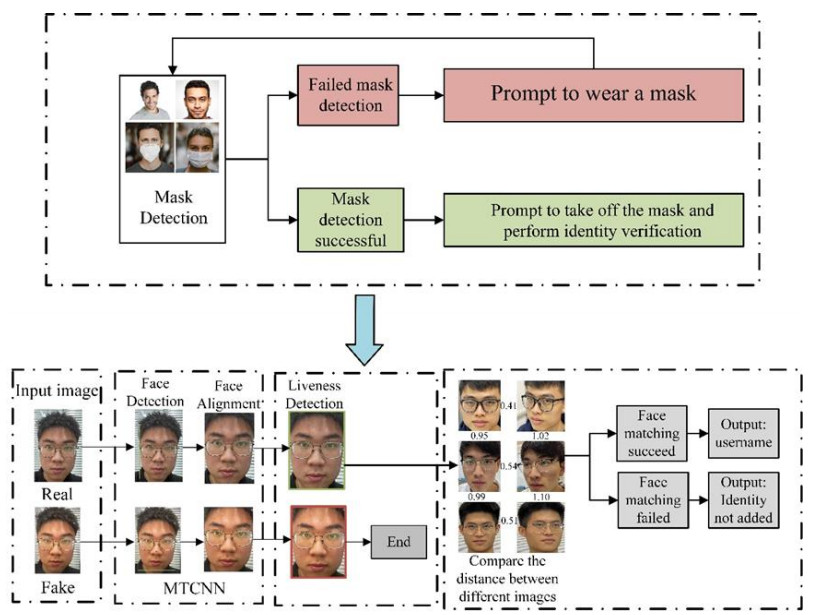
In this study, we deal with the problem of extended dissipativity analysis for memristive neural networks (MNNs) with two-delay components. The goal is to get less conservative extended dissipativity criteria for delayed MNNs. An improved Lyapunov-Krasovskii functional (LKF) with some generalized delay-product-type terms is constructed based on the dynamic delay interval (DDI) method. Moreover, the derivative of the created LKF is estimated using the integral inequality technique, which includes the information of higher-order time-varying delay. Then, sufficient conditions are attained in terms of linear matrix inequalities (LMIs) to pledge the extended dissipative of MNNs via the new negative definite conditions of matrix-valued cubic polynomials. Finally, a numerical example is shown to prove the value and advantage of the presented approach.
Citation: Zirui Zhao, Wenjuan Lin. Extended dissipative analysis for memristive neural networks with two-delay components via a generalized delay-product-type Lyapunov-Krasovskii functional[J]. AIMS Mathematics, 2023, 8(12): 30777-30789. doi: 10.3934/math.20231573
[1] | Lijun Hao . New proofs for three identities of seventh order mock theta functions. AIMS Mathematics, 2023, 8(2): 4806-4813. doi: 10.3934/math.2023238 |
[2] | Aslıhan ILIKKAN CEYLAN, Canan HAZAR GÜLEÇ . A new double series space derived by factorable matrix and four-dimensional matrix transformations. AIMS Mathematics, 2024, 9(11): 30922-30938. doi: 10.3934/math.20241492 |
[3] | Fei Hou, Bin Chen . Triple correlation sums of coefficients of θ-series. AIMS Mathematics, 2023, 8(10): 25275-25287. doi: 10.3934/math.20231289 |
[4] | Chuanan Wei, Chun Li . New q-supercongruences arising from a summation of basic hypergeometric series. AIMS Mathematics, 2022, 7(3): 4125-4136. doi: 10.3934/math.2022228 |
[5] | Long Li . Double series expansions for π. AIMS Mathematics, 2021, 6(5): 5000-5007. doi: 10.3934/math.2021294 |
[6] | Tie-Hong Zhao, Zai-Yin He, Yu-Ming Chu . On some refinements for inequalities involving zero-balanced hypergeometric function. AIMS Mathematics, 2020, 5(6): 6479-6495. doi: 10.3934/math.2020418 |
[7] | Savin Treanţă, Cristina-Florentina Marghescu, Laura-Gabriela Matei . Efficiency conditions in multiple-objective optimal control models under generalized hypotheses. AIMS Mathematics, 2024, 9(9): 25184-25204. doi: 10.3934/math.20241228 |
[8] | Nadia N. Li, Wenchang Chu . Explicit formulae for Bernoulli numbers. AIMS Mathematics, 2024, 9(10): 28170-28194. doi: 10.3934/math.20241366 |
[9] | Robert Reynolds, Allan Stauffer . Extended Prudnikov sum. AIMS Mathematics, 2022, 7(10): 18576-18586. doi: 10.3934/math.20221021 |
[10] | Jiaye Lin . Evaluations of some Euler-type series via powers of the arcsin function. AIMS Mathematics, 2025, 10(4): 8116-8130. doi: 10.3934/math.2025372 |
In this study, we deal with the problem of extended dissipativity analysis for memristive neural networks (MNNs) with two-delay components. The goal is to get less conservative extended dissipativity criteria for delayed MNNs. An improved Lyapunov-Krasovskii functional (LKF) with some generalized delay-product-type terms is constructed based on the dynamic delay interval (DDI) method. Moreover, the derivative of the created LKF is estimated using the integral inequality technique, which includes the information of higher-order time-varying delay. Then, sufficient conditions are attained in terms of linear matrix inequalities (LMIs) to pledge the extended dissipative of MNNs via the new negative definite conditions of matrix-valued cubic polynomials. Finally, a numerical example is shown to prove the value and advantage of the presented approach.
