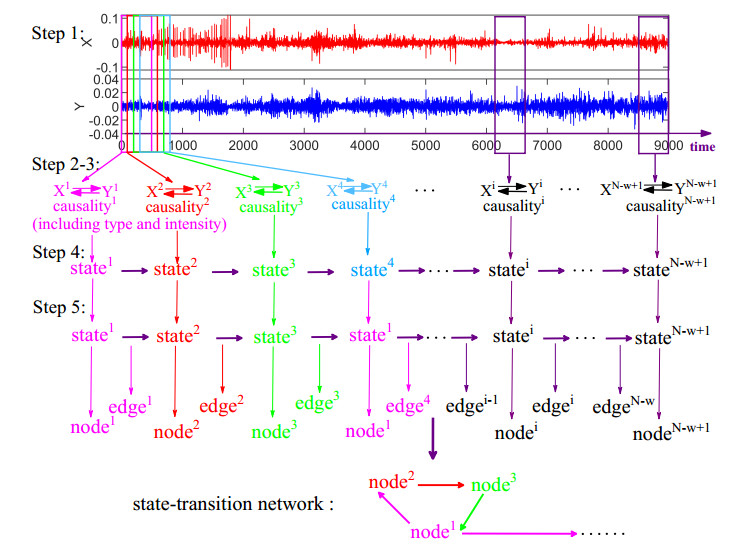
This study provides a systematic literature review of research (2001–2020) in the field of teaching and learning a foreign language and intercultural learning using immersive technologies. Based on 2507 sources, 54 articles were selected according to a predefined selection criteria. The review is aimed at providing information about which immersive interventions are being used for foreign language learning and teaching and where potential research gaps exist. The papers were analyzed and coded according to the following categories: (1) investigation form and education level, (2) degree of immersion, and technology used, (3) predictors, and (4) criterions. The review identified key research findings relating the use of immersive technologies for learning and teaching a foreign language and intercultural learning at cognitive, affective, and conative levels. The findings revealed research gaps in the area of teachers as a target group, and virtual reality (VR) as a fully immersive intervention form. Furthermore, the studies reviewed rarely examined behavior, and implicit measurements related to inter- and trans-cultural learning and teaching. Inter- and transcultural learning and teaching especially is an underrepresented investigation subject. Finally, concrete suggestions for future research are given. The systematic review contributes to the challenge of interdisciplinary cooperation between pedagogy, foreign language didactics, and Human-Computer Interaction to achieve innovative teaching-learning formats and a successful digital transformation.
Citation: Rebecca M. Hein, Carolin Wienrich, Marc E. Latoschik. A systematic review of foreign language learning with immersive technologies (2001-2020)[J]. AIMS Electronics and Electrical Engineering, 2021, 5(2): 117-145. doi: 10.3934/electreng.2021007
[1] | YongKyung Oh, JiIn Kwak, Sungil Kim . Time delay estimation of traffic congestion propagation due to accidents based on statistical causality. Electronic Research Archive, 2023, 31(2): 691-707. doi: 10.3934/era.2023034 |
[2] | Ming Wei, Shaopeng Zhang, Bo Sun . Airport passenger flow, urban development and nearby airport capacity dynamic correlation: 2006-2019 time-series data analysis for Tianjin city, China. Electronic Research Archive, 2022, 30(12): 4447-4468. doi: 10.3934/era.2022226 |
[3] | Yuxia Liu, Qi Zhang, Wei Xiao, Tianguang Chu . Characteristic period analysis of the Chinese stock market using successive one-sided HP filter. Electronic Research Archive, 2023, 31(10): 6120-6133. doi: 10.3934/era.2023311 |
[4] | Jiping Xing, Xiaohong Jiang, Yu Yuan, Wei Liu . Incorporating mobile phone data-based travel mobility analysis of metro ridership in aboveground and underground layers. Electronic Research Archive, 2024, 32(7): 4472-4494. doi: 10.3934/era.2024202 |
[5] | Moutian Liu, Lixia Duan . In-phase and anti-phase spikes synchronization within mixed Bursters of the pre-Bözinger complex. Electronic Research Archive, 2022, 30(3): 961-977. doi: 10.3934/era.2022050 |
[6] | Zhihui Wang, Yanying Yang, Lixia Duan . Dynamic mechanism of epileptic seizures induced by excitatory pyramidal neuronal population. Electronic Research Archive, 2023, 31(8): 4427-4442. doi: 10.3934/era.2023226 |
[7] | Li Li, Zhiguo Zhao . Inhibitory autapse with time delay induces mixed-mode oscillations related to unstable dynamical behaviors near subcritical Hopf bifurcation. Electronic Research Archive, 2022, 30(5): 1898-1917. doi: 10.3934/era.2022096 |
[8] | Jianmin Hou, Quansheng Liu, Hongwei Yang, Lixin Wang, Yuanhong Bi . Stability and bifurcation analyses of p53 gene regulatory network with time delay. Electronic Research Archive, 2022, 30(3): 850-873. doi: 10.3934/era.2022045 |
[9] | Jieqiong Yang, Panzhu Luo . Study on the spatial correlation network structure of agricultural carbon emission efficiency in China. Electronic Research Archive, 2023, 31(12): 7256-7283. doi: 10.3934/era.2023368 |
[10] | Ariel Leslie, Jianzhong Su . Modeling and simulation of a network of neurons regarding Glucose Transporter Deficiency induced epileptic seizures. Electronic Research Archive, 2022, 30(5): 1813-1835. doi: 10.3934/era.2022092 |
This study provides a systematic literature review of research (2001–2020) in the field of teaching and learning a foreign language and intercultural learning using immersive technologies. Based on 2507 sources, 54 articles were selected according to a predefined selection criteria. The review is aimed at providing information about which immersive interventions are being used for foreign language learning and teaching and where potential research gaps exist. The papers were analyzed and coded according to the following categories: (1) investigation form and education level, (2) degree of immersion, and technology used, (3) predictors, and (4) criterions. The review identified key research findings relating the use of immersive technologies for learning and teaching a foreign language and intercultural learning at cognitive, affective, and conative levels. The findings revealed research gaps in the area of teachers as a target group, and virtual reality (VR) as a fully immersive intervention form. Furthermore, the studies reviewed rarely examined behavior, and implicit measurements related to inter- and trans-cultural learning and teaching. Inter- and transcultural learning and teaching especially is an underrepresented investigation subject. Finally, concrete suggestions for future research are given. The systematic review contributes to the challenge of interdisciplinary cooperation between pedagogy, foreign language didactics, and Human-Computer Interaction to achieve innovative teaching-learning formats and a successful digital transformation.
The dollar is an international currency issued and endorsed by the Federal Reserve. As a stable currency, it is used for the most extensive payment and settlement. Gold is a precious metal, which contains commodity and monetary attributes. Accordingly, both have integrated vital risk aversion functions, whose has always been a concern to the financial media and the investors. So far, there have been multiple studies on the relationship between the gold price and the dollar index [1,2,3,4,5,6,7]. Most studies showed a significant negative correlation between them [8,9,10]; when the dollar depreciates, the nominal price of gold will rise. Therefore, gold behaves as a hedge against the dollar and provides exchange rate hedging for investors holding the dollar. On the contrary, observers have long noted a positive correlation of them, although this correlation will not last long [3,11,12,13,14]. Moreover, the relationship is not always stable [1,15]. When the financial markets run smoothly, gold and the dollar will show a trend of going up and falling on the other; and when there is a local political crisis or economic crisis in the world, the correlation will be positive.
