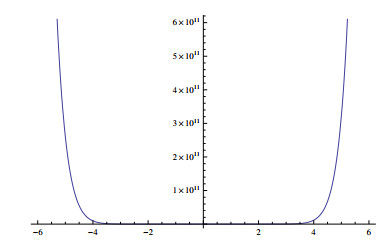
The South African financial market is developing with periods of high and low volatility. Employing an adequate volatility model is essential to manage market risk. This research study was designed to investigate the effectiveness of the fractionally integrated asymmetric power autoregressive conditional heteroskedasticity contrasted with long-memory GARCH-type models, such as the fractionally integrated generalized autoregressive conditional heteroskedasticity and the hyperbolic generalized autoregressive conditional heteroskedasticity for producing the measure of market risk known as the value at risk. These long-memory GARCH-type models assume that the distributions of the index returns follow normal, student-t, skewed student-t and generalized error distributions. The historical closing price time series of the Johannesburg Stock Exchange all share, the mining and the banking indices are considered. The value at risk and its backtesting for short and long trading positions on the different confident levels are computed and they correspond to the right and left quantiles of the return distributions, respectively. The results reveal that FIAPARCH with a standard student-t distribution is an appropriate model for producing a robust value at risk in the context of mining and banking indices. Alternatively, FIGARCH with the assumed skewed student-t distribution model is a good fit to produce a value at risk for the Johannesburg Stock Exchange All Share Index.
Citation: Moses Khumalo, Hopolang Mashele, Modisane Seitshiro. Quantification of the stock market value at risk by using FIAPARCH, HYGARCH and FIGARCH models[J]. Data Science in Finance and Economics, 2023, 3(4): 380-400. doi: 10.3934/DSFE.2023022
[1] | Irina Di Ruocco . A political concept for the Gragnano Valley of Mills (Valle dei Mulini). Urban redevelopment of cultural-industrial heritage. Urban Resilience and Sustainability, 2023, 1(4): 278-308. doi: 10.3934/urs.2023018 |
[2] | Aibin Yan, Dinghan Zheng . Restoration and integration of the Huang Family Garden within the contemporary urban fabric of Shanghai. Urban Resilience and Sustainability, 2024, 2(1): 27-44. doi: 10.3934/urs.2024003 |
[3] | Elham Zabetian Targhi, Niusha Fardnava, Saba Saghafi . Urban development criteria with a focus on resilience to pandemics: A case study of coronavirus (Covid-19). Urban Resilience and Sustainability, 2023, 1(1): 66-85. doi: 10.3934/urs.2023005 |
[4] | Jichao Wang, Xiaoning Sui, Jie Zhang, Wenjie Shi, Wayne L. Thompson . Relocating disaster-prone villages and improving villager well-being: Evidence from Beijing, China. Urban Resilience and Sustainability, 2024, 2(3): 236-255. doi: 10.3934/urs.2024012 |
[5] | João C. G. Lanzinha . Rehabilitation of existing building parks and its relationship with urban agglomerates–An urgent and demanding task for our common future. Urban Resilience and Sustainability, 2023, 1(2): 86-90. doi: 10.3934/urs.2023006 |
[6] | Adoyo Laji, Jeremiah N. Ayonga . Mainstreaming resilience to flood risk among households in informal settlements in Kisumu City, Kenya. Urban Resilience and Sustainability, 2024, 2(4): 326-347. doi: 10.3934/urs.2024017 |
[7] | Maria Helena Luengo-Duque . Erasing roots: The impact of urban development on historical memory and identity in San Juan. Urban Resilience and Sustainability, 2025, 3(1): 26-56. doi: 10.3934/urs.2025002 |
[8] | Erik Velasco . Circular economy in Singapore: waste management, food and agriculture, energy, and transportation. Urban Resilience and Sustainability, 2024, 2(2): 110-150. doi: 10.3934/urs.2024007 |
[9] | Cheikh Cisse . At the crossroads of datas: Using artificial intelligence for efficient and resilient planning in African cities. Urban Resilience and Sustainability, 2023, 1(4): 309-313. doi: 10.3934/urs.2023019 |
[10] | Belhaj Naoufel . Determinants of cities' financial governance for sustainable urban development in Morocco—Case of the regions of Casablanca-Settat and Rabat-Sale-Kenitra. Urban Resilience and Sustainability, 2023, 1(1): 1-19. doi: 10.3934/urs.2023001 |
The South African financial market is developing with periods of high and low volatility. Employing an adequate volatility model is essential to manage market risk. This research study was designed to investigate the effectiveness of the fractionally integrated asymmetric power autoregressive conditional heteroskedasticity contrasted with long-memory GARCH-type models, such as the fractionally integrated generalized autoregressive conditional heteroskedasticity and the hyperbolic generalized autoregressive conditional heteroskedasticity for producing the measure of market risk known as the value at risk. These long-memory GARCH-type models assume that the distributions of the index returns follow normal, student-t, skewed student-t and generalized error distributions. The historical closing price time series of the Johannesburg Stock Exchange all share, the mining and the banking indices are considered. The value at risk and its backtesting for short and long trading positions on the different confident levels are computed and they correspond to the right and left quantiles of the return distributions, respectively. The results reveal that FIAPARCH with a standard student-t distribution is an appropriate model for producing a robust value at risk in the context of mining and banking indices. Alternatively, FIGARCH with the assumed skewed student-t distribution model is a good fit to produce a value at risk for the Johannesburg Stock Exchange All Share Index.