With Covid-19 spreading across the world, epidemic prevention has become an essential part of public life and more and more people begin to be concerned about their health of themselves and turn to adapt to a new lifestyle. Covid-19 is a kind of respiratory infectious disease that spreads mainly through droplets and close contact. To protect people in public places from infection, one practical suggestion for people is to wear masks as possible, keep social distance, and so on. However, most public facilities cannot be directly compatible with these new normal actions under epidemic. Taking the entry scene for example, most public entries are equipped with ACS to effectively prevent idle personnel, recognize people and check their identities. In order to prevent entry procedures causing the spread of the epidemic, it is not suggested people to taking off masks or sojourn on the ACS. Therefore, it needs to enable ACS with high-level biometric recognition capability and passage efficiency. As one popular direction of computer vision research, biometric recognition is widely used in security systems due to its safety and convenience. Nowadays common biometric recognition methods include face recognition, fingerprint recognition, iris recognition, voice recognition, etc. Among these methods, face recognition is supposed to be the most convenient compared with others, especially its non-contact characteristics match the requirement of Covid-19 epidemic prevention. In most public entry conditions, a face recognition module is implemented based on a monocular camera. In practice, this design has to confront several challenges, such as mask-wearing reorganization and Face Anti-Spoofing Detection (FASD). The article will discuss the solutions we think of for the above two problems.
In terms of mask-wearing recognition, this paper focuses on the fusion between mask detection and ACS based on face recognition in order to remind users to wear masks correctly. Qin et al. [1] proposed a facemask-wearing condition identification method, which is divided into three conditions for face mask detection and includes a face with masks correctly, faces with masks incorrectly and faces without masks. This method achieves relatively satisfying results in experiments but lacks robustness under complex background. Ejaz et al. [2] proposed another face masks detection method based on Principal Component Analysis (PCA). This method meets the application requirement on the embedded devices, but the accuracy of face-mask detection is not good. In order to deal with the lack of datasets, Jiang et al. [3] designed one kind of mask detector which uses transfer learning to enhance networks robustness which increases the capability of image feature extraction in the Backone. Through using feature pyramid networks (FPN) [4] and Convolutional Block Attention Module (CBAM) [5], this method increases the accuracy of classification results and enables the frame compatible with embedded systems too.
Completing face recognition is divided into two tasks: Face identification task and face verification task. The face identification task compares one face with face datasets from which the most possible identity will be found. The face verification task is a binary classifier, it makes use of a similarity metric algorithm to get confidence whether this identity indeed matches the tested face. Thanks to the recent developments of Convolutional Neural Networks (CNNs), face recognition has achieved great progress, but there are many challenges, such as illumination, pixel resolution, and so on. There are two ideas for face recognition in the era of deep learning: Metric learning [6] and margin-based classification [9,10,11,12]. Metric learning is also called distance metric learning, which classifies input images by calculating the similarity between two input images. However, the model training relies on so large data that the efficiency of the fitting is very slow. Margin-based classification is margin limited to feature layer by modifying softmax formula indirectly. This method makes the network obtain a feature that is more discriminative than the feature obtained after training. But the model has the instrict of overfitting because of harsh constraints. Yi Sun et al. [7] proposed a DeepID model that achieves 99.15% face verification accuracy on the LFW dataset by designing deep CNNs and using both face identification and verification signals as supervision. The advantage of DeepID is its high accuracy, but its training process is also prone to be overfitted. Dung Nguyen et al. [8] proposed a framework called FaceNet that directly learns a mapping through transferring face images to a compact Euclidean distance which directly corresponds to face similarity. This method achieves an accuracy of 99.63% on the LFW and accuracy of 95.12% on YouTube FacesDB respectively. However, there are many facial features lost in the process, which makes it easy to focus on the local feature rather than the global feature. Besides, another algorithm called ArcFace Jiankang was proposed by Deng et al [9]. It has a geometric interpretation to obtain highly discriminative features for face recognition. The verification accuracy of this method is 99.83% on the LFW dataset and 98.02% on the YTF dataset. ArcFace 9has high performance, but its weight model is so large that it is much more difficult to apply in transfer learning. MV-softmax [10,11] integrates the feature margin and feature mining into one unified loss function, it implements the discriminative learning by adaptively coding the feature vector of false classification. The main disadvantage of MV-softmax is to stress hard samples or half-hard samples, this may cause a negative influence on the model coverage. CurricularFace [12] adopts one kind of adaptive strategy to train loss function using margin samples and hard samples mining, which has great value in practice.
Generally speaking, although face recognition technology has been widely studied, there still exists a balance among the network size, computation cost and generalization. In addition, new face recognition also needs to complete tasks when people wear masks as possible, this is also the focus in the research field.
Compared with mask-wearing recognition, FASD is a long-term and widely-discussed problem because nowadays the biometric information of the face is easier to capture and be utilized for spoofing. Usually, Face spoofing attacks method includes photo attacks [13,14], video attacks [15,16] driven by reinforcement learning model, and 3D mask attacks [17,18,19] within low cost, hyper-real 3D color masks. Compared with widely studied 2D face presentation attacks, 3D face spoofing attacks are more challenging because present 3D face recognition systems are difficult to balance the effectiveness and cost. A typical 3D anti-spoofing system based on holographic imaging has little availability for most public entry scenes due to its high expense. Consequently, conventional face recognition systems are vulnerable to the above proofing attacks, most widely-applied face anti-spoofing methods up to now are still Human-computer interaction (HCI) methods that verify the user through some behavior interaction, such as winking, head moving, and lip language. Therefore, it is necessary to endow ACS with the capability of verifying the authenticity of facial information, and reject illegal users who present an imitation or fake face of the enrolled user.
