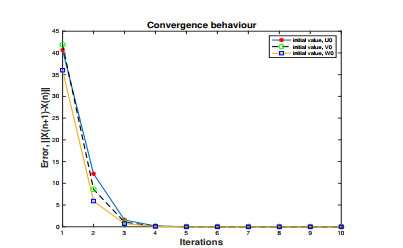
Many systems in real world can be represented as network, and network analysis can help us understand these systems. Node centrality is an important problem and has attracted a lot of attention in the field of network analysis. As the rapid development of information technology, the scale of network data is rapidly increasing. However, node centrality computation in large-scale networks is time consuming. Parallel computing is an alternative to speed up the computation of node centrality. GPU, which has been a core component of modern computer, can make a large number of core tasks work in parallel and has the ability of big data processing, and has been widely used to accelerate computing. Therefore, according to the parallel characteristic of GPU, we design the parallel algorithms to compute three widely used node centralities, i.e., closeness centrality, betweenness centrality and PageRank centrality. Firstly, we classify the three node centralities into two groups according to their definitions; secondly, we design the parallel algorithms by mapping the centrality computation of different nodes into different blocks or threads in GPU; thirdly, we analyze the correlations between different centralities in several networks, benefited from the designed parallel algorithms. Experimental results show that the parallel algorithms designed in this paper can speed up the computation of node centrality in large-scale networks, and the closeness centrality and the betweenness centrality are weakly correlated, although both of them are based on the shortest path.
Citation: Siyuan Yin, Yanmei Hu, Yuchun Ren. The parallel computing of node centrality based on GPU[J]. Mathematical Biosciences and Engineering, 2022, 19(3): 2700-2719. doi: 10.3934/mbe.2022123
[1] | Yajun Xie, Changfeng Ma, Qingqing Zheng . On the nonlinear matrix equation Xs+AHF(X)A=Q. AIMS Mathematics, 2023, 8(8): 18392-18407. doi: 10.3934/math.2023935 |
[2] | Muhammad Tariq, Eskandar Ameer, Amjad Ali, Hasanen A. Hammad, Fahd Jarad . Applying fixed point techniques for obtaining a positive definite solution to nonlinear matrix equations. AIMS Mathematics, 2023, 8(2): 3842-3859. doi: 10.3934/math.2023191 |
[3] | Xi-Ming Fang . General fixed-point method for solving the linear complementarity problem. AIMS Mathematics, 2021, 6(11): 11904-11920. doi: 10.3934/math.2021691 |
[4] | Reena Jain, Hemant Kumar Nashine, Jung Rye Lee, Choonkil Park . Unified relational-theoretic approach in metric-like spaces with an application. AIMS Mathematics, 2021, 6(8): 8959-8977. doi: 10.3934/math.2021520 |
[5] | Muhammad Tariq, Muhammad Arshad, Mujahid Abbas, Eskandar Ameer, Saber Mansour, Hassen Aydi . A relation theoretic m-metric fixed point algorithm and related applications. AIMS Mathematics, 2023, 8(8): 19504-19525. doi: 10.3934/math.2023995 |
[6] | Md Hasanuzzaman, Mohammad Imdad . Relation theoretic metrical fixed point results for Suzuki type ZR-contraction with an application. AIMS Mathematics, 2020, 5(3): 2071-2087. doi: 10.3934/math.2020137 |
[7] | Pattrawut Chansangiam, Arnon Ploymukda . Riccati equation and metric geometric means of positive semidefinite matrices involving semi-tensor products. AIMS Mathematics, 2023, 8(10): 23519-23533. doi: 10.3934/math.20231195 |
[8] | Mohammad Sajid, Lucas Wangwe, Hemanta Kalita, Santosh Kumar . Fixed point theorem on CATp(0) metric spaces with applications in solving matrix equations and fractional differential equations. AIMS Mathematics, 2025, 10(5): 11131-11158. doi: 10.3934/math.2025505 |
[9] | Ahmed Alamer, Faizan Ahmad Khan . Boyd-Wong type functional contractions under locally transitive binary relation with applications to boundary value problems. AIMS Mathematics, 2024, 9(3): 6266-6280. doi: 10.3934/math.2024305 |
[10] | Changzhou Li, Chao Yuan, Shiliang Chen . On positive definite solutions of the matrix equation X−∑mi=1A∗iX−piAi=Q. AIMS Mathematics, 2024, 9(9): 25532-25544. doi: 10.3934/math.20241247 |
Many systems in real world can be represented as network, and network analysis can help us understand these systems. Node centrality is an important problem and has attracted a lot of attention in the field of network analysis. As the rapid development of information technology, the scale of network data is rapidly increasing. However, node centrality computation in large-scale networks is time consuming. Parallel computing is an alternative to speed up the computation of node centrality. GPU, which has been a core component of modern computer, can make a large number of core tasks work in parallel and has the ability of big data processing, and has been widely used to accelerate computing. Therefore, according to the parallel characteristic of GPU, we design the parallel algorithms to compute three widely used node centralities, i.e., closeness centrality, betweenness centrality and PageRank centrality. Firstly, we classify the three node centralities into two groups according to their definitions; secondly, we design the parallel algorithms by mapping the centrality computation of different nodes into different blocks or threads in GPU; thirdly, we analyze the correlations between different centralities in several networks, benefited from the designed parallel algorithms. Experimental results show that the parallel algorithms designed in this paper can speed up the computation of node centrality in large-scale networks, and the closeness centrality and the betweenness centrality are weakly correlated, although both of them are based on the shortest path.
Nonlinear matrix equations (NME) were initially studied in the literature in relation to the algebraic Riccati problem. These equations appear in a wide range of problems in control theory, dynamical programming, ladder networks, stochastic filtering, queuing theory, statistics, and many other fields.
Let H(n) (resp. K(n), P(n)) denote the set of all n×n Hermitian (resp. positive semi-definite, positive definite) matrices over C and M(n) the set of all n×n matrices over C. In [20], Ran and Reurings discussed the existence of solutions of the following equation:
X+B∗F(X)B=Q | (1.1) |
in K(n), where B∈M(n), Q is positive definite and F is a mapping from K(n) into M(n). Note that X is a solution of (1.1) if and only if it is a fixed point of the mapping G(X)=Q−B∗F(X)B. In [21], they used the notion of partial ordering and established a modification of Banach Contraction Principle, which they applied for solving a class of NMEs of the form X=Q+∑mi=1B∗iF(X)Bi using the Ky Fan norm in M(n).
Theorem 1.1. [21] Let F:H(n)→H(n) be an order-preserving, continuous mapping which maps P(n) into itself and Q∈P(n). If Bi,B∗i∈P(n) and ∑mi=1BiB∗i<M⋅In for some M>0 (In – the unit matrix in M(n)) and if |tr(F(Y)−F(X))|≤1M|tr(Y−X)|, for all X,Y∈H(n) with X≤Y, then the equation X=Q+∑mi=1B∗iF(X)Bi has a unique positive definite solution (PDS).
On the other hand, many authors have obtained a large number of fixed point and common fixed point results over the course of the last few decades and have applied these results to obtain solutions of different kinds of equations arising in different situations in a wide range of mathematical problems.
Several mathematicians have recently established fixed point findings for contraction type mappings in partial order metric spaces. Turinici developed some early results in this technique in [23,24]; it should be emphasised, however, that their starting points were amorphous contributions in the field due to Matkowski [15,16]. These types of discoveries have been investigated by Ran and Reurings, and also Nieto and Rodriguez-López, who [17,18]. Turinici's findings were broadened and enhanced in subsequent papers [17,18]. Samet and Turinici refer to Bessem's new discovery of a fixed point theorem for nonlinear contraction when an arbitrary relation is symmetrically closed. Recently, Ahmadullah et al. [1,2], and Alam and Imdad [4] established a relation-theoretic equivalent of the Banach Contraction Principle using an amorphous relation, which incorporates a number of well-known relevant order-theoretic fixed point theorems. In the paper [25], Wardowski created the term F-contractions to describe a new type of contraction. He introduced the F family of functions F:R+→R that share certain properties. This concept has been used by many researchers in a variety of abstract situations.
One of the most visually appealing uses of contraction mapping is found in the field of nonlinear matrix equation to solve it. The question now is whether the aforementioned F-contraction can be enhanced and generalized. We explore a general class of contraction comprised of FG-contractions, extending certain fixed point findings from the conventional fixed point theory consisting of Banach contraction, F-contraction, Geraghty-type-contraction, to provide an affirmative response. Additionally, two novel rational-type contraction are deduced. Two examples are offered to illustrate the topic.
The following is the overview of the paper's structure. In Section 2, some notions related to relational metric spaces are discussed. In Section 3, we introduce a FG-contraction mapping on metric spaces equipped with an arbitrary binary relation (not necessarily partial order) and then show existence and uniqueness of fixed point findings under weaker conditions, and establish fixed point results. Section 4 provides two nontrivial instances to support the conclusion made here. In the final Section 5, we apply this conclusion to NMEs and examine their convergence behaviour with regard to three alternative initializations using graphical representations and solution by surface plot in MATLAB. Two randomly (real and complex) generated matrices of different orders are used to solve the nonlinear matrix equations.
We fix, the notations Z, N, R, R+ have their customary meanings, and N∗=N∪{0}.
A relational set is defined as (W,R) if (i) W≠∅ is a set and (ii) R is a binary relation on W.
In addition, if (W,d) is a metric space, we call (W,d,R) a relational metric space (RMS, for short).
The following are some commonly used terminology in relational set theory (see, for example, [4,12,13,14,22]).
