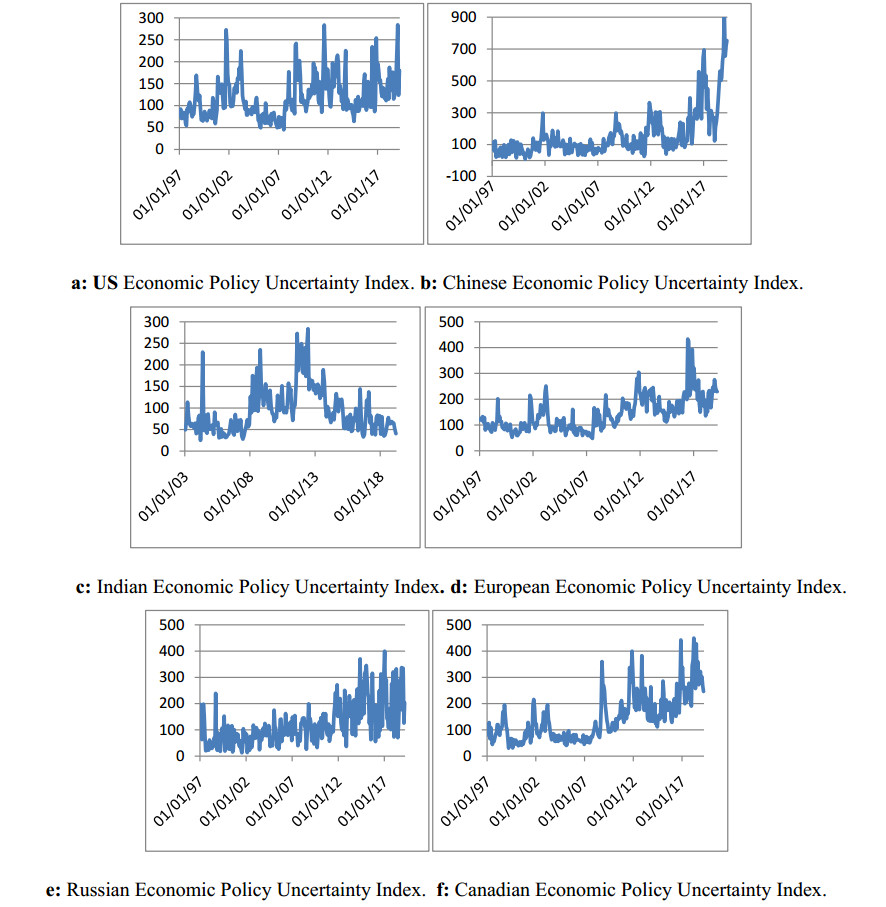
Citation: Md Akther Uddin, Mohammad Enamul Hoque, Md Hakim Ali. International economic policy uncertainty and stock market returns of Bangladesh: evidence from linear and nonlinear model[J]. Quantitative Finance and Economics, 2020, 4(2): 236-251. doi: 10.3934/QFE.2020011
[1] | Thomas C. Chiang . Economic policy uncertainty and stock returns—evidence from the Japanese market. Quantitative Finance and Economics, 2020, 4(3): 430-458. doi: 10.3934/QFE.2020020 |
[2] | Arifenur Güngör, Hüseyin Taştan . On macroeconomic determinants of co-movements among international stock markets: evidence from DCC-MIDAS approach. Quantitative Finance and Economics, 2021, 5(1): 19-39. doi: 10.3934/QFE.2021002 |
[3] | Fisnik Morina, Simon Grima . The impact of pension fund assets on economic growth in transition countries, emerging economies, and developed countries. Quantitative Finance and Economics, 2022, 6(3): 459-504. doi: 10.3934/QFE.2022020 |
[4] | David G. McMillan . The information content of the stock and bond return correlation. Quantitative Finance and Economics, 2018, 2(3): 757-775. doi: 10.3934/QFE.2018.3.757 |
[5] | Simiso Msomi, Damien Kunjal . Industry-specific effects of economic policy uncertainty on stock market volatility: A GARCH-MIDAS approach. Quantitative Finance and Economics, 2024, 8(3): 532-545. doi: 10.3934/QFE.2024020 |
[6] | Korhan Gokmenoglu, Baris Memduh Eren, Siamand Hesami . Exchange rates and stock markets in emerging economies: new evidence using the Quantile-on-Quantile approach. Quantitative Finance and Economics, 2021, 5(1): 94-110. doi: 10.3934/QFE.2021005 |
[7] | Thomas C. Chiang . Stock returns and inflation expectations: Evidence from 20 major countries. Quantitative Finance and Economics, 2023, 7(4): 538-568. doi: 10.3934/QFE.2023027 |
[8] | Mohsen Bahmani-Oskooee, Sujata Saha . On the effects of policy uncertainty on stock prices: an asymmetric analysis. Quantitative Finance and Economics, 2019, 3(2): 412-424. doi: 10.3934/QFE.2019.2.412 |
[9] | Albert A. Agyemang-Badu, Fernando Gallardo Olmedo, José María Mella Márquez . Conditional macroeconomic and stock market volatility under regime switching: Empirical evidence from Africa. Quantitative Finance and Economics, 2024, 8(2): 255-285. doi: 10.3934/QFE.2024010 |
[10] | James P. Gander . Market Value Volatility and the Volume of Traded Stock for U.S. Industrial Corporations. Quantitative Finance and Economics, 2017, 1(4): 403-410. doi: 10.3934/QFE.2017.4.403 |
Economic policy uncertainty (EPU) refers to the non-zero probability of changes in the existing economic policies that determine the rules of the game for economic agents (Baker et al., 2014). Theoretically, it is postulated that economic policy uncertainty affects equity market returns (Pastor and Veronesi, 2012). Baker et al. (2016) empirically argued that there exists a significant dynamic relationship among EPU, real macroeconomic variables, and stock markets. Economic policy uncertainty affects stock market return since it appears to be both push and pull factors to affect capital inflows and outflows in the cross-border countries (Julio & Yook, 2016; Choi & Furceri, 2019). Cross-border capital flows are influenced by market size of source and destination, existing technology in the market, distance and demography and trading cost (Portes & Rey, 2005). Capital flows, asset prices, credit growth and stock market volatility move together (Rey, 2015). More specifically, economic policy uncertainties in big bilateral trade partners create huge capital inflow and outflow that could cause financial instabilities in the host economy (Julio & Yook, 2016). Moreover, psychologically, stock market returns respond under or overreaction to good or bad news caused by policy uncertainty (Barberis et al., 1998).
From the empirical side, Arouri et al. 2016 found that increased in policy uncertainty reduced significantly stock returns and this effect is stronger and persistent during extreme volatility periods. Consequently, Phan et al. (2018) discuss the ability of economic policy uncertainty on the stock market return from a global perspective and document the effect to be asymmetric. It is argued that the stock market return varies with the economic integration leading to close economic ties (Guo et al., 2018). Hence, crisis and policy uncertainty turn out to be equity market contagion and their gravity of that depends on economic fundamentals (Bekaert et al., 2014) as well as economic ties. More recently, Bahmani-Oskooee and Saha (2019a) found that increased uncertainty has adverse short-run effects but not long-run effects on stock prices. However, later they also found long-run effects by using non-linear estimation (Bahmani-Oskooee and Saha, 2019b).
Li et al. (2016) found weak relationship between EPU and stock market of China and India. The effect of US economic policy uncertainty on Chinese and Indian stock market is weak in the short-run but gradually become stronger in the long run (Li et al., 2020). Alqahtani & Martinez (2020) found Global and US EPU have significant negative effect on stock price in Bahrain and Kuwait but not on other GCC countries. Tsai (2017) concluded that US EPU is less influential than China and Chinese EPU has become the most influential and its contagion risk spread to different regional markets except for Europe. The existing empirical works of literature of EPU focus largely on advanced economies and BRICs countries. Moreover, there are only a few studies which study the effect of EPU on developing or emerging equity markets in the context of increasing global trade linkage. On this backdrop, we have taken Bangladesh as a case study. Bangladesh, a member of next eleven (N-11) countries, is one of the fastest growing economies with above average growth of six percent for the last two decades. Bangladesh’s equity market liberalization1 has begun at the beginning of the 90s (Bekaert et al., 2003). Its economy has been gradually integrating with the global economy through trade liberalization (Manni & Afzal, 2012). Therefore, economic policy uncertainty in big bilateral trade partners could spill over to the Bangladesh economy and the stock market. A recent demutualization of the premier bourse of the country has brought new perspectives and challenges on stock market development (Islam and Islam, 2011; Mahmud, 2019).