The core task of the FASD is to find the intrinsic difference between the sampled information and fake spoofing information. With the development of the reinforcement learning technique, it has not been difficult to produce dynamic face movement completely driven by data, which presents a challenge to the anti-spoofing method based on human-machine interaction. Up to now, the main attention is increasingly drawn on the research involved in color texture analyzing [13,14,20,21] and adaptive recognition based on deep learning [22,23], etc. Määttä et al. [19] utilized multi-scale LBP to analyze the texture of face images. Bouklenafe et al. [13,14] proposed a face anti-spoofing algorithm based on color texture. This algorithm considered the three-color space, RGB, YCbCr and HSV, which improves the model reliability in various conditions. After that, Bouklenafe et al. [13,14] started to focus on the luminance and chrominance channels and utilized the fusion mechanism of color and texture. Although FASD based on texture has drawn much attention, its performance is easy to be affected by illumination and image resolution. When the above factor decreases in quality, this method may be difficult to maintain self-stability. Another category of FASD is to monitor face movement. Usually, a genuine face has lots of movement characteristics, such as blinking, mouth opening and head-turning, while fake faces are difficult to formulate similar movements. Pan et al. [24] proposed a real-time liveness detection approach by recognizing spontaneous eyeblinks to prevent photos attack in face recognition. Zadeh et al. [25] also proposed a method utilizing eye and mouth movements to conduct liveness detection. Despite these ways tend to have high accuracy, it is still necessary to highly interact with users. On the other hand, the time cost of this detection is relatively long and not friendly to users. In recent years, there has appeared simulated dynamic faces driven by the reinforcement learning model [26], which presents challenges to FASD research. It is also found that the CNNs have great potential for learning faced with various spoofing ways and improved the robustness of anti-spoofing algorithms. Atoum et al. [22] proposed a two-stream CNNs-based approach for face anti-spoofing. Liu et al. [23] assumed liveness detection as a binary classification problem to be directly learned by Deep Neural Networks (DNNs). Despite CNNs [27,28] having potential for face liveness detection, it has to face the problem of overfitting and needs further studies.
Based on the above discussion, it is not difficult to find that the liveness detection research has competed with a variety of spoofing approaches all the time. In this paper, we focus on these issues and present an intelligent access control framework with two improvements:
1) A novel authentication system fusing the mask detection algorithm and face authentication algorithm with face anti-spoofing algorithm is proposed. The mask detection algorithm employs the mask detector called RetinaFaceMask, and uses MobileNetV3 as the feature extractor; the face authentication algorithm makes use of Tripletloss for training the neural network model;
2) In order to realize the anti-spoofing function, this paper makes use of a lightweight neural network and uniform Local Binary Patterns (LBP) method to extract the color texture of the image frame. The accuracy and training efficiency of the above model is tested on the CASIA-FASD dataset and Replay-Attack dataset.
This paper is organized as follows. Sections Ⅱ and Ⅲ discuss the design of the network framework in detail, the selection of benchmark datasets, and experiment setups respectively. Then the analysis and discussion of experimental results are presented in Section Ⅳ.
The proposed framework of the intelligent access control framework is composed of three parts: Mask detection module, face detection and recognition module and face anti-spoofing module (as shown in Figure 1). During the operation process, the passenger is sampled using videos and flagged the mask-wearing status by the mask detection module. Then it makes use of a multi-task convolutional neural network (MTCNN) [29] to process captured video frames and align them within the user's face. Through MTCNN the detected face is centrally located in alignment pictures with a size of 160*160 pixel). The alignment pictures are then filled into the face anti-spoofing module in which the liveness detection algorithm can judge its authenticity. If one face is judged as real, it will enter the face recognition module for identity matching. In the face recognition module, if the real face matches one in the database, this face and its identity will be flagged by this system. Otherwise, the face recognition will end and present an alarm message.
This paper designs mask and face detection module, which includes mask detection unit and face detection unit. The mask detection unit is designed based on a kind of face-mask detector called RetinaFaceMask (as shown in Figure 2). In order to apply to embedded applications, the feature extractor is replaced with MobileNetV3 which is featured with its low parameter quantity and lightweight architecture. On the other hand, the high-level semantic information is extracted by FPN from the feature maps of different sizes while fusing this information into previous layers' feature maps by adding operation with a coefficient. In Figure 2, the head part of mask detection architecture represents classifiers, predictors, estimators which complete inference function and indicate the mask-wearing status. The test image input into networks model in the testing, which outputs two results are face confidence and mask confidence by removing proposal which is less than the set threshold. The remaining results are filtered using Non-Maximum Suppression (NMS). The prediction results of faces and masks are retained, whose weighted logistic loss is defined as (1). In the Eq (1), di denoted a detection, yi∈{−1,1} indicate whether or not di was successfully matched to an object, and let f denote the scoring function (RetinaFaceMask) that jointly scores all detections on an image f([di]ni=1)=[si]ni=1. For a variety potential detection results, here loss per detections is coupled to the other detections through the marching produces yi. By this way, the prediction results of faces and masks are retained.
L(si,yi)=∑Ni=1ωyi⋅log(1+exp(−si⋅yi)). | (1) |
Due to the limited data set of face masks, it is difficult for the network to be trained through the training set to get good results so that we train a more robust network model through transfer learning. In RetinaFaceMask, only the parameters of the backbone and neck are transferred from Wider Face, a dataset consisting of 32,203 images and 393,703 annotated faces. RetinaFaceMask proposed a new contextual attention module as Heads in order to improve the prediction performance of face masks. The context attention module has three branches, each branch has a 3×3, two 3×3, and three 3×3 convolution kernels, and using different numbers of 3×3 convolution kernels to obtain different receptive fields from the same feature map. The original context module does not consider the face or mask, so it needs to feature an abstraction component put it into retraining. Here the abstraction component is designed to be one simplified convolutional block attention module (CBAM). Given an intermediate feature map from the original RetinaFaceMask architecture, the CBAM can sequentially infer a 1D channel attention map and a 2D spatial attention map. Although this structure is suitable to save the memory cost, the spatial attention module in the CBAM still can occupy a variety of computation costs [30,31]. Therefore, in this paper, we replace the attention module of CBAM with on Maxpool-Convolution-AveragePool component. This simplification is equivalent to cascading the shared spatial perception of the feature abstraction. After it, the RetinaFaceMask is prompted to pay attention to the face and mask features.
