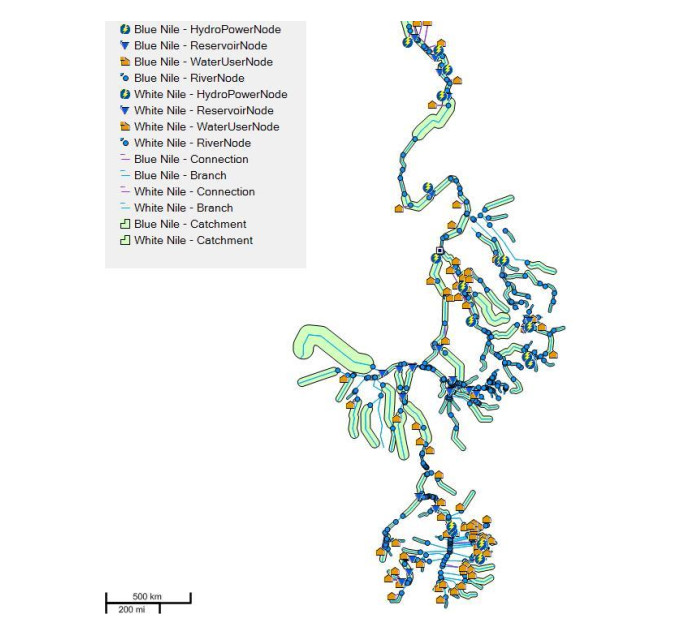
One of the most significant challenges of the mining industry is resource yield estimation from visual data. An example would be identification of the rock chunk distribution parameters in an open pit. Solution of this task allows one to estimate blasting quality and other parameters of open-pit mining. This task is of the utmost importance, as it is critical to achieving optimal operational efficiency, reducing costs and maximizing profits in the mining industry. The mentioned task is known as rock fragmentation estimation and is typically tackled using computer vision techniques like instance segmentation or semantic segmentation. These problems are often solved using deep learning convolutional neural networks. One of the key requirements for an industrial application is often the need for real-time operation. Fast computation and accurate results are required for practical tasks. Thus, the efficient utilization of computing power to process high-resolution images and large datasets is essential. Our survey is focused on the recent advancements in rock fragmentation, blast quality estimation, particle size distribution estimation and other related tasks. We consider most of the recent results in this field applied to open-pit, conveyor belts and other types of work conditions. Most of the reviewed papers cover the period of 2018-2023. However, the most significant of the older publications are also considered. A review of publications reveals their specificity, promising trends and best practices in this field. To place the rock fragmentation problems in a broader context and propose future research topics, we also discuss state-of-the-art achievements in real-time computer vision and parallel implementations of neural networks.
Citation: Mikhail V. Ronkin, Elena N. Akimova, Vladimir E. Misilov. Review of deep learning approaches in solving rock fragmentation problems[J]. AIMS Mathematics, 2023, 8(10): 23900-23940. doi: 10.3934/math.20231219
[1] | Stefano De Falco, Giulia Fiorentino . The GERD dam in the water dispute between Ethiopia, Sudan and Egypt. A scenario analysis in an ecosystem approach between physical and geopolitical geography. AIMS Geosciences, 2022, 8(2): 233-253. doi: 10.3934/geosci.2022014 |
[2] | Stanley C. Nwokebuihe, Abdulrahman M. Alotaibi, Adel Elkrry, Evgeniy V. Torgashov, Neil L. Anderson . Dam Seepage Investigation of an Earthfill Dam in Warren County, Missouri Using Geophysical Methods. AIMS Geosciences, 2017, 3(1): 1-13. doi: 10.3934/geosci.2017.1.1 |
[3] | Khalid Ahmad, Umair Ali, Khalid Farooq, Syed Kamran Hussain Shah, Muhammad Umar . Assessing the stability of the reservoir rim in moraine deposits for a mega RCC dam. AIMS Geosciences, 2024, 10(2): 290-311. doi: 10.3934/geosci.2024017 |
[4] | Serin Değerli Şimşek, Ömer Faruk Çapar, Evren Turhan . Assessment of Hydrological Drought Index change over long period (1990–2020): The case of İskenderun Gönençay Stream, Türkiye. AIMS Geosciences, 2023, 9(3): 441-454. doi: 10.3934/geosci.2023024 |
[5] | Piero Farabollini, Francesca Romana Lugeri, Emy Fuffa . Environmental knowledge, risk prevention, renaissance suggestions in the time of COVID-19. AIMS Geosciences, 2020, 6(3): 258-270. doi: 10.3934/geosci.2020015 |
[6] | Evgeniy V. Torgashov, Aleksandra V. Varnavina . Site Characterization during Bridge Foundation Construction Using Electrical Resistivity Tomography. AIMS Geosciences, 2016, 2(3): 201-213. doi: 10.3934/geosci.2016.3.201 |
[7] | Valentina E. Albanese, Teresa Graziano . Disentangling rural smartness in European policies: a critical overview of the Smart Village concept. AIMS Geosciences, 2024, 10(4): 882-906. doi: 10.3934/geosci.2024041 |
[8] | Luis M. Sandoval, Philip C. Goodell, Hector Gonzalez-Huizar, Munazzam Ali Mahar . Rayleigh wave group velocity model of the southeast flank of the Rio Grande Rift using Cross-Correlation. AIMS Geosciences, 2018, 4(1): 1-20. doi: 10.3934/geosci.2018.1.1 |
[9] | Ana Casado, Natalia C López . Comparison of synthetic unit hydrograph methods for flood assessment in a dryland, poorly gauged basin (Napostá Grande, Argentina). AIMS Geosciences, 2025, 11(1): 27-46. doi: 10.3934/geosci.2025003 |
[10] | Joseph J. Kelly, Christopher I. McDermott . Numerical modelling of a deep closed-loop geothermal system: evaluating the Eavor-Loop. AIMS Geosciences, 2022, 8(2): 175-212. doi: 10.3934/geosci.2022011 |
One of the most significant challenges of the mining industry is resource yield estimation from visual data. An example would be identification of the rock chunk distribution parameters in an open pit. Solution of this task allows one to estimate blasting quality and other parameters of open-pit mining. This task is of the utmost importance, as it is critical to achieving optimal operational efficiency, reducing costs and maximizing profits in the mining industry. The mentioned task is known as rock fragmentation estimation and is typically tackled using computer vision techniques like instance segmentation or semantic segmentation. These problems are often solved using deep learning convolutional neural networks. One of the key requirements for an industrial application is often the need for real-time operation. Fast computation and accurate results are required for practical tasks. Thus, the efficient utilization of computing power to process high-resolution images and large datasets is essential. Our survey is focused on the recent advancements in rock fragmentation, blast quality estimation, particle size distribution estimation and other related tasks. We consider most of the recent results in this field applied to open-pit, conveyor belts and other types of work conditions. Most of the reviewed papers cover the period of 2018-2023. However, the most significant of the older publications are also considered. A review of publications reveals their specificity, promising trends and best practices in this field. To place the rock fragmentation problems in a broader context and propose future research topics, we also discuss state-of-the-art achievements in real-time computer vision and parallel implementations of neural networks.
Population growth and economic development have sparked water demands for hydropower and irrigation developments in the river basin [1]. On the basis of utilizing these water resources for socioeconomic transformation and the alleviation of poverty, the Government of Ethiopia, with the support of the United Sates Government Bureau of Reclamation (USBR) [2], made the first assessment of potential irrigation and hydropower in Ethiopia's Abbay River basin in 1964. They identified that the basin has considerable irrigation and hydropower potential. The border dam site on the Nile River, which the USBR initially identified as having relatively less water storage at 14.5 billion cubic meters (BCM), a hydropower generation capacity of 800 mega watts (MW), and a height of approximately 80 meters above sea level (masl), is the current site of the Grand Ethiopian Renaissance Dam (GERD). The GERD is 1,874 km2 in area and 170 masl in height, with a 5,150 MW hydropower generation capacity and 74 BCM water storage capacities. The GERD's minimum operating level (MOL) is 590 masl. In contrast, the height of the downstream High Aswan Dam (HAD), also located on the Nile River but within Egypt, is 183 masl, with a hydropower generation capacity of 21,000 MW and a MOL of 147 masl. The HAD's full reservoir capacity is 164 BCM with an active storage capacity of 107 BCM [3,4,5].
Before implementing the USBR recommendations on the utilization of the Abbay River basin, the then Ethiopian Imperial regime was overthrown by the Dergue regime in 1974; subsequently, the Dergue regime was engaged in active war for seventeen years, from which guerilla fighters came to be known as the Tigray People Liberation Front (TPLF) and Eritrean Peoples Liberation Front (EPLF). In 1991, the TPLF led the coalition of the Ethiopian People's Revolutionary Democratic Front (EPRDF) and seized the capital city of Addis Ababa, while the EPLF controlled the city of Asmara. After reconstructing and rehabilitating the war-torn economies and infrastructures, in pursuit of the United Nations Millennium Development Goals (MDGs) to improve the lives of impoverished peoples by 2015, Ethiopia created the Plan for Accelerated and Sustained Development to End Poverty (PASDEP) under the EPRDF regime. The PASDEP was a medium-term development plan based on eight pillars, some of which were included in subsequent five-year plans. In line with Ethiopia's principle of exploiting indigenous resources, the PASDEP planned to construct three hydropower plants: the Tekeze, Gilbgel Gibe II, and Tana Belese plants. According to the Ministry of Finance and Economic Development (MoFED) [6], the PASDEP is the first of four comprehensive economic development plans launched since 2000 and was relatively effective until 2010 when, subsequently, the Growth and Transformation Plan I (GTP I), a five-year plan, was adopted by the MoFED to achieve the MDGs by 2015. Currently, through GTP I, the MoFED intends that Ethiopia should attain middle-income status during 2020–2023 and continue to prioritize infrastructure developments. For power generation, Ethiopia signed contracts for the GERD and the Gilgel Gibe III before the completion of GTP I. However, to commence construction of the GERD, Ethiopia was required to rely on its existing economic and financial capacities and improve development of its technical capacities [7,8,9], since constructing the GERD with financial and technical support from the international financial institution was not possible. The World Bank Operational Directive 7:50 requires the approval of downstream countries for any construction envisioned by an upstream country if the upstream country seeks financial and technical support from the World Bank [10]. Therefore, global and internal circumstances compounded Ethiopia to delay the construction of the GERD until 2011 (Nile Basin Initiative [3,4].
