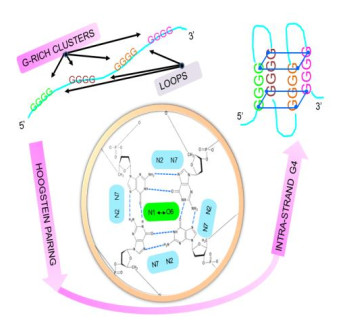
Citation: Eric Ariel L. Salas, Sakthi Kumaran Subburayalu. Implications of climate change on nutrient pollution: a look into the nitrogen and phosphorus loadings in the Great Miami and Little Miami watersheds in Ohio[J]. AIMS Environmental Science, 2019, 6(3): 186-221. doi: 10.3934/environsci.2019.3.186
[1] | Wenjun Xia, Jinzhi Lei . Formulation of the protein synthesis rate with sequence information. Mathematical Biosciences and Engineering, 2018, 15(2): 507-522. doi: 10.3934/mbe.2018023 |
[2] | Chaudry Masood Khalique, Kentse Maefo . A study on the (2+1)–dimensional first extended Calogero-Bogoyavlenskii- Schiff equation. Mathematical Biosciences and Engineering, 2021, 18(5): 5816-5835. doi: 10.3934/mbe.2021293 |
[3] | Sixing Tao . Lie symmetry analysis, particular solutions and conservation laws of a (2+1)-dimensional KdV4 equation. Mathematical Biosciences and Engineering, 2023, 20(7): 11978-11997. doi: 10.3934/mbe.2023532 |
[4] | Alexander N. Gorban, Annick Harel-Bellan, Nadya Morozova, Andrei Zinovyev . Basic, simple and extendable kinetic model of protein synthesis. Mathematical Biosciences and Engineering, 2019, 16(6): 6602-6622. doi: 10.3934/mbe.2019329 |
[5] | Mahmoud El-Morshedy, Zubair Ahmad, Elsayed tag-Eldin, Zahra Almaspoor, Mohamed S. Eliwa, Zahoor Iqbal . A new statistical approach for modeling the bladder cancer and leukemia patients data sets: Case studies in the medical sector. Mathematical Biosciences and Engineering, 2022, 19(10): 10474-10492. doi: 10.3934/mbe.2022490 |
[6] | Lan Huang, Shuyu Guo, Ye Wang, Shang Wang, Qiubo Chu, Lu Li, Tian Bai . Attention based residual network for medicinal fungi near infrared spectroscopy analysis. Mathematical Biosciences and Engineering, 2019, 16(4): 3003-3017. doi: 10.3934/mbe.2019149 |
[7] | Luis L. Bonilla, Vincenzo Capasso, Mariano Alvaro, Manuel Carretero, Filippo Terragni . On the mathematical modelling of tumor-induced angiogenesis. Mathematical Biosciences and Engineering, 2017, 14(1): 45-66. doi: 10.3934/mbe.2017004 |
[8] | J. A. Méndez-Bermúdez, José M. Rodríguez, José L. Sánchez, José M. Sigarreta . Analytical and computational properties of the variable symmetric division deg index. Mathematical Biosciences and Engineering, 2022, 19(9): 8908-8922. doi: 10.3934/mbe.2022413 |
[9] | Pu Yang, Zhenbo Li, Yaguang Yu, Jiahui Shi, Ming Sun . Studies on fault diagnosis of dissolved oxygen sensor based on GA-SVM. Mathematical Biosciences and Engineering, 2021, 18(1): 386-399. doi: 10.3934/mbe.2021021 |
[10] | Micaela Morettini, Christian Göbl, Alexandra Kautzky-Willer, Giovanni Pacini, Andrea Tura, Laura Burattini . Former gestational diabetes: Mathematical modeling of intravenous glucose tolerance test for the assessment of insulin clearance and its determinants. Mathematical Biosciences and Engineering, 2020, 17(2): 1604-1615. doi: 10.3934/mbe.2020084 |
