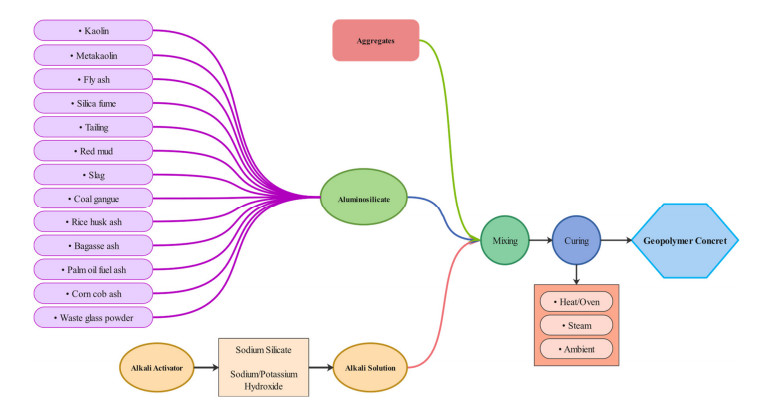
Mixing is the basis of stable and efficient combustion in air-breathing power systems, and it is also an important problem in fluid mechanics, which has been extensively studied from various perspectives. The purpose of this review is to investigate mixing mechanisms based on two commonly-used mixing indicators, namely ci (c refers to concentration, and i is either 1 or 2, indicating first- or second-order statistics), with a focus on passive-scalar (PS) and variable-density (VD) mixing. For PS mixing, the flow is not influenced by the mixing process. By using first-order statistics with concentration as the core, the PS mixing mechanisms on lamella structures can be described as stretching enhancing diffusion and promoting mixing. On the other hand, second-order statistics represented by the scalar dissipation rate can investigate mixing mechanisms on specific type of flow structures described by the invariants of velocity gradient tensors and the rotation of principal strain axis. As such, it has been found that strain-dominated flow structures can promote mixing, while rotation-dominated flow structures hinder it. For VD mixing, it has two distinct characteristics: flow changes due to baroclinic vorticity, and the inherent velocity divergence alters the mixing indicators. Studies using first-order statistics center on the mixing time in different types of VD flows, leading to the discovery of new phenomena. For instance, the second baroclinic vorticity can promote stretching in shock bubble interactions. Studies on second-order statistics for VD mixing have defined several mixing indicators from the component-transport equation, which have been utilized in phenomenological studies on VD mixing. This review aims to provide an overview of mixing phenomena, mixing indicators, and mixing mechanisms, and proposes research directions for understanding the mixing characteristics, flow structures, and their relationship with specific combustion phenomena particularly by second-order statistics.
Citation: Xu Han, Bin Yu, Hong Liu. Mixing mechanisms in the view of mixing indicators: from passive-scalar mixing to variable-density mixing[J]. Metascience in Aerospace, 2024, 1(1): 1-37. doi: 10.3934/mina.2024001
[1] | Sakthieswaran Natarajan, Shiny Brintha Gnanadurai . An approach to study the inter-relationship between mechanical and durability properties of ternary blended cement concrete using linear regression analysis. Mathematical Biosciences and Engineering, 2019, 16(5): 3734-3752. doi: 10.3934/mbe.2019185 |
[2] | Yu Jin, Zhe Ren, Wenjie Wang, Yulei Zhang, Liang Zhou, Xufeng Yao, Tao Wu . Classification of Alzheimer's disease using robust TabNet neural networks on genetic data. Mathematical Biosciences and Engineering, 2023, 20(5): 8358-8374. doi: 10.3934/mbe.2023366 |
[3] | Jie Bai, Heru Xue, Xinhua Jiang, Yanqing Zhou . Recognition of bovine milk somatic cells based on multi-feature extraction and a GBDT-AdaBoost fusion model. Mathematical Biosciences and Engineering, 2022, 19(6): 5850-5866. doi: 10.3934/mbe.2022274 |
[4] | Yunyun Liang, Shengli Zhang, Huijuan Qiao, Yinan Cheng . iEnhancer-MFGBDT: Identifying enhancers and their strength by fusing multiple features and gradient boosting decision tree. Mathematical Biosciences and Engineering, 2021, 18(6): 8797-8814. doi: 10.3934/mbe.2021434 |
[5] | Dan Zhu, Liru Yang, Xin Liang . Gender classification in classical fiction: A computational analysis of 1113 fictions. Mathematical Biosciences and Engineering, 2022, 19(9): 8892-8907. doi: 10.3934/mbe.2022412 |
[6] | Jian-xue Tian, Jue Zhang . Breast cancer diagnosis using feature extraction and boosted C5.0 decision tree algorithm with penalty factor. Mathematical Biosciences and Engineering, 2022, 19(3): 2193-2205. doi: 10.3934/mbe.2022102 |
[7] | Jian Cao, Tao Liu, Ziyang Han, Bin Tu . Sulfate ions diffusion in concrete under coupled effect of compression load and dry-wet circulation. Mathematical Biosciences and Engineering, 2023, 20(6): 9965-9991. doi: 10.3934/mbe.2023437 |
[8] | Hanyu Zhao, Chao Che, Bo Jin, Xiaopeng Wei . A viral protein identifying framework based on temporal convolutional network. Mathematical Biosciences and Engineering, 2019, 16(3): 1709-1717. doi: 10.3934/mbe.2019081 |
[9] | Zhihao Zhang, Ting Zeng, Yijia Wang, Yinxia Su, Xianghua Tian, Guoxiang Ma, Zemin Luan, Fengjun Li . Prediction Model of hospitalization time of COVID-19 patients based on Gradient Boosted Regression Trees. Mathematical Biosciences and Engineering, 2023, 20(6): 10444-10458. doi: 10.3934/mbe.2023459 |
[10] | Lili Jiang, Sirong Chen, Yuanhui Wu, Da Zhou, Lihua Duan . Prediction of coronary heart disease in gout patients using machine learning models. Mathematical Biosciences and Engineering, 2023, 20(3): 4574-4591. doi: 10.3934/mbe.2023212 |
Mixing is the basis of stable and efficient combustion in air-breathing power systems, and it is also an important problem in fluid mechanics, which has been extensively studied from various perspectives. The purpose of this review is to investigate mixing mechanisms based on two commonly-used mixing indicators, namely ci (c refers to concentration, and i is either 1 or 2, indicating first- or second-order statistics), with a focus on passive-scalar (PS) and variable-density (VD) mixing. For PS mixing, the flow is not influenced by the mixing process. By using first-order statistics with concentration as the core, the PS mixing mechanisms on lamella structures can be described as stretching enhancing diffusion and promoting mixing. On the other hand, second-order statistics represented by the scalar dissipation rate can investigate mixing mechanisms on specific type of flow structures described by the invariants of velocity gradient tensors and the rotation of principal strain axis. As such, it has been found that strain-dominated flow structures can promote mixing, while rotation-dominated flow structures hinder it. For VD mixing, it has two distinct characteristics: flow changes due to baroclinic vorticity, and the inherent velocity divergence alters the mixing indicators. Studies using first-order statistics center on the mixing time in different types of VD flows, leading to the discovery of new phenomena. For instance, the second baroclinic vorticity can promote stretching in shock bubble interactions. Studies on second-order statistics for VD mixing have defined several mixing indicators from the component-transport equation, which have been utilized in phenomenological studies on VD mixing. This review aims to provide an overview of mixing phenomena, mixing indicators, and mixing mechanisms, and proposes research directions for understanding the mixing characteristics, flow structures, and their relationship with specific combustion phenomena particularly by second-order statistics.
Cement concretes are the construction component that is employed on a global scale with the highest rate [1,2]. Ordinary Portland cement is one of the most common forms of binding agent used in cement concretes. Additional kinds of aggregate, water, and binding agents are other components of cement concretes. After aluminum and steel, oxidized polycyclic aromatic hydrocarbon is regarded as the third most incredibly energy-demanding chemical in the world. Ordinary Portland cement is responsible for seven percent of the overall energy that is needed by industries [3]. Unfortunately, the manufacturing of ordinary Portland cement results in the emission of enormous quantities of greenhouse gases like carbon dioxide, which has a significant role in the progression of global warming [4,5,6]. It is anticipated that the production of ordinary Portland cement would result in the release of 1,400,000,000 tons of greenhouse gases on a yearly basis [7,8]. Because of this, scientists have focused their attention on finding ways to lessen the amount of ordinary Portland cement that is used thanks to the development of alternative binders. There is some evidence that suggests that alkali-activated components, including geo-polymers, are preferred to cement concrete [9,10,11,12]. The reaction of precursor and activator results in the formation of alkali-activated compounds. In accordance with the amount of calcium present in the products of the chemical process, these were divided into two categories: 1) those that are high in calcium and have a Ca/(Si + Al) proportion that is higher than one (geo-polymers) and 2) those that are weak in calcium [13].
A geo-polymer is an innovative kind of binder produced for the production of concretes instead of ordinary Portland cement [13,14,15,16,17]. This was performed in order to improve the efficiency of production. The objective is to develop construction supplies that are sustainable-based, eco-friendly, and do not contain ordinary Portland cement. There is a significant increase in the number of distinct kinds of waste products that are being produced and deposited in landfills as a direct result of the ongoing expansion of industry and population. Rice husk ash, waste glass powder, ground granulated glass furnace slag, silica fume, fly ash, etc., are included in this category of wastes. It is detrimental to the environment to dispose of the mentioned wastes in landfills since they contribute to contamination in the environment [18,19]. Because geo-polymer concrete (GPoC) requires basic components with higher aluminum silicate concentrations present in waste materials, recycling these types of materials to produce GPoC would reduce the volume of pollutants that are released into the atmosphere [20,21]. Figure 1 provides a visual representation of the GPoC manufacturing procedure, in which a variety of different kinds of components and curing regimens are shown to be utilized during the making of GPoC. As can be seen in Figure 2, the utilization of such kinds of waste materials will be beneficial to both the natural environment and the economic system. This is because these materials are abundant and the need for reasonably priced housing is expected to increase in tandem with the growth of populations [8,22,23,24]. In general, the use of GPoC for studies is becoming more common, and it has the potential to overtake other environmentally friendly construction materials [25,26]. Despite this, GPoC has an opportunity to produce a substantial contribution to the continued existence of cement concrete technologies as well as the construction sector in the years to come.
Recent advancements in artificial intelligence (AI) have provided an explanation for the widespread use of artificial intelligence techniques for anticipating the properties of a variety of materials in civil engineering [29,30,31,32,33,34,35]. Also, varying AI techniques have been employed to predict the mechanical properties of engineering materials [36,37,38,39,40,41]. In a study that was conducted by Huang et al. [42], a comparison was conducted between three different artificial intelligence techniques known as decision trees (DT), AdaBoost, and bagging regressor in order to predict the compressive strength of GPoC (CSGPoC) that included fly ash materials. In comparison to the other systems examined, it proved that the bagging regressor approach showed the highest level of accuracy. In a separate study conducted by Ahmad et al. [43], artificial neural networks and gene expression programming (GEP) models were used to generate an estimate of the compressive strength of concretes that included recycled aggregates. In the study, the GEP model provided a more accurate forecast than the artificial neural network. A study conducted by Song et al. [44] used an artificial neural network approach to explore the compressive strength of concretes including waste materials, and they were able to correctly anticipate the needed conclusion. According to the findings of the study, it is possible to effectively use machine learning methods to anticipate any kind of mechanical feature that is associated with concretes. The tensile and compressive strengths of concretes with high performance were predicted using a number of artificial intelligence methods, as detailed by Nguyen et al. [45]. They concluded that the approaches of combined artificial intelligence were more accurate than the methods of artificial intelligence used in standalone form. This is due to the fact that the various machine learning techniques, in order to produce a more accurate model, frequently utilize the abilities of weak learners including decision trees and multi-layer perception neural networks. Therefore, several researchers have documented different artificial intelligence systems that have better degrees of precision in their evaluation of the attributes of materials. In light of this, it is absolutely necessary to carry out more in-depth research in order to shed light on this particular issue. Some literature models for predicting different characteristics of concretes are reported in Table 1.