1 Purchases of Bangladesh shares and securities by nonresidents, including nonresident Bangladeshis, in stock exchange in Bangladesh were allowed, subject to meeting procedural requirements
Therefore, the main aim of our paper is to extend the empirical work of Arouri et al. (2016), Tsai (2017) and Bahmani-Oskooee and Saha’s (2019b). We hypothesize that EPU from major trading partners (exporters and importers) would affect stock market homogeneously. Moreover, we study whether affect of EPU on stock market is regime dependent. Consequently, we examine the impact of economic policy uncertainty of US, Canada, EU, Russia, China, and India on the stock market return of Bangladesh by using time and state varying linear and non-linear econometric techniques.
The rest of the paper is organized as follows: The next section deals with the data, models and methodology. This is followed by a presentation of results and analysis. The final section provides concluding remarks and policy implications.
This study has selected S & P Bangladesh BMI—Price Index to capture monthly changes in Bangladesh stock market return, and the data related to that has been extracted from the DataStream2. This study has selected the economic policy uncertainty3 index of US, China, India, EU, Russia, and Canada as these countries are the biggest trading partners4 of Bangladesh. The economic policy uncertainty indices of trading partners are from the website of policy uncertainty https://www.policyuncertainty.com/, the indices are formed on assumptions of Baker et al. (2016) and collected data from DataStream.
2 We could have used Bangladesh Broad Index or DSE Broad index but du shorter time series we had to exclude these series.
3 Baker et al. (2016) develop policy-related economic uncertainty index based on newspaper coverage frequency. This index capture uncertainty about who will make economic policy decisions, what economic policy actions will be undertaken and when, and the economic effects of policy actions(or inaction)—including uncertainties related to the economic ramifications of “non-economic” policy matters, e.g., military actions. Our measures capture both near-term concerns (e.g., when will the Fed adjust its policy rate) and longer-term concerns (e.g., how to fund entitlement programs), as reflected in newspaper articles.
4 China and India are the largest import partners while the US and EU are the largest export partners of Bangladesh. Canada and Russia have enjoyed more balanced trade position with Bangladesh.
The sample of this study covers monthly data from the period of January 2003 to April 2019. The fact of starting from January 2003 is that the Indian economic policy uncertainty index starts from January 2003. Henceforth, for keeping the same data span, this study had to employ monthly data from January 2003 to April 2019.
We adopt the factor model approach to capture the effects of international economic policy uncertainties on stock market returns of Bangladesh, which shown in Equation 1. The current factor model is supported by the international capital asset pricing model. In addition, there are several empirical studies also have employed multifactor type models to investigate the effects of EPU on stock returns (e.g., Arouri et al., 2016; Hoque et al., 2019). Henceforth, the current factor model can be used as a baseline model for time-varying linear and nonlinear models.
Rt=αt+∅0Rt−i+∅1ΔCH+∅2ΔCHt−i+∅3ΔCNt+∅4ΔCNt−i+∅5ΔEUt+∅6ΔEUt−i+∅7ΔINt+∅8ΔINt−i+∅9ΔRSt+∅10ΔRSt−i+∅11ΔUSt+∅12ΔUSt−i+εt;εt→N(0,σ2t)t=1,⋯,T. | (1) |
where, Rt denotes stock market returns. t-i represents lag point up to the ith period, ∆CH, ∆CN, ∆EU, ∆IN, ∆RS, and ∆US change in economic policy uncertainty of China, Canada, European Union, India, Russia, and the United States, respectively. εt denotes error term. Lag will be selected based on AIC and SIC criteria.
The stock market evolves with time. Therefore, the structural changes may occur in the market with policy changes, economic boost, and political regime. Henceforth, the structural changes of financial markets have promoted to re-specify Equation (1) with the presence of structural breaks. This study adopts the approach Bai and Perron(1998, 2003). This approach allows testing for multiple structural breaks in a linear model and can detect breaks at a priori unknown date. Additionally, this approach is able to take care of heterogeneity within the return distribution. Hence, in the following Equation (2) modeled with m breaks (m + 1 regimes).
Rt=αj+∅0,jRt−i+∅1,jΔCH+∅2,jΔCHt−i+∅3,jΔCNt+∅4,jΔCNt−i+∅5,jΔEUt+∅6,jΔEUt−i+∅7,jΔINt+∅8,jΔINt−i+∅9,jΔRSt+∅10,jΔRSt−i+∅11,jΔUSt+∅12,jΔUSt−i+εt;εt→N(0,σ2t)t=1,…,T. | (2) |
where, j = 1, …….., m+1. J and T are the segment index and total sample size, respectively. The breakpoints (T1……Tm) are treated as unknown and by convention T0 = 0 Tm+1 = T. All other specifications are the same as in the specification Equation (1).
Andrews (1993) and Andrews and Ploberger (1994) have designed F-statistic for selecting a specific alternative and testing against the null hypothesis of one break with unknown timing. With breakpoint i, this study needs to compare OLS residuals ˆei of regression among each subsample and OLS residuals ˆei of each subsample with whole e sample, which is presented below:
Fi=ˆeTˆe−ˆeTiˆeiˆeTiˆei/(n−2k)i=nh,…,nh(nh≥k) | (3) |
Bai and Perron(1998, 2003) have extended this given method to test 0 break against L break and L+1 break. Before confirming the of breaks, Bai and Perron(1998, 2003) recommend that UDmax and WDmax tests should be done to confirm at one break exists in the relationships. Henceforth, sequential estimation of SUP FT = (L+1)/L statistics should be done to select appropriate numbers of breaks.
We adopt the Markov regime-switching model of Hamilton’s(1989, 2010) for estimating the regime varying effects of international economic policy uncertainties on stock market returns. This approach is one of the most prevalent non-linear time series models which allows and captures time-varying effects of exogenous factors across volatility regimes5. The study considers the influences of transition variables on stock returns is state (st) dependent. Henceforth, as shown below, Equation (1) is re-formulated within Markov switching framework.
5 See Hamilton(1989, 2010), Janina et al. (2018) and Hoque et al. (2019) for details of markov switching regression and estimation approach.
Rt,st=αj+∅0,stRt−i+∅1,stΔCH+∅2,stΔCHt−i+∅3,stΔCNt+∅4,stΔCNt−i+∅5,stΔEUt+∅6,stΔEUt−i+∅7,stΔINt+∅8,stΔINt−i+∅9,stΔRSt+∅10,stΔRSt−i+∅11,stΔUSt+∅12,stΔUSt−i+εt;εt∼N(0,σ2s)t=1,…,T. | (4) |
wheres denotes regime states and all other specifications are the same as in specification Equation (1).
Figure 1 shows that economic policy uncertainty of China, Russia, and EU are highly volatile compare to other countries. Table 1 presents the summary statistics. It has been observed that returns and/or changes of variables are more or less normally distributed. Interestingly, the standard deviations indicate that economic policy uncertainty of China, Russia, Canada, and EU exhibits greater volatility than other economies in our sample. This might be resonated by the fact that Russia and China are having high growth prospectus uncertainty6 among the sample economies.
6 Please see the report to view the lowest to highest volatile economies.
RET | IN | EU | CN | CH | US | RS | |
Mean | −0.001 | 93.209 | 143.487 | 144.631 | 160.270 | 118.675 | 122.151 |
Median | 0.000 | 79.001 | 132.868 | 123.371 | 109.093 | 108.634 | 103.439 |
Maximum | 0.327 | 283.689 | 433.277 | 449.623 | 935.310 | 284.135 | 400.016 |
Minimum | −0.369 | 24.939 | 47.692 | 30.0970 | 9.066 | 44.782 | 12.3987 |
Std. Dev. | 0.0839 | 51.904 | 65.121 | 87.718 | 145.52 | 46.130 | 79.135 |
Skewness | −0.574 | 1.289 | 1.1642 | 1.0468 | 2.295 | 1.074 | 1.0872 |
Kurtosis | 7.590 | 4.577 | 5.140 | 3.657 | 9.0371 | 4.114 | 3.790 |
Jarque-Bera | 250.059 | 74.660 | 111.694 | 53.775 | 642.315 | 65.447 | 59.779 |
Probability | 0.000 | 0.000 | 0.000 | 0.000 | 0.000 | 0.000 | 0.000 |
In order to have a preliminary understanding, this study performed a pair-wise Pearson correlation test and the results are presented in Table 2. This study has observed that economic policy uncertainties of selected economies are having a positive relationship, suggesting one economy’s policy uncertainty may create policy uncertainty in other economies or they may follow the same direction, this can be due to the level of trade openness, economic and political tie with one another country. We have also looked at the magnitude of the relationship among the independent variables of the study. We find that EU and Canadian economic policy uncertainty has the highest correlation of r = 0.7837 among others, which is lower than the cut-value of r = 0.90. So, it can be said that the model may not suffer due to statistical issues. Hence, we can proceed to the estimation stage.