Another module of the mask and face detection module is the face detection unit, which uses the MTCNN model. This model is built as a three-layer neural network, which is P-Net, R-Net, O-Net. In the operation, the obtained images are processed by these three networks to detect faces and face landmarks. When input images come, they will be resized continuously to produce the image pyramid. The pyramid is filled into P-Net to obtain lots of candidates that are filtered by R-Net more precisely afterward. Finally, the filtered data is filled into O-Net to calculate the landmarks' position of potential faces. This model realizes face detection and alignment functions.
Boulkenafet et al. [14] propose that face anti-spoofing could be distinguished by analyzing color texture. The color is analyzed by utilizing uniform LBP in the luminance and chrominance channels. Uniform LBP extracts the histogram from a single image band and concatenates these histograms into the final descriptor which be calculated by Support Vector Machine (SVM) to distinguish real face and fake face.
LBP is a feature descriptor of local texture for gray-scale images proposed by Ojala et al. [32]. The traditional extraction approach of the LBP feature descriptor makes the original image be divided into blocks of n*n and each block is divided into cells of a*a. Then, the feature of each cell is extracted by LBP. Considering the center pixel value as a threshold to compare with 8 pixels from the yield of threshold around. The LBP descriptor is defined as Eq (2):
LBP(xc,yc)=∑7p=02ps(ip−ic). | (2) |
(xc, yc) is the center point in the 3*3 area, which pixel value is ic. ip represents the value of other pixels in the area. p is the number of pixel points. s(x) is a symbolic function which is defined as Eq (3):
s(x)={1,x≥00,x<0. | (3) |
s(x)=1, if x≥0, otherwise s(x)=0. The values of each pixel point be calculated by exploiting LBP descriptor. The result is connected to a binary value and converted to a decimal value which is the value of the LBP descriptor. Generating situations are 256 by this approach, but lots of situations are very complex so it is not good for the extraction of facial features. Thereby, Ojala, et al. [33] proposed Uniform LBP to optimize the traditional LBP descriptor for implementing dimensionality reduction through the transition approach. The uniform LBP descriptor is defined as Eq (4):
LBP(i)P,R(x,y)={∑P−1n=0δ(r(i)n−r(i)c)×2n,U(i)≤2P(P−1),U(i)>2. | (4) |
(x,y) is the center point of the neighborhood, rn(n=0,⋯,P−1) is the pixel value of surrounding neighborhood, rc is the pixel value of center point, where R is the radius of the surrounding neighborhood, P is the number of points in the surrounding neighborhood, where U(i) is defined as Eq (5):
U(i)=|δ(r(i)P−1−r(i)c)−δ(r(i)0−r(i)c)|+∑Pn=1|δ(r(i)n−r(i)c)−δ(r(i)n−1−r(i)c)| | (5) |
where, δ(x) is the symbolic function which is defined as Eq (6):
δ(x)={1,x≥00,x<0. | (6) |
δ(x)=1, if x≥0, otherwise δ(x)=0.
Uniform LBP extracts the histogram from a single image band, and then concatenates these histograms into the final descriptor which be calculated by SVM [34] to distinguish real face and fake face. The whole process is shown as Figure 3. The Figure 3 contains two options to complete liveness detection task. The option 1 is face anti-spoofing based on color texture analysis. It extracts the faces in the dataset and crops them to the unified format of 224 * 224 pixels. These unified face frames are converted into the color spaces of HSV and YCbCr. Then the LBP descriptor extracts the feature histogram from color spaces, and connects them in series. In the end, serialized feature histogram is input in SVM to classify as real or fake face.
Option 2 in Figure 3 completes face anti-spoofing based on a lightweight neural network. The advantages of the lightweight neural network [35,36,37,38,39] are high accuracy, smaller parameter, detection faster and can be well applied to intelligent access control systems. Face liveness detection is defined as a binary classification question. Using the face data set of size 224×224 to train a lightweight neural network, and change the linear activation function of the last layer of the network structure to the Tanh activation function. Because the weights of multi-type image classification and living detection two classifications are different, the two-classification model suitable for living detection is used for pre-training. In terms of hyperparameter selection, Epoch is 150, Batch Size is 64, the cross-entropy loss function is used, the optimizer selects random gradient descent, the weight attenuation is 4×10-5, and the Momentum is 0.9. According to the above conditions, the lightweight neural network is trained.
The function of face detection and verification is a hot spot in the field of security. In this paper, we propose a neural network called Face-net. This neural network encodes face images into digital vectors (or called face-net vectors). In order to calculate the confidence between the face image and preset identity, this paper uses Euclidean distance to compare two face-net vectors and determine whether these two vectors belong to the same person. As shown in Figure 4(b) represents distances between images. The distance between the same person's images is basically below one threshold which is demarcated a visa experiment.