Author | Year | Technique | Number of data |
Huang et al. [46] | 2021 | SVM | 114 |
Sarir et al. [47] | 2019 | GEP | 303 |
Balf et al. [48] | 2021 | DEA | 114 |
Ahmad et al. [49] | 2021 | GEP, ANN, DT | 642 |
Azimi-Pour et al. [50] | 2020 | SVM | - |
Saha et al. [51] | 2020 | SVM | 115 |
Hahmansouri et al. [52] | 2020 | GEP | 351 |
Hahmansouri et al. [53] | 2019 | GEP | 54 |
Aslam et al. [54] | 2020 | GEP | 357 |
Farooq et al. [55] | 2020 | RF and GEP | 357 |
Asteris and Kolovos [56] | 2019 | ANN | 205 |
Selvaraj and Sivaraman [57] | 2019 | IREMSVM-FR with RSM | 114 |
Zhang et al. [58] | 2019 | RF | 131 |
Kaveh et al. [59] | 2018 | M5MARS | 114 |
Sathyan et al. [60] | 2018 | RKSA | 40 |
Vakhshouri and Nejadi [61] | 2018 | ANFIS | 55 |
Belalia Douma et al. [62] | 2017 | ANN | 114 |
Abu Yaman et al. [63] | 2017 | ANN | 69 |
Ahmad et al. [64] | 2021 | GEP, DT and Bagging | 270 |
Farooq et al. [65] | 2021 | ANN, bagging and boosting | 1030 |
Bušić et al. [66] | 2020 | MV | 21 |
Javad et al. [67] | 2020 | GEP | 277 |
Nematzadeh et al. [68] | 2020 | RSM, GEP | 108 |
Güçlüer et al. [69] | 2021 | ANN, SVM, DT | 100 |
Ahmad et al. [70] | 2021 | ANN, DT, GB | 207 |
Asteris et al. [71] | 2021 | ANN, GPR, MARS | 1030 |
Emad et al. [72] | 2022 | ANN, M5P, | 306 |
Shen et al. [73] | 2022 | XGBoost, AdaBoost, and Bagging | 372 |
Kuma et al. [74] | 2022 | GPR, SVMR | 194 |
Jaf et al. [75] | 2023 | NLR, MLR, ANN | 236 |
Mahmood et al. [76] | 2023 | NLR, M5P, ANN | 280 |
Ali et al. [77] | 2023 | LR, MLR, NLR, PQ, IA, FQ | 420 |
SVM: Support vector machine; GEP: Gene expression programming; ANN: Artificial neural network; DT: Decision tree; RF: Random Fores; DEA: Data envelopment analysis; RSM: Response surface methodology; ANFIS: Adaptive neuro fuzzy inference system; MV: Micali-Vazirani algorithm; RKSA: Retina key scheduling algorithm; GB: gradient boosting; GPR: Gaussian Process Regression; MARS: Multivariate Adaptive Regression Splines; SVMR: Support Vector Machine Regression; NLR: Nonlinear regression; MLR: Multi-linear regression; LR: linear regression; PQ: pure quadratic; IA: interaction; FQ: full quadratic. |
This research differs from experimentation-based research in that it examines the CSGPoC using both base models of artificial intelligence methods as well as their ensemble form for predicting the CSGPoC. Experiment-based studies require considerable quantities of personal effort in addition to costly and lengthy experiments. By tackling the aforementioned challenges, using advanced technology such as artificial intelligence will help the building industry [13,78,79]. It is challenging to determine how several factors, such as precursor materials, activator solution, aggregates amount, and others, affect the strength of GPoC utilizing experimental procedures. Machine learning approaches may quickly and easily determine the combined impact of its constituent parts. Given that numerous studies have been accomplished to ascertain the determination of CSGPoC, machine learning models need a dataset, which could have been acquired from previous studies. Following data gathering, machine learning models can be trained to predict material attributes. Recent research has used machine learning techniques with a constrained set of effective parameters and databases to determine the intensity of GPoC. For instance, Dao et al.'s [80] use of machine learning approaches to forecast the CSGPoC employing three inputs and 210 data rows. Similar to this research, [81] employed 210 data rows and 4 inputs. In order to examine the effectiveness of various machine learning approaches used to anticipate the CSGPoC, the current study used nine effective parameters on CSGPoC and 295 data points based on literature review. The results of this study are also contrasted with those of related earlier investigations. The superior accuracy of machine learning approaches is anticipated to come from employing more input parameters and data points. The main goal of the present work is to identify the best machine learning method for calculating the CSGPoC using anticipated results and the impact of different parameters on GPoC strength. The computational flowchart of the study is depicted in Figure 3.
The composition of the various alkali excitation components and solid waste from the industry that are used to make gels are the basic components, and the amount of these initial components used in the production of gels have an effect on the efficiency of the gels [24,79,82,83]. It is necessary to have adequate Na+ and OH in order to finish every step of the polymerization of gels, and the amount of both of these ions has a direct bearing on the amount of force that can be exerted by the gels [84]. In light of the two aforementioned explanations, as well as the impact that the properties and ratios of the initial materials have on the compressive strength of concretes, the authors of the present study came to the conclusion that GGBS, sodium silicate, fly-ash, gravel stones (4–10 mm to 10–20 mm), water/solids proportion, sodium hydroxide, sodium hydroxide molarity, and fine aggregates are the effective parameters to determine and predict the CSGPoC to complete a dataset comprising 295 data points. The required CSGPoC data of developing models is gathered from a study conducted by Yong et al. [28]. This data includes nine parameters as inputs and CSGPoC as the output of the models. Statistical analysis of the inputs and output is reported in Table 2. Furthermore, a better view for variations of inputs as well as CSGPoC in 295 various points are demonstrated in Figure 4.
Parameter | Symbol | Unit | Median | Min | Mean | Max | StD | |
Inputs | Fly ash | FA | kg/m3 | 170 | 0 | 178.265 | 523 | 173.979 |
GGBS | GGBS | kg/m3 | 225 | 0 | 209.831 | 450 | 163.271 | |
Na2SiO3 | Na2SiO3 | kg/m3 | 100 | 18 | 104.059 | 342 | 44.9000 | |
NaOH | NaOH | kg/m3 | 64 | 6.300 | 60.042 | 147 | 30.391 | |
Fine aggregate | FAg | kg/m3 | 721 | 459 | 731.209 | 1360 | 138.078 | |
Gravel 4–10 mm | Gravel 4–10 | kg/m3 | 309 | 0 | 335.828 | 1293.400 | 373.884 | |
Gravel 10–20 mm | Gravel 10–20 | kg/m3 | 815 | 0 | 741.556 | 1298 | 361.336 | |
Water/solids ratio | WS | N/A | 0.330 | 0.120 | 0.330 | 0.630 | 0.095 | |
NaOH molarity | NaOH molarity | N/A | 10 | 1 | 8.193 | 20 | 4.596 | |
Output | Compressive strength of geo-polymer concrete | CSGPoC | MPa | 43 | 10 | 44.474 | 86.080 | 18.010 |
Before model developing, the correlation coefficient between parameters should be evaluated [13,85,86,87]. If the correlation between two parameters is high, the multicollinearity problem appears in the model. Therefore, the Spearman correlation coefficient between CSGPoC and effective parameters is calculated as shown in Figure 5. This figure is a heatmap of Spearman correlation coefficient that can be determined by following equation [88,89,90,91]:
r=n∑i=1(xi−xm)(yi−ym)√n∑i=1(xi−xm)2×n∑i=1(yi−ym)2 | (1) |
where n, xm, and ym stand for the number of datasets, average value across all x data, and the average value across all y data, respectively. When the r value is r > 0, r = 0, r ≃ 1, r < 0, or r ≃ -1, then there is positive linear correlation, no correlation, stronger positive linear correlation, negative linear correlation, or stronger negative linear correlation, respectively [8,87,92]. From Figure 5, there exist medium negative and positive linear correlations between CSGPoC and FA and GGBS at -0.43 and 0.46, respectively. Moreover, the correlation between CSGPoC and NaoH, Gravel 4–10 mm, and NaOH molarity is a weak negative linear correlation with r equal to -0.22, -0.27, and -0.12, respectively, and the correlation between CSGPoC and other parameters is a weak positive linear correlation. Based on these results, the investigation of developed models impact will not be influenced by the occurrence of multicollinearity between effective parameters on CSGPoC. In the following, the violin plot of parameters is showed in Figure 6. In this figure, the median, Q1, Q2, Q3, minimum, and maximum values of parameters are presented.
The DT is an artificial intelligence method widely employed for classifying issues, including those involving regression. Classes are included inside the trees. On the other hand, if there is not already a class for the data, researchers can employ the regression approach to develop predictions about the result based on the effective parameters [13,87,93]. A DT is a hierarchical classification algorithm, and the internal node of a DT is equivalent to databases properties. The branches of the tree reflect the results of the regulations, and the leaf nodes stand for different outcomes. A DT is constructed from two different nodes: the decision nodes and the leaf nodes. Leaf nodes do not possess branches and are regarded as the decision's result. In contrast, a decision node is capable of making a choice since they contain multiple decision-making branches. As its name implies, a DT is a kind of data architecture of trees, with a root node and it increases in size based on the number of branches [94]. The DT splits the data points into different sections. The target and the projected numbers are compared at every splitting point, and the difference is determined by the procedure being carried out. The error values are calculated at each division point, and the parameter with the least fit function is selected as a division point. The operation is then iterated as necessary. Figure 7 presents the DT flowchart.
Breiman [95] first put out the idea of using the RF technique, which is a common approach to soft computing. The RF method relies on decision-trees computations, and it has the ability to assemble numerous decision trees into a complicated structure in order to arrive at a conclusion on the classifications or regressions that have been presented to them. Throughout this phase of computation, the DTs that create the RF architecture receive training by randomly choosing parameters and data points from the primary CSGPoC database. Breiman [95] and Liaw and Wiener [96] both provide thorough overviews of the RF technique. The conceptual view of the RF model is demonstrated in Figure 8.
XGBoost is a recommendation system developed by Chen et al. [97]. The lifting technique consists of training many of the base models (learners) using specific approaches, such as the simple decision trees that have low depth, and then combining the forecasting outcomes of these weak base learners using specific techniques in order to significantly enhance the estimation impact [8,98]. As its weak learners, XGBoost employs regressive trees with a short depth. Let the learners that were acquired in the first phase be y; 0′. This will apply to the initial shallow regression tree models that were created during the training phase. It is assumed that the resulting model appears as F0(t) and that t denotes the instance vector in the space of features. XGBoost continues by computing and obtaining the first and second derivatives, which are hi and gi, of the loss function of the errors among the classifiers. This is done after the function has been evaluated. The value that was anticipated in the process before this one, which was m − 1, the objective function of Fm(t), may be calculated using the second-order expansions of the Taylor function as follows:
Obj(m)=∑Ni=1[giFm(ti)+12hiF2m(ti)]+Ω(Fm) | (2) |
The regularizing component is denoted by the symbol Ω(Fm), and its purpose is to prevent the technique from unnecessarily enhancing the degree of complexity of the models in an effort to enhance its precision, which would result in the overfitting problem. (Fm) is equivalent to the following expressions:
Ω(Fm)=γT+12λ‖w‖2 | (3) |
In which γ and λ stand for penalty coefficients, w represents the weighting of the regression leaves node, T indicates the number of regression leaves node, and ‖w‖2 shows the effect that the weight of the regression leaves node has on the level of complexity of the models. Equation (3) reveals that the fitted objective in each repetition of the XGBoost objective function is the difference between the value that was anticipated and the actual value of the data. This can be observed by comparing the projected values to the actual values. The goal of the training procedure is to reduce obj(m) to the smallest possible value. The MSE value may be used by the regression trees node dividing to choose which dividing features to use. Subsequently, it is possible to generate an additional shallower trees model called Fm(t), and the learner may be updated as follows:
y'm(t)=y'm−1(t)+Fm(t) | (4) |
The XGBoost regression flowchart is illustrated in Figure 9.
Before developing machine learning models and predicting CSGPoC, two main steps are implemented. The closer the distance between the inputs and CSGPoC parameters is, the machine learning techniques can better learn the relationships among parameters. Also, the training of machine learning techniques is only performed based on the parameters' values, not their unit. Therefore, the input values should be normalized in the range [0, 1] in the first step of pre-analysis using Eq (5) to achieve a rational output [99,100,101].
xnorm=(xi−xmaximun)(xmaximun−xminimun) | (5) |
In which xnorm, xi, xmaximum, and xminimun signify the standardized value, actual value, maximum value, and minimum value, respectively [102].
