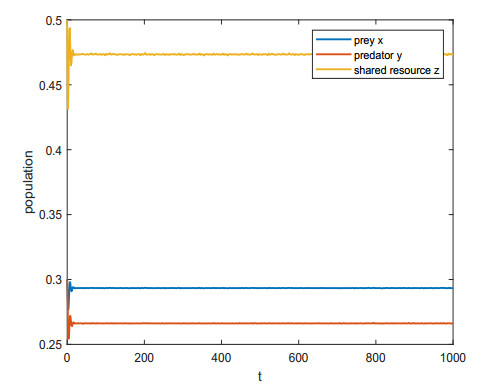
Electricity markets have been liberalized worldwide, but the success of country specific experiences varied widely. Consumers' behavior is among the key factors for successful liberalization experiences namely regarding the decision to switch operator. This decision has been shown to be influenced by a multiplicity of factors. The goal of this article is to explore the analysis of the drivers for switching operator in a liberalized electricity market. With that purpose, we focused on the residential Portuguese case using a questionnaire. The logit estimation showed that men are more likely to switch supplier than women and that larger families are less likely to do so probably, due to the perception of high information search costs. Other sociodemographic variables were not found to be statistically significant. Regarding specific determinants, our results showed that past experiences with a supplier, dissatisfaction with the current operator, and family and friends' experiences were the most important determining factor for the decision to switch operator. Hence, the price was not the most important determinant. We also explored if different income groups had differentiated responses regarding the main drivers but concluded that there was no evidence that the income group affected the importance given to the price or to the other determinants for the decision.
Citation: Débora Maravilha, Susana Silva, Erika Laranjeira. Consumer's behavior determinants after the electricity market liberalization: the Portuguese case[J]. Green Finance, 2022, 4(4): 436-449. doi: 10.3934/GF.2022021
[1] | Xiaoling Han, Xiongxiong Du . Dynamics study of nonlinear discrete predator-prey system with Michaelis-Menten type harvesting. Mathematical Biosciences and Engineering, 2023, 20(9): 16939-16961. doi: 10.3934/mbe.2023755 |
[2] | Xin-You Meng, Yu-Qian Wu . Bifurcation analysis in a singular Beddington-DeAngelis predator-prey model with two delays and nonlinear predator harvesting. Mathematical Biosciences and Engineering, 2019, 16(4): 2668-2696. doi: 10.3934/mbe.2019133 |
[3] | Guangxun Sun, Binxiang Dai . Stability and bifurcation of a delayed diffusive predator-prey system with food-limited and nonlinear harvesting. Mathematical Biosciences and Engineering, 2020, 17(4): 3520-3552. doi: 10.3934/mbe.2020199 |
[4] | Hongqiuxue Wu, Zhong Li, Mengxin He . Dynamic analysis of a Leslie-Gower predator-prey model with the fear effect and nonlinear harvesting. Mathematical Biosciences and Engineering, 2023, 20(10): 18592-18629. doi: 10.3934/mbe.2023825 |
[5] | Rongjie Yu, Hengguo Yu, Chuanjun Dai, Zengling Ma, Qi Wang, Min Zhao . Bifurcation analysis of Leslie-Gower predator-prey system with harvesting and fear effect. Mathematical Biosciences and Engineering, 2023, 20(10): 18267-18300. doi: 10.3934/mbe.2023812 |
[6] | Shunyi Li . Hopf bifurcation, stability switches and chaos in a prey-predator system with three stage structure and two time delays. Mathematical Biosciences and Engineering, 2019, 16(6): 6934-6961. doi: 10.3934/mbe.2019348 |
[7] | Fang Liu, Yanfei Du . Spatiotemporal dynamics of a diffusive predator-prey model with delay and Allee effect in predator. Mathematical Biosciences and Engineering, 2023, 20(11): 19372-19400. doi: 10.3934/mbe.2023857 |
[8] | Kawkab Al Amri, Qamar J. A Khan, David Greenhalgh . Combined impact of fear and Allee effect in predator-prey interaction models on their growth. Mathematical Biosciences and Engineering, 2024, 21(10): 7211-7252. doi: 10.3934/mbe.2024319 |
[9] | Ting Yu, Qinglong Wang, Shuqi Zhai . Exploration on dynamics in a ratio-dependent predator-prey bioeconomic model with time delay and additional food supply. Mathematical Biosciences and Engineering, 2023, 20(8): 15094-15119. doi: 10.3934/mbe.2023676 |
[10] | Christopher M. Kribs-Zaleta . Sharpness of saturation in harvesting and predation. Mathematical Biosciences and Engineering, 2009, 6(4): 719-742. doi: 10.3934/mbe.2009.6.719 |
Electricity markets have been liberalized worldwide, but the success of country specific experiences varied widely. Consumers' behavior is among the key factors for successful liberalization experiences namely regarding the decision to switch operator. This decision has been shown to be influenced by a multiplicity of factors. The goal of this article is to explore the analysis of the drivers for switching operator in a liberalized electricity market. With that purpose, we focused on the residential Portuguese case using a questionnaire. The logit estimation showed that men are more likely to switch supplier than women and that larger families are less likely to do so probably, due to the perception of high information search costs. Other sociodemographic variables were not found to be statistically significant. Regarding specific determinants, our results showed that past experiences with a supplier, dissatisfaction with the current operator, and family and friends' experiences were the most important determining factor for the decision to switch operator. Hence, the price was not the most important determinant. We also explored if different income groups had differentiated responses regarding the main drivers but concluded that there was no evidence that the income group affected the importance given to the price or to the other determinants for the decision.
Lotka and Volterra introduced a system of differential equations known as the Lotka-Volterra equations, which have served as a foundation for studying predator-prey dynamics in ecology [1,2,3]. Intraguild predation, an ecological phenomenon where competition and predation coexist in the food web, has garnered significant attention for its profound influence on populations, impacting their abundance, distribution, and even evolution [4,5,6]. In a seminal paper, Polis et al. [7] initially introduced a concept involving a two-species predator system, where one species consumes the other while they compete for shared resources. Subsequent scholars have made substantial advancements in this area [8]. For instance, Hart [9] focused on marine ecosystems, investigating top-down and bottom-up characteristics in model food webs to explain outcomes observed in trophic cascade experiments in lakes. Moeller et al.[10] proposed a mathematical model demonstrating how two plankton species, acting as mixotrophs, can coexist through resource competition. Safuan et al. [11] developed an intraguild predation model where resources are shared among predators and prey, introducing a carrying capacity proportional to biological resources. Resource availability, impacted by both predators and prey, plays a crucial role in system behavior [12,13,14]. Abundant and high-quality resources lead to stability, while scarce or degraded resources result in chaotic dynamics, limit cycles, or even chaos [15,16].
External factors such as harvesting also influence this ecological interaction [17,18]. Harvesting's impact on resource and fishery management is significant both economically and ecologically [19,20]. Chaudhuri [21] examined harvesting in the context of two competing species, providing a detailed analysis of equilibrium solutions. Das et al. [22] explored bioeconomic fish extraction in predator-prey fishery models, while Ang and Safuan [23] considered independent harvesting strategies for predators and prey with their own economic values. To address limitations of the unit harvest concept, Clark introduced Michaelis-Menten-type harvesting [24]. Research on the combination of this harvesting type in ecological models has revealed various bifurcation behaviors [25,26,27]. Sharif and Mohd [28] conducted a comprehensive analysis of harvesting and enrichment effects on intraguild predator fishery ecosystems, highlighting diverse dynamic behaviors.
In predator-prey systems, factors like food digestion, gestation, disease transmission, capture, and defense involve time delays [29,30,31]. Time delay differential equations exhibit intricate dynamics, especially when delays exceed a critical threshold, leading to Hopf bifurcation and oscillations in population sizes [32,33]. For example, Li et al. [34] investigated spatial memory and Allee effects in prey-predator systems with time delays. Other researchers have explored various delays and analyzed local stability, Hopf bifurcation, and stability paths using methods like the center manifold and paradigm theory [35,36,37].
To our knowledge, prior research has not explored a double-delayed predator-prey model with a Michaelis-Menten-type harvesting. This article specifically focuses on gestation delays in prey and predator populations. In Section 2, we establish the model's framework. Section 3 examines equilibrium points and their local stability under varying time delays, including Hopf bifurcation analysis. Section 4 investigates conditions affecting bifurcation direction and stability factors crucial to Hopf bifurcation. In Section 5, we employ numerical simulations to explore the system's complex behavior. Finally, we summarize key findings, draw conclusions, and discuss the significance of our analytical results to conclude the paper in Section 6.