The previous studies have obtained time-varying causal relationships between gold and the dollar based on the econometric framework [1,15], such as the error correction model, the standard bivariate GARCH models and the extension to the structural BEKK model. In addition, Lin et al. [16] utilized wavelet analysis to decompose the pair relations between oil-US dollar and gold-US dollar into short-term and long-term parts, they found that the pair relationship becomes weaker in the long run and the short-run correlations are much higher after the early 1990s. Moreover, Mo et al. [3] investigated the long-term relationship by means of both fractional cointegration and DCC−MGARCH method and employed non-linear asymmetric causality to examine the effect of the 2007 global financial crisis on the short-term interdependence. However, both the causality's evolution rule and motif structure were ignored in these studies. It is still an open issue to discover the motifs and their relationships. The combined application of time-series analysis and complex network theory effectively describes the temporal evolution of relationships in complex systems [17,18,19]. Methods of complex networks have been proposed to analyze time series, such as visibility graph methods [20], recurrence network method [21], coarse-graining method [22], pseudo-periodic time series [23], quasi-isometric transformation [24], correlation matrix [25] and the state-transition network [26,27,28]. These methods can map the time series into the network. In this article, we apply the state-transition network method of easy-to-operate to convert the time series into a complex network. The method can reveal dynamic information as well as complicated behaviour in complex systems.
In recent years, causal analysis has attracted the attention of many researchers because it can reveal the internal laws between variables. For example, the Granger causality test has successfully revealed the non-linear causal relationship of separable variables [29,30]; transfer entropy can detect causality based on the information flowing between variables, by which the large sample size is required to estimate accurately [31]. Moreover, the cross-convergent mapping (CCM) indicates that the time-series variables are causally linked if they are from the same dynamical system. Namely, they share a common attractor [32,33]. It can distinguish the causality from the correlation in inseparable systems, which complements the Granger causality test. The weakness for the CCM method is that it is sensitive to noise of which the influence inevitably exists in natural systems.
In order to decrease the impact of noise, Stavroglou et al. proposed the pattern causality algorithm (PC) to investigate the symbolic dynamics based on the CCM [34,35]. The PC method can judge not only the causality intensity but also the causality type, by which the causality is divided into three types, i.e., positive, negative, and dark (a more complex causal relationship). The targeted partition allows the unique identification for both persistent causal structures and dominant influences that would otherwise be lost in the noise of disparate causalities(if we did not discern the three types of interactions). Moreover, this method reveals causality between variables from the perspective of non-linear dynamics.
In this article, a state-transition network was built to detect the evolutional or time-varying characteristics in the causality between gold price and the dollar index. Specifically, the idea is to extract all possible segments from the initial bivariate time-series of gold price and the dollar index by a sliding window. Then, for each segment, the non-linear causality is identified by the PC method, containing the causality type as well as causality intensity. The causality pattern is used to describe the state. We mapped all the states defined as nodes to a unique state chain. An edge is added between two states(nodes) if they appear successively. The resulting network is called a state-transition network whose topological structure can represent the dynamic characteristics of the causal relationship. Thus, the results of this paper provide a reference for studying the time-varying characteristics of causality between gold and the dollar. The structure of this article is as follows. In Section Ⅱ, we present (ⅰ) the data description of gold and the dollar analyzed in this study; (ⅱ) the steps of the method that combines PC and state-transition network; (ⅲ) definitions of concepts; Section Ⅲ shows (ⅰ) determination of an appropriate window size; (ⅱ) identification of important causality states; (ⅲ) identification of the transitions among nodes and among clusters, respectively; Section Ⅳ summarizes and discusses the results.
The daily closing prices of gold futures and the dollar index futures, respectively, were downloaded from the website, https://cn.investing.com/. They are issued by the Intercontinental Exchange of the United States, of which stock exchange codes are ZG and DX, respectively. The data was selected from November 21, 1985 to June 22, 2022. The length of the bivariate series is 9248 after deleting a small number of missing data (63 data points of gold and 83 data points of the dollar). Note that Saturdays and Sundays are excluded from the time series. Because the original data is unstable and there is a large difference between gold and the dollar, we performed the analysis based on the logarithmic return rate of daily closing prices.
The specific process for building the state-transition network consists of the following five steps: (ⅰ) dividing the time series into segments of the equal length; (ⅱ) obtaining the reconstructed phase space for each fragment; (ⅲ) calculating the causality types as well as the causality intensities by the PC method; (ⅳ) transforming the causality types and the causality intensities into causality states; (ⅴ) constructing the state-transition network. The whole process is shown in Figure 1.
Step 1. Dividing the time series into segments of equal length.
For simplicity, let X and Y represent gold and the dollar, respectively. X and Y can be expressed as time series X=X(1),⋯,X(N) and Y=Y(1),⋯,Y(N), respectively, where N is the length of time series. Let the window size be w and the sliding step be 1. Accordingly, N−w+1 fragments are obtained for each time series. Then, the ith fragment can be expressed as:
Xi={X(i),X(i+1),⋯,X(i+w−1)},1≤i≤N−w+1,Yi={Y(i),Y(i+1),⋯,Y(i+w−1)},1≤i≤N−w+1. | (2.1) |
Step 2. Obtaining the reconstructed phase space for each fragment.
We reconstructed the phase space for each fragment defined by Eq (2.1) [36,37]. E and τ represent the embedded dimension and delay time, respectively. A data point is an E-dimensional vector in the phase space [37]. The jth point in the pase space of the ith fragment can be expressed as
x(j)={Xi(j),Xi(j+τ),Xi(j+2τ),⋯,Xi(j+(E−1)τ))},1≤i≤N−w+1,1≤j≤w−(E−1)τ, | (2.2) |
where E and τ are the two parameters of which values are required to be determined. Their values will affect the accuracy of the results. If the values are too large, the system will lose much important information; and if the values are too small, the system will be easily disturbed by noise. A great deal of work have been done around this issue. In this article, the C−C method [38] is utilized to determine the values of E and τ, simultaneously. By this method, the optimal embedding dimensions were obtained for the two variables X, Y are 2 and 3, respectively. The values of the dimensions are also limited by the application of PC method, i.e., the embedding dimension of each variable should be the same and the missing information of the system should be as little as possible. Therefore, the values of E and τ were set to 3 and 1 in this article, respectively. According to Eq (2.2), ith fragment of X can be expressed in the phase space by the matrix MXi:
Xi={X(i),X(i+1),⋯,X(i+w−1)}⇒MXi=(x(1)={Xi(1),Xi(1+τ),⋯,Xi(1+(E−1)τ)}x(2)={Xi(2),Xi(2+τ),⋯,Xi(2+(E−1)τ)}⋮x(w−(E−1)τ)={Xi(w−(E−1)τ),Xi(w−(E−2)τ),⋯,Xi(w)}), | (2.3) |
Similarly, we can obtain the matrix MYi by Eq (2.3).
Step 3. Calculating the causality patterns as well as the causality intensities.