Upon the construction of the GERD, riparian states have not yet agreed on nor established a filling strategy for the GERD reservoir. Therefore, to inform on possible filling strategies, we use historical and projected streamflow data to examine whether two filling strategies of the GERD (five- and six-year policies) could harm the downstream HAD operations. We selected historical flow data that are representative of low, medium, and high flows. The study objective was to exhaustively compare the two filling strategies and determine whether they cause any significant harm to HAD, and instigate Egypt to invoke its drought management policy (DMP). The study does not cover post-filling management and operational strategies. Nonetheless, given the impacts of climate change, climate variability, and uncertainties with respect to the directions and magnitudes of future climate change, the need to coordinate the GERD and HAD is imperative for maintaining the equitable utilization of shared water resources and benefitting from them. In conclusion, we highlighted the associated behavioral perceived and endowment effects of gains and losses under hawkish and nationalist policy debates over these transboundary water resources.
The construction of the GERD in the Abbay River basin, which launched in 2011 [3,4,11], was anticipated to be a platform for cooperation among Ethiopia, Sudan, and Egypt. The benefits provided by the GERD include electricity trading, water flow regulation, and sediment and flood control. However, due to perceived and real associated impacts induced on the HAD, some of which were undesired, controversial issues have persisted among the riparian countries [12,13,14]. As a result, various GERD filling policies and potential impacts have been previously examined [15,16,17,18,19,20,21,22,23]. However, although studies do encourage cooperation and benefit sharing, they disagree on the potential impacts of GERD filling strategies on HAD. The related irrigation and hydropower potential of the Abbay River basin for Ethiopia have been illustrated [11,12,24], and the Nile River's climate sensitivity and variability have been documented [25,26]. Similarly, studies on other environmental and socioeconomic impacts of Nile River flow on the HAD have also been documented [27,28,29,30,31,32,33,34].
Yet the future state of climate change in the Abbay River basin remains uncertain [26,35,36]. Forecast models have used different simulations, including synthetic river flows, to forecast future states, but the directions and scope of future climate changes largely remain unclear. Moreover, it has been recognized that high spatial and temporal interannual variability are the main characteristics of the Ethiopian highlands and the Abbay River basin's climatic conditions [37,38,39] which puts pressure on the availability of the basin's water resources.
Meanwhile, in addition to climate change pressure on water resources, both the Abbay and Nile River basins are experiencing high population growth. As a result, energy access and food supply are among the major issues in the riparian states [1]. Rapid population growth, food insecurity, and urbanization promoted the riparian states to develop competitive water resource development plans [40,41]. The GERD is the largest among the numerous project plans developed by the riparian countries in recent history. As the construction of GERD led to intense debate among Ethiopia, Sudan, and Egypt, the GERD's filling policies and long-term operations have similarly ignited controversial debates and prolonged negotiation between the riparian states. Due to the challenges in coming to mutually acceptable agreements, Ethiopia unilaterally completed its first GERD filling in the summer of 2020.
The second round of GERD filling has been scheduled for the summer of 2021, yet the riparian countries have yet to reach to an agreement in terms of the filling strategy. Assuming that the parties do not reach an agreement or fail to establish frameworks before the filling period commences, Ethiopia will be required to subsequently conduct the second round of filling as originally scheduled since the activity of filling is not considered illicit according to existing international customary law or general principle. In fact, this second round, as well as the presumed upcoming third round of filling, are in accordance with international customary laws, as exemplified by two similar disputes; one between France and Spain over Lake Lanoux and another between Argentina and Uruguay regarding the construction of a pulp mill on the Uruguay River. In the first case, Spain objected and resisted the basin development in Lanoux Lake by France, which was carried out without prior agreement. Similarly, Argentina originally resisted and objected to the development of a pulp mill on the Uruguay River before reaching an agreement. In both cases, the international court dismissed the objections because they were neither backed by general principles nor customary law [42,43]. In both cases, the court demonstrated the inappropriateness of insisting on having a prior agreement, as it would otherwise curtail the state's right to act unilaterally and unconditionally within its jurisdiction. Considering this, Ethiopia is expected to pursue its second round of filling as originally planned. Therefore, assessing the impacts of the two shortest-timeframe GERD filling policies (five- and six-year policies) is essential to searching for potential interventions.
The Nile River is shared by 11 African riparian states. The Blue Nile (hereinafter the Abbay River) is major tributary of the Nile River, and its basin temperature spatial distribution is strongly related to altitude. Within the Abbay River basin, the highest temperatures are observed in the northwestern part of the basin (in the areas of Rihad, Dinder, Beles, and Dabus), where the maximum temperature is 28–38 ℃ and minimum temperature is 15–20 ℃. Lower temperatures are observed in the highlands of Ethiopia, that is, in the central and eastern parts of the basin, where the maximum and minimum temperature ranges from 12–20 ℃ and from −1 to 8 ℃, respectively. Potential evapotranspiration (PET) in the basin ranges between 1,056 and 2,232 mm per year. High PET is observed between 1,800 and 2,232 mm per year in the northwestern parts of the basin, that is, in Dinder, Rahad, and parts of the Beles and Didessa sub-basins. The eastern and southern parts have lower PET, ranging between 1,200 and 1,800 mm per year. The lowest PET (below 1,200 mm per year) is observed in parts of the highlands [38,44]
The basin has considerable inter-annual rainfall variability. It increases from approximately 1,000 mm near the Ethiopia and Sudan border to between 1,400 and 1,800 mm over parts of the upper basin, and exceeds 2,200 mm in some places in the south with a mean of approximately 1,420 mm/year. Around 85% of annual precipitation occurs during the summer, from July to October [45,46]. The basin contributes 54.5 BCM/year to the Nile River flow.
The basin's 11 riparian states: Burundi, the Democratic Republic of Congo, Egypt, Eritrea, Ethiopia, Kenya, Rwanda, South Sudan, Sudan, Tanzania, and Uganda. The Nile River is the result of two principal tributaries: the Abbay River and the White Nile, which originates in the Equatorial Lakes. The two rivers merge in Sudan near Khartoum. The Abbay River originates from a small spring called Gish Abay in the Ethiopian highlands, located 117 km from Lake Tana; however, multiple studies have proposed that the source of the Abbay River is Lake Tana itself. The Abbay River flows clockwise and enters a canyon through the Ethiopian gorge, joining the White Nile in Sudan. Monsoon rainfall contributes to the major rainy season in the Ethiopian plateau. Most of the water is produced upstream, and the downstream countries utilize significant portions of the river flow for both hydropower and irrigations (Please see Figure 1, for schematic view). For example, Ethiopia's present annual water consumption water from the Abbay River basin is less than 5% for irrigation and less than 3% for hydropower. These consumption rates have been a source of contention, but also have potential to be a source of collaboration among the riparian states [11,17,47,48,49]
This study employed a comprehensive analytical MIKE Hydro model, which simulated the Nile River from 2020 to 2060. The precipitation-runoff for every catchment area was simulated using the MIKE-NAM model. MIKE-NAM is used to conceptualizing the estimation and correct the bias based on the time series data of the climate research unit time series version 4.03 (CRU TS4.03) associated with potential evapotranspiration, precipitation, and temperature. In this study, MIKE-NAM observed the streamflow calibrated at each of the sub-catchments and developed a simulation of ungauged catchments, which aided the estimation of the streamflow at an outlet for each sub-catchment. The Nile River streamflow was required to meet the municipal and agricultural irrigation water demand, the power generation water requirement, and minimum monthly water flow requirements. The Nile Basin Decision Support System, which is generally tailored to the Nile River basin regime, has been used to combine temporal and spatial variability of precipitation and historical data of stream flow across the entire river basin; the major assumption of the MIKE Hydro model has been extensively reported in previous studies including those conducted by Yao and Georgakakos [50,51].
The sets of historical data were obtained from the NBI-ENTRO dataset from the Eastern Nile Technical Regional Office (ENTRO) [52]. The streamflow, hydrology, and hydropower models require inputs of monthly precipitation, daily mean temperature, and the diurnal temperature range. This historical period was selected to minimize the possible effects of climate change (i.e., post 2000). Accordingly, we purposefully selected the following scenarios for this study: a) two GERD filling inflow scenarios (average and dry flow sequence obtained from historical data), b) four of the HAD's starting water level conditions (obtained from historical water level conditions of the HAD), and c) different HAD drought management policies, adapted from [21].
The two GERD filling scenarios, which are five- and six-year filling policies (Figure 4), were analyzed as follows: i) a five-year annual filling policy that retains 4.5, 17.5, 17.5, 17.5, and 17.0 BCM each year by considering the average inflow conditions of the Abbay River from 1987 to 1992; ii) a six-year annual filling policy that retains 4.5, 15, 15, 15, 12, and 12 BCM of water by considering the dry inflow conditions of the Abbay River from 1979 to 1987. The filling was allowed in July, August, September (minimum), and October. The release in September was considered to be either minimal or zero to manage the filling of Sudan's Rosieres Dam during September.
The four different HAD starting water levels studied were 158.2,160.0,165.0,170.0, and 175.0 masl. Water levels abstracted from historical water level data were considered for both dry and average flow conditions. It should be noted that these water level conditions represent possible realizations of the historical water levels at the beginning of HAD filling. Finally, for different HAD drought management policies, the historical annual release from the HAD reservoir ranged between 35.0 BCM (representing the dry season of 1984) and 86.0 BCM (the wettest season of 1988) (Figure 2). Despite the release rate of 55.5 BCM/year indicated in various documents, the outflow from HAD varies between 35 BCM (dry year) and 86 BCM (wet year), which indicates the implementation of water management policies at HAD for flood and drought years. Furthermore, Wheeler et al. documented Egypt's drought management policy in their recent study; a 5–10% release reduction was reported during the drought year [25]. A similar magnitude (5–10%) was observed for the increase in release during wet years.
It is well known that examination of the impacts of filling on downstream countries during the filling period is complicated by the filling strategies' multifacetedness and correlations. Therefore, our study is based on the features and operations of two major factors: average historical water release (Figures 2, 6, and 8) and the starting water level at HAD (Figures 5 and 8), which was 178.4 MASL in August 2020 [5].
In the summer of 2020, Ethiopia completed the first round of GERD filling by securing 4.9 BCM of water. During all planned filling periods, a certain portion of the river flow is intended be diverted to fill the reservoir, while the rest makes its way downstream to the HAD through its turbine. The flow of the Abbay River differs from that observed without the GERD. With the existence of the GERD, water flow at the HAD decreases, and the hydropower generation at the HAD also declines due to the lowering of the hydraulic head on its turbine. However, the river flow will return to normalcy upon completion of GERD Reservoir filling [53].
