϕ(Nkx)=ϕ(x+n−1N)+αf(x) |
and, thus,
Cf=N⋃n=1Wn(Cf). |
Now, we consider the following perturbed system
{Ln(x)=xN+n−1NFn(x,y)=(α+δ)y+ϕ(x+n−1N)+λn, |
where δ=(1−α)/2 and λ1=0, and we obtain
λn+1=n2(1−α)yN−n2(1−α)y0=n2(1−α)[yN−y0]. |
Furthermore, using Remark 1, we get
‖˜f−f‖∞≤21−α(‖ϕ‖∞+λ∞)≤21−α(‖ϕ‖∞+N2(1−α)(yN−y0)). |
Finally, we get the desired result since ϕ is a Z-periodic function with ϕ(0)=0, which implies that f(0)=f(1) and yN=y0.
Let f∈C(I), the normed space of real valued endowed with the uniform norm continuous function on I. We will say that the function f is Hölder continuous with exponent β and Hölder constant c if
|f(x)−f(y)|≤c|x−y|θ,x,y∈I. |
We denote by Hθ(I) the set of all Hölder continuous functions on I with exponent θ. We consider the interpolation points P={(nN,yn)∈R2,n∈J} and we denote D={nN∈I,n∈J0}. We set
L0(D)=D,L(D)=N⋃n=1Ln(D),and Lk(D)=L∘⋯∘L(D), |
k times composition. In this section, we consider
{Ln(x)=anx+enFn(x,y)=αn(x)y+f(Ln(x))−αn(x)b(x), | (4.1) |
where the real constants an and en are determined by condition (2.1), the functions αn:I⟶R are Lipschitz functions, with Lipschitz constant Cn such that α:=maxn‖αn‖∞<1 and b∈C(I) such that b(x0)=f(x0) and b(xN)=f(xN). The FIF generated by (4.1) will be denoted by fα, which interpolates f at the nodes of the partition. Moreover, using (2.4), the function fα satisfies
fα(x)=f(x)+αn(L−1n(x))(fα−b)(L−1n(x)),for allx∈In,n∈J. | (4.2) |
We will denote by ˜fα the FIF generated by the perturbed system; that is
˜Fn(x,y)=βn(x)y+f(Ln(x))−αn(x)b(x)+λn(x). | (4.3) |
Lemma 1. Assume that β:=maxn‖βn‖∞<1. For all x∈In and n∈J, we have
‖˜fα−f‖∞≤α‖f−b‖∞+δ‖f‖∞+‖λ‖∞1−β |
and
‖˜fα−fα‖∞≤β‖fα‖∞+‖λ‖∞1−β, |
where ‖λ‖∞=maxn‖λn‖∞, α=maxn‖αn‖∞ and δ=maxn‖δn‖∞.
Proof. Using (2.4), we obtain
˜fα(x)=f(x)+βn(L−1n(x))˜fα(L−1n(x))−αn(L−1n(x))b(L−1n(x))+λn(L−1n(x)) | (4.4) |
and
(1−β)‖˜fα(x)−f(x)‖∞≤α‖f−b‖∞+δ‖f‖∞+‖λ‖∞ |
as required. Moreover, from (4.4) and (4.2), ˜fα(x)=fα(x)+βn(L−1n(x))˜fα(L−1n(x))+λn(L−1n(x)) and
‖˜fα−fα‖∞≤β‖˜fα−fα‖∞+β‖fα‖∞+‖λ‖∞. |
Now, assume that f∈Hθ1(I) and b∈Hθ2(I) with Hölder constant Hf, Hb respectively.