In the second step of pre-analysis, the data points were randomly categorized into two main phases: training samples (80% of whole CSGPoC, 236 data out of 295 CSGPoC data) and testing samples (20% of whole CSGPoC, 59 data out of 295 CSGPoC data). Then the train samples were applied for models learning and the testing samples were applied for the evaluation of models performance.
Overfitting is a common challenge in machine learning models, where a model performs exceptionally well on the training data but struggles with unseen data. To address this concern, the study has meticulously incorporated a safeguard in its model development process. They employed rigorous cross-validation techniques, ensuring that the model's performance is evaluated on diverse subsets of the dataset. Additionally, the authors applied regularization methods, such as dropout or weight decay, to prevent the model from becoming overly complex and fitting noise in the data. Furthermore, the use of a diverse and representative dataset, along with extensive hyperparameter tuning, contributes to the generalization capability of the model [103].
The construction of the base learner (DT) and super learner (RF and XGBoost) predictive models on 295 CSGPoC data is highlighted and discussed in this section. The performance and efficiency of the developed DT, XGBoost, and RF models were evaluated utilizing 12 statistical metrics involving mean absolute error (MAE), mean absolute percentage error (MAPE), Nash–Sutcliffe (NS), correlation coefficient (R), root mean square error (RMSE), R2, Willmott's index (WI), weighted mean absolute percentage error (WMAPE), bias index, square index (SI), p, mean relative error (MRE), and a20 index [98,104,105,106,107,108,109]. The determination of these metrics can be performed by the following equations. It should be noted that the performance of the developed predictive models is analyzed and described using scatter plots, ribbon charts, violin plots, Taylor diagrams, and error plots.
RMSE=√1n∑ni=1(O−P)2 | (6) |
MAE=1n∑ni=1|(P−O)| | (7) |
R2=∑ri=1(O−¯O)2−∑ri=1(O−P)2∑ri=1(O−¯O)2 | (8) |
R=∑(Oi−−P)(Pi−−P)√∑(Oi−−P)2∑(Oi−−P)2 | (9) |
MAPE=1n∑ni=1|O−PO|∗100 | (10) |
WMAPE=∑ni=1|O−PO|∗α∑ni=1O | (11) |
NS=1−∑ni=1(O−P)2∑ni=1(O−¯P)2 | (12) |
Bias=1n∑ni=1(P−O)2 | (13) |
SI=RMSE1n∑ni=1O | (14) |
ρ=SI1+R | (15) |
MRE=1n∑ni=1(|O−P|O) | (16) |
a20−index=m20M | (17) |
where Oi and
Some evaluation metrics including error indices of MRE, RSME, MAPE, MAE, SI, p, WI, bias, and WMAPE are applied to error analysis and evaluation of the relationships between the measured CSGPoC and predicted one with base learner and super learner models. The minimum value for error indices reveals the highest prediction capability. The R2, NS, and R determine model precision within a range of 0–1, and an amount higher than 0.95 for these metrics indicates that the proposed models present a highly reliable and accurate prediction. The obtained performance evaluation metrics of all the developed RF, XGBoost, and RF models are summarized in Figure 10. The predictive model can be specified as the most accurate system when the errors of MRE, RSME, MAPE, MAE, SI, p, WI, bias, and WMAPE are the lowest, and the values of accuracy of R2, NS, and R are higher. From Figure 10, the XGBoost model presents the highest performance prediction level based on the evaluation metrics. The highest degree of accuracy yielded by the XGBoost model achieved MAE of 2.073, MAPE of 5.547, NS of 0.981, R of 0.991, R2 of 0.982, RMSE of 2.458, WI of 0.795, WMAPE of 0.046, bias of 2.073, SI of 0.054, p of 0.027, MRE of -0.014, and a20 of 0.983 for the training model and MAE of 2.06, MAPE of 6.553, NS of 0.985, R of 0.993, R2 of 0.986, RMSE of 2.307, WI of 0.818, WMAPE of 0.05, bias of 2.06, SI of 0.056, p of 0.028, MRE of -0.015, and a20 of 0.949 for the testing model. Furthermore, the highest R2 (0.9819 for training part and 0.9857 for testing part) is achieved by the XGBoost model, while the lowest R2 (0.8859 for training part and 0.8969 for testing part) is obtained by the DT model. Therefore, the DT model has the worst performance and accuracy concerning the evaluation indices with MAE of 4.666, MAPE of 12.117, NS of 0.871, R of 0.941, R2 of 0.886, RMSE of 6.333, WI of -0.335, WMAPE of 0.103, bias of 4.666, SI of 0.143, p of 0.074, MRE of 0.029, and a20 of 0.826 for the training set and MAE of 5.103, MAPE of 15.736, NS of 0.896, R of 0.947, R2 of 0.897, RMSE of 6.111, WI of -0.265, WMAPE of 0.125, bias of 5.103, SI of 0.149, p of 0.076, MRE of -0.036, and a20 of 0.729 for the testing set.
The obtained R2 and correlation between measured and predicted CSGPoC by XGBoost, RF, and DT is illustrated in Figures 11–13, respectively. As can be seen, the highest R2 value is relevant to the XGBoost model in both the training and testing sets.
In the following, the performance of the models is evaluated by adding testing sets to trained models. The capability of the prediction performance is specified by testing data. An acceptable value for statistical metrics in the training phase does not mean that the models are predicting correctly, and the efficiency of the models should be evaluated using test data. If the model error is reduced by importing the test data into modeling process, it can be concluded that the model training is not performed well and the model is not able to predict the CSGPoC values in the real world. The testing results are revealed in Figure 14. It can be seen that the prediction of CSGPoC is conducted correctly, as the results of the testing phase are approximately close to the results of the training phase for all DT, RF, and XGBoost models. Specially, the results of the XGBoost model are acceptable and its accuracy is confirmed for predicting CSGPoC in other projects.
The distribution of error for all models is revealed from the violin plot, which is demonstrated in Figure 15. The violin diagram shows the range of errors relevant to the DT, XGBoost, and RF models. From Figure 15, it can be found that the range of errors in the XGBoost model is lower for both the training and testing phases compared to the RF and DT models. Of the models, the DT model has the lowest accuracy due to its higher error ranges on the violin plot. The schematic demonstration of the standard deviation, coefficient determination, and RMSE values can be shown using Taylor's diagram, which is displayed for the training and testing parts of the models in Figure 16. This figure assigns the best-fitted model. In this figure, the red dashed line represents the standard deviation of the data. As depicted in Figure 16, both the standard deviation and R2 are close to 1 for reference point. The XGBoost, DT, and RF models are illustrated in this figure using green, orange and purple colored squares. The XGBoost symbol is very close to the reference symbol (red square), which shows that the XGBoost model reflects the reality-based results and presents better results in terms of performance and precision values. It can be concluded that although the accuracy of the DT and RF models is acceptable, XGBoost is the superior model for predicting CSGPoC.
In the last step of modeling processes, a sensitivity analysis on the effective parameters is performed. Sensitivity analysis techniques, such as the cosine amplitude method (CAM), evaluate the impact of input parameters or assumptions on the output of a model or system. This method involves systematically varying individual input parameters while keeping other factors constant and measuring the resulting changes in the model's output. By applying this method, researchers can quantify the model's sensitivity to specific input variations and identify the parameters that had the most critical impacts on the model's behaviors. Through this analysis, researchers can identify critical parameters that contribute the most to output variability, allowing for the prioritization of resources and efforts toward addressing and optimizing these influential factors. Also, this method evaluates how sensitive the model is to small or large fluctuations in input parameters [113], as follows:
sij=m∑k=1xik⋅xjk√(m∑k=1x2ik)⋅(m∑k=1x2jk) | (18) |
where xik and xjk represent the inputs and output variables, and m stands the number of data points.
In accordance with the devised methodology of CAM, emphasis was placed on assessing the sensitivity of output variables to input variables. As illustrated in Figure 17, the influence of input parameters (X) on objective functions (outputs) was investigated. A higher value of rij, closer to 1, signifies a more pronounced impact of the input parameters on the objectives (outputs). The outcomes depicted in Figure 17 reveal that a majority of the input parameters exhibit significant effects on CSGPoC. Specifically, the parameters Fag and WS demonstrated the most substantial impacts on CSGPoC, with strengths of 0.928 and 0.904, respectively. In the second rank, Na2SiO3, gravel 10/20, and GGBS parameters exhibited comparable strengths of 0.872, 0.863, and 0.838, indicating approximately similar levels of influence. Additionally, the parameters NaOH and NaOH molarity exerted notable effects on CSGPoC, with strengths of 0.791 and 0.787, respectively. Also, the impact of FA on CSGPoC was moderate, as evidenced by a strength value of 0.552.
Despite the promising results obtained from the XGBoost model in predicting CSGPoC, it is essential to acknowledge certain limitations within this study. First, the generalization of the developed models may be constrained by the specific composition and characteristics of the dataset used for training and testing. The reliance on a singular dataset, consisting of 259 CSGPoC samples, may not fully encapsulate the diverse range of conditions and materials encountered in real-world scenarios. Additionally, the study focuses on a specific set of input parameters, and the exclusion of other potentially influential factors could limit the model's applicability to a broader spectrum of geo-polymer concrete formulations. Furthermore, the current research primarily addresses the prediction aspect, and considering various external factors, the practical implementation of the proposed models in an industrial setting remains a subject for future exploration.
To advance the field of geo-polymer concrete compressive strength estimation, future research endeavors should aim to address the identified limitations and explore new avenues. First, expanding the dataset to include a more extensive variety of geo-polymer concrete formulations and considering additional influential factors could enhance the robustness and generalization of the developed models. The incorporation of real-world complexities, such as environmental conditions and variations in raw materials, would contribute to the models' reliability in practical applications. Moreover, a comparative analysis with other advanced machine learning algorithms and the integration of hybrid models could provide further insights into optimizing the accuracy and efficiency of CSGPoC prediction. The scalability and adaptability of the models for different scales of construction projects and manufacturing processes should also be investigated. Hence, validation through large-scale field trials would validate the models' effectiveness and facilitate their seamless integration into the decision-making processes of the green concretes industry.
The main purpose of the current research is to establish a robust predictive system for predicting CSGPoC. The creation of environmentally friendly building supplies may be helped along by the increased use of geo-polymer concrete in the construction sector, which is also helping to popularize this material. This research has a beneficial effect on advancing the use of geopolymer concrete by boosting its use. An efficient super learner technique for predicting the CSGPoC was proposed with the use of the XGBoost and RF models, which allowed for the development of a high-performance model. A database comprised of 259 CSGPoC data points was gathered from literature for developing DT, RF, and XGBoost models as well as accurately predicting CSGPoC. For developing models, nine effective parameters, including FA, GGBS, Na2SiO3, NaOH, FAg, Gravel 4/10, Gravel 10/20, WS, and NaOH molarity, were considered. The obtained results clarified that the highest R2 was determined by the XGBoost model as 0.9819 and 0.9857 for the training and testing parts, respectively. Hence, the XGBoost outperformed DT with R2 of 0.8859 and 0.8969 and RF with R2 of 0.9492 and 0.9424 for training and testing phases, respectively. It can be concluded that the XGBoost super learner model is significantly more efficient in establishing estimation models of CSGPoC than the DT and RF models.
The authors declare they have not used Artificial Intelligence (AI) tools in the creation of this article.
This research was supported by the Guangdong provincial science and technology plan project (Grant No. 2021B1111610002), Natural Science Foundation of Hunan (Grant No. 2023JJ50418) and Hunan Provincial transportation technology project (Grant No. 202109). The writers are grateful for this support.
The authors declare no conflict of interest.