The special polynomials and numbers play an important role in many branches of mathematics such as combinatorics, computer science, statistics, etc. The generating functions of the special polynomials are used in the investigation of numerous properties satisfied by polynomials. A large number of indispensable special polynomials such as the Bernoulli, Euler and monic Hermite polynomials belong to the Appell class of sequences. These polynomials play crucial roles in many aspects, most importantly, they provide solutions to certain specified problems of physics, engineering and mathematics (see for more details [5,6,7,8,9,21,22,34,35]).
Throughout this paper, we use the following standard notations: N:={1,2,3,⋯},N0:=N∪{0}:={0,1,2,3,⋯},Z−:={−1,−2,−3,⋯},Z denotes the set of integers and R denotes the set of real numbers.
The generating function of the Euler polynomials Eq(v) [23], which arise in various classical and numerical analysis (and also of analytic number theory) is defined as:
2ew+1evw=∞∑q=0Eq(v)wqq!,(|w|<π). | (1.1) |
The corresponding Euler numbers (appear in the Taylor series expansions of the secant and the hyperbolic secant functions) Eq:=Eq(0) are given as:
2ew+1=∞∑q=0Eqwqq!,(|w|<π). | (1.2) |
Recently, Kim et al., [31] introduced the Changhee polynomials and numbers and established certain explicit formulae and identities for these polynomials. The Changhee polynomials Chq(v) are specified by the following generating function [31]:
22+w(1+w)v=∞∑q=0Chq(v)wqq!, | (1.3) |
which for v=0 gives the corresponding Changhee numbers Chq:=Chq(0). Thereafter, Lee et al., [32] introduced a new form of Changhee polynomials, called the Appell-type Changhee polynomials Ch∗q(v), and defined by the following generating equation [32]:
22+wevw=∞∑q=0Ch∗q(v)wqq!, | (1.4) |
and have the following explicit relation [32,P3,Theorem 1]:
Ch∗q(v)=q∑p=0(qp)Ch∗qvq−p, | (1.5) |
where Ch∗q:=Ch∗q(0) are the corresponding Appell-type Changhee numbers:
22+w=∞∑q=0Ch∗qwqq!. | (1.6) |
The above Appell-type Changhee numbers Ch∗q satisfy the following relation [32,P4,Theorem 4]:
2Ch∗q+qCh∗q−1=0,in the case whenq≥1, | (1.7) |
with initial condition Ch∗0=1.
Motivated by the work of Kim et al., [24,26,27,28,29,30,31] and Lee et al., [31,32], in this paper we introduce the generating function of the Appell-type Changhee-Euler polynomials (ATCEP) and derive its properties.
In this section, the Appell-type Changhee-Euler polynomials (ATCEP), denoted by ChE∗q(v) are introduced and certain properties of these polynomials are established.