PC is a method proposed by Stavroglou et al. to explore nonlinear causality between time series developed from CCM [34]. It can judge not only the the causality intensity but also the causality type. The PC method is described as below. The distance matrix DXi is defined as:
DXi=(d(x(1),x(1))d(x(1),x(2))⋯d(x(1),x(w−(E−1)τ))d(x(2),x(1))d(x(2),x(2))⋯d(x(2),x(w−(E−1)τ))⋮⋮⋮⋮d(x(w−(E−1)τ),x(1))d(x(w−(E−1)τ),x(2))⋯d(x(w−(E−1)τ),x(w−(E−1)τ))), | (2.4) |
where d(x(t),x(t+1)) is the Euclidean distance from x(t) to x(t+1) for a focused time t. According to Eq (2.4), E+1 real nearest neighbors for x(t) represented by NNx(t) were found in Eq (2.5). The reason why we chose E+1 nearest neighbor was that it requires at least E+1 point to form bounded simplicity in E-dimensional phase space. From these E+1 nearest neighbors, two pieces of information were recorded, i.e., their time indexes tx1,tx2,⋯,txE+1, and their distance from a focused point x(t). Similarly, we can obtain y(t)'s E+1 nearest neighbors and their time indices ty1,ty2,⋯,tyE+1. These time indices corresponding to the nearest neighbors to y(t) on MYi are used to identify the points in MXi, i.e., x(t)'s E+1 estimated nearest neighbors by y(t). Thus, the distances from x(t) to x(t)'s E+1 real nearest neighbors and the distances from x(t) to x(t)'s E+1 estimated nearest neighbors by y(t) are shown in Eq (2.6).
NNx(t)=min(E+1){d(x(t),x(1)),d(x(t),x(2)),⋯,d(x(t),x(t−(E−1)τ))}={NNx(t1),NNx(t2),⋯,NNx(tE+1)}⇒<tx1,tx2,⋯,txE+1>, | (2.5) |
dj=d(x(t),x(txj)),1≤j≤E+1ˆdj=d(x(t),x(tyj)),1≤j≤E+1 | (2.6) |
The real pattern of the MXi's tth point x(t) is:
Patternx(t)=signature(sigx(t)), | (2.7) |
where
sigx(t)=E+1∑j=1Wjsj, |
Wj=edj∑E+1j=1edj,1≤j≤E+1, |
sj=(X(tj−τ)−X(tj)X(tj),X(tj−2τ)−X(tj−τ)X(tj−τ),⋯,X(tj−(E−1)τ)−X(tj−(E−2)τ)X(tj−(E−2)τ)),1≤j≤E+1, |
where the function signature stands for symbolization. In particular, when sigy(t) is greater than 0, Patterny(t) stands for ↗; when sigy(t) is less than 0, Patterny(t) stands for ↘; when sigy(t) is equal to 0, Patterny(t) stands for →. Furthermore, the estimated pattern of the MXi's tth point x(t) by MYi's tth point y(t) is:
^Patternx(t)=signature(^sigx(t)), | (2.8) |
where
^sigx(t)=E+1∑j=1ˆWjsj, |
ˆWj=eˆdj∑E+1j=1eˆdj,1≤j≤E+1. |
Similarly, the real pattern and the estimated pattern of each point in MXi and MYi can also be obtained, i=1,2,...,N−w+1. All the situations of PC pattern are shown for E=3 in Figure 2. The left blue striped, right red striped, and purple boxes represent positive causalities, negative causalities and dark causalities, respectively. We recorded the point with the same pattern of Patternx(t) and ^Patternx(t), i.e., the accurately estimated point. Each cell (the box in Figure 2) is filled with proportion of each pattern in the total accurately predicted points. Thus, the value of each cell is in the range from 0 to 1. The causality type of X on Y is the largest number's pattern in the ith fragment denoted as piX→Y; and the causality intensity is the proportion of the largest number's pattern in all patterns denoted as siX→Y. By repeating this process, we can obtain both the causality type and the causality intensity from X to Y and from Y to X for all fragments.
Step 4. Transforming causality types and causality intensities into causality states.
The causality type and the causality intensity are transformed into a particular state, i.e., coarse-grained local features while retaining only some large-scale features. A four-letter string represents the state of each fragment. The first and third letters represent the causality type from X to Y and from Y to X, respectively, and the second and fourth letters represent the causality intensity from X to Y and from Y to X. For example, the first and second letters can be obtained by the following formula,
TypeiX→Y={P,piX→Y=PositiveN,piX→Y=Negative,1≤i≤N−w+1,D,piX→Y=Dark | (2.9) |
IntensityiX→Y={a,0.8<siX→Y≤1b,0.6<siX→Y≤0.8c,0.4<siX→Y≤0.6,1≤i≤N−w+1.d,0.2<siX→Y≤0.4e,0≤siX→Y≤0.2 | (2.10) |
Similarly, TypeiY→X and IntensityiY→X can be obtained by Eqs (2.9) and (2.10). Therefore, the state of the ith fragment is defined. If TypeiX→Y=N, IntensityiX→Y=b, TypeiY→X=P, IntensityiY→X=d, the state of ith fragment is NbPd. It means that the causality type from X to Y is negative, and the intensity is between 0.6 and 0.8; the causality type from Y to X is positive, and the intensity is between 0.2 and 0.4. According to this definition, the number of possible states is 225, and the combination rule is shown in Table 1.
(the type of gold's influence on the dollar) | The second letter (the strength of gold's influence on the dollar) | The third letter (the type of dollar's influence on the gold) | The fourth letter (the strength of dollar's influence on the gold) |
P | a, (0.8, 1] | P | a, (0.8, 1] |
b, (0.6, 0.8] | b, (0.6, 0.8] | ||
c, (0.4, 0.6] | c, (0.8, 0.6] | ||
d, (0.2, 0.4] | d, (0.8, 0.4] | ||
e, [0, 0.2] | e, [0, 0.2] | ||
N | a, (0.8, 1] | N | a, (0.8, 1] |
b, (0.6, 0.8] | b, (0.6, 0.8] | ||
c, (0.4, 0.6] | c, (0.8, 0.6] | ||
d, (0.2, 0.4] | d, (0.8, 0.4] | ||
e, [0, 0.2] | e, [0, 0.2] | ||
D | a, (0.8, 1] | D | a, (0.8, 1] |
b, (0.6, 0.8] | b, (0.6, 0.8] | ||
c, (0.4, 0.6] | c, (0.8, 0.6] | ||
d, (0.2, 0.4] | d, (0.8, 0.4] | ||
e, [0, 0.2] | e, [0, 0.2] |
Step 5. Constructing the state-transition network.
We arranged states into a state chain according to the temporal order of appearance,
state1→state2→⋯→stateN−w+1, | (2.11) |
in order to visualize the state chain, we mapped Eq (2.11) to a network by numbering each state, i.e., the first state PaPa as node 1, the second state PaPb as node 2, ..., the last state DeDe as node 225. Then, if two nodes appear adjacently, a directed edge is added between them. The network is called the directed and weighted state-transition network in which the direction is the order of the nodes appearing and the weight is the number of the edge between nodes.
1) Degree: incoming degree or outgoing degree is defined as the number of incoming or outgoing links for a focused node. Note that the incoming (or outgoing) degree for a focused state (node) is equal to the number of occurrences of this state, except the state which appears in the first or last position in the state chain.
2) Hub node: nodes with extremely large degrees in the network.
3) Edge: if two states appear adjacently (i before j), a directed edge is added from i to j. The directed linkage has a clear physical meaning as the occurrence of the transition from i to j in the state chain [27,28].
4) Edge weight: the edge weight indicates the strength of the relationship between the two connected nodes. Herein, the number of transfers between two nodes is defined as the weight of the edges between them. Note that this concept is very important, because we can obtained the transfer preferences between nodes based on this number. Moreover, according to the transition preferences from the focused state to other states, we can predict the subsequent state.