As shown in the part (a) of Figure 4 In this paper, Face-net model is designed to realize the face verification and recognition, architecture of Face-net model is shown in Figure 4(a), batch refers to the input face image sample which is found and then is cropped to a fixed size of 160×160 pixel. Deep architecture refers to a deep learning architecture, the deep architecture of Face-net uses the GoogleNet network, which combines the traditional convolutional neural network with Inception structure. This configuration can get the balance between the size and over-fitting characteristics of the deep neural network. In practice, the main disadvantage of this method is the over-high dimensions of output features. So, optimization is conducted by replacing the last layer of the softmax classifier with one layer of L2 feature normalization. Through the normalization of the L2 layer, the revised module will output a 128-dimensional feature vector which is then optimized with triple loss. The triplet loss is one kind of loss function in Figure 4(c). The input of the triplet loss function is three groups of images. These three image groups are called Achor(A), Negative(N) and Positive(P) respectively. The A and P are captured from the same person with different imaging angles. The N is captured by other people. The similarity between image groups is quality by Euclidean distance. When training face-net, the Euclidean distance between A and P will gradually decrease, and the distance between A and N will gradually increase. Through this mechanism, the face-net learns the separability between features, and builds connections between every single image from a specific person. This is the basis for identity verification. The definition of triplet loss is shown as Eq (7):
||xAi−xPi||22+α<||xAi−xNi||22,∀(xAi,xPi,xNi)∈T. | (7) |
In the Eq (6), xAi (Anchor) represents the image of A, xPi (Positive) represents the different image of A, xNi (Negative) represents the image of B. α represents constant, and T represents the set of triplets in the training set. The loss function minimization L is defined as Eq (8).
L=∑Mi[||f(xAi)−f(xPi)||22−||f(xAi)−f(xNi)||22+α]+. | (8) |
In the Eq (7), f(x)∈Rd represents a face image x that is embedded in d-dimensional European space. Among them, the second norm on the left represents the intraclass distance, the second norm on the right represents the distance between classes, and α is a constant. The "+" at bottom right represents that when the value in the brackets is greater than zero, the loss is taken, and when it is less than zero, the loss is zero. M is the base. The optimization process is to use the gradient descent method to make the loss function continuously decrease which means the distance within the class continuously decreasing, and the distance between classes continuously increased.
To draw a conclusion, aiming at the possibility of infection and negligence in human supervision in public places, at the meantime, in order to meet the needs of embedded device platform, this paper proposes an intelligent access control framework composed of the mask detection algorithm, MTCNN algorithm, face anti-spoofing based on color texture analysis and Face-net identity verification. This framework can meet the requirements of embedded device applications and effectively solve the security problem of the public place access control system under the background of the spreading coronavirus.
In this section, we present the experimental evaluation of the proposed scheme for the intelligent access control system. The two data sets used in our experimental evaluation are introduced in detail. Experiments are performed on different anti-spoofing datasets. Details are given in the subsequent sections.
In order to evaluate proposed system objectively, we have chosen widely accepted CASIA-FASD [40] and Replay Attack [41] Datasets for experiments, both of which have well-described evaluation protocols. The CASIA-FASD datasets consist of 600 videos including 50 objects, each of the objects has 12 videos including 3 videos of legal access and 9 videos of illegal access. There are 3 different types of videos in the datasets which is low, normal, high, respectively. Illegal access videos have 3 different types of attack methods including warped photo attacks, cut photo attacks, and video attacks. The CASIA-FASD datasets are shown as Figure 5. The Reply-Attack datasets include 1200 videos which have 50 objects. Each of the objects has 24 videos which include 4 videos of legal access and 20 videos of illegal access, each of the videos has about 10 seconds in length. All videos are classified to 3 different backgrounds and 2 different illuminations. Illegal access videos have 3 different types of attack methods including print attack, digital images attack, videos attack. The datasets have 3 parts of datasets including train datasets, development datasets, text datasets.
In order to obtain a face recognition security system with a low calculation amount and a high recognition rate, the live detection is more user-friendly. The experiment verifies the performance of the algorithm by comparing the size of the model parameters, the recognition accuracy, the time required for the system to process a frame of pictures through the entire process, and the CPU occupancy.
This section focuses on the experiments to verify the anti-spoofing performance of the algorithm, using the CASIA-FASD and Replay-Attack datasets to conduct experiments. The datasets are shown in Table 1.
Dataset | Training Set | Testing Set | Total |
CASIA-FASD | 7200 | 10800 | 18000 |
Replay-Attack | 10800 | 14400 | 25200 |
a) The videos contained in the CASIA-FASD and Replay-Attack datasets are read and saved by OpenCV for 30 frames. After each frame is obtained, the image will be aligned and cropped by MTCNN and normalized to a 224×224 RGB picture.
b) This chapter uses datasets for training and testing separately. In the quantitative analysis, the experiment uses accuracy rate, EER, HTER, and receiver operating characteristic (ROC) curve as the evaluation indicators for evaluating the algorithm. When quantitatively analyzing the real-time performance of the face security system, we verify the performance of the algorithm through the time required for the system to process a frame of pictures and CPU occupancy rate.
c) All experiments have been conducted on a computer with PyCharm® installed in Windows 10. The processor model is Intel Core i5-8250U@ 1.60 GHz, and the memory is 8 GB RAM. The algorithm in this chapter is based on PyTorch 1.5 and TensorFlow 1.12 deep-learning framework, using Python 3.7 language implementation.
We did experiments on CASIA and Replay-Attack datasets to compare with the EER, HTER, ROC curves of different algorithms, the time required for the system to process a frame of pictures, and the experimental results of the CPU occupancy rate. The advantages and disadvantages of the algorithm are analyzed.
The CASIA dataset has rich attack methods and complex datasets. The Replay-Attack dataset has a total of 25,200 pictures, the attack method is video attack, the control variable is light, and the data set attack method is relatively simple. From Figures 6 and 7, we can see that under the Replay-Attack dataset with a single attack method, the algorithm can perform better classification, while under the complex CASIA data set, the LBP algorithm can still maintain good classification performance.