Definition 1. The Appell-type Changhee-Euler polynomials ChE∗q(v) are defined by the following generating relation:
4(ew+1)(2+w)evw=∞∑q=0ChE∗q(v)wqq!. | (2.1) |
Utilizing Eqs (1.1) and (1.6) in the left hand side of generating function (2.1) and then using Cauchy-product rule in the left hand side of the obtained equation, we get
∞∑q=0q∑l=0(ql)El(v)Ch∗q−lwqq!=∞∑q=0ChE∗q(v)wqq!. |
Comparing the coefficients of identical powers of w in both sides of the foregoing equation, we get the following series expansion for Appell-type Changhee-Euler polynomials ChE∗q(v):
q∑l=0(ql)El(v)Ch∗q−l=ChE∗q(v). | (2.2) |
Motivated by the importance of the Euler numbers [36,38] in various branches of mathematics specially in number theory and combinatorics, we introduce the numbers related to the polynomial families introduced here. Taking v=0 in series definition (2.2) of the ATCEP ChE∗q(v) and using notation:
ChE∗q:=ChE∗q(0), | (2.3) |
in the left hand side, we get the following series form for Appell-type Changhee-Euler number (ATCEN) ChE∗q:
q∑l=0(ql)ElCh∗q−l=ChE∗q. | (2.4) |
Also, we find the following generating functions for ATCEN ChE∗q:
4(ew+1)(2+w)=∞∑q=0ChE∗qwqq!. | (2.5) |
Theorem 2.1. Let u,v∈C and q∈N0, then the following addition formulas hold:
ChE∗q(u+v)=q∑p=0(qp)Ep(u)Ch∗q−p(v). | (2.6) |
ChE∗q(u+v)=q∑p=0(qp)uq−pChE∗p(v). | (2.7) |
Proof. Replacing v by u+v in Eq (2.1), we get
(2ew+1)(22+w)e(v+u)w=∞∑q=0ChE∗q(v+u)wqq!, | (2.8) |
which on using Eqs (1.4) and (1.1) in the l.h.s. and then on comparing the coefficients of the identical powers of w in both sides of the obtained relation, we get assertion (2.6).
Equation (2.8) can be written as
∞∑q=0ChE∗q(v+u)wqq!=4(ew+1)(2+w)evweuw, | (2.9) |
which on using Eq (2.1) and expanding the second exponential of the right side gives
∞∑q=0ChE∗q(v+u)wqq!=∞∑q=0(uw)qq!∞∑p=0ChE∗p(v)wpp!. | (2.10) |
Using the Cauchy product rule on the right side of Eq (2.10) and on comparing the coefficients of w, we are lead to assertion (2.7).
Theorem 2.2. Let q∈N0 and v∈C, then the following identities hold true:
2Eq(v)=2Ch∗Eq(v)+qChE∗q−1(v). | (2.11) |
2Ch∗q(v)=Ch∗Eq(v)+q∑p=o(qp)ChE∗q−p(v). | (2.12) |
ChE∗q(v)=q∑p=0(qp)(−1)pp!Eq−p(v)2p. | (2.13) |
ChE∗q(v)=q∑p=0(qp)ChE∗q−p(v)p. | (2.14) |
ChE∗q(v)=q∑p=0(qp)Ch∗q−p(v2)Ep(v2). | (2.15) |
ddvChE∗q(v)=qChE∗q−1(v). | (2.16) |
dpdvpChE∗q(v)=q!(q−p)!ChE∗q−p(v). | (2.17) |
∫vaChE∗q−1(u)du=1q(ChE∗q(v)−ChE∗q(a)). | (2.18) |
ChE∗q=q∑p=0(qp)(−v)pChE∗q−p(v). | (2.19) |
2Ch∗q=q∑p=0(qp)ChE∗q−p(v)(−v)p(1+(1−1v)p). | (2.20) |
Proof. These identities are easily derivable with the help of generating Eqs (1.1), (1.4) and (2.1) by manipulating double series. Now, rewriting the generating Eq (2.1) as
4(ew+1)(2+w)=∞∑q=0ChE∗q(v)wqq!(e−vw), | (2.21) |
which on using generating relation (2.5) in the l.h.s. and expanding the exponential on the r.h.s. and then on equating the coefficients of identical powers of w in both sides, we are led to assertion (2.19).
From Eq (2.21), we have
42+w=∞∑q=0ChE∗q(v)wqq!(e−vw(ew+1)), | (2.22) |
which on using generating relation (1.6) in l.h.s. and expanding the exponentials on the r.h.s. and then on equating the coefficients of identical powers of w in both sides of the obtained result, yields assertion (2.20).