5) Shuffled data: the sequence is obtained by randomly disrupting the positions of the data in the original sequence.
6) Motif: if a node's degree for the original data is significantly larger than it in the shuffled data, the node is called a motif [39,40].
7) Node's position sequence: the sequence of positions where the focused state (node) appears in the state chain.
8) Hurst index (H): a long-range correlation is common in actual data, which can be evaluated by estimating its Hurst index (scaling exponent). Different values of H indicate different evolution behaviors for the sequence. When 0<H<0.5, the sequence shows anti-persistent evolution behavior, i.e., the state of the next moment is the opposite state of the previous one with large possibility; when H=0.5, the characteristic of the sequence is a random walk, i.e., the two successive states of the sequence have no correlation; when 0.5<H<1, a positive long-range correlation exists in the sequence, i.e., the next moment tends to maintain the same state as the previous one. The methods for estimating the Hurst index can be divided into the time-domain and frequency-domain methods. The time-domain methods include the variance-time method [41], the absolute values of the aggregated series method [41], the rescaled range analysis method (R/S) [42,43] and the detrended fluctuation analysis method [44]; the frequency-domain methods include the periodic-diagram method [41], the whittle estimation method [45] and the wavelet analysis method [46].
9) Cluster: the cluster in the network is a sub-network for which the internal edges are denser and the external edges are sparse [47], i.e., nodes in the same cluster are more similar in specific attributes.
In general, the window size w impacts results [48]. If w is too large, the rules of causality evolution between gold and the dollar will be obscured; while if w is too small, it will be more vulnerable to noise, and both the complexity and research cost will be higher. In addition, w also affects the number of states, that is, the larger w is, the less the number will be. In order to ensure the stability as well as diversity of the number of states, a balanced value for w is required.
The effect of the size w is examined from 100 to 1000 (the interval is 100) in that we obtain 10 state-transition networks. The numbers of nodes Nn and the numbers of edges Ne in state-transition networks are shown in Figure 3(a). The results show that both Nn and Ne for the shuffled sequence are more than that of the original sequence. In addition, both Nn and Ne for the original sequence decrease sharply with the increase of w when w is less than 600. When w is around 600, both Nn and Ne tends to be stable. On the contrary, both Nn and Ne for the shuffled sequence do not tend to be stable as that of the original sequence when w is around 600, i.e., the network structures of the shuffled sequence are not stable. Moreover, when w is 600 or 900, rn or re are the smallest (i.e., the radio is the most deviated from 1), respectively (see Figure 3(b)). Herein, rn and re represent the ratio of number of nodes of the original sequence to it of the shuffled sequence and the ratio of number of edges of the original sequence to it of the shuffled sequence, respectively. Accordingly, the difference between the original network and the shuffled network is the largest. Since the larger the w is, the more the information is lost and the higher the complexity is. Therefore, we assume that the reasonable value for w is 600 (i.e., a segment of around 840 days). Without special statement, w is selected as 600 through this article.
Figure 4 shows the state-transition networks for the original data (a) and the shuffled data (b), respectively. There are 23 nodes and 86 edges in (a) that are much smaller than 225 nodes and 225 × 225 = 50,625 edges for the all-to-all network. With regard to causality types, 10 nodes correspond to the states of negative causality from both directions (from gold to dollar and from dollar to gold), 4 nodes correspond to the states of dark causality from both directions, 9 nodes correspond to the state of negative causality from one direction and the state of dark causality from the other direction, and only one node 58 (i.e., State PdDc) corresponds to the state of positive causality from one direction and state of dark causality from the other direction. With respect to the causality intensity, only it of negative causality can be strong, i.e., larger than 0.6, whereas it of dark causality or positive causality is relatively low.
The state-transition network is heterogenous in (a). Most nodes have small degrees, whereas several hubs have large degrees. For example, both the incoming degree and outgoing degree are equal to 8 for Node 113 (i.e., State NcNc), and both the incoming degree and outgoing degree are 6 for Node 97 (i.e., State NbNb). Both Nodes 113 and 97 correspond to the states of negative causality from both directions. The edge weights are extremely heterogenous because the total weights of the two hubs' self-connecting edges are large, which ratio to the sum of the edge weights for the whole network is 68%. The self-connecting edges represent that when the focused state (node) appears, the state of the next moment remains the focused one. The state-transition network for the shuffled time series is shown in (b). The network is less heterogenous for the shuffled data. There are 33 nodes and 227 edges in (b). Both the numbers of nodes and edges are relatively large and messy in this network, where only Node 193 (i.e., State DcDc) appears more frequently. State DcDc corresponds to the dark causality from both directions, which exists between two independently random sequences. In a word, the hubs of state-transition networks for the original data (a) and the shuffled data (b) correspond to the states of negative causality and dark causality from both directions, respectively.
Figure 5 shows the comparison in the occurrence number of each state between the original data and the shuffled data. The numbers of occurrences for the two hubs (i.e., the incoming or outgoing degrees of Nodes 97 and 113) in the original data are apparently more than theirs in the shuffled data (a). Therefore, Nodes 113 and 97 are motifs. In addition, the number of occurrences for Node 193 in the shuffled data is visibly more than it in original data (a). Next, we examine whether the occurrence of the motifs are accidental or not. By the R/S method, we estimate the Hurst index for the time sequence composed of the positions where the nodes appear in turn in Figure 5(b)–(d). The Hurst indexes of Nodes 97 and 113 in the original data are larger than 0.5 (H97,O=0.58 and H113,O=0.69), respectively, and it of Node 193 in the shuffled data is close to 0.5 (H193,S=0.51). The results are entirely in line with intuitive expectations, i.e., the occurrences of Nodes 97 or 113 for the original data is long-range related, while the occurrence of Node 193 for the shuffled data is random.
The importance of a node is reflected not only in the occurrence number but also in the probability of it transferring to other nodes. If the transition probability from one focused node to another is relatively large, the node of the next moment can be predicted when the focused node appears. Furthermore, if several nodes often transfer to each other, these nodes form a cluster.
In this article, a fast heuristic algorithm [49] is utilized to find the clusters. There are 4 clusters (see Figure 6), each of which mainly contains the same causality types as well as causality intensities. For simplicity, we let "weak" represents the causality intensity "d" or "e", "medium" represents the intensity "c", and "strong" represents the intensity "a" or "b". The largest cluster (Cluster I) is composed of 13 nodes that are mainly the states of weak dark causalities or the states of weak negative causalities. Although both the numbers of nodes and edges in Cluster I are the largest that are more than half of the whole network, the sum of its edge weights is meagre (see Figure 7(a)). In the second largest cluster (Cluster II), four out of five nodes are the states of medium or strong negative causalities. Both the numbers of nodes and edges in Cluster II account for a small proportion, but the sum of its edge weights reaches 84% of the whole network (see Figure 7(a)). In the third largest cluster (Cluster III), all of the three nodes correspond to the states of strong negative causalities. In the smallest cluster (Cluster IV), both of the two nodes are the states of strong negative causalities. In order to show the transition within each cluster more clearly, (b)–(e) show the structures of the four clusters, respectively. It is visible that most edges within each cluster are bi-directional, in other words, they can mutually transfer to each other within the cluster.