The lightweight neural network is limited in the size of the dataset. On the one hand, it is subject to fewer model parameters. Even if it is pre-trained under the live detection model, the network convergence process is still slow; on the other hand, under the multi-image classification It can achieve good accuracy, but live detection pays more attention to the image texture, spectral information, motion information, depth information and other nuances between the real face and the fraudulent face. This is a lightweight neural network for live detection under higher requirements.
It can be seen from Figures 6 and 7, and Table 2 that the size of the model parameters also affects the accuracy of live detection to a certain extent. Figures 8 and 9 verify that the LBP algorithm takes the least time when using SVM for facial feature classification, and the CPU usage is low. Because SVM has a better classification effect for small sample data and has a faster calculation speed.
Algorithm | CASIA | Replay-Attack | Parameter | ||
HTER (%) | EER (%) | HTER (%) | EER (%) | ||
MobileNetV2 | 16.7 | 9.4 | 12.6 | 3.6 | 3.4MB |
MobileNetV3 | 19.3 | 14.4 | 10.9 | 6.5 | 3.87MB |
ShuffleNetV2 | 21.9 | 14.9 | 21.8 | 6.33 | 1.4MB |
SqueezeNet | 28.3 | 19.1 | 24.7 | 13.7 | 736.45KB |
Xception | 20.5 | 9.4 | 20.5 | 4.2 | 20.81MB |
LBP | 9.7 | 5.5 | 10.8 | 5.6 | - |
The overall results of the experiments show that the system fused with the LBP algorithm and the FaceNet algorithm can effectively identify the attacks of forged faces on the face recognition security system while the real-time detection efficiency is high, and the lightweight neural network exists in the real-time live detection feasibility.
In this paper, we have proposed a novel security classification framework based on face recognition which will possibly contribute to ACS in public condition. This framework is composed of a mask detection algorithm and face authentication algorithm with an anti-spoofing function. According to the epidemic prevention problem, the mask detection algorithm is integrated with a dexterous structure which contains a novel context attention head module to focus on the face and mask features, and a series of lightweight units to remove object cross-class. This configuration enables the mask detection module to meet the operation requirement of embedded devices. Besides, we proposed one novel liveness detection method that analyzes the color and texture of image frames using the uniform LBP method. In order to test the performance of the framework, this paper employs CASIA-FASD and Reply-Attack datasets as a benchmark. The test indicates that the HTER is 9.7%, the EER is 5.5%. The average process time of a single frame is 0.12 seconds. The above results demonstrate that this framework has a high anti-spoofing capability and can be employed on the embedded system to complete the mask detection and face authentication task in real-time, which can effectively support the public entry task under an epidemic background.
This work is supported by the National Key Research and Development Program of China (No. 2020YFB1313600).
All authors declare no conflicts of interest in this paper.
[1] |
L. O. Chua, L. Yang, Cellular neural networks: Applications, IEEE Trans. Circuits Syst., 35 (1998), 1273–1290. https://doi.org/10.1109/31.7601 doi: 10.1109/31.7601
![]() |
[2] |
L. O. Chua, Memristor-the missing circuit element, IEEE Trans. Circuit Theory, 18 (1971), 507–519. https://doi.org/10.1109/tct.1971.1083337 doi: 10.1109/tct.1971.1083337
![]() |
[3] | D. B. Strukov, G. S. Snider, D. R. Stewart, R. S. Williams, The missing memristor found, Nature, 453 (2008), 80. https://doi.org/10.1038/nature08166 |
[4] |
Y. Zhang, X. Wang, E. G. Friedman, Memristor-based circuit design for multilayer neural networks, IEEE Trans. Circuits Syst. I-Regular Papers, 65 (2018), 677–686. https://doi.org/10.1109/TCSI.2017.2729787 doi: 10.1109/TCSI.2017.2729787
![]() |
[5] |
S. Duan, X. Hu, Z. Dong, L. Wang, P. Mazumder, Memristor-based cellular nonlinear/neural network: Design, analysis, and applications, IEEE Trans. Neural Netw. Learn. Syst., 26 (2015), 1202–1213. https://doi.org/10.1109/TNNLS.2014.2334701 doi: 10.1109/TNNLS.2014.2334701
![]() |
[6] |
W. J. Lin, Y. He, C. K. Zhang, L. Wang, M. Wu, Event-triggered fault detection filter design for discrete-time memristive neural networks with time delays, IEEE Trans. Cybern., 52 (2022), 3359–3369. https://doi.org/10.1109/TCYB.2020.3011527 doi: 10.1109/TCYB.2020.3011527
![]() |
[7] | L. Wang, C. K. Zhang, Exponential synchronization of memristor-based competitive neural networks with reaction-diffusions and infinite distributed delays, IEEE Trans. Neural Netw. Learn. Syst., 2022, 1–14. https://doi.org/10.1109/TNNLS.2022.3176887 |