RET | US | EU | CN | RS | CH | IN | |
RET | 1 | ||||||
US | 0.4605 | 1 | |||||
EU | 0.5470 | 0.7168 | 1 | ||||
CN | −0.5993 | 0.6996 | 0.7837 | 1 | |||
RS | −0.3904 | 0.2709 | 0.4675 | 0.4763 | 1 | ||
CH | −0.4109 | 0.5077 | 0.6840 | 0.6780 | 0.4536 | 1 | |
IN | −0.3177 | 0.4333 | 0.3311 | 0.3165 | 0.1509 | 0.0456 | 1 |
The study has employed PP and ADF unit-root testing approach and the results are presented in Table 3. The unit root tests show that some variables are not stationary in level from but all are stationary in the first difference level. That is consistent with the empirical assumptions and empirical model of the study. Afterward, this study has performed the BDS test of Brock et al. (1996) to examine patterns and non-linearity in the stock market returns of Bangladesh, which presented in Table 4. The findings imply that there is two or more embedded dimension in the stock market returns movements which is consistent with the earlier studies of Anagnostidis and Emmanouilides (2015) & Apergis et al. (2018). Hence, the model with structural breaks and time-varying setting could capture a clearer picture of the relationship. Therefore, in this study, we have employed break least square and Markov switching regression approach to examine the international economic policy uncertainty on stock market returns of Bangladesh. The results of break least square and Markov switching regression are reported and discussed in the following.
Panel A: PP | |||||||
Panel A1:At Level | |||||||
RET | CH | CN | EU | IN | RS | US | |
With Constant | −16.408*** | −3.231** | −3.954*** | −4.666*** | −5.831*** | −10.002*** | −7.086*** |
With Constant & Trend | −16.471*** | −5.410*** | −6.749*** | −7.041*** | −5.824*** | −13.129*** | −7.718*** |
Without Constant & Trend | −16.431*** | −1.496 | −1.328 | −1.231 | −1.787* | −3.404*** | −1.825* |
Panel A2: At First Difference | |||||||
With Constant | −56.398*** | −26.574*** | −36.112*** | −37.986*** | −30.069*** | −64.867*** | −46.03*** |
With Constant & Trend | −56.845*** | −27.946*** | −36.702*** | −38.243*** | −30.358*** | −65.553*** | −45.894*** |
Without Constant & Trend | −56.422*** | −25.849*** | −35.195*** | −37.188*** | −30.169*** | −64.374*** | −45.712*** |
Panel B:ADF | |||||||
Panel B1: At Level | |||||||
With Constant | −16.176*** | −0.387 | −2.155 | −2.518 | −2.681* | −0.768 | −2.57* |
With Constant & Trend | −16.320*** | −1.991 | −5.357*** | −4.046*** | −2.65 | −3.0479 | −3.24* |
Without Constant & Trend | −16.205*** | 0.6816 | 0.0621 | 0.0206 | −1.29* | 0.75 | −0.193 |
Panel B2: At First Difference | |||||||
With Constant | −9.153*** | −6.22*** | −8.029*** | −5.899*** | −17.042*** | −6.197*** | −8.963*** |
With Constant & Trend | −9.196*** | −6.359*** | −8.028*** | −5.886*** | −17.002*** | −6.18*** | −8.944*** |
Without Constant & Trend | −9.162*** | −6.083*** | −7.996*** | −5.871*** | −17.086*** | −6.084*** | −8.965*** |
Note: *, **, and *** denote statistical signifies at 10%, 5%, and 1% respectively. |
M | Ɛ(1) | Ɛ(2) | Ɛ(3) |
2 | 0.07*** | 0.123*** | 0.1169*** |
3 | 0.08*** | 0.174*** | 0.1671*** |
Note: *, **, and *** denote statistical signifies at 10%, 5%, and 1% respectively. |
We have employed the approach of Bai and Perron(1983, 2003) for detecting multiple structural breaks in the relationship between international economic policy uncertainty and stock market returns of Bangladesh. We have allowed a maximum of five breaks with a trimming parameter of 0.05. First, we have estimated the UDmax and WDmax tests(at the 5% level) for conforming at least one break that exists between international EPU and stock market returns. In the second step, we have estimated the sequential test supFT (L + 1/L) statistics for determining appropriate breaks in the nexus. The results of the multiple breaks in the relationship between international economic policy uncertainty and stock market returns of Bangladesh are presented in Table 5. The break test has detected four structural breaks in the relationships. To this end, several researchers documented more than one break in the stock prices, oil prices (Andreou and Ghysels, 2002; Arouri and Roubaud, 2016; Balcilar et al., 2016; Balcilar et al., 2017). The break dates/points are 2007M06, 2009M11, 2012M06, and 2016M12. Based on these points, this study has estimated the Equation (2) with the break least square regression and the results are presented in Table 6. The model has captured the 49.28% variation of stock market returns. The model is also significant with an F-statistic of 11.760 (p value = 0.0031). The post-estimation tests of LM and ARCH show that the estimated model is free from the serial-correlation and heteroscedasticity. Henceforth, we can proceed to interpret the estimated results.
udmax | wdmax | supr(0/1) | supr(1/2) | supr(2/3) | supr(3/4) | numbers of breaks select | optimal break | break date | |||
seq | bic | lwz | |||||||||
ret | 31.13** | 32.8*** | 28.92** | 56.90** | 31.711** | 31.71** | 4 | 0 | 0 | 4 | 2007m06, 2009m11, 2012m06, 2016m12 |
Note:** denotes statistical signifies at 5%. This table reports the results of the procedure developed by bai and perron(1998, 2003) to search endogenously for structural breaks. the effective sample size is 938. a maximum of five breaks are allowed and a trimming parameter (minimum size of a segment with respect to the sample size) of 0.15 is used, so each segment has at least 140 observations. the double maximum tests (udmax and wdmax) test the null of no structural breaks against the alternative of an unknown number of breaks. the supft (L + 1/L) is a sequential test of the null of l breaks versus the alternative of l + 1 breaks. sequential, bic and lwz denote the sequential procedure, Bayesian information criterion and information criterion suggested by liu et al. (1997), respectively. as usual *, ** and *** indicate statistical significance at 10%, 5% and 1% levels, respectively. |
Breaks | C | RET(-1) | CH | CH(-1) | CN | CN(-1) | EU | EU(-1) | IN | IN(-1) | RS | RS(-1) | US | US(-1) | |||
2003M02−2007M05 | Coefficients | 0.019 | −0.092 | 0 | −0.001 | −0.001 | 0 | 0 | −0.001 | 0 | −0.001 | 0.001 | 0 | 0 | 0.001 | R-squared | 0.4928 |
T-statistic | 0.283 | −0.795 | −0.608 | −1.99** | −1.419 | 0.685 | 0.747 | −1.013 | 0.617 | −3.37** | 1.376 | −1.416 | 0.34 | 1.332 | Adj R−squared | 0.2128 | |
2007M06−2009M10 | Coefficients | 0.297 | −0.314 | −0.001 | −0.001 | 0 | 0.001 | 0 | 0 | 0 | 0 | 0.001 | −0.001 | −0.001 | −0.001 | Log likelihood | 293.855 |
T-statistic | 2.349*** | −1.372 | −1.254 | −1.391 | 0.23 | 1.858* | 0.47 | −0.722 | −0.37 | −0.595 | 1.375 | −1.195 | −0.831 | −1.89 | F-statistic | 11.7601 | |
2009M11−2012M05 | Coefficients | 0.054 | −0.215 | 0.001 | −0.001 | −0.002 | 0.001 | 0.002 | −0.003 | 0 | −0.001 | 0 | 0.002 | 0.001 | 0 | Prob(F-statistic) | 0.0031 |
T-statistic | 0.811 | −2.10** | 3.56*** | −2.354*** | −6.59*** | 2.60** | 4.12*** | −4.06*** | −0.23 | −3.36*** | 0.22 | 4.11*** | 2.67*** | −1.24 | Prob(F-statistic) | 0.0031 | |
2012M06−2016M11 | Coefficients | 0.058 | −0.115 | 0 | 0 | 0 | 0 | 0 | 0 | 0 | 0 | 0 | 0 | 0 | 0 | Durbin-Watson stat | 1.998 |
T-statistic | 1.235 | −0.958 | 0.404 | −0.714 | −0.935 | 0.557 | 1.063 | −0.428 | −0.292 | 0.024 | 0.272 | −1.223 | −0.416 | −0.125 | LM test | 0.858 | |
2016M12−2019M04 | Coefficients | −0.135 | −0.039 | 0.002 | 0.0003 | 0.004 | −.001 | 0.0001 | 0.0003 | 0.009 | 0.0001 | 0.0002 | −0.001 | 0.0001 | 0.0001 | ARCH Test | 1.031 |
T-statistic | −2.148*** | −0.11 | 0.024 | −0.769 | 0.651 | −2.139** | 1.388 | −0.531 | 0.52 | 0.417 | 3.119*** | −1.964** | 1.656* | 2.101*** | |||
Note: *, **, and *** denote statistical signifies at 10%, 5%, and 1% respectively. |
During the time span starting from February 2003 to May 2007, the Bangladesh stock market was relatively stable and internal factors were mostly dominant in determining stock market return (Hassan & Chowdhury, 2008). Additionally, macroeconomic conditions were also conducive for the stock market. Most importantly, Bangladesh’s trade link with China and India grew significantly in this period7. Thus, Chinese economic policy uncertainty has lag negative significant effect on the stock market return and Indian economic policy uncertainty has a concurrent negative significant effect on stock market return. Bangladesh’s import dependence on China and India grew significantly in this period. Even though the US and EU are the largest markets for Bangladesh’s export mainly Ready-Made Garments (RMG) but we didn’t observe any significant policy uncertainty effect from them.