Ang and Safuan in [27] presented an intricate ecological model that comprehensively captures the complex interplay among different species and their respective habitats:
{dXdt=r1X(1−XmZ)−aXY,dYdt=r2Y(1−YnZ)+bXY−cEYd1E+d2Y,dZdt=Z(g−uX−vY). | (2.1) |
This model not only elucidates the dynamics of predator and prey but also incorporates the dimension of human predation on the predator species, where X(t) and Y(t) denote the populations of a prey species and predator species over time, respectively. r1 and r2 represent the intrinsic growth rates of the prey, denoted as X, and the predator, denoted as Y, respectively. In this context, Z is used to signify a shared resource accessible to both prey and predator populations, with growth rates of m and n, respectively (0<m<1, 0<n<1), satisfying the constraint m+n=1. The parameter g characterizes the growth rate of biotic resources, and u and v represent the consumption rates of resources by prey and predator species, respectively. Furthermore, parameters a and b quantify the strength or intensity of the interaction between the prey and predator species. The catchability coefficient of predator fish is denoted as c, while E represents the harvesting effort applied to predator fish, with d1 and d2 as positive constants.
The primary focus of this work centered on exploring the long-term behavior of the system, utilizing the harvest of predator fish as a bifurcation parameter and examining the associated economic implications using Pontryagin's maximum principle. The bistable behavior within the system was leveraged to derive the optimal threshold for predator harvest, aiming to strike a balance between maintaining fishery resources and maximizing economic profit. In this study, our objective was to delve into an unexplored aspect of system dynamics, with a particular emphasis on the impact of time delays. We have thoroughly investigated the predator-prey model within an intraguild setting, incorporating the Michaelis-Menten harvest style and introducing two significant time delays. A key highlight of our research lied in our careful consideration of the gestation time delays for both predator and prey. This approach represents a promising avenue for gaining deeper insights into ecological relationships and mechanisms that yields the following model:
{dXdT=r1X(1−X(T−T1)mZ(T−T1))−aXY,dYdT=r2Y(1−Y(T−T2)nZ(T−T2))+bXY−cEYd1E+d2Y,dZdT=Z(g−uX−vY). | (2.2) |
Here, the constants T1 and T2 account for the gestation time delays of X and Y, respectively. T1(T2) represents the gestation delay in the presence of the prey (predator), i.e., after consuming the shared resource, the prey X (the predator Y) takes some time to convert the shared resource Z into prey (predator) biomass [38]. Now, let's introduce dimensionless variables: x=anX/mr2, y=aY/r2, z=anZ/r2, and t=r2T so we obtain the following dimensionless system:
{dxdt=αx(1−x(t−τ1)z(t−τ1))−xy,dydt=y(1−y(t−τ2)z(t−τ2))+βxy−δyσ+y,dzdt=z(ρ−εx−μy), | (2.3) |
where the dimensionless parameters are α=r1/r2, β=bm/an, δ=acE/d2r22, ρ=g/r2, ε=mu/an and μ=v/a. To ensure that the system is biologically feasible, it is assumed that all the parameters are positive. By the biological senses, the initial conditions are chosen as
x(θ)=ϕ1(θ)≥0,y(θ)=ϕ2(θ)≥0,z(θ)=ϕ3(θ)≥0,ϕi≥0,i=1,2,3,θ∈[−τ,0], | (2.4) |
where τ=max{τ1,τ2},(ϕ1(θ),ϕ2(θ),ϕ3(θ)∈C([−τ,0],R3+) is a continuous vector function in the Banach space mapping [−τ,0]→R3+ and R3+={(x1,x2,x3):xi≥0,i=1,2,3}.
Notice that in the absence of delays, i.e., τ1=τ2=0, model (2.3) is reduced to the one considered by Sharif and Mohd in [28]. Since the presence of delays does not affect the number and existence of equilibria, we can have the following lemma (see [28] for details).
Lemma 2.1. For model (2.3) with τ1=0 and τ2=0, the following statements are true.
(a) If μσ+ρ−δμ>0, there is a prey-free equilibrium E1=(0,ρμ,ρ(μσ+ρ)μ(ρ+μσ−δμ)), which is locally stable provided ρ−αμ>0 and (ρ+μσ)2−δμ2σ−2δμρ>0 hold.
(b) There is always a predator-free equilibrium E2=(ρε,0,ρε), which is locally stable provided δε−βρσ−εσ>0 holds.
(c) Coexistence equilibrium E∗ is given by the expression: E∗=(ρ−μˆyε,ˆy,α(μˆy−ρ)ε(ˆy−α)), where ˆy is the positive solution to the cubic equation Aˆy3+Bˆy2+Cˆy+D=0, satisfying ρ−μˆy>0 and α−ˆy>0. Here,
A=αβμ2+ε2, B=αβμ2σ+ε2σ−2αβμρ−αε2−αεμ,C=αβρ2+αδεμ+αερ−2αβμρσ−αε2σ−αεμσ, D=αβρ2σ+αερσ−αδερ. |
It has been revealed in [28] that in the absence of delay, model (2.3) can have up to three possible equilibria and exhibit complex dynamics, including tri- or bistability phenomena and multi-type bifurcations. In this paper, we will turn our main attention to the investigation of the effects of delay on the dynamics of model (2.3).
In this section, we will explore the local stability of the equilibrium point/s of model (2.3), as well as investigate whether or not a Hopf bifurcation can occur at any arbitrary equilibrium point where coexistence is achieved. By linearizing model (2.3), we can derive the characteristic equation at the equilibrium point E∗(x∗,y∗,z∗). This equation determines the behavior of the system near the equilibrium point and provides insight into the stability properties of the system. The representation of the characteristic equation can be stated as follows:
det(λ−α+y∗+αx∗z∗(e−λτ1+1)x∗−αx2∗z2∗e−λτ1−βy∗λ−1−βx∗+δσ(σ+y∗)2+y∗z∗(e−λτ2+1)−y2∗z2∗e−λτ2εz∗μz∗λ−ρ+εx∗+μy∗)=0. | (3.1) |
According to (3.1), we first study the local stability of the prey-free equilibrium E1 and the predator-free equilibrium E2.
Theorem 3.1. Consider system (2.3) for any τ1>0 and τ2>0. We have
(i) If there exists a prey-free equilibrium E1 (i.e., μσ+ρ−δμ>0), the prey-free equilibrium E1=(0,ρμ,ρ(μσ+ρ)μ(ρ+μσ−δμ)) is stable when ρ−αμ>0 and 0<τ1<τ20. Otherwise, it is unstable.
(ii) If εδ−εσ−βρσ>0 and 0<τ1<τ10, the predator-free equilibrium E2=(ρε,0,ρε) is stable. Otherwise, it is unstable.