Figure 2 shows the historical HAD release from 1970 to 2002, while Figure 3 demonstrates the HAD level without GERD, and Figures 3 and 8 show the HAD water level at various conditions. When the model flow is subjected to the historical flow data of 1970–2002 under the five-year strategy, the scale of the annual water shortfall in the HAD before and after the GERD could be calculated in relation to Egypt's historical average annual water release of 55.5 BCM. In the model, when the HAD reservoir received water based on the historical data series from 1987 to 1992 under the five-year strategy of 4.5, 17.5, 17.5, and 17.0 BCM, the filling of the GERD had no adverse impacts on Egypt (Figures 4 and 5). Moreover, the model shows that the GERD's storage would be full in five years. Upon achieving maximum storage capacity, the flow of the river resumed its regular flow pattern, and the HAD's storage recovered speedily. The simulation shows that the HAD storage capacity did not drop below its MOL, which is 60 BCM. Therefore, initiating the DMP of HAD would not be necessary under the five-year strategy, and Egypt could potentially maintain its historical average annual release of 55.5 BCM (Figures 5 and 6).
Similarly, based on the historical average annual flow from 1979–1987 and the six-year annual filling strategy of 4.5, 15, 15, 15, 12, and 12 BCM, modeling results show that the filling of the GERD had no adverse effect on the HAD reservoir, and that again, Egypt could potentially retain its annual release of 55.5 BCM without the need to invoke the DMP. The GERD was full after six years. According to the model results, a stable and regular flow of the river was established and the HAD recovered quickly to 74 BCM.
In both the five- and six-year scenarios, the HAD did not reach its lowest recorded reservoir level of 34.7 BCM (147 masl). Therefore, both the five- and six-year filling strategies would not call for the establishment of the DMP in Egypt. Furthermore, the likelihood of drought occurrence during the filling period would be considered remote; this is because drought conditions were historically observed only in two years, 1978 and 1987, and the probability of the re-occurrence of such conditions is estimated to occur only once in more than 20 years [51]. Although such a drought may occur during either of the two filling strategies, a dynamic filling model can be developed in the ongoing negotiations by using past scientific evidence to account for this possibility. Furthermore, the majority of climate models for the Abbay River basin exhibit wetter climate conditions in the future with high interannual variabilities of rainfall, while related studies have recommended the construction of additional storage in the Abbay River basin [26,53,54,55].
Based on the filling models, filling was allowed in July, August, September (minimum) and October. The release in September was considered to either be minimal or nonexistent, with regard to Sudan's Rosieres Dam filling during this period. Based on the five-year strategy under average inflow conditions, model results show that GERD storage volume was able to almost reach its full capacity of 69.5 BCM safely without affecting the HAD, as shown in Figure 5. Based on the six-year strategy and dry flow conditions the starting water level conditions of the HAD should be considered in the filling of the GERD. In the unlikely event of consecutive dry conditions such as those observed in 1980s, the filling of the GERD could be initiated if the initial water level of the HAD is maintained at 175 m or above to avoid significant negative consequences.
Using the average inflow conditions, the proposed GERD filling policy under the five-year strategy suggests that the HAD can be operated above the MOL of 147 m under all starting water level conditions (Figure 5). Figure 5 shows the dry and wet historical flows, as January is the dry season of the year while July is the wet season. Regardless of the average inflow sequence and the starting water level conditions, the model shows that the water level did not fall below the MOL.
As shown in Figure 6, the modeled outflow/release downstream of the HAD was in accordance with the HAD management policy regime and remained within the historical release sequence illustrated in Figure 5. Similarly, as presented in Figure 7, regardless of the HAD starting period, the energy produced may be reduced by 10–25%, particularly if the initial level of the HAD reaches 158.2 m before it recovers. Moreover, considerations of annual operation policies based on seasonal forecasting and joint optimization of rules may further decrease the reduction rate of energy through a water transportation mechanism. Water transportation involves retaining more water during the wet season to compensate for the low supply of water during the dry seasons.
When calibrating the dry inflow conditions of the GERD filling under the six-year strategy, we found that the HAD can be operated above the MOL of 147 m when the starting level of HAD exceeds 170 m. When the HAD starting water level conditions during the start of filling are below 170 m, the water level may exceed the MOL. However, conditions similar to those observed in the 1984 drought are not likely to recur in the same sequence, and as shown from the historical water level conditions, HAD exceeded 170 m. In a dry scenario, the combination of a joint safeguard policy and stringent drought management measures can be implemented to foster cooperation and goodwill.
This study attempts to elucidate the challenges of the ongoing and future negotiations associated with sharing a water resource and managing the two biggest African dams (i.e., the GERD and HAD) built in a single river system in two different countries. We also determine that upon completion of the GERD construction (it was 90% complete at the time of this study), the dynamics of the Nile River basin will change; therefore, a "new normal" in terms of operation as well as a novel hydrological order and approach utilizing revised logic will be needed [56]. We demonstrated negligible impacts on the HAD during the five- and six-year GERD filling strategies. Therefore, in addition to the potential impacts of GERD filling, it is deemed that the ongoing problematic and complicated negotiation surrounding the operation of the GERD is one example of the onset of a new normal owing to the construction of the GERD, which necessitates continual negotiation to manage the perceptions and definition of gains, biases and losses based on a given point of reference,
As we have demonstrated by analyzing the two shortest-timescale filling policies, although Ethiopia is currently pursuing longer-timescale filling policy, filling the GERD would not prompt Egypt to invoke the HAD's DMP. Thus, the challenge to reach an agreement goes beyond hydrological matters. We suggest that the challenge is based on the perceptions and biases that originated with the 1902 Nile treaty signed by Great Britain, the 1929 Anglo-Egyptian treaty, and the 1959 Nile Waters treaty signed between Egypt and Sudan. The 1929 treaty allocated 48 BCM waters to Egypt and 4 BCM to Sudan, while the 1959 treaty allocated Egypt 55.5 BCM of Nile River waters and allotted 18.5 BCM to Sudan while leaving only 10 BCM for evaporation. Moreover, the treaty provided Egypt veto power over any constructions in the Nile River system. Both treaties excluded Ethiopia, which hosts the origin of the Abbay River (i.e., Blue Nile) that contributes approximately 80% of overall Nile River flows. In turn, Ethiopia deemed the treaties null and void and dubbed them as colonial treaties. Thus, the factors mentioned above could be the basis for the ongoing debate that commenced upon construction of the GERD. Since that time, the riparian states have not been able to agree on either the short-term filing policies or the long-term operations and continue to be reluctant to fully endorse the international panel of experts' recommendations. The ensuing disagreement on the mediating roles of the African Union Commission negotiation, along with Egypt's and Sudan's calls for the Arab League Nations to become involved in the matter, and the subsequent internationalization of the diplomacy by taking the dispute to the United Nations Security Council calls for an even more comprehensive research investigation including physical sciences, further historical data, and decisions science to work towards a resolve. Such an investigation may provide a framework for resolving similar issues arising elsewhere in the world.
As discussed, it was found that the water flow from Abbay River basin to Egypt under the two filling policies should not drop below the MOL of the HAD. Therefore, the associated impacts of the GERD on the two shortest-timescale filling polices should be negligible during both average and drier conditions. However, the mere construction of the GERD may have caused uneasiness and frustration among policymakers and Egyptians at large [57,58]. Behavioral scientists and researchers have highlighted the negative impacts of random variability and systematic errors in decision-making and opinion formations. Endowment factors of losses or gains and decision-making biases and noises are considered to be among other factors that cause policy debate polarization in international negotiation and decisions [59,60,61]. Any perceived losses would elicit strong reactions and prompt resistance among those who feel affected; in turn, the endowment effect will reinforce this into a vicious cycle [59,60,61,62,63]
Similarly, confirmation bias causes both policymakers to become resistant to change by establishing their status quo. In the era of climate change, the past does not necessarily reflect the future, thus water resource strategies need to adapt to the associated changes, thereby recognizing the new normal of a changing climate. However, attaining the required change and adapting to the new normal at regional levels for transboundary water regimes will be an uphill task. This is because each party continually affirms the subjective narratives of its domestic political elites. Such behavioral and psychological factors thereby cause differences in policymakers' view of the world, particularly with respect to the reasonable sharing of resources through the "echo chamber" phenomenon [64]. This is because people tend to accept information and data that resonate with their beliefs and disregard information and data that conflict with their beliefs and cognitive modeling processes [62,63,65,66]. The relevance of this hypothesis to our study result is that without verifying the reduction in the water flow owing to the GERD, the mere construction of the GERD could lead to a perception of water loss in Egypt and trigger confirmation biases, such as those provided by Herodotus's inaccurate comment that Egypt is the gift of the Nile [67], and generates gaps in terms of policy cooperation and benefit sharing mechanisms among all 11 Nile River basin riparian states.
The perception of water loss is more extensive and deeply rooted as compared to that of the actual loss of related commodities [68]. In this way, confirmation biases to the status quo "do not form in a vacuum but rather correspond to the way water issues are integrated in pre-existing political dynamics" [69]. Accordingly, the political denotation and connotation of the Nile River basin have been deeply integrated into the cultures and national symbols of affected countries. Therefore, the decision of political elites to cooperate with each other or with peer riparian states could depend on their analysis of domestic political benefits or reprisal costs in their respective political domains [70]. Still more, besides the cognitive biases of losses and gains, the new concept of noise and its roles in decision variability should also be a factor in future research and search for an adaptable solution. Noise is a random and unexplainable error in decisions and can be triggered by various emotional and relational factors. In our case, the perceived and natural use of water resources by any of the riparian states may trigger a certain country's policymaker to make a noisy decision [62,63,64,65,66]. Noise is defined as judgment variability between experts whose judgments are expected to be interchangeable. Thus, substantial judgment variability between experts has recently been brought to attention by researchers. For example, Jaya Ramji-Nogales et al. [71] researched the Miami courthouse to understand decision variability where the clerk randomly tabled similar asylum cases to different judges. The research found that a judge granted asylum to 88% of the asylum seekers, while another judge in the same building granted asylum to only 5% of the applicants [62,71]. Moreover, their research result noted that the asylum seekers' origin was unable to explain the variations. Therefore sources of significant variability between experts in interpreting the same data while following the same measurement criteria cause poor decision qualities and disagreements.
Finally, the circumstances may render it difficult for government officials to make decisions or form agreements based on scientific results. Such an agreement would require a broader comprehensive regional approach that fosters and enhances solidarity among the Nile River basin riparian states and addresses policies and technicalities by focusing primarily on sustainability. In the future, international communities can help demystify the perception and definitions of losses, gains, and biases of the societies of Ethiopia, Sudan, and Egypt by targeted intervention through education. The international community can also help to maintain the integrity of negotiators by playing the impartial and genuine role of a mediator.