7 Please see the report of United Nations Conference on Trade and Development (UNCTAD).
In the second phase starting from June 2007 to October 2009, which covers the Global Financial Crisis (GFC). Political uncertainty had increased following the1/11 (One eleven8) event that took place in this period. In Bangladesh’s case, there were also drastic economic and financial policy changes in this period as the government was not elected and the investors feared to invest in that period. Thus, Bangladesh’s stock market was largely disintegrated with the world market hence not affected by the Global Financial Crisis (GFC) unfolding in the rest of the world. Exports, remittances, and imports are identified as key transmission channels for contagion. As the capital account has not been liberalized in Bangladesh, capital flows play less significant role (Murshid et al., 2009). Therefore, the empirical results are showing that international policy uncertainties had little or no additional effect on stock market return during the crisis period.
8 2006–2008 Bangladeshi political crisis is commonly known as One Eleven.
In the next time span of November 2009 to May 2012, we observed a bubble burst in the Bangladesh stock market. The effect spilled over to the real-estate sector and financial sector. It is well-documented that internal factors were mainly responsible for the crash (Rahman et al., 2017; Islam and Ahmed, 2015; Rahman, Hossain and Habibullah, 2017), but interestingly we found the significant effects of economic policy shock from major trading partners in this period. We have found mostly negative significant contemporaneous and lag effects of international economic policy uncertainties on stock market return during volatile period which is supported by (Arouri et al, 2017; Bahmani-Oskooee and Sujata 2019). Following the global financial crisis, the world experienced unprecedented fiscal and monetary policy measures and policy-related uncertainty was increasing in developed and developing countries (Blanchard et al., 2010). Interestingly, there is no significant economic policy uncertainty affecting the stock market during the Global Financial Crisis. However, after the Asian Financial Crisis foreign investors withdrew from the Bangladesh market in large numbers (Mollah, 2011). However, during post GFC Bangladesh's external trade increased significantly, and the stock market attracts international portfolio investment. Bangladesh became one of the largest RMG exporting countries in Europe and the US. China was gradually becoming one of the largest trading partners of Bangladesh9. Therefore, post-global financial crisis, the stock market was affected negatively.
9 Bangladesh’s trade deficit with China reached to USD 7.5 billion in 2012.
In the next phase (June 2012 to November 2016), no significant effect has been identified and the stock market was largely bearish in this time period. In addition to increasing political instability following the 2014 election, lack of investor confidence, and tight credit policy can be the reason behind it. Finally, the recent past consisting of the time frame from December 2016 to April 2019, the stock market has shown mixed performance. Although private sector investment growth is low, the economy is growing at a steady rate, this could be due to the rise of government investment expenditure. A great number of mega infrastructure projects have attracted foreign and local investment. In the meantime, China has become the largest trading partner10 of Bangladesh by beating India. While, Russia has come forward to invest and build the first nuclear power-plant in Bangladesh. Recent demutualization of the leading stock market of the country has brought a new perspective on stock market development. Bangladesh’s economy has gradually integrated with the global economy and the global economic policy uncertainty index reached to 311 in 2018 point from 155 points in 2016. Brexit, the sovereign debt crisis in Eurozone, financial instability in China, US-China trade war have contributed significantly to this increase in global economic policy uncertainty. However, only Russian and Canadain economic policy uncertainty impacts the stock market return significantly in this period. All in all, global economic policy uncertainty affect stock market returns of Bangladesh significantly negatively from November 2009 to May 2012 when the second stock market bubble burst.
10 Bangladesh’s trade deficit with China reached to USD 16.8 billion in 2018.
The prevalence of several volatility structures led us to examine the non-linear effect of the economic policy uncertainty on stock market returns using Markov Switching Regression. Based on the graphical presentation and BDS test, this study specifies the appearance of a two-regime model with low volatility and high volatility. The estimated results of the two-regime model are presented in Table 7. The finding indicates that volatility has a negative impact on stock returns regardless of their structure. However, high volatility has a greater negative influence on stock returns. Such findings are in line with market behavior, market theory and theory of economic policy uncertainty (Baker et al, 2016; Pastor and Veronesi, 2012) and also similar with previous studies by Basher et al., (2018) and Chung and Chuwonganant, (2018) Bahmani-Oskooee and Sujata (2019b); Arouri et al., (2016). The time varying transition parameter world-P11 found to be significant hence it confirms that the regime tends to change with development in the world economic policy uncertainties. Henceforth, the estimated results are generalizable about the Bangladesh stock market returns.
C | CH | CH(-1) | CN | CN(-1) | EU | EU(-1) | IN | IN(-1) | RS | RS(-1) | US | US(-1) | LOG(SIGMA) | ||||
Low Volatile Regime | Coefficients | 0.037 | 0 | 0.001 | 0.001 | 0.003 | 0.004 | 0.001 | 0.005 | 0.005 | 0.003 | 0.001 | 0.001 | 0.001 | −2.463 | P11-WORLD | −3.506** |
T-statistic | 1.097 | 1.706 | −2.116** | −1.612 | −0.401 | 0.159 | −0.171 | −1.429 | −0.133 | 1.051 | −0.376 | 0.945 | 0.713 | −32.40*** | P12-WORLD | 0.03 | |
High Volatile Regime | Coefficients | 0.024 | −0.02 | 0.007 | −0.065 | −0.001 | −0.054 | 0.001 | 0.004 | 0.003 | 0.024 | 0.002 | 0.001 | 0.001 | −3.348 | ||
T-statistic | 1.19 | −1.56 | 3.203*** | −0.7 | −0.01 | −1.98** | −1.396 | −2.1** | 2.54** | 1.143 | −2.5** | −0.527 | −1.355 | −33.34*** | |||
Note: *, **, and *** denote statistical signifies at 10%, 5%, and 1% respectively. |
In a low volatility regime, this study has not found any significant contemporaneous or lag effect of the international EPU on stock market returns, except for the Chinese EPU. The lagged effect of Chinese EPU is found to be negatively significant on DSE stock returns. However, in high volatility regimes, Chinese, Indian, European and Russian EPU have a significant negative lag effect on stock market return. The exposures are higher than those in the low volatility regime (Arouri et al., 2016). These findings are similar to Hoque & Zaidi (2019 and 2020) as they presented that the global economic policy uncertainty has greater impacts on stock returns in the high‐volatility regime, and the impacts also are time and regime varying.
Henceforth, the empirical results imply that the effects are time and volatile varying. One possible explanation for these findings could be that, during a high volatility period, external policy shocks could agammaavate the situation in addition to internal factors, and thus the market is highly volatile and exposed to external shocks. Additionally, the overall negative impacts of international EPU have further strengthened our findings from linear estimation. Bangladesh and China have developed a strong bilateral politico-economic relationship (Islam, 2012). In the last decade or so, Chinese economic policy uncertainty has become the most influential and its contagion risk spread to different regional markets (Tsai, 2017). However, India, the second largest trading partner followed by China and a giant neighbor, still plays a dominant role in politico-economic development of Bangladesh (Ullah & Uddin, 2018).
Bangladesh’s economy has been gradually integrating with the global economy through different linkages. In this way, economic policy uncertainty from major economies and trading partners could spill over to the Bangladesh stock market due to greater financial liberalization, regional and global integration (Bekaert et al., 2003). To this end, we study how global economic policy uncertainty affects the stock market return of Bangladesh. We have applied Break least square and Markov switching regression on monthly data covering from January 2003 to April 2019.
Our key findings are as follows. Firstly, we have identified four significant structural breaks in the stock market return series in our sample period. The premier bourse of the country has been turmoil and suffered from frequent major stock market crashes. This finding is corroborated by the existing literature (Basher et al., 2007; Banerjee et al., 2017). Secondly, our findings also confirm that EPU from major trading partners (exporters and importers) would not homogeneously affect stock market returns of Bangladesh. As Bangladesh is a predominantly import dependent country, economic policy uncertainty from major importing countries (China and India) affects stock market return more significantly than major exporting countries (US and EU). Alqhatani & Martinez (2020) also did not find significant effect of Global and US EPU on many GCC countries. Thirdly, Indian and Chinese policy shocks tend to significantly affect stock market return negatively during high volatility regime (stock market crash from 2010 to 2012). Tsai (2017) argued that the US EPU effect is less influential than Chinese EPU and China spreads contagious risk to different regional markets. Moreover, China has become more influential in several key international markets, namely, stock, credit, energy, and commodity markets (Zhang et al., 2019). Finally, our study found that the effect of EPU on stock market is regime dependent i.e., during high volatility EPU has a greater negative influence on stock returns than low volatility. Our findings are supported by Arouri et al. (2016) and Bahmani-Oskooee and Sujata (2019b).