Let (W,R) be a relational set, (W,d,R) be an RMS, and let ℑ be a self-mapping on W. Then:
1) u∈W is R-related to v∈W if and only if (u,v)∈R.
If for all u,v∈W, [u,v]∈R, where [u,v]∈R means either (u,v)∈R or (v,u)∈R, the set (W,R) is said to be comparable.
2)The symmetric closure of R, denoted by Rs, is defined to be the set R∪R−1, that is, Rs:=R∪R−1. Indeed, Rs is the smallest symmetric relation on W containing R.
3) A sequence (un) in W is said to be R-preserving if (un,un+1)∈R, ∀n∈N∪{0}.
4) (W,d,R) is said to be R-complete if every R-preserving Cauchy sequence converges in W.
5) R is said to be ℑ-closed if (u,v)∈R⇒(ℑu,ℑv)∈R. It is said to be weakly ℑ-closed if (u,v)∈R⇒[ℑu,ℑv]∈R.
6) R is said to be d-self-closed if there is a subsequence (unk) of (un) for every R-preserving sequence with un→u, such that [unk,u]∈R, for all k∈N∗.
7) If for each u,v∈M, there exists μ∈W such that (u,μ)∈R and (v,μ)∈R, then the subset M of W is termed R-directed. If for any u,v∈M, there exists μ∈W such that (u,ℑμ)∈R and (v,ℑμ)∈R, it is said to be (ℑ,R)-directed.
8)ℑ is said to be R-continuous at u if we get ℑ(un)→ℑ(u) as n→∞ for every R-preserving sequence (un) converging to u. Furthermore, ℑ is said to be R-continuous if it is R-continuous at all points of W.
9) For u,v∈W, a path of length k (where k is a natural number) in R from u to v is a finite sequence {w0, w1, w2, …, wk}⊂W satisfying the following conditions:
(i) z0=u and μk=v,
(ii) (wi, wi+1)∈R for each i (0≤i≤k−1),
then this finite sequence is called a path of length k joining u to v in R.
10) If for a pair of u,v∈W, there is a finite sequence {w0, w1, w2, …, wk}⊂W satisfying the following conditions:
(i) ℑw0=u and ℑwk=v,
(ii) (ℑwi, ℑwi+1)∈R for each i (0≤i≤k−1),
then this finite sequence is called a ℑ-path of length k joining u to v in R.
It is worth noting that a path of length k contains k+1 components of W, which are not necessarily distinct.
For a relational space (W,R), a self-mapping ℑ on W and an R-directed subset D of W, we use the following notation:
(i) Fix(ℑ):= the set of all fixed points of ℑ,
(ii) N(ℑ,R):={u∈W:(u,ℑu)∈R},
(iii) Λ(u,v,R):= the class of all paths in R from u to v in R, where u,v∈W.
Wardowski [25] introduced the family F of functions F:R+→R with the following properties:
(F1) F is strictly increasing;
(F2) for each sequence {ξn} of positive numbers,
limn→∞ξn=0 if and only if limn→∞F(ξn)=−∞. |
(F3) There exists k∈(0,1) such that limt→0+ξkF(ξ)=0.
Parvaneh et al. [19] used following set of slightly modified family of functions.
Definition 3.1. [19] The collection of all functions F:R+→R satisfying:
(F1) F is continuous and strictly increasing;
(F2) for each {ξn}⊆R+, limn→∞ξn=0 iff limn→∞F(ξn)=−∞,
will be denoted by F.
The collection of all pairs of mappings (G,β), where G:R+→R, β:R+→[0,1), satisfying:
(F3) for each {ξn}⊆R+, lim supn→∞G(ξn)≥0 iff lim supn→∞ξn≥1;
(F4) for each {ξn}⊆R+, lim supn→∞β(ξn)=1 implies limn→∞ξn=0;
(F5) for each {ξn}⊆R+, ∑∞n=1G(β(ξn))=−∞,
will be denoted by Gβ.
Definition 3.2. Let (W,d,R) be an RMS and ℑ:W→W be a given mapping. A mapping ℑ is said to be a FG-contractive mapping, if there exist F∈F and (G,β)∈Gβ, such that for (u,v)∈W2 with (u,v)∈R∗,
F(d(ℑu,ℑv))≤F(Δ(u,v))+G(β(Δ(u,v))), | (3.1) |
where
Δ(u,v)=max{d(u,v),d(u,ℑu),d(v,ℑv),d(u,ℑv)+d(v,ℑu)2}, | (3.2) |
R∗={(u,v)∈R∣ℑu≠ℑv}. |
We denote by (FG)R the collection of all FG-contractive mappings on (W,d,R).
We present and verify our conclusions on (FG)R-contractive mappings described in Sub-Section 3.1. The following is the first main outcome.
Theorem 3.3. Let (W,d,R) be an RMS and ℑ:W→W. Suppose that the following conditions hold:
(C1) N(ℑ,R)≠∅;
(C2) R is ℑ-closed and ℑ-transitive;
(C3) W is ℑ-R-complete;
(C4) ℑ∈(FG)R;
(C5) ℑ is R-continuous or
(C′5) R is d-self-closed.
Then there exists a point u∗∈Fix(ℑ).
Proof. Starting with u0∈W given by (C1), we construct a sequence {un} of Picard iterates un+1=ℑn(u0) for all n∈N∗.
Using (C1) and (C2), we have that (ℑu0,ℑ2u0)∈R. Continuing this process inductively, we obtain
(ℑnu0,ℑn+1u0)∈R | (3.3) |
for any n∈N∗. Hence, {un} is an R-preserving sequence.
Now, if there exists some n0∈N∗ such that d(un0, ℑun0)=0, then the result follows immediately. Otherwise, for all n∈N∗, d(un, ℑun)>0 so that ℑun≠ℑun+1 which implies that (un, un+1)∈R∗. Therefore, using (C4) for u=un, v=un+1, we have
F(d(ℑun,ℑun+1))≤F(Δ(un,un+1))+G(β(un,un+1))), |
where
Δ(un,un+1))=max{d(un,un+1),d(un,ℑun),d(un+1,ℑun+1),d(un,ℑun+1)+d(un+1,ℑun)2}=max{d(un,un+1),d(un,un+1),d(un+1,un+2),d(un,un+2)2}≤max{d(un,un+1),d(un,un+1),d(un+1,un+2),d(un,un+1)+d(un+1,un+2)2}=max{d(un,un+1),d(un+1,un+2)}. |
If Δ(un,un+1))=d(un+1,un+2), then
F(d(un+1,un+2))≤F(d(un+1,un+2))+G(β(d(un+1,un+2))) |
which implies G(β(d(un+1,un+2)))≥0 i.e. β(d(un+1,un+2))≥1, a contradiction. Therefore
d(un+1,un+2)≤d(un,un+1) for all n∈N | (3.4) |
and so
F(d(un+1,un+2))≤F(d(un,un+1))+G(β(d(un,un+1))) |
for all n∈N. Consequently
F(d(un,un+1))≤F(d(un−1,un))+G(β(d(un−1,un)))≤…≤F(d(u0,u1))+i=n∑i=1G(β(d(ui,ui−1))). | (3.5) |
Letting n→∞ gives limn→∞F(d(un,un+1))=−∞ and F∈F gives
limn→∞d(un,un+1)=0. | (3.6) |
We will now show that the sequence {un} is a R preserving Cauchy sequence in (W,d). On the contrary, we suppose that there exist ζ>0 and two subsequences {un(j)} and {um(j)} of {un} such that m(j) is the smallest index for which m(j)>n(j)>j and
d(um(j),un(j))≥ζ. | (3.7) |
This means that m(j)>n(j)>j and
d(um(j)−1,un(j))<ζ. | (3.8) |
On the other hand
ζ≤d(um(j),un(j))≤d(um(j),um(j)−1)+d(um(j)−1,unj))≤d(um(j),um(j)−1)+ζ. |
Taking j→∞ and using (3.6), we get
limj→∞d(um(j),un(j))=ζ, | (3.9) |
and hence
limj→∞d(um(j)+1,un(j)+1)=ζ. | (3.10) |
As the sequence {un} is R-preserving and R is ℑ-transitive, therefore (um(j),un(j))∈R∗ and we get
F(lim supj→∞d(um(j)+1,un(j)+1))≤F(lim supj→∞Δ(um(j),un(j)))+lim supȷ→∞G(β(Δ(um(j),un(j)))) | (3.11) |
where
Δ(um(j),un(j))=max{d(um(j),un(j)),d(um(j),ℑum(j)),d(un(j),ℑun(j)),d(un(j),ℑum(j))+d(um(j),ℑun(j))2}=max{d(um(j),un(j)),d(um(j),um(j)+1),d(un(j),un(j)+1),d(un(j),um(j)+1)+d(um(j),un(j)+1)2}≤max{d(um(j),un(j)),d(um(j),um(j)+1),d(un(j),un(j)+1),d(un(j),um(j))+d(um(j),um(j)+1)+d(um(j),un(j))+d(un(j),un(j)+1)2}. |
Taking upper limit as j→∞ and making use of (3.6), (3.9) and (3.10), we get
lim supj→∞Δ(um(j),un(j))=lim supj→∞d(um(j),un(j)). | (3.12) |
Therefore, from (3.11), (3.10) and (3.12), we have
F(ζ)=F(lim supj→∞d(um(j)+1,un(j)+1))≤F(lim supj→∞d(um(j),un(j)))+lim supȷ→∞G(β(d(um(j),un(j))))=F(ζ)+lim supȷ→∞G(β(d(um(j),un(j))), |
which implies that lim supȷ→∞G(β(d(um(j),un(j))))≥0, which gives \\ lim supj→∞β(d(um(j),un(j)))≥1, and taking in account that β(ξ)<1 for all ξ≥0, we have lim supȷ→∞β(d(um(j),un(j)))=1. Therefore, lim supȷ→∞d(um(j),un(j))=0, a contradiction. Hence, {un} is R preserving Cauchy sequence in W.
