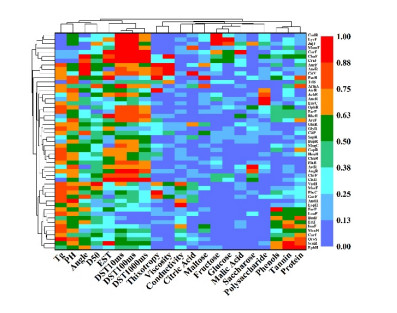
Fluidized bed granulation (FBG) is a widely used granulation technology in the pharmaceutical industry. However, defluidization caused by the formation of large aggregates poses a challenge to FBG, particularly in traditional Chinese medicine (TCM) due to its complex physicochemical properties of aqueous extracts. Therefore, this study aims to identify the complex relationships between physicochemical characteristics and defluidization using data mining methods. Initially, 50 types of TCM were decocted and assessed for their potential influence on defluidization using a set of 11 physical properties and 10 chemical components, utilizing the loss rate as an evaluation index. Subsequently, the random forest (RF) and Apriori algorithms were utilized to uncover intricate association rules among physicochemical characteristics and defluidization. The RF algorithm analysis revealed the top 8 critical factors associated with defluidization. These factors include physical properties like glass transition temperature (Tg) and dynamic surface tension (DST) of DST100ms, DST1000ms, DST10ms and conductivity, in addition to chemical components such as fructose, glucose and protein contents. The results from Apriori algorithm demonstrated that lower Tg and conductivity were associated with an increased risk of defluidization, resulting in a higher loss rate. Moreover, DST100ms, DST1000ms and DST10ms exhibited a contrasting trend in the physical properties Specifically, defluidization probability increases when Tg and conductivity dip below 29.04℃ and 6.21 ms/m respectively, coupled with DST10ms, DST100ms and DST1000ms values exceeding 70.40 mN/m, 66.66 mN/m and 61.58 mN/m, respectively. Moreover, an elevated content of low molecular weight saccharides was associated with a higher occurrence of defluidization, accompanied by an increased loss rate. In contrast, protein content displayed an opposite trend regarding chemical properties. Precisely, the defluidization likelihood amplifies when fructose and glucose contents surpass 20.35 mg/g and 34.05 mg/g respectively, and protein concentration is less than 1.63 mg/g. Finally, evaluation criteria for defluidization were proposed based on these results, which could be used to avoid this situation during the granulation process. This study demonstrated that the RF and Apriori algorithms are effective data mining methods capable of uncovering key factors affecting defluidization.
Citation: Sai Fu, Yuting Luo, Yuling Liu, Qian Liao, Shasha Kong, Anhui Yang, Longfei Lin, Hui Li. Mining association rules between the granulation feasibility and physicochemical properties of aqueous extracts from Chinese herbal medicine in fluidized bed granulation[J]. Mathematical Biosciences and Engineering, 2023, 20(11): 19065-19085. doi: 10.3934/mbe.2023843
[1] | Muhammad Akram, Ahmad N. Al-Kenani, Anam Luqman . Degree based models of granular computing under fuzzy indiscernibility relations. Mathematical Biosciences and Engineering, 2021, 18(6): 8415-8443. doi: 10.3934/mbe.2021417 |
[2] | David Moreno-Martos, Sean Foley, Benjamin Parcell, Dumitru Trucu, Raluca Eftimie . A computational investigation of COVID-19 transmission inside hospital wards and associated costs. Mathematical Biosciences and Engineering, 2022, 19(7): 6504-6522. doi: 10.3934/mbe.2022306 |
[3] | Sakthieswaran Natarajan, Shiny Brintha Gnanadurai . An approach to study the inter-relationship between mechanical and durability properties of ternary blended cement concrete using linear regression analysis. Mathematical Biosciences and Engineering, 2019, 16(5): 3734-3752. doi: 10.3934/mbe.2019185 |
[4] | Huihui Hu, Fengjie Wu, Jisong Zhang, Enguo Chen . Treatment of secondary benign airway stenosis after tracheotomy with Montgomery T-tube. Mathematical Biosciences and Engineering, 2019, 16(6): 7839-7849. doi: 10.3934/mbe.2019394 |
[5] | Fabiana Russo, Alberto Tenore, Maria Rosaria Mattei, Luigi Frunzo . Multiscale modelling of the start-up process of anammox-based granular reactors. Mathematical Biosciences and Engineering, 2022, 19(10): 10374-10406. doi: 10.3934/mbe.2022486 |
[6] | Wangping Xiong, Kaiqi Wang, Shixiong Liu, Zhaoyang Liu, Yimin Zhu, Peng Liu, Ming Yang, Xian Zhou . Multiple prescription pattern recognition model based on Siamese network. Mathematical Biosciences and Engineering, 2023, 20(10): 18695-18716. doi: 10.3934/mbe.2023829 |
[7] | David Moreno Martos, Benjamin J. Parcell, Raluca Eftimie . Modelling the transmission of infectious diseases inside hospital bays: implications for COVID-19. Mathematical Biosciences and Engineering, 2020, 17(6): 8084-8104. doi: 10.3934/mbe.2020410 |
[8] | Xiao Wang, Jianbiao Zhang, Ai Zhang, Jinchang Ren . TKRD: Trusted kernel rootkit detection for cybersecurity of VMs based on machine learning and memory forensic analysis. Mathematical Biosciences and Engineering, 2019, 16(4): 2650-2667. doi: 10.3934/mbe.2019132 |
[9] | Shuilong Zou, Zhaoyang Liu, Kaiqi Wang, Jun Cao, Shixiong Liu, Wangping Xiong, Shaoyi Li . A study on pharmaceutical text relationship extraction based on heterogeneous graph neural networks. Mathematical Biosciences and Engineering, 2024, 21(1): 1489-1507. doi: 10.3934/mbe.2024064 |
[10] | Ji Zhou, Zhanlin Su, Shahab Hosseini, Qiong Tian, Yijun Lu, Hao Luo, Xingquan Xu, Chupeng Chen, Jiandong Huang . Decision tree models for the estimation of geo-polymer concrete compressive strength. Mathematical Biosciences and Engineering, 2024, 21(1): 1413-1444. doi: 10.3934/mbe.2024061 |
Fluidized bed granulation (FBG) is a widely used granulation technology in the pharmaceutical industry. However, defluidization caused by the formation of large aggregates poses a challenge to FBG, particularly in traditional Chinese medicine (TCM) due to its complex physicochemical properties of aqueous extracts. Therefore, this study aims to identify the complex relationships between physicochemical characteristics and defluidization using data mining methods. Initially, 50 types of TCM were decocted and assessed for their potential influence on defluidization using a set of 11 physical properties and 10 chemical components, utilizing the loss rate as an evaluation index. Subsequently, the random forest (RF) and Apriori algorithms were utilized to uncover intricate association rules among physicochemical characteristics and defluidization. The RF algorithm analysis revealed the top 8 critical factors associated with defluidization. These factors include physical properties like glass transition temperature (Tg) and dynamic surface tension (DST) of DST100ms, DST1000ms, DST10ms and conductivity, in addition to chemical components such as fructose, glucose and protein contents. The results from Apriori algorithm demonstrated that lower Tg and conductivity were associated with an increased risk of defluidization, resulting in a higher loss rate. Moreover, DST100ms, DST1000ms and DST10ms exhibited a contrasting trend in the physical properties Specifically, defluidization probability increases when Tg and conductivity dip below 29.04℃ and 6.21 ms/m respectively, coupled with DST10ms, DST100ms and DST1000ms values exceeding 70.40 mN/m, 66.66 mN/m and 61.58 mN/m, respectively. Moreover, an elevated content of low molecular weight saccharides was associated with a higher occurrence of defluidization, accompanied by an increased loss rate. In contrast, protein content displayed an opposite trend regarding chemical properties. Precisely, the defluidization likelihood amplifies when fructose and glucose contents surpass 20.35 mg/g and 34.05 mg/g respectively, and protein concentration is less than 1.63 mg/g. Finally, evaluation criteria for defluidization were proposed based on these results, which could be used to avoid this situation during the granulation process. This study demonstrated that the RF and Apriori algorithms are effective data mining methods capable of uncovering key factors affecting defluidization.
Abbreviations: FBG: Fluidized bed granulation; QbD: Quality by Design; TCM: Traditional Chinese medicine; RF: Random forest; Tg: Transition temperature; DST: Dynamic surface tension; Work: Work of adhesion; FGSM: The sum of low molecular weight saccharides; DOE: Design of experiment; CFD: Computational Fluid Dynamics; DEM: Discrete element method; PBM: Population balance model; NIR: Near-infrared reflectance; MD: Maltodextrin; EST: Equilibrium surface tension; ZS: The sum content of malic acid and citric acid; ECR: The aqueous extract of Codonopsis radix; ECH: The aqueous extract of Cistanches Herba; HPMC: Hydroxypropyl methyl cellulose; HPLC-ELSD: High-performance liquid chromatography with evaporative light scattering detection
FBG is a size enlargement process integrating mixing, wetting, granulating and drying in a single piece of equipment. The formation process proceeds as follows. Atomized feed solutions are sprayed onto a fluidization solid particle bed, causing the particles to become wetter and stickier, facilitating mutual particle bonding and the creation of a liquid bridge through a combination of capillary and viscous forces. Subsequently, solid bridges are formed through either a drying or sintering processes, resulting in particle enlargement [1]. Due to its advantages, such as high production efficiency, uniform temperature distribution, large solid-gas contact area, high heat transfer rate with low material loss during transfer and uniform mixing of the product, among others, FBG has been widely used as a granule fabrication technology in the pharmaceutical industry [2].