Proof. (ⅰ) If the feasible criterion of μσ+ρ−δμ>0 is met, equilibrium E1 always exists. The characteristic equation (3.1) at E1 is of the form
(λ−α+ρμ)[λ2−ρδμ(μσ+ρ)2λ−μσ+ρ−δμμσ+ρ(λ−ρ)e−λτ2]=0. | (3.2) |
Obviously, α−ρμ is one root of Eq (3.2). Let G(λ):=λ2−ρδμ(μσ+ρ)2λ−μσ+ρ−δμμσ+ρ(λ−ρ)e−λτ2 and assume that λ=iω(ω>0) is a root of G(λ)=0. Then it follows that
{μσ+ρ−δμμσ+ρρcos(ωτ2)−μσ+ρ−δμμσ+ρωsin(ωτ2)=ω2,μσ+ρ−δμμσ+ρωcos(ωτ2)+μσ+ρ−δμμσ+ρρsin(ωτ2)=−ρδμ(μσ+ρ)2ω, | (3.3) |
which leads to
ω4+[ρ2δ2μ2(μσ+ρ)4−(μσ+ρ−δμμσ+ρ)2]ω2−(μσ+ρ−δμ)2(μσ+ρ)2ρ2=0. | (3.4) |
Notice that when −(μσ+ρ−δμ)2ρ2(μσ+ρ)2<0, Equation (3.4) has a unique positive root ω20=12[(μσ+ρ−δμμσ+ρ)2−ρ2δ2μ2(μσ+ρ)4+√Δ1], where Δ1=[(μσ+ρ−δμμσ+ρ)2−ρ2δ2μ2(μσ+ρ)4]2+4(μσ+ρ−δμ)2ρ2(μσ+ρ)2. Substituting ω20 into Eqs (3.3), we obtain
τ2n=1ω0{arccosω20[ρ−ρδμ(μσ+ρ)2]μσ+ρ−δμμσ+ρ(ρ2+ω20)+2nπ},n=0,1,2,…. | (3.5) |
Substituting λ(τ2) into the left-hand side of G=0 and taking the derivative with respect to τ2, we have
(dλdτ2)−1=μσ+ρ−δμμσ+ρ−(2λ−ρδμ(μσ+ρ)2)eλτ2μσ+ρ−δμμσ+ρ(λ−ρ)λ−τ2λ, | (3.6) |
which leads to
ℜ[(dλdτ2)−1]λ=iω0=ℜ[1(λ−ρ)λ]λ=iω0−ℜ[(2λ−ρδμ(μσ+ρ)2)eλτ2μσ+ρ−δμμσ+ρ(λ−ρ)λ]λ=iω0=2ω20+ρ2δ2μ2(μσ+ρ)4−(μσ+ρ−δμμσ+ρ)2(ω20+ρ2)(μσ+ρ−δμμσ+ρ)2=√Δ1(ω20+ρ2)(μσ+ρ−δμμσ+ρ)2>0. |
This suggests that the root crosses the imaginary axis from left to right at τ2=τ20, indicating that E1 becomes unstable.
(ⅱ) The characteristic equation of system (2.3) at E2 is
(λ−εσ+βρσ−εδεσ)(λ2+(αλ+ρα)e−λτ1)=0. | (3.7) |
Obviously, εσ+βρσ−εδεσ is a characteristic root of Eq (3.7), which is positive if εσ+βρσ−εδ>0, indicating that E2 is unstable. If εσ+βρσ−εδ<0, we only need to consider T(λ):=λ2+(αλ+ρα)e−λτ1=0. Assume that λ=iξ(ξ>0) is a root of T(λ)=0. Then we have
{αρcos(ξτ1)+αξsin(ξτ1)=ξ2,αξcos(ξτ1)−αρsin(ξτ1)=0, | (3.8) |
which leads to
ξ4−α2ξ2−α2ρ2=0. | (3.9) |
Because −α2ρ2<0, Equation (3.9) has a unique positive root ξ20=α2+√Δ22,Δ2=α4+4α2ρ2. Substituting ξ20 into Eq (3.8), we obtain
τ1n=1ξ0{arccosρξ20α(ρ2+ξ20)+2nπ},n=0,1,2,… | (3.10) |
Moreover, we can compute from T(λ)=0 that
(dλdτ1)−1=2λeλτ1+α(αλ+ρα)λ−τ1λ, | (3.11) |
which leads to
ℜ[(dλdτ1)−1λ=iξ0]=2αξ20(ρcos(ξ0τ1)+ξ0sin(ξ0τ1))+α2ξ20α2ξ40−ρ2α2ξ20=2ξ20−α2α2ξ20+ρ2α2=√Δ2α2ξ20+ρ2α2>0. |
This suggests that the root crosses the imaginary axis from left to right at τ1=τ10, indicating that E2 becomes unstable.
Next, let us delve into the local stability of E∗(x∗,y∗,z∗) and explore the possible Hopf bifurcations at E∗. E∗ satisfies the equation
{α(1−x∗z∗)−y∗=0,(1−y∗z∗)+βx∗−δσ+y∗=0,ρ−εx∗−μy∗=0. | (3.12) |
To simplify the analysis, let ˉx(t)=x(t)−x∗,ˉy(t)=y(t)−y∗,ˉz(t)=z(t)−z∗, and still denote x(t),y(t),z(t), respectively. The linearized part of the system (2.3) at E∗(x∗,y∗,z∗) is
{dxdt=−x∗y(t)−αx∗z∗x(t−τ1)+αx∗2z∗2z(t−τ1),dydt=βy∗x(t)+δy∗(σ+y∗)2y(t)−y∗z∗y(t−τ2)+y∗2z∗2z(t−τ2),dzdt=−εz∗x(t)−μz∗y(t). | (3.13) |
Therefore, the corresponding characteristic equation of system (3.10) is given by:
λ3+m2λ2+m1λ+(b2λ2+b1λ+b0)e−λτ1+(c2λ2+c1λ+c0)e−λτ2+(d1λ+d0)e−λ(τ1+τ2)=0, | (3.14) |
where
m2=−δy∗(σ+y∗)2,m1=βx∗y∗,b2=αx∗z∗,b1=εαx∗2z∗−αδx∗y∗(σ+y∗)2z∗,b0=αβμx∗2y∗z∗−αδεx∗2y∗(σ+y∗)2z∗,c2=y∗z∗,c1=μy∗2z∗,c0=−εx∗y∗2z∗,d1=αx∗y∗z∗2,and d0=αεx∗2y∗+μαx∗y∗2z∗2. |
In the following, we apply the method used in Ruan and Wei [39] to investigate the distribution of roots of the transcendental equation (3.14). As system (2.3) has two time delays, τ1 and τ2, we consider the following four cases.
Case 1: τ1=τ2=0.
The characteristic equation (3.14) becomes:
λ3+m12λ2+m11λ+m10=0, | (3.15) |
where
m10=αβμx∗2y∗z∗+αεx∗2y∗+μαx∗y∗2z∗2−αδx∗y∗(σ+y∗)2z∗−εx∗y∗2z∗,m11=x∗βy∗+αx∗z∗+μy∗2z∗+εαx∗2z∗−αδx∗y∗(σ+y∗)2z∗, m12=αx∗z∗+y∗z∗−δy∗(σ+y∗)2. |
By Routh-Hurwitz criterion, we can conclude that all of the roots of Eq (3.15) have negative real parts if and only if
(H1) m12>0,m10>0, m11m12>m10
are satisfied. Namely, when τ1=τ2=0, the coexistence equilibrium E∗ is locally asymptotically stable provided (H1) is satisfied.
Case 2: τ1>0, τ2=0.
The characteristic equation (3.14) becomes:
λ3+m22λ2+m21λ+m20+(b22λ2+b21λ+b20)e−λτ1=0, | (3.16) |
where
m22=m2+c2, m21=m1+c1, m20=c0, b22=b2, b21=b1+d1,and b20=b0+d0. |
Assume that λ=iω1(ω1>0) is a root of Eq (3.16). By separating the real part and imaginary part, we obtain
{(b20−b22ω21)cos(ω1τ1)+b21ω1sin(ω1τ1)=m22ω21−m20,b21ω1cos(ω1τ1)+(b22ω21−b20)sin(ω1τ1)=ω31−m21ω1, | (3.17) |
which yields
ω61+e22ω41+e21ω21+e20=0, | (3.18) |
where
e22=m220−b220, e21=m221−b221−2m20m22+2b20b22, and e20=m222−b222−2m21. |
Let ω21=x. Then Eq (3.18) becomes
h(x):=x3+e22x2+e21x+e20=0. | (3.19) |
We need only study the existence and number of positive roots for Eq (3.19). We can compute that
dh(x)dx=3x2+2e22x+e21. | (3.20) |
If △=e222−3e21≤0, we have dh(x)dx≥0, and thus h(x) monotonically increases for x∈[0,+∞). If △>0, Equation (3.20) has two different real roots:
x1∗=−e22+√e222−3e213, x2∗=−e22−√e222−3e213. | (3.21) |
Obviously, x∗2<x∗1, and x∗2 and x∗1 are respectively the local maximum point and local minimum point. Notice the geometric characteristics of the cubic polynomial equation and also that h(0)=e20 and limx→+∞h(x)=+∞. We can make clear the number and existence of positive roots of Eq (3.19). The detailed analyses are provided in Appendix A. Without loss of generality, we assume the conditions in (H2) hold, that is,
(H2) e20<0, Δ>0 and x∗2>0; h(x∗2)>0 and h(x∗1)<0.