[1] |
Cetegen BM, Mohamad N (1993) Experiments on liquid mixing and reaction in a vortex. J Fluid Mech 249: 391–414. https://doi.org/10.1017/S0022112093001223 doi: 10.1017/S0022112093001223
![]() |
[2] |
Verzicco R, Orlandi P (1995) Mixedness in the formation of a vortex ring. Phys Fluids 7: 1513–1515. https://doi.org/10.1063/1.868538 doi: 10.1063/1.868538
![]() |
[3] |
Urzay J (2018) Supersonic combustion in air-breathing propulsion systems for hypersonic flight. Annu Rev Fluid Mech 50: 593–627. https://doi.org/10.1146/annurev-fluid-122316-045217 doi: 10.1146/annurev-fluid-122316-045217
![]() |
[4] | Ferri A, Libby PA, Zakkay V (1964) Theoretical and experimental investigation of supersonic combustion, in High Temperatures in Aeronautics, Elsevier, 55–118. https://doi.org/10.1016/B978-0-08-010558-1.50011-6 |
[5] |
Ferri A (1973) Mixing-controlled supersonic combustion. Annu Rev Fluid Mech 5: 301–338. https://doi.org/10.1146/annurev.fl.05.010173.001505 doi: 10.1146/annurev.fl.05.010173.001505
![]() |
[6] | Gupta AK, Lilley DG, Syred N (1984) Swirl flows. Tunbridge Wells. |
[7] |
Candel S, Durox D, Schuller T, et al. (2014) Dynamics of swirling flames. Annu Rev Fluid Mech 46: 147–173. https://doi.org/10.1146/annurev-fluid-010313-141300 doi: 10.1146/annurev-fluid-010313-141300
![]() |
[8] | Bahr D (1987) Technology for the design of high temperature rise combustors. J Propuls Power 3: 179–186. |
[9] |
An Q, Steinberg AM (2019) The role of strain rate, local extinction, and hydrodynamic instability on transition between attached and lifted swirl flames. Combusti Flame 199: 267–278. https://doi.org/10.1016/j.combustflame.2018.10.029 doi: 10.1016/j.combustflame.2018.10.029
![]() |
[10] |
Caulfield C (2021) Layering, instabilities, and mixing in turbulent stratified flows. Annu Rev Fluid Mech 53: 113–145. https://doi.org/10.1146/annurev-fluid-042320-100458 doi: 10.1146/annurev-fluid-042320-100458
![]() |
[11] |
Crimaldi JP, Zimmer RK (2014) The physics of broadcast spawning in benthic invertebrates. Annu Rev Mar Sci 6: 1. https://doi.org/10.1146/annurev-marine-010213-135119 doi: 10.1146/annurev-marine-010213-135119
![]() |
[12] |
Mingotti N, Wood R, Noakes C, et al. (2020) The mixing of airborne contaminants by the repeated passage of people along a corridor. J Fluid Mech 903: A52. https://doi.org/10.1017/jfm.2020.671 doi: 10.1017/jfm.2020.671
![]() |
[13] |
Lohse D, Xia KQ (2010) Small-scale properties of turbulent rayleigh-bénard convection. Annu Rev Fluid Mech 42: 335–364. https://doi.org/10.1146/annurev-fluid-10908.165152 doi: 10.1146/annurev-fluid-10908.165152
![]() |
[14] |
Kolmogorov AN (1941) The local structure of turbulence in incompressible viscous fluid for very large reynolds numbers. Cr Acad Sci URSS 30: 301–305. https://doi.org/10.1007/978-94-011-3030-1_45 doi: 10.1007/978-94-011-3030-1_45
![]() |
[15] |
Batchelor GK (1959) Small-scale variation of convected quantities like temperature in turbulent fluid Part 1. general discussion and the case of small conductivity. J Fluid Mech 5: 113–133. https://doi.org/10.1017/S002211205900009X doi: 10.1017/S002211205900009X
![]() |
[16] |
Warhaft Z (2000) Passive scalars in turbulent flows. Annu Rev Fluid Mech 32: 203–240. https://doi.org/10.1146/annurev.fluid.32.1.203 doi: 10.1146/annurev.fluid.32.1.203
![]() |
[17] |
Schwertfirm F, Manhart M (2007) Dns of passive scalar transport in turbulent channel flow at high schmidt numbers. Int J Heat Fluid Fl 28: 1204–1214. https://doi.org/10.1016/j.ijheatfluidflow.2007.05.012 doi: 10.1016/j.ijheatfluidflow.2007.05.012
![]() |
[18] |
Dimotakis PE (2000) The mixing transition in turbulent flows. J Fluid Mech 409: 69–98. https://doi.org/10.1017/S0022112099007946 doi: 10.1017/S0022112099007946
![]() |
[19] |
Meunier P, Villermaux E (2003) How vortices mix. J Fluid Mech 476: 213–222. https://doi.org/10.1017/S0022112002003166 doi: 10.1017/S0022112002003166
![]() |
[20] |
Souzy M, Zaier I, Lhuissier H, et al. (2018) Mixing lamellae in a shear flow. J Fluid Mech 838: R3. https://doi.org/10.1017/jfm.2017.916 doi: 10.1017/jfm.2017.916
![]() |
[21] |
Buaria D, Clay MP, Sreenivasan KR, et al. (2021) Turbulence is an ineffective mixer when schmidt numbers are large. Phys Rev Lett 126: 074501. https://doi.org/10.1103/PhysRevLett.126.074501 doi: 10.1103/PhysRevLett.126.074501
![]() |
[22] |
Raynal F, Gence JN (1997) Energy saving in chaotic laminar mixing. Int J Heat Mass Trans 40: 3267–3273. https://doi.org/10.1016/S0017-9310(96)00383-3 doi: 10.1016/S0017-9310(96)00383-3
![]() |
[23] |
Dimotakis PE (2005) Turbulent mixing. Annu Rev Fluid Mech 37: 329–356. https://doi.org/10.1146/annurev.fluid.36.050802.122015 doi: 10.1146/annurev.fluid.36.050802.122015
![]() |
[24] |
Thiffeault JL (2012) Using multiscale norms to quantify mixing and transport. Nonlinearity 25: R1. https://doi.org/10.1088/0951-7715/25/2/R1 doi: 10.1088/0951-7715/25/2/R1
![]() |
[25] |
Duplat J, Jouary A, Villermaux E (2010) Entanglement rules for random mixtures. Phys Rev Lett 105: 034504. https://doi.org/10.1103/PhysRevLett.105.034504 doi: 10.1103/PhysRevLett.105.034504
![]() |
[26] |
Kree M, Duplat J, Villermaux E (2013) The mixing of distant sources. Phys Fluids 25: 091103. https://doi.org/10.1063/1.4820015 doi: 10.1063/1.4820015
![]() |
[27] |
Villermaux E, Rehab H (2000) Mixing in coaxial jets. J Fluid Mech 425: 161–185. https://doi.org/10.1017/S002211200000210X doi: 10.1017/S002211200000210X
![]() |
[28] | Vidick B (1989) Critical mixing parameters for good control of cement slurry quality. in SPE Production Operations Symposium. OnePetro. https://doi.org/10.2118/18895-PA |
[29] |
Poulain S, Villermaux E, Bourouiba L (2018) Ageing and burst of surface bubbles. J Fluid Mech 851: 636–671. https://doi.org/10.1017/jfm.2018.471 doi: 10.1017/jfm.2018.471
![]() |
[30] | Marble F (1985) Growth of a diffusion flame in the field of a vortex, in Recent advances in the aerospace sciences, Springer, 395–413. https://doi.org/10.1007/978-1-4684-4298-4_19 |
[31] |
Villermaux E (2019) Mixing versus stirring. Annu Rev Fluid Mech 51: 245–273. https://doi.org/10.1146/annurev-fluid-010518-040306 doi: 10.1146/annurev-fluid-010518-040306
![]() |
[32] |
Ranz WE (1979) Applications of a stretch model to mixing, diffusion, and reaction in laminar and turbulent flows. AIChE J 25: 41–47. https://doi.org/10.1002/aic.690250105 doi: 10.1002/aic.690250105
![]() |
[33] | Marble FE, Broadwell JE (1977) The coherent flame model for turbulent chemical reactions. Purdue Univ Lafayette in project squid head quaters. Tech Rep. Available from: https://api.semanticscholar.org/CorpusID:45411925. |
[34] |
Villermaux E, Duplat J (2003) Mixing is an aggregation process. Comptes Rendus Mécanique 331: 515–523. https://doi.org/10.1016/S1631-0721(03)00110-4 doi: 10.1016/S1631-0721(03)00110-4
![]() |
[35] |
Duplat J, Villermaux E (2008) Mixing by random stirring in confined mixtures. J Fluid Mech 617: 51–86. https://doi.org/10.1017/S0022112008003789 doi: 10.1017/S0022112008003789
![]() |
[36] |
Duplat J, Innocenti C, Villermaux E (2010) A nonsequential turbulent mixing process. Phys Fluids 22: 035104. https://doi.org/10.1063/1.3319821 doi: 10.1063/1.3319821
![]() |
[37] |
Haller G (2015) Lagrangian coherent structures. Annu Rev Fluid Mech 47: 137–162. https://doi.org/10.1146/annurev-fluid-010313-141322 doi: 10.1146/annurev-fluid-010313-141322
![]() |
[38] |
Hang H, Yu B, Xiang Y, et al. (2020) An objective-adaptive refinement criterion based on modified ridge extraction method for finite-time lyapunov exponent (ftle) calculation. J Visual 23: 81–95. https://doi.org/10.1007/S12650-019-00605-1 doi: 10.1007/S12650-019-00605-1
![]() |
[39] |
Liang G, Yu B, Zhang B, et al. (2019) Hidden flow structures in compressible mixing layer and a quantitative analysis of entrainment based on lagrangian method. J Hydrodyn 31: 256–265. https://doi.org/10.1017/jfm.2020.295 doi: 10.1017/jfm.2020.295
![]() |
[40] |
Zheng Z, Fan Z, Wang Z, et al. (2021) Lagrangian visualization of mixing enhancement induced by finite-time stretching in compressible vortex interaction. J Visual 24: 19–28. https://doi.org/10.1007/s12650-020-00698-z doi: 10.1007/s12650-020-00698-z
![]() |
[41] |
Götzfried P, Emran MS, Villermaux E, et al. (2019) Comparison of lagrangian and eulerian frames of passive scalar turbulent mixing. Phys Rev Fluids 4: 044607. https://doi.org/10.1103/PhysRevFluids.4.044607 doi: 10.1103/PhysRevFluids.4.044607
![]() |
[42] |
Meunier P, Villermaux E (2022) The diffuselet concept for scalar mixing. J Fluid Mech 951: A33. https://doi.org/10.1017/jfm.2022.771 doi: 10.1017/jfm.2022.771
![]() |
[43] |
Meunier P, Villermaux E (2010) The diffusive strip method for scalar mixing in two dimensions. J Fluid Mechanics 662: 134–172. https://doi.org/10.1017/S0022112010003162 doi: 10.1017/S0022112010003162
![]() |
[44] |
Martínez-Ruiz D, Meunier P, Favier B, et al.(2018) The diffusive sheet method for scalar mixing. J Fluid Mech 837: 230–257. https://doi.org/10.1017/S0022112010003162 doi: 10.1017/S0022112010003162
![]() |
[45] |
Sen S, Singh P, Heyman J, et al. (2020) The impact of stretching-enhanced mixing and coalescence on reactivity in mixing-limited reactive flows. Phys Fluids 32: 106602, 2020. https://doi.org/10.1063/5.0022798 doi: 10.1063/5.0022798
![]() |
[46] |
Heyman J, Lester DR, Turuban R, et al. (2020) Stretching and folding sustain microscale chemical gradients in porous media. P Natl Acad Sci 117: 13 359–13 365. https://doi.org/10.1073/pnas.2002858117 doi: 10.1073/pnas.2002858117
![]() |
[47] |
Guilbert E, Almarcha C, Villermaux E (2021) Chemical reaction for mixing studies. Phys Rev Fluids 6: 114501. https://doi.org/10.1103/PhysRevFluids.6.114501 doi: 10.1103/PhysRevFluids.6.114501