[8] |
L. Wang, H. B. He, Z. Zeng, Global synchronization of fuzzy memristive neural networks with discrete and distributed delays, IEEE Trans. Fuzzy Syst., 28 (2020), 2022–2034. https://doi.org/10.1109/TFUZZ.2019.2930032 doi: 10.1109/TFUZZ.2019.2930032
![]() |
[9] | X. Hu, L. Wang, C. K. Zhang, X. Wan, Y. He, Fixed-time stabilization of discontinuous spatiotemporal neural networks with time-varying coefficients via aperiodically switching control, Sci. China Inf. Sci., 66, (2023), 152204. https://doi.org/10.1007/s11432-022-3633-9 |
[10] | J. Hu, G. Tan, L. Liu, A new result on H∞ state estimation for delayed neural networks based on an extended reciprocally convex inequality, IEEE Trans. Circuits Syst. II-Express Briefs, 2023. https://doi.org/10.1109/TCSII.2023.3323834 |
[11] |
W. J. Lin, Q. L. Han, X. M. Zhang, J. Yu, Reachable set synthesis of markov jump systems with time-varying delays and mismatched modes, IEEE Trans. Circuits Syst. II-Express Briefs, 69 (2022), 2186–2190. https://doi.org/10.1109/TCSII.2021.3126262 doi: 10.1109/TCSII.2021.3126262
![]() |
[12] |
Y. He, C. K. Zhang, H. B. Zeng, M. Wu, Additional functions of variable-augmented-based free-weighting matrices and application to systems with time-varying delay, Int. J. Syst. Sci., 54 (2023), 991–1003. https://doi.org/10.1080/00207721.2022.2157198 doi: 10.1080/00207721.2022.2157198
![]() |
[13] |
C. K. Zhang, W. Chen, C. Zhu, Y. He, M. Wu, Stability analysis of discrete-time systems with time-varying delay via a delay-dependent matrix-separation-based inequality, Automatica, 156 (2023), 111192. https://doi.org/10.1016/j.automatica.2023.111192 doi: 10.1016/j.automatica.2023.111192
![]() |
[14] |
C. Qin, W. J. Lin, Adaptive event-triggered fault-tolerant control for Markov jump nonlinear systems with time-varying delays and multiple faults, Commun. Nonlinear. Sci. Numer. Simul., 128 (2024), 107655. https://doi.org/10.1016/j.cnsns.2023.107655 doi: 10.1016/j.cnsns.2023.107655
![]() |
[15] | C. Qin, W. J. Lin, J. Yu, Adaptive event-triggered fault detection for Markov jump nonlinear systems with time delays and uncertain parameters, Int. J. Robust Nonlinear Control, 2023. https://doi.org/10.1002/rnc.7062 |
[16] |
C. K. Zhang, K. Xie, Y. He, J. She, M. Wu, Matrix-injection-based transformation method for discrete-time systems with time-varying delay, Sci. China Inf. Sci., 66 (2023), 159201. https://doi.org/10.1007/s11432-020-3221-6 doi: 10.1007/s11432-020-3221-6
![]() |
[17] |
Y. Zhao, H. Gao, S. Mou, Asymptotic stability analysis of neural networks with successive time delay components, Neurocomputing, 71 (2008), 2848–2856. https://doi.org/10.1016/j.neucom.2007.08.015 doi: 10.1016/j.neucom.2007.08.015
![]() |
[18] |
Q. Fu, J. Cai, S. Zhong, Y. Yu, Y. Shan, Input-to-state stability of discrete-time memristive neural networks with two delay components, Neurocomputing, 329 (2019), 1–11. https://doi.org/10.1016/j.neucom.2018.10.017 doi: 10.1016/j.neucom.2018.10.017
![]() |
[19] |
Y. Sheng, T. Huang, Z. Zeng, Exponential stabilization of fuzzy memristive neural networks with multiple time delays via intermittent control, IEEE Trans. Syst. Man Cybern. Syst., 52 (2022), 3092–3101. https://doi.org/10.1109/TSMC.2021.3062381 doi: 10.1109/TSMC.2021.3062381
![]() |
[20] |
R. Wei, J. Cao, W. Qian, C. Xue, X. Ding, Finite-time and fixed-time stabilization of inertial memristive Cohen-Grossberg neural networks via non-reduced order method, AIMS Math., 6 (2021), 6915–6932. https://doi.org/10.3934/math.2021405 doi: 10.3934/math.2021405
![]() |
[21] |
W. Zhang, H. Zhang, J. Cao, F. E. Alsaadi, D. Chen, Synchronization in uncertain fractional-order memristive complex-valued neural networks with multiple time delays, Neural Netw., 110 (2019), 186–198. https://doi.org/10.1016/j.neunet.2018.12.004 doi: 10.1016/j.neunet.2018.12.004
![]() |
[22] |
Q. Chang, J. H. Park, Y. Yang, The Optimization of control parameters: finite-time bipartite synchronization of memristive neural networks with multiple time delays via saturation function, IEEE Trans. Neural Netw. Learn. Syst., 34 (2023), 7861–7872. https://doi.org/10.1109/TNNLS.2022.3146832 doi: 10.1109/TNNLS.2022.3146832
![]() |
[23] |
Y. Qian, L. Duan, H. Wei, New results on finite-/fixed-time synchronization of delayed memristive neural networks with diffusion effects, AIMS Math., 7 (2022), 16962–16974. https://doi.org/10.3934/math.2022931 doi: 10.3934/math.2022931
![]() |
[24] |