Lemma 2. Let ˜fα be the FIF generated by the system (4.3) and we assume that β=maxn‖βn‖∞<1. We denote α=maxn‖αn‖∞, δ=maxn‖δn‖∞ and A1=βΓ1+Hf+αHb+y0δ1−β, then
|˜fα(x)−yn−1|≤A1,x∈In,∀n∈J. |
Proof. We define, for k=1,2,…,
Γk=max{|˜fα(x)−y0|,x∈Lk−1(D)}andγk=maxn{|˜fα(x)−yn−1|,x∈Lk−1(D)∩In}. |
First, observe that
Γk≤maxn{|˜fα(x)−yn−1|,x∈Lk−1(D)∩In}+maxn{|yn−1−y0|}≤Γ1+γk. | (4.5) |
For x∈Lk(D)∩In, we have
˜fα(x)=f(x)+βn(L−1n(x))˜fα(L−1n(x))−αn(L−1n(x))b(L−1n(x))+λn(L−1n(x)) |
and then
˜fα(x)−yn−1=f(x)−yn−1+βn(L−1n(x))[˜fα(L−1n(x))−y0]−αn(L−1n(x))[b(L−1n(x))−y0]+λn(L−1n(x))+y0δn(L−1n(x))≤Hf+βΓk−1+αHb+y0δ. |
We denote by A=Hf+αHb+y0δ, which does not depend on k. It follows by using (4.5) that
γk+1≤βΓk+A≤βγk+βΓ1+A≤β(βΓk−1+A)+βΓ1+A≤β2γk−1+β2Γ1+βΓ1+βA+A⋮≤k∑j=1βjΓ1+k−1∑j=0βjA≤βΓ1+A1−β=A1. |
For any x∈In, there exits a sequence {xj}j∈In∩(⋃kLk(D)) such that xj⟶x and limj→∞|˜fα(xj)−yn−1|=|˜fα(x)−yn−1|, by continuity of the function ˜fα. Therefore, we get
|˜fα(x)−yn−1|≤A1,x∈In. |
Given a function S defined on I, we define the maximum range RS of S as
RS(I)=sups1,s2∈I|S(s1)−S(s2)|. |
Theorem 3. Let fα be the α-FIF the IFS (4.1) with interpolation points P and ˜fα as the perturbed FIF defined by (4.3). Assume that β=maxn‖βn‖∞<1, then
R˜fα(I)≤min{NA1,21−β(α‖b‖∞+‖λ‖∞+‖f‖∞(1+α+δ−β))} |
where A1=βΓ1+Hf+αHb+y0δ1−β, α=maxn‖αn‖∞ and δ=maxn‖δn‖∞.
Proof. From Lemma 2, we have
supIn|˜fα(x)−yn−1|≤A1, |
Now, let s1,s2∈I, then there exists n1≤n2∈J such that s1∈In1 and s2∈In2. It follows that
|˜fα(s1)−˜fα(s2)|≤|˜fα(s1)−yn1−1|+|yn1−1−yn1|+⋯+|yn2−1−˜fα(s2)|.≤NA1. |
On the other hand, we may estimate the upper bound of the maximum range R˜fα not depending on N. Indeed, using Lemma 1 we get
R˜fα≤2‖˜fα‖∞≤2‖˜fα−f‖∞+2‖f‖∞≤2α‖f−b‖∞+δ‖f‖∞+‖λ‖∞1−β+2‖f‖∞≤21−β(α‖b‖∞+‖λ‖∞+‖f‖∞(1+α+δ−β)) |
as required.
Remark 2. Let fα be the α-FIF the IFS (4.1) with interpolation points P such that α<1, then
Rfα(I)≤min{NA2,21−α(α‖b‖∞+‖f‖∞)} |
where A2=αΓ1+Hf+αHb1−α.
Example 3. Let I=[0,1] and f(x)=x−x2. Observe that for any x,y∈I, we have
|f(x)−f(y)|≤|x−y|+|x2−y2|≤3|x−y|, |
then the function f is Hölder continuous with exponent one and Hölder constant Hf=3. In this example, we consider the following perturbed system
{Ln(x)=xN+n−1NFn(x,y)=(α+δ)y+f(Ln(x))−αb(x)+λn, |
where δ=(1−α)/2, λ1=0 and b(x)=f(x)/3. Since f(0)=f(1)=0, we obtain λn=0 for all n∈J. Therefore, using Lemma 1, we have
‖˜fα−f‖∞≤α‖f−b‖∞+δ‖f‖∞1−β≤α/3+(1−α)/41−α=α+312(1−α) |
and
‖˜fα−fα‖∞≤1+α1−α‖fα‖∞. |
In particular, if α=1/4, we obtain
‖˜fα−f‖∞≤1336and‖˜fα−fα‖∞≤53‖fα‖∞. |
Therefore, we have
R˜fα(I)≤21−β(α‖b‖∞+‖λ‖∞+‖f‖∞(1+α+δ−β))≤11−α(α/12+1/4), |
then R˜fα(I)≤1336 for α=1/4.
In this paper, a class of generalized affine FIFs with variable parameters, where ordinate scaling is substituted by real-valued control function, is investigated. More precisely, we considered the FIF generated through the IFS defined by the functions Wn(x,y)=(an(x)+en,αn(x)y+ψn(x)), n=1,…,N. We computed the error estimate in response to a small perturbation on αn(x) and we gave a sufficient condition on the perturbed IFS so that it satisfies the continuity condition.
The authors declare they have not used Artificial Intelligence (AI) tools in the creation of this article.
The authors extend their appreciation to the Deanship of Scientific Research, Vice Presidency for Graduate Studies and Scientific Research at King Faisal University, Saudi Arabia, for financial support under the annual funding track [GRANT 5416].
The authors declare no conflicts of interest.