The R-completeness of W implies that there exists u∗∈W such that limn→∞un=u∗. Now first by (C5), we have
u∗=limn→∞un+1=limn→∞ℑun=ℑu∗ | (3.13) |
and hence u∗ is a fixed point of ℑ.
Alternatively, suppose that R is d-self-closed. Then, there exists a subsequence {unk} of {un} with [unk, u∗]∈R for all k∈N∗.
Now, we distinguish two cases for Γ={k∈N:ℑunk=ℑu∗}. If Γ is finite, then there exists k0∈N such that ℑunk≠ℑu∗ for all k>k0. It follows from (3.1), (for all k>k0) that
F(d(ℑunk,ℑu∗))≤F(Δ(unk,u∗))+G(β(Δ(unk,u∗))) |
where
Δ(unk,u∗)=max{d(unk,u∗),d(unk,ℑunk),d(u∗,ℑu∗),d(unk,ℑu∗)+d(u∗,ℑunk)2}. |
Applying limit as n→∞, we get limn→∞Δ(unk,u∗)=d(u∗,ℑu∗) which implies that lim supn→∞G(β(Δ(unk,u∗))≥0, which gives lim supn→∞β(Δ(unk,u∗))≥1, and taking in account that β(ξ)<1 for all ξ≥0, we have lim supn→∞β(Δ(unk,u∗))=1. Therefore, lim supn→∞Δ(unk,u∗)=0. Hence, d(u∗,ℑu∗)=0, we get u∗=ℑu∗.
Otherwise, if Γ is not finite, a subsequence exists. {un(k(ς))} of {unk} such that
un(k(ς))+1=ℑun(k(ς))=ℑu∗,∀ς∈N. |
As unk→du∗, therefore ℑu∗=u∗.
Theorem 3.4. In addition to the assumptions of Theorem 3.3, let Λ(u,v;R|ℑ(W))≠∅ for all u,v∈ℑ(W). Then ℑ has a unique fixed point.
Proof. In view of Theorem 3.3, Fix(ℑ)≠∅. If Fix(ℑ) is a singleton, then we concluded the proof. Otherwise, let u∗≠ϖ∈Fix(ℑ). Then ℑu∗=u∗≠ϖ=ℑϖ. Since Λ(u,v;R|ℑ(W))≠∅ for all v, u∈ℑ(W), there exists a path {ℑz0, ℑz1, ⋯, ℑzk} of some length k in R|ℑ(W) such that ℑz0=u∗, ℑzk=ϖ and (ℑzj, ℑzj+1)∈R|ℑ(W) for each j=0,1,2, ⋯, k−1. Since R is ℑ-transitive, we have
(u∗, ℑz1)∈R, (ℑz1, ℑz2)∈R, ⋯, (ℑzk−1, ϖ)∈R⇒(u∗, ϖ)∈R. |
Therefore from (u∗, ϖ)∈R and ℑu∗≠ℑϖ, we have (u∗, ϖ)∈R∗. Using (3.1) for (u∗, ϖ)∈R∗, we have
F(d(ℑu∗,ℑϖ))≤F(Δ(ϖ,u∗)+G(β(Δ(ϖ,u∗))), | (3.14) |
where
Δ(ϖ,u∗)=max{d(u∗,ϖ),d(u∗,ℑu∗),d(ϖ,ℑϖ),d(u∗,ℑϖ)+d(ϖ,ℑu∗)2}=d(u∗,ϖ) |
which on substituting in (3.14) gives
F(d(u∗,ϖ))≤F(d(u∗,ϖ))+G(β(d(u∗,ϖ))), |
which gives G(β(d(u∗,ϖ))≥0 implies β(d(u∗,ϖ)≥1, a contradiction. Thus, d(u∗,ϖ)=0.
Theorem 3.5. In addition to the hypotheses of Theorem 3.3, if any of the following conditions is fulfilled:
(I) for all u,v∈W, there exists a z∈W such that
{(z,ℑz),(z,u),(z,v)}⊆R; | (3.15) |
(II) the set ℑ(W) is R-directed;
(III) R|ℑ(E) is complete;
(IV) Λ(u,v,Fix(ℑ),Rs) is nonempty, for each u,v∈Fix(ℑ),
then ℑ has a unique fixed point.
Proof. In view of Theorem 3.3, Fix(ℑ)≠∅.
● Assume (I). Suppose u,v∈W are the two distinct fixed points of ℑ, that is, ℑu=u≠v=ℑv. We will consider the following two cases.
Case (A): We have (u,v)∈R, then ℑnu=u and ℑnv=v such that (ℑnu,ℑnv)∈R∗ for n=0,1,…. Therefore, using condition (3.1),
F(d(ℑn+1u,ℑn+1v)≤F(Δ(ℑnu,ℑnv))+G(β(Δ(ℑnu,ℑnv))) |
where
Δ(ℑnu,ℑnv))=max{d(ℑnu,ℑnv),d(ℑnu,ℑn+1u),d(ℑnv,ℑn+1v),d(ℑnu,ℑn+1v)+d(ℑnv,ℑn+1u)2}. |
Since u and v are fixed points of ℑ, we have
Δ(ℑnu,ℑnv)=d(u,v) |
and we get
F(d(u,v)≤F(d(u,v)+G(β(d(u,v)) |
which gives G(β(d(u,v))≥0 and so β(d(u,v)≥1, a contradiction. Thus, the fixed point is unique. Case (B): By the assumption (I), there exists a distinct element z in W such that ℑu=u≠z≠v=ℑv, satisfying condition (3.15), otherwise proof follows from Case (A). Next due to ℑ-closedness of R, we get
(ℑn−1z,u)∈R,(ℑn−1z,v)∈R. |
Also distinctness of z from u and v, we have
(ℑn−1z,ℑn−1u)=(ℑn−1z,u)∈R∗,(ℑn−1z,ℑn−1v)=(ℑn−1z,v)∈R∗. |
Therefore using condition (3.1) for (ℑn−1z,u)∈R∗, we have
F(d(ℑnz,u))≤F(Δ(ℑn−1z,u))+G(β(Δ(ℑn−1z,u))), | (3.16) |
where
Δ(ℑn−1z,u)=max{d(ℑn−1z,u),d(ℑn−1z,ℑnz),d(u,ℑu),d(ℑn−1z,ℑu)+d(u,ℑnz)2}≤max{d(ℑn−1z,u),d(ℑn−1z,ℑnz),d(u,ℑu),2d(ℑn−1z,u)+d(ℑn−1z,ℑnz)2}≤max{d(ℑn−1z,u),d(ℑn−1z,ℑnz),d(u,ℑu)}. |
Using (z,ℑz)∈R, similarly as in the proof of Theorem 3.3, it can be shown that d(ℑn−1z,ℑnz)→0 as n→∞. Therefore, for n sufficiently large,
max{d(ℑn−1z,u),d(ℑn−1z,ℑnz),d(u,ℑu)}=d(ℑn−1z,u) |
and, from (3.16), we have
F(d(ℑnz,u))≤F(d(ℑn−1z,u))+G(β(d(ℑn−1z,u))). |
As in the proof of Theorem 3.3, it can be shown that d(ℑnz,u)≤d(ℑn−1z,u). It follows that the sequence {d(ℑnz,u)} is nonincreasing. As earlier, we have
limn→∞d(ℑnz,u)=0. |
Also, since (z,v)∈R, proceeding as earlier, we can prove that
limn→∞d(ℑnz,v)=0, |
and by using limit uniqueness, we infer that u=v; i.e., the fixed point of ℑ is unique.
● Assume (II). For any two fixed points u,v of ℑ, there must be an element z∈ℑ(W), such that
(z,u)∈R and (z,v)∈R. |
As R is ℑ-closed, so for all n∈N∪{0},
(ℑnz,u)∈R and (ℑnz,v)∈R. |
In the line of proof of Case(B) (I), we obtain u=v, i.e., ℑ has a unique fixed point.
● Assume (III). Suppose u,v are two fixed points of ℑ. Then, we must have (u,v)∈R and since u≠ℑv, we have (v,u)∈R∗. Therefore, using condition (3.1),
F(d(ℑu,ℑv)≤F(Δ(u,v)+G(β(Δ(u,v))) |
where
Δ(u,v)=max{d(u,v),d(u,ℑu),d(v,ℑv),d(u,ℑv)+d(v,ℑu)2}=d(u,v) |
which gives G(β(d(u,v))≥0 and so β(d(u,v)≥1, a contradiction. Thus, the fixed point is unique. In a similar way, if (v,u)∈R, we have u=v.
● Assume (IV). Suppose u,v are two fixed points of ℑ. Let {z0,z1,…,zk} be an Rs-path in Fix(ℑ) connecting u and v. As in Case (I, A), it must be zi−1=zi for each i=1,2,…,k, and it follows that u=v.
If we take R={(u, u)∈W×W∣u⪯u}, then we have more new results as consequences of Theorem 3.3.