The connections across different clusters are different with the connections within each cluster. The edges across different clusters are much less than edges within the same cluster. The edges between clusters are very important, because they act as bridges in the transition across different clusters. Figure 7(b) shows the transition frequencies between clusters. Cluster II can transfer to the other three clusters, and the other three can also transfer to Cluster II, whereas the other three can not transfer to each other. Therefore, Cluster II acts as a bridge in the transition among clusters. It means that even if there is a positive or dark causality (i.e., Cluster I, Cluster III or Cluster IV) between gold and the dollar, it is temporary and will soon transfer to Cluster II. Thus, we suggest that the relationship between gold and the dollar is the negative causality of being relatively stable for a long time. In a word, we can more accurately predict the causality between gold and the dollar next moment by these transition preferences of intra-cluster and inter-clusters.
In this article, a method which combines the PC method [34,35] and the state-transition network was developed to identify the characteristics of the causality evolution between gold and the dollar. Based on the PC method, we can identify not only the causality intensity but also the causality type in the segments of the bivariate time series, including the types of positive causality, negative causality and the dark causality. Then, both the causality type and the causality intensity were transformed into a particular state in each segment, i.e., a four-letter string. Finally, the state-transition network was built to detect the characteristics in the causality evolution between them, where the node is the causality state and the edge is the transition between states.
Two nodes (States NbNb and NCNC) were referred to motifs in the state-transition network. Surprisingly, both motifs corresponds to the strong or medium negative causality. The sum of the edge weights for these two motifs accounts for 68% of the total edge weights for the whole network, in other words, the number of appearances for these two states also accounts for 68% for the whole network. Thus, we suggest that the relationship between gold and the dollar is the negative causality of relatively stable for a long time, which corresponds to the previous research results [1,15].
Several nodes form a cluster because they often transfer to each other. The transition preferences between causality states are compared between intra-cluster and inter-cluster. With regard to the intra-cluster, the transitions of nodes are not only frequent but also bidirectional. The nodes within the cluster can transfer to each other, but it is rare to transfer from one cluster to another. It is the other reason why the relationship between gold and the dollar is negative causality of being relatively stable for a long time. With respect to the inter-cluster, although the transitions across different clusters are much less than transitions within clusters, they play a key role in the connections (transitions) between different clusters. We found that Cluster II was especially essential. It can transfer to the other three clusters, and the other three can also transfer to Cluster II. However, the other three clusters can not transfer to each other. Therefore, Cluster II play a bridge role in the connections between clusters. It means that even if there is a positive or dark causality (i.e., Cluster I, Cluster III or Cluster IV) between gold and the dollar, it is temporary and will soon transfer to Cluster II. In a word, these transition preferences of intra-cluster and inter-clusters provide helpful information to evaluate the current causality between gold and the dollar, and to predict the causality of the next moment.
Different clusters are different in both the causality type and causality intensity of the nodes (states). Cluster I is mainly composed of states of weak dark causality, II states of strong or medium negative causality, III states of strong negative causality, and IV states of weak negative causality. It means that not only the causality type of nodes but also the causality intensity within each cluster are roughly the same. From this perspective, we can also explain the long-term stability of the negative causality between gold and the dollar.
As mentioned in [48], the window size w impacts results. By comparing the properties of the network constructed under different w, we found that the network structure tends to be stable when the w is around 600 (i.e., a segment of around 840 days). This discovery can provide a suitable time scale for future study.
The following suggestions may be of assistance to investors. Firstly, the number of appearances for two states accounts for 68% for the whole network. This indicates a negative correlation between gold and the dollar. That is, gold prices rise as the dollar depreciates, when investors are more likely to make more profits by investing in gold. Conversely, gold prices fall as the dollar appreciates, accordingly, it is a good time for investors to be short gold. Secondly, the existence of many self-connected edges in the network indicates that the relationship between them is difficult to change in short-term. Thus, it is risky for long-term investment, but for adventurous investors it can yield high returns in a short-term. Thirdly, investors should also consider the transition preferences of intra-cluster and inter-cluster when making investment decisions to reduce investment risk. For example, if the current relationship between gold and the dollar belongs to Cluster II, conservatives will likely believe the relationship will remain in Cluster II at the next moment (the self-connected edge weight is 7200), while adventurers will be more inclined to believe it will transfer to Cluster III (the transfer weight is 19). Finally, the length of 600 research window (i.e., a segment of around 840 days) is more conducive to the discovery of stable evolutionary rules that can assist investors in forming effective judgments.
Our research provides a new perspective to study the evolutional causality between two variables, i.e., gold and the dollar. In future, it is worth studying the evolutional causality between multiple variables, such as gold, the dollar, the price of crude oil, the financial crisis, and the political situation.
This work is supported by the National Natural Science Foundation of China under Grant No.11875042 and and the Natural Science Foundation of Shanghai under Grant No. 21ZR1443900.
The authors declare there is no conflicts of interest.
[1] |
Ahn SJ, Bostick J, Ogle E, et al. (2016) Experiencing nature: Embodying animals in immersive virtual environments increases inclusion of nature in self and involvement with nature. Journal of Computer-Mediated Communication 21: 399–419. doi: 10.1111/jcc4.12173
![]() |
[2] |
Banakou D, Groten R, Slater M (2013) Illusory ownership of a virtual child body causes overestimation of object sizes and implicit attitude changes. Proceedings of the National Academy of Sciences 110: 12846–12851. doi: 10.1073/pnas.1306779110
![]() |