[1] | M. Barnsley, S. Demko, Iterated function systems and the global construction of fractals, Proc Roy Soc London, 1985. |
[2] |
M. F. Barnsley, Fractal functions and interpolation, Constr. Approx., 2 (1986), 303–329. https://doi.org/10.1007/BF01893434 doi: 10.1007/BF01893434
![]() |
[3] | M. Barnsley, A. Harrington, The calculus of fractal interpolation functions, J Approx Th 1989. |
[4] |
J. E. Hutchinson, Fractals and self-similarity, Indiana Univ. Math. J., 30 (1981), 713–747. https://doi.org/10.1512/iumj.1981.30.30055 doi: 10.1512/iumj.1981.30.30055
![]() |
[5] | M. F. Barnsley, Fractals everywhere, Boston: Academic Press, 1988. |
[6] |
G. E. Hardy, T. D. Rogers, A generalization of a fixed point theorem of Reich, Can. Math. Bull., 16 (1973), 201–206. https://doi.org/10.4153/CMB-1973-036-0 doi: 10.4153/CMB-1973-036-0
![]() |
[7] |
A. Petrusel, I. A. Rus, M. A. Serban, Fixed points, fixed sets and iterated multifunction systems for nonself multivalued operators, Set-Valued Var. Anal., 23 (2015), 223–237. https://doi.org/10.1007/s11228-014-0291-6 doi: 10.1007/s11228-014-0291-6
![]() |
[8] |
X. Y. Wang, F. P. Li, A class of nonlinear iterated function system attractors, Nonlinear Anal. Theor., 70 (2009), 830–838. https://doi.org/10.1016/j.na.2008.01.013 doi: 10.1016/j.na.2008.01.013
![]() |
[9] | K. R. Wicks, Fractals and hyperspaces, 1991. Berlin, Heidelberg: Springer. https://doi.org/10.1007/BFb0089156 |
[10] | Mihail, R. Miculescu, The shift space for an infinite iterated function system, Math. Rep., 11 (2009), 21–32. |
[11] |
A. Mihail, R. Miculescu, Generalized IFSs on non-compact spaces, Fixed Point Theory Appl., 2010 (2010), 584215. https://doi.org/10.1155/2010/584215 doi: 10.1155/2010/584215
![]() |
[12] |
F. Strobin, J. Swaczyna, On a certain generalization of the iterated function system, Bull. Aust. Math. Soc., 87 (2013), 37–54. https://doi.org/10.1017/S0004972712000500 doi: 10.1017/S0004972712000500
![]() |
[13] |
K. Leśniak, Infinite iterated function systems: A multivalued approach, Bulletin Polish Acad. Sci. Math., 52 (2004), 1–8. https://doi.org/10.4064/ba52-1-1 doi: 10.4064/ba52-1-1
![]() |
[14] | N. A. Secelean, Countable iterated function systems, Far East J. Dyn. Syst., 3 (2001), 149–167. |
[15] |
N. Attia, H. Jebali, On the construction of recurrent fractal interpolation functions using Geraghty contractions, Electron. Res. Arch., 31 (2023), 6866–6880. https://doi.org/10.3934/era.2023347 doi: 10.3934/era.2023347
![]() |
[16] |
N. Attia, M. Balegh, R. Amami, R. Amami, On the Fractal interpolation functions associated with Matkowski contractions, Electron. Res. Arch., 31 (2023), 4652–4668. https://doi.org/10.3934/era.2023238 doi: 10.3934/era.2023238
![]() |
[17] |
S. Ri, A new nonlinear fractal interpolation function, Fractals, 25 (2017), 1750063. https://doi.org/10.1142/S0218348X17500633 doi: 10.1142/S0218348X17500633
![]() |
[18] |
S. Ri, New types of fractal interpolation surfaces, Chaos Solitons Fractals, 119 (2019), 291–297. https://doi.org/10.1016/j.chaos.2019.01.010 doi: 10.1016/j.chaos.2019.01.010
![]() |
[19] | M. A. Navascués, C. Pacurar, V. Drakopoulos, Scale-free fractal interpolation, Fractal Fract., 6 (2022), 602. |
[20] |
J. Kim, H. Kim, H. Mun, Nonlinear fractal interpolation curves with function vertical scaling factors, Indian J. Pure Ap. Mat., 51 (2020), 483–499. https://doi.org/10.1007/s13226-020-0412-x doi: 10.1007/s13226-020-0412-x
![]() |
[21] |
A. K. B, Chand, G. P. Kapoor, Generalized cubic spline fractal interpolation functions, SIAM J. Numer. Anal., 44 (2006), 655–676. https://doi.org/10.1137/040611070 doi: 10.1137/040611070
![]() |
[22] | M. A. Navascuès, M. V. Sebastian, Smooth fractal interpolation, Journal of Inequalities and Applications Volume 2006, Article ID 78734, 1–20, https://doi.org/10.1155/JIA/2006/78734 |