Corollary 3.6. Let (W,d,⪯) be an ordered complete metric space. Let ℑ:W→W be increasing and (FG)R on W⪯. Suppose there exists u0∈W such that u0⪯ℑu0. If ℑ is W⪯-continuous or W⪯ is d-self-closed, then u∗∈Fix(ℑ). Moreover, for each u0∈W with u0⪯ℑu0, the Picard sequence ℑn(u0) for all n∈N converges to a u∗∈Fix(ℑ).
Considering a range of concrete functions F∈F and (G,β)∈Gβ in the condition (1.1) of Theorems 3.3–3.5 and Corollary 3.6, we can get various classes of (FG)R-contractive conditions in an RMS. We state just a few examples (recall that Δ(u,v) is defined in (3.2)).
Corollary 3.7. Let all the hypothesis of Theorem 3.3 hold except (C4) is replaced by
(C4 ') ℑ satisfy Wardowski-type [25] condition, that is, for (u,v)∈W with (u,v)∈R∗, τ>0
τ+F(d(ℑu,ℑv))≤F(Δ(u,v)). |
Then there exists a point u∗∈Fix(ℑ).
Proof. If we take β(t)=e−τ, and G(t)=lnt (t>0) in the Eq (3.1), then the result follows from Theorem 3.3.
Corollary 3.8. Let all the hypothesis of Theorem 3.3 hold except (C4) is replaced by
(C4 ') ℑ satisfy Geraghty-type [9,10] condition, that is, for (u,v)∈W with (u,v)∈R∗,
d(ℑu,ℑv)≤β(Δ(u,v))Δ(u,v))). |
Then there exists a point u∗∈Fix(ℑ).
Proof. If we take F(t)=G(t)=lnt (t>0) in the Eq (3.1), then the result follows from Theorem 3.3.
Corollary 3.9. Let all the hypothesis of Theorem 3.3 hold except (C4) is replaced by
(C_4′) ℑ satisfy mixed type-I condition, that is, for (u,v)∈W with (u,v)∈R∗,
d(ℑu,ℑv)≤Δ(u,v)[1−√Δ(u,v)ln(β(Δ(u,v)))]2. |
Then there exists a point u∗∈Fix(ℑ).
Proof. If we take F(t)=−1√t, G(t)=lnt (t>0) in Eq (3.1), then the result follows from Theorem 3.3.
Corollary 3.10. Let all the hypothesis of Theorem 3.3 hold except (C4) is replaced by
(C4 ') ℑ satisfy mixed type-II condition, that is, for (u,v)∈W with (u,v)∈R∗, τ>0
d(ℑu,ℑv)≤Δ(u,v)[1+τ√Δ(u,v)]2. |
Then there exists a point u∗∈Fix(ℑ).
Proof. If we take β(t)=e−τ, G(t)=lnt and F(t)=−1/√t (t>0) in the Eq (3.1), then the result follows from Theorem 3.3.
Remark 3.11. If we take R={(u, u)∈W×W∣u⪯u} in the Corollarys 3.7–3.10, then it belong to [4,9,21].
Remark 3.12. If we replace (C3) by relatively weaker notions, namely, R-precompleteness [3] of ℑ(W), our results will be true.
Example 4.1 Let W =[0,9) be equipped with usual metric d. Consider the binary relation on W as follows:
R={(0,2), (3,2), (3,3), (3,6), (4,2), (4,3), (4,4), (4,6), (6,2), (6,3), (6,6)}. |
Define a mapping ℑ:W→W by
ℑu={2, 0≤u<1;4, u=1;6, 1<u<8. |
Then ℑ is not continuous while ℑ is R-continuous, R is ℑ-closed, and ℑ-transitive; W is ℑ-R-complete. Also R∗={(0,2), (6,2)} and N(ℑ;R)≠∅ as (6, ℑ6)=(6,6)∈R.
Now we take F(ξ)=−1√ξ, G(ξ)=lnξ (ξ>0) and β(ξ)=λ∈(0,1), τ=−lnλ>0, then (3.1) converted to
d(ℑu,ℑv)≤Δ(u,v)(1+τ√Δ(u,v))2 | (4.1) |
where Δ(u,v) given in (3.2).
Consider (u,v)=(6,2)∈R∗. Then d(ℑu,ℑv)=2 and Δ(u,v)=4. Therefore, the condition (4.1) reduces to 2≤4(1+τ√4)2, which is true for τ=0.1. Thus, all the conditions of Theorem 3.3 are satisfied, hence ℑ has a fixed point. Moreover, R|ℑ(W?) is transitive while R is not and for all u, v∈ℑ(W), we have (u, v)∈R, so Λ(u,v,R)|ℑ(W)) is nonempty for all u, v∈ℑ(W). Following Theorem 3.4, ℑ has a unique fixed point which is u∗=5.
Now for (0,2)∈R,
d(ℑu,ℑv)=2≰2k=k max{d(u,v),d(u,ℑu),d(v,ℑv),12[d(u,ℑv)+d(v,ℑu)]} |
which is not true for any k∈(0,1), and hence ℑ is not contraction mapping (Ćirić type contraction) on (W,d,R). Hence Ćirić et al. [8] cannot be applied to the present example.
Also, as 2,0∈W, (2,0)∉R with ℑ2=4≠2=ℑ0 such that d(ℑ2, ℑ0)≰d(2,0)(1+τ√d(2,0))2 and d(ℑu,ℑv)≰Δ(u,v)(1+τ√Δ(u,v))2. Also
d(ℑu,ℑv)=2≰2k=k max{d(u,v),d(u,ℑu),d(v,ℑv),12[d(u,ℑv)+d(v,ℑu)]}, |
which shows that ℑ is neither contraction nor generalized contraction for any k∈[0,1). Hence results of Wardowski [25] and Ćirić [7] cannot be applied to the present example, while our Theorems 3.3 and 3.4 are applicable. This shows that our results are genuine improvements over the corresponding results contained in Wardowski [25], Ćirić [7] and Ćirić et al. [8].
Example 4.2. Consider the set W=[15,1] with the usual metric d. Define a binary relation R by
R={(15,15),(15,1)(14,1),(14,15),(15,14),(14,14)}. |
Consider the ℑ self-mapping on W defined by
ℑ(v)={15,15≤v≤1414,34<v≤1. |
It is obvious that W is ℑ−R is ℑ-closed and R is ℑ-complete. Also R∗={(15,1), (14,1)} and N(ℑ;R)≠∅ as (15, ℑ15)=(15,15)∈R.
We consider (4.1) of previous Example 4.1 to verify ℑ∈(FG)R.
● Let (v,u)=(15,1). Then d(ℑv,ℑu)=120 and Δ(u,v)=45. Therefore, the condition (4.1) reduces to 120≤4/5(1+2τ√1/5)2.
● Let (v,u)=(14,1). Then d(ℑv,ℑu)=120 and Δ(u,v)=4/5. Therefore, the condition (4.1) reduces to 120≤4/5(1+2τ√1/5)2.
It is reasonable to verify that the aforementioned instances hold true for τ>0 (especially τ=0.1). Thus, ℑ∈(FG)R.
Let (vn) be a sequence that preserves R and converges to v as n→∞. Then we'll need
(vn,vn+1)∈{(14,15),(14,15),(15,14),(14,14)} |
implies that
vn∈{15,14}. |
This means that either vn→0 or vn→15 is n→∞, and we have [vn,v]∈R for all n∈N, where v=15 and 14. This shows that R is d-self-closed. Thus, all the conditions of Theorem 3.3 are satisfied, hence ℑ has a fixed point (u∗=1/5).
Remark 4.3. ● Take note that the binary relation R used in Examples 4.1 and 4.2 is not one of the more well-known conventional binary relations such as reflexive, irreflexive, symmetric, antisymmetric, complete, or weakly complete.
● It is worth noting that the comparable theorems in [6,17,18,20,21,22,23,24] cannot be used in the context of the preceding examples (i.e., Example 4.1 and 4.2), which illustrate the superiority of Theorem 3.3 over many other conclusions. As a result, all of the traditional discoveries have been extended to an arbitrary binary connection.
For a matrix B∈H(n), we will denote by s(B) any of its singular values and by s+(B) the sum of all of its singular values, that is, the trace norm ‖. For \mathcal{C}, \mathcal{D}\in \mathcal{H}(n) , \mathcal{C}\succeq \mathcal{D} (resp. \mathcal{C}\succ \mathcal{D} ) will mean that the matrix \mathcal{C}-\mathcal{D} is positive semi-definite (resp. positive definite).
In [21], Ran and Raurings derived the Lemma 3.1 to get positive solution of linear and nonlinear matrix equations. We state in the following which is needed in the subsequent discussion.
Lemma 5.1. [21, Lemma 3.1] If \mathcal{A} \succeq {O} and \mathcal{B}\succeq {O} are n\times n matrices, then
0\leq tr(\mathcal{AB})\leq\Vert \mathcal{A}\Vert tr(\mathcal{B}). |
Lemma 5.2. [5] If \mathcal{A}\in \mathcal{H}(n) such that \mathcal{A} \prec I_{n} , then \Vert \mathcal{A}\Vert < 1 .
Here we present an example that satisfying the above lemmas.
Example 5.3. Consider the matrices
\mathcal{A} = \begin{bmatrix} 0.1444 & 0.1089& 0.0766\\ 0.1089 & 0.2697& 0.2064\\ 0.0766& 0.2064& 0.2039 \end{bmatrix}, \mathcal{B} = \begin{bmatrix} 0.1864 & 0.1352& 0.0923\\ 0.1352 & 0.1292 & 0.0585\\ 0.0923 & 0.0585& 0.1563 \end{bmatrix} |
Both the matrices are positive definite having the minimum eigenvalues as 0.0259 and 0.0180 respectively. Also, satisfying Lemma 5.1, since
0\leq tr(\mathcal{AB}) = 0.1614\leq\Vert \mathcal{A}\Vert tr(\mathcal{B}) = 0.2917. |
In addition, here \mathcal{A} \prec I_{n} , and \Vert \mathcal{A}\Vert = 0.6181 < 1 which validate the Lemma 5.2.