Defluidization, resulting from the formation of significant agglomerates, represents an adverse occurrence in the FBG granulation process, potentially precipitating process halts, heightened loss rates and the rejection of complete batches, among other complications. It is a phenomenon shaped by a myriad of factors ranging from formulation architecture and the physical-chemical attributes of feed solutions to the primal particle dimensions, operational stipulations, droplet dimensions and the machinery's geometric design [3,4,5]. Given the intrinsic complexity of the FBG procedure, improper formation parameters can catalyze undesirable granulation dynamics, escalating to the point of defluidization. Consequently, fostering a nuanced understanding of the interrelations among the critical material attributes, critical process parameters and critical quality attributes becomes indispensable. Establishing this understanding within a quality by design (QbD) framework for manufacturing processes assumes paramount importance in safeguarding the quality and efficacy of the production cycle [6,7].
Numerous studies have substantiated that procedural parameters critically influence agglomeration kinetics, thereby facilitating a macro-control strategy for FBG grounded in macro-scale perspectives. Ming et al. [8] underscored the pronounced impact of inlet air temperature, binder solution rate and binder-to-powder ratio on pivotal quality attributes including flowability, temperature regulation, moisture content, aggregation indices and compactability. Additionally, Krzywanski et al. [9] leveraged a fuzzy logic methodology to optimize fluidized bed jet milling processes, demonstrating that augmenting working air pressure and test duration while reducing rotational speed amplifies process effectiveness, yielding optimal predictive performance. In parallel, the advent of computer simulations and large-scale parallelization techniques have catalyzed an enriched understanding of the FBG process through mechanism models [10] and in-line process analytical technology [11]. These advancements permit the high-definition visualization of simulation outcomes based on various mechanistic models, illuminating the effects of equipment geometry and process parameters on elements such as pressure drop, velocity fluctuations, temperature variations and alterations in solid volume fractions across different fluid flow domains [12]. This analytical paradigm extends to detail the dispersion of the solid phase and delineates collision energy and frequency, alongside contact force dynamics [13]. Furthermore, mechanistic rate expressions forge connections between particle growth phases and the aforementioned determinants [14], facilitating in-line techniques to monitor transformations in transport velocity [15], moisture retention [16] and granule surface compositions [17], thus enhancing the depth of understanding in FBG processes. A growing body of evidence, supported by emerging technologies, corroborates the substantial role of process factors in dictating agglomeration kinetics [18,19].
In the context of feed solutions, a considerable number of researchers have honed in on analyzing the ramifications of nozzle configuration and varied process parameters on the spraying procedure. The collective findings highlight those factors such as spray air pressure [20,21], and the differences in velocity between liquid and gas phases or liquid volume flow rates [22,23] exert the most substantial influence on droplet dimensions. The drying trajectory of these droplets is principally dictated by gas parameters including velocity, viscosity and density, alongside binder attributes and ambient temperature. It is observed that smaller droplets undergo rapid desiccation before interacting with primary particles, thereby yielding a proliferation of diminutive particles constituted of purely dried feed solution. This stands in contrast to larger droplets, which demonstrate a protracted drying cycle. Instances where droplets are excessively large and sprayed at high velocities may provoke a collapse [24]. Under conditions of reduced atomization pressures and elevated temperatures paired with high spray speeds and large droplets, there is a tendency to foster increased surface ruggedness, whereas the reverse conditions facilitate the formation of smooth and densely structured granules [25]. Concurrent research dictates that particle characteristics are fundamentally shaped by process parameters and the inherent properties of the feed solution [26]. In a pertinent study, Düsenberg et al. [27] posited that it is the agglomerate morphology and stability predominantly driven by material properties that hold a higher sway over the outcome, compared to spray parameters. In scenarios with unsuitable parameters, the risk of collapse increases [24]. However, in terms of material properties, the influence remains somewhat limited to a handful of aspects such as viscosity, surface tension or contact angle impacting the particle aggregation mechanics, a topic explored in further depth in subsequent studies [28,29,30,31].
However, the FBG process, when applied to TCM utilizing aqueous extracts from Chinese medicinal herbs as feed solutions, faces substantial challenges. Desired agglomeration often occurs alongside unfavorable outcomes such as the formation of large agglomerates and pronounced sticking, outcomes spurred by excessive wetting and nucleation, culminating in defluidization. Simple adjustments to the previously mentioned physical and chemical parameters, or alternations in equipment and process parameters, fall short in fully mitigating the defluidization issue. While the incorporation of excipients serves to somewhat alleviate this concern, the fundamental underlying mechanism remains elusive. Consequently, predicting defluidization persists as a daunting challenge, and a concrete delineation of the pivotal feed solution physical parameters that dictate process viability remains undefined.
This study endeavors to enhance the comprehension of the influence exerted by the physicochemical parameters of the feed solution on the granulation procedure. It involved an extensive assessment of the feed solutions extracted from 50 varieties of Chinese medicinal materials, wherein 11 physical attributes and 10 chemical components were meticulously identified. Moreover, the granulation loss rate was instituted as a benchmark for evaluating granulation feasibility. Subsequently, essential indicators influencing granulation feasibility were discerned from the pool of potential physicochemical parameters under consideration. This was followed by an exploration of the association rules between these critical parameters and loss rates. Lastly, evaluation criteria for defluidization were established based on the association rules. These criteria aid in achieving a deeper understanding of the process and elucidate the factors influencing FBG.
50 herbs were purchased from Beijing Qiancao Medicinal Materials Electuary Co. Ltd. (Beijing, China). The herbs were extracted by reflux extraction. In brief, an appropriate amount of the herbs was placed into a round-bottom flask, and eight times the volume of deionized water (w/v) was added. The reflux extraction method was used for extraction with 1.5 hours per cycle, and the process was repeated once following the procedure mentioned above. Subsequently, the aqueous extract was concentrated to a density of 1.15 g/cm3 (50℃) to obtain the traditional Chinese medicine decoctions.
The feed solution contains a large number of small molecules of sugars, polysaccharides and proteins, which are in an amorphous state, and has a glass transition phenomenon. In order to maintain the feed solution properties [32], a freeze-drying technique was used to dry 50 types of Chinese medical aqueous decoction into solid powder, and Tg was measured for each sample to reflect the thermodynamic properties of the material in the drying or granulation. The thermograms of the samples were determined by DSC-Q2000 (TA Instruments, USA), equipped with a double scanning procedure to improve the measurement accuracy. The scanning procedures were based on Shi's report [33]. About 5 mg of samples were used, and the endpoint temperature in the heating procedure was 200℃ for each program. The midpoint temperature was considered to be Tg [34].
Wettability, an essential variable during the nucleation stage in FBG, was evaluated via contact angle measurements. To minimize measurement error, maltodextrin (MD) powders, with a fixed weight of 300 mg, were compacted using an infrared tablet pressing mechanism (Tianjin Botianshengda Technology Development Ltd. Co., China) with 13 mm diameter punching and die sets. The load was maintained at 1MT, and the load was held for 1 minute during pressing.
The contact angles between the MD tablet and feed solution were measured by the DSA100 contact angle tester (Krüss Ltd. Co., Hamburg, Germany). The sessile drop method was used with a dripping velocity of 2 μl/s, and droplet volume of 2 μl. All analyses were carried out in quintuplicate.
The EST values of feed solutions were measured with a K100 surface tension meter (Krüss Ltd. Co., Hamburg, Germany). The EST calibration value was 72.00 ± 0.50 mN/m with approximately 36 ml distilled water at 25℃. Subsequently, the sample EST was recorded over time using the ADVANCE software. The result was presented as an average of five readings for each sample.
The DST values over 10~1000 ms of samples were conducted using a BP100 bubble pressure tension meter (KRÜSS Ltd. Co. Hamburg, Germany). The experimental conditions were detailed in the report of Cheng et al. [35]. Diagrams illustrating the DST over 10 ~ 1000 ms were recorded.
The rheological properties were determined using a rotational rheometer (MCR2, Anton Paar, Austria) equipped with a CC-27 rotor system (Coaxial cylinder type). Before measurement, samples were submerged in a water bath (EYELA, Japan) heated up to 50℃. The measurements included plotting viscosity vs. time curves at a shear rate 50 s-1 at 50℃, recording 50 data points every 300 s. The result was presented as an average of five values for each sample.
The thixotropy was measured using a rotational rheometer, with the thixotropic ring area representing the energy required to disrupt the internal structure of the aqueous extracts. The three-stage measurement procedure is as follows. In the first step, a shear rate range of 0.01 ~ 1000 s-1 was used, and 30 data points were recorded throughout the 300 s. In the second step, a shear rate of 1000 s-1 was maintained for 5 s, and 5 data points were recorded. In the third step, the shear rate ranged from 1000 to 0.01 s-1, and 30 data points were recorded over a period of 300 s. In the rheological curve diagram, an upper and lower rheological curve formed a closed "shuttle-type" thixotropic loop representing its thixotropy.
PH value was measured using an S20 PH meter (SevenEasy. Mettler-Toledo), and conductivity was determined using an FE38-Standard conductivity meter (Mettler-Toledo) at 25℃.
The droplet size was measured using a laser particle size analyzer (winner 319, Winner Particle Jinan, China). Data was obtained by measuring the intensity of light scattered as a laser beam passes through a spray. The process conditions included atomization pressure set at 1.5 kg/cm2, spray rate at 10 rad/min, a measured distance of 100 mm from the nozzle tip and a nozzle with a 0.8 mm diameter. The nozzle was secured using a method similar to that in Zeng's report [36].