G-quadruplex or G-tetrad (G4), is a thermodynamically stable structural element that is formed between clusters/stretches/tracts of Guanine (G) residues (|x|≥3) and is intra- or inter-molecular [1,2,3]. The intervening loops whence applicable are composed of one or more nucleotide(s) (N∈{A, U, T, G, C}) (Figure 1). G4 is found in DNA (telomeres, double-strand break sites, transcription start sites) and in the untranslated region(s) (5'-, 3'-UTR, introns) of mRNA [4,5]. In vivo, G4 may function to preserve the telomeric ends of chromosomes, repress or promote transcription and regulate translation [4,5]. The generic representation of an intra-strand G4 may be described as follows:
(((Gt,k)t≥3(Nh,k)h≥1)k=3((Gt,k)t≥3)k=1)m=1 | (Def. 1) |
𝑡 ≔ 𝑁𝑢𝑚𝑏𝑒𝑟 𝑜𝑓 𝐺𝑢𝑎𝑛𝑖𝑛𝑒𝑠 𝑝𝑒𝑟 𝐺 − 𝑟𝑖𝑐ℎ 𝑐𝑙𝑢𝑠𝑡𝑒𝑟
ℎ ≔ 𝑁𝑢𝑚𝑏𝑒𝑟 𝑜𝑓 𝑙𝑜𝑜𝑝 − 𝑓𝑜𝑟𝑚𝑖𝑛𝑔 𝑔𝑒𝑛𝑒𝑟𝑖𝑐 𝑖𝑛𝑡𝑒𝑟𝑣𝑒𝑛𝑖𝑛𝑔 𝑛𝑢𝑐𝑙𝑒𝑜𝑡𝑖𝑑𝑒𝑠
𝑘 ≔ 𝐶𝑙𝑢𝑠𝑡𝑒𝑟 𝑖𝑛𝑑𝑒𝑥
𝑚 ≔ 𝑁𝑢𝑚𝑏𝑒𝑟 𝑜𝑓 𝑠𝑡𝑟𝑎𝑛𝑑𝑠
𝐺 ≔ 𝐺𝑢𝑎𝑛𝑖𝑛𝑒
𝐴 ≔ 𝐴𝑑𝑒𝑛𝑖𝑛𝑒
𝑇 ≔ 𝑇ℎ𝑦𝑚𝑖𝑛𝑒
𝐶 ≔ 𝐶𝑦𝑡𝑜𝑠𝑖𝑛𝑒
𝑁 ≔ 𝐴𝑛𝑦 𝑛𝑢𝑐𝑙𝑒𝑜𝑡𝑖𝑑𝑒
The high melting temperature (Tm~600C) of G4 implies that the mature quadruplex is stable and refractory to unfolding. This is partly due to stabilizing Hoogsteen (N7gu1-N2gu2; O6gu1-N1gu2) and reverse Hoogsteen (N7gu1-N1gu2; O6gu1-N2gu2) hydrogen bonding as well as π-orbital stacking between the purine rings of non-contiguous guanine pairs (gu1, gu2) (Figure 1) [6,7]. Additionally, the presence of Adenine residues in the intervening loops, variable loop length (h~1-30 Mer) and permutation have all been shown to contribute to the stability and thence persistence of the mature quadruplex [8,9,10,11].
Tm∝(#Adenine/h)=τ.(#Adenine/h)Tm:=Melting temperatureτ:=constant of proportionalityh:=Length of intervening loops | (1) |
Despite the wide range of methods available that can predict G4 formation in DNA/RNA, there is poor agreement between sequence-based motif locators and empirically derived biophysical data [12,13,14,15]. Motif-independent methods such as those that directly measure the GC-content or the GC-/AT-skew of a query sequence and utilize this data to train machine learning algorithms may address some of these discrepancies [16,17,18].
Investigations into transcribed RNA suggests that secondary and tertiary forms (5'- and 3'-UTRs) may not only coexist with stretches of unfolded ribonucleotides, but can also be read by the ribosomal machinery. Non-canonical translation is described as: a) translation from atypical start sites AUG→{CUG, GUG} or b) peptides (≤100 aa) of short open reading frames (sORF)-encoded polypeptides (SEPs) and upstream open reading frames (uORFs) [19,20,21,22]. The latter are rarely silent and can function as modulators of metabolism (S-Adenosylmethionine decarboxylase, AMD1) or transcription (activating transcription factor, ATF4, H19; yeast AP-1 like, YAP1) and as generic transcription factors (general control protein, GCN4) [19]. G4 has also been observed in one or more exons of the prion protein (PRNP, exon 2), zinc finger protein (ZNF669, exon 1), β-amyloid secretase (BACE1, exon 3) and the estrogen receptor 1 (ESR1, exon 4) among several others [16,23,24,25,26,27,28,29].