In the following, we demonstrate that the solution to the nonlinear matrix problem exists and is unique
\begin{equation} \mathcal{X} = \mathcal{Q} + \sum\limits_{i = 1}^{m} \mathcal{B}_{i}^{*}\mathcal{G}(\mathcal{X})\mathcal{B}_{i}, \end{equation} | (5.1) |
where \mathcal{Q} is a Hermitian positive definite matrix, \mathcal{B}_{i}^{*} stands for the conjugate transpose of an n\times n matrix \mathcal{B}_{i} and \mathcal{G} an order-preserving continuous mapping from the set of all Hermitian matrices to the set of all positive definite matrices such that \mathcal{G}(O) = O .
Theorem 5.4. Consider NME (5.1). Assume that there exists a positive real number \eta { such that}
(H_{1}) there exists \mathcal{Q} \in \mathcal{P}(n) such that \sum_{i = 1}^{m} \mathcal{B}_{i}^{*} \mathcal{G} (\mathcal{Q})\mathcal{B}_{i}\succ 0 ;
(H_{2}) \sum_{i = 1}^{m}\mathcal{B}_{i}\mathcal{B}_{i}^{*}\prec\eta I_{n} .
(H_{3}) for every \mathcal{X}, \ \mathcal{Y}\in \mathcal{P}(n) such that \mathcal{X}\preceq \mathcal{Y} with,
\sum\limits_{i = 1}^{m} \mathcal{B}_{i}^{*}\mathcal{G}(\mathcal{X})\mathcal{B}_{i} \neq\sum\limits_{i = 1}^{m} \mathcal{B}_{i}^{*}\mathcal{G}(\mathcal{Y})\mathcal{B}_{i} |
for \tau > 0 , we have
\begin{eqnarray*} && |s^+(\mathcal{G}(\mathcal{X})-\mathcal{G}(\mathcal{Y}))| \\ &&\leq \frac{1}{\eta} \times \max \left\{\begin{array}{cc} \frac{|s^+(\mathcal{X}-\mathcal{Y})|}{[1+ \tau |s^+(\mathcal{X}-\mathcal{Y})|^{1/2}]^2}, \frac{ |s^+\left(\mathcal{X}-\mathcal{Q} - \sum_{i = 1}^{m}\mathcal{B}_{i}^{*}\mathcal{G}(\mathcal{X})\mathcal{B}_{i}\right)|}{[1+\tau |s^+\left(\mathcal{X}-\mathcal{Q} - \sum_{i = 1}^{m}\mathcal{B}_{i}^{*}\mathcal{G}(\mathcal{X})\mathcal{B}_{i}\right)|^{1/2}]^2}, \\ \frac{|s^+\left(\mathcal{Y}-\mathcal{Q} - \sum_{i = 1}^{m}\mathcal{B}_{i}^{*}\mathcal{G}(\mathcal{Y})\mathcal{B}_{i} \right)|}{[1+\tau |s^+\left(\mathcal{Y}-\mathcal{Q} - \sum_{i = 1}^{m}\mathcal{B}_{i}^{*}\mathcal{G}(\mathcal{Y})\mathcal{B}_{i} \right)|^{1/2}]^2}, \\ \frac{1}{2}\left[\begin{array}{cc}\frac{ |s^+\left(\mathcal{X} - \mathcal{Q} - \sum_{i = 1}^{m}\mathcal{B}_{i}^{*}\mathcal{G}(\mathcal{Y})\mathcal{B}_{i}\right)|}{[1+\tau |s^+\left(\mathcal{X} - \mathcal{Q} - \sum_{i = 1}^{m}\mathcal{B}_{i}^{*}\mathcal{G}(\mathcal{Y})\mathcal{B}_{i}\right)|^{1/2}]^2}\\ + \frac{ |s^+ \left(\mathcal{Y}- \mathcal{Q} - \sum_{i = 1}^{m}\mathcal{B}_{i}^{*}\mathcal{G}(\mathcal{X})\mathcal{B}_{i}\right)|}{[1+\tau |s^+ \left(\mathcal{Y}- \mathcal{Q} - \sum_{i = 1}^{m}\mathcal{B}_{i}^{*}\mathcal{G}(\mathcal{X})\mathcal{B}_{i}\right)|^{1/2}]^2} \end{array}\right] \end{array} \right\}. \end{eqnarray*} |
Then the NME (5.1) has a unique solution. Moreover, the iteration
\begin{equation} \mathcal{X}_{n} = \mathcal{Q}+\sum\limits_{i = 1}^{m} \mathcal{B}_{i}^{*} \mathcal{G}(\mathcal{X}_{n-1}) \mathcal{B}_{i} \end{equation} | (5.2) |
where \mathcal{X}_{0}\in \mathcal{P}(n) satisfies
\mathcal{X}_{0}\preceq \mathcal{Q}+\sum\limits_{i = 1}^{m} \mathcal{B}_{i}^{*} \mathcal{G}(\mathcal{X}_{0}) \mathcal{B}_{i}, |
converges in the sense of trace norm \Vert.\Vert_{tr} to the solution of the matrix equation (5.1).
Proof. Define a mapping \Im :\mathcal{P}(n)\rightarrow \mathcal{P}(n) by
\begin{equation*} \Im (\mathcal{X}) = \mathcal{Q} + \sum\limits_{i = 1}^{m} \mathcal{B}_{i}^{*}\mathcal{G}(\mathcal{X})\mathcal{B}_{i}, ~~\text{ for all }~~ \mathcal{X}\in \mathcal{P}(n), \end{equation*} |
and a binary relation
\mathcal{R} = \{(\mathcal{X}, \ \mathcal{Y})\in \mathcal{P}(n)\times \mathcal{P}(n): \mathcal{X} \preceq \mathcal{Y}\}. |
Then a solution of the matrix Eq (5.1) is a fixed point of the mapping \Im . It's worth noting that \Im is well defined, \mathcal{R} is \Im -closed, and \mathcal{R} is \mathcal{R} -continuous.
\sum\limits_{i = 1}^{m} \mathcal{B}_{i}^{*} \mathcal{G}(\mathcal{Q})\mathcal{B}_{i}\succ 0, |
for some \mathcal{Q} \in \mathcal{P}(n) , we have (\mathcal{Q}, \Im (\mathcal{K}))\in \mathcal{R} and hence \mathcal{P}(n)(\Im; \mathcal{R})\neq\emptyset .
Now, let (\mathcal{X}, \ \mathcal{Y})\in \mathcal{R}^{*} = \{(\mathcal{X}, \ \mathcal{Y})\in \mathcal{R}:\Im (\mathcal{X})\neq \Im (\mathcal{Y})\} . Then
\begin{align} &\Vert \Im (\mathcal{X})-\Im (\mathcal{Y})\Vert_{tr}\\ & = s^+(\Im (\mathcal{X})-\Im (\mathcal{Y}))\\ & = s^+(\sum\limits_{i = 1}^{m} \mathcal{B}_{i}^{*}(\mathcal{G}(\mathcal{X})- \mathcal{G}(\mathcal{Y}))\mathcal{B}_{i})\\ & = \sum\limits_{i = 1}^{m} s^+(\mathcal{B}_{i}^{*}(\mathcal{G}(\mathcal{X})- \mathcal{G}(\mathcal{Y}))\mathcal{B}_{i})\\ & = \sum\limits_{i = 1}^{m} s^+(\mathcal{B}_{i}\mathcal{B}_{i}^{*}(\mathcal{G}(\mathcal{X})-\mathcal{G}(\mathcal{Y})))\\ & = s^+(\sum\limits_{i = 1}^{m}\mathcal{B}_{i}\mathcal{B}_{i}^{*}) s^+(\mathcal{G}(\mathcal{X})-\mathcal{G}(\mathcal{Y}))\\ &\leq \frac{\Vert \sum\limits_{i = 1}^{m}\mathcal{B}_{i}\mathcal{B}_{i}^{*}\Vert}{\eta } \times \max \left\{\begin{array}{cc} \frac{\|\mathcal{X}-\mathcal{Y}\|_{tr}}{[1+\tau \|\mathcal{X}-\mathcal{Y}\|_{tr}^{1/2}]^2}, \frac{\|\mathcal{X}-\Im \mathcal{X}\|_{tr}}{[1+\tau \|\mathcal{X}-\Im \mathcal{X}\|_{tr}^{1/2}]^2}, \frac{\|\mathcal{Y}-\Im \mathcal{Y}\|_{tr}}{[1+\tau \|\mathcal{Y}-\Im \mathcal{Y}\|_{tr}^{1/2}]^2}, \\ \frac{1}{2}\left[\frac{\|\mathcal{X}-\Im \mathcal{Y}\|_{tr}}{[1 + \tau \|\mathcal{X}-\Im \mathcal{Y}\|_{tr}^{1/2}]^2}+ \frac{\|\mathcal{Y}-\Im \mathcal{X}\|_{tr}}{[1 + \tau \|\mathcal{Y}-\Im \mathcal{X}\|_{tr}^{1/2}]^2} \right] \end{array} \right\}\\ &\leq \frac{\Theta(\mathcal{U, V})}{[1+\tau (\Theta(\mathcal{U, V}))^{1/2}]^2} \end{align} | (5.3) |
where
\begin{align} \Theta(\mathcal{U, V}) = \max \left\{\begin{array}{cc} \frac{\|\mathcal{X}-\mathcal{Y}\|_{tr}}{[1+\tau \|\mathcal{X}-\mathcal{Y}\|_{tr}^{1/2}]^2}, \frac{\|\mathcal{X}-\Im \mathcal{X}\|_{tr}}{[1+\tau \|\mathcal{X}-\Im \mathcal{X}\|_{tr}^{1/2}]^2}, \frac{\|\mathcal{Y}-\Im \mathcal{Y}\|_{tr}}{[1+\tau \|\mathcal{Y}-\Im \mathcal{Y}\|_{tr}^{1/2}]^2}, \\ \frac{1}{2}\left[\frac{\|\mathcal{X}-\Im \mathcal{Y}\|_{tr}}{[1 + \tau \|\mathcal{X}-\Im \mathcal{Y}\|_{tr}^{1/2}]^2}+ \frac{\|\mathcal{Y}-\Im \mathcal{X}\|_{tr}}{[1 + \tau \|\mathcal{Y}-\Im \mathcal{X}\|_{tr}^{1/2}]^2} \right] \end{array} \right\}. \end{align} | (5.4) |
Consider \mathcal{F}(t) = - \frac{1}{\sqrt{ t}} , \mathcal{G}(t) = \ln t ( t > 0 ) and \beta(t) = \lambda\in(0, 1) , \tau = -\ln\lambda > 0 , then (5.3) converted to
\begin{align*} \mathcal{F}(\Vert \Im (\mathcal{X})-\Im (\mathcal{Y})\Vert_{tr}) \leq \mathcal{F}(\Theta(\mathcal{U, V})) + \mathcal{G}(\beta(\Theta(\mathcal{U, V}))) \end{align*} |
where \Theta(\mathcal{U, V}) given in (5.4). Thus, all the hypotheses of Theorem 3.3 are satisfied, therefore there exists \hat{\mathcal{X}}\in \mathcal{P}(n) such that \Im (\hat{\mathcal{X}}) = \hat{\mathcal{X}} , and hence the matrix Eq (5.1) has a solution in \mathcal{P}(n) . Furthermore, we have \Lambda(\mathcal{X}, \mathcal{Y}; \mathcal{R}|_{\Im (\mathcal{P}(n))})\neq \emptyset for every \mathcal{X}, \mathcal{Y} \in \Im (\mathcal{P}(n)) owing to the presence of least upper bound and largest lower bound for each \mathcal{X}, \mathcal{Y} \in \Im (\mathcal{P}(n)) . As a result of using Theorem 3.4, we may infer that \Im has a unique fixed point, and that the matrix Eq (5.1) has a unique solution in \mathcal{P}(n) .