The contents of fructose, glucose, sucrose and maltose in feed solutions were determined using a high-performance liquid chromatography with evaporative light scattering detection (HPLC-ELSD) method. The analysis was performed on a Waters XBridge® amide column (250 mm × 4.6 mm, 5 μm) with isocratic elution of 80 : 20 acetonitrile/water containing 0.2% triethylamine(v/v). The drift tube temperature was set at 95℃ and the flow rate was maintained at 2.6 L/min.
A modified method, as described by Liu [37], was utilized to evaluate malic acid and citric acid. In brief, the method was as follows: column-a Waters XSelect® HSS T3 column (250 mm × 4.6 mm, 5 μm); mobile phases-5:95 acetonitrile/water with 0.5% ammonium dihydrogen phosphate (PH set to 2.35 with 1mol/L Phosphoric Acid solution); flow rate-0.5 mL/min; column temperature-25℃; detection wavelength-214 nm.
Polysaccharide contents were determined using the phenol-sulfuric acid method [38]. According to the 2020 edition of the Chinese Pharmacopoeia, polyphenols and tannins were determined following the requirements of protocol 2202, while proteins were assessed using protocol 0731.
Before granulation, the 50 types of aqueous extract from Chinese medicine as feed solutions were heated and preserved at 50℃ throughout the entire process. A total of 150 g of MD, sieved through a 180 µm sieve, was introduced as the initial particles in the bed chamber. The batch size was maintained at a fixed value of 300 g.
A lab-scale batch fluidized bed granulator (WBF-2G, Chongqing Enger Granulating & Coating Technology Co., Ltd., China) was used in the granulation process. Prior to the experiment, MD was preheated in the reactor under dry fluidization conditions at a constant inlet air temperature of 80℃ and a flow rate of 60 m3/h for 10 minutes, reaching a preheat temperature of approximately 40℃. The feed solution injection began once thermal equilibrium was reached. The process parameters were as follows: inlet air temperature, 75℃; flow rate, 60−80 m3/h; atomizing pressure, 1.5 kg/cm2; ambient humidity, 20−35%; peristaltic pump speed, 10 rad/min (equivalent to 10 ml/min). Similarly, the following parameters were used during the drying process: drying time, 5 min; inlet fluidizing velocity, 80−50 m3/h based on visually acceptable fluidization. Samples were collected after completion of the process and dried in a drier until residual moisture content dropped below 3%. These process parameters ensured consistent levels of fluidization state and particle trajectory, as observed in previous studies.
The defluidization phenomena were observed in the FBG. The loss rate was calculated as the ratio of the reduction in the dried mass of solids collected to the sum of MD and the mass in the feed solution.
Data mining is utilized to uncover patterns and rules within intricate datasets. Considering the complexity of the data, a random forest algorithm was used to screen the vital characteristic variables affecting the particle loss rate. Then, an association algorithm was used to quantitatively examine the association rules between different physicochemical parameters and the loss rates using Python (version 3.9).
The RF algorithm has gained popularity in various scientific fields as a machine learning technique. It is employed not only for constructing prediction models through classification or regression, but also for permutating the importance of variables in both high-dimensional data and computational models. It is appropriate for analyzing continuous variables, discrete variables or missing data. In this study, the RF classification algorithm was used to uncover nonlinear and complex relationships between physicochemical parameters and loss rates, as well as identify fundamental properties that contribute to defluidization effects and influence granulation feasibility [39].
RF is an integrated learning method that consists of multiple decision trees. The corresponding steps were summarized as follows: The original dataset was assumed to be composed of input variables with n-dimensional vectors and an output variable. First, training data was generated from the original dataset to establish the RF model. Second, for each tree in the forest, an in-bag dataset was randomly selected from the upper dataset. This process was repeated until n-trees were grown using the CART algorithm, which employs binary recursive partitioning. Lastly, Gini importance, permutation importance or conditional permutation importance were used to permutate variable importance measures. Accuracy, precision, recall and F1-score were performance metrics to evaluate classification models [40].
Association rule analysis was utilized to reflect the dependence and correlation between two variables, enabling the prediction of event Y based on event X when a specific correlation exists between events XY. The Apriori algorithm is a well-known method that utilizes an item-based, discovery-oriented approach to uncover association rules. Its core is an iterative method of hierarchical search to find frequent itemsets and reveal all relationships among items. To boost computational efficiency and filter out invalid candidate itemsets and generated association rules, an optimized Apriori algorithm [41] was applied to reveal multidimensional association rules with the combination of multiple factors. In this approach, the front and rear items of the dataset were first fixed according to physicochemical parameters and granular properties. Subsequently, all the front and rear items were stored separately. In this study, the itemsets with physicochemical parameters were brought into the front itemset, while those relating to granular properties were brought into the rear itemset. Through data scanning, the candidate items were calculated and filtered according to the predefined support, confidence and lift threshold. Support indicates the probability of simultaneously containing both X and Y within the entire database. Confidence represents the probability of Y occurring given that X has occurred. Lift represents the ratio of the probability of simultaneous occurrence of both X and Y to the probability of Y occurring alone. The lift value greater than 1 suggests a strong relationship between the two items. The corresponding equations for support, confidence and lift threshold were given below.
(1) |
(2) |
(3) |
where P(X) is the probability of X occurring alone; P(Y) is the probability of Y occurring alone; P(X, Y) is the probability of both X and Y co-occurring. X represents specific-range physicochemical parameters, and Y represents specific-range loss rate. Finally, I refers to the entire database.
The strong association rules meeting the criteria were ultimately obtained, ensuring each rule is presented in the anticipated format for research purposes. Therefore, the optimized Apriori algorithm was utilized to mine relationships between physical parameters, chemical properties and loss rate of granulation from a dataset of 50 samples. Moreover, it was aimed to discover the fundamental properties that affect the feasibility of granulation by the analysis of these association rules.
The physical properties and the content of the chemical components of 50 herbal solutions were determined. Figure 1 illustrated the wide distribution of physicochemical properties across all samples without extreme outliers. It indicated that the selected samples and the subsequent analysis results were reasonably representative.
The RF method was employed to analyze the importance of the 21 variables affecting the granulation loss rate across about 50 types of herbs. A random grid search method with 10-fold cross-validation was used to avoid overfitting to determine the optimal hyperparameter values before constructing the model. Figure 2(a) depicted the importance of independent variables, while Figure 2(b) presented performance metrics such as accuracy, precision, recall and F1-score used to confirm the optimal number of variables. The variables ranked in descending order of importance are: Tg, DST100ms, Fructose, DST1000ms, DST10ms, Glucose, Protein, Conductivity. Figure 2(b) illustrated performance metrics that demonstrated the RF model's accurate classification of loss rates, achieving an accuracy of 0.86, a precision of 0.85, a recall of 0.79 and an F1-score of 0.81 in the best model utilizing the top 8 variables. Selecting these top 8 variables only in the model development process ensured its robustness. Among these variables are factors related to physical properties like Tg, DST100ms, DST1000ms, DST10ms and Conductivity. Factors related to chemical properties like Fructose, Glucose and Protein. These factors were listed among the top 8 most important variables related to particle loss rate.
Normalizing and discretizing the data is necessary to adhere to the Apriori algorithm, Figure 3 illustrated the results of discretization, where each impact factor was divided into six categories based on different ranges, and loss rate was separated into three categories.
The loss rates in the granulation process were divided into three grades. When the defluidization phenomenon occurred by the formation of large agglomerates, the loss rate was classified as level 3 (50,100). When larger aggregates adhere to the wall but do not lead to a collapse, the loss rate was classified as level 2 (15, 50). When abrasion, fragmentation, slight wall adhesion, spray drying or normal fluildization result in the desired granulation, the loss rate was classified as level 1 (0, 15). It is generally accepted as a satisfactory production result in the FBG process. Next, the items with physicochemical parameters were brought into the front itemset, and the loss rate was brought into the rear itemset. Finally, strong association rules meeting the requirements were obtained. Figure 4 depicted the association rules of the top 8 impact factors to the loss rate satisfying the pre-defined metrics. These top 8 impact factors provide essential insights into the relationship between physicochemical parameters and loss rate.
The Tg was brought into the front itemset, and the loss rate was brought into the rear itemset. The association rules that met the requirement were as follows. T1→L3 > T2→L2 > T6→L1 > T5→L1 > T3→L1 > T4→L1 (Table 1). The results indicated a decrease in loss rates with an increase in Tg (Figure 5(a)). A confidence level of 1 was observed for Tg (11.42, 29.04) → loss (50,100), suggesting a 100% probability of defluidization occurring when Tg was below 29.04 ℃. Similarly, a confidence level of 1 was found for Tg (29.04, 53.80) → loss (15, 50), indicating a complete occurrence of larger aggregates or sticking to the wall but not bed collapses when Tg fell within the range of (29.04, 53.80). Furthermore, a confidence level of 0.82 was obtained for Tg (53.80,123.10) → loss (0, 15), implying an 82% probability of normal granulation when Tg exceeded 53.80℃. In conclusion, Tg at 29.04℃ might be a transitional point influencing defluidization during FBG under material temperatures around 40 ± 2℃ in the granulation process. Considering these findings, Tg at approximately 53.80℃ might be another transitional point affecting granulation feasibility.