Many researchers have established the determinant form of certain special and q-special polynomials [4,12,16,18,19,20,33]. Costabile [10,11,13] has given several approaches to Bernoulli polynomials. An important approach based on a determinant definition was given in [10]. This approach is further extended to provide determinant definitions of the Appell and Sheffer polynomial sequences by Costabile and Longo in [12] and [14] respectively. Motivated by the recent work on the determinant approaches, here we give the determinant representation of ATCEP ChE∗q(v). We recall the determinant form of the Euler polynomials Eq(v):
The Euler polynomials Eq(v) of degree q=0,1,2,⋯ are defined by
E0(v)=1,Eq(v)=(−1)q|1vv2⋯vq−1vq11212⋯12120112(21)⋯12(q−11)12(q1)001⋯12(q−12)12(q2)...⋯.....⋯..000⋯112(qq−1)|,q=1,2,.... | (2.23) |
Definition 2. The Appell-type Changhee-Euler polynomials ChE∗q(v) of degree q are defined by
ChE∗0(v)=1,ChE∗q(v)=(−1)q|1Ch∗1(v)Ch∗2(v)⋯Ch∗q−1(v)Ch∗q(v)11212⋯12120112(21)⋯12(q−11)12(q1)001⋯12(q−12)12(q2)...⋯.....⋯..000⋯112(qq−1)|,q=1,2,.... | (2.24) |
where the Appell-type Changhee polynomials Ch∗q(v)(q=0,1,2,...) are given by Eq (1.4).
In the forthcoming section, a family of linear differential equations arising from the generating function of ATCEN ChE∗q is derived by following the approach presented in [26].
Differential equations, besides playing important role in pure mathematics, constitute fundamental part of mathematical description of physical processes. Thus, obtaining the solutions for differential equations is of paramount importance. Few types of differential equations allow explicit and straightforward analytical solutions. Many researchers obtained the differential equations for special polynomials and numbers utilizing their generating functions [17,25,30].
Theorem 3.1. For n∈N the following family of differential equations for Appell-type Changhee-Euler polynomials ChE∗q(v) holds true:
n∑j=1βj(n)ewG(j−1)(w)+(ew+1)G(n)(w)=(−1)nβ0(n)(2+w)(n+1), | (3.1) |
where G(n)(w):=(ddw)nG(w),
G=G(w)=4(ew+1)(2+w), | (3.2) |
β0(n)=4n!,β1(n)=1,βn(n)=n, | (3.3) |
and
βi(n+1)=1+n−i+1∑j1=0βi−1(n−j1),(2≤i≤n). | (3.4) |
Proof. Let
G=G(w)=4(ew+1)(2+w), |
which can be rewritten as:
(ew+1)G(w)=42+w. | (3.5) |
Taking the derivative of Eq (3.5) with respect to w, it follows that:
(ew+1)G(1)(w)+ewG(w)=(−1)4(2+w)2, | (3.6) |
Again, by taking the derivative with respect to w of Eq (3.6), we find
(ew+1)G(2)(w)+2ewG(1)(w)+ewG(w)=(−1)28(2+w)3, | (3.7) |
and consequently
(ew+1)G(3)(w)+3ewG(2)(w)+3ewG(1)(w)+ewG(w)=(−1)324(2+w)4. | (3.8) |
Continuing this process, we find
n∑j=1βj(n)ewG(j−1)(w)+(ew+1)G(n)(w)=(−1)nβ0(n)(2+w)(n+1). | (3.9) |
Further, taking the derivative with respect to w of Eq (3.9), we get
β1(n)ewG(w)+n∑j=2ew(βj(n)+βj−1(n))G(j−1)(w)+(βn(n)+1)ewG(n)(w)+(ew+1)G(n+1)(w)=(−1)n+1(n+1)β0(n)(2+w)−(n+2). | (3.10) |
Replacing n by n+1, Eq (3.9) may be rewritten as:
n+1∑j=1βj(n+1)ewG(j−1)(w)+(ew+1)G(n+1)(w)=(−1)n+1β0(n+1)(2+w)(n+2). | (3.11) |
Comparison of the coefficients of G(i)'s on both sides of Eqs (3.10) and (3.11), yields the following recursive formulas:
β0(n+1)=(n+1)β0(n), | (3.12) |
β1(n+1)=β1(n), | (3.13) |
βj(n+1)=βj(n)+βj−1(n),(2≤j≤n) | (3.14) |
and
βn+1(n+1)=βn(n)+1. | (3.15) |
From Eqs (3.6) and (3.9), we have
β0(1)=4,β1(1)=1. | (3.16) |
Again, from Eq (3.12), we note that
β0(n+1)=(n+1)β0(n)=(n+1)nβ0(n)=...=(n+1)n(n−1)...2.1β0(1), |
that is
β0(n+1)=4(n+1)!. | (3.17) |
In view of Eq (3.13), we have
β1(n+1)=β1(n)=β1(n−1)=...=β1(1)=1. | (3.18) |
Further, from Eq (3.15), it follows that:
βn+1(n+1)=βn(n)+1=βn−1(n−1)+2=...=β1(1)+n, |
that is
βn+1(n+1)=1+n. | (3.19) |
Taking j=2 in Eq (3.14), we find
β2(n+1)=β2(n)+β1(n)=β2(n−1)+β1(n−1)+β1(n) |
=...=n−2∑j=0β1(n−j)+β2(2), |
that is
β2(n+1)=1+n−1∑j=0β1(n−j). | (3.20) |
Similarly, for j=3 Eq (3.14) gives
β3(n+1)=1+n−2∑j=0β2(n−j), | (3.21) |
consequently for j=4, we have
β4(n+1)=1+n−3∑j=0β3(n−j). | (3.22) |
Continuing this process, we deduce that
βi(n+1)=1+n−i+1∑j1=0βi−1(n−j1),(2≤j≤n). | (3.23) |
Equation (3.9) together with Eqs (3.17), (3.18) and (3.23) completes the proof of Theorem 3.1.