Whilst the presence of segments of folded mRNA may have a significant influence on the yield of the protein product(s), the effect on sequence whence part of the protein coding segment (PCS) is largely unknown [4,5,30,31,32,33]. Proteopathies are diseases that result directly from agammaegates of truncated and misfolded proteins. These may occur secondary to a faulty translation machinery such as a ribosome that has stalled on encountering a secondary or tertiay folded mRNA sub segment. Recent data suggests ~45% of the human genome may code for proteins that are either intrinsically disordered (IDPs) or comprise one or more sub-segments that are disordered (IDRs) [34]. The absence of delineable structural features notwithstanding, disordered regions are characterized by short linear motifs (SLiMS) and/or molecular recognition features (MoRFs) [34,35]. The improper folding and heightened degradation rates could lead to perturbed proteostasis and thence contribute to the pathogenesis of proteopathies [34,35]. Primary proteopathies are likely to result directly from mutations (point, chromosomal translocations) in the PCS of a gene. These include sickle cell disease (βE6→V6-mediated defective polymerization), amylin-based type Ⅱ Diabetes Mellitus, Cystic Fibrosis (cystic fibrosis transmembrane conductance regulator), Alzheimer's disease (Amyloid β-peptide) and Parkinson's disease (α-synuclein) [36,37]. Secondary proteopathies, in contrast, result from motif or molecular mimicry of a host protein(s) by a pathogen. These are further classified into acute and chronic variants depending on the onset, genesis and/or resolution of the resultant infection or infestation [34,35].
G4 is known to stall the ribosome during translation and the resultant protein is truncated and/or degraded at an accelerated rate. The manuscript subsumes ribosomal read-through of mRNA with a G-quadruplex and assesses influence of the translated product to proteostasis. Here, I present a mathematical model of a short G4 (20–60 Mer) in the PCS, i.e., translatable G-quadruplex (TG4), in the mRNA of a hypothetical gene. The mapping uses several novel indices to annotate, classify and select suitable Guanine-containing codons (α) and amino acids (β). A generic algorithm then computes and validates, as proof-of-principle, possible peptides (pTG4ij) that correspond to the modeled TG4 (pTG4ij∈PTG4~TG4). Co-occurrence, homology and the distribution of overlapping/shared amino acids between PTG4 and the disorder promoting SLiMS are used to infer probable mechanisms of TG4~PTG4 facilitated misfolding. Standard bioinformatics indices (accuracy, precision, recall, p-value) are used to arrive at these conclusions.
The objective of this investigation is to model a short G4 in an arbitrary PCS (TG4) which when translated will result in a set of peptides (PTG4) with an average length that is less than 100 amino acids. The hypothesis explored in this manuscript is that in the event of a ribosomal read-through, the translated mRNA, with its G4 will result in a modified protein product. This protein will then exhibit considerable propensity to misfold on account of the presence of one or more members of the PTG4.