Egypt will not be compelled to invoke the DMP based on the five- and six-year annual filling policies of the GERD. Although filling of the GERD increases the generation of hydropower for Ethiopia, the energy generation at the HAD could decrease by 5%, or by 20% in the worst-case scenarios. Thus, collaborations among the riparian states could help realize the potential economic and environmental benefits through benefit sharing within trade and responsibility sharing within mitigation and adaption measures.
The GERD, being located in the Ethiopian highlands, has a low-level evaporation rate of 0.09 m, while the HAD evaporation rate is 0.199 m. Therefore, the GERD does not contribute to overall evaporation losses within the basin and saves water for the benefit of all. Furthermore, the GERD helps to regulate water flow, reduce sedimentation, arrest floods, enhance dam operations, and mitigate and reduce the impacts of climate change. Nevertheless, to materialize the shared benefit and collaboratively mitigate the future impacts of climate change, the effected riparian countries of Ethiopia, Sudan, and Egypt need to coordinate their respective development plans for the basin based on their comparative advantageous and environmental suitability. In light of this, the political and technical leaders of these countries must build mutual trust and adhere to evidence-based decision-making practices so as to develop climate-proof Nile River basin agreements. In doing so, these countries may be able to reach a climate-proof and future-oriented Nile River basin governance system at both policy and technical levels. At the policy level, a vision for benefit sharing and economic cooperation should be fostered, while at the technical level, the countries may need to cooperate on data and skill sharing that aims to reduce the impacts of climate change by undertaking mitigation and adaptation strategies through the use and application of water efficient and effective technologies. Such technologies may involve deploying water-efficient irrigation infrastructure, water-efficient and drought-tolerant crops grown using biotechnology and/or new breeding techniques, and training programs for farmers to encourage effective and efficient use of irrigation water.
The authors suggest the importance of having a dynamic, phased-based, procedural, and substantive filling agreement in the short term to de-escalate associated tensions. While parties continue working towards reaching a long-term basin-wide agreement, the phased-based short-term agreement could also be extended to a medium-term river-based agreement, similar to that of the Uruguay and Rio de La Plata statutes signed between Uruguay and Argentina in 1975.
To achieve the long-term basin-wide agreement, experience can be considered from other relevant locations within Africa. For example, there are at least 20 effective international agreements across 63 river basins, and effective institutionalized systems exist for 16 river basins that coordinate countries' river basin development initiatives and activities including the Niger Basin Authority, Organisation pour la Mise en Valeur du Fleuve Sénégal, and Lake Chad Basin Commission. Nevertheless the authors suggest investigating the underlined cause of the impasse while the international community continues to collaborate in providing integrity-saving opportunities for policymakers that foster the development of a basin-wide, long-term, climate-proof operations agreement.
Based on the findings of this research, we suggest that future studies should examine the upstream countries' actual water utilizations under a changing climate, as well as their actual and perceived impacts on downstream countries. We believe that insight into such intervention would help to avoid water conflicts and foster cooperation through by demystifying the perceived losses and gains of the transboundary water resources.
The authors declare no conflict of interest.
[1] |
W. Zhou, H. Wang, Z. Wan, Ore image classification based on improved CNN, Comput. Electr. Eng., 99 (2022), 107819. https://doi.org/10.1016/j.compeleceng.2022.107819 doi: 10.1016/j.compeleceng.2022.107819
![]() |
[2] |
S. S. Amiripallia, G. N. Rao, J. Beharaa, K. Sanjay, Mineral rock classification using convolutional neural network, Adv. Parallel Comput., 39 (2021), 499–505. https://doi.org/10.3233/APC210235 doi: 10.3233/APC210235
![]() |
[3] |
X. Liu, H. Wang, H. Jing, A. Shao, L. Wang, Research on intelligent identification of rock types based on Faster R-CNN method, IEEE Access, 8 (2020), 21804–21812. https://doi.org/10.1109/ACCESS.2020.2968515 doi: 10.1109/ACCESS.2020.2968515
![]() |
[4] |
S. Karimpouli, P. Tahmasebi, Segmentation of digital rock images using deep convolutional autoencoder networks, Comput. Geosci., 126 (2019), 142–150. https://doi.org/10.1016/j.cageo.2019.02.003 doi: 10.1016/j.cageo.2019.02.003
![]() |
[5] |
J. Maitre, K. Bouchard, L. P. Bédard, Mineral grains recognition using computer vision and machine learning, Comput. Geosci., 130 (2019), 84–93. https://doi.org/10.1016/j.cageo.2019.05.009 doi: 10.1016/j.cageo.2019.05.009
![]() |
[6] |
M. He, Z. Zhang, J. Ren, J. Huan, G. Li, Y. Chen, et al., Deep convolutional neural network for fast determination of the rock strength parameters using drilling data, Int. J. Rock Mech. Min. Sci., 123 (2019), 104084. https://doi.org/10.1016/j.ijrmms.2019.104084 doi: 10.1016/j.ijrmms.2019.104084
![]() |
[7] |
F. Alzubaidi, P. Mostaghimi, P. Swietojanski, S. R. Clark, R. T. Armstrong, Automated lithology classification from drill core images using convolutional neural networks, J. Pet. Sci. Eng., 197 (2021), 107933. https://doi.org/10.1016/j.petrol.2020.107933 doi: 10.1016/j.petrol.2020.107933
![]() |
[8] | F. Zeng, A. Jacobson, D. Smith, N. Boswell, T. Peynot, M. Milford, Lookup: Vision-only real-time precise underground localisation for autonomous mining vehicles, In: 2019 International conference on robotics and automation (ICRA), IEEE, 2019, 1444–1450. https://doi.org/10.1109/ICRA.2019.8794453 |
[9] |
T. Chen, N. Hu, R. Niu, N. Zhen, A. Plaza, Object-oriented open-pit mine mapping using Gaofen-2 satellite image and convolutional neural network, for the Yuzhou City, China, Remote Sens., 12 (2020), 3895. https://doi.org/10.3390/rs12233895 doi: 10.3390/rs12233895
![]() |
[10] |
J. Baek, Y. Choi, Deep neural network for predicting ore production by truck-haulage systems in open-pit mines, Appl. Sci., 10 (2020), 1657. https://doi.org/10.3390/app10051657 doi: 10.3390/app10051657
![]() |
[11] |
J. Williams, J. Singh, M. Kumral, J. Ramirez Ruiseco, Exploring deep learning for dig-limit optimization in open-pit mines, Nat. Resour. Res., 30 (2021), 2085–2101. https://doi.org/10.1007/s11053-021-09864-y doi: 10.1007/s11053-021-09864-y
![]() |
[12] |
T. Vu, T. Bao, Q. V. Hoang, C. Drebenstetd, P. V. Hoa, H. H. Thang, Measuring blast fragmentation at Nui Phao open-pit mine, Vietnam using the Mask R-CNN deep learning model, Min. Technol., 130 (2021), 232–243. https://doi.org/10.1080/25726668.2021.1944458 doi: 10.1080/25726668.2021.1944458
![]() |
[13] | G. Somua-Gyimah, S. Frimpong, W. Nyaaba, E. Gbadam, A computer vision system for terrain recognition and object detection tasks in mining and construction environments, In: SME Annual Conference, 2019. |
[14] |
R. Gao, Z. Sun, W. Li, L. Pei, Y. Hu, L. Xiao, Automatic coal and gangue segmentation using U-Net based fully convolutional networks, Energies, 13 (2020), 829. https://doi.org/10.3390/en13040829 doi: 10.3390/en13040829
![]() |
[15] |
X. Liu, Y. Zhang, H. Jing, L. Wang, S. Zhao, Ore image segmentation method using U-Net and Res_Unet convolutional networks, RSC Adv., 10 (2020), 9396–9406. https://doi.org/10.1039/C9RA05877J doi: 10.1039/C9RA05877J
![]() |
[16] | L. Si, X. Xiong, Z. Wang, C. Tan, A deep convolutional neural network model for intelligent discrimination between coal and rocks in coal mining face, Math. Probl. Eng., 2020. https://doi.org/10.1155/2020/2616510 |
[17] |
C. Su, S. Xu, K. Zhu, X. Zhang, Rock classification in petrographic thin section images based on concatenated convolutional neural networks, Earth Sci. Inform., 13 (2020), 1477–1484. https://doi.org/10.1007/s12145-020-00505-1 doi: 10.1007/s12145-020-00505-1
![]() |
[18] | V. Zyuzin, M. Ronkin, S. Porshnev, A. Kalmykov, Computer vision system for the automatic asbestos content control in stones, In: Journal of Physics: Conference Series, vol. 1727, IOP Publishing, 2021, 012014. 10.1088/1742-6596/1727/1/012014 |
[19] |
A. Azizi, H. Moomivand, A new approach to represent impact of discontinuity spacing and rock mass description on the median fragment size of blasted rocks using image analysis of rock mass, Rock Mech. Rock Eng., 54 (2021), 2013–2038. https://doi.org/10.1007/s00603-020-02360-4 doi: 10.1007/s00603-020-02360-4
![]() |
[20] |