In this situation, Equation (3.18) has three positive roots denoted respectively by ω1,i,i=1,2,3 (ω1,1<ω1,2<ω1,3). It then follows from Eq (3.17) that
cos(ω1τ1)=(m22ω21−m20)(b20−b22ω21)+b21ω1(ω31−m21ω1)(b20−b22ω21)2+b221ω21, | (3.22) |
from which we obtain that
τ(κ)1,i=1ω1,iarccos[(m22ω21,i−m20)(b20−b22ω21,i)+b21ω1,i(ω31,i−m21ω1,i)(b20−b22ω21,i)2+b221ω21,i]+2κπω1,i | (3.23) |
for κ=0,1,2,⋯;i=1,2,3.
Now we verify that the transversality condition holds. Differentiating both sides of Eq (3.16) with respect to τ1, we can obtain
(dλdτ1)−1=3λ2+2m22λ+m21−λ(λ3+m22λ2+m21λ+m20)+2b22λ+b21λ(b22λ2+b21λ+b20)−τ1λ. | (3.24) |
Through some sophisticated calculations, we get
ℜ{d(λ)dτ1}−1λ=iω1=h′(ω21)b221ω21+(b20−b22ω21)2. | (3.25) |
Lemma 3.2. Assume the conditions in (H2) hold. Then
sign{d(ℜλ)dτ1}λ=iω1,1=signh′(ω21,1)>0, |
sign{d(ℜλ)dτ1}λ=iω1,2=signh′(ω21,2)<0, |
sign{d(ℜλ)dτ1}λ=iω1,3=signh′(ω21,3)>0. |
Proof. 1) Based on (H2), we have h(0)=e20<0, h(x∗2)>0, and h′(x)>0∈[0,x∗2]. By the zero existence theorem, we know that there exists a unique ω21,1∈(0,x∗2), and, in addition, we have h′(ω21,1)>0.
2) From (H2), we may obtain h(x∗2)>0,h(x∗1)<0, and h′(x)<0∈[x∗2,x∗1]. By the zero existence theorem, we know that there exists a unique ω21,2∈(x∗2,x∗1), so h′(ω21,2)<0 is verified.
3) Finally, verify the symbol at h′(ω21,3). By the zero existence theorem, h(x∗1)<0,limx→+∞h(x)=+∞, and h′(x)>0∈[x∗2,+∞), so we know that there exists a unique ω21,3∈(x∗2,+∞), and in addition we have h′(ω21,3)>0.
For the case when the characteristic equation (3.16) has three pairs of conjugate complex roots, the process of analyzing stability and switching phenomena can be relatively complex. As the time delay τ1 increases gradually and passes through the critical values of τ(κ)1,1 and τ(κ)1,3, the system may undergo a transition from stable to unstable, and as the time delay τ1 passes through the critical values of τ(κ)1,2, the system may be switched from unstable back to stable. Thus, stability switch phenomena may occur and the specific situations of stabilizing switches depend on the relative positions of these critical time-delay values.
For the sake of narrative convenience, we reorder all the critical values {τ(κ)1,i}, i=1,2,3;κ=0,1,2,⋯ as {τ(κ)1}, κ=0,1,2,⋯ such that 0<τ(0)1<τ(1)1<τ(2)1<⋯, and assume that the system undergoes a stability switch from stable to unstable and then from unstable to stable, and after finite times like this, the system becomes unstable eventually. We summarize these as the following theorem.
Theorem 3.3. For system (2.3) with τ1>0 and τ2=0, if (H1) and (H2) hold, then there exists a M∈N such that when τ1∈(0,τ(0)1) ⋃(τ(1)1,τ(2)1)⋃⋯⋃ (τ(M−1)1,τ(M)1), all the roots of Eq (3.16) have negative real part, and thus the system of (2.3) is locally asympotically stable; when τ1∈(τ(0)1,τ(1)1)⋃ (τ(2)1,τ(3)1)⋃⋯⋃(τ(M)1,+∞), at least one root of Eq (3.16) has a positive real part, and thus the system of (2.3) is unstable. In addition, the model undergoes a Hopf bifurcation at E∗ when τ1=τ(κ)1,κ∈N.
Case 3: τ2>0,τ1=0.
Analyzing similarly as in Case 2, we have the following theorem.
Theorem 3.4. For system (2.3) with τ2>0 and τ1=0, the equilibrium E∗ may undergo a finite number of stability switches, that is there is a N∈N such that it is locally asymptotically stable for τ2∈(0,τ(0)2)⋃(τ(1)2,τ(2)1)⋃⋯⋃(τ(N−1)2,τ(N)2); and when τ2∈(τ(0)2,τ(1)2)⋃(τ(2)2,τ(3)2)⋃⋯⋃(τ(N)2,+∞), the equilibrium point E∗ is unstable. Additionally, model (2.3) undergoes a Hopf bifurcation at E∗ for each τ2=τ(κ)2,κ∈N.
Case 4: τ1>0,τ2>0.
In this case, we choose τ1 as the bifurcation parameter and τ2 in one of its stable intervals, say (0,τ(0)2) (the same below). Assume that λ=iω∗1(ω∗1>0) is a characteristic root of Eq (3.14), then we can obtain
N1(ω∗1,τ2)=K1(ω∗1,τ2)cosω∗1τ∗1−K2(ω∗1,τ2)sinω∗1τ∗1,N2(ω∗1,τ2)=K2(ω∗1,τ2)cosω∗1τ∗1+K1(ω∗1,τ2)sinω∗1τ∗1, | (3.26) |
where
K1(ω∗1,τ2)=b1ω∗1+d1ω∗1cos(ω∗1τ2)−d0sin(ω∗1τ2),K2(ω∗1,τ2)=−b2ω∗21+b0+d1ω∗1sin(ω∗1τ2)+d0cos(ω∗1τ2),N1(ω∗1,τ2)=ω∗31−m1ω∗1−c1ω∗1cos(ω∗1τ2)−c2ω∗21sin(ω∗1τ2)+c0sin(ω∗1τ2),N2(ω∗1,τ2)=m2ω∗21+c2ω∗21cos(ω∗1τ2)−c1ω∗1sin(ω∗1τ2)−c0cos(ω∗1τ2). |
Then, adding the squares of the above two equations, we obtain
G1(ω∗1)+G2(ω∗1)sin(ω∗1τ2)+G3(ω∗1)cos(ω∗1τ2)=0, | (3.27) |
where
G1(ω∗1)=ω∗61+(c22+m22−b22−2m1)ω∗41+(m21+c21−2c0c2−b21−d21+2b0b2)ω∗21+c20−d20−b20,G2(ω∗1)=−2c2ω∗51+(2c0+2c2m1−2c1m2+2b2d1)ω∗31+(2b1d0−2b0d1)ω∗1,G3(ω∗1)=(−2c1+2c2m2)ω∗41+(2c1m1−2c0m2−2b1d1+2b2d0)ω∗21. |
It is difficult to discuss the roots of Eq (3.27). To get the main conclusion, we assume that Eq (3.27) has a finite number of positive real roots ω∗1,i(i=1,2,⋯,h) and that for each ω∗1,i, there is a series of {τ(ζ)1,i|i=1,2,⋯,h;ζ=0,1,2,⋯} satisfying Eq (3.27), where
τ(ζ)1,i(τ2)=1ω∗1,iarccos(N1(ω∗1,i,τ2)K1(ω∗(i)1,τ2)+N2(ω∗1,i,τ2)K2(ω∗1,i,τ2)K21(ω∗1,i,τ2)+K22(ω∗1,i,τ2))+2ζπω∗1,i. | (3.28) |
Let τ∗10(τ2)=min{τ(ζ)1,i(τ2)|i=1,2,⋯,h;ζ=0,1,2,⋯}. When τ1=τ∗10(τ2), Equation (3.28) has a pair of purely imaginary roots iω∗10 for τ2 in its stable intervals. Of course, we should verify the transversality condition. Taking the derivative on both sides of Eq (3.14) with respect to τ1 and substituting λ=iω∗10, we get
ℜ(dλdτ1)−1λ=iω∗10=ℜ(R1+iM1R2+iM2)−1λ=iω∗10=R1R2+M1M2R22+M22, | (3.29) |
where
R1=(−3ω∗210+m1)cos(ω∗10τ∗10)−2m2ω∗10sin(ω∗10τ∗10)+(c2τ2ω∗210−c0τ2+c1)cos(ω∗10(τ∗10−τ2))+(c1ω∗10τ2−2c2ω∗10)sin(ω∗10(τ∗10−τ2))+(d1−d0τ∗10)cos(ω∗10τ2)−d1ω∗10τ∗10sin(ω∗10τ2)+b1,M1=2m2ω∗10cos(ω∗10τ∗10)+(−3ω∗210+m1)sin(ω∗10τ∗10)(2c2ω∗10−c1ω∗10τ2)cos(ω∗10(τ∗10−τ2))+(ω∗210c2τ2−c0τ2+c1)sin(ω∗10(τ∗10−τ2))−d1ω∗10cos(ω∗10τ2)+(τ∗10d0−d1)sin(ω∗10τ2)+2b2ω∗10,R2=−b1ω∗210−d1ω∗210cos(ω∗10τ2)+d0ω∗10sin(ω∗10τ2),M2=−b2ω∗310+b0ω∗10+d1ω∗210sin(ω∗10τ2)+d0ω∗10cos(ω∗10τ2). |
We make the following hypothesis:
(H3) sign{[d(ℜλ)(dτ1)]−1}λ=iω∗10=R1R2+M1M2≠0.