Example 5.5. Consider the NME (5.1) for m = 3 , \eta = 4 , n = 3 , with \mathcal{G}(\mathcal{X}) = \mathcal{X}^{1/4} , i.e.,
\begin{equation} \mathcal{X} = \mathcal{Q} + \mathcal{B}_1^* \mathcal{X}^{1/4} \mathcal{B}_1 + \mathcal{B}_2^*\mathcal{X}^{1/4}\mathcal{B}_2+\mathcal{B}_3^*\mathcal{X}^{1/4}\mathcal{B}_3, \end{equation} | (5.5) |
where
\mathcal{Q} = \begin{bmatrix} 12.722272690000000 & 1.464788500000000 & 2.414163701250000\\ 1.464788500000000 & 11.328605119375000 & 2.000862796281250\\ 2.414163701250000 & 2.000862796281250 & 13.332179887689062 \end{bmatrix}, |
\mathcal{B}_1 = \begin{bmatrix} 0.070500000000000 & 0.094800000000000 & 0.187200000000000\\ 0.076200000000000 & 0.046200000000000 & 0.191400000000000\\ 0.196200000000000 & 0.077400000000000 & 0.036600000000000 \end{bmatrix}, |
\mathcal{B}_2 = \begin{bmatrix} 0.022400000000000 & 0.029000000000000 & 0.033000000000000\\ 0.047000000000000 & 0.031400000000000 & 0.036800000000000\\ 0.049000000000000 & 0.047800000000000 & 0.031800000000000 \end{bmatrix}, |
\mathcal{B}_3 = \begin{bmatrix} 0.859375000000000 & 1.343750000000000 & 0.421875000000000\\ 0.718750000000000 & 0.375000000000000 & 0.812500000000000\\ 1.500000000000000 & 0.562500000000000 & 0.875000000000000 \end{bmatrix}. |
The conditions of Theorem 5.4 can be checked numerically, taking various special values for matrices involved. For example, they can be tested (and verified to be true) for
\mathcal{X} = \begin{bmatrix} 2.722167968750000 & 1.464355468750000 & 2.414062500000000\\ 1.464355468750000 & 1.317382812500000 & 2.000000000000000\\ 2.414062500000000 & 2.000000000000000 & 3.332031250000000 \end{bmatrix}, |
\mathcal{Y} = \begin{bmatrix} 10.000104721250000 & 0.000433031250000 & 0.000101201250000\\ 0.000433031250000 & 10.011222306875000 & 0.000862796281250\\ 0.000101201250000 & 0.000862796281250 & 10.000148637689062 \end{bmatrix}. |
To see the convergence of the sequence \{\mathcal{X}_n\} defined in (5.2), we start with three different initial values
\mathcal{X}_0 = \begin{bmatrix} 0.025970559290683 & 0.014219828729812 & 0.004760641350592\\ 0.014219828729812 & 0.055823355744100 & 0.011986278815522\\ 0.004760641350592 & 0.011986278815522 & 0.024342909184651 \end{bmatrix} |
with \Vert \mathcal{X}_0 \Vert = 0.106136824219434 , \mathcal{Y}_0 = \begin{bmatrix} 1 & 0 & 0\\ 0 & 1 & 0\\ 0 & 0 & 1 \end{bmatrix} with \Vert \mathcal{Y}_0 \Vert = 3 , \mathcal{W}_0 = \begin{bmatrix} 4 & 0 & 0\\ 0 & 4 & 0\\ 0 & 0 & 4 \end{bmatrix} with \Vert \mathcal{W}_0 \Vert = 12 . \newline We have the following approximation of the unique positive definite solution of the system (5.1) after 10 iterations:
\widehat{\mathcal{X}}\approx\mathcal{X}_{10} = \begin{bmatrix} 20.940543248755521 & 7.041955400915334 & 7.763256211115045\\ 7.041955400915334 & 16.607981685744864 & 5.475739411366025\\ 7.763256211115045 & 5.475739411366025 & 17.183592428336805 \end{bmatrix} |
\widehat{\mathcal{Y}}\approx\mathcal{Y}_{10} = \begin{bmatrix} 20.940543256819559 & 7.041955407550640 & 7.763256216731211\\ 7.041955407550640 & 16.607981691214334 & 5.475739415981650\\ 7.763256216731211 & 5.475739415981650 & 17.183592432298781 \end{bmatrix} |
\widehat{\mathcal{W}}\approx\mathcal{W}_{10} = \begin{bmatrix} 20.940543262336771 & 7.041955412090349 & 7.763256220573650\\ 7.041955412090350 & 16.607981694956411 & 5.475739419139545\\ 7.763256220573650 & 5.475739419139543 & 17.183592435009462 \end{bmatrix}. |
Also, the elements of each sequence are order preserving. The Figure 1 represents the convergence analysis of sequence and Figure 2 represents surface plot of solution.
Example 5.6. Consider the NME
\mathcal{X} = \mathcal{Q}+\mathcal{B}_1^{*}\mathcal{X}^{0.1}\mathcal{B}_1+\mathcal{B}_2^{*}\mathcal{X}^{0.1}\mathcal{B}_2 |
where \mathcal{Q}, \mathcal{B}_1, \mathcal{B}_2 are uniformly distributed positive definite matrices.