S. Shrivastava, S. Bhattacharjee, D. Deb, Segmentation of mine overburden dump particles from images using Mask R CNN, Sci. Rep., 13 (2023), 2046. https://doi.org/10.1038/s41598-023-28586-0 doi: 10.1038/s41598-023-28586-0
![]() |
[21] |
M. Babaeian, M. Ataei, F. Sereshki, F. Sotoudeh, S. Mohammadi, A new framework for evaluation of rock fragmentation in open pit mines, J. Rock Mech. Geotech. Eng., 11 (2019), 325–336. https://doi.org/10.1016/j.jrmge.2018.11.006 doi: 10.1016/j.jrmge.2018.11.006
![]() |
[22] |
S. Mkwelo, V. Nicolls, G. De Jager, Watershed-based segmentation of rock scenes and proximity-based classification of watershed regions under uncontrolled lighting, SAIEE Afr. Res. J., 96 (2005), 28–34. https://doi.org/10.23919/SAIEE.2005.9488146 doi: 10.23919/SAIEE.2005.9488146
![]() |
[23] |
T. Bamford, K. Esmaeili, A. P. Schoellig, A deep learning approach for rock fragmentation analysis, Int. J. Rock Mech. Min. Sci., 145 (2021), 104839. https://doi.org/10.1016/j.ijrmms.2021.104839 doi: 10.1016/j.ijrmms.2021.104839
![]() |
[24] |
D. Jung, Y. Choi, Systematic review of machine learning applications in mining: exploration, exploitation, and reclamation, Minerals, 11 (2021), 148. https://doi.org/10.3390/min11020148 doi: 10.3390/min11020148
![]() |
[25] | J. A. Franklin, T. Katsabanis, Measurement of blast fragmentation, Rotterdam: CRC Press, 1996. |
[26] |
A. Tosun, A modified Wipfrag program for determining muckpile fragmentation, J. S. Afr. Inst. Min. Metall., 118 (2018), 1113–1199. https://doi.org/10.17159/2411-9717/2018/v118n10a13 doi: 10.17159/2411-9717/2018/v118n10a13
![]() |
[27] |
J. P. Latham, J. Kemeny, N. Maerz, M. Noy, J. Schleifer, S. Tose, A blind comparison between results of four image analysis systems using a photo-library of piles of sieved fragments, Fragblast, 7 (2003), 105–132. https://doi.org/10.1076/frag.7.2.105.15899 doi: 10.1076/frag.7.2.105.15899
![]() |
[28] |
Y. LeCun, B. Boser, J. S. Denker, D. Henderson, R. E. Howard, W. Hubbard, et al., Backpropagation applied to handwritten zip code recognition, Neural Comput., 1 (1989), 541–551. https://doi.org/10.1162/neco.1989.1.4.541 doi: 10.1162/neco.1989.1.4.541
![]() |
[29] | Y. LeCun, B. Boser, J. Denker, D. Henderson, R. Howard, W. Hubbard, et al., Handwritten digit recognition with a back-propagation network, In: Advances in Neural Information Processing Systems (Ed. D. Touretzky), vol. 2, Morgan-Kaufmann, 1989. |
[30] |
C. Lu, Z. Wang, B. Zhou, Intelligent fault diagnosis of rolling bearing using hierarchical convolutional network based health state classification, Mech. Syst. Signal Process., 32 (2017), 139–151. https://doi.org/10.1016/j.aei.2017.02.005 doi: 10.1016/j.aei.2017.02.005
![]() |
[31] |
W. Zhang, C. Li, G. Peng, Y. Chen, Z. Zhang, A deep convolutional neural network with new training methods for bearing fault diagnosis under noisy environment and different working load, Mech. Syst. Signal Process., 100 (2018), 439–453. https://doi.org/10.1016/j.ymssp.2017.06.022 doi: 10.1016/j.ymssp.2017.06.022
![]() |
[32] |
Y. J. Cha, W. Choi, O. Büyüköztürk, Deep learning-based crack damage detection using convolutional neural networks, Comput. Aided Civ. Infrastruct. Eng., 32 (2017), 361–378. https://doi.org/10.1111/mice.12263 doi: 10.1111/mice.12263
![]() |
[33] |
H. V. Dang, M. Raza, T. V. Nguyen, T. Bui-Tien, H. X. Nguyen, Deep learning-based detection of structural damage using time-series data, Struct. Infrastruct. Eng., 17 (2021), 1474–1493. https://doi.org/10.1080/15732479.2020.1815225 doi: 10.1080/15732479.2020.1815225
![]() |
[34] |
F. Yessoufou, J. Zhu, One-class convolutional neural network (OC-CNN) model for rapid bridge damage detection using bridge response data, KSCE J. Civ. Eng., 27 (2023), 1640–1660. https://doi.org/10.1007/s12205-023-0063-7 doi: 10.1007/s12205-023-0063-7
![]() |
[35] |
D. Ai, J. Cheng, A deep learning approach for electromechanical impedance based concrete structural damage quantification using two-dimensional convolutional neural network, Mech. Syst. Signal Pr., 183 (2023), 109634. https://doi.org/10.1016/j.ymssp.2022.109634 doi: 10.1016/j.ymssp.2022.109634
![]() |
[36] |
Y. LeCun, L. Bottou, Y. Bengio, P. Haffner, Gradient-based learning applied to document recognition, Proc. IEEE, 86 (1998), 2278–2324. https://doi.org/10.1109/5.726791 doi: 10.1109/5.726791
![]() |
[37] | I. Goodfellow, Y. Bengio, A. Courville, Deep Learning, Cambridge, MA: MIT Press, 2016. |
[38] | A. Zhang, Z. C. Lipton, M. Li, A. J. Smola, Dive into deep learning, (2023). https://doi.org/10.48550/arXiv.2106.11342 |
[39] |
H. Li, C. Pan, A. Chen, Z. Wulamu, A. Yang, Ore image segmentation method based on U-Net and Watershed, Comput. Mater. Contin., 65 (2020), 563–578. https://doi.org/10.32604/cmc.2020.09806 doi: 10.32604/cmc.2020.09806
![]() |
[40] |
D. Yang, X. Wang, H. Zhang, Z. Yin, D. Su, J. Xu, A Mask R-CNN based particle identification for quantitative shape evaluation of granular materials, Powder Technol., 392 (2021), 296–305. https://doi.org/10.1016/j.powtec.2021.07.005 doi: 10.1016/j.powtec.2021.07.005
![]() |
[41] |
M. Li, X. Wang, H. Yao, H. Saxén, Y. Yu, Analysis of particle size distribution of coke on blast furnace belt using object detection, Processes, 10 (2022), 1902. https://doi.org/10.3390/pr10101902 doi: 10.3390/pr10101902
![]() |
[42] | M. Ronkin, A. Kalmykov, K. Reshetnikov, V. Zyuzin, Investigation of object detection based method for open-pit blast quality estimation, In: 2022 Ural-Siberian Conference on Biomedical Engineering, Radioelectronics and Information Technology (USBEREIT), IEEE, 2022,248–251. https://doi.org/10.1109/USBEREIT56278.2022.9923353 |
[43] |
G. Xue, S. Li, P. Hou, S. Gao, R. Tan, Research on lightweight Yolo coal gangue detection algorithm based on resnet18 backbone feature network, Internet Things, 22 (2023), 100762. https://doi.org/10.1016/j.iot.2023.100762 doi: 10.1016/j.iot.2023.100762
![]() |
[44] | A. K. Sangaiah, Deep learning and parallel computing environment for bioengineering systems, St. Louis, MO: Academic Press, 2019. |
[45] | S. Beucher, Use of watersheds in contour detection, In: Proceedings of the International Workshop on Image Processing, CCETT, 1979. |
[46] |
Z. Yang, H. Ding, L. Guo, M. Lian, Superpixel image segmentation-based particle size distribution analysis of fragmented rock, IEEE Access, 9 (2021), 59048–59058. https://doi.org/10.1109/ACCESS.2021.3072998 doi: 10.1109/ACCESS.2021.3072998
![]() |
[47] |
Q. Guo, Y. Wang, S. Yang, Z. Xiang, A method of blasted rock image segmentation based on improved watershed algorithm, Sci. Rep., 12 (2022), 7143. https://doi.org/10.1038/s41598-022-11351-0 doi: 10.1038/s41598-022-11351-0
![]() |
[48] | O. Ronneberger, P. Fischer, T. Brox, U-Net: Convolutional networks for biomedical image segmentation, In: International Conference on Medical image computing and computer-assisted intervention, Springer, 2015,234–241. https://doi.org/10.1007/978-3-319-24574-4_28 |
[49] |
N. Siddique, S. Paheding, C. P. Elkin, V. Devabhaktuni, U-Net and its variants for medical image segmentation: A review of theory and applications, IEEE Access, 9 (2021), 82031–82057. https://doi.org/10.1109/ACCESS.2021.3086020 doi: 10.1109/ACCESS.2021.3086020
![]() |
[50] |
X. X. Yin, L. Sun, Y. Fu, R. Lu, Y. Zhang, U-Net-based medical image segmentation, J. Healthc. Eng., 2022 (2022), 4189781. https://doi.org/10.1155/2022/4189781 doi: 10.1155/2022/4189781
![]() |
[51] |
J. Wu, Y. Guo, F. Gou, Z. Dai, A medical assistant segmentation method for MRI images of osteosarcoma based on DecoupleSegNet, Int. J. Intell. Syst., 37 (2022), 8436–8461. https://doi.org/10.1002/int.22949 doi: 10.1002/int.22949
![]() |
[52] |
Z. Ling, S. Yang, F. Gou, Z. Dai, J. Wu, Intelligent assistant diagnosis system of osteosarcoma mri image based on transformer and convolution in developing countries, IEEE J. Biomed. Health Inform., 26 (2022), 5563–5574. https://doi.org/10.1109/JBHI.2022.3196043 doi: 10.1109/JBHI.2022.3196043
![]() |
[53] |
Z. Zhou, F. Gou, Y. Tan, J. Wu, A cascaded multi-stage framework for automatic detection and segmentation of pulmonary nodules in developing countries, IEEE J. Biomed. Health Inform., 26 (2022), 5619–5630. https://doi.org/10.1109/JBHI.2022.3198509 doi: 10.1109/JBHI.2022.3198509
![]() |
[54] | J. Wu, W. Liu, C. Li, T. Jiang, I. M. Shariful, H. Sun, et al., A state-of-the-art survey of U-Net in microscopic image analysis: from simple usage to structure mortification, (2022). https://doi.org/10.48550/arXiv.2202.06465 |
[55] |
W. Qiao, Y. Zhao, Y. Xu, Y. Lei, Y. Wang, S. Yu, et al., Deep learning-based pixel-level rock fragment recognition during tunnel excavation using instance segmentation model, Tunn. Undergr. Space Technol., 115 (2021), 104072. https://doi.org/10.1016/j.tust.2021.104072 doi: 10.1016/j.tust.2021.104072
![]() |
[56] |
Z. Xue, L. Chen, Z. Liu, F. Lin, W. Mao, Rock segmentation visual system for assisting driving in tbm construction, Mach. Vis. Appl., 32 (2021), 77. https://doi.org/10.1007/s00138-021-01203-8 doi: 10.1007/s00138-021-01203-8