Using the same logic as above, model (2.3) can exhibit the phenomenon of stability switches. For convenience, here we assume that once E∗ loses its stability, it will be unstable forever. Then we will see the following results.
Theorem 3.5. For model (2.3) with τ1>0 and τ2 in its stable intervals, if (H1) and (H3) hold, then the equilibrium E∗ is asymptotically stable when τ1∈(0,τ∗10(τ2)) and unstable for τ1∈(τ∗10(τ2),+∞). Moreover, model (2.3) undergoes a Hopf bifurcation at the equilibrium E∗ for each τ1=τ∗10(τ2).
In this section, we shall discuss the direction and stability of the Hopf bifurcation periodic solution of system (2.3) with respect to τ1 and τ2∈[0,τ(0)2). Using the normal method of Hassard [40] and the center manifold theory, for τ2∈[0,τ(0)2), we derive explicit formulas to determine the properties of the Hopf bifurcation at the critical value τ1=τ∗10. For the readability of the article, we defer the detailed derivation to Appendix B.
Using Hassard's method [40], we can calculate g21 and compute the following values determining the qualitative behavior of the bifurcating periodic solutions at τ=τ∗10:
c1(0)=i2τ∗10ω∗10(g11g20−|2g11|2−|g02|23)+g212,μ2=−ℜ{c1(0)}ℜ{λ′(τ∗10)},β2=2ℜ{c1(0)},T2=−ℑ{c1(0)}+μ2ℑ{λ′(τ∗10)}τ∗10ω∗10, | (4.1) |
where μ2 determines the direction of the Hopf bifurcation: if μ2>0 (μ2<0), then the Hopf bifurcation is supercritical (subcritical). The stability of the bifurcating periodic solutions is determined by the sign of β2: if β2<0 (β2>0), the bifurcating periodic solutions are stable (unstable). The period of the bifurcating periodic solutions is determined by the sign of T2: if T2>0 (T2<0), the bifurcating periodic solutions increase (decrease).
Based on the results of the stability analysis and bifurcation discussed in Sections 2 and 3, we will conduct numerical simulations to examine the impact of time delays on the stability and periodic solutions of system (2.3). These simulations will allow us to observe the effect of delay on the behavior of the system and the effect of changes in harvest rate on latency. By studying these aspects, we can better understand the dynamics of the system in different situations [41,42].
Let the parameters of system (2.3) be α=0.7, β=0.08, δ=0.215, σ=0.2, ρ=1.165, ε=0.75, and μ=3.55. (If more data details are required, please refer to [27]), then we can get the following prey-free equilibrium E1=(0,0.32817,0.55347), predator-free equilibrium E2=(1.6643,0,1.6643) and the coexistence equilibrium E∗=(0.293,0.2704,0.4774). By Theorem 3.1, because α−ρμ≈0.3718>0, and εσ+βρσ−εδ≈0.0074>0, we know prey-free E1 and predator-free E2 are unstable. For τ1=0,τ2=0, under the above parameters, we can check that the conditions in (H1) are satisfied, and therefore the coexistence equilibrium E∗ of system (2.3) is asymptotically stable (see Figure 1).
When the time delay τ1 is within the range [0,τ(0)1), the coexistence equilibrium E∗ is still asymptotically stable (see Figure 2). However, once τ1 surpasses the critical value τ(0)1=2.7415 (at this point ω1,1=0.5618), the coexistence equilibrium E∗ becomes unstable, leading to a Hopf bifurcation. This bifurcation results in the emergence of a family of periodic solutions originating from the coexistence equilibrium E∗. In summary, the stability of E∗ changes at τ(0)1, and this change triggers the appearance of periodic solutions (see Figure 3).
Similarly, we have ω1,2=0.8895,τ(0)2=0.5856, and the corresponding figures are Figures 4 and 5.
For τ1>0,τ2=0.2∈(0,τ(0)2), we have ω∗10=0.1269,τ∗10=0.2885. According to Theorem 3.5, E∗ is asymptotically stable when τ1∈[0,0.2885) (see Figure 6) and unstable when τ1>τ∗10. After the computation of formula (4.1), we can obtain c1(0)=−0.2225+1.3699i, β2=−0.4449<0, μ2=3.2038>0, and T2=−0.5009<0. The Hopf bifurcation is characterized as a supercritical bifurcation, where the periodic solutions are stable. This can be visually represented in Figure 7.
For an intraguild predator-prey fishery model, a stable positive equilibrium indicates a balance among the prey, predators, and the resource they are competing for. This essentially means the fishery resources are being used in a sustainable way. On the flip side, when the positive equilibrium E∗ loses its stability, it will lead to an emergence of a stable periodic solution.
Next, we consider the effect of the predator harvest parameter δ on the dynamics of the system. We can see from Figure 8 that the predator harvest parameter δ can have a significant effect on the sizes of two species and the quantity of resources, and meanwhile it can also affect the critical values of two delays: as δ increases, x∗ and z∗ increase, and y∗ decreases, suggesting that the degree of depredation by the predator changes the numbers of predators and prey. Keeping τ2=0.2 constant, the higher the degree of predator capture, the smaller the corresponding τ1 critical value τ∗10 will be, indicating that the degree of capture destabilizes the corresponding system (2.3).
In this paper, we have explored an intraguild prey-predator model (2.3) featuring two delays and a Michaelis-Menten-type harvesting. First, we analyzed the corresponding characteristic equation and discussed the stability of the prey-free equilibrium E1 and the predator-free equilibrium E2. According to the Hopf bifurcation theorem, we investigated the conditions for equilibrium stability and the existence of Hopf bifurcations. We employed the normal form theory and the center manifold theorem and obtained some explicit results. Specifically, when τ2∈[0,τ(0)2), we explored the stability and direction of Hopf bifurcations for varying values of τ1. Finally, we validated our theoretical findings through numerical simulations.
In ecological systems, the predator, the prey, and the shared resources are expected to persist within a given set of parameters. The local bifurcation observed in such systems has ecological significance. This means that changes in the parameters can result in ecologically relevant shifts in the population dynamics of the predator and prey, along with their interactions with shared resources. In addition, when both delays surpass their critical values, they can have a significant impact on the stability of the system and cause various changes in its properties and behaviors. An increase in predator harvesting parameter δ may lead to unstable behaviors and phenomena that will influence the efficient use of shared resources by prey and predators, even if the number of predators has decreased.
To maintain sustainable fishing practices without depleting resources or driving predator species to extinction, it is crucial to employ qualitative analysis and numerical simulations in research. These methods provide insights into the dynamic behavior of ecosystems, enabling us to set limits and strike a balance. In essence, they help determine the level of fishing that is sustainable without harming the ecosystem. Overfishing can disrupt marine ecosystems, so selecting an appropriate value for δ to achieve a balance between resource sustainability and maximizing benefits in the presence of time delays is a critical concern, which we leave for future research to address.
The authors declare they have not used Artificial Intelligence (AI) tools in the creation of this article.
The authors would like to thank the National Natural Science Foundation of China (11671260; 12071293) for supporting this work.
The authors announce that there are no conflicts of interest. Sanling Yuan and Tonghua Zhang are the editorial board members for Mathematical Biosciences and Engineering and were not involved in the editorial review or the decision to publish this article.
1) If e20>0, then we have
(a) If Δ≤0, then Eq (3.19) has no positive roots.