Factor code | Front itemset | Rear itemset | Support | Confidence | Lift |
T1→L3 | Tg = (11.42, 29.04) | Loss = (50.0,100.0) | 0.18 | 1.00 | 5.67 |
T2→L2 | Tg = (29.04, 53.80) | Loss = (15.0, 50.0) | 0.16 | 1.00 | 3.64 |
T3→L1 | Tg = (53.80, 88.39) | Loss = (0.0, 15.0) | 0.14 | 0.78 | 1.42 |
T4→L1 | Tg = (88.39, 94.46) | Loss = (0.0, 15.0) | 0.12 | 0.75 | 1.37 |
T5→L1 | Tg = (94.46, 99.06) | Loss = (0.0, 15.0) | 0.14 | 0.88 | 1.59 |
T6→L1 | Tg = (99.06,123.10) | Loss = (0.0, 15.0) | 0.16 | 0.89 | 1.62 |
Different concentrations of MD were added to the aqueous extract of Codonopsis radix (ECR). The Tg values of ECR over the concentrations of the MD were shown in Figure 6. The Tg value of ECR alone was 13.46℃, which was significantly lower compared to the Tg values observed for ECR + 5%MD, ECR + 10%MD, and ECR + 15%MD, where Tg increased significantly to 23.10℃, 47.92℃ and 57.06℃, respectively. The corresponding loss rate decreased, ranging from 100% to 29.47%, then 13.67% (Figure 5(b)). Furthermore, this transition shifted the granulation state from a collapsed bed to a larger wet mass toward achieving a normal fluidization state. Consequently, materials with high Tg exhibited reduced wall adhesion or defluidization during FBG processing, underscoring the critical role of Tg in determining FBG feasibility.
During the granulation process, the solutions were dispersed into droplets through the atomizer within a few seconds, and the surface tension was always in an unbalanced state. Studying DST is of great significance. The DST was brought into the front itemset, and the loss rate was in the rear itemset. Table 2 and Figure 7 showed association rules meeting the requirement. The impact factors from strong to weak were in the following order. DST100ms > DST1000ms > DST10ms. As for DST100ms, the rules from strong to weak were as follows. D21→L1 > D26→L3 > D22→L1 > D23→L1 > D25→L2 > D24→L2. Confidence was 1 for D21→L1 (DST100 ms (44.46, 53.16) → loss (0, 15), indicating that if the DST100ms ranged from 44.46 to 53.16, the probability of the granulation within the normal loss rate equals 100%. It can be summarized by analyzing Figure 7(a). As the DST100ms of the feed solutions increases, the loss rate also increases, which is not conducive to the granulation process. This trend was consistent for DST10ms and DST1000ms, as shown in Figures 7(b), (c). It means that as the DST increases, the chances of defluidization also increase.
Factors | Factor code | Front itemset | Rear itemset | Support | Confidence | Lift |
DST100ms | D21→L1 | DST100ms = (44.46, 53.16) | Loss = (0.0, 15.0) | 0.18 | 1.00 | 1.82 |
D22→L1 | DST100ms = (53.16, 54.90) | Loss = (0.0, 15.0) | 0.14 | 0.88 | 1.59 | |
D23→L1 | DST100ms = (54.90, 59.27) | Loss = (0.0, 15.0) | 0.14 | 0.78 | 1.42 | |
D24→L2 | DST100ms = (59.27, 62.95) | Loss = (15.0, 50.0) | 0.10 | 0.63 | 2.28 | |
D25→L2 | DST100ms = (62.95, 66.66) | Loss = (15.0, 50.0) | 0.12 | 0.75 | 2.73 | |
D26→L3 | DST100ms = (66.66, 70.16) | Loss = (50.0,100.0) | 0.16 | 0.89 | 5.04 | |
DST1000ms | D31→L1 | DST1000ms = (42.83, 49.51) | Loss = (0.0, 15.0) | 0.16 | 0.89 | 1.62 |
D32→L1 | DST1000ms = (49.51, 52.48) | Loss = (0.0, 15.0) | 0.16 | 1.00 | 1.82 | |
D33→L1 | DST1000ms = (52.48, 54.57) | Loss = (0.0, 15.0) | 0.16 | 0.89 | 1.62 | |
D34→L2 | DST1000ms = (54.57, 59.47) | Loss = (15.0, 50.0) | 0.10 | 0.63 | 2.28 | |
D35→L2 | DST1000ms = (59.47, 61.58) | Loss = (15.0, 50.0) | 0.10 | 0.63 | 2.28 | |
D36→L3 | DST1000ms = (61.58, 69.25) | Loss = (50.0,100.0) | 0.12 | 0.67 | 3.78 | |
DST10ms | D11→L1 | DST10ms = (46.48, 56.06) | Loss = (0.0, 15.0) | 0.18 | 1.00 | 1.82 |
D12→L1 | DST10ms = (56.06, 60.04) | Loss = (0.0, 15.0) | 0.14 | 0.88 | 1.59 | |
D13→L1 | DST10ms = (60.04, 63.57) | Loss = (0.0, 15.0) | 0.14 | 0.78 | 1.42 | |
D14→L2 | DST10ms = (63.57, 67.17) | Loss = (15.0, 50.0) | 0.10 | 0.63 | 2.28 | |
D15→L2 | DST10ms = (67.17, 70.4) | Loss = (15.0, 50.0) | 0.12 | 0.75 | 2.73 | |
D16→L3 | DST10ms = (70.4, 72.71) | Loss = (50.0,100.0) | 0.14 | 0.78 | 4.41 |
Different concentrations of HPMC were added to the aqueous extract of Cistanches Herba (ECH). The DST values of ECH alone ranged from 71 ± 0.5 to 64.5 ± 0.5 mN/m over 2000 ms. However, the DST values of ECH containing 5~13% HPMC significantly decreased, and the loss rates were significantly reduced by adding HPMC (Figure 7(e)). Therefore, low surface activity was conducive to effective FBG, which was consistent with the results in Wang's report [42].
The conductivity was brought into the front itemset, and the loss rate was brought into the rear itemset. Table 3 depicted the association rules meeting the requirement. The rules from strong to weak were as follows. C6→L1 > C5→L1 > C2→L2 > C4→L1 > C1→L3 > C3→L1. Figure 7(d) showed that the loss rate increased with the decrease in conductivity.
Factor code | Front itemset | Rear itemset | Support | Confidence | Lift |
C1→L3 | Conductivity = (2.5, 6.21) | Loss = (50.0,100.0) | 0.10 | 0.56 | 3.15 |
C2→L2 | Conductivity = (6.21, 8.36) | Loss = (15.0, 50.0) | 0.10 | 0.63 | 2.28 |
C3→L1 | Conductivity = (8.36, 10.55) | Loss = (0.0, 15.0) | 0.10 | 0.56 | 1.01 |
C4→L1 | Conductivity = (10.55, 13.32) | Loss = (0.0, 15.0) | 0.10 | 0.63 | 1.14 |
C5→L1 | Conductivity = (13.32, 20.92) | Loss = (0.0, 15.0) | 0.12 | 0.75 | 1.37 |
C6→L1 | Conductivity = (20.92, 53.87) | Loss = (0.0, 15.0) | 0.18 | 1.00 | 1.82 |
The analysis of small molecular saccharides, including fructose, glucose, saccharose and maltose, revealed that fructose and glucose had a strong association with the loss rate. However, the saccharose and maltose contents had no significant effects. Fructose content was brought into the front itemset, and loss rate into the rear itemset. Table 4 and Figure 8 depicted association rules meeting the requirement. In terms of the fructose content, the rules from strong to weak were as follow: F1→L1 = F3→L1 > F5→L2 > F2→L1 > F6→L3 > F4→L1. The result showed that the loss rate increased with increased fructose content. When the fructose content was more than 20.35 mg/g, defluidization and sticky wall phenomenon occurred easily. Similarly, the loss rate greatly decreased when the fructose content was less than 20.35 mg/g. A fructose content of 20.35 mg/g might be a transition point impacting granulation feasibility similar to glucose, as shown in Figure 8(b).
Factors | Factor code | Front itemset | Rear itemset | Support | Confidence | Lift |
Fructose | F1→L1 | Fructose = (0.00, 6.86) | Loss = (0.0, 15.0) | 0.16 | 0.89 | 1.62 |
F2→L1 | Fructose = (6.86, 10.33) | Loss = (0.0, 15.0) | 0.14 | 0.88 | 1.59 | |
F3→L1 | Fructose = (10.33, 12.80) | Loss = (0.0, 15.0) | 0.16 | 0.89 | 1.62 | |
F4→L1 | Fructose = (12.80, 20.35) | Loss = (0.0, 15.0) | 0.10 | 0.63 | 1.14 | |
F5→L2 | Fructose = (20.35, 39.73) | Loss = (15.0, 50.0) | 0.14 | 0.88 | 3.19 | |
F6→L3 | Fructose = (39.73,104.93) | Loss = (50.0,100.0) | 0.14 | 0.78 | 4.41 | |
Glucose | G1→L1 | Glucose = (0.00, 2.42) | Loss = (0.0, 15.0) | 0.16 | 0.89 | 1.62 |
G2→L1 | Glucose = (2.42, 7.13) | Loss = (0.0, 15.0) | 0.12 | 0.75 | 1.37 | |
G3→L1 | Glucose = (7.13, 10.43) | Loss = (0.0, 15.0) | 0.14 | 0.78 | 1.42 | |
G4→L1 | Glucose = (10.43, 12.42) | Loss = (0.0, 15.0) | 0.12 | 0.75 | 1.37 | |
G5→L2 | Glucose = (12.42, 34.05) | Loss = (15.0, 50.0) | 0.10 | 0.63 | 2.28 | |
G6→L3 | Glucose = (34.05,117.57) | Loss = (50.0,100.0) | 0.14 | 0.78 | 4.41 |
The association rules for glucose were consistent with those for fructose (Figure 8(b)). Higher saccharides content easily led to defluidization or sticky wall phenomenon, significantly impacting the granulate process.