Most importantly, from a policy point of view, policy makers, investors, fund and portfolio managers who have significant exposure in emerging markets like Bangladesh should closely follow the global EPU related events unfolding across the world and more importantly economic policy changes confronted by China and India. The financial contagion has become a widespread phenomenon in the post-global financial crisis. Therefore, future research could focus on how Global policy uncertainty can affect stock markets through macroeconomic channels like exchange rate shocks, remittance, FDI and commodity prices.
We would like to thank you three esteemed reviewers and the editor of QFE. The earlier version of the paper was presented in the 13th International Conference on Business Innovation for Inclusive Development (ICBIID 2019) and authors are thankful to the participants and reviewers for their valuable comments and suggestions.
We, hereby, declare that the submitted paper is not associated with any kind of conflict of interest.
[1] | Alqahtani A, Miguel M (2020) US Economic Policy Uncertainty and GCC Stock Market. Asia-Paci Financ Markets, 1-11. |
[2] |
Anagnostidis P, Emmanouilides CJ (2015) Nonlinearity in high-frequency stock returns: Evidence from the Athens Stock Exchange. Phys A 421: 473-487. doi: 10.1016/j.physa.2014.11.056
![]() |
[3] |
Andreou E, Ghysels E (2002) Detecting multiple breaks in financial market volatility dynamics. J Appl Econometrics 17: 579-600. doi: 10.1002/jae.684
![]() |
[4] |
Andrews DW, Ploberger W (1994) Optimal tests when a nuisance parameter is present only under the alternative. Econometrica J Econometric Society, 1383-1414. doi: 10.2307/2951753
![]() |
[5] |
Andrews DW (1993) Tests for parameter instability and structural change with unknown change point. Econometrica J Econometric Society, 821-856. doi: 10.2307/2951764
![]() |
[6] | Apergis N, Bonato M, Gupta R, et al. (2018) Does geopolitical risks predict stock returns and volatility of leading defense companies? Evidence from a nonparametric approach. Def Peace Econ 29: 684-696. |
[7] |
Arouri M, Estay C, Rault C, et al. (2016) Economic policy uncertainty and stock markets: Long-run evidence from the US. Financ Res Lett 18: 136-141. doi: 10.1016/j.frl.2016.04.011
![]() |
[8] | Arouri M, Roubaud D (2016) On the determinants of stock market dynamics in emerging countries: the role of economic policy uncertainty in China and India. Econ Bull 36: 760-770. |
[9] | Bahmani-Oskooee M, Saha S (2019) On the Effects of Policy Uncertainty on Stock Prices. J Econ Financ. [In press]. |
[10] |
Bahmani-Oskooee M, Sujata S (2019) On the effects of policy uncertainty on stock prices: an asymmetric analysis. Quant Financ Econ 3: 412-424. doi: 10.3934/QFE.2019.2.412
![]() |
[11] |
Bai J, Perron P (1998) Estimating and testing linear models with multiple structural changes. Econometrica, 47-78. doi: 10.2307/2998540
![]() |
[12] |
Bai J, Perron P (2003) Computation and analysis of multiple structural change models. J Appl Econometrics 18: 1-22. doi: 10.1002/jae.659
![]() |
[13] |
Baker SR, Bloom N, Canes-Wrone B, et al. (2014) Why has US policy uncertainty risen since 1960? Am Econ Rev 104: 56-60. doi: 10.1257/aer.104.5.56
![]() |
[14] |
Baker SR, Bloom N, Davis SJ (2016) Measuring economic policy uncertainty. Q J Econ 131: 1593-1636. doi: 10.1093/qje/qjw024
![]() |
[15] |
Balcilar M, Bekiros S, Gupta R (2017) The role of news-based uncertainty indices in predicting oil markets: a hybrid nonparametric quantile causality method. Empirica Econ 53: 879-889. doi: 10.1007/s00181-016-1150-0
![]() |
[16] | Balcilar M, Gupta R, Kyei C, et al. (2016) Does economic policy uncertainty predict exchange rate returns and volatility? Evidence from a nonparametric causality-in-quantiles test. Open Econ Rev 27: 229-250. |
[17] |
Banerjee PK, Ahmed MN, Hossain MM (2017) Bank, stock market and economic growth: Bangladesh perspective. J Dev Areas 51: 17-29. doi: 10.1353/jda.2017.0028
![]() |
[18] |
Barberis N, Shleifer A, Vishny R (1998) A model of investor sentiment. J Financ Econ 49: 307-343. doi: 10.1016/S0304-405X(98)00027-0
![]() |
[19] |
Basher SA, Hassan MK, Islam AM (2007) Time-varying volatility and equity returns in Bangladesh stock market. Appl Financ Econ 17: 1393-1407. doi: 10.1080/09603100600771034
![]() |
[20] |
Basher SA, Haug AA, Sadorsky P (2018) The impact of oil-market shocks on stock returns in major oil-exporting countries. J Int Money Financ 86: 264-280. doi: 10.1016/j.jimonfin.2018.05.003
![]() |
[21] |
Bekaert G, Ehrmann M, Fratzscher M, et al. (2014) The global crisis and equity market contagion. J Financ 69: 2597-2649. doi: 10.1111/jofi.12203
![]() |
[22] |
Bekaert G, Harvey CR, Lundblad CT (2003) Equity market liberalization in emerging markets. J Financ Res 26: 275-299. doi: 10.1111/1475-6803.00059
![]() |
[23] |
Blanchard O, Dell'Ariccia G, Mauro P (2010) Rethinking macroeconomic policy. J Money Credit Bank 42: 199-215. doi: 10.1111/j.1538-4616.2010.00334.x
![]() |
[24] |
Choi S, Furceri D (2019) Uncertainty and cross-border banking flows. J In Money Financ 93: 260-274. doi: 10.1016/j.jimonfin.2019.01.012
![]() |
[25] |
Chung KH, Chuwonganant C (2018) Market volatility and stock returns: The role of liquidity providers. J Financ Markets 37: 17-34. doi: 10.1016/j.finmar.2017.07.002
![]() |
[26] |
Guo P, Zhu H, You W (2018) Asymmetric dependence between economic policy uncertainty and stock market returns in G7 and BRIC: A quantile regression approach. Financ Res Lett 25: 251-258. doi: 10.1016/j.frl.2017.11.001
![]() |
[27] |
Hamilton JD (1989) A new approach to the economic analysis of nonstationary time series and the business cycle. Econometrica: J Econometric Society, 357-384. doi: 10.2307/1912559
![]() |
[28] | Hamilton JD (2010) Regime switching models, In Macroeconometrics and time series analysis, Palgrave Macmillan UK, 202-209. |
[29] |
Hassan MK, Chowdhury SSH (2008) Efficiency of Bangladesh stock market: evidence from monthly index and individual firm data. Appl Financ Econ 18: 749-758. doi: 10.1080/09603100701320178
![]() |
[30] | Hoque ME, Zaidi AS (2020) Impacts of Global Economic Policy Uncertainty 0n Emerging Stock Markets: Evidence From Linear and Non-Linear Models. Prague Econ Pap, 1-12. |
[31] |
Hoque ME, Zaidi MAS (2019) The impacts of global economic policy uncertainty on stock market returns in regime switching environment: Evidence from sectoral perspectives. Int J Financ Econ 24: 991-1016. doi: 10.1002/ijfe.1702
![]() |
[32] | Islam KM, Ahmed SF (2015) Stock market crash and stock return volatility: empirical evidence from Dhaka stock exchange. Bangladesh Dev Stud 38: 25-34. |
[33] | Islam MS (2012) China-Bangladesh relations: contemporary convergence. Daily Star 25. |