Theorem 3.2. For i,n∈N,p∈N0 the following identity for the Appell-type Changhee-Euler numbers ChE∗q holds true:
n∑j=1i∑p=0(ip)βj(n)ChE∗p+j−1+i∑p=0(ip)ChE∗p+n+ChE∗i+n=(−1)n+i(n+1)i(β0(n)2n+i+1), | (3.24) |
where (n+1)i is the pochhammer symbol [37,P21] and βj(n),(0≤j≤n) defined in Eqs (3.3) and (3.4).
Proof. Making use of Eq (2.5) in the first term of the left hand side of Eq (3.1), we find
n∑j=1βj(n)ewG(j−1)(w)=n∑j=1βj(n)∞∑l=0wll!∞∑p=0ChE∗p+j−1wpp!, |
or equivalently
n∑j=1βj(n)ewG(j−1)(w)=∞∑i=0(n∑j=1i∑p=0(ip)βj(n)ChE∗p+j−1)wii!. | (3.25) |
Again, using Eq (2.5) in the second term of the left hand side of Eq (3.1), we find
(ew+1)G(n)(w)=(∞∑p=0wpp!+1)((ddw)n∞∑l=0ChE∗lwll!), |
that is
(ew+1)G(n)(w)=∞∑i=0(i∑p=0(ip)ChE∗p+n+ChE∗i+n)wii!. | (3.26) |
Finally, from the right hand side of Eq (3.1), we have
β0(n)(−1)n(2+w)−(n+1)=β0(n)(−1)n2−(n+1)(1+w/2)−(n+1), |
which on using the following identity [37,P34]:
(1−z)−a=∞∑n=0(a)nznn!, |
gives
β0(n)(−1)n(2+w)−(n+1)=∞∑i=0((−1)n+iβ0(n)(n+1)i2n+i+1)wii!. | (3.27) |
Use of Eqs (3.25)–(3.27) in Eq (3.1), yields assertion (3.24).
In the next section, the graphical representations of the ATCEP ChE∗q(v) and distribution of their zeros are discussed.
Over the years, there has been increasing interest in solving mathematical problems with the aid of computers. The software Mathematica is used to show the behaviour of these newly introduced polynomials using the graphs plotted for the special values of indices. The manual computation of the zeros is too complicated, therefore, we use Mathematica to investigate the zeros of the ATCEP ChE∗q(v). By using numerical investigations and computer experiments, we find the real and complex zeros for certain values of index q. The investigation in this direction will lead to a new approach employing numerical methods in the field of the hybrid special polynomials to appear in mathematics and physics, see for example [18,19].
With the help of Mathematica and using Eqs (1.5) and (1.7) in relation (2.2), Figures 1 and 2 are drawn for odd values of index q:
Similarly, for even values of index q, Figures 3 and 4 are drawn:
Next, a remarkably regular structure of the zeros of ATCEP ChE∗q(v) are explored with the help of Mathematica in Figures 5–8.
Further properties, applications and theoretical investigation of scattering of zeros of the ATCEP ChE∗q(v) introduced here are left to the authors and the interested researchers, for future study.
Exclusive role have been played by special polynomials in applied mathematics. It is not astonishing when new classes of special polynomials are established as the issues associated with special polynomials are too immense. In this paper, by incorporating the Appell-type Changhee polynomials Ch∗q(v) and the Euler polynomials Eq(v) in a natural way, the so-called Appell-type Changhee-Euler polynomials ChE∗q(v) are introduced. Then, we investigate certain properties and identities for these new polynomials such as series representation, determinant form and some novel identities. We also present non-homogeneous linear differential equations for the Appell-type Changhee-Euler numbers ChE∗q. Further we discuss the shape and zero distributions of these polynomials by observing their graphs drawn by Mathematica. The ATCEP ChE∗q(v) were found to be the member of well-known Appell family and therefore many important properties and identities for this new polynomials were easily established by employing those in the well-developed theory of Appell polynomials. A unifying tool for studying polynomial sequences, namely the representation of Appell polynomials in matrix form has been studied in [1] and extended in [3] to the hypercomplex case. After that, Aceto and Caçāo [2] extends this approach to find the matrix representation of the Sheffer polynomials. The matrix which represents the Sheffer polynomial coefficients can be factorized into two matrices, one associated to Appell polynomials and the other linked to the binomial type polynomial sequences. This approach can be further extended to find the matrix representations of mixed special polynomials related to the Appell polynomials.