![]() |
[48] |
Guilbert E, Metzger B, Villermaux E (2022) Chemical production on a deforming substrate. J Fluid Mech 934: R1. https://doi.org/10.1017/jfm.2021.1122 doi: 10.1017/jfm.2021.1122
![]() |
[49] |
Buch KA, Dahm WJ (1996) Experimental study of the fine-scale structure of conserved scalar mixing in turbulent shear flows. part 1. sc [dbl greater-than sign] 1. J Fluid Mech 317: 21–71. https://doi.org/10.1017/s0022112096000651 doi: 10.1017/s0022112096000651
![]() |
[50] |
Cetegen B, Aguirre J (1990) Analysis of molecular mixing and chemical reaction in a vortex pair. Phys Fluids 2: 2211–2216. https://doi.org/10.1063/1.857807 doi: 10.1063/1.857807
![]() |
[51] |
Basu S, Barber T, Cetegen B (2007) Computational study of scalar mixing in the field of a gaseous laminar line vortex. Phys Fluids 19: 053601. https://doi.org/10.1063/1.2732454 doi: 10.1063/1.2732454
![]() |
[52] |
Flohr P, Vassilicos J (1997) Accelerated scalar dissipation in a vortex. J Fluid Mech 348: 295–317. https://doi.org/10.1017/S0022112097006927 doi: 10.1017/S0022112097006927
![]() |
[53] |
Bilger R (1976) The structure of diffusion flames. Combust Sci Technol 13: 155–170. https://doi.org/10.1080/00102207608946733 doi: 10.1080/00102207608946733
![]() |
[54] |
Bilger RW (1989) Turbulent diffusion flames. Annu Rev Fluid Mech 21: 101–135. https://doi.org/10.1146/annurev.fl.21.010189.000533 doi: 10.1146/annurev.fl.21.010189.000533
![]() |
[55] |
Yeung P, Girimaji S, Pope S (1990) Straining and scalar dissipation on material surfaces in turbulence: implications for flamelets. Combust Flame 79: 340–365. https://doi.org/10.1016/0010-2180(90)90145-H doi: 10.1016/0010-2180(90)90145-H
![]() |
[56] |
Kim SH, Pitsch H (2007) Scalar gradient and small-scale structure in turbulent premixed combustion. Phys Fluids 19: 115104. https://doi.org/10.1063/1.2784943 doi: 10.1063/1.2784943
![]() |
[57] |
Boratav O, Elghobashi S, Zhong R (1996) On the alignment of the alpha-strain and vorticity in turbulent nonpremixed flames. Phys Fluids 8: 2251–2253. https://doi.org/10.1063/1.869000 doi: 10.1063/1.869000
![]() |
[58] |
Boratav O, Elghobashi S, Zhong R (1998) On the alignment of strain, vorticity and scalar gradient in turbulent, buoyant, nonpremixed flames. Phys Fluids 10: 2260–2267. https://doi.org/10.1063/1.869747 doi: 10.1063/1.869747
![]() |
[59] |
Attili A, Bisetti F (2019) Statistics of scalar dissipation and strain/vorticity/scalar gradient alignment in turbulent nonpremixed jet flames. Flow Turbul Combust 103: 625–642. https://doi.org/10.1007/s10494-019-00044-w doi: 10.1007/s10494-019-00044-w
![]() |
[60] |
McManus TA, Sutton JA (2022) Conditional analysis of temperature and strain rate effects on dissipation structure in turbulent non-premixed jet flames. P Combust Inst. https://doi.org/10.1016/j.proci.2022.07.052 doi: 10.1016/j.proci.2022.07.052
![]() |
[61] |
Chakraborty N, Swaminathan N (2007) Influence of the damköhler number on turbulence-scalar interaction in premixed flames. i. physical insight. Phys Fluids 19: 045103. https://doi.org/10.1063/1.2714070 doi: 10.1063/1.2714070
![]() |
[62] |
Zhao S, Er-Raiy A, Bouali Z, et al. (2018) Dynamics and kinematics of the reactive scalar gradient in weakly turbulent premixed flames. Combust Flame 198: 436–454. https://doi.org/10.1016/j.combustflame.2018.10.002 doi: 10.1016/j.combustflame.2018.10.002
![]() |
[63] |
Batchelor GK (1952) The effect of homogeneous turbulence on material lines and surfaces. Proc Math Phys Sci 213: 349–366. https://doi.org/10.1098/rspa.1952.0130 doi: 10.1098/rspa.1952.0130
![]() |
[64] |
Girimaji S, Pope S (1990) 'Material-element deformation in isotropic turbulence. J Fluid Mech 220: 427–458. https://doi.org/10.1017/S0022112090003330 doi: 10.1017/S0022112090003330
![]() |
[65] |
Dresselhaus E, Tabor M (1992) The kinematics of stretching and alignment of material elements in general flow fields. J Fluid Mech 236: 415–444. https://doi.org/10.1017/S0022112092001460 doi: 10.1017/S0022112092001460
![]() |
[66] |
Lapeyre G, Klein P, Hua B (1999) Does the tracer gradient vector align with the strain eigenvectors in 2d turbulence? Phys Fluids 11: 3729–3737. https://doi.org/10.1063/1.870234 doi: 10.1063/1.870234
![]() |
[67] |
Klein P, Hua BL, Lapeyre G (2000) Alignment of tracer gradient vectors in 2d turbulence. Physica D 146: 246–260. https://doi.org/10.1016/S0167-2789(00)00119-6 doi: 10.1016/S0167-2789(00)00119-6
![]() |
[68] |
Swaminathan N, Grout R (2006) Interaction of turbulence and scalar fields in premixed flames. Phys Fluids 18: 045102. https://doi.org/10.1063/1.2186590 doi: 10.1063/1.2186590
![]() |
[69] |
Minamoto Y, Jigjid K, Igari R, et al. (2022) Effect of flame–flame interaction on scalar pdf in turbulent premixed flames. Combust Flame 239: 111660. https://doi.org/10.1016/j.combustflame.2021.111660 doi: 10.1016/j.combustflame.2021.111660
![]() |
[70] |
Holzner M, Lüthi B (2011) Laminar superlayer at the turbulence boundary. Phys Rev Lett 106, : 134503. https://doi.org/10.1103/PhysRevLett.106.134503 doi: 10.1103/PhysRevLett.106.134503
![]() |
[71] |
Townsend AA (1951) The diffusion of heat spots in isotropic turbulence. Proc Math Phys Sci 209: 418–430. https://doi.org/10.1098/rspa.1951.0216 doi: 10.1098/rspa.1951.0216
![]() |
[72] |
Cocke W (1969) Turbulent hydrodynamic line stretching: consequences of isotropy. Phys Fluids 12: 2488–2492. https://doi.org/10.1063/1.1692385 doi: 10.1063/1.1692385
![]() |
[73] |
Ashurst WT, Kerstein A, Kerr R, et al. (1987) Alignment of vorticity and scalar gradient with strain rate in simulated navier–stokes turbulence. Phys Fluids 30: 2343–2353. https://doi.org/10.1063/1.866513 doi: 10.1063/1.866513
![]() |
[74] |
Vincent A, Meneguzzi M (1991) The spatial structure and statistical properties of homogeneous turbulence. J Fluid Mech 225: 1–20. https://doi.org/10.1017/S0022112091001957 doi: 10.1017/S0022112091001957
![]() |
[75] |
Carter HH, Okubo A (1972) Longitudinal dispersion in non-uniform flow. Water Resour Res 8: 648–660. https://doi.org/10.1029/WR008i003p00648 doi: 10.1029/WR008i003p00648
![]() |
[76] |
Weiss J (1991) The dynamics of enstrophy transfer in two-dimensional hydrodynamics. Physica D 48: 273–294. https://doi.org/10.1016/0167-2789(91)90088-Q doi: 10.1016/0167-2789(91)90088-Q
![]() |
[77] | Hunt JC, Wray AA, Moin P (1988) Eddies, streams, and convergence zones in turbulent flows. Studying turbulence using numerical simulation databases, 2. Proceedings of the 1988 summer program. Available from: https://ntrs.nasa.gov/citations/19890015184 |
[78] |
Basdevant C, Philipovitch T (1994) On the validity of the "weiss criterion" in two-dimensional turbulence. Physica D 73: 17–30. https://doi.org/10.1016/0167-2789(94)90222-4 doi: 10.1016/0167-2789(94)90222-4
![]() |
[79] |
Hua B, Klein P (1998) An exact criterion for the stirring properties of nearly two-dimensional turbulence. Physica D 113: 98–110. https://doi.org/10.1016/S0167-2789(97)00143-7 doi: 10.1016/S0167-2789(97)00143-7
![]() |
[80] |
Galanti B, Gibbon J, Heritage M (1997) Vorticity alignment results for the three-dimensional euler and navier-stokes equations. Nonlinearity 10: 1675. https://doi.org/10.1088/0951-7715/10/6/013 doi: 10.1088/0951-7715/10/6/013
![]() |
[81] |
Ohkitani K, Kishiba S (1995) Nonlocal nature of vortex stretching in an inviscid fluid. Phys Fluids 7: 411–421. https://doi.org/10.1063/1.868638 doi: 10.1063/1.868638
![]() |
[82] |
Tsinober A, Ortenberg M, Shtilman L (1999) On depression of nonlinearity in turbulence. Phys Fluids 11: 2291–2297. https://doi.org/10.1063/1.870091 doi: 10.1063/1.870091
![]() |
[83] |
Wacks DH, Chakraborty N, Klein M, et al. (2016) Flow topologies in different regimes of premixed turbulent combustion: A direct numerical simulation analysis. Phys Rev Fluids 1: 083401. https://doi.org/10.1103/PhysRevFluids.1.083401 doi: 10.1103/PhysRevFluids.1.083401
![]() |
[84] |
Meneveau C (2011) Lagrangian dynamics and models of the velocity gradient tensor in turbulent flows. Annu Rev Fluid Mech 43: 219–245. https://doi.org/10.1146/annurev-fluid-122109-160708 doi: 10.1146/annurev-fluid-122109-160708
![]() |
[85] |
Jeong J, Hussain F (1995) On the identification of a vortex. J Fluid Mech 285: 69–94. https://doi.org/10.1017/S0022112095000462 doi: 10.1017/S0022112095000462
![]() |
[86] |
Chong MS, Perry AE, Cantwell BJ (1990) A general classification of three-dimensional flow fields. Phys Fluids A 2: 765–777. https://doi.org/10.1063/1.857730 doi: 10.1063/1.857730
![]() |
[87] |
Perry AE, Chong MS (1987) A description of eddying motions and flow patterns using critical-point concepts. Annu Rev Fluid Mech 19: 125–155. https://doi.org/10.1146/annurev.fl.19.010187.001013 doi: 10.1146/annurev.fl.19.010187.001013
![]() |
[88] |
Danish M, Suman S, Girimaji SS (2016) Influence of flow topology and dilatation on scalar mixing in compressible turbulence. J Fluid Mech 793: 633–655. https://doi.org/10.1017/jfm.2016.145 doi: 10.1017/jfm.2016.145
![]() |
[89] |
Gao X, Bermejo-Moreno I, Larsson J (2020) Parametric numerical study of passive scalar mixing in shock turbulence interaction. J Fluid Mech 895: A21. https://doi.org/10.1017/jfm.2020.292 doi: 10.1017/jfm.2020.292
![]() |
[90] |
Cifuentes L, Dopazo C, Martin J, et al. (2014) Local flow topologies and scalar structures in a turbulent premixed flame. Phys Fluids 26: 065108. https://doi.org/10.1063/1.4884555 doi: 10.1063/1.4884555
![]() |
[91] |
Han W, Scholtissek A, Dietzsch F, et al. (2019) Influence of flow topology and scalar structure on flame-tangential diffusion in turbulent non-premixed combustion. Combust Flame 206: 21–36. https://doi.org/10.1016/j.combustflame.2019.04.038 doi: 10.1016/j.combustflame.2019.04.038