The protein content was brought into the front itemset, and the loss rate into the rear itemset. Table 5 illustrated the association rules meeting the requirement. The rules from strong to weak were as follows. P6→L1 > P4→L1 = P5→L1 > P1→L2 > P3→L1 > P2→L2. The loss rate decreased as the protein contents increased (Figure 8(c)).
Factor code | Front itemset | Rear itemset | Support | Confidence | Lift |
P1→L2 | Protein = (0.29, 1.63) | Loss = (15.0, 50.0) | 0.12 | 0.67 | 2.43 |
P2→L2 | Protein = (1.63, 2.93) | Loss = (15.0, 50.0) | 0.10 | 0.63 | 2.28 |
P3→L1 | Protein = (2.93, 3.66) | Loss = (0.0, 15.0) | 0.12 | 0.67 | 1.21 |
P4→L1 | Protein = (3.66, 5.84) | Loss = (0.0, 15.0) | 0.12 | 0.75 | 1.37 |
P5→L1 | Protein = (5.84, 8.06) | Loss = (0.0, 15.0) | 0.12 | 0.75 | 1.37 |
P6→L1 | Protein = (8.06, 12.01) | Loss = (0.0, 15.0) | 0.16 | 0.89 | 1.62 |
Regarding physical properties, association rules indicated that increasing Tg promotes a smooth granulation process. When the material temperature during granulation is 40 ± 2℃, there is a significant risk of defluidization and granulation failure if Tg is under 29.04℃. Similarly, when Tg ranges from 29.04 to 53.80℃, there is a great risk of forming larger aggregates or sticking to the wall, resulting in a higher loss rate. Previous research has shown that for a given rigid condition, temperatures for sticky conditions range from 20 to 30℃ above Tg attributed to the amorphous components [43]. One possible mechanism explanation for these results is that when the temperature exceeds the Tg of the amorphous material by more than 10℃, it transitions into a rubbery state with increased adhesion. It leads to the formation of rubbery bridges between particles and causing wet mass or collapse and over-granulation due to the formation of large agglomerates. Adding excipients with high glass transition temperature to materials with lower glass transition temperature can improve the yield and reduce the stickiness by increasing the Tg of the original liquid [44]. It is in agreement with the results obtained in this study. The DST was another critical parameter that affected FBG. The results showed that a lower DST was conducive to effective FBG. Cheng et al. [35] found that, by analyzing the DST of the feed solution and the surface chemical elements of the powder, the addition of HPMC can significantly reduce sticking and improve the yield due to its surface activity, reducing the DST and achieving an anti-sticking effect. It is consistent with the results of this study. Additionally, conductivity was another vital parameter affecting FBG. The results showed that the loss rate decreased with the decrease in conductivity. However, further investigation is required to understand the reason behind this phenomenon deeply.
In terms of chemical properties, the result showed that the loss rate increased with the increase in the contents of low molecular weight saccharides, especially fructose. A 20.35 mg/g fructose content might be critical to granulation feasibility. One possible mechanism explaining these results is attributed to the soluble and sticky characteristics of sugars. These properties lead to the formation of viscous liquid bridges, contributing to sticky behavior or a larger wet mass. Importantly, they adhere easily to the inner wall and nozzle, often resulting in over granulation. The same conclusion was obtained in Liu's study [45].
Additionally, the protein content was another important parameter. The results showed that higher protein contents were conducive to effective FBG. The mechanism of spray drying encapsulation technology might explain this result. High loss rates of feed solutions due to the low Tg or uncontrolled stickiness are severe problems with high contents of small molecule sugars, acids or phenolic compounds [46]. Thus, utilizing protein as the wall material enables the rapid formation of a glassy layer with a high Tg on the surface. This layer exhibits surfactant and film-forming agent characteristics, effectively preventing excessive adherence, reducing loss and minimizing hygroscopicity [47]. In addition to that, it also prevents the deterioration of phenolic compounds, extending shelf life and minimizing bitterness and astringency [48]. State-of-the-art research regarding plant proteins has shown their potential as natural ingredients due to their less allergenic structure and functionality.
In this study, 11 physical properties and 10 chemical properties were comprehensively determined. It was done utilizing the RF and association algorithms based on data mining to explore the relationship between physicochemical characteristics and the process feasibility of FBG. RF algorithm identified the top 8 important physicochemical parameters such as Tg, DST100ms, DST1000ms, DST10ms and conductivity. Furthermore, chemical properties such as fructose content, glucose content and protein content were also identified. The association algorithm revealed that Tg was the most critical factor affecting the feasibility of FBG among all physical parameters in this study. Moreover, for a bed temperature higher than 10℃ above the Tg, there was a high possibility of bed collapse or wall sticking. The higher the DST, the higher the loss rate, and DST10ms, DST100ms and DST1000ms showed a similar trend, while conductivity had an opposite trend. Regarding chemical compositions, low molecular weight saccharides and protein exhibited different trends. A higher content of the low molecular weight saccharides resulted in a greater impact on loss rate. In particular, a 20.35 mg/g content in feed solution might be a transition point impacting granulation feasibility, while protein contents showed an opposite trend.
Data mining is useful for investigating the association between physicochemical characteristics and the feasibility of FBG by uncovering hidden rules. Overall, based on the RF and Apriori algorithm approaches, the established association rules were beneficial for better understanding the process by controlling material properties and providing valuable guidance for improving FBG-based product development.
These phenomena depend on the combination of various physicochemical properties and process parameters. However, this work still has limitations, as only physicochemical properties were used as influencing factors. Further research should be carried out to determine more influential variables on the granule growth mechanism from a broader perspective and to comprehensively develop a control strategy for FBG. This part of the research is currently in progress.
The authors declare they have not used Artificial Intelligence (AI) tools in the creation of this article.
This research is supported by the Scientific and technological innovation project of China Academy of Chinese Medical Sciences (CI2021A04301), National Key Research and Development Program (2022YFC3501900) and Fundamental Research Funds for the Central Public Welfare Research Institutes (ZXKT21012, ZZ13-YQ-059). The author thanks co-authors for Software, Validation, Formal Analysis, Investigation and Visualization of the manuscript.
The authors declare there is no conflict of interest.
[1] |