Posing a problem
If we consider the Genocchi polynomials [15] in place of Euler polynomials in Eq (2.1), we get the following generating function for the Appell-type Changhee-Genocchi polynomials (ATCGP) ChG∗q(v):
4w(ew+1)(2+w)evw=∞∑q=0ChG∗q(v)wqq!. | (5.1) |
We are believed to be able to establish the corresponding results for the Appell-type Changhee-Genocchi polynomials ChG∗q(v) as those established in this paper. This posed problem is left to the interested researchers and authors for further investigation.
The authors are thankful to the reviewers for several useful comments and suggestions towards the improvement of this paper.
No conflict of interest was declared by the authors.
[1] |
Abuzayed B, Al-Fayoumi N, Charfeddine L (2018) Long range dependence in an emerging stock market's sectors: volatility modelling and var forecasting. Appl Econ 50: 2569-2599. https://doi.org/10.1080/00036846.2017.1403559 doi: 10.1080/00036846.2017.1403559
![]() |
[2] |
Arouri MEH, Hammoudeh S, Lahiani A, et al. (2012) Long memory and structural breaks in modeling the return and volatility dynamics of precious metals. Q Rev Econ Financ 52: 207-218. https://doi.org/10.1016/j.qref.2012.04.004 doi: 10.1016/j.qref.2012.04.004
![]() |
[3] |
Baillie RT (1996) Long memory processes and fractional integration in econometrics. J Econometrics 73: 5-59. https://doi.org/10.1016/0304-4076(95)01732-1 doi: 10.1016/0304-4076(95)01732-1
![]() |
[4] |
Barkoulas JT, Baum CF, Travlos N (2000) Long memory in the greek stock market. Appl Financ Econ 10: 177-184. https://doi.org/10.1080/096031000331815 doi: 10.1080/096031000331815
![]() |
[5] | Basel Committee on Banking Supervision (2013) Fundamental review of the trading book: A revised market risk framework. Available from: https://www.bis.org/publ/bcbs265.pdf. |
[6] |
Bentes SR (2015) Forecasting volatility in gold returns under the garch, igarch and figarch frameworks: New evidence. Physica A 438: 355-364. https://doi.org/10.1016/j.physa.2015.07.011 doi: 10.1016/j.physa.2015.07.011
![]() |
[7] | Beran J (2017) Statistics for long-memory processes, Routledge. https://doi.org/10.1201/9780203738481 |
[8] |
Bollerslev T (1986) Generalized autoregressive conditional heteroskedasticity. J Econometrics 31: 307-327. https://doi.org/10.1016/0304-4076(86)90063-1 doi: 10.1016/0304-4076(86)90063-1
![]() |
[9] |
Cavanaugh JE, Neath AA (2019) The akaike information criterion: Background, derivation, properties, application, interpretation, and refinements. Wires Computat Stat 11: e1460. https://doi.org/10.1002/wics.1460 doi: 10.1002/wics.1460
![]() |
[10] |
Cerqueti R, Giacalone M, Panarello D (2019) A generalized error distribution copula-based method for portfolios risk assessment. Physica A 524: 687-695. https://doi.org/10.1016/j.physa.2019.04.077 doi: 10.1016/j.physa.2019.04.077
![]() |
[11] |
Cheung YW (1993) Long memory in foreign-exchange rates. J Bus Econ Stat 11: 93-101. https://doi.org/10.1080/07350015.1993.10509935 doi: 10.1080/07350015.1993.10509935
![]() |
[12] |
Chinhamu K, Chifurira R, Ranganai E (2022) Value-at-risk estimation of precious metal returns using long memory garch models with heavy-tailed distribution. J Stat Appl Probab 11: 89-107. http://dx.doi.org/10.18576/jsap/110107 doi: 10.18576/jsap/110107
![]() |
[13] |
Chkili W, Hammoudeh S, Nguyen DK (2014) Volatility forecasting and risk management for commodity markets in the presence of asymmetry and long memory. Energ Econ 41: 1-18. https://doi.org/10.1016/j.eneco.2013.10.011 doi: 10.1016/j.eneco.2013.10.011
![]() |
[14] |