![]() |
[92] |
Heyman J, Lester D, Le Borgne T (2021) Scalar signatures of chaotic mixing in porous media. Phys Rev Lett 126: 034505. https://doi.org/10.1103/PhysRevLett.126.034505 doi: 10.1103/PhysRevLett.126.034505
![]() |
[93] |
Waitz I, Qiu Y, Manning T, et al. (1997) Enhanced mixing with streamwise vorticity. Prog Aerosp Sci 33: 323–351. https://doi.org/10.1016/S0376-0421(96)00008-5 doi: 10.1016/S0376-0421(96)00008-5
![]() |
[94] |
Vergine F, Ground C, Maddalena L (2016) Turbulent kinetic energy decay in supersonic streamwise interacting vortices. J Fluid Mech 807: 353–385. https://doi.org/10.1017/jfm.2016.611 doi: 10.1017/jfm.2016.611
![]() |
[95] |
Wu Z, He M, Yu B, et al. (2022) A circulation prediction model for ramp and vortex generator in supersonic flow: A numerical study. Aerosp Sci Technol 127: 107688. https://doi.org/10.1016/j.ast.2022.107688 doi: 10.1016/j.ast.2022.107688
![]() |
[96] |
Wang Z, Yu B, Zhang B, et al. (2021) Kinematic and mixing characteristics of vortex interaction induced by a vortex generator model: a numerical study. Appl Math Mech 42: 387–404. https://doi.org/10.1007/s10483-021-2711-5 doi: 10.1007/s10483-021-2711-5
![]() |
[97] |
Schetz JA, Maddalena L, Burger SK (2010) Molecular weight and shock-wave effects on transverse injection in supersonic flow. J Propuls Power 26: 1102–1113. https://doi.org/10.2514/1.49355 doi: 10.2514/1.49355
![]() |
[98] |
Tew DE, Hermanson JC, Waitz IA (2004) Impact of compressibility on mixing downstream of lobed mixers. AIAA J 42: 2393–2396. https://doi.org/10.2514/1.11004 doi: 10.2514/1.11004
![]() |
[99] |
Brouillette M (2002) The richtmyer-meshkov instability. Annu Rev Fluid Mech 34: 445–468. https://doi.org/10.1146/annurev.fluid.34.090101.162238 doi: 10.1146/annurev.fluid.34.090101.162238
![]() |
[100] |
Zhou Y (2017) Rayleigh–taylor and richtmyer–meshkov instability induced flow, turbulence, and mixing. ii. Phys Rep 723: 1–160. https://doi.org/10.1016/j.physrep.2017.07.008 doi: 10.1016/j.physrep.2017.07.008
![]() |
[101] |
Zhou Y (2017) Rayleigh–taylor and richtmyer–meshkov instability induced flow, turbulence, and mixing. i. Phys Rep 720-722: 1–136. https://doi.org/10.1016/j.physrep.2017.07.005 doi: 10.1016/j.physrep.2017.07.005
![]() |
[102] |
Peng G, Zabusky NJ, Zhang S (2003) Vortex-accelerated secondary baroclinic vorticity deposition and late-intermediate time dynamics of a two-dimensional richtmyer–meshkov interface. Phys Fluids 15: 3730–3744. https://doi.org/10.1063/1.1621628 doi: 10.1063/1.1621628
![]() |
[103] |
Livescu D (2020) Turbulence with large thermal and compositional density variations. Annu Rev Fluid Mech 52: 309-341. https://doi.org/10.1146/annurev-fluid-010719-060114 doi: 10.1146/annurev-fluid-010719-060114
![]() |
[104] |
Weber C, Haehn N, Oakley J, et al. (2012) Turbulent mixing measurements in the richtmyer-meshkov instability. Phys Fluids 24: 074105. https://doi.org/10.1063/1.4733447 doi: 10.1063/1.4733447
![]() |
[105] |
Mikaelian KO (1998) Analytic approach to nonlinear rayleigh-taylor and richtmyer-meshkov instabilities. Phys Rev Lett 80: 508–511. https://doi.org/10.1103/PhysRevLett.80.508 doi: 10.1103/PhysRevLett.80.508
![]() |
[106] |
Zabusky NJ (1999) Vortex paradigm for accelerated inhomogeneous flows: Visiometrics for the rayleigh-taylor and richtmyer-meshkov environments. Annu Rev Fluid Mech 31: 495–536. https://doi.org/10.1146/annurev.fluid.31.1.495 doi: 10.1146/annurev.fluid.31.1.495
![]() |
[107] |
Kumar S, Orlicz G, Tomkins C, et al. (2005) Stretching of material lines in shock-accelerated gaseous flows. Phys Fluids 17: 082107. https://doi.org/10.1063/1.2031347 doi: 10.1063/1.2031347
![]() |
[108] |
Zhou Y, Cabot WH, Thornber B (2016) Asymptotic behavior of the mixed mass in rayleigh–taylor and richtmyer–meshkov instability induced flows. Phys Plasmas 23: 052712. https://doi.org/10.1063/1.4951018 doi: 10.1063/1.4951018
![]() |
[109] |
Richtmyer RD (1960) Taylor instability in shock acceleration of compressible fluids. Commun Pure Appl Math 13: 297–319. https://doi.org/10.1002/cpa.3160130207 doi: 10.1002/cpa.3160130207
![]() |
[110] |
Meshkov E (1969) Instability of the interface of two gases accelerated by a shock wave. Fluid Dyn 4: 101–104. https://doi.org/10.1007/BF01015969 doi: 10.1007/BF01015969
![]() |
[111] |
Klein RI, McKee CF, Colella P (1994) On the hydrodynamic interaction of shock waves with interstellar clouds. 1: Nonradiative shocks in small clouds. Astrophys J 420: 213–236. https://doi.org/10.1086/173554 doi: 10.1086/173554
![]() |
[112] |
Cabot WH, Cook AW (2006) Reynolds number effects on rayleigh–taylor instability with possible implications for type ia supernovae. Nat Phys 2: 562–568. https://doi.org/10.1038/nphys361 doi: 10.1038/nphys361
![]() |
[113] |
Lindl JD, McCrory RL, Campbell EM (1992) Progress toward ignition and burn propagation in inertial confinement fusion. Phys Today 45: 32–40. https://doi.org/10.1063/1.881318 doi: 10.1063/1.881318
![]() |
[114] |
EOran ES, Gamezo VN (2007) Origins of the deflagration-to-detonation transition in gas-phase combustion. Combust Flame 148: 4–47. https://doi.org/10.1016/j.combustflame.2006.07.010 doi: 10.1016/j.combustflame.2006.07.010
![]() |
[115] |
Yang J, Kubota T, Zukoski EE (1994) A model for characterization of a vortex pair formed by shock passage over a light-gas inhomogeneity. J Fluid Mech 258: 217–244. https://doi.org/10.1017/S0022112094003307 doi: 10.1017/S0022112094003307
![]() |
[116] |
Yu B, He M, Zhang B, et al. (2020) Two-stage growth mode for lift-off mechanism in oblique shock-wave/jet interaction. Phys Fluids 32: 116105. https://doi.org/10.1063/5.0022449 doi: 10.1063/5.0022449
![]() |
[117] |
Zhang B, Liu H, Yu B, et al. (2022) Numerical investigation on combustion-enhancement strategy in shock–fuel jet interaction. AIAA J 60: 393–410. https://doi.org/10.2514/1.J060168 doi: 10.2514/1.J060168
![]() |
[118] |
Zhang B, Chen H, Yu B, et al. (2019) Molecular simulation on viscous effects for microscale combustion in reactive shock-bubble interaction. Combust Flame 208: 351–363. https://doi.org/10.1016/j.combustflame.2019.07.001 doi: 10.1016/j.combustflame.2019.07.001
![]() |
[119] |
Liu H, Yu B, Chen H, et al. (2020) Contribution of viscosity to the circulation deposition in the richtmyer–meshkov instability. J Fluid Mech 895: A10. https://doi.org/10.1017/jfm.2020.295 doi: 10.1017/jfm.2020.295
![]() |
[120] |
Wang Z, Yu B, Chen H, et al. (2018) Scaling vortex breakdown mechanism based on viscous effect in shock cylindrical bubble interaction. Phys Fluids 30: 126103. https://doi.org/10.1063/1.5051463 doi: 10.1063/1.5051463
![]() |
[121] |
Ding J, Si T, Yang J, et al. (2017) Measurement of a richtmyer-meshkov instability at an air-sf 6 interface in a semiannular shock tube. Phys Rev Lett 119: 014501. https://doi.org/10.1103/PhysRevLett.119.014501 doi: 10.1103/PhysRevLett.119.014501
![]() |
[122] |
Cook AW, Dimotakis PE (2001) Transition stages of rayleigh–taylor instability between miscible fluids. J Fluid Mech 443: 69–99. https://doi.org/10.1017/S0022112002007802 doi: 10.1017/S0022112002007802
![]() |
[123] |
Cook AW, Cabot W, Miller PL (2004) The mixing transition in rayleigh–taylor instability. J Fluid Mech 511: 333–362. https://doi.org/10.1017/S0022112004009681 doi: 10.1017/S0022112004009681
![]() |
[124] |
Ristorcelli J, Clark T (2004) Rayleigh–taylor turbulence: self-similar analysis and direct numerical simulations. J Fluid Mech 507: 213–253. https://doi.org/10.1017/S0022112004008286 doi: 10.1017/S0022112004008286
![]() |
[125] |
Samtaney R, Zabusky NJ (1993) On shock polar analysis and analytical expressions for vorticity deposition in shock-accelerated density-stratified interfaces. Phys Fluids A: Fluid Dyn 5: 1285–1287. https://doi.org/10.1063/1.858618 doi: 10.1063/1.858618
![]() |
[126] |
Marugan-Cruz C, Rodriguez-Rodriguez J, Martinez-Bazan C (2013) Formation regimes of vortex rings in negatively buoyant starting jets. J Fluid Mech 716: 470–486. https://doi.org/10.1017/jfm.2012.554 doi: 10.1017/jfm.2012.554
![]() |
[127] |
Soteriou MC, Ghoniem AF (1995) Effects of the free stream density ratio on free and forced spatially developing shear layers. Phys Fluids 7: 2036–2051. https://doi.org/10.1063/1.868451 doi: 10.1063/1.868451
![]() |
[128] |
Livescu D, Ristorcelli J, Petersen M, et al. (2010) New phenomena in variable-density rayleigh–taylor turbulence. Phys Scripta 2010: 014015. https://doi.org/10.1088/0031-8949/2010/T142/014015 doi: 10.1088/0031-8949/2010/T142/014015
![]() |
[129] |
Zhai Z, Si T, Luo X, et al. (2011) On the evolution of spherical gas interfaces accelerated by a planar shock wave. Phys Fluids 23: 084104. https://doi.org/10.1063/1.3623272 doi: 10.1063/1.3623272
![]() |
[130] |
Si T, Long T, Zhai Z, et al. (2015) Experimental investigation of cylindrical converging shock waves interacting with a polygonal heavy gas cylinder. J Fluid Mech 784: 225–251. https://doi.org/10.1017/jfm.2015.581 doi: 10.1017/jfm.2015.581
![]() |
[131] |
Luo X, Wang M, Si T, et al. (2015) On the interaction of a planar shock with an polygon. J Fluid Mech 773: 366–394. https://doi.org/10.1017/jfm.2015.257 doi: 10.1017/jfm.2015.257
![]() |
[132] |
Ding J, Si T, Chen M, et al. (2017) On the interaction of a planar shock with a three-dimensional light gas cylinder. J Fluid Mech 828: 289–317. https://doi.org/10.1017/jfm.2017.528 doi: 10.1017/jfm.2017.528
![]() |
[133] |
Li D, Guan B, Wang G (2022) Effects of interface diffusion and shock strength on shock-accelerated sf6 cylinder. Phys Fluids 34: 076109. https://doi.org/10.1063/5.0099353 doi: 10.1063/5.0099353