[23] |
P. Viswanathan, A. K. B. Chand, M. A. Navascuès, Fractal perturbation preserving fundamental shapes: Bounds on the scale factors, J. Math. Anal. Appl., 419 (2014), 804–817. https://doi.org/10.1016/j.jmaa.2014.05.019 doi: 10.1016/j.jmaa.2014.05.019
![]() |
[24] |
N. Vijender, Bernstein fractal trigonometric approximation, Acta Appl. Math., 159 (2018), 11–27. https://doi.org/10.1007/s10440-018-0182-1 doi: 10.1007/s10440-018-0182-1
![]() |
[25] | E. Mihailescu, Unstable directions and fractal dimension for skew products with overlaps in fibers, Math Zeit, 2011. |
[26] | E. Mihailescu, M. Urbanski, Hausdorff dimension of the limit set of conformal iterated function systems with overlaps, Proc Amer Math Soc, 2011. |
[27] | C. Gang, The smoothness and dimension of fractal interpolation functions, Appl. Math. JCU, 11 (1996), 409–418. |
[28] |
Md. Nasim Akhtar, M. Guru Prem Prasad, M. A. Navascués, Box dimension of α-fractal function with variable scaling factors in subintervals, Chaos Soliton. Fract., 103 (2017), 440–449. https://doi.org/10.1016/j.chaos.2017.07.002 doi: 10.1016/j.chaos.2017.07.002
![]() |
[29] |
N. Attia, T. Moulahi, R. Amami, N. Saidi, Note on Fractal interpolation function with variable parameters, AIMS Math., 9 (2024), 2584–2601. https://doi.org/10.3934/math.2024127 doi: 10.3934/math.2024127
![]() |
[30] | A. K. B. Chand, G. P. Kapoor, Smoothness analysis of coalescence hidden variable fractal interpolation functions, Int. J. Nonlinear Sci., 3 (2007), 15–26. |
[31] |
A. K. B. Chand, G. P. Kapoor, Stability of affine coalescence hidden variable fractal interpolation functions, Nonlinear Anal. Theor., 68 (2008), 3757–3770. https://doi.org/10.1016/j.na.2007.04.017 doi: 10.1016/j.na.2007.04.017
![]() |
[32] | H. Y. Wang, J. S. Yu, Fractal interpolation functions with variable parameters and their analytical proper ties, J. Approx. Theory, 175 (2013), 1–18. |
[33] | M. A. Navascués, Fractal trigonometric approximation, Electron. Trans. Numer. Anal., 20 (2005), 64–74. |
[34] |
M. A. Navascués, Fractal polynomial interpolation, Z. Anal. Anwend., 24 (2005), 401–418. https://doi.org/10.4171/ZAA/1248 doi: 10.4171/ZAA/1248
![]() |
[35] | M. A. Navascués, Fractal functions on the sphere, J. Comput. Anal. Appl., 9 (2007), 257–270. |
[36] |
H. Y. Wang, X. J. Li, Perturbation error analysis for fractal interpolation functions and their moments, Appl. Math. Lett., 21 (2008), 441–446. https://doi.org/10.1016/j.aml.2007.03.026 doi: 10.1016/j.aml.2007.03.026
![]() |
[37] |
H. J. Ren, W. X. Shen, A dichotomy for the Weierstrass-type functions, Invent. Math., 226 (2021), 1057–1100. https://doi.org/10.1007/s00222-021-01060-2 doi: 10.1007/s00222-021-01060-2
![]() |
[38] | T. Y. Hu, K. S. Lau, Fractal dimensions and singularities of the Weierstrass type functions, Trans. Amer. Math. Soc., 335 (1993), 649–665. |
[39] | W. Shen, Hausdorff dimension of the graphs of the classical Weierstrass functions, Math. Z., 289 (2018), 223–266. |
[40] |
L. Jiang, H. J. Ruan, Box dimension of generalized affine fractal interpolation functions, J. Fractal Geom., 10 (2023), 279–302. https://doi.org/10.4171/JFG/136 doi: 10.4171/JFG/136
![]() |
[41] | J. Xu, Z. Feng, Fractal Interpolation Functions on the Stability of Vertical Scale Factor, Int. J. Nonlin. Sci., 13 (2012), 380–384. |
1. | Najmeddine Attia, Rim Amami, On linear transformation of generalized affine fractal interpolation function, 2024, 9, 2473-6988, 16848, 10.3934/math.2024817 |
δ | λ | box dimension | |
Initial system | δn=0 | λn=0 | 1.369 |
First perturbation | δn=1.25 | λn=0 | 1.738 |
Second perturbation | δ1=0.65 δ2=0.6 δ3=0.85 |
λn=0 | 1 |
Third perturbation | δ1=1 δ2=0.65 δ3=−0.3 |
λn=0 | 1.338 |