Conrad C (2010) Non-negativity conditions for the hyperbolic garch model. J Econometrics 157: 441-457. https://doi.org/10.1016/j.jeconom.2010.03.045 doi: 10.1016/j.jeconom.2010.03.045
![]() |
[15] |
Conrad C, Haag BR (2006) Inequality constraints in the fractionally integrated garch model. J Financ Econometrics 4: 413-449. https://doi.org/10.1093/jjfinec/nbj015 doi: 10.1093/jjfinec/nbj015
![]() |
[16] |
Cont R (2001) Empirical properties of asset returns: stylized facts and statistical issues. Quant Financ 1: 223. https://dx.doi.org/10.1088/1469-7688/1/2/304 doi: 10.1088/1469-7688/1/2/304
![]() |
[17] |
Davidson J (2004) Moment and memory properties of linear conditional heteroscedasticity models, and a new model. J Bus Econ Stat 22: 16-29. https://doi.org/10.1198/073500103288619359 doi: 10.1198/073500103288619359
![]() |
[18] |
Ding Z, Granger CW, Engle RF (1993) A long memory property of stock market returns and a new model. J Empir Financ 1: 83-106. https://doi.org/10.1016/0927-5398(93)90006-D doi: 10.1016/0927-5398(93)90006-D
![]() |
[19] | Enow ST (2023) Exploring volatility clustering financial markets and its implication. J Econ Soc Dev Resilient Society 10. |
[20] | Fama EF (1965) The behavior of stock-market prices. J Bus 38: 34-105. https://www.jstor.org/stable/2350752 |
[21] |
Gaye Gencer H, Demiralay S (2016) Volatility modeling and value-at-risk (var) forecasting of emerging stock markets in the presence of long memory, asymmetry, and skewed heavy tails. Emerg Mark Financ Tr 52: 639-657. https://doi.org/10.1080/1540496X.2014.998557 doi: 10.1080/1540496X.2014.998557
![]() |
[22] |
Geweke J, Porter-Hudak S (1983) The estimation and application of long memory time series models. J Time Ser Anal 4: 221-238. https://doi.org/10.1111/j.1467-9892.1983.tb00371.x doi: 10.1111/j.1467-9892.1983.tb00371.x
![]() |
[23] |
Haque ME, Farzana N (2021) Modeling asymmetric effects and long memory in conditional volatility of dhaka stock exchange: New evidence from family of figarch models. Account Financ 9: 1103-15. https://dx.doi.org/10.13189/ujaf.2021.090520 doi: 10.13189/ujaf.2021.090520
![]() |
[24] | Härdle WK, Mungo J (2008) Value-at-risk and expected shortfall when there is long range dependence. |
[25] |
Hurst HE (1951) Long-term storage capacity of reservoirs. T Am Soc Civil Eng 116: 770-799. https://doi.org/10.1061/TACEAT.0006518 doi: 10.1061/TACEAT.0006518
![]() |
[26] | Jorion P (2007) Value at risk: the new benchmark for managing financial risk, The McGraw-Hill Companies, Inc. Available from: https://thuvienso.hoasen.edu.vn/handle/123456789/10569. |
[27] | Kasman A (2009) Estimating value-at-risk for the turkish stock index futures in the presence of long memory volatility. Cent Bank Rev 9: 1. Available from: https://core.ac.uk/reader/6462820. |
[28] |
Kaya Soylu P, Okur M, Çatıkkaş Ö, et al. (2020) Long memory in the volatility of selected cryptocurrencies: Bitcoin, ethereum and ripple. J Risk Financ Manag 13: 107. https://doi.org/10.3390/jrfm13060107 doi: 10.3390/jrfm13060107
![]() |
[29] |
Klar B, Lindner F, Meintanis SG (2012) Specification tests for the error distribution in garch models. Computat Stat Data Anal 56: 3587-3598. https://doi.org/10.1016/j.csda.2010.05.029 doi: 10.1016/j.csda.2010.05.029
![]() |
[30] |
Kupiec PH (1995) Techniques for verifying the accuracy of risk measurement models. J Deriv 3: 73-84. https://doi.org/10.3905/jod.1995.407942 doi: 10.3905/jod.1995.407942
![]() |
[31] |
Li M, Li WK, Li G (2015) A new hyperbolic garch model. J Econometrics 189: 428-436. https://doi.org/10.1016/j.jeconom.2015.03.034 doi: 10.1016/j.jeconom.2015.03.034
![]() |