2.1.1 Model of a translatable G-quadruplex (TG4)
SEPs-derived peptides with the lowest molecular weight (~2.5 KDa) and with lengths varying from ~7–20 aa were identified and used to define the boundaries of the peptides that comprise PTG4 [20,21]. The TG4 (m = 1) is therefore, modeled as an intra-strand sub sequence of the mRNA of a hypothetical gene and has a length of ~20–60 Mer. This is represented (with symbols and variable names as explained in Def. 1) as follows:
TG4:=(((Gt,k)3≤t≤9(Nh,k)2≤h≤7)k=3((Gt,k)3≤t≤9)k=1)m=1 | (Def. 2) |
Since the Guanine-rich clusters and loops are contiguous, the aforementioned model (Def.2) of the TG4 may be approximated with a sequence of codons and is as under:
TG4:=(CODq)Lq∈N|COD∈COD | (Def. 3) |
The algorithm to compute L, which is the number of codons needed to model TG4 is presented and is as follows:
1:N←{u∈[20,60)}2:r←N mod 33:e←N−((N mod 3)/3)4:If e<(⌊e⌋+⌈e⌉)/2 then5:L=⌈e/3⌉6:else If e≥(⌊e⌋+⌈e⌉)/2 then7:L=⌊e/3⌋8:end If |
𝑁 ≔ 𝑁𝑢𝑚𝑏𝑒𝑟 𝑜𝑓 𝑟𝑖𝑏𝑜𝑛𝑢𝑐𝑙𝑒𝑜𝑡𝑖𝑑𝑒𝑠 𝑟𝑒𝑞𝑢𝑖𝑟𝑒𝑑 𝑡𝑜 𝑚𝑜𝑑𝑒𝑙 𝑇𝐺4
𝐿 : = 𝑇𝑜𝑡𝑎𝑙 𝑛𝑢𝑚𝑏𝑒𝑟 𝑜𝑓 𝑐𝑜𝑑𝑜𝑛𝑠 𝑛𝑒𝑒𝑑𝑒𝑑 𝑡𝑜 𝑚𝑜𝑑𝑒𝑙 𝑇𝐺4 (7 ≤ 𝐿 < 21)
𝑞 ≔ 𝑞𝑡ℎ 𝑐𝑜𝑑𝑜𝑛
𝑟 ≔ 𝑅𝑒𝑚𝑎𝑖𝑛𝑑𝑒𝑟 = {0, 1, 2}
𝑒, 𝑢 : = 𝐺𝑒𝑛𝑒𝑟𝑖𝑐 𝑣𝑎𝑟𝑖𝑎𝑏𝑙𝑒𝑠
𝑪𝑶𝑫 ≔ 𝑆𝑒𝑡 𝑜𝑓 𝑣𝑒𝑟𝑡𝑒𝑏𝑟𝑎𝑡𝑒 𝑐𝑜𝑑𝑜𝑛𝑠
The codons selected for modelling TG4 (Def. 3) comprised suitably scored Guanine-containing vertebrate codons (gCOD+n⊂COD) for the Guanine-rich clusters/stretches/tracks (3≤t≤9;Defs. 1 and 2) and generic/no-stop codons for the intervening loops (Figures 2 and 3). Briefly, a Guanine-containing codon (gCODn) is scored by considering its association with two similar flanking codons, i.e., gCODn-1, gCODn, gCODn+1 such that there is at least one occurrence of 'GGGG' (δ≥1.0) (Figures 2 and 3). This non-trivial case (4≤t≤9) is chosen since its trivial equivalent t = 3, is already subsumed (Defs. 1 and 2). Numerically,
αaminocodon=γ.θ.δ+Ω | (2) |
𝛾 : = 𝑃𝑟𝑜𝑏𝑎𝑏𝑖𝑙𝑖𝑡𝑦 𝑜𝑓 𝑐𝑜𝑑𝑜𝑛 𝑜𝑐𝑐𝑢𝑟𝑟𝑒𝑛𝑐𝑒 (𝛾 = 1/64 ≈ 0.02)
𝜃 ≔ 𝑃𝑟𝑜𝑏𝑎𝑏𝑖𝑙𝑖𝑡𝑦 𝑜𝑓 𝑐𝑜𝑑𝑜𝑛 𝑜𝑐𝑐𝑢𝑟𝑟𝑒𝑛𝑐𝑒 𝑤𝑖𝑡ℎ𝑖𝑛 𝑎 𝑔𝑟𝑜𝑢𝑝 (𝜃 = {0.04, 0.11, 0.33, 1})
𝛿 ≔ 𝐷𝑖𝑠𝑡𝑖𝑛𝑐𝑡 𝑜𝑐𝑐𝑢𝑟𝑟𝑒𝑛𝑐𝑒𝑠 𝑜𝑓 ′𝐺𝐺𝐺𝐺′(𝛿 = {0, 1, 2, 6})
Ω ≔ 𝑁𝑢𝑚𝑏𝑒𝑟 𝑜𝑓 𝑎𝑑𝑗𝑎𝑐𝑒𝑛𝑡 𝑐𝑜𝑑𝑜𝑛𝑠 𝑤𝑖𝑡ℎ 𝛿 (Ω = {0, 1, 2})
Since the genetic code is degenerate, amino acids mapped from the selected codons are further scored and grouped (g1, g2, g3) (Figures 3 and 4).
βamino=|gCOD+amino|/|CODamino| | (6) |
gCOD+amino:= set of optimal codons for each amino acid (αaminocodon>0.0000)
CODamino:= Set of codons for each amino acid
Whilst amino acids from groups 1 and 2 (β > 0.00) (3) can represent the modeled G-rich clusters (y∈g1∪g2 = Y), no constraint was imposed on the amino acids (z) used to model the loops (z∈g1∪g2∪g3 = Z) (Figures 3 and 4). The peptidome (PTG4) evaluated by this study is a combinatorial association of peptides such that the molecular weight is ~0.8–2.3 KDa and length of any arbitrary member is ~7–20 aa (Figure 4). This may be represented as follows:
pTG4ij=((((yi,k)1≤i≤3(zi,k)1≤i≤2)k=3(yi)1≤i≤3)(zi)1≤i≤2)j | (Def. 4) |
PTG4=⋃i=20i=7⋃j=Jj=1|pTG4ij| | (Def. 5) |
𝑷𝑻𝑮𝟒 : = 𝑃𝑒𝑝𝑡𝑖𝑑𝑜𝑚𝑒 𝑐𝑜𝑟𝑟𝑒𝑠𝑝𝑜𝑛𝑑𝑖𝑛𝑔 𝑡𝑜 𝑇𝐺4
𝑝𝑇𝐺4𝑖𝑗 : = 𝑗𝑡ℎ 𝑐𝑎𝑛𝑜𝑛𝑖𝑐𝑎𝑙 𝑎𝑚𝑖𝑛𝑜 𝑎𝑐𝑖𝑑 𝑓𝑜𝑟𝑚 𝑜𝑓 𝑃𝑇𝐺4 𝑤