\mathcal{B}_1 = \begin{bmatrix} 1.0000 & -0.3863& 0.0275& -0.1669& 0.0528\\ -0.3863& 1.0000 & 0.4274 & -0.9587 & 0.6466\\ 0.0275 &0.4274 & 1.0000 & 0.7799& -0.9775\\ -0.1669 &-0.9587& 0.7799 & 1.0000& 0.7176\\ 0.0528& 0.6466& -0.9775& 0.7176& 1.0000 \end{bmatrix}, |
\mathcal{B}_2 = \begin{bmatrix} 1.0000 & 0.2678 & -0.0068& -0.2905& 0.1674\\ 0.2678& 1.0000& -0.6979& 0.3640 & -0.1111\\ -0.0068& -0.6979& 1.0000& 0.6944& 0.9336\\ -0.2905& 0.3640& 0.6944 & 1.0000 & 0.8174\\ 0.1674& -0.1111& 0.9336 & 0.8174 & 1.0000 \end{bmatrix}, |
\mathcal{Q} = \begin{bmatrix} 1.0000 & -0.1515& 0.0282& 0.2155& 0.6334\\ -0.1515& 1.0000 & 0.0855& -0.9102& -0.5035\\ 0.0282& 0.0855 & 1.0000 & 0.1743 &-0.0878\\ 0.2155 & -0.9102 & 0.1743 & 1.0000 & 0.4105\\ 0.6334& -0.5035& -0.0878& 0.4105 & 1.0000 \end{bmatrix}. |
Using three approximations as a starting point
\mathcal{X}_0 = 5 \times \begin{bmatrix} 1.0000 & -0.1061 & -0.3330 & 0.3496& 0.6669\\ -0.1061 & 1.0000& 0.6168& 0.4456& -0.0913\\ -0.3330 & 0.6168& 1.0000& 0.2424& -0.0166\\ 0.3496 & 0.4456 & 0.2424 & 1.0000 & 0.1357\\ 0.6669& -0.0913& -0.0166& 0.1357& 1.0000 \end{bmatrix}, |
\mathcal{Y}_0 = 2\times\begin{bmatrix} 1.0000 & 0.5814 & -0.2994& -0.3206 &-0.3171\\ 0.5814& 1.0000 & 0.4472& 0.6869& -0.0376\\ -0.2994& 0.4472 & 1.0000& -0.2630 & -0.2886\\ -0.3206& 0.6869& -0.2630 & 1.0000 & -0.3170\\ -0.3171 & -0.0376& -0.2886& -0.3170 & 1.0000 \end{bmatrix} |
\mathcal{Z}_0 = 4\times\begin{bmatrix} 1.0000 & -0.8225 &0.4813 & 0.7906& 0.1445\\ -0.8225 & 1.0000 & 0.7100& 0.0642& -0.0427\\ 0.4813 & 0.7100& 1.0000 & 0.4340 &0.3744\\ 0.7906 & 0.0642 & 0.4340 & 1.0000 & -0.5953\\ 0.1445 & -0.0427 & 0.3744& -0.5953 & 1.0000 \end{bmatrix}. |
We take \eta = \Vert \mathcal{B}_1^{*}\mathcal{B}_1+\mathcal{B}_2^{*}\mathcal{B}_2\Vert_{tr} = 16.602 , and \mathcal{G}(\mathcal{X}) = \mathcal{X}^{0.1} to test our algorithm. The numerical results are discussed in Table 1.
Initial Matrix | \mathcal{G(U)} | Iter no. | Error(1.e-10) | CPU |
\mathcal{X}_0 | \mathcal{X}_0^{0.1} | 12 | 0.9491 | 0.030092 |
\mathcal{Y}_0 | \mathcal{Y}_0^{0.1} | 13 | 0.1432 | 0.036789 |
\mathcal{Z}_0 | \mathcal{W}_0^{0.1} | 13 | 0.1085 | 0.028577 |
The following positive-definite solution is obtained after 12 iterations.
\widehat{\mathcal{X}} = \begin{bmatrix} 3.7159 & -0.3242& -0.6653& -0.0887 & 0.3253\\ -0.3242& 6.1770& -2.1357 & -2.2443 & -1.0107\\ -0.6653& -2.1357& 7.9363 & 3.3075 & 1.8312\\ -0.0887& -2.2443 & 3.3075& 7.9845 & 3.3266\\ 0.3253 & -1.0107 & 1.8312 & 3.3266 & 7.8012 \end{bmatrix}, |
with min eigenvalue 3.3143. The Figure 3 represents the convergence analysis of sequence and Figure 4 represents surface plot of solution \widehat{\mathcal{X}} .
Next, we introduce a new example consisting of randomly generated real coefficient matrices with various dimensions.
Example 5.7. Consider a randomly generated real coefficient matrices of the equation
\begin{equation} \mathcal{X} = \mathcal{Q} + \mathcal{B}_1^* \mathcal{X}^{3/10} \mathcal{B}_1 + \mathcal{B}_2^*\mathcal{X}^{3/10}\mathcal{B}_2+\mathcal{B}_3^*\mathcal{X}^{3/10}\mathcal{B}_3, \end{equation} | (5.6) |
where the coefficients \mathcal{B}_{j} ( j = 1, 2, 3 ) are chosen by \mathcal{B}_{j} = rand(n) , \mathcal{Q} = \mathcal{B}_{1}\mathcal{B}_{1}^{*}, \tau = 0.008 . All the experimental data such as iteration number, cpu time, error shown in the Table 2. The last column shows that the solution is positive definite. The Figure 5 represents the convergence analysis of sequence for various dimensions.
Dimension | No. of Iteration | Error | CPU Time | Min. Eigen Value |
4 | 20 | 5.7919e-11 | 0.055063 | 0.7974 |
8 | 22 | 9.7623e-11 | 0.072524 | 0.5615 |
12 | 25 | 3.5907e-11 | 0.109079 | 1.2494 |
16 | 26 | 4.5219e-11 | 0.139981 | 1.4793 |
20 | 27 | 3.1658e-11 | 0.262055 | 1.7858 |
30 | 28 | 5.0170e-11 | 0.439142 | 2.872 |
64 | 30 | 8.4997e-11 | 5.319442 | 5.8014 |
Definition 5.8. [11] For a complex matrix \mathcal{X} to be positive definite if and only if the Hermitian portion \mathcal{X}_{H}=\frac{1}{2}(\mathcal{X}+\mathcal{X}^{*}) be positive definite, where \mathcal{X}^{*} denotes the conjugate transpose.
Using this above Definition 5.8, a new example is illustrated below:
Example 5.9. Consider a randomly generated complex coefficient matrices of the equation
\begin{equation} \mathcal{X} = \mathcal{Q} + \mathcal{B}_1^* \mathcal{X}^{1/2} \mathcal{B}_1 + \mathcal{B}_2^*\mathcal{X}^{1/2}\mathcal{B}_2+\mathcal{B}_3^*\mathcal{X}^{1/2}\mathcal{B}_3, \end{equation} | (5.7) |
where the coefficients \mathcal{B}_{j} ( j = 1, 2, 3 ) are chosen by \mathcal{B}_{j} = rand(n)+ i \ rand(n) , \mathcal{Q} = \mathcal{B}_{1}\mathcal{B}_{1}^{*}, \tau = 0.01 . All the experimental data such as iteration number, cpu time, error shown are reported in the Table 3. The last column presents the minimum eigenvalue of the solution matrix to ensure the solution to be positive definite. The Figure 6 represents the convergence analysis of sequence for various dimensions.
Dimension | No. of Iteration | Error | CPU Time | Min. Eigen Value |
5 | 43 | 8.6462e-11 | 0.116591 | 2.5393 |
8 | 47 | 7.8654e-11 | 0.213147 | 3.7129 |
10 | 43 | 5.9279e-11 | 0.303800 | 3.4470 |
16 | 52 | 7.8891e-11 | 0.664103 | 6.9888 |
20 | 54 | 6.7639e-11 | 1.213817 | 9.8417 |
22 | 128 | 9.8101e-11 | 3.262496 | 12.0391 |
It is clear from the discussion in the Examples 5.5–5.7 and 5.9 that the solutions of NMEs are positive definite, as the lowest eigenvalues are positive for any starting matrices. Additionally, it is also clear from the solution's surface plot as it is pointing upward. Convergence analysis demonstrates that for any starting guess, it will convergence to a common value. Finally from Tables 2 and 3, it is obvious that result is true for real and complex coefficient matrices for any dimension.
The authors are thankful to the Science and Engineering Research Board, India, for providing funds under the project-CRG/2018/000615. We thank the editor for his kind support. The authors are thankful to the learned reviewers for their valuable comments.