[32] | Ma J (2019) Test and application of persistence point of long memory time series, in '1st International Conference on Business, Economics, Management Science (BEMS 2019), Atlantis Press, 1-8. https://doi.org/10.2991/bems-19.2019.1 |
[33] |
Mabrouk S, Saadi S (2012) Parametric value-at-risk analysis: Evidence from stock indices. Q Rev Econ Financ 52: 305-321. https://doi.org/10.1016/j.qref.2012.04.006 doi: 10.1016/j.qref.2012.04.006
![]() |
[34] |
McMillan D, Thupayagale P (2010) Evaluating stock index return value-at-risk estimates in south africa: Comparative evidence for symmetric, asymmetric and long memory garch models. J Emerg Mark Financ 9: 325-345. https://doi.org/10.1177/097265271000900304 doi: 10.1177/097265271000900304
![]() |
[35] |
Mighri Z, Jaziri R (2023) Long-memory, asymmetry and fat-tailed garch models in value-at-risk estimation: Empirical evidence from the global real estate markets. J Q Econ 21: 41-97. https://doi.org/10.1007/s40953-022-00331-w doi: 10.1007/s40953-022-00331-w
![]() |
[36] |
Patra B, Padhi P (2015) Backtesting of value at risk methodology: Analysis of banking shares in india. J Appl Econ Res 9: 254-277. https://doi.org/10.1177/0973801015583739 doi: 10.1177/0973801015583739
![]() |
[37] | Peters JP (2001) Estimating and forecasting volatility of stock indices using asymmetric garch models and (skewed) student-t densities. Preprint, University of Liege, Belgium 3: 2. |
[38] |
Purczyński J, Bednarz-Okrzyńska K (2014) Estimation of the shape parameter of ged distribution for a small sample size. Folia Oecon Stetinensia 14: 35-46. https://doi.org/10.2478/foli-2014-0103 doi: 10.2478/foli-2014-0103
![]() |
[39] |
Sethapramote Y, Prukumpai S, Kanyamee T (2014) Evaluation of value-at-risk estimation using long memory volatility models: Evidence from stock exchange of thailand. Available at SSRN 2396531. https://dx.doi.org/10.2139/ssrn.2396531 doi: 10.2139/ssrn.2396531
![]() |
[40] |
So MK (2000) Long-term memory in stock market volatility. Appl Financ Econ 10: 519-524. https://doi.org/10.1080/096031000416398 doi: 10.1080/096031000416398
![]() |
[41] | Szczygielski JJ, Chipeta C (2023) Properties of returns and variance and the implications for time series modelling: Evidence from south africa. Mod Financ 1: 35-55. Available from: https://mf-journal.com/index.php/mf/article/download/8/61. |
[42] |
Tang TL, Shieh SJ (2006) Long memory in stock index futures markets: A value-at-risk approach. Physica A 366: 437-448. https://doi.org/10.1016/j.physa.2005.10.017 doi: 10.1016/j.physa.2005.10.017
![]() |
[43] |
Tayefi M, Ramanathan T (2012) An overview of figarch and related time series models. Austrian J Stat 41: 175-196. https://doi.org/10.17713/ajs.v41i3.172 doi: 10.17713/ajs.v41i3.172
![]() |
[44] |
Thupayagale P (2011) Long memory in the volatility of an emerging fixed-income market: Evidence from south africa. S Afr J Econ 79: 290-300. https://doi.org/10.1111/j.1813-6982.2011.01274.x doi: 10.1111/j.1813-6982.2011.01274.x
![]() |
[45] |
Tripathy N (2022) Long memory and volatility persistence across brics stock markets. Res Int Bus Financ 63: 101782. https://doi.org/10.1016/j.ribaf.2022.101782 doi: 10.1016/j.ribaf.2022.101782
![]() |
[46] | Tse YK (1998) The conditional heteroscedasticity of the yen-dollar exchange rate. J Appl Econometrics 13: 49-55. |
[47] |
Wu PT, Shieh SJ (2007) Value-at-risk analysis for long-term interest rate futures: Fat-tail and long memory in return innovations. J Empir Financ 14: 248-259. https://doi.org/10.1016/j.jempfin.2006.02.001 doi: 10.1016/j.jempfin.2006.02.001
![]() |
[48] | Yaya OS (2013) Nigerian stock index: a search for optimal garch model using high frequency data', CBN J Appl Stat 4: 69-85. http://hdl.handle.net/10419/142079 |