[3] | Barreira J, Bessa M, Pereira LC, et al. (2012) MOW: Augmented Reality game to learn words in different languages: Case study: Learning English names of animals in elementary school. In 7th Iberian conference on information systems and technologies (CISTI 2012). 1–6. IEEE. |
[4] | Barrett MD (2011) Intercultural competence. EWC Statement Series 2: 23–27. |
[5] | Bazzaza MW, Alzubaidi M, Zemerly MJ, et al. (2016) Impact of smart immersive mobile learning in language literacy education. In 2016 IEEE Global Engineering Education Conference (EDUCON). 443–447. IEEE. |
[6] | Berns A, Mota JM, Ruiz-Rube I, et al. (2018) Exploring the potential of a 360 video application for foreign language learning. In Proceedings of the Sixth International Conference on Technological Ecosystems for Enhancing Multiculturality. 776–780. |
[7] | Blell G, Doff S (2014) Mehrsprachigkeit und Mehrkulturalität: Einführung in das Thema. Zeitschrift für Interkulturellen Fremdsprachenunterricht 19. |
[8] |
Bond M, Marín VI, Dolch C, et al. (2018) Digital transformation in German higher education: student and teacher perceptions and usage of digital media. Int J Educ Technol H 15: 1–20. doi: 10.1186/s41239-017-0083-9
![]() |
[9] |
Buhl H, Winter R (2009) Full virtualization – BISE's contribution to a vision. Business and Information Systems Engineering 1: 133–136. doi: 10.1007/s12599-008-0023-2
![]() |
[10] | Byram M, Doyé P (1999) Intercultural competence and foreign language learning in the primary school. The teaching of modern foreign languages in the primary school, 138–151. |
[11] | Chabot S, Drozdal J, Peveler M, et al. (2020) A collaborative, immersive language learning environment using augmented panoramic imagery. In 2020 6th International Conference of the Immersive Learning Research Network (iLRN), 225–229. |
[12] | Chang YJ, Chen CH, Huang WT, et al. (2011) Investigating students' perceived satisfaction, behavioral intention, and effectiveness of English learning using augmented reality. In 2011 IEEE International Conference on Multimedia and Expo. 1–6. |
[13] | Chen G, Starosta W (1999) A review of the concept of intercultural awareness. Human Communication 2: 27–54. |
[14] | Chen CP, Wang CH (2015) The effects of learning style on mobile augmented-reality-facilitated English vocabulary learning. In 2015 2nd International Conference on Information Science and Security (ICISS). 1–4. |
[15] |
Chen YL (2016) The effects of virtual reality learning environment on student cognitive and linguistic development. The Asia-Pacific Education Researcher 25: 637–646. doi: 10.1007/s40299-016-0293-2
![]() |
[16] | Chen SY, Hung CY, Chang YC, et al. (2018) A study on integrating augmented reality technology and game-based learning model to improve motivation and effectiveness of learning English vocabulary. In 2018 1st International Cognitive Cities Conference (IC3). 24–27. |
[17] | Chen MP, Wang LC, Zou D, et al. (2020) Effects of captions and English proficiency on learning effectiveness, motivation and attitude in augmented-reality-enhanced theme-based contextualized EFL learning. Computer Assisted Language Learning, 1–31. |
[18] | Chen MRA, Hwang GJ (2020) Effects of experiencing authentic contexts on English speaking performances, anxiety and motivation of EFL students with different cognitive styles. Interactive Learning Environments, 1–21. |
[19] |
Chen Y, Smith TJ, York CS, et al. (2020) Google Earth Virtual Reality and expository writing for young English Learners from a Funds of Knowledge perspective. Computer Assisted Language Learning 33: 1–25. doi: 10.1080/09588221.2018.1544151
![]() |
[20] |
Chen CH (2020) AR videos as scaffolding to foster students' learning achievements and motivation in EFL learning. British Journal of Educational Technology 51: 657–672. doi: 10.1111/bjet.12902
![]() |
[21] | Chew SW, Jhu JY, Chen NS (2018) The effect of learning English idioms using scaffolding strategy through situated learning supported by augmented reality. In 2018 IEEE 18th International Conference on Advanced Learning Technologies (ICALT). 390–394. |
[22] | Chung LY (2011) Using avatars to enhance active learning: Integration of virtual reality tools into college English curriculum. In The 16th North-East Asia Symposium on Nano, Information Technology and Reliability. 29–33. |
[23] | Chung LY (2012) Virtual Reality in college English curriculum: Case study of integrating second life in freshman English course. In 2012 26th International Conference on Advanced Information Networking and Applications Workshops. 250–253. |
[24] |
Cooper C, Varley-Campbell J, Booth A, et al. (2018) Systematic review identifies six metrics and one method for assessing literature search effectiveness but no consensus on appropriate use. J clin epidemiol 99: 53–63. doi: 10.1016/j.jclinepi.2018.02.025
![]() |
[25] |
Cornillie F, Clarebout G, Desmet P (2012) Between learning and playing? Exploring learners' perceptions of corrective feedback in an immersive game for English pragmatics. ReCALL: Journal of Eurocall 24: 257–278. doi: 10.1017/S0958344012000146
![]() |
[26] | Dalim CSC, Dey A, Piumsomboon T, et al. (2016) TeachAR: An interactive augmented reality tool for teaching basic English to non-native children. In 2016 IEEE International Symposium on Mixed and Augmented Reality (ISMAR-Adjunct). 82–86. |
[27] | De Freitas S (2006) Learning in immersive worlds: A review of game-based learning. 2–60. |
[28] | De Grove F, Van Looy J, Courtois C (2010) Towards a serious game experience model: Validation, extension and adaptation of the GEQ for use in an educational context. In Playability and player experience. 10: 47–61. Breda University of Applied Sciences. |
[29] | Draxler F, Labrie A, Schmidt A, et al. (2020) Augmented reality to enable users in learning case grammar from their real-world interactions. In Proceedings of the 2020 CHI Conference on Human Factors in Computing Systems. 1–12. |
[30] |
Eisenmann M, Grimm N, Volkmann L (2010) 92. Teaching the new English cultures and literatures. English and American Studies in German 2009: 164–166. doi: 10.1515/9783484431225.164
![]() |
[31] | Eisenmann M (2019) Teaching English: Differentiation and Individualisation. utb GmbH. |
[32] |
Fast-Berglund Å, Gong LL (2018) Testing and validating extended reality (xR) technologies in manufacturing. Procedia Manuf 25: 31–38. doi: 10.1016/j.promfg.2018.06.054
![]() |
[33] |
Fernández SS, Pozzo MI (2017) Intercultural competence in synchronous communication between native and non-native speakers of Spanish. Language Learning in Higher Education 7: 109–135. doi: 10.1515/cercles-2017-0003
![]() |
[34] |
Garzon J, Acevedo J (2019) Meta-analysis of the impact of Augmented Reality on students' learning gains. Educational Research Review 27: 244–260. doi: 10.1016/j.edurev.2019.04.001
![]() |
[35] | Gelsomini M, Leonardi G, Garzotto F (2020) Embodied learning in immersive smart spaces. In Proceedings of the 2020 CHI Conference on Human Factors in Computing Systems. 1–14. |
[36] | Giraudeau P, Olry A, Roo JS, et al. (2019) CARDS: a mixed-reality system for collaborative learning at school. In Proceedings of the 2019 ACM International Conference on Interactive Surfaces and Spaces. 55–64. |
[37] | Grünewald A (2016) Üben und Übungen im Fremdsprachenunterricht. Üben und Übungen beim Fremdsprachenlernen: Perspektiven und Konzepte für Unterricht und Forschung. Arbeitspapiere der 36. Frühjahrskonferenz zur Erforschung des Fremdsprachenunterrichts, 84. |