S. M. Iveson, J. D. Litster, K. Hapgood, B. J. Ennis, Nucleation, growth and breakage phenomena in agitated wet granulation processes: A review, Powder Technol., 117 (2001), 3–39. https://doi.org/10.1016/S0032-5910(01)00313-8 doi: 10.1016/S0032-5910(01)00313-8
![]() |
[2] |
S. V. Moraga, M. P. Villa, D. E. Bertín, I. M. Cotabarren, J. Piña, M. Pedernera, et al., Fluidized-bed melt granulation: The effect of operating variables on process performance and granule properties, Powder Technol., 286 (2015), 654–667. https://doi.org/10.1016/j.powtec.2015.09.006 doi: 10.1016/j.powtec.2015.09.006
![]() |
[3] |
G. Strenzke, R. Dürr, A. Bück, E. Tsotsas, Influence of operating parameters on process behavior and product quality in continuous spray fluidized bed agglomeration, Powder Technol., 375 (2020), 210–220. https://doi.org/10.1016/j.powtec.2020.07.083 doi: 10.1016/j.powtec.2020.07.083
![]() |
[4] |
H. Aziz, S. N. Ahsan, G. De Simone, Y. J. Gao, B. Chaudhuri, Computational modeling of drying of pharmaceutical wet granules in a fluidized bed dryer using coupled CFD-DEM approach, AAPS PharmSciTech, 59 (2022). https://doi.org/10.1208/s12249-021-02180-x doi: 10.1208/s12249-021-02180-x
![]() |
[5] |
K. Vorlander, L. Bahlmann, A. Kwade, J. H. Finke, I. Kampen, Effect of process parameters, protectants and carrier materials on the survival of yeast cells during fluidized bed granulation for tableting, Pharmaceutics, 15 (2023), 884. https://doi.org/10.3390/pharmaceutics15030884 doi: 10.3390/pharmaceutics15030884
![]() |
[6] | ICH Harmonised Tripartite Guideline Pharmaceutical Development Q8 R2 Guideline; ICH: Geneva, Switzerland, 2009, 1–24. |
[7] |
F. Sun, B. Xu, S. Dai, Y. Zhang, Z. Lin, Y. Qiao, A novel framework to aid the development of design space across multi-unit operation pharmaceutical processes—A case study of panax notoginseng saponins immediate release tablet, Pharmaceutics, 11 (2019), 474. https://doi.org/10.3390/pharmaceutics11090474 doi: 10.3390/pharmaceutics11090474
![]() |
[8] |
L. S. Ming, Z. Li, F. Wu, R. F. Du, Y. Feng, A two-step approach for fluidized bed granulation in pharmaceutical processing: Assessing different models for design and control, PloS One, 12 (2017). https://doi.org/10.1371/journal.pone.0180209 doi: 10.1371/journal.pone.0180209
![]() |
[9] |
J. Krzywanski, D. Urbaniak, H. Otwinowski, T. Wylecial, M. Sosnowski, Fluidized bed jet milling process optimized for mass and particle size with a fuzzy logic approach, Materials, 13 (2020), 3303. https://doi.org/10.3390/ma13153303 doi: 10.3390/ma13153303
![]() |
[10] |
M. Sen, D. Barrasso, R. Singh, R. Ramachandran, A multi-scale hybrid CFD-DEM-PBM description of a fluid-bed granulation process, Processes, 2 (2014), 89–111. https://doi.org/10.3390/pr2010089 doi: 10.3390/pr2010089
![]() |
[11] |
H. Aoki, Y. Hattori, T. Sasaki, M. Otsuka, Comparative study on the real-time monitoring of a fluid bed drying process of extruded granules using near-infrared spectroscopy and audible acoustic emission, Int. J. Pharm., 619 (2022), 121689. https://doi.org/10.1016/j.ijpharm.2022.121689 doi: 10.1016/j.ijpharm.2022.121689
![]() |
[12] |
M. Nadda, S. K. Shah, S. Roy, A. Yadav, CFD-based deep neural networks (DNN) model for predicting the hydrodynamics of fluidized beds, Digit. Chem. Eng., 8 (2023), 100113. https://doi.org/10.1016/j.dche.2023.100113 doi: 10.1016/j.dche.2023.100113
![]() |
[13] |
A. Tamrakar, R. Ramachandran, CFD-DEM-PBM coupled model development and validation of a 3D top-spray fluidized bed wet granulation process, Chem. Eng. Sci., 125 (2019), 249–270. https://doi.org/10.1016/j.compchemeng.2019.01.023 doi: 10.1016/j.compchemeng.2019.01.023
![]() |
[14] |
S. V. Muddu, A. Tamrakar, P. Pandey, R. Ramachandran, Model development and validation of fluid bed wet granulation with dry binder addition using a population balance model methodology, Processes, 6 (2018), 154. https://doi.org/10.3390/pr6090154 doi: 10.3390/pr6090154
![]() |
[15] |
K. K. Win, W. Nowak, H. Matsuda, M. Hasatani, Z. Bis, J. Krzywanski, et al., Transport velocity of coarse particles in multi-solid fluidized bed, JCEJ, 28 (1995), 535–540. https://doi.org/10.1252/jcej.28.535 doi: 10.1252/jcej.28.535
![]() |
[16] |
J. Liu, T. Liu, G. Mu, J. Chen, Wavelet based calibration model building of NIR spectroscopy for in-situ measurement of granule moisture content during fluidized bed drying, Chem. Eng. Sci., 226 (2020), 115867. https://doi.org/10.1016/j.ces.2020.115867 doi: 10.1016/j.ces.2020.115867
![]() |
[17] |
R. F. Nascimento, M. F. Ávila, A. G. P. da Silva, O. P. Taranto, L. E. Kurozawa, The formation of solid bridges during agglomeration in a fluidized bed: Investigation by Raman spectroscopy and image analyses, Powder Technol., 420 (2023), 118377. https://doi.org/10.1016/j.powtec.2023.118377 doi: 10.1016/j.powtec.2023.118377
![]() |
[18] |
H. Otwinowski, J. Krzywanski, D. Urbaniak, T. Wylecial, M. Sosnowski, Comprehensive knowledge-driven ai system for air classification process, Materials, 15 (2021), 45. https://doi.org/10.3390/ma15010045 doi: 10.3390/ma15010045
![]() |
[19] |
V. Todaro, A. M, Healy Development and characterization of ibuprofen co-crystals granules prepared via fluidized bed granulation in a one-step process–A design of experiment approach, Drug Dev. Ind. Pharm., 47 (2021), 292–301. https://doi.org/10.1080/03639045.2021.1879836 doi: 10.1080/03639045.2021.1879836
![]() |
[20] |
K. Kramm, M. Orth, A. Teiwes, J. C. Kammerhofer, V. Meunier, S. Pietsch‐Braune, et al., Influence of nozzle parameters on spray pattern and droplet characteristics for a two‐fluid nozzle, Chem. Ing. Tech., 95 (2023), 151–159. https://doi.org/10.1002/cite.202200152 doi: 10.1002/cite.202200152
![]() |
[21] |
R. Xue, Y. Ruan, X. Liu, L. Chen, X. Zhang, Y. Hou, et al., Experimental study of liquid nitrogen spray characteristics in atmospheric environment, Appl. Therm. Eng., 142 (2018), 717–722. https://doi.org/10.1016/j.applthermaleng.2018.07.056 doi: 10.1016/j.applthermaleng.2018.07.056
![]() |
[22] |
P. D. Hede, P. Bach, A. D. Jensen Two-fluid spray atomisation and pneumatic nozzles for fluid bed coating/agglomeration purposes: A review, Chem. Eng. Sci., 63 (2008), 3821–3842. https://doi.org/10.1016/j.ces.2008.04.014 doi: 10.1016/j.ces.2008.04.014
![]() |
[23] | C. Vesvey, J. Cronlein, A. Breuer, S. Gerstner, Fluid bed nozzle spray characterization of an aqueous ethylcellulose dispersion for particle taste-masking applications, AAPS Poster Reprint, (2014), 3–6. |
[24] |
R. Zhang, T. Hoffmann, E. Tsotsas, Novel technique for coating of fine particles using fluidized bed and aerosol atomizer, Processes, 8 (2020), 1525. https://doi.org/10.3390/pr8121525 doi: 10.3390/pr8121525
![]() |
[25] |
M. Orth, P. Kieckhefen, S. Pietsch, S. Heinrich, Correlating granule surface structure morphology and process conditions in fluidized bed layering spray granulation, KONA Powder Part. J., 39 (2022), 230–239. https://doi.org/10.14356/kona.2022016 doi: 10.14356/kona.2022016
![]() |
[26] |
R. Maharjan, S. H. Jeong Application of different models to evaluate the key factors of fluidized bed layering granulation and their influence on granule characteristics, Powder Technol., 408 (2022), 117737. https://doi.org/10.1016/j.powtec.2022.117737 doi: 10.1016/j.powtec.2022.117737
![]() |
[27] |
B. Düsenberg, A. K. Singh, J. Schmidt, A. Bück, Spray agglomeration of polymer particles: Influence of spray parameters on shape factors, Powder Technol., 422 (2023), 118491. https://doi.org/10.1016/j.powtec.2023.118491 doi: 10.1016/j.powtec.2023.118491