[34] | Islam MS, Islam MR (2011) Demutualization: Pros and Cons for Dhaka Stock Exchange (DSE). Eur J Bus Manage 3: 24-33. |
[35] |
Janina E, Markus W, Rudi Z (2018) Forecasting turbulence in the Asian and European stock market using regime-switching models. Quant Financ Econ 2: 388-406. doi: 10.3934/QFE.2018.2.388
![]() |
[36] |
JulioB, Yook Y (2016) Policy uncertainty, irreversibility, and cross-border flows of capital. J Int Econ 103: 13-26. doi: 10.1016/j.jinteco.2016.08.004
![]() |
[37] | Li R, Sufang L, Di Yuan, et al. (2020) Does economic policy uncertainty in the US influence stock markets in China and India? Time-frequency evidence. Appl Econ, 1-17. |
[38] |
Li X, Mehmet B, Rangan G, et al. (2016) The causal relationship between economic policy uncertainty and stock returns in China and India: evidence from a bootstrap rolling window approach. Emerging Markets Financ Trade 52: 674-689. doi: 10.1080/1540496X.2014.998564
![]() |
[39] | Mahmud N (2019) Chinese consortium in DSE: Performance not as per expectation after a year. Dhaka Tribune. Retrieved on 5th October, 2019 . Available from: https://www.dhakatribune.com/business/stock/2019/05/13/chinese-consortium-in-dse-performance-not-as-per-expectation-after-a-year |
[40] | Manni UH, Afzal MNI (2012) Effect of trade liberalization on economic growth of developing countries: A case of Bangladesh economy. J Bus Econ 1: 37-44. |
[41] | Mollah S (2011) Do emerging market firms follow different dividend policies? Empirical investigation on the pre-and post-reform dividend policy and behaviour of Dhaka Stock Exchange listed firms. Stud Econ Financ 28: 118-135. |
[42] | Murshid KAS, Zohir SC, Ahmed M, et al. (2009) The global financial crisis implications for Bangladesh. BIDS-PRP Working Paper Series, 1. |
[43] |
Pastor L, Veronesi P (2012) Uncertainty about government policy and stock prices. J Financ 67: 1219-1264. doi: 10.1111/j.1540-6261.2012.01746.x
![]() |
[44] |
Phan DHB, Sharma SS, Tran VT (2018) Can economic policy uncertainty predict stock returns? Global evidence. J Int Financ Markets Inst Money 55, 134-150. doi: 10.1016/j.intfin.2018.04.004
![]() |
[45] |
Portes R, Rey H (2005) The determinants of cross-border equity flows. J Int Econ 65: 269-296. doi: 10.1016/j.jinteco.2004.05.002
![]() |
[46] |
Rahman T, Hossain S, Habibullah M (2017) Stock market crash in Bangladesh: The moneymaking psychology of domestic investors. Am J Theor Appl Bus 3: 43-53. doi: 10.11648/j.ajtab.20170303.12
![]() |
[47] | Rey H (2015) Dilemma not trilemma: the global financial cycle and monetary policy independence. National Bureau of Economic Research (No. w21162). |
[48] |
Tsai I (2017) The source of global stock market risk: A viewpoint of economic policy uncertainty. Econ Model 60: 122-131. doi: 10.1016/j.econmod.2016.09.002
![]() |
[49] | Ullah N, Uddin MA (2018) Does Indian Economic Growth Affect Bangladesh? An Application of ARDL. J South Asian Stud 6: 69-84. |
[50] |
Zhang D, Lei L, Ji Q, et al. (2019) Economic policy uncertainty in the US and China and their impact on the global markets. Econ Model 79: 47-56. doi: 10.1016/j.econmod.2018.09.028
![]() |
1. | Xuehong Zhu, Jianhui Liao, Ying Chen, Time-varying effects of oil price shocks and economic policy uncertainty on the nonferrous metals industry: From the perspective of industrial security, 2021, 97, 01409883, 105192, 10.1016/j.eneco.2021.105192 | |
2. | Yaya Su, Yi Qu, Yuxuan Kang, Online public opinion and asset prices: a literature review, 2021, 1, 2769-2140, 60, 10.3934/DSFE.2021004 | |
3. | Shuanglian Chen, Junhao Zhong, Pierre Failler, Does China transmit financial cycle spillover effects to the G7 countries?, 2022, 35, 1331-677X, 5184, 10.1080/1331677X.2021.2025123 | |
4. | Kexian Zhang, Yan Wang, Zimei Huang, Do the Green Credit Guidelines Affect Renewable Energy Investment? Empirical Research from China, 2021, 13, 2071-1050, 9331, 10.3390/su13169331 | |
5. | Fenghua Wen, Aojie Shui, Yuxiang Cheng, Xu Gong, Monetary policy uncertainty and stock returns in G7 and BRICS countries: A quantile-on-quantile approach, 2022, 78, 10590560, 457, 10.1016/j.iref.2021.12.015 | |
6. | Fenghua Wen, Zhen Liu, jiahui Cao, Yun Zhang, Zhujia Yin, Mood seasonality: Evidence from the Chinese A-share market, 2022, 46, 15446123, 102232, 10.1016/j.frl.2021.102232 | |
7. | Kaiming Zhong, Hongyan Fu, Tinghui Li, Can the Digital Economy Facilitate Carbon Emissions Decoupling? An Empirical Study Based on Provincial Data in China, 2022, 19, 1660-4601, 6800, 10.3390/ijerph19116800 | |
8. | Xuehong Zhu, Ying Chen, Jinyu Chen, Effects of non-ferrous metal prices and uncertainty on industry stock market under different market conditions, 2021, 73, 03014207, 102243, 10.1016/j.resourpol.2021.102243 | |
9. | Rongwu Zhang, Yanzhen Lin, Yingxu Kuang, Will the Governance of Non-State Shareholders Inhibit Corporate Social Responsibility Performance? Evidence from the Mixed-Ownership Reform of China’s State-Owned Enterprises, 2022, 14, 2071-1050, 527, 10.3390/su14010527 | |
10. | Junjie Guo, Youshu Li, Qinglong Shao, Cross-category spillover effects of economic policy uncertainty between China and the US: Time and frequency evidence, 2022, 80, 10490078, 101462, 10.1016/j.asieco.2022.101462 | |
11. | Rui Huang, Hao Xiao, Guoqin Pan, Authority decentralization and air pollution reduction: Evidence from China, 2022, 19, 1551-0018, 14212, 10.3934/mbe.2022661 | |
12. | Jinyu Chen, Yilin Wang, Xiaohang Ren, Asymmetric effects of non-ferrous metal price shocks on clean energy stocks: Evidence from a quantile-on-quantile method, 2022, 78, 03014207, 102796, 10.1016/j.resourpol.2022.102796 | |
13. | Yueting Jiang, Marwan Mohamed Abdeldayem, Prediction and Analysis of ChiNext Stock Price Based on Linear and Non-linear Composite Model, 2022, 0, 2444-8656, 10.2478/amns.2022.2.0055 | |
14. | Mohammad Enamul Hoque, Low Soo-Wah, Md Akther Uddin, Ashiqur Rahman, International trade policy uncertainty spillover on stock market: Evidence from fragile five economies, 2023, 32, 0963-8199, 104, 10.1080/09638199.2022.2072520 | |
15. | Joseph Chukwudi Odionye, Ethelbert Ukachukwu Ojiaku, Godwin Chigozie Okpara, Ndubuisi Agoh, Roy M. Okpara, Economic Policy Uncertainty and Stock Market Index: Fresh Insights from Augmented-ARDL and Multiple Structural Breaks, 2024, 15, 1793-9933, 10.1142/S1793993324500108 | |
16. | Oana Panazan, Catalin Gheorghe, Emilia Calefariu, Geopolitical Risks and Stock Market Volatility in the SAARC Region, 2024, 18, 1864-6042, 10.1515/econ-2022-0124 | |
17. | Sitara Jabeen, The asymmetric effects of exchange rate volatility on UK–Pakistan trade flows: New evidence from nonlinear ARDL approach, 2024, 0963-8199, 1, 10.1080/09638199.2024.2379572 | |