(b) If Δ>0, then we have
● when x∗1≤0, then Eq (3.19) has no positive roots.
● when x∗1>0 and h(x∗1)>0, then Eq (3.19) has no positive roots.
● when x∗1>0 and h(x∗1)=0, then Eq (3.19) has one positive double root (i.e., x∗1).
● when x∗1>0 and h(x∗1)<0, then Eq (3.19) has two different positive roots.
2) If e20=0, then we have
(a) If Δ≤0, then Eq (3.19) has no positive roots.
(b) If Δ>0, then we have
● when x∗1≤0, then Eq (3.19) has no positive roots.
● when x∗2≤0<x∗1, then Eq (3.19) has one positive root.
● when x∗2>0 and h(x∗1)>0, then Eq (3.19) has no positive roots.
● when x∗2>0 and h(x∗1)=0, then Eq (3.19) has one positive double root (i.e., x∗1).
● when x∗2>0 and h(x∗1)<0, then Eq (3.19) has two different positive roots.
3) If e20<0, then we have
(a) If Δ≤0, then Eq (3.19) has one positive root.
(b) If Δ>0, then we have
● when x∗2≤0, then Eq (3.19) has one positive root.
● when x∗2>0 and h(x∗1)h(x∗2)>0, then Eq (3.19) has one positive root.
● when x∗2>0, h(x∗2)=0 (respectively, h(x∗1)=0), then Eq (3.19) has one positive double root x∗2 (respectively, x∗1) and one positive root.
● when x∗2>0, h(x∗2)>0 and h(x∗1)<0, then Eq (3.19) has three positive roots.
To summarize, we can have the following results on Eq (3.18).
Lemma A.1. The following statements are true.
1) If one of the following four conditions holds, Equation (3.18) will have on positive roots.
(i) e20≥0 and Δ≤0;
(ii) e20≥0, Δ>0 and x∗1≤0;
(iii) e20>0, Δ>0 and min{x∗1,h(x∗1)}>0;
(iv) e20=0, Δ>0 and min{x∗2,h(x∗1)}>0.
2) If one of the following three conditions is satisfied, Equation (3.18) will have only one positive root.
(i) e20=0, Δ>0 and x∗2≤0<x∗1.
(ii) e20<0, Δ>0 and x∗2≤0.
(iii) e20<0, Δ>0 and min{x∗2,h(x∗1)h(x∗2)}>0.
3) If either of the following two conditions is satisfied, Equation (3.18) will possess two positive roots:
(i) e20>0, Δ>0 and x∗1>0 and h(x∗1)<0;
(ii) e20=0, Δ>0 and x∗2>0 and h(x∗1)<0;
4) Equation (3.18) has three positive roots provided the following conditions hold:
(H2) e20<0, Δ>0, x∗2>0, h(x∗2)>0, and h(x∗1)<0.
5) Equation (3.18) has one double positive root provided one of the following two conditions holds:
(i) e20>0, Δ>0, x∗1>0, and h(x∗1)=0; (ii) e20=0, Δ>0, x∗2>0, and h(x∗1)=0;
6) Equation (3.18) has two positive roots (a single root and a double root) provided the following conditions hold:
e20<0, Δ>0, x∗2>0, and h(x∗2)=0 (or h(x∗1)=0).
Let τ1=τ∗10+μ,μ∈R, then μ=0 is the Hopf bifurcation value of the system. Let t=sτ1, x(sτ1)=ˆx(s), y(sτ1)=ˆy(s), z(sτ1)=ˆz(s), and denote x=ˆx(s), y=ˆy(s), z=ˆz(s), and t=s, then system (2.3) can be written as a functional differential equation in C=C([−1,0],R3):
˙X(t)=LμXt+f(μ,Xt), | (B.1) |
where X(t)=(x(t),y(t),z(t))T∈R3, Xt(θ)=X(t+θ)=(x(t+θ),y(t+θ),z(t+θ))T∈C, and Lμ:C→R3, F:R×C→R3 are given by
Lμ(ϕ)=(τ∗10+μ)[Aϕ(0)+Bϕ(−1)+Cϕ(−τ2τ∗10)], | (B.2) |
and
F(μ,ϕ)=(τ∗10+μ)(F1F2F3), | (B.3) |
where
A=(0−x∗0βy∗δy∗(σ+y∗)20−εz∗−μz∗0),B=(−αx∗z∗0αx∗2z∗2000000),C=(0000−y∗z∗y∗2z∗2000), |
F1=h1ϕ1(0)ϕ2(0)−h2ϕ1(0)ϕ1(−1)+h3ϕ1(0)ϕ3(−1)+h3ϕ1(−1)ϕ3(−1)+h4ϕ23(−1)+h5ϕ1(0)ϕ1(−1)ϕ3(−1)+h6ϕ1(0)ϕ23(−1)+h6ϕ1(−1)ϕ23(−1)+h7ϕ33(−1),F2=k1ϕ1(0)ϕ2(0)+k2ϕ22(0)+k3ϕ2(0)ϕ2(−τ2τ∗10)+k4ϕ2(−τ2τ∗10)ϕ3(−τ2τ∗10)+k4ϕ2(0)ϕ2(−τ2τ∗10)+k5ϕ23(−τ2τ∗10)+k6ϕ32(0)+k7ϕ2(0)ϕ2(−τ2τ∗10)ϕ3(−τ2τ∗10)+k8ϕ2(0)ϕ23(−τ2τ∗10)+k8ϕ2(−τ2τ∗10)ϕ23(−τ2τ∗10)+k9ϕ33(−τ2τ∗10),F3=l1ϕ2(0)ϕ3(0)+l2ϕ1(0)ϕ3(0), |
where
h1=−1,h2=−αz∗,h3=αx∗z∗2,h4=−αx∗2z∗3,h5=αz∗2,h6=−αx∗z∗3,h7=−αx∗2z∗4,k1=β,k2=σδ(σ+y∗)3,k3=−1z∗,k4=y∗z∗2,k5=−y∗2z∗2,k6=−δσ(σ+y∗)4,k7=−1z∗2,k8=−y∗z∗3,k9=−y∗2z∗4,l1=−μ,l2=−ε. |
By the Riesz representation theorem, there exists a 3×3 matrix function η(θ,μ) of bounded variatation for θ∈[−1,0], such that
Lμϕ=∫0−1dη(θ,μ)ϕ(θ),for ϕ∈C. | (B.4) |
In fact, we can choose
η(θ,μ)=(τ∗10+μ)[Aδ(θ)+Bδ(θ+1)+Cδ(θ+τ2τ∗10)], | (B.5) |
where δ(θ) is the Dirac delta function.
For ϕ∈C([−1,0],R3), define
A(μ)ϕ={dϕ(θ)dθ,−1≤θ<0,∫0−1dη(θ,μ)ϕ(θ),θ=0, |
and
R(μ)ϕ={0,−1≤θ<0,F(μ,ϕ),θ=0. |
Then this can be transformed into the following operator equation
˙Xt=A(μ)Xt+R(μ)Xt, | (B.6) |
which is a functional differential equation in C([−1,0];R3).