[38] | Guillén-Nieto V, Aleson-Carbonell M (2012) Serious games and learning effectiveness: The case of It'sa Deal! Computers and Education 58: 435–448. |
[39] |
Hamilton D, McKechnie J, Edgerton E (2020) Immersive virtual reality as a pedagogical tool in education: a systematic literature review of quantitative learning outcomes and experimental design. Journal of Computer Education 8: 1–32. doi: 10.1007/s40692-020-00169-2
![]() |
[40] | Hammer M (2012) The intercultural development inventory: A new frontier in assessment and development of intercultural competence. Sterling, VA: Stylus Publishing. In M. Vande Berg, R. M. Paige, and K. H. Lou (Eds.). Student learning abroad, 115–136. |
[41] | Hao KC, Lee LC (2019) The development and evaluation of an educational game integrating augmented reality, ARCS model, and types of games for English experiment learning: an analysis of learning. Interactive Learning Environments, 1–14. |
[42] |
Hassani K, Nahvi A, Ahmadi A (2016) Design and implementation of an intelligent virtual environment for improving speaking and listening skills. Interactive Learning Environments 24: 252–271. doi: 10.1080/10494820.2013.846265
![]() |
[43] | He J, Ren J, Zhu G, et al. (2014) Mobile-based AR application helps to promote EFL children's vocabulary study. In 2014 IEEE 14th International Conference on Advanced Learning Technologies. 431–433. |
[44] |
Herrera F, Bailenson J, Weisz E, et al. (2018) Building long-term empathy: A large-scale comparison of traditional and virtual reality perspective-taking. PLOS ONE 13: e0204494. doi: 10.1371/journal.pone.0204494
![]() |
[45] | Ho SC, Hsieh SW, Sun PC, et al. (2017) To activate English learning: Listen and speak in real life context with an AR featured u-learning system. Journal of Educational Technology and Society 20: 176–187. |
[46] | Hsieh M (2016) Development and evaluation of a mobile AR assisted learning system for English learning. 2016 International Conference on Applied System Innovation (ICASI), Okinawa, 1-4. |
[47] | Hsieh MC (2016) Teachers' and students' perceptions toward augmented reality materials. In 2016 5th IIAI International Congress on Advanced Applied Informatics (IIAI-AAI). 1180–1181. |
[48] |
Hsu TC (2019) Effects of gender and different augmented reality learning systems on English vocabulary learning of elementary school students. Universal Access in the Information Society 18: 315–325. doi: 10.1007/s10209-017-0593-1
![]() |
[49] | Huang X, Han G, He J, et al. (2018) Design and Application of a VR English Learning Game Based on the APT Model. In 2018 Seventh International Conference of Educational Innovation through Technology (EITT). 68–72. |
[50] |
Hung HC, Young SSC (2015) An investigation of game-embedded handheld devices to enhance English learning. Journal of Educational Computing Research 52: 548–567. doi: 10.1177/0735633115571922
![]() |
[51] |
Ibrahim A, Huynh B, Downey J, et al. (2018) Arbis pictus: A study of vocabulary learning with augmented reality. IEEE T Vis Comput Gr 24: 2867–2874. doi: 10.1109/TVCG.2018.2868568
![]() |
[52] | Ji S, Li K, Zou L (2019) The Effect of 360-Degree Video Authentic Materials on EFL Learners' Listening Comprehension. In 2019 International Joint Conference on Information, Media and Engineering (IJCIME). 288–293. |
[53] |
Johnson-Glenberg MC, Birchfield DA, Tolentino L, et al. (2014) Collaborative embodied learning in mixed reality motion-capture environments: Two science studies. Journal of Educational Psychology 106: 86. doi: 10.1037/a0034008
![]() |
[54] | Khatoony S (2019) An Innovative Teaching with Serious Games through Virtual Reality Assisted Language Learning. In 2019 International Serious Games Symposium (ISGS). 100–108. |
[55] | Kincheloe Joe L (2008) Critical Pedagogy Primer 2nd edition. English: New York, NY: Peter Lang. |
[56] | Küçük S, Yylmaz RM, Göktap Y (2014) Augmented reality for learning English: Achievement, attitude and cognitive load levels of students. Education and Science/Egitim ve Bilim 39. |
[57] | Küster L (2014) Zur Einführung in den Themenschwerpunkt. Fremdsprachen lehren und lernen 43: 2. |
[58] | Lan YJ (2015) Contextual EFL learning in a 3D virtual environment. Language Learning and Technology 19: 16–31. |
[59] |
Lee K, Kweon SO, Lee S, et al. (2014) POSTECH immersive English study (POMY): Dialog-based language learning game. IEICE T Inf Syst 97: 1830–1841. doi: 10.1587/transinf.E97.D.1830
![]() |
[60] |
Lee SM, Park M (2020) Reconceptualization of the context in language learning with a location-based AR app. Computer Assisted Language Learning 33: 936–959. doi: 10.1080/09588221.2019.1602545
![]() |
[61] | Leyva F, Plummer CJ (2015) National Institute for Health and Care Excellence 2014 guidance on cardiac implantable electronic devices: health economics reloaded. |
[62] | Li KC, Tsai CW, Chen CT, et al. (2015) The design of immersive English learning environment using augmented reality. In 2015 8th International Conference on Ubi-Media Computing (UMEDIA). 174-179. |
[63] | Liaw ML (2019) EFL learners' intercultural communication in an open social virtual environment. Journal of Educational Technology and Society 22: 38–55. |
[64] |
Liou HC (2012) The roles of Second Life in a college computer-assisted language learning (CALL) course in Taiwan, ROC. Computer Assisted Language Learning 25: 365–382. doi: 10.1080/09588221.2011.597766
![]() |
[65] |
Liu IF, Chen MC, Sun YS, et al. (2010) Extending the TAM model to explore the factors that affect intention to use an online learning community. Computers and education 54: 600–610. doi: 10.1016/j.compedu.2009.09.009
![]() |
[66] | Liu E, Liu C, Yang Y, et al. (2018) Design and implementation of an augmented reality application with an English Learning Lesson. In 2018 IEEE International Conference on Teaching, Assessment, and Learning for Engineering (TALE) 494–499. |
[67] | Lorenzo CM, Lezcano L, Alonso SS (2013) Language Learning in Educational Virtual Worlds-a TAM Based Assessment. J UCS 19: 1615–1637. |
[68] | Matveev AV (2002) The perception of intercultural communication competence by American and Russian managers with experience on multicultural teams (Doctoral dissertation, Ohio University). |
[69] | Milgram P, Kishino F (1994) A taxonomy of mixed reality visual displays. IEICE T Inf Syst 77: 1321–1329. |
[70] |
Moher D, Liberati A, Tetzlaff J, et al. (2011) Bevorzugte Report Items für systematische Übersichten und Meta-Analysen: Das PRISMA-Statement. DMW-Deutsche Medizinische Wochenschrift 136: e9–e15. doi: 10.1055/s-0031-1272982
![]() |
[71] |
Neumeier P (2005) A closer look at blended learning–parameters for designing a blended learning environment for language teaching and learning. ReCALL: the Journal of EUROCALL 17: 163. doi: 10.1017/S0958344005000224
![]() |
[72] |
Oberdörfer S, Latoschik ME (2019) Predicting learning effects of computer games using the Gamified Knowledge Encoding Model. Entertain Comput 32: 100315. doi: 10.1016/j.entcom.2019.100315
![]() |
[73] | Oberdörfer S, Elsässer A, Schraudt D, et al. (2020) Horst-The teaching frog: learning the anatomy of a frog using tangible AR. In Proceedings of the Conference on Mensch und Computer. 303–307. |
[74] |
Peck T, Seinfeld S, Aglioti S, et al. (2013) Putting yourself in the skin of a black avatar reduces implicit racial bias. Consciousness and cognition 22: 779–787. doi: 10.1016/j.concog.2013.04.016