R. Rakkiyappan, A. Chandrasekar, J. Cao, Passivity and passification of memristor-based recurrent neural networks with additive time-varying delays, IEEE Trans. Neural Netw. Learn. Syst., 26 (2015), 2043–2057. https://doi.org/10.1109/TNNLS.2014.2365059 doi: 10.1109/TNNLS.2014.2365059
![]() |
[25] |
J. C. Willems, Dissipative dynamical systems part I: General theory, Arch. Ration. Mech. Anal., 45 (2015), 321–351. https://doi.org/10.1007/bf00276493 doi: 10.1007/bf00276493
![]() |
[26] |
B. Zhang, W. Zheng, S. Xu, Filtering of Markovian jump delay systems based on a new performance index, IEEE Trans. Circuits Syst. I-Regular Papers, 60 (2013), 1250–1263. https://doi.org/10.1109/TCSI.2013.2246213 doi: 10.1109/TCSI.2013.2246213
![]() |
[27] | C. Lu, X. M. Zhang, Y. He, Extended dissipativity analysis of delayed memristive neural networks based on a parameter-dependent lyapunov functional, In: 2018 Australian & New Zealand Control Conference, 2018,194–198. https://doi.org/10.1109/ANZCC.2018.8606585 |
[28] |
H. Wei, R. Li, C. Chen, Z. Tu, Extended dissipative analysis for memristive neural networks with two additive time-varying delay components, Neurocomputing, 216 (2016), 321–351. https://doi.org/10.1002/rnc.5118 doi: 10.1002/rnc.5118
![]() |
[29] |
T. H. Lee, M. J. Park, J. H. Park, O. M. Kwon, S. M. Lee, Extended dissipative analysis for neural networks with time-varying delays, IEEE Trans. Neural Netw. Learn. Syst., 25 (2014), 1936–1941. https://doi.org/10.1109/TNNLS.2013.2296514 doi: 10.1109/TNNLS.2013.2296514
![]() |
[30] |
P. Park, W. I. Lee, S. Y. Lee, Auxiliary function-based integral inequalities for quadratic functions and their applications to time-delay systems, J. Franklin Inst. Eng. Appl. Math., 352 (2015), 1378–1396. https://doi.org/10.1016/j.jfranklin.2015.01.004 doi: 10.1016/j.jfranklin.2015.01.004
![]() |
[31] |
A. Seuret, F. Gouaisbaut, Wirtinger-based integral inequality: application to time-delay systems, Automatica, 49 (2013), 2860–2866. https://doi.org/10.1016/j.automatica.2013.05.030 doi: 10.1016/j.automatica.2013.05.030
![]() |
[32] |
A. Seuret, F. Gouaisbaut, Allowable delay sets for the stability analysis of linear time-varying delay systems using a delay-dependent reciprocally convex lemma, IFAC Papersonline, 50 (2017), 1275–1280. https://doi.org/10.1016/j.ifacol.2017.08.131 doi: 10.1016/j.ifacol.2017.08.131
![]() |
[33] |
Z. Zhai, H. Yan, S. Chen, H. Zeng, M. Wang, Improved stability analysis results of generalized neural networks with time-varying delays, IEEE Trans. Neural Netw. Learn. Syst., 34 (2023), 9404–9411. https://doi.org/10.1109/TNNLS.2022.3159625 doi: 10.1109/TNNLS.2022.3159625
![]() |
1. | Zhenzhen Wang, Hao Dong, Zhehao Huang, Carbon spot prices in equilibrium frameworks associated with climate change, 2023, 19, 1547-5816, 961, 10.3934/jimo.2021214 | |
2. | Shuaishuai Jia, Cunyi Yang, Mengxin Wang, Pierre Failler, Heterogeneous Impact of Land-Use on Climate Change: Study From a Spatial Perspective, 2022, 10, 2296-665X, 10.3389/fenvs.2022.840603 | |
3. | Meng Yang, Hujun Li, Fangzhao Deng, Qinchen Yang, Ning Ba, Yunxia Guo, Haitao Wu, Muhammad Irfan, Yu Hao, Inclusivity between internet development and energy conservation in Henan, China, 2023, 16, 1570-646X, 10.1007/s12053-023-10144-2 | |
4. | Michele Azzone, Roberto Baviera, Is (Independent) Subordination Relevant in Equity Derivatives?, 2024, 1524-1904, 10.1002/asmb.2904 | |
5. | Xin Yun, Yang Hu, An Overview of the Evolution in the Research Landscape of Green Finance, 2024, 5, 2673-4060, 1335, 10.3390/world5040068 | |
6. | Maria Carannante, Stefania Corsaro, Vincenzo Di Sauro, Zelda Marino, Salvatore Scognamiglio, A spatially-continuous neural network temperature model for weather derivatives evaluation, 2025, 0254-5330, 10.1007/s10479-025-06624-6 |
Dataset | Training Set | Testing Set | Total |
CASIA-FASD | 7200 | 10800 | 18000 |
Replay-Attack | 10800 | 14400 | 25200 |
Algorithm | CASIA | Replay-Attack | Parameter | ||
HTER (%) | EER (%) | HTER (%) | EER (%) | ||
MobileNetV2 | 16.7 | 9.4 | 12.6 | 3.6 | 3.4MB |
MobileNetV3 | 19.3 | 14.4 | 10.9 | 6.5 | 3.87MB |
ShuffleNetV2 | 21.9 | 14.9 | 21.8 | 6.33 | 1.4MB |
SqueezeNet | 28.3 | 19.1 | 24.7 | 13.7 | 736.45KB |
Xception | 20.5 | 9.4 | 20.5 | 4.2 | 20.81MB |
LBP | 9.7 | 5.5 | 10.8 | 5.6 | - |
Dataset | Training Set | Testing Set | Total |
CASIA-FASD | 7200 | 10800 | 18000 |
Replay-Attack | 10800 | 14400 | 25200 |
Algorithm | CASIA | Replay-Attack | Parameter | ||
HTER (%) | EER (%) | HTER (%) | EER (%) | ||
MobileNetV2 | 16.7 | 9.4 | 12.6 | 3.6 | 3.4MB |
MobileNetV3 | 19.3 | 14.4 | 10.9 | 6.5 | 3.87MB |
ShuffleNetV2 | 21.9 | 14.9 | 21.8 | 6.33 | 1.4MB |
SqueezeNet | 28.3 | 19.1 | 24.7 | 13.7 | 736.45KB |
Xception | 20.5 | 9.4 | 20.5 | 4.2 | 20.81MB |
LBP | 9.7 | 5.5 | 10.8 | 5.6 | - |