Denote A=A(0),
A∗(μ)ϕ={−dψ(s)ds,0<s⩽1,∫0−1dηT(t,0)ψ(−t),s=0, |
and
<ψ(s),ϕ(θ)>=¯ψ(0)ϕ(0)−∫0−1∫θξ=0¯ψ(ξ−θ)dη(θ,0)ψ(ξ)dξ, |
where ψ∈C∗([0,1],(R3)∗). Then A∗ are adjoint operators of A. If ±iω1kτ1k are eigenvalues of A, they are eigenvalues of A∗. Suppose that q(θ)=(1,q2,q3)Teiω10∗τ10∗θ is the eigenvector of A corresponding to iω∗10τ∗10, that is Aq(θ)=iω∗10τ∗10q(θ). Then we can obtain that
q2=−(αεx∗2z∗+αx∗z∗iω∗10)e−iω∗10τ∗10−ω∗10z∗2x∗z∗2iω∗10+αμx∗2z∗e−iω∗10τ∗10,q3=iω∗10(−εx∗z∗2+αμx∗z∗e−iω∗10τ∗10+iω∗10)−x∗z∗ω∗210+αμx∗2iω∗10e−iω∗10τ∗10. |
Let q∗(s)=D(1,q∗2,q∗3)eiω∗10τ∗10s be an eigenvector of A∗corresponding to −iω∗10τ∗10, then we have
q2∗=ω∗210z∗2+(iω∗10αx∗z∗−αεx∗2z∗)eiω∗10τ∗10βy∗z∗2iω∗10+εy∗2z∗eiω∗10τ∗2,q3∗=(−αx∗y∗2eiω∗10τ∗10+iω∗10)eiω∗10τ2+αβx∗2y∗z∗eiω∗10τ∗10βy∗z∗3iω∗10+εy∗2z∗2eiω∗10τ2. |
For this equation, we can get
<q∗(s),q(θ)>=¯q∗(0)q(0)−∫0−1∫θξ=0¯q∗(ξ−θ)dη(θ)q(ξ)dξ=¯D(1,¯q∗2,¯q∗3)(1,q2,q3)T−¯D∫0−1∫θξ=0(1,¯q∗2,¯q∗3)eiω∗10τ∗10(θ−ξ)dη(θ)(1,q2,q3)Teiω∗10τ∗10ξdξ=¯D[1+¯q∗2q2+¯q∗3q3−∫0−1(1,¯q∗2,¯q∗3)θeiω∗10τ∗10θdη(θ)(1,q2,q3)T]=¯D[1+¯q∗2q2+¯q∗3q3+τ∗10(αx∗z∗−αx∗2z∗2q3)e−iω∗10τ∗10+τ2(y∗z∗ q2¯q∗2−y∗2z∗2q3¯q∗2)e−iω∗10τ2]. |
Thus, one can choose D as
D=[1+¯q2q2∗+¯q3q∗3+τ∗10(αx∗z∗−αx∗2z∗2q3)eiω∗10τ∗10+τ2(y∗z∗q2¯q∗2−y∗2z∗2q3¯q∗2)eiω∗10τ2]−1, | (B.7) |
which satisfies <q∗(s),q(θ)>=1.
In the rest of this section, applying the methods from [40] along with similar computational procedures should enable us to ascertain the coefficients for determining both the Hopf bifurcation's direction and the stability of the bifurcating periodic solutions.
g20=2τ∗10¯D(h4q23e−2iω∗10τ∗10+h3q3e−iω∗10τ∗10+h1q2+((k4q2q3+k5q23)e−2iω∗10τ2+(k3q22+k4q2q3)e−iω∗10τ2+k2q22+k6q22+k1q2)¯q∗2+(l1q2q3+l2q3)¯q∗3),g11=τ∗10¯D(2h4¯q3q3+(h3¯q3+h3q3)e−iω∗10τ∗10+k4q2¯q3+2Re(h1q2)+k4¯q2q3e−iω10τ2+(k4q3¯q2+2k5q3¯q3+k3q2¯q2(e−iω∗10τ2+eiω∗10τ2)+k4q2¯q3eiω∗10τ2+2(k2+k6)q2¯q2+k1¯q2+k1q2)¯q∗2+(l1¯q2q3+l1q2¯q3+l22¯q3q3)¯q∗3),g02=2τ∗10¯D(h4¯q23e2iω∗10τ∗10+h3¯q3eiω∗10τ∗10+h1¯q2+(k4¯q2¯q3+k5¯q23)e2iω∗10τ2+k3¯q22eiω∗10τ2+k4¯q2¯q3eiω∗10τ2+(k2+k6)¯q22+k1¯q2)¯q∗2+(l1¯q2¯q3+l2¯q3)¯q∗3),g21=τ∗10¯D(2h3q3W(1)11(0)e−iω∗10τ∗10+h3¯q3W(1)20(0)eiω∗10τ∗10h3¯q3W(1)20(−1)eiω∗10τ∗10+2h4¯q3W(3)20(−τ2τ∗10)eiω∗10τ∗10+4h4q3W(3)11(−τ2τ∗10)e−iω∗10τ∗10+2h3q3e−iω∗10τ∗10+2h3q3W(1)11(−1)e−iω∗10τ∗10−2h2W(1)11(−1)+h1W(2)20(0)−h2W(1)20(−1)+h3W(3)20(−τ2τ∗10)+2h1W(2)11(0)+2h3W(3)11(−τ2τ∗10)−2h2+2h6q23e2iω∗10τ∗10+2h1q2W(1)11(0)+h1¯q2W(1)20(0)(4h6q3¯q3+6h7q23¯q3e−iω∗10τ∗10))+(6k9¯q3q23e−iω∗10τ2+2k7q22¯q3+2k8¯q2q23e−iω∗10τ2+2k7q2¯q2q3e−2iω∗10τ2+k1W(2)20(0)+2k1W(2)11(0)+k4¯q3W(2)20(−τ2τ∗10)eiω∗10τ2+4k5q3W(3)11(−τ2τ∗10)e−iω2τ∗10+2k5¯q3W(3)20(−τ2τ∗10)eiω∗10τ2+2k8¯q2q23e−2iω∗10τ2+2k3q2W(2)11(0)eiω∗10τ2+2k3q2W(2)11(0)e−iω∗10τ2+k3¯q2W(2)20(0)eiω∗10τ2+2k4q3W(2)11(0)e−iω∗10τ2+k4¯q3W(2)20(0)eiω∗10τ2+2k4q2e−iω∗10τ2W(3)11(−τ2τ∗10)+k4¯q2W(3)20(−τ2τ∗10)eiω∗10τ2+2k4q2W(2)11(−τ2τ∗10)eiω∗10τ2+2k1q2W(1)11(0)+4k2q2W(2)11(0)+2k3q2W(2)11(−τ2τ∗10)+2k4q2W(3)11(−τ2τ∗10)+4k6q2W(2)11(0)+k1¯q2W(1)20(0)+2k2¯q2W(2)20(0)+k3¯q2W(2)20(−τ2τ∗10)+k4¯q2W(3)20(−τ2τ∗10)+2k6¯q2W(2)20(0)+2k7q2¯q2q3+4k8q2q3¯q3+4k8q2q3¯q3e−iω∗10τ2)¯q∗2+(l2W(3)20(0)+2l2W(3)11(0)+2l1q2W(3)11(0)+2l1q3W(2)11(0)+2l2q3W(1)11(0)¯q3+l1¯q2W(3)20(0)+l1¯q3W(2)20(0)+l2¯q3W(1)20(0))¯q∗3. |
However,
W20(θ)=ig20ω∗10τ∗10q(0)eiω∗10τ∗10θ+i¯g023ω∗10τ∗10¯q(0)e−iω∗10τ∗10θ+M1e2iω∗10τ∗10θ,W11(θ)=−ig11ω∗10τ∗10q(0)eiω∗10τ∗10θ+i¯g11ω∗10τ∗10¯q(0)e−iω∗10τ∗10θ+M2. | (B.8) |
Here M1=(M11,M21,M31)T∈R3 and M2=(M12,M22,M32)T∈R3 are also constant vectors and can be determined by the following equations, respectively.