![]() |
[57] |
X. Zhou, Q. Gong, Y. Liu, L. Yin, Automatic segmentation of tbm muck images via a deep-learning approach to estimate the size and shape of rock chips, Autom. Constr., 126 (2021), 103685. https://doi.org/10.1016/j.autcon.2021.103685 doi: 10.1016/j.autcon.2021.103685
![]() |
[58] |
W. Wang, Q. Li, C. Xiao, D. Zhang, L. Miao, L. Wang, An improved boundary-aware U-Net for ore image semantic segmentation, Sensors, 21 (2021), 2615. https://doi.org/10.3390/s21082615 doi: 10.3390/s21082615
![]() |
[59] |
D. Xiao, X. Liu, B. T. Le, Z. Ji, X. Sun, An ore image segmentation method based on RDU-Net model, Sensors, 20 (2020), 4979. https://doi.org/10.3390/s20174979 doi: 10.3390/s20174979
![]() |
[60] |
J. Chen, M. Zhou, H. Huang, D. Zhang, Z. Peng, Automated extraction and evaluation of fracture trace maps from rock tunnel face images via deep learning, Int. J. Rock Mech. Min. Sci., 142 (2021), 104745. https://doi.org/10.1016/j.ijrmms.2021.104745 doi: 10.1016/j.ijrmms.2021.104745
![]() |
[61] |
W. Gu, S. Bai, L. Kong, A review on 2D instance segmentation based on deep neural networks, Image Vis. Comput., 120 (2022), 104401. https://doi.org/10.1016/j.imavis.2022.104401 doi: 10.1016/j.imavis.2022.104401
![]() |
[62] |
A. M. Hafiz, G. M. Bhat, A survey on instance segmentation: state of the art, Int. J. Multimed. Inf. Retr., 9 (2020), 171–189. https://doi.org/10.1007/s13735-020-00195-x doi: 10.1007/s13735-020-00195-x
![]() |
[63] | K. He, G. Gkioxari, P. Dollár, R. Girshick, Mask R-CNN, In: 2017 IEEE International Conference on Computer Vision (ICCV), 2017, 2980–2988. https://doi.org/10.1109/ICCV.2017.322 |
[64] | K. He, X. Zhang, S. Ren, J. Sun, Deep residual learning for image recognition, In: 2016 IEEE Conference on Computer Vision and Pattern Recognition (CVPR), 2016,770–778. https://doi.org/10.1109/CVPR.2016.90 |
[65] | S. Ramesh C., A review on instance segmentation using mask R-CNN, In: Proceedings of the International Conference on Systems, Energy & Environment (ICSEE), 2021. |
[66] | F. Schenk, A. Tscharf, G. Mayer, F. Fraundorfer, Automatic muck pile characterization from UAV images, In: ISPRS Annals of the Photogrammetry, Remote Sensing and Spatial Information Sciences, vol. IV-2/W5, 2019,163–170. https://doi.org/10.5194/isprs-annals-IV-2-W5-163-2019 |
[67] |
V. Zyuzin, M. Ronkin, S. Porshnev, A. Kalmykov, Automatic asbestos control using deep learning based computer vision system, Appl. Sci., 11 (2021), 10532. https://doi.org/10.3390/app112210532 doi: 10.3390/app112210532
![]() |
[68] |
S. Shrivastava, D. Deb, S. Bhattacharjee, Prediction of particle size distribution curves of dump materials using convolutional neural networks, Rock Mech. Rock Eng., 55 (2022), 471–479. https://doi.org/10.1007/s00603-021-02657-y doi: 10.1007/s00603-021-02657-y
![]() |
[69] | L. Fu, X. Xu, F. Jin, H. Zhou, Evaluation of the particle size distribution of on-site rockfill using mask r-cnn deep learning model, In: 2021 7th International Conference on Hydraulic and Civil Engineering & Smart Water Conservancy and Intelligent Disaster Reduction Forum (ICHCE & SWIDR), 2021,291–297. https://doi.org/10.1109/ICHCESWIDR54323.2021.9656248 |
[70] |
H. Fan, Z. Tian, X. Xu, X. Sun, Y. Ma, H. Liu, et al., Rockfill material segmentation and gradation calculation based on deep learning, Case Stud. Constr. Mater., 17 (2022), e01216. https://doi.org/10.1016/j.cscm.2022.e01216 doi: 10.1016/j.cscm.2022.e01216
![]() |
[71] |
H. Huang, J. Luo, E. Tutumluer, J. M. Hart, A. J. Stolba, Automated segmentation and morphological analyses of stockpile aggregate images using deep convolutional neural networks, Transp. Res. Rec., 2674 (2020), 285–298. https://doi.org/10.1177/0361198120943887 doi: 10.1177/0361198120943887
![]() |
[72] | J. Redmon, S. Divvala, R. Girshick, A. Farhadi, You only look once: Unified, real-time object detection, In: 2016 IEEE Conference on Computer Vision and Pattern Recognition (CVPR), 2016,779–788. https://doi.org/10.1109/CVPR.2016.91 |
[73] | J. Redmon, A. Farhadi, YOLOv3: An incremental improvement, (2018). https://doi.org/10.48550/arXiv.1804.02767 |
[74] | A. Bochkovskiy, C. Y. Wang, H. Y. M. Liao, YOLOv4: Optimal speed and accuracy of object detection, (2020). https://doi.org/10.48550/arXiv.2004.10934 |
[75] | T. Diwan, G. Anirudh, J. V. Tembhurne, Object detection using YOLO: Challenges, architectural successors, datasets and applications, Multimed. Tools Appl., 82 (2023) 9243–9275. https://doi.org/10.1007/s11042-022-13644-y |
[76] |
P. Jiang, D. Ergu, F. Liu, Y. Cai, B. Ma, A review of Yolo algorithm developments, Procedia Comput. Sci., 199 (2022), 1066–1073. https://doi.org/10.1016/j.procs.2022.01.135 doi: 10.1016/j.procs.2022.01.135
![]() |
[77] |
D. Li, G. Wang, Y. Zhang, S. Wang, Coal gangue detection and recognition algorithm based on deformable convolution YOLOv3, IET Image Process., 16 (2022), 134–144. https://doi.org/10.1049/ipr2.12339 doi: 10.1049/ipr2.12339
![]() |
[78] |
X. Wang, Y. Guo, Y. Yu, An appropriate approach to recognize coke size distribution in a blast furnace, Processes, 11 (2023), 187. https://doi.org/10.3390/pr11010187 doi: 10.3390/pr11010187
![]() |
[79] |
H. Pan, Y. Shi, X. Lei, Z. Wang, F. Xin, Fast identification model for coal and gangue based on the improved tiny YOLO v3, J. Real-Time Image Proc., 19 (2022), 687–701. https://doi.org/10.1007/s11554-022-01215-1 doi: 10.1007/s11554-022-01215-1
![]() |
[80] | J. Terven, D. Cordova-Esparza, A comprehensive review of YOLO: From YOLOv1 and beyond, (2023). https://doi.org/10.48550/arXiv.2304.00501 |
[81] |
Z. Yang, B. He, Y. Liu, D. Wang, G. Zhu, Classification of rock fragments produced by tunnel boring machine using convolutional neural networks, Autom. Constr., 125 (2021), 103612. https://doi.org/10.1016/j.autcon.2021.103612 doi: 10.1016/j.autcon.2021.103612
![]() |
[82] |
L. E. Olivier, M. G. Maritz, I. K. Craig, Estimating ore particle size distribution using a deep convolutional neural network, IFAC-PapersOnLine, 53 (2020), 12038–12043. https://doi.org/10.1016/j.ifacol.2020.12.740 doi: 10.1016/j.ifacol.2020.12.740
![]() |
[83] |
J. Chen, T. Yang, D. Zhang, H. Huang, Y. Tian, Deep learning based classification of rock structure of tunnel face, Geosci. Front., 12 (2021), 395–404. https://doi.org/10.1016/j.gsf.2020.04.003 doi: 10.1016/j.gsf.2020.04.003
![]() |
[84] | D. Bolya, C. Zhou, F. Xiao, Y. J. Lee, Yolact: Real-time instance segmentation, In: 2019 IEEE/CVF International Conference on Computer Vision (ICCV), 2019, 9156–9165. https://doi.org/10.1109/ICCV.2019.00925 |
[85] | T. Cheng, X. Wang, S. Chen, W. Zhang, Q. Zhang, C. Huang, et al., Sparse instance activation for real-time instance segmentation, In: Proceedings of the IEEE/CVF Conference on Computer Vision and Pattern Recognition, 2022, 4433–4442. https://doi.org/10.1109/CVPR52688.2022.00439 |
[86] | C. Y. Wang, A. Bochkovskiy, H. Y. M. Liao, Yolov7: Trainable bag-of-freebies sets new state-of-the-art for real-time object detectors, In: Proceedings of the IEEE/CVF Conference on Computer Vision and Pattern Recognition (CVPR), 2023, 7464–7475. |
[87] | M. Tan, Q. Le, EfficientNet: Rethinking model scaling for convolutional neural networks, In: International conference on machine learning, PMLR, 2019, 6105–6114. |
[88] | M. Tan, Q. Le, EfficientNetv2: Smaller models and faster training, In: International Conference on Machine Learning, PMLR, 2021, 10096–10106. |
[89] | A. G. Howard, M. Zhu, B. Chen, D. Kalenichenko, W. Wang, T. Weyand, et al., MobileNets: Efficient convolutional neural networks for mobile vision applications, (2017). https://doi.org/10.48550/arXiv.1704.04861 |
[90] | M. Sandler, A. Howard, M. Zhu, A. Zhmoginov, L. C. Chen, Mobilenetv2: Inverted residuals and linear bottlenecks, In: Proceedings of the IEEE conference on computer vision and pattern recognition, 2018, 4510–4520. https://doi.org/10.1109/CVPR.2018.00474 |
[91] | A. Howard, M. Sandler, G. Chu, L. C. Chen, B. Chen, M. Tan, et al., Searching for MobileNetV3, In: Proceedings of the IEEE/CVF international conference on computer vision, 2019, 1314–1324. https://doi.org/10.1109/ICCV.2019.00140 |
[92] | S. Mehta, M. Rastegari, MobileViT: Light-weight, general-purpose, and mobile-friendly vision transformer, (2022). https://doi.org/10.48550/arXiv.2110.02178 |
[93] | K. He, X. Zhang, S. Ren, J. Sun, Identity mappings in deep residual networks, In: European conference on computer vision, Springer, 2016,630–645. https://doi.org/10.1007/978-3-319-46493-0_38 |
[94] | A. Supani, Y. Andriani, H. Indarto, Enhancing deeper layers with residual network on CNN architecture: A review, In: Proceedings of the 6th FIRST 2022 International Conference (FIRST 2022), vol. 14, Springer Nature, 2023,449. https://doi.org/10.2991/978-94-6463-118-0_46 |
[95] | H. Naveed, S. Anwar, M. Hayat, K. Javed, A. Mian, Survey: Image mixing and deleting for data augmentation, (2023). https://doi.org/10.48550/arXiv.2106.07085. |