![]() |
[75] |
Qu C, Ling Y, Heynderickx I, et al. (2015) Virtual bystanders in a language lesson: examining the effect of social evaluation, vicarious experience, cognitive consistency and praising on students' beliefs, self-efficacy and anxiety in a virtual reality environment. PloS one 10: e0125279. doi: 10.1371/journal.pone.0125279
![]() |
[76] | Quintín E, Sanz C, Zangara A (2016) The impact of role-playing games through Second Life on the oral practice of linguistic and discursive sub-competences in English. In 2016 International Conference on Collaboration Technologies and Systems (CTS). 148–155. |
[77] |
Ratan R, Beyea D, Li B, et al. (2020) Avatar characteristics induce 'users' behavioral conformity with small-to-medium effect sizes: A meta-analysis of the proteus effect. Media Psychology 23: 651–675. doi: 10.1080/15213269.2019.1623698
![]() |
[78] |
Redondo B, Cózar-Gutiérrez R, González-Calero JA, et al. (2020) Integration of augmented reality in the teaching of English as a foreign language in early childhood education. Early Childhood Education Journal 48: 147–155. doi: 10.1007/s10643-019-00999-5
![]() |
[79] | Ripka G, Grafe S, Latoschik ME (2020) Preservice Teachers' encounter with Social VR–Exploring Virtual Teaching and Learning Processes in Initial Teacher Education. In SITE Interactive Conference. 549–562. Association for the Advancement of Computing in Education (AACE). |
[80] | Sherman WR, Craig AB (2003) Understanding virtual reality. San Francisco, CA: Morgan Kauffman. |
[81] | Shih YC, Yang MT (2008) A collaborative virtual environment for situated language learning using VEC3D. Educational Technology and Society 11: 56–68. |
[82] |
Shih YC (2015) A virtual walk through London: Culture learning through a cultural immersion experience. Computer Assisted Language Learning 28: 407–428. doi: 10.1080/09588221.2013.851703
![]() |
[83] | Skarbez R, Frederick PB, Mary CW (2018) Immersion and Coherence in a Stressful Virtual Environment. In Proceedings of the 24th Acm Symposium on Virtual Reality Software and Technology. 1–11. |
[84] |
Slater M, Wilbur S (1997) A framework for immersive virtual environments (FIVE): Speculations on the role of presence in virtual environments. Presence: Teleoperators and Virtual Environments 6: 603–616. doi: 10.1162/pres.1997.6.6.603
![]() |
[85] |
Slater M (1999) Measuring presence: A response to the Witmer and Singer presence questionnaire. Presence 8: 560–565. doi: 10.1162/105474699566477
![]() |
[86] | Slater M (2003) A note on presence terminology. Presence connect 3: 1–5. |
[87] |
Sanchez-Vives MV, Mel S (2005) From presence to consciousness through virtual reality. Nat Rev Neurosci 6: 332–339. doi: 10.1038/nrn1651
![]() |
[88] |
Slater M (2009) Place illusion and plausibility can lead to realistic behaviour in immersive virtual environments. Philos T R Soc B 364: 3549–3557. doi: 10.1098/rstb.2009.0138
![]() |
[89] | Tulodziecki G, Grafe S (2012) Approaches to learning with media and media literacy education–trends and current situation in Germany. Journal of Media Literacy Education 4: 5. |
[90] | Tulodziecki G, Grafe S, Herzig B (2019) Medienbildung in Schule und Unterricht: Grundlagen und Beispiele. UTB GmbH. |
[91] | Vate-U-Lan P (2012) An augmented reality 3d pop-up book: the development of a multimedia project for English language teaching. In 2012 IEEE International Conference on Multimedia and Expo. 890–895. |
[92] | Vedadi S, Abdullah ZB, Cheok AD (2019) The Effects of Multi-Sensory Augmented Reality on Students' Motivation in English Language Learning. In 2019 IEEE Global Engineering Education Conference (EDUCON). 1079–1086. |
[93] |
Wang CX, Calandra B, Hibbard ST, et al. (2012) Learning effects of an experimental EFL program in Second Life. Educational Technology Research and Development 60: 943–961. doi: 10.1007/s11423-012-9259-0
![]() |
[94] |
Wang YF, Petrina S, Feng F (2017) VILLAGE—V irtual I mmersive L anguage L earning and G aming E nvironment: Immersion and presence. British Journal of Educational Technology 48: 431–450. doi: 10.1111/bjet.12388
![]() |
[95] |
Wienrich C, Johanna G (2020) AppRaiseVR–An Evaluation Framework for Immersive Experiences. I-Com 19: 103–121. doi: 10.1515/icom-2020-0008
![]() |
[96] | Wienrich C, Döllinger NI, Hein R (2020) Mind the Gap: A Framework (BehaveFIT) Guiding The Use of Immersive Technologies in Behavior Change Processes. arXiv preprint arXiv: 2012.10912. |
[97] | Wienrich C, Eisenmann M, Latoschik ME, et al. (2020) CoTeach - Connected Teacher Education. VRinSight Greenpaper 53–55. |
[98] |
Witmer BG, Singer MJ (1998) Measuring presence in virtual environments: A presence questionnaire. Presence 7: 225–240. doi: 10.1162/105474698565686
![]() |
[99] |
Wohlgenannt I, Simons A, Stieglitz S (2020) Virtual Reality. Business and Information Systems Engineering 5: 455–461. doi: 10.1007/s12599-020-00658-9
![]() |
[100] | Wu MH (2019) The applications and effects of learning English through augmented reality: A case study of Pokémon go. Computer Assisted Language Learning, 1–35. |
[101] |
Yang MT, Liao WC (2014) Computer-assisted culture learning in an online augmented reality environment based on free-hand gesture interaction. IEEE T Learn Technol 7: 107–117. doi: 10.1109/TLT.2014.2307297
![]() |
[102] | Yeh HC, Tseng SS, Heng L (2020) Enhancing EFL students' intracultural learning through virtual reality. Interactive Learning Environments, 1–10. |
[103] |
Zhang X, Zhou M (2019) Interventions to promote 'learners' intercultural competence: A meta-analysis. International Journal of Intercultural Relations 71: 31–47. doi: 10.1016/j.ijintrel.2019.04.006
![]() |
1. | Ping Wang, Changgui Gu, Huijie Yang, Haiying Wang, Identify causality by multi-scale structural complexity, 2024, 633, 03784371, 129398, 10.1016/j.physa.2023.129398 |
(the type of gold's influence on the dollar) | The second letter (the strength of gold's influence on the dollar) | The third letter (the type of dollar's influence on the gold) | The fourth letter (the strength of dollar's influence on the gold) |
P | a, (0.8, 1] | P | a, (0.8, 1] |
b, (0.6, 0.8] | b, (0.6, 0.8] | ||
c, (0.4, 0.6] | c, (0.8, 0.6] | ||
d, (0.2, 0.4] | d, (0.8, 0.4] | ||
e, [0, 0.2] | e, [0, 0.2] | ||
N | a, (0.8, 1] | N | a, (0.8, 1] |
b, (0.6, 0.8] | b, (0.6, 0.8] | ||
c, (0.4, 0.6] | c, (0.8, 0.6] | ||
d, (0.2, 0.4] | d, (0.8, 0.4] | ||
e, [0, 0.2] | e, [0, 0.2] | ||
D | a, (0.8, 1] | D | a, (0.8, 1] |
b, (0.6, 0.8] | b, (0.6, 0.8] | ||
c, (0.4, 0.6] | c, (0.8, 0.6] | ||
d, (0.2, 0.4] | d, (0.8, 0.4] | ||
e, [0, 0.2] | e, [0, 0.2] |
(the type of gold's influence on the dollar) | The second letter (the strength of gold's influence on the dollar) | The third letter (the type of dollar's influence on the gold) | The fourth letter (the strength of dollar's influence on the gold) |
P | a, (0.8, 1] | P | a, (0.8, 1] |
b, (0.6, 0.8] | b, (0.6, 0.8] | ||
c, (0.4, 0.6] | c, (0.8, 0.6] | ||
d, (0.2, 0.4] | d, (0.8, 0.4] | ||
e, [0, 0.2] | e, [0, 0.2] | ||
N | a, (0.8, 1] | N | a, (0.8, 1] |
b, (0.6, 0.8] | b, (0.6, 0.8] | ||
c, (0.4, 0.6] | c, (0.8, 0.6] | ||
d, (0.2, 0.4] | d, (0.8, 0.4] | ||
e, [0, 0.2] | e, [0, 0.2] | ||
D | a, (0.8, 1] | D | a, (0.8, 1] |
b, (0.6, 0.8] | b, (0.6, 0.8] | ||
c, (0.4, 0.6] | c, (0.8, 0.6] | ||
d, (0.2, 0.4] | d, (0.8, 0.4] | ||
e, [0, 0.2] | e, [0, 0.2] |