(2iω∗10+αx∗z∗e−2iω∗10τ∗10x∗−αx∗2z∗2e−2iω∗10τ∗10−βy∗2iω∗10−δy∗(σ+y∗)2+y∗z∗e−2iω∗10τ2−y∗2z∗2e−2iω∗10τ∗2εz∗μz∗2iω∗10)M1=2(Q1Q2Q3), |
(−αx∗z∗−x∗αx∗2z∗2βy∗δy∗(σ+y∗)2−y∗z∗y∗2z∗2−εz∗−μz∗0)M2=−(P1P2P3), | (B.9) |
with
Q1=h4q23e−2iω∗10τ∗10+h3q3e−iω∗10τ∗10+h1q2,Q2=(k4q2q3+k5q23)e−2iω∗10τ2+(k3q22+k4q2q3)e−iω∗10τ2+k2q22+k6q22+k1q2,Q3=l1q2q3+l2q3,P1=2h4¯q3q3+(h3¯q3+h3q3)e−iω∗10τ∗10+2ℜ(h1q2),P2=(k4q3¯q2+k4q2¯q3+2k5q3¯q3+k3q2¯q2(e−iω∗10τ2+eiω∗10τ2)+k4¯q2q3e−iω∗10τ2+k4q2¯q3eiω∗10τ2+2(k2+k6)q2¯q2+k1¯q2+k1q2),P3=l1¯q2q3+l1q2¯q3+l2¯q3l2q3. |
[1] |
Aliabadi DE, Chan K (2022) The emerging threat of artificial intelligence on competition in liberalized electricity markets: A deep Q-network approach. Appl Energy 32: 119813. https://doi.org/10.1016/j.apenergy.2022.119813 doi: 10.1016/j.apenergy.2022.119813
![]() |
[2] |
Al-Sunaidy A, Green R (2006) Electricity deregulation in OECD countries. Energy 31: 769–787. https://doi.org/10.1016/j.energy.2005.02.017 doi: 10.1016/j.energy.2005.02.017
![]() |
[3] | Ariu T, Lewis PE, Goto H, et al. (2012) Impacts and Lessons from the Fully Liberalized European Electricity Market - Residential Customer Price, Switching and Services. Central Research Institute of Electric Power Industry Report, No. Y11018. |
[4] |
Armstrong M, Sappington D (2006) Regulation, Competition, and Liberalization. J Econ Lit 44: 325–366. https://doi.org/10.1257/jel.44.2.325 doi: 10.1257/jel.44.2.325
![]() |
[5] | Borenstein S, Bushnell J (2000) Electricity restructuring: deregulation or reregulation. Regulation 23: 46–52. |
[6] |
Defeuilley C (2009) Retail competition in electricity markets. Energy Policy 37: 377–386. https://doi.org/10.1016/j.enpol.2008.07.025. doi: 10.1016/j.enpol.2008.07.025
![]() |
[7] | Drosos D, Grigorios LK, Arabatzis G, et al. (2020) Evaluating Customer Satisfaction in Energy Markets Using a Multicriteria Method: The Case of Electricity Market in Greece. Sustainability 12: 3862. https://doi.org/10.3390/su12093862 |
[8] | ERSE (2013) Extinction of regulated tariffs for electricity and natural gas. Available from: http://www.erse.pt/eng/Paginas/extinctiontariffs.aspx. |
[9] | ERSE (2021) Tarifas e preços para a energia elétrica e outros serviços em 2022 e parâmetros para o período de regulação 2022–2025. |
[10] | ERSE (2021) Relatório sobre os mercados retalhistas de eletricidade e de gás natural em Portugal. |
[11] | Entidade Reguladora dos Serviços Energético (ERSE) (2021) Síntese Mensal, November 2021. |
[12] |
Ek K, Söderholm P (2008) Households' switching behavior between electricity suppliers in Sweden. Util Policy 16: 254–261. https://doi.org/10.1016/j.jup.2008.04.005. doi: 10.1016/j.jup.2008.04.005
![]() |
[13] |
Ferreira P, Araújo M, O'Kelly MEJ (2006) An overview of the portuguese electricity market. Energy Policy 35: 1967–1977. https://doi.org/10.1016/j.enpol.2006.06.003 doi: 10.1016/j.enpol.2006.06.003
![]() |
[14] | Flores M, Waddams PC (2013) Consumer behaviour in the British retail electricity market. Centre for Competition Policy Working Paper, University of East Anglia, Norwich (UK). https://ueaeco.github.io/working-papers/papers/ccp/CCP-13-10.pdf |
[15] |
Gamble A, Juliusson E, Gärling T (2009) Consumer attitudes towards switching supplier in three deregulated markets. J Socio Econ 38: 814–819. https://doi.org/10.1016/j.socec.2009.05.002. doi: 10.1016/j.socec.2009.05.002
![]() |
[16] |
Gärling T, Gamble A, Juliusson E (2008) Consumers' switching inertia in a fictitious electricity market. Int J Consum Stud 32: 613–618. https://doi.org/10.1111/j.1470-6431.2008.00728.x doi: 10.1111/j.1470-6431.2008.00728.x
![]() |
[17] | Ghazvini M, Ramos S, Soares J, et al. (2019) Liberalization and customer behavior in the Portuguese residential retail electricity market. Util Policy 59: 100919. https://doi.org/10.1016/j.jup.2019.05.005 |
[18] |
Giulietti M, Price C, Waterson M (2005) Consumer choice and competition policy: a study of UK energy markets. Econ J 115: 949–968. https://doi.org/10.1111/j.1468-0297.2005.01026.x doi: 10.1111/j.1468-0297.2005.01026.x
![]() |
[19] |
Hartmann P, Ibáñez VA (2007) Managing customer loyalty in liberalized residential energy markets: The impact of energy branding. Energy Policy 35: 2661–2672. https://doi.org/10.1016/j.enpol.2006.09.016 doi: 10.1016/j.enpol.2006.09.016
![]() |
[20] | Joskow PL (2011) Deregulation and regulatory reform. In Deregulation of Network Industries: What's Next? Sam Peltzman and Clifford Winston (Eds.). Washington D.C, USA, 113–188. |
[21] |
Juliusson EA, Gamble A, Gärling T (2007) Loss aversion and price volatility as determinants of attitude towards variable price agreements in the Swedish electricity market. Energy Policy 35: 5953–5957. https://doi.org/10.1016/j.enpol.2007.06.019 doi: 10.1016/j.enpol.2007.06.019
![]() |
[22] |
Kaenzig J, Heinzle SL, Wüstenhagen R (2013) Whatever the customer wants, the customer gets? Exploring the gap between consumer preferences and default electricity products in Germany. Energy Policy 53: 311–322. https://doi.org/10.1016/j.enpol.2012.10.061 doi: 10.1016/j.enpol.2012.10.061
![]() |
[23] | Littlechild SC (2000) Why do we need electricity retailers: A reply to Joskow on wholesale spot price pass-through. University of Cambridge, Cambridge (UK). |
[24] | Macedo DP, Marques AC, Damette O (2020) The impact of the integration of renewable energy sources in the electricity price formation: is the Merit-Order Effect occurring in Portugal? Util Policy 101080. https://doi.org/10.1016/j.jup.2020.101080 |
[25] | Morey MJ, Kirsch LD (2016) Retail choice in electricity: What have we learned in 20 years? Electric Markets Research Foundation report. |
[26] |
Pollitt M (2012) The role of policy in energy transitions: Lessons from the energy liberalisation era. Energy Policy 50: 128–137. https://doi.org/10.1016/j.enpol.2012.03.004 doi: 10.1016/j.enpol.2012.03.004
![]() |
[27] |
Roe B, Teisl M, Levy A, et al. (2001) US consumers' willingness to pay for green electricity. Energy policy 29: 917–925. https://doi.org/10.1016/S0301-4215(01)00006-4 doi: 10.1016/S0301-4215(01)00006-4
![]() |
[28] |
Shin KJ, Managi S (2017) Liberalization of a retail electricity market: Consumer satisfaction and household switching behavior in Japan. Energy Policy 110: 675–685. https://doi.org/10.1016/j.enpol.2017.07.048 doi: 10.1016/j.enpol.2017.07.048
![]() |
[29] | Sioshansi F (2006) Electricity Market Reform: What has the Experience taught us thus far? Util Policy 14: 63–75. https://doi.org/10.1016/j.jup.2005.12.002 |
[30] |
Six M, Wirl F, Wolf J (2017) Information as potential key determinant in switching electricity suppliers. J Bus Econ 87: 263–290. https://doi.org/10.1007/s11573-016-0821-9 doi: 10.1007/s11573-016-0821-9
![]() |
[31] |
Vihalemm T, Keller M (2016) Consumers, citizens or citizen-consumers? Domestic users in the process of Estonian electricity market liberalization. Energy Res Soc Sci 13: 38–48. https://doi.org/10.1016/j.erss.2015.12.004 doi: 10.1016/j.erss.2015.12.004
![]() |
[32] |
Waterson M (2003) The role of consumer in competition and competition policy. Ind J Ind Organ 21: 129–150. https://doi.org/10.1016/S0167-7187(02)00054-1 doi: 10.1016/S0167-7187(02)00054-1
![]() |
[33] |
Woo CK, Lloyd D, Tishler A (2003) Electricity Market Reform Failures: UK, Norway, Alberta and California. Energy Policy 31: 1103–1115. https://doi.org/10.1016/S0301-4215(02)00211-2 doi: 10.1016/S0301-4215(02)00211-2
![]() |
[34] |
Yang Y (2014) Understanding household switching behavior in the retail electricity market. Energy Policy 69: 406–414. https://doi.org/10.1016/j.enpol.2014.03.009 doi: 10.1016/j.enpol.2014.03.009
![]() |