[96] | J. L. Ba, J. R. Kiros, G. E. Hinton, Layer normalization, (2016). https://doi.org/10.48550/arXiv.1607.06450 |
[97] |
T. M. Hospedales, A. Antoniou, P. Micaelli, A. J. Storkey, Meta-learning in neural networks: A survey, IEEE Trans. Pattern Anal. Mach. Intell., 44 (2022), 5149–5169. https://doi.org/10.1109/TPAMI.2021.3079209 doi: 10.1109/TPAMI.2021.3079209
![]() |
[98] | C. J. Holder, M. Shafique, On efficient real-time semantic segmentation: A survey, (2022). https://doi.org/10.48550/arXiv.2206.08605 |
[99] | J. Xu, Z. Xiong, S. P. Bhattacharyya, PIDNet: A real-time semantic segmentation network inspired by PID controllers, In: Proceedings of the IEEE/CVF Conference on Computer Vision and Pattern Recognition (CVPR), 2023, 19529–19539. |
[100] | J. Peng, Y. Liu, S. Tang, Y. Hao, L. Chu, G. Chen, et al., PP-LiteSeg: A superior real-time semantic segmentation model, (2022). https://doi.org/10.48550/arXiv.2204.02681 |
[101] | C. Li, L. Li, H. Jiang, K. Weng, Y. Geng, L. Li, et al., YOLOv6: A single-stage object detection framework for industrial applications, (2022). https://doi.org/10.48550/arXiv.2209.02976 |
[102] | C. Li, L. Li, Y. Geng, H. Jiang, M. Cheng, B. Zhang, et al., YOLOv6 v3.0: A full-scale reloading, (2023). https://doi.org/10.48550/arXiv.2301.05586 |
[103] |
D. Bolya, C. Zhou, F. Xiao, Y. J. Lee, Yolact++ better real-time instance segmentation, IEEE Trans. Pattern Anal. Mach. Intell., 44 (2022), 1108–1121. https://doi.org/10.1109/TPAMI.2020.3014297. doi: 10.1109/TPAMI.2020.3014297
![]() |
[104] | X. Wang, R. Zhang, T. Kong, L. Li, C. Shen, SOLOv2: Dynamic and fast instance segmentation, In: Advances in Neural Information Processing Systems (Eds. H. Larochelle, M. Ranzato, R. Hadsell, M. Balcan and H. Lin), vol. 33, Curran Associates, Inc., 2020, 17721–17732. |
[105] | X. Wang, T. Kong, C. Shen, Y. Jiang, L. Li, Solo: Segmenting objects by locations, In: European Conference on Computer Vision, Springer, 2020,649–665. https://doi.org/10.1007/978-3-030-58523-5_38 |
[106] | J. He, P. Li, Y. Geng, X. Xie, FastInst: A simple query-based model for real-time instance segmentation, In: Proceedings of the IEEE/CVF Conference on Computer Vision and Pattern Recognition, 2023, 23663–23672. |
[107] | C. Lyu, W. Zhang, H. Huang, Y. Zhou, Y. Wang, Y. Liu, et al., RTMDet: An empirical study of designing real-time object detectors, (2022). https://doi.org/10.48550/arXiv.2212.07784 |
[108] | T. He, Z. Zhang, H. Zhang, Z. Zhang, J. Xie, M. Li, Bag of tricks for image classification with convolutional neural networks, In: Proceedings of the IEEE/CVF Conference on Computer Vision and Pattern Recognition, 2019,558–567. https://doi.org/10.1109/CVPR.2019.00065 |
[109] |
B. Bischl, M. Binder, M. Lang, T. Pielok, J. Richter, S. Coors, et al., Hyperparameter optimization: Foundations, algorithms, best practices, and open challenges, WIREs Data Min. Knowl. Discov., 13 (2023), e1484. https://doi.org/10.1002/widm.1484 doi: 10.1002/widm.1484
![]() |
[110] | A. Kirillov, E. Mintun, N. Ravi, H. Mao, C. Rolland, L. Gustafson, et al., Segment Anything, (2023). https://doi.org/10.48550/arXiv.2304.02643 |
[111] | Z. Gu, H. Chen, Z. Xu, J. Lan, C. Meng, W. Wang, DiffusionInst: Diffusion model for instance segmentation, (2022). https://doi.org/10.48550/arXiv.2212.02773 |
[112] | T. Amit, T. Shaharbany, E. Nachmani, L. Wolf, SegDiff: Image segmentation with diffusion probabilistic models, (2022). https://doi.org/10.48550/arXiv.2112.00390 |
[113] | W. Wu, Y. Zhao, M. Z. Shou, H. Zhou, C. Shen, DiffuMask: Synthesizing images with pixel-level annotations for semantic segmentation using diffusion models, (2023). https://doi.org/10.48550/arXiv.2303.11681 |
[114] | A. Gholami, S. Kim, Z. Dong, Z. Yao, M. W. Mahoney, K. Keutzer, A survey of quantization methods for efficient neural network inference, In: Low-Power Computer Vision, Chapman and Hall/CRC, 2022,291–326. |
[115] |
T. Liang, J. Glossner, L. Wang, S. Shi, X. Zhang, Pruning and quantization for deep neural network acceleration: A survey, Neurocomputing, 461 (2021), 370–403. https://doi.org/10.1016/j.neucom.2021.07.045 doi: 10.1016/j.neucom.2021.07.045
![]() |
[116] | M. Rakka, M. E. Fouda, P. Khargonekar, F. Kurdahi, Mixed-precision neural networks: A survey, (2022). https://doi.org/10.48550/arXiv.2208.06064 |
[117] | R. Tian, Z. Zhao, W. Liu, H. Liu, W. Mao, Z. Zhao, SAMP: A toolkit for model inference with self-adaptive mixed-precision, (2022). https://doi.org/10.48550/arXiv.2209.09130 |
[118] | NVIDIA Corporation: A PyTorch Extension: Tools for easy mixed precision and distributed training in Pytorch, 2022. Available from: https://github.com/nvidia/apex. |
[119] | Linux Foundation: Automatic Mixed Precision package - torch.amp, 2022. Available from: https://pytorch.org/docs/stable/amp.html. |
[120] | T. Honka, Automatic Mixed Precision Quantization of Neural Networks using Iterative Correlation Coefficient Adaptation, PhD thesis, Tampere University, (2021). |
[121] | C. Pham-Quoc, X. Q. Nguyen, T. N. Thinh, Hardware/software co-design for convolutional neural networks acceleration: A survey and open issues, In: Context-Aware Systems and Applications (Eds. P. Cong Vinh, A. Rakib), Springer International Publishing, Cham, 2021,164–178. https://doi.org/10.1007/978-3-030-93179-7_13 |
[122] |
J. Gou, B. Yu, S. J. Maybank, D. Tao, Knowledge distillation: A survey, Int. J. Comput. Vis., 129 (2021), 1789–1819. https://doi.org/10.1007/s11263-021-01453-z doi: 10.1007/s11263-021-01453-z
![]() |
[123] | W. Xu, Y. Zhang, X. Tang, Parallelizing DNN training on GPUs: Challenges and opportunities, In: Companion Proceedings of the Web Conference 2021, WWW '21, Association for Computing Machinery, New York, NY, USA, 2021,174–178. https://doi.org/10.1145/3442442.3452055 |
[124] |
Y. Fu, C. Aldrich, Online particle size analysis on conveyor belts with dense convolutional neural networks, Miner. Eng., 193 (2023), 108019. https://doi.org/10.1016/j.mineng.2023.108019 doi: 10.1016/j.mineng.2023.108019
![]() |
[125] |
H. Li, G. Asbjörnsson, M. Lindqvist, Image process of rock size distribution using dexined-based neural network, Minerals, 11 (2021), 736. https://doi.org/10.3390/min11070736 doi: 10.3390/min11070736
![]() |
[126] | V. Suprunenko, Ore particles segmentation using deep learning methods, In: Journal of Physics: Conference Series, vol. 1679, IOP Publishing, 2020, 042089. https://doi.org/10.1088/1742-6596/1679/4/042089 |
[127] |
X. Ma, P. Zhang, X. Man, L. Ou, A new belt ore image segmentation method based on the convolutional neural network and the image-processing technology, Minerals, 10 (2020), 1115. https://doi.org/10.3390/min10121115 doi: 10.3390/min10121115
![]() |
[128] |
Y. Zhan, G. Zhang, An improved OTSU algorithm using histogram accumulation moment for ore segmentation, Symmetry, 11 (2019), 431. https://doi.org/10.3390/sym11030431 doi: 10.3390/sym11030431
![]() |
[129] | Z. Tungol, Y. Kawamura, I. Kitahara, H. D. Jang, Development of a remote rock fragmentation size distribution measurement system for surface mines using 3D photogrammetry, In: The 10th International Conference on Explosives and Blasting, 2019. |
[130] |
Y. Wang, W. Tu, H. Li, Fragmentation calculation method for blast muck piles in open-pit copper mines based on three-dimensional laser point cloud data, Int. J. Appl. Earth Obs. Geoinf., 100 (2021), 102338. https://doi.org/10.1016/j.jag.2021.102338 doi: 10.1016/j.jag.2021.102338
![]() |
[131] |
F. Bai, M. Fan, H. Yang, L. Dong, Image segmentation method for coal particle size distribution analysis, Particuology, 56 (2021), 163–170. https://doi.org/10.1016/j.partic.2020.10.002 doi: 10.1016/j.partic.2020.10.002
![]() |
[132] |
C. Xie, H. Nguyen, X. N. Bui, Y. Choi, J. Zhou, T. Nguyen-Trang, Predicting rock size distribution in mine blasting using various novel soft computing models based on meta-heuristics and machine learning algorithms, Geosci. Front., 12 (2021), 101108. https://doi.org/10.1016/j.gsf.2020.11.005 doi: 10.1016/j.gsf.2020.11.005
![]() |
[133] | T. Vu, T. Bao, Development of a predictive model of rock fragmentation for Nui Phao open-pit mine in vietnam using multiple-output neural networks and Monte Carlo dropout technique, Research Square, https://doi.org/10.21203/rs.3.rs-171960/v1 |
[134] |
N. Koteleva, S. Khokhlov, I. Frenkel, Digitalization in open-pit mining: A new approach in monitoring and control of rock fragmentation, Appl. Sci., 11 (2021), 10848. https://doi.org/10.3390/app112210848 doi: 10.3390/app112210848
![]() |
[135] |
W. Chen, X. Li, H. He, L. Wang, A review of fine-scale land use and land cover classification in open-pit mining areas by remote sensing techniques, Remote Sens., 10 (2017), 15. https://doi.org/10.3390/rs10010015 doi: 10.3390/rs10010015
![]() |
[136] |