18. | Joseph Chukwudi Odionye, Ethelbert Ukachukwu Ojiaku, Ndubuisi Agoh, Chikeziem F. Okorontah, Roy M. Okpara, Callistus Ogu, Economic policy uncertainty and equity index in sub-Saharan African (SSA) countries: accounting for multiple structural breaks in a panel framework, 2024, 4, 2662-9399, 10.1007/s43546-024-00664-z | |
19. | Chenxi Wang, Ran Duan, Impact of Economic Policy Uncertainty on Bank Loan Restructuring: Empirical Evidence from Industry-Level Loan Distribution in China, 2025, 15, 2158-2440, 10.1177/21582440241310313 |
RET | IN | EU | CN | CH | US | RS | |
Mean | −0.001 | 93.209 | 143.487 | 144.631 | 160.270 | 118.675 | 122.151 |
Median | 0.000 | 79.001 | 132.868 | 123.371 | 109.093 | 108.634 | 103.439 |
Maximum | 0.327 | 283.689 | 433.277 | 449.623 | 935.310 | 284.135 | 400.016 |
Minimum | −0.369 | 24.939 | 47.692 | 30.0970 | 9.066 | 44.782 | 12.3987 |
Std. Dev. | 0.0839 | 51.904 | 65.121 | 87.718 | 145.52 | 46.130 | 79.135 |
Skewness | −0.574 | 1.289 | 1.1642 | 1.0468 | 2.295 | 1.074 | 1.0872 |
Kurtosis | 7.590 | 4.577 | 5.140 | 3.657 | 9.0371 | 4.114 | 3.790 |
Jarque-Bera | 250.059 | 74.660 | 111.694 | 53.775 | 642.315 | 65.447 | 59.779 |
Probability | 0.000 | 0.000 | 0.000 | 0.000 | 0.000 | 0.000 | 0.000 |
RET | US | EU | CN | RS | CH | IN | |
RET | 1 | ||||||
US | 0.4605 | 1 | |||||
EU | 0.5470 | 0.7168 | 1 | ||||
CN | −0.5993 | 0.6996 | 0.7837 | 1 | |||
RS | −0.3904 | 0.2709 | 0.4675 | 0.4763 | 1 | ||
CH | −0.4109 | 0.5077 | 0.6840 | 0.6780 | 0.4536 | 1 | |
IN | −0.3177 | 0.4333 | 0.3311 | 0.3165 | 0.1509 | 0.0456 | 1 |
Panel A: PP | |||||||
Panel A1:At Level | |||||||
RET | CH | CN | EU | IN | RS | US | |
With Constant | −16.408*** | −3.231** | −3.954*** | −4.666*** | −5.831*** | −10.002*** | −7.086*** |
With Constant & Trend | −16.471*** | −5.410*** | −6.749*** | −7.041*** | −5.824*** | −13.129*** | −7.718*** |
Without Constant & Trend | −16.431*** | −1.496 | −1.328 | −1.231 | −1.787* | −3.404*** | −1.825* |
Panel A2: At First Difference | |||||||
With Constant | −56.398*** | −26.574*** | −36.112*** | −37.986*** | −30.069*** | −64.867*** | −46.03*** |
With Constant & Trend | −56.845*** | −27.946*** | −36.702*** | −38.243*** | −30.358*** | −65.553*** | −45.894*** |
Without Constant & Trend | −56.422*** | −25.849*** | −35.195*** | −37.188*** | −30.169*** | −64.374*** | −45.712*** |
Panel B:ADF | |||||||
Panel B1: At Level | |||||||
With Constant | −16.176*** | −0.387 | −2.155 | −2.518 | −2.681* | −0.768 | −2.57* |
With Constant & Trend | −16.320*** | −1.991 | −5.357*** | −4.046*** | −2.65 | −3.0479 | −3.24* |
Without Constant & Trend | −16.205*** | 0.6816 | 0.0621 | 0.0206 | −1.29* | 0.75 | −0.193 |
Panel B2: At First Difference | |||||||
With Constant | −9.153*** | −6.22*** | −8.029*** | −5.899*** | −17.042*** | −6.197*** | −8.963*** |
With Constant & Trend | −9.196*** | −6.359*** | −8.028*** | −5.886*** | −17.002*** | −6.18*** | −8.944*** |
Without Constant & Trend | −9.162*** | −6.083*** | −7.996*** | −5.871*** | −17.086*** | −6.084*** | −8.965*** |
Note: *, **, and *** denote statistical signifies at 10%, 5%, and 1% respectively. |
M | Ɛ(1) | Ɛ(2) | Ɛ(3) |
2 | 0.07*** | 0.123*** | 0.1169*** |
3 | 0.08*** | 0.174*** | 0.1671*** |
Note: *, **, and *** denote statistical signifies at 10%, 5%, and 1% respectively. |
udmax | wdmax | supr(0/1) | supr(1/2) | supr(2/3) | supr(3/4) | numbers of breaks select | optimal break | break date | |||
seq | bic | lwz | |||||||||
ret | 31.13** | 32.8*** | 28.92** | 56.90** | 31.711** | 31.71** | 4 | 0 | 0 | 4 | 2007m06, 2009m11, 2012m06, 2016m12 |
Note:** denotes statistical signifies at 5%. This table reports the results of the procedure developed by bai and perron(1998, 2003) to search endogenously for structural breaks. the effective sample size is 938. a maximum of five breaks are allowed and a trimming parameter (minimum size of a segment with respect to the sample size) of 0.15 is used, so each segment has at least 140 observations. the double maximum tests (udmax and wdmax) test the null of no structural breaks against the alternative of an unknown number of breaks. the supft (L + 1/L) is a sequential test of the null of l breaks versus the alternative of l + 1 breaks. sequential, bic and lwz denote the sequential procedure, Bayesian information criterion and information criterion suggested by liu et al. (1997), respectively. as usual *, ** and *** indicate statistical significance at 10%, 5% and 1% levels, respectively. |
Breaks | C | RET(-1) | CH | CH(-1) | CN | CN(-1) | EU | EU(-1) | IN | IN(-1) | RS | RS(-1) | US | US(-1) | |||
2003M02−2007M05 | Coefficients | 0.019 | −0.092 | 0 | −0.001 | −0.001 | 0 | 0 | −0.001 | 0 | −0.001 | 0.001 | 0 | 0 | 0.001 | R-squared | 0.4928 |
T-statistic | 0.283 | −0.795 | −0.608 | −1.99** | −1.419 | 0.685 | 0.747 | −1.013 | 0.617 | −3.37** | 1.376 | −1.416 | 0.34 | 1.332 | Adj R−squared | 0.2128 | |
2007M06−2009M10 | Coefficients | 0.297 | −0.314 | −0.001 | −0.001 | 0 | 0.001 | 0 | 0 | 0 | 0 | 0.001 | −0.001 | −0.001 | −0.001 | Log likelihood | 293.855 |
T-statistic | 2.349*** | −1.372 | −1.254 | −1.391 | 0.23 | 1.858* | 0.47 | −0.722 | −0.37 | −0.595 | 1.375 | −1.195 | −0.831 | −1.89 | F-statistic | 11.7601 | |
2009M11−2012M05 | Coefficients | 0.054 | −0.215 | 0.001 | −0.001 | −0.002 | 0.001 | 0.002 | −0.003 | 0 | −0.001 | 0 | 0.002 | 0.001 | 0 | Prob(F-statistic) | 0.0031 |
T-statistic | 0.811 | −2.10** | 3.56*** | −2.354*** | −6.59*** | 2.60** | 4.12*** | −4.06*** | −0.23 | −3.36*** | 0.22 | 4.11*** | 2.67*** | −1.24 | Prob(F-statistic) | 0.0031 | |
2012M06−2016M11 | Coefficients | 0.058 | −0.115 | 0 | 0 | 0 | 0 | 0 | 0 | 0 | 0 | 0 | 0 | 0 | 0 | Durbin-Watson stat | 1.998 |
T-statistic | 1.235 | −0.958 | 0.404 | −0.714 | −0.935 | 0.557 | 1.063 | −0.428 | −0.292 | 0.024 | 0.272 | −1.223 | −0.416 | −0.125 | LM test | 0.858 | |
2016M12−2019M04 | Coefficients | −0.135 | −0.039 | 0.002 | 0.0003 | 0.004 | −.001 | 0.0001 | 0.0003 | 0.009 | 0.0001 | 0.0002 | −0.001 | 0.0001 | 0.0001 | ARCH Test | 1.031 |
T-statistic | −2.148*** | −0.11 | 0.024 | −0.769 | 0.651 | −2.139** | 1.388 | −0.531 | 0.52 | 0.417 | 3.119*** | −1.964** | 1.656* | 2.101*** | |||
Note: *, **, and *** denote statistical signifies at 10%, 5%, and 1% respectively. |
C | CH | CH(-1) | CN | CN(-1) | EU | EU(-1) | IN | IN(-1) | RS | RS(-1) | US | US(-1) | LOG(SIGMA) | ||||
Low Volatile Regime | Coefficients | 0.037 | 0 | 0.001 | 0.001 | 0.003 | 0.004 | 0.001 | 0.005 | 0.005 | 0.003 | 0.001 | 0.001 | 0.001 | −2.463 | P11-WORLD | −3.506** |
T-statistic | 1.097 | 1.706 | −2.116** | −1.612 | −0.401 | 0.159 | −0.171 | −1.429 | −0.133 | 1.051 | −0.376 | 0.945 | 0.713 | −32.40*** | P12-WORLD | 0.03 | |
High Volatile Regime | Coefficients | 0.024 | −0.02 | 0.007 | −0.065 | −0.001 | −0.054 | 0.001 | 0.004 | 0.003 | 0.024 | 0.002 | 0.001 | 0.001 | −3.348 | ||