![]() |
[134] |
Vorobieff P, Rightley PM, Benjamin RF (1998) Power-law spectra of incipient gas-curtain turbulence. Phys Rev Lett 81: 2240. https://doi.org/10.1103/PhysRevLett.81.2240 doi: 10.1103/PhysRevLett.81.2240
![]() |
[135] |
Niederhaus JH, Greenough J, Oakley J, et al. (2008) A computational parameter study for the three-dimensional shock–bubble interaction. J Fluid Mech 594: 85–124. https://doi.org/10.1017/S0022112007008749 doi: 10.1017/S0022112007008749
![]() |
[136] |
Lombardini M, Pullin D, Meiron D (2012) Transition to turbulence in shock-driven mixing: a mach number study. J Fluid Mech 690: 203–226. https://doi.org/10.1017/jfm.2011.425 doi: 10.1017/jfm.2011.425
![]() |
[137] |
Oggian T, Drikakis D, Youngs D, et al. (2015) Computing multi-mode shock-induced compressible turbulent mixing at late times. J Fluid Mech 779: 411–431. https://doi.org/10.1017/jfm.2015.392 doi: 10.1017/jfm.2015.392
![]() |
[138] |
Liu H, Yu B, Zhang B, et al. (2020) On mixing enhancement by secondary baroclinic vorticity in shock bubble interaction. J Fluid Mech 931: A17. https://doi.org/10.1017/jfm.2021.923 doi: 10.1017/jfm.2021.923
![]() |
[139] |
Li Y, Wang Z, Yu B, et al. (2019) Gaussian models for late-time evolution of two-dimensional shock–light cylindrical bubble interaction. Shock Waves 1–16. https://doi.org/10.1007/s00193-019-00928-w doi: 10.1007/s00193-019-00928-w
![]() |
[140] |
Thornber B, Griffond J, Poujade O, et al. (2017) Late-time growth rate, mixing, and anisotropy in the multimode narrowband richtmyer–meshkov instability: The θ-group collaboration. Phys Fluids 29: 105107. https://doi.org/10.1063/1.4993464 doi: 10.1063/1.4993464
![]() |
[141] |
Li H, He Z, Zhang Y, et al. (2019) On the role of rarefaction/compression waves in richtmyer-meshkov instability with reshock. Phys Fluids 31: 054102. https://doi.org/10.1063/1.5083796 doi: 10.1063/1.5083796
![]() |
[142] |
Bin Y, Xiao M, Shi Y, et al. (2021) A new idea to predict reshocked richtmyer–meshkov mixing: constrained large-eddy simulation. J Fluid Mech 918: R1. https://doi.org/10.1017/jfm.2021.332 doi: 10.1017/jfm.2021.332
![]() |
[143] |
Ruan YC, Zhang YS, Tian BL, et al. (2020) Density-ratio-invariant mean-species profile of classical rayleigh-taylor mixing. Phys Rev Fluids 5: 054501. https://doi.org/10.1103/PhysRevFluids.5.054501 doi: 10.1103/PhysRevFluids.5.054501
![]() |
[144] |
Yu B, Liu H, Liu H (2021) Scaling behavior of density gradient accelerated mixing rate in shock bubble interaction. Phys Rev Fluids 6: 064502. https://doi.org/10.1103/PhysRevFluids.6.064502 doi: 10.1103/PhysRevFluids.6.064502
![]() |
[145] |
Yu B, Li L, Xu H, et al. (2022) Effects of reynolds number and schmidt number on variable density mixing in shock bubble interaction. Acta Mech Sinica 38: 121256. https://doi.org/10.1007/s10409-022-09011-9 doi: 10.1007/s10409-022-09011-9
![]() |
[146] |
Tian Y, Jaberi FA, Li Z, et al. (2017) Numerical study of variable density turbulence interaction with a normal shock wave. J Fluid Mech 829: 551–588. https://doi.org/10.1017/jfm.2017.542 doi: 10.1017/jfm.2017.542
![]() |
[147] |
Wong ML, Livescu D, Lele SK (2019) High-resolution navier-stokes simulations of richtmyer-meshkov instability with reshock. Phys Rev Fluids 4: 104609. https://doi.org/10.1103/PhysRevFluids.4.104609 doi: 10.1103/PhysRevFluids.4.104609
![]() |
[148] |
Livescu D, Ryu J (2016) Vorticity dynamics after the shock–turbulence interaction. Shock Waves 26: 241–251. https://doi.org/10.1007/s00193-015-0580-5 doi: 10.1007/s00193-015-0580-5
![]() |
[149] |
Aslangil D, Livescu D, Banerjee A (2020) Variable-density buoyancy-driven turbulence with asymmetric initial density distribution. Physica D 406: 132444. https://doi.org/10.1016/j.physd.2020.132444 doi: 10.1016/j.physd.2020.132444
![]() |
[150] |
Tian Y, Jaberi FA, Livescu D (2019) Density effects on post-shock turbulence structure and dynamics. J Fluid Mech 880: 935–968. https://doi.org/10.1017/jfm.2019.707 doi: 10.1017/jfm.2019.707
![]() |
[151] |
Orlicz G, Balasubramanian S, Prestridge K (2013)Incident shock mach number effects on richtmyer-meshkov mixing in a heavy gas layer. Phys Fluids 25: 114101. https://doi.org/10.1063/1.4827435 doi: 10.1063/1.4827435
![]() |
[152] |
Tomkins C, Kumar S, Orlicz G, et al. (2008) An experimental investigation of mixing mechanisms in shock-accelerated flow. J Fluid Mech 611: 131–150. https://doi.org/10.1017/S0022112008002723 doi: 10.1017/S0022112008002723
![]() |
[153] |
Marble FE, Zukoski EE, Jacobs JW, et al. (1990) Shock enhancement and control of hypersonic mixing and combustion. AIAA Pap 1981: 1990. https://doi.org/10.2514/6.1990-1981 doi: 10.2514/6.1990-1981
![]() |
[154] |
Ottino JM (1990) Mixing, chaotic advection, and turbulence. Annu Rev Fluid Mech 22: 207–254. https://doi.org/10.1146/annurev.fl.22.010190.001231 doi: 10.1146/annurev.fl.22.010190.001231
![]() |
[155] |
Sreenivasan KR (2019) Turbulent mixing: A perspective. P Natl Acad Sci 116: 18 175–18 183. https://doi.org/10.1073/pnas.1800463115 doi: 10.1073/pnas.1800463115
![]() |
[156] |
Ranjan D, Oakley J, Bonazza R (2011) Shock-bubble interactions. Annu Rev Fluid Mech 43: 117–140. https://doi.org/10.1146/annurev-fluid-122109-160744 doi: 10.1146/annurev-fluid-122109-160744
![]() |
[157] |
Mulla IA, Hardalupas Y (2022) Measurement of instantaneous fully 3d scalar dissipation rate in a turbulent swirling flow. Exp Fluids 63: 173. https://doi.org/10.1007/s00348-022-03518-2 doi: 10.1007/s00348-022-03518-2
![]() |
1. | Jun Zhang, Ranran Wang, Yijun Lu, Jiandong Huang, Prediction of Compressive Strength of Geopolymer Concrete Landscape Design: Application of the Novel Hybrid RF–GWO–XGBoost Algorithm, 2024, 14, 2075-5309, 591, 10.3390/buildings14030591 | |
2. | Shahab Hosseini, Shima Entezam, Behshad Jodeiri Shokri, Ali Mirzaghorbanali, Hadi Nourizadeh, Amin Motallebiyan, Alireza Entezam, Kevin McDougall, Warna Karunasena, Naj Aziz, Predicting grout’s uniaxial compressive strength (UCS) for fully grouted rock bolting system by applying ensemble machine learning techniques, 2024, 36, 0941-0643, 18387, 10.1007/s00521-024-10128-y | |
3. | Qiong Tian, Yijun Lu, Ji Zhou, Shutong Song, Liming Yang, Tao Cheng, Jiandong Huang, Compressive strength of waste-derived cementitious composites using machine learning, 2024, 63, 1605-8127, 10.1515/rams-2024-0008 | |
4. | Areeba Ishtiaq, Kashif Munir, Ali Raza, Nagwan Abdel Samee, Mona M. Jamjoom, Zahid Ullah, Product Helpfulness Detection With Novel Transformer Based BERT Embedding and Class Probability Features, 2024, 12, 2169-3536, 55905, 10.1109/ACCESS.2024.3390605 | |
5. | Sesha Choudary Yeluri, Karan Singh, Akshay Kumar, Yogesh Aggarwal, Parveen Sihag, Estimation of Compressive Strength of Rubberised Slag Based Geopolymer Concrete Using Various Machine Learning Techniques Based Models, 2024, 2228-6160, 10.1007/s40996-024-01569-5 | |
6. | Qiong Tian, Yijun Lu, Ji Zhou, Shutong Song, Liming Yang, Tao Cheng, Jiandong Huang, Supplementary cementitious materials-based concrete porosity estimation using modeling approaches: A comparative study of GEP and MEP, 2024, 63, 1605-8127, 10.1515/rams-2023-0189 | |
7. | Xuyang Shi, Shuzhao Chen, Qiang Wang, Yijun Lu, Shisong Ren, Jiandong Huang, Mechanical Framework for Geopolymer Gels Construction: An Optimized LSTM Technique to Predict Compressive Strength of Fly Ash-Based Geopolymer Gels Concrete, 2024, 10, 2310-2861, 148, 10.3390/gels10020148 | |
8. | Ranran Wang, Jun Zhang, Yijun Lu, Jiandong Huang, Towards Designing Durable Sculptural Elements: Ensemble Learning in Predicting Compressive Strength of Fiber-Reinforced Nano-Silica Modified Concrete, 2024, 14, 2075-5309, 396, 10.3390/buildings14020396 | |
9. | Fei Zhu, Xiangping Wu, Yijun Lu, Jiandong Huang, Understanding Penetration Attenuation of Permeable Concrete: A Hybrid Artificial Intelligence Technique Based on Particle Swarm Optimization, 2024, 14, 2075-5309, 1173, 10.3390/buildings14041173 | |
10. | Seyed Amir Mohammad Lahaghi, Behrooz Zaker, Gevork B. Gharehpetian, Venera Nurmanova, Mehdi Bagheri, 2024, A Hybrid Approach Based on LMP and VCG Mechanism to Create a Positive Interaction Between Distribution Companies and Owners of DG Units, 979-8-3503-6161-2, 192, 10.1109/icSmartGrid61824.2024.10578141 | |
11. | Feng Bin, Shahab Hosseini, Jie Chen, Pijush Samui, Hadi Fattahi, Danial Jahed Armaghani, Proposing Optimized Random Forest Models for Predicting Compressive Strength of Geopolymer Composites, 2024, 9, 2412-3811, 181, 10.3390/infrastructures9100181 | |
12. | Qiang Wang, Tao Cheng, Yijun Lu, Haichuan Liu, Runhua Zhang, Jiandong Huang, Underground Mine Safety and Health: A Hybrid MEREC–CoCoSo System for the Selection of Best Sensor, 2024, 24, 1424-8220, 1285, 10.3390/s24041285 | |
13. | Ranran Wang, Jun Zhang, Yijun Lu, Shisong Ren, Jiandong Huang, Towards a Reliable Design of Geopolymer Concrete for Green Landscapes: A Comparative Study of Tree-Based and Regression-Based Models, 2024, 14, 2075-5309, 615, 10.3390/buildings14030615 | |
14. | Zhiguo Chang, Xuyang Shi, Kaidan Zheng, Yijun Lu, Yunhui Deng, Jiandong Huang, Soft Computing Techniques to Model the Compressive Strength in Geo-Polymer Concrete: Approaches Based on an Adaptive Neuro-Fuzzy Inference System, 2024, 14, 2075-5309, 3505, 10.3390/buildings14113505 | |
15. | Runhua Zhang, Tao Cheng, Yijun Lu, Hao Luo, Jiandong Huang, Evaluating and correlating asphalt binder and mixture fatigue properties considering aging conditions, 2024, 436, 09500618, 136356, 10.1016/j.conbuildmat.2024.136356 | |