![]() |
[28] |
J. Tang, D. Chen, R. Wang, Study on the bed collapse in the shaping process of Shenmai granules, Pharm. Care Res., 21 (2020), 23–25. https://doi.org/10.5428/pcar20210105 doi: 10.5428/pcar20210105
![]() |
[29] |
P. Stähle, V. Gaukel, H. P. Schuchmann, Influence of feed viscosity on the two-phase flow inside the exit orifice of an effervescent atomizer and on resulting spray characteristics, Food Res. Int., 77 (2015), 55–62. https://doi.org/10.1016/j.foodres.2015.04.047 doi: 10.1016/j.foodres.2015.04.047
![]() |
[30] |
I. M. Andersson, M. Glantz, M. Alexander, A. Millqvist-Fureby, M. Paulsson, B. Bergenståhl, Impact of surface properties on morphology of spray-dried milk serum protein/lactose systems, Int. Dairy J., 85 (2018), 86–95. https://doi.org/10.1016/j.idairyj.2018.04.011 doi: 10.1016/j.idairyj.2018.04.011
![]() |
[31] |
M. Hemati, R. Cherif, K. Saleh, V. Pont. Fluidized bed coating and granulation: influence of process-related variables and physicochemical properties on the growth kinetics, Powder Technol., 130 (2003), 18–34. https://doi.org/10.1016/S0032-5910(02)00221-8 doi: 10.1016/S0032-5910(02)00221-8
![]() |
[32] |
T. Y. Du, J. C. Xu, S. N. Zhu, X. J. Yao, J. Guo, W. Q. Lv, Effects of spray drying, freeze drying, and vacuum drying on physicochemical and nutritional properties of protein peptide powder from salted duck egg white, Front. Nutr., 9 (2022). https://doi.org/10.3389/fnut.2022.1026903 doi: 10.3389/fnut.2022.1026903
![]() |
[33] |
Q. L. Shi, Z. X. Fang, B. Bhandari, Effect of addition of whey protein isolate on spray-drying behavior of honey with maltodextrin as a carrier material, Dry. Technol., 31 (2013), 1681–1692. https://doi.org/10.1080/07373937.2013.783593 doi: 10.1080/07373937.2013.783593
![]() |
[34] |
A. C. Karaca, O. Guzel, M. M. Ak, Effects of processing conditions and formulation on spray drying of sour cherry juice concentrate, J. Sci. Food Agric., 96 (2016), 449–455. https://doi.org/10.1002/jsfa.7110 doi: 10.1002/jsfa.7110
![]() |
[35] |
H. Cheng, C. H. Lu, G. F. Xu, L. J. Zhao, M. Lu, Y. J. Wang, Optimization of spray-drying process parameters to study anti-sticking effect of hydroxypropyl methyl cellulose-VLV on corni fructus extracts, AAPS PharmSciTech, 23 (2022). https://doi.org/10.1208/s12249-022-02215-x doi: 10.1208/s12249-022-02215-x
![]() |
[36] |
J. Zeng, L. S. Ming, J. M. Wang, T. Huang, B. B. Liu, L. L. Feng, et al., Empirical prediction model-based process optimization for droplet size and spraying angle during pharmaceutical fluidized bed granulation, Pharm. Devt. Technol., 25 (2020), 720–728. https://doi.org/10.1080/10837450.2020.1738461 doi: 10.1080/10837450.2020.1738461
![]() |
[37] | H. Liu, Q. Zhang, J. Wang, J. Wu, H. Cui, Determination of six main components of organic acids in eight medicines by HPLC, J. Chin. Med. Mater., 40 (2017), 1130–1133. |
[38] | H. Qu, C. Feng, M. Xie, J. Zhu, Y. Sun, P. Cui, et al., Effect of different processing methods on the content of polygahatous polysaccharides, J. Hunan Univ. CM, 35 (2015), 53–55. |
[39] |
G. Shanmugasundar, M. Vanitha, R. Čep, V. Kumar, K. Kalita, M. Ramachandran, A comparative study of linear, random forest and adaboost regressions for modeling non-traditional machining, Processes, 9 (2021), 2015. https://doi.org/10.3390/pr9112015 doi: 10.3390/pr9112015
![]() |
[40] |
R. García-Carretero, R. Holgado-Cuadrado, Ó. Barquero-Pérez, Assessment of classification models and relevant features on nonalcoholic steatohepatitis using random forest, Entropy, 23 (2021), 763. https://doi.org/10.3390/e23060763 doi: 10.3390/e23060763
![]() |
[41] |
L. W. Li, Y. P. Wu, Y. P. Huang, B. Li, M. F. S.Miao, Z. Q. Deng, Optimized apriori algorithm for deformation response analysis of landslide hazards, Comput. Geosci., 170 (2023), 105261. https://doi.org/10.1016/j.cageo.2022.105261 doi: 10.1016/j.cageo.2022.105261
![]() |
[42] |
Y. J. Wang, Y. L. Xie, D. S. Xu, X. Lin, Y. Feng, Y. L. Hong, Hydroxypropyl methylcellulose reduces particle adhesion and improves recovery of herbal extracts during spray drying of Chinese herbal medicines, Dry. Technol., 32 (2014), 557–566. https://doi.org/10.1080/07373937.2013.843543 doi: 10.1080/07373937.2013.843543
![]() |
[43] |
C. Avilés-Avilés, E. Dumoulin, C. Turchiuli, Fluidised bed agglomeration of particles with different glass transition temperatures, Powder Technol., 270 (2015), 445–452. https://doi.org/10.1016/j.powtec.2014.03.026 doi: 10.1016/j.powtec.2014.03.026
![]() |
[44] |
M. B. Braga, S. C. D. Rocha, M. D. Hubinger, Spray-Drying of milk-blackberry pulp mixture: Effect of carrier agent on the physical properties of powder, water sorption, and glass transition temperature, J. Food Sci., 83 (2018), 1650–1659. https://doi.org/10.1111/1750-3841.14187 doi: 10.1111/1750-3841.14187
![]() |
[45] |
P. D. Liu, J. X. Li, C. H. Lu, L. J. Zhao, X. Lin, Y. J. Wang, et al., Data mining-based detection of the physical and chemical characteristics of Chinese medical herbs aqueous decoction in spray drying yield, Dry. Technol., 39 (2021), 896–904. https://doi.org/10.1080/07373937.2020.1728304 doi: 10.1080/07373937.2020.1728304
![]() |
[46] |
S. Jafari, S. M. Jafari, M. Ebrahimi, I. Kijpatanasilp, K. Assatarakul, A decade overview and prospect of spray drying encapsulation of bioactives from fruit products: Characterization, food application and in vitro gastrointestinal digestion, Food Hydrocoll, 134 (2023). https://doi.org/10.1016/j.foodhyd.2022.108068 doi: 10.1016/j.foodhyd.2022.108068
![]() |
[47] |
Z. X. Fang, B. Bhandari, Comparing the efficiency of protein and maltodextrin on spray drying of bayberry juice, Food Res. Int., 48(2012), 478–483. https://doi.org/10.1016/j.foodres.2012.05.025 doi: 10.1016/j.foodres.2012.05.025
![]() |
[48] |
R. T. Hoskin, M. H. Grace, J. Xiong, M. A. Lila, Spray-drying microencapsulation of blackcurrant and cocoa polyphenols using underexplored plant-based protein sources, J. Food Sci., 88 (2023), 2665–2678. https://doi.org/10.1111/1750-3841.16590 doi: 10.1111/1750-3841.16590
![]() |
1. | Ziyang Geng, Ting Zeng, Tao Wang, Likaiying Deng, 2024, Chapter 55, 978-981-97-5097-9, 802, 10.1007/978-981-97-5098-6_55 |
Factor code | Front itemset | Rear itemset | Support | Confidence | Lift |
T1→L3 | Tg = (11.42, 29.04) | Loss = (50.0,100.0) | 0.18 | 1.00 | 5.67 |
T2→L2 | Tg = (29.04, 53.80) | Loss = (15.0, 50.0) | 0.16 | 1.00 | 3.64 |
T3→L1 | Tg = (53.80, 88.39) | Loss = (0.0, 15.0) | 0.14 | 0.78 | 1.42 |
T4→L1 | Tg = (88.39, 94.46) | Loss = (0.0, 15.0) | 0.12 | 0.75 | 1.37 |
T5→L1 | Tg = (94.46, 99.06) | Loss = (0.0, 15.0) | 0.14 | 0.88 | 1.59 |
T6→L1 | Tg = (99.06,123.10) | Loss = (0.0, 15.0) | 0.16 | 0.89 | 1.62 |
Factors | Factor code | Front itemset | Rear itemset | Support | Confidence | Lift |
DST100ms | D21→L1 | DST100ms = (44.46, 53.16) | Loss = (0.0, 15.0) | 0.18 | 1.00 | 1.82 |
D22→L1 | DST100ms = (53.16, 54.90) | Loss = (0.0, 15.0) | 0.14 | 0.88 | 1.59 | |
D23→L1 | DST100ms = (54.90, 59.27) | Loss = (0.0, 15.0) | 0.14 | 0.78 | 1.42 | |
D24→L2 | DST100ms = (59.27, 62.95) | Loss = (15.0, 50.0) | 0.10 | 0.63 | 2.28 | |
D25→L2 | DST100ms = (62.95, 66.66) | Loss = (15.0, 50.0) | 0.12 | 0.75 | 2.73 | |
D26→L3 | DST100ms = (66.66, 70.16) | Loss = (50.0,100.0) | 0.16 | 0.89 | 5.04 | |