A. Sayadi, M. Monjezi, N. Talebi, M. Khandelwal, A comparative study on the application of various artificial neural networks to simultaneous prediction of rock fragmentation and backbreak, J. Rock Mech. Geotech. Eng., 5 (2013), 318–324. https://doi.org/10.1016/j.jrmge.2013.05.007 doi: 10.1016/j.jrmge.2013.05.007
![]() |
[137] | F. I. Siddiqui, Measurement of size distribution of blasted rock using digital image processing, Eng. Sci., 20 (2009), 81–93. |
[138] |
S. H. Cho, M. Nishi, M. Yamamoto, K. Kaneko, Fragment size distribution in blasting, Mater. Trans., 44 (2003), 951–956. https://doi.org/10.2320/matertrans.44.951 doi: 10.2320/matertrans.44.951
![]() |
[139] | M. J. Thurley, Automated image segmentation and analysis of rock piles in an open-pit mine, In: 2013 International Conference on Digital Image Computing: Techniques and Applications (DICTA), IEEE, 2013, 1–8. https://doi.org/10.1109/DICTA.2013.6691484 |
[140] |
W. D. Lei, K. Li, X. P. Wang, Improved watershed segmentation method in rock fragmentation analysis on digital photos, Adv. Mater. Res., 261 (2011), 1734–1737. https://doi.org/10.4028/www.scientific.net/AMR.261-263.1734 doi: 10.4028/www.scientific.net/AMR.261-263.1734
![]() |
[141] |
W. Wang, Q. Li, D. Zhang, J. Fu, Image segmentation of adhesive ores based on MSBA-Unet and convex-hull defect detection, Eng. Appl. Artif. Intell., 123 (2023), 106185. https://doi.org/10.1016/j.engappai.2023.106185 doi: 10.1016/j.engappai.2023.106185
![]() |
[142] | X. Tang, X. Wang, N. Yan, S. Fu, W. Xiong, Q. Liao, A new ore image segmentation method based on Swin-Unet, In: 2022 China Automation Congress (CAC), IEEE, 2022, 1681–1686. https://doi.org/10.1109/CAC57257.2022.10055952 |
[143] |
W. Wang, C. Su, H. Zhang, Automatic segmentation of concrete aggregate using convolutional neural network, Autom. Constr., 134 (2022), 104106. https://doi.org/10.1016/j.autcon.2021.104106 doi: 10.1016/j.autcon.2021.104106
![]() |
[144] |
R. Amoako, A. Jha, S. Zhong, Rock fragmentation prediction using an artificial neural network and support vector regression hybrid approach, Mining, 2 (2022), 233–247. https://doi.org/10.3390/mining2020013 doi: 10.3390/mining2020013
![]() |
[145] | H. Li, X. Wang, C. Yang, W. Xiong, Ore image segmentation method based on GAN-UNet, Control Theory Appl. |
[146] |
Y. Liu, Z. Zhang, X. Liu, L. Wang, X. Xia, Ore image classification based on small deep learning model: Evaluation and optimization of model depth, model structure and data size, Miner. Eng., 172 (2021), 107020. https://doi.org/10.1016/j.mineng.2021.107020 doi: 10.1016/j.mineng.2021.107020
![]() |
[147] |
J. Duan, X. Liu, X. Wu, C. Mao, Detection and segmentation of iron ore green pellets in images using lightweight u-net deep learning network, Neural Comput. Appl., 32 (2020), 5775–5790. https://doi.org/10.1007/s00521-019-04045-8 doi: 10.1007/s00521-019-04045-8
![]() |
[148] |
F. Sereshki, S. Hoseini, M. Ataei, Blast fragmentation analysis using image processing, Int. J. Min. Geo-Eng., 50 (2016), 211–218. http://dx.doi.org/10.22059/ijmge.2016.59831 doi: 10.22059/ijmge.2016.59831
![]() |
[149] |
H. Yaghoobi, H. Mansouri, M. A. E. Farsangi, H. Nezamabadi-Pour, Determining the fragmented rock size distribution using textural feature extraction of images, Powder Technol., 342 (2019), 630–641. https://doi.org/10.1016/j.powtec.2018.10.006 doi: 10.1016/j.powtec.2018.10.006
![]() |
[150] | M. Firla, P. Lipnicki, D. Lewandowski, Image processing algorithm for the assessment of the ore fragmentation size distribution, In: 2019 24th IEEE International Conference on Emerging Technologies and Factory Automation (ETFA), IEEE, 2019,505–512. https://doi.org/10.1109/ETFA.2019.8869462 |
[151] | M. Liancheng, Y. ZHANG, S. Guoqing, M. Zhen, L. Tianqi, Ore granularity detection and analysis system based on image processing, In: 2019 Chinese Control And Decision Conference (CCDC), IEEE, 2019,359–366. https://doi.org/10.1109/CCDC.2019.8832862 |
[152] |
H. Chen, Y. Jin, G. Li, B. Chu, Automated cement fragment image segmentation and distribution estimation via a holistically-nested convolutional network and morphological analysis, Powder Technol., 339 (2018), 306–313. https://doi.org/10.1016/j.powtec.2018.08.015 doi: 10.1016/j.powtec.2018.08.015
![]() |
[153] | R. Wang, W. Zhang, L. Shao, Research of ore particle size detection based on image processing, In: Proceedings of 2017 Chinese Intelligent Systems Conference: Volume II, Springer, 2018,505–514. https://doi.org/10.1007/978-981-10-6499-9_48 |
[154] |
X. Liu, C. Mao, W. Sun, X. Wu, Image-based method for measuring pellet size distribution in the stable area of disc pelletizer, ISIJ Int., 58 (2018), 2088–2094. https://doi.org/10.2355/isijinternational.ISIJINT-2018-384 doi: 10.2355/isijinternational.ISIJINT-2018-384
![]() |
[155] |
M. Heydari, R. Amirfattahi, B. Nazari, P. Rahimi, An industrial image processing-based approach for estimation of iron ore green pellet size distribution, Powder Technol., 303 (2016), 260–268. https://doi.org/10.1016/j.powtec.2016.09.020 doi: 10.1016/j.powtec.2016.09.020
![]() |
[156] | M. Roy, R. Paswan, M. Sarim, S. Kumar, R. R. Jha, P. Singh, Rock fragmentation by blasting – a review, J. Mines Met. Fuels, 64 (2016), 424–431. |
[157] |
E. Ebrahimi, M. Monjezi, M. R. Khalesi, D. J. Armaghani, Prediction and optimization of back-break and rock fragmentation using an artificial neural network and a bee colony algorithm, Bull. Eng. Geol. Environ., 75 (2016), 27–36. https://doi.org/10.1007/s10064-015-0720-2 doi: 10.1007/s10064-015-0720-2
![]() |
[158] |
I. Enayatollahi, A. Aghajani Bazzazi, A. Asadi, Comparison between neural networks and multiple regression analysis to predict rock fragmentation in open-pit mines, Rock Mech. Rock Eng., 47 (2014), 799–807. https://doi.org/10.1007/s00603-013-0415-6 doi: 10.1007/s00603-013-0415-6
![]() |
[159] |
F. Faramarzi, H. Mansouri, M. E. Farsangi, A rock engineering systems based model to predict rock fragmentation by blasting, Int. J. Rock Mech. Min. Sci., 60 (2013), 82–94. https://doi.org/10.1016/j.ijrmms.2012.12.045 doi: 10.1016/j.ijrmms.2012.12.045
![]() |
[160] |
X. Shi, Z. Jian, B. Wu, D. Huang, W. Wei, Support vector machines approach to mean particle size of rock fragmentation due to bench blasting prediction, Trans. Nonferrous Met. Soc. China, 22 (2012), 432–441. https://doi.org/10.1016/S1003-6326(11)61195-3 doi: 10.1016/S1003-6326(11)61195-3
![]() |
[161] |
M. J. Thurley, Automated online measurement of limestone particle size distributions using 3D range data, J. Process Control, 21 (2011), 254–262. https://doi.org/10.1016/j.jprocont.2010.11.011 doi: 10.1016/j.jprocont.2010.11.011
![]() |
[162] |
G. Zhang, G. Liu, H. Zhu, Segmentation algorithm of complex ore images based on templates transformation and reconstruction, Int. J. Miner. Metall. Mater., 18 (2011), 385. https://doi.org/10.1007/s12613-011-0451-8 doi: 10.1007/s12613-011-0451-8
![]() |
[163] | A. Amankwah, C. Aldrich, Rock image segmentation using watershed with shape markers, In: 2010 IEEE 39th Applied Imagery Pattern Recognition Workshop (AIPR), 2010, 1–7. http://doi.org/10.1109/AIPR.2010.5759719 |
[164] |
D. P. Mukherjee, Y. Potapovich, I. Levner, H. Zhang, Ore image segmentation by learning image and shape features, Pattern Recognit. Lett., 30 (2009), 615–622. https://doi.org/10.1016/j.patrec.2008.12.015 doi: 10.1016/j.patrec.2008.12.015
![]() |
[165] |
M. Monjezi, M. Rezaei, A. Y. Varjani, Prediction of rock fragmentation due to blasting in Gol-E-Gohar iron mine using fuzzy logic, Int. J. Rock Mech. Min. Sci., 46 (2009), 1273–1280. https://doi.org/10.1016/j.ijrmms.2009.05.005 doi: 10.1016/j.ijrmms.2009.05.005
![]() |
[166] | W. Wang, Rock particle image segmentation and systems, In: Pattern recognition techniques, technology and applications, I-Tech Vienna, Austria, 2008,197–226. |
[167] |
M. J. Thurley, K. C. Ng, Identifying, visualizing, and comparing regions in irregularly spaced 3d surface data, Comput. Vis. Image Underst., 98 (2005), 239–270. https://doi.org/10.1016/j.cviu.2003.12.002 doi: 10.1016/j.cviu.2003.12.002
![]() |
[168] | C. Perez, A. Casali, G. Gonzalez, G. Vallebuona, R. Vargas, Lithological composition sensor based on digital image feature extraction, genetic selection of features and neural classification, In: Proceedings 1999 International Conference on Information Intelligence and Systems (Cat. No. PR00446), IEEE, 1999,236–241. https://doi.org/10.1109/ICIIS.1999.810267 |
[169] | N. H. Maerz, Image sampling techniques and requirements for automated image analysis of rock fragmentation, In: Measurement of Blast Fragmentation, Routledge, 2018,115–120. |
1. | Ashebir Dingeto Hailu, Ethiopia hydropower development and Nile basin hydro politics, 2022, 10, 2333-8334, 87, 10.3934/energy.2022006 | |
2. | Kassa Abera Tareke, Admasu Gebeyehu Awoke, Roberto Coscarelli, Hydrological and Meteorological Drought Monitoring and Trend Analysis in Abbay River Basin, Ethiopia, 2022, 2022, 1687-9317, 1, 10.1155/2022/2048077 |