[1] | N. Safari-Alighiarloo, M. Taghizadeh, M. Rezaei-Tavirani, Protein-protein interaction networks (PPI) and complex diseases, Gastroenterol. Hepatol. Bed. Bench., 7 (2014), 17–31. |
[2] |
B. Duo, Q. Wu, X. Yuan, Anti-jamming 3D trajectory design for UAV-enabled wireless sensor networks under probabilistic LoS channel, IEEE Trans. Veh. Technol., 69 (2020), 16288–16293. https://doi.org/10.1109/TVT.2020.3040334 doi: 10.1109/TVT.2020.3040334
![]() |
[3] | R. Zafarani, M. A. Abbasi, H. Liu, Social media mining: an introduction, Cambridge University Press, (2014), 41–49. https://doi.org/10.1017/CBO9781139088510 |
[4] |
Y. LeCun, Y. Bengio, G. Hinton, Deep learning, Nature, 521 (2015), 436–444. https://doi.org/10.1038/nature14539 doi: 10.1038/nature14539
![]() |
[5] |
X. Li, M. Yin, Hybrid differential evolution with biogeography-based optimization for design of a reconfigurable antenna array with discrete phase shifters, Int. J. Antennas. Propag., (2011), 685629. https://doi.org/10.1155/2011/685629 doi: 10.1155/2011/685629
![]() |
[6] | X. Li, M. Yin, Design of a reconfigurable antenna array with discrete phase shifters using differential evolution algorithm, Prog. Electromagn. Res., 31 (2011), 29–43. http://dx.doi.org/10.2528/PIERB11032902 |
[7] |
X. Li, S. Ma, Multi-objective memetic search algorithm for multi-objective permutation flow shop scheduling problem, IEEE Access, 4 (2016), 2154–2165. https://doi.org/10.1109/ACCESS.2016.2565622 doi: 10.1109/ACCESS.2016.2565622
![]() |
[8] | R. Cypher, J. Sanz, The SIMD model of parallel computation, Springer, 1994. http://dx.doi.org/10.1007/978-1-4612-2612-3 |
[9] | D. A. Bader, K. Madduri, Designing multithreaded algorithm for breadth-first search and st-connectivity on the Cray MTA-2, in International Conference on Parallel Processing, IEEE, (2006), 523–530. https://doi.org/10.1109/ICPP.2006.34 |
[10] | A. Siriam, K. Gautham, K. Kothapalli, Evaluating centrality metrics in real-world networks on GPU, 2009. Available from: http://citeseerx.ist.psu.edu/viewdoc/summary?doi=10.1.1.622.9442 |
[11] | A. McLaughlin, D. A. Bader, Scalable and high performance betweenness centrality on the GPU, in International Conference for High Performance Computing, Networking, Storage and Analysis, IEEE, (2014), 572–583. https://doi.org/10.1109/SC.2014.52 |
[12] | Y. Jia, V. Lu, J. Hoberock, et al., Edge v.s node parallelism for graph centrality metrics, in GPU Computing Gems (eds. W. W. Hwu), Elsevier, (2012), 15–28. https://doi.org/10.1016/B978-0-12-385963-1.00002-2 |
[13] | H. Yin, A. R. Benson, J. Leskovec, The local closure coefficient: a new perspective on network clustering, in Proceedings of the Twelfth ACM International Conference on Web Search and Data Mining, ACM, (2019), 303–311. https://doi.org/10.1145/3289600.3290991 |
[14] |
Y. M. Hu, B. Yang, H. S. Wong, A weighted local view method based on observation over ground truth for community detection, Inform. Sci., 355 (2016), 37–57. https://doi.org/10.1016/j.ins.2016.03.028 doi: 10.1016/j.ins.2016.03.028
![]() |
[15] |
Y. M. Hu, B. Yang, Enhanced link clustering with observations on ground truth to discover social circles, Knowl-Based. Syst., 73 (2015), 227–235. https://doi.org/10.1016/j.knosys.2014.10.006 doi: 10.1016/j.knosys.2014.10.006
![]() |
[16] | B. Elbirt, The nature of networks: a structural census of degree centrality across multiple network sizes and edge densities, Ph.D. thesis, State University of New York at BuffaloIn, 2007. |
[17] |
K. F. Cheung, M. G. H. Bell, J. J. Pan, An eigenvector centrality analysis of world container shipping network connectivity, Transport. Res. E-Log., 140 (2020), 101991. https://doi.org/10.1016/j.tre.2020.101991 doi: 10.1016/j.tre.2020.101991
![]() |
[18] |
T. Ioanna, B. Y. Ricardo, B. Francesco, Temporal betweenness centrality in dynamic graphs, Int. J. Data Sci. Anal., 9 (2020), 257–272. https://doi.org/10.1007/s41060-019-00189-x doi: 10.1007/s41060-019-00189-x
![]() |
[19] | I. G. Adebayo, Y. X. Sun, A novel approach of closeness centrality measure for voltage stability analysis in an electric power grid, Int. J. Emerg. Electr. Power Syst. 3 (2020). http://doi.org/10.1515/ijeeps-2020-0013 |
[20] |
A. Hashemi, M. B. Dowlatshahi, H. Nezamabadi-pour, MGFS: A multi-label graph-based feature selection algorithm via PageRank centrality, Expert Syst. Appl., 142 (2019), 113024. https://doi.org/10.1016/j.eswa.2019.113024 doi: 10.1016/j.eswa.2019.113024
![]() |
[21] |
U. Brandes, A faster algorithm for betweenness centrality, J. Math. Sociol., 25 (2001), 163–177. https://doi.org/10.1080/0022250X.2001.9990249 doi: 10.1080/0022250X.2001.9990249
![]() |
[22] |
G. Zhao, P. Jia, A. Zhou, InfGCN: Identifying influential nodes in complex networks with graph convolutional networks, Neurocomputing, 414 (2020), 18–26. https://doi.org/10.1016/j.neucom.2020.07.028 doi: 10.1016/j.neucom.2020.07.028
![]() |
[23] | S. Buß, H. Molter, R. Niedermeier, Algorithmic aspects of temporal betweenness, in Proceedings of the 26th ACM SIGKDD International Conference on Knowledge Discovery & Data Mining, ACM, (2020), 2084–2092. https://doi.org/10.1145/3394486.3403259 |
[24] |
E. Y. Yu, Y. P Wang, Y. Fu, Identifying critical nodes in complex networks via graph convolutional networks, Knowl-Based. Syst., 198 (2020), 105893. https://doi.org/10.1016/j.knosys.2020.105893 doi: 10.1016/j.knosys.2020.105893
![]() |
[25] | S. Ahamed, M. Samad, Information mining for covid-19 research from a large volume of scientific literature, preprint, arXiv: 2004.02085. http://arXiv.org/abs/2004.02085 |
[26] |
L. E. Suárez, B. A. Richards, G. Lajoie, Learning function from structure in neuromorphic networks, Nat. Mach. Intell., 3 (2021), 771–786. https://doi.org/10.1038/s42256-021-00376-1 doi: 10.1038/s42256-021-00376-1
![]() |
[27] |
S. Brin, L. Page, The anatomy of a large-scale hypertextual Web search engine, Comput. Support. Coop. Work, 30 (1998), 107–117. https://doi.org/10.1016/S0169-7552(98)00110-X doi: 10.1016/S0169-7552(98)00110-X
![]() |
[28] |
U. Brandes, C. Pich, Centrality estimation in large networks, Int. J. Bifurcat. Chaos., 17 (2007), 2303–2318. https://doi.org/10.1142/S0218127407018403 doi: 10.1142/S0218127407018403
![]() |
[29] |
J. Liu, Z. Ren, Q. Guo, Node importance ranking of complex networks, Acta Phys. Sinica., 62 (2013), 178901. https://doi.org/10.7498/aps.62.178901 doi: 10.7498/aps.62.178901
![]() |
[30] | G. Csárdi, T. Nepusz, The igraph software package for complex network research, Int. J. Complex Syst., 1695 (2006), 1–9. http://igraph.sf.net |
1. | Koti N. V. V. V. Prasad, Vinay Mishra, Zoran D. Mitrović, Ahmad Aloqaily, Nabil Mlaiki, Fixed point results for generalized almost contractions and application to a nonlinear matrix equation, 2024, 9, 2473-6988, 12287, 10.3934/math.2024600 | |
2. | Reena Jain, Hemant Kumar Nashine, RESULTS ON EXTENDED BRANCIARI GENERALIZED b-DISTANCE SPACES, WITH APPLICATIONS TO FRACTIONAL INTEGRALS AND NONLINEAR MATRIX EQUATIONS, 2025, 55, 0035-7596, 10.1216/rmj.2025.55.167 |
Initial Matrix | \mathcal{G(U)} | Iter no. | Error(1.e-10) | CPU |
\mathcal{X}_0 | \mathcal{X}_0^{0.1} | 12 | 0.9491 | 0.030092 |
\mathcal{Y}_0 | \mathcal{Y}_0^{0.1} | 13 | 0.1432 | 0.036789 |
\mathcal{Z}_0 | \mathcal{W}_0^{0.1} | 13 | 0.1085 | 0.028577 |
Dimension | No. of Iteration | Error | CPU Time | Min. Eigen Value |
4 | 20 | 5.7919e-11 | 0.055063 | 0.7974 |
8 | 22 | 9.7623e-11 | 0.072524 | 0.5615 |
12 | 25 | 3.5907e-11 | 0.109079 | 1.2494 |
16 | 26 | 4.5219e-11 | 0.139981 | 1.4793 |
20 | 27 | 3.1658e-11 | 0.262055 | 1.7858 |
30 | 28 | 5.0170e-11 | 0.439142 | 2.872 |
64 | 30 | 8.4997e-11 | 5.319442 | 5.8014 |
Dimension | No. of Iteration | Error | CPU Time | Min. Eigen Value |
5 | 43 | 8.6462e-11 | 0.116591 | 2.5393 |
8 | 47 | 7.8654e-11 | 0.213147 | 3.7129 |
10 | 43 | 5.9279e-11 | 0.303800 | 3.4470 |
16 | 52 | 7.8891e-11 | 0.664103 | 6.9888 |
20 | 54 | 6.7639e-11 | 1.213817 | 9.8417 |
22 | 128 | 9.8101e-11 | 3.262496 | 12.0391 |
Initial Matrix | \mathcal{G(U)} | Iter no. | Error(1.e-10) | CPU |
\mathcal{X}_0 | \mathcal{X}_0^{0.1} | 12 | 0.9491 | 0.030092 |
\mathcal{Y}_0 | \mathcal{Y}_0^{0.1} | 13 | 0.1432 | 0.036789 |
\mathcal{Z}_0 | \mathcal{W}_0^{0.1} | 13 | 0.1085 | 0.028577 |
Dimension | No. of Iteration | Error | CPU Time | Min. Eigen Value |
4 | 20 | 5.7919e-11 | 0.055063 | 0.7974 |
8 | 22 | 9.7623e-11 | 0.072524 | 0.5615 |
12 | 25 | 3.5907e-11 | 0.109079 | 1.2494 |
16 | 26 | 4.5219e-11 | 0.139981 | 1.4793 |
20 | 27 | 3.1658e-11 | 0.262055 | 1.7858 |
30 | 28 | 5.0170e-11 | 0.439142 | 2.872 |
64 | 30 | 8.4997e-11 | 5.319442 | 5.8014 |
Dimension | No. of Iteration | Error | CPU Time | Min. Eigen Value |
5 | 43 | 8.6462e-11 | 0.116591 | 2.5393 |
8 | 47 | 7.8654e-11 | 0.213147 | 3.7129 |
10 | 43 | 5.9279e-11 | 0.303800 | 3.4470 |
16 | 52 | 7.8891e-11 | 0.664103 | 6.9888 |
20 | 54 | 6.7639e-11 | 1.213817 | 9.8417 |
22 | 128 | 9.8101e-11 | 3.262496 | 12.0391 |