T-statistic | 1.19 | −1.56 | 3.203*** | −0.7 | −0.01 | −1.98** | −1.396 | −2.1** | 2.54** | 1.143 | −2.5** | −0.527 | −1.355 | −33.34*** | |||
Note: *, **, and *** denote statistical signifies at 10%, 5%, and 1% respectively. |
RET | IN | EU | CN | CH | US | RS | |
Mean | −0.001 | 93.209 | 143.487 | 144.631 | 160.270 | 118.675 | 122.151 |
Median | 0.000 | 79.001 | 132.868 | 123.371 | 109.093 | 108.634 | 103.439 |
Maximum | 0.327 | 283.689 | 433.277 | 449.623 | 935.310 | 284.135 | 400.016 |
Minimum | −0.369 | 24.939 | 47.692 | 30.0970 | 9.066 | 44.782 | 12.3987 |
Std. Dev. | 0.0839 | 51.904 | 65.121 | 87.718 | 145.52 | 46.130 | 79.135 |
Skewness | −0.574 | 1.289 | 1.1642 | 1.0468 | 2.295 | 1.074 | 1.0872 |
Kurtosis | 7.590 | 4.577 | 5.140 | 3.657 | 9.0371 | 4.114 | 3.790 |
Jarque-Bera | 250.059 | 74.660 | 111.694 | 53.775 | 642.315 | 65.447 | 59.779 |
Probability | 0.000 | 0.000 | 0.000 | 0.000 | 0.000 | 0.000 | 0.000 |
RET | US | EU | CN | RS | CH | IN | |
RET | 1 | ||||||
US | 0.4605 | 1 | |||||
EU | 0.5470 | 0.7168 | 1 | ||||
CN | −0.5993 | 0.6996 | 0.7837 | 1 | |||
RS | −0.3904 | 0.2709 | 0.4675 | 0.4763 | 1 | ||
CH | −0.4109 | 0.5077 | 0.6840 | 0.6780 | 0.4536 | 1 | |
IN | −0.3177 | 0.4333 | 0.3311 | 0.3165 | 0.1509 | 0.0456 | 1 |
Panel A: PP | |||||||
Panel A1:At Level | |||||||
RET | CH | CN | EU | IN | RS | US | |
With Constant | −16.408*** | −3.231** | −3.954*** | −4.666*** | −5.831*** | −10.002*** | −7.086*** |
With Constant & Trend | −16.471*** | −5.410*** | −6.749*** | −7.041*** | −5.824*** | −13.129*** | −7.718*** |
Without Constant & Trend | −16.431*** | −1.496 | −1.328 | −1.231 | −1.787* | −3.404*** | −1.825* |
Panel A2: At First Difference | |||||||
With Constant | −56.398*** | −26.574*** | −36.112*** | −37.986*** | −30.069*** | −64.867*** | −46.03*** |
With Constant & Trend | −56.845*** | −27.946*** | −36.702*** | −38.243*** | −30.358*** | −65.553*** | −45.894*** |
Without Constant & Trend | −56.422*** | −25.849*** | −35.195*** | −37.188*** | −30.169*** | −64.374*** | −45.712*** |
Panel B:ADF | |||||||
Panel B1: At Level | |||||||
With Constant | −16.176*** | −0.387 | −2.155 | −2.518 | −2.681* | −0.768 | −2.57* |
With Constant & Trend | −16.320*** | −1.991 | −5.357*** | −4.046*** | −2.65 | −3.0479 | −3.24* |
Without Constant & Trend | −16.205*** | 0.6816 | 0.0621 | 0.0206 | −1.29* | 0.75 | −0.193 |
Panel B2: At First Difference | |||||||
With Constant | −9.153*** | −6.22*** | −8.029*** | −5.899*** | −17.042*** | −6.197*** | −8.963*** |
With Constant & Trend | −9.196*** | −6.359*** | −8.028*** | −5.886*** | −17.002*** | −6.18*** | −8.944*** |
Without Constant & Trend | −9.162*** | −6.083*** | −7.996*** | −5.871*** | −17.086*** | −6.084*** | −8.965*** |
Note: *, **, and *** denote statistical signifies at 10%, 5%, and 1% respectively. |
M | Ɛ(1) | Ɛ(2) | Ɛ(3) |
2 | 0.07*** | 0.123*** | 0.1169*** |
3 | 0.08*** | 0.174*** | 0.1671*** |
Note: *, **, and *** denote statistical signifies at 10%, 5%, and 1% respectively. |
udmax | wdmax | supr(0/1) | supr(1/2) | supr(2/3) | supr(3/4) | numbers of breaks select | optimal break | break date | |||
seq | bic | lwz | |||||||||
ret | 31.13** | 32.8*** | 28.92** | 56.90** | 31.711** | 31.71** | 4 | 0 | 0 | 4 | 2007m06, 2009m11, 2012m06, 2016m12 |
Note:** denotes statistical signifies at 5%. This table reports the results of the procedure developed by bai and perron(1998, 2003) to search endogenously for structural breaks. the effective sample size is 938. a maximum of five breaks are allowed and a trimming parameter (minimum size of a segment with respect to the sample size) of 0.15 is used, so each segment has at least 140 observations. the double maximum tests (udmax and wdmax) test the null of no structural breaks against the alternative of an unknown number of breaks. the supft (L + 1/L) is a sequential test of the null of l breaks versus the alternative of l + 1 breaks. sequential, bic and lwz denote the sequential procedure, Bayesian information criterion and information criterion suggested by liu et al. (1997), respectively. as usual *, ** and *** indicate statistical significance at 10%, 5% and 1% levels, respectively. |
Breaks | C | RET(-1) | CH | CH(-1) | CN | CN(-1) | EU | EU(-1) | IN | IN(-1) | RS | RS(-1) | US | US(-1) | |||
2003M02−2007M05 | Coefficients | 0.019 | −0.092 | 0 | −0.001 | −0.001 | 0 | 0 | −0.001 | 0 | −0.001 | 0.001 | 0 | 0 | 0.001 | R-squared | 0.4928 |
T-statistic | 0.283 | −0.795 | −0.608 | −1.99** | −1.419 | 0.685 | 0.747 | −1.013 | 0.617 | −3.37** | 1.376 | −1.416 | 0.34 | 1.332 | Adj R−squared | 0.2128 | |
2007M06−2009M10 | Coefficients | 0.297 | −0.314 | −0.001 | −0.001 | 0 | 0.001 | 0 | 0 | 0 | 0 | 0.001 | −0.001 | −0.001 | −0.001 | Log likelihood | 293.855 |
T-statistic | 2.349*** | −1.372 | −1.254 | −1.391 | 0.23 | 1.858* | 0.47 | −0.722 | −0.37 | −0.595 | 1.375 | −1.195 | −0.831 | −1.89 | F-statistic | 11.7601 | |
2009M11−2012M05 | Coefficients | 0.054 | −0.215 | 0.001 | −0.001 | −0.002 | 0.001 | 0.002 | −0.003 | 0 | −0.001 | 0 | 0.002 | 0.001 | 0 | Prob(F-statistic) | 0.0031 |
T-statistic | 0.811 | −2.10** | 3.56*** | −2.354*** | −6.59*** | 2.60** | 4.12*** | −4.06*** | −0.23 | −3.36*** | 0.22 | 4.11*** | 2.67*** | −1.24 | Prob(F-statistic) | 0.0031 | |
2012M06−2016M11 | Coefficients | 0.058 | −0.115 | 0 | 0 | 0 | 0 | 0 | 0 | 0 | 0 | 0 | 0 | 0 | 0 | Durbin-Watson stat | 1.998 |
T-statistic | 1.235 | −0.958 | 0.404 | −0.714 | −0.935 | 0.557 | 1.063 | −0.428 | −0.292 | 0.024 | 0.272 | −1.223 | −0.416 | −0.125 | LM test | 0.858 | |
2016M12−2019M04 | Coefficients | −0.135 | −0.039 | 0.002 | 0.0003 | 0.004 | −.001 | 0.0001 | 0.0003 | 0.009 | 0.0001 | 0.0002 | −0.001 | 0.0001 | 0.0001 | ARCH Test | 1.031 |
T-statistic | −2.148*** | −0.11 | 0.024 | −0.769 | 0.651 | −2.139** | 1.388 | −0.531 | 0.52 | 0.417 | 3.119*** | −1.964** | 1.656* | 2.101*** | |||
Note: *, **, and *** denote statistical signifies at 10%, 5%, and 1% respectively. |
C | CH | CH(-1) | CN | CN(-1) | EU | EU(-1) | IN | IN(-1) | RS | RS(-1) | US | US(-1) | LOG(SIGMA) | ||||
Low Volatile Regime | Coefficients | 0.037 | 0 | 0.001 | 0.001 | 0.003 | 0.004 | 0.001 | 0.005 | 0.005 | 0.003 | 0.001 | 0.001 | 0.001 | −2.463 | P11-WORLD | −3.506** |
T-statistic | 1.097 | 1.706 | −2.116** | −1.612 | −0.401 | 0.159 | −0.171 | −1.429 | −0.133 | 1.051 | −0.376 | 0.945 | 0.713 | −32.40*** | P12-WORLD | 0.03 | |
High Volatile Regime | Coefficients | 0.024 | −0.02 | 0.007 | −0.065 | −0.001 | −0.054 | 0.001 | 0.004 | 0.003 | 0.024 | 0.002 | 0.001 | 0.001 | −3.348 | ||
T-statistic | 1.19 | −1.56 | 3.203*** | −0.7 | −0.01 | −1.98** | −1.396 | −2.1** | 2.54** | 1.143 | −2.5** | −0.527 | −1.355 | −33.34*** | |||
Note: *, **, and *** denote statistical signifies at 10%, 5%, and 1% respectively. |