16. | Qiong Tian, Yijun Lu, Ji Zhou, Shutong Song, Liming Yang, Tao Cheng, Jiandong Huang, Exploring the viability of AI-aided genetic algorithms in estimating the crack repair rate of self-healing concrete, 2024, 63, 1605-8127, 10.1515/rams-2023-0179 | |
17. | Muhammad Fawad, Hisham Alabduljabbar, Furqan Farooq, Taoufik Najeh, Yaser Gamil, Bilal Ahmed, Indirect prediction of graphene nanoplatelets-reinforced cementitious composites compressive strength by using machine learning approaches, 2024, 14, 2045-2322, 10.1038/s41598-024-64204-3 | |
18. | Tran Nhat Minh, Nguyen Tan Khoa, Nguyen Ninh Thuy, Le Anh Tuan, Evaluating Flexural Strength of Steel Fiber Reinforced Geopolymer Concrete using the ResNet Approach and Sensitivity Analysis, 2024, 14, 1792-8036, 18099, 10.48084/etasr.8912 | |
19. | Enhui Li, Zixi Wang, Jin Liu, Jiandong Huang, Sustainable Smart Education Based on AI Models Incorporating Firefly Algorithm to Evaluate Further Education, 2024, 16, 2071-1050, 10845, 10.3390/su162410845 | |
20. | Xiaoyan Wang, Yantao Zhong, Fei Zhu, Jiandong Huang, Digital Industrial Design Method in Architectural Design by Machine Learning Optimization: Towards Sustainable Construction Practices of Geopolymer Concrete, 2024, 14, 2075-5309, 3998, 10.3390/buildings14123998 | |
21. | Fahimeh Ahmadi, Mohsen Hajihassani, Stefanos Papanikolaou, Panagiotis G. Asteris, Data-driven optimized XGBoost and LightGBM for predicting the Judd-Ofelt parameters in rare-earth doped phosphate-based glasses, 2025, 02728842, 10.1016/j.ceramint.2025.01.177 | |
22. | Malika Ouacifi, Karim Ferroudji, Fouad Chebbara, Mounir Amir, Mohamed Lashab, Raed A. Abd-Alhameed, 2024, A Decision Tree Model for Inset-Fed Microstrip Patch Antenna, 979-8-3503-8848-0, 1, 10.1109/ECTE-Tech62477.2024.10851155 | |
23. | Hongchao Li, Shahab Hosseini, Behrouz Gordan, Jian Zhou, Sajid Ullah, Dimensionless Machine Learning: Dimensional Analysis to Improve LSSVM and ANN models and predict bearing capacity of circular foundations, 2025, 58, 1573-7462, 10.1007/s10462-024-11099-1 | |
24. | Wei Ge, Ramindu De Silva, Yanan Fan, Scott A. Sisson, Martina H. Stenzel, Machine Learning in Polymer Research, 2025, 0935-9648, 10.1002/adma.202413695 | |
25. | Ezzeddin Bakhtavar, Shahab Hosseini, Haroon R. Mian, Kasun Hewage, Rehan Sadiq, Robust prediction of water arsenic levels downstream of gold mines affected by acid mine drainage using hybrid ensemble machine learning and soft computing, 2025, 489, 03043894, 137665, 10.1016/j.jhazmat.2025.137665 | |
26. | Charuta Waghmare, Mohammad Gulfam Pathan, Syed Aamir Hussain, Tripti Gupta, Anshul Nikhade, Monali Wagh, Khalid Ansari, Machine learning based prediction of compressive strength in roller compacted concrete: a comparative study with PDP analysis, 2025, 1563-0854, 10.1007/s42107-025-01310-1 | |
27. | Patryk Ziolkowski, Influence of Optimization Algorithms and Computational Complexity on Concrete Compressive Strength Prediction Machine Learning Models for Concrete Mix Design, 2025, 18, 1996-1944, 1386, 10.3390/ma18061386 | |
28. | Mohammed Salem Atoum, Ala Abdulsalam Alarood, Eesa Alsolami, Adamu Abubakar, Ahmad K. Al Hwaitat, Izzat Alsmadi, Cybersecurity Intelligence Through Textual Data Analysis: A Framework Using Machine Learning and Terrorism Datasets, 2025, 17, 1999-5903, 182, 10.3390/fi17040182 | |
29. | Yin Junjia, Aidi Hizami Alias, Nuzul Azam Haron, Nabilah Abu Bakar, Predicting Building Primary Energy Use Based on Machine Learning: Evidence from Portland, 2024, 11, 2409-9821, 124, 10.15377/2409-9821.2024.11.7 | |
30. | David Sinkhonde, Derrick Mirindi, Ismael Dabakuyo, Tajebe Bezabih, Destine Mashava, Frederic Mirindi, Applications of machine learning algorithms on the compressive strength of laterite blocks made with metakaolin-based geopolymer and sugarcane molasses, 2025, 3, 29497507, 100212, 10.1016/j.wmb.2025.100212 |
Author | Year | Technique | Number of data |
Huang et al. [46] | 2021 | SVM | 114 |
Sarir et al. [47] | 2019 | GEP | 303 |
Balf et al. [48] | 2021 | DEA | 114 |
Ahmad et al. [49] | 2021 | GEP, ANN, DT | 642 |
Azimi-Pour et al. [50] | 2020 | SVM | - |
Saha et al. [51] | 2020 | SVM | 115 |
Hahmansouri et al. [52] | 2020 | GEP | 351 |
Hahmansouri et al. [53] | 2019 | GEP | 54 |
Aslam et al. [54] | 2020 | GEP | 357 |
Farooq et al. [55] | 2020 | RF and GEP | 357 |
Asteris and Kolovos [56] | 2019 | ANN | 205 |
Selvaraj and Sivaraman [57] | 2019 | IREMSVM-FR with RSM | 114 |
Zhang et al. [58] | 2019 | RF | 131 |
Kaveh et al. [59] | 2018 | M5MARS | 114 |
Sathyan et al. [60] | 2018 | RKSA | 40 |
Vakhshouri and Nejadi [61] | 2018 | ANFIS | 55 |
Belalia Douma et al. [62] | 2017 | ANN | 114 |
Abu Yaman et al. [63] | 2017 | ANN | 69 |
Ahmad et al. [64] | 2021 | GEP, DT and Bagging | 270 |
Farooq et al. [65] | 2021 | ANN, bagging and boosting | 1030 |
Bušić et al. [66] | 2020 | MV | 21 |
Javad et al. [67] | 2020 | GEP | 277 |
Nematzadeh et al. [68] | 2020 | RSM, GEP | 108 |
Güçlüer et al. [69] | 2021 | ANN, SVM, DT | 100 |
Ahmad et al. [70] | 2021 | ANN, DT, GB | 207 |
Asteris et al. [71] | 2021 | ANN, GPR, MARS | 1030 |
Emad et al. [72] | 2022 | ANN, M5P, | 306 |
Shen et al. [73] | 2022 | XGBoost, AdaBoost, and Bagging | 372 |
Kuma et al. [74] | 2022 | GPR, SVMR | 194 |
Jaf et al. [75] | 2023 | NLR, MLR, ANN | 236 |
Mahmood et al. [76] | 2023 | NLR, M5P, ANN | 280 |
Ali et al. [77] | 2023 | LR, MLR, NLR, PQ, IA, FQ | 420 |
SVM: Support vector machine; GEP: Gene expression programming; ANN: Artificial neural network; DT: Decision tree; RF: Random Fores; DEA: Data envelopment analysis; RSM: Response surface methodology; ANFIS: Adaptive neuro fuzzy inference system; MV: Micali-Vazirani algorithm; RKSA: Retina key scheduling algorithm; GB: gradient boosting; GPR: Gaussian Process Regression; MARS: Multivariate Adaptive Regression Splines; SVMR: Support Vector Machine Regression; NLR: Nonlinear regression; MLR: Multi-linear regression; LR: linear regression; PQ: pure quadratic; IA: interaction; FQ: full quadratic. |
Parameter | Symbol | Unit | Median | Min | Mean | Max | StD | |
Inputs | Fly ash | FA | kg/m3 | 170 | 0 | 178.265 | 523 | 173.979 |
GGBS | GGBS | kg/m3 | 225 | 0 | 209.831 | 450 | 163.271 | |
Na2SiO3 | Na2SiO3 | kg/m3 | 100 | 18 | 104.059 | 342 | 44.9000 | |
NaOH | NaOH | kg/m3 | 64 | 6.300 | 60.042 | 147 | 30.391 | |
Fine aggregate | FAg | kg/m3 | 721 | 459 | 731.209 | 1360 | 138.078 | |
Gravel 4–10 mm | Gravel 4–10 | kg/m3 | 309 | 0 | 335.828 | 1293.400 | 373.884 | |
Gravel 10–20 mm | Gravel 10–20 | kg/m3 | 815 | 0 | 741.556 | 1298 | 361.336 | |
Water/solids ratio | WS | N/A | 0.330 | 0.120 | 0.330 | 0.630 | 0.095 | |
NaOH molarity | NaOH molarity | N/A | 10 | 1 | 8.193 | 20 | 4.596 | |
Output | Compressive strength of geo-polymer concrete | CSGPoC | MPa | 43 | 10 | 44.474 | 86.080 | 18.010 |
Author | Year | Technique | Number of data |
Huang et al. [46] | 2021 | SVM | 114 |
Sarir et al. [47] | 2019 | GEP | 303 |
Balf et al. [48] | 2021 | DEA | 114 |
Ahmad et al. [49] | 2021 | GEP, ANN, DT | 642 |
Azimi-Pour et al. [50] | 2020 | SVM | - |
Saha et al. [51] | 2020 | SVM | 115 |
Hahmansouri et al. [52] | 2020 | GEP | 351 |
Hahmansouri et al. [53] | 2019 | GEP | 54 |
Aslam et al. [54] | 2020 | GEP | 357 |
Farooq et al. [55] | 2020 | RF and GEP | 357 |
Asteris and Kolovos [56] | 2019 | ANN | 205 |
Selvaraj and Sivaraman [57] | 2019 | IREMSVM-FR with RSM | 114 |
Zhang et al. [58] | 2019 | RF | 131 |
Kaveh et al. [59] | 2018 | M5MARS | 114 |
Sathyan et al. [60] | 2018 | RKSA | 40 |
Vakhshouri and Nejadi [61] | 2018 | ANFIS | 55 |
Belalia Douma et al. [62] | 2017 | ANN | 114 |
Abu Yaman et al. [63] | 2017 | ANN | 69 |
Ahmad et al. [64] | 2021 | GEP, DT and Bagging | 270 |
Farooq et al. [65] | 2021 | ANN, bagging and boosting | 1030 |
Bušić et al. [66] | 2020 | MV | 21 |
Javad et al. [67] | 2020 | GEP | 277 |
Nematzadeh et al. [68] | 2020 | RSM, GEP | 108 |
Güçlüer et al. [69] | 2021 | ANN, SVM, DT | 100 |
Ahmad et al. [70] | 2021 | ANN, DT, GB | 207 |
Asteris et al. [71] | 2021 | ANN, GPR, MARS | 1030 |
Emad et al. [72] | 2022 | ANN, M5P, | 306 |
Shen et al. [73] | 2022 | XGBoost, AdaBoost, and Bagging | 372 |
Kuma et al. [74] | 2022 | GPR, SVMR | 194 |
Jaf et al. [75] | 2023 | NLR, MLR, ANN | 236 |
Mahmood et al. [76] | 2023 | NLR, M5P, ANN | 280 |
Ali et al. [77] | 2023 | LR, MLR, NLR, PQ, IA, FQ | 420 |
SVM: Support vector machine; GEP: Gene expression programming; ANN: Artificial neural network; DT: Decision tree; RF: Random Fores; DEA: Data envelopment analysis; RSM: Response surface methodology; ANFIS: Adaptive neuro fuzzy inference system; MV: Micali-Vazirani algorithm; RKSA: Retina key scheduling algorithm; GB: gradient boosting; GPR: Gaussian Process Regression; MARS: Multivariate Adaptive Regression Splines; SVMR: Support Vector Machine Regression; NLR: Nonlinear regression; MLR: Multi-linear regression; LR: linear regression; PQ: pure quadratic; IA: interaction; FQ: full quadratic. |
Parameter | Symbol | Unit | Median | Min | Mean | Max | StD | |
Inputs | Fly ash | FA | kg/m3 | 170 | 0 | 178.265 | 523 | 173.979 |
GGBS | GGBS | kg/m3 | 225 | 0 | 209.831 | 450 | 163.271 | |
Na2SiO3 | Na2SiO3 | kg/m3 | 100 | 18 | 104.059 | 342 | 44.9000 | |
NaOH | NaOH | kg/m3 | 64 | 6.300 | 60.042 | 147 | 30.391 | |
Fine aggregate | FAg | kg/m3 | 721 | 459 | 731.209 | 1360 | 138.078 | |
Gravel 4–10 mm | Gravel 4–10 | kg/m3 | 309 | 0 | 335.828 | 1293.400 | 373.884 | |
Gravel 10–20 mm | Gravel 10–20 | kg/m3 | 815 | 0 | 741.556 | 1298 | 361.336 | |
Water/solids ratio | WS | N/A | 0.330 | 0.120 | 0.330 | 0.630 | 0.095 | |
NaOH molarity | NaOH molarity | N/A | 10 | 1 | 8.193 | 20 | 4.596 | |
Output | Compressive strength of geo-polymer concrete | CSGPoC | MPa | 43 | 10 | 44.474 | 86.080 | 18.010 |