DST1000ms | D31→L1 | DST1000ms = (42.83, 49.51) | Loss = (0.0, 15.0) | 0.16 | 0.89 | 1.62 |
D32→L1 | DST1000ms = (49.51, 52.48) | Loss = (0.0, 15.0) | 0.16 | 1.00 | 1.82 | |
D33→L1 | DST1000ms = (52.48, 54.57) | Loss = (0.0, 15.0) | 0.16 | 0.89 | 1.62 | |
D34→L2 | DST1000ms = (54.57, 59.47) | Loss = (15.0, 50.0) | 0.10 | 0.63 | 2.28 | |
D35→L2 | DST1000ms = (59.47, 61.58) | Loss = (15.0, 50.0) | 0.10 | 0.63 | 2.28 | |
D36→L3 | DST1000ms = (61.58, 69.25) | Loss = (50.0,100.0) | 0.12 | 0.67 | 3.78 | |
DST10ms | D11→L1 | DST10ms = (46.48, 56.06) | Loss = (0.0, 15.0) | 0.18 | 1.00 | 1.82 |
D12→L1 | DST10ms = (56.06, 60.04) | Loss = (0.0, 15.0) | 0.14 | 0.88 | 1.59 | |
D13→L1 | DST10ms = (60.04, 63.57) | Loss = (0.0, 15.0) | 0.14 | 0.78 | 1.42 | |
D14→L2 | DST10ms = (63.57, 67.17) | Loss = (15.0, 50.0) | 0.10 | 0.63 | 2.28 | |
D15→L2 | DST10ms = (67.17, 70.4) | Loss = (15.0, 50.0) | 0.12 | 0.75 | 2.73 | |
D16→L3 | DST10ms = (70.4, 72.71) | Loss = (50.0,100.0) | 0.14 | 0.78 | 4.41 |
Factor code | Front itemset | Rear itemset | Support | Confidence | Lift |
C1→L3 | Conductivity = (2.5, 6.21) | Loss = (50.0,100.0) | 0.10 | 0.56 | 3.15 |
C2→L2 | Conductivity = (6.21, 8.36) | Loss = (15.0, 50.0) | 0.10 | 0.63 | 2.28 |
C3→L1 | Conductivity = (8.36, 10.55) | Loss = (0.0, 15.0) | 0.10 | 0.56 | 1.01 |
C4→L1 | Conductivity = (10.55, 13.32) | Loss = (0.0, 15.0) | 0.10 | 0.63 | 1.14 |
C5→L1 | Conductivity = (13.32, 20.92) | Loss = (0.0, 15.0) | 0.12 | 0.75 | 1.37 |
C6→L1 | Conductivity = (20.92, 53.87) | Loss = (0.0, 15.0) | 0.18 | 1.00 | 1.82 |
Factors | Factor code | Front itemset | Rear itemset | Support | Confidence | Lift |
Fructose | F1→L1 | Fructose = (0.00, 6.86) | Loss = (0.0, 15.0) | 0.16 | 0.89 | 1.62 |
F2→L1 | Fructose = (6.86, 10.33) | Loss = (0.0, 15.0) | 0.14 | 0.88 | 1.59 | |
F3→L1 | Fructose = (10.33, 12.80) | Loss = (0.0, 15.0) | 0.16 | 0.89 | 1.62 | |
F4→L1 | Fructose = (12.80, 20.35) | Loss = (0.0, 15.0) | 0.10 | 0.63 | 1.14 | |
F5→L2 | Fructose = (20.35, 39.73) | Loss = (15.0, 50.0) | 0.14 | 0.88 | 3.19 | |
F6→L3 | Fructose = (39.73,104.93) | Loss = (50.0,100.0) | 0.14 | 0.78 | 4.41 | |
Glucose | G1→L1 | Glucose = (0.00, 2.42) | Loss = (0.0, 15.0) | 0.16 | 0.89 | 1.62 |
G2→L1 | Glucose = (2.42, 7.13) | Loss = (0.0, 15.0) | 0.12 | 0.75 | 1.37 | |
G3→L1 | Glucose = (7.13, 10.43) | Loss = (0.0, 15.0) | 0.14 | 0.78 | 1.42 | |
G4→L1 | Glucose = (10.43, 12.42) | Loss = (0.0, 15.0) | 0.12 | 0.75 | 1.37 | |
G5→L2 | Glucose = (12.42, 34.05) | Loss = (15.0, 50.0) | 0.10 | 0.63 | 2.28 | |
G6→L3 | Glucose = (34.05,117.57) | Loss = (50.0,100.0) | 0.14 | 0.78 | 4.41 |
Factor code | Front itemset | Rear itemset | Support | Confidence | Lift |
P1→L2 | Protein = (0.29, 1.63) | Loss = (15.0, 50.0) | 0.12 | 0.67 | 2.43 |
P2→L2 | Protein = (1.63, 2.93) | Loss = (15.0, 50.0) | 0.10 | 0.63 | 2.28 |
P3→L1 | Protein = (2.93, 3.66) | Loss = (0.0, 15.0) | 0.12 | 0.67 | 1.21 |
P4→L1 | Protein = (3.66, 5.84) | Loss = (0.0, 15.0) | 0.12 | 0.75 | 1.37 |
P5→L1 | Protein = (5.84, 8.06) | Loss = (0.0, 15.0) | 0.12 | 0.75 | 1.37 |
P6→L1 | Protein = (8.06, 12.01) | Loss = (0.0, 15.0) | 0.16 | 0.89 | 1.62 |
Factor code | Front itemset | Rear itemset | Support | Confidence | Lift |
T1→L3 | Tg = (11.42, 29.04) | Loss = (50.0,100.0) | 0.18 | 1.00 | 5.67 |
T2→L2 | Tg = (29.04, 53.80) | Loss = (15.0, 50.0) | 0.16 | 1.00 | 3.64 |
T3→L1 | Tg = (53.80, 88.39) | Loss = (0.0, 15.0) | 0.14 | 0.78 | 1.42 |
T4→L1 | Tg = (88.39, 94.46) | Loss = (0.0, 15.0) | 0.12 | 0.75 | 1.37 |
T5→L1 | Tg = (94.46, 99.06) | Loss = (0.0, 15.0) | 0.14 | 0.88 | 1.59 |
T6→L1 | Tg = (99.06,123.10) | Loss = (0.0, 15.0) | 0.16 | 0.89 | 1.62 |
Factors | Factor code | Front itemset | Rear itemset | Support | Confidence | Lift |
DST100ms | D21→L1 | DST100ms = (44.46, 53.16) | Loss = (0.0, 15.0) | 0.18 | 1.00 | 1.82 |
D22→L1 | DST100ms = (53.16, 54.90) | Loss = (0.0, 15.0) | 0.14 | 0.88 | 1.59 | |
D23→L1 | DST100ms = (54.90, 59.27) | Loss = (0.0, 15.0) | 0.14 | 0.78 | 1.42 | |
D24→L2 | DST100ms = (59.27, 62.95) | Loss = (15.0, 50.0) | 0.10 | 0.63 | 2.28 | |
D25→L2 | DST100ms = (62.95, 66.66) | Loss = (15.0, 50.0) | 0.12 | 0.75 | 2.73 | |
D26→L3 | DST100ms = (66.66, 70.16) | Loss = (50.0,100.0) | 0.16 | 0.89 | 5.04 | |
DST1000ms | D31→L1 | DST1000ms = (42.83, 49.51) | Loss = (0.0, 15.0) | 0.16 | 0.89 | 1.62 |
D32→L1 | DST1000ms = (49.51, 52.48) | Loss = (0.0, 15.0) | 0.16 | 1.00 | 1.82 | |
D33→L1 | DST1000ms = (52.48, 54.57) | Loss = (0.0, 15.0) | 0.16 | 0.89 | 1.62 | |
D34→L2 | DST1000ms = (54.57, 59.47) | Loss = (15.0, 50.0) | 0.10 | 0.63 | 2.28 | |
D35→L2 | DST1000ms = (59.47, 61.58) | Loss = (15.0, 50.0) | 0.10 | 0.63 | 2.28 | |
D36→L3 | DST1000ms = (61.58, 69.25) | Loss = (50.0,100.0) | 0.12 | 0.67 | 3.78 | |
DST10ms | D11→L1 | DST10ms = (46.48, 56.06) | Loss = (0.0, 15.0) | 0.18 | 1.00 | 1.82 |
D12→L1 | DST10ms = (56.06, 60.04) | Loss = (0.0, 15.0) | 0.14 | 0.88 | 1.59 | |
D13→L1 | DST10ms = (60.04, 63.57) | Loss = (0.0, 15.0) | 0.14 | 0.78 | 1.42 | |
D14→L2 | DST10ms = (63.57, 67.17) | Loss = (15.0, 50.0) | 0.10 | 0.63 | 2.28 | |
D15→L2 | DST10ms = (67.17, 70.4) | Loss = (15.0, 50.0) | 0.12 | 0.75 | 2.73 | |
D16→L3 | DST10ms = (70.4, 72.71) | Loss = (50.0,100.0) | 0.14 | 0.78 | 4.41 |
Factor code | Front itemset | Rear itemset | Support | Confidence | Lift |
C1→L3 | Conductivity = (2.5, 6.21) | Loss = (50.0,100.0) | 0.10 | 0.56 | 3.15 |
C2→L2 | Conductivity = (6.21, 8.36) | Loss = (15.0, 50.0) | 0.10 | 0.63 | 2.28 |
C3→L1 | Conductivity = (8.36, 10.55) | Loss = (0.0, 15.0) | 0.10 | 0.56 | 1.01 |
C4→L1 | Conductivity = (10.55, 13.32) | Loss = (0.0, 15.0) | 0.10 | 0.63 | 1.14 |
C5→L1 | Conductivity = (13.32, 20.92) | Loss = (0.0, 15.0) | 0.12 | 0.75 | 1.37 |
C6→L1 | Conductivity = (20.92, 53.87) | Loss = (0.0, 15.0) | 0.18 | 1.00 | 1.82 |
Factors | Factor code | Front itemset | Rear itemset | Support | Confidence | Lift |
Fructose | F1→L1 | Fructose = (0.00, 6.86) | Loss = (0.0, 15.0) | 0.16 | 0.89 | 1.62 |
F2→L1 | Fructose = (6.86, 10.33) | Loss = (0.0, 15.0) | 0.14 | 0.88 | 1.59 | |
F3→L1 | Fructose = (10.33, 12.80) | Loss = (0.0, 15.0) | 0.16 | 0.89 | 1.62 | |
F4→L1 | Fructose = (12.80, 20.35) | Loss = (0.0, 15.0) | 0.10 | 0.63 | 1.14 | |
F5→L2 | Fructose = (20.35, 39.73) | Loss = (15.0, 50.0) | 0.14 | 0.88 | 3.19 | |
F6→L3 | Fructose = (39.73,104.93) | Loss = (50.0,100.0) | 0.14 | 0.78 | 4.41 | |
Glucose | G1→L1 | Glucose = (0.00, 2.42) | Loss = (0.0, 15.0) | 0.16 | 0.89 | 1.62 |
G2→L1 | Glucose = (2.42, 7.13) | Loss = (0.0, 15.0) | 0.12 | 0.75 | 1.37 | |
G3→L1 | Glucose = (7.13, 10.43) | Loss = (0.0, 15.0) | 0.14 | 0.78 | 1.42 | |
G4→L1 | Glucose = (10.43, 12.42) | Loss = (0.0, 15.0) | 0.12 | 0.75 | 1.37 | |
G5→L2 | Glucose = (12.42, 34.05) | Loss = (15.0, 50.0) | 0.10 | 0.63 | 2.28 | |
G6→L3 | Glucose = (34.05,117.57) | Loss = (50.0,100.0) | 0.14 | 0.78 | 4.41 |
Factor code | Front itemset | Rear itemset | Support | Confidence | Lift |
P1→L2 | Protein = (0.29, 1.63) | Loss = (15.0, 50.0) | 0.12 | 0.67 | 2.43 |
P2→L2 | Protein = (1.63, 2.93) | Loss = (15.0, 50.0) | 0.10 | 0.63 | 2.28 |
P3→L1 | Protein = (2.93, 3.66) | Loss = (0.0, 15.0) | 0.12 | 0.67 | 1.21 |
P4→L1 | Protein = (3.66, 5.84) | Loss = (0.0, 15.0) | 0.12 | 0.75 | 1.37 |
P5→L1 | Protein = (5.84, 8.06) | Loss = (0.0, 15.0) | 0.12 | 0.75 | 1.37 |
P6→L1 | Protein = (8.06, 12.01) | Loss = (0.0, 15.0) | 0.16 | 0.89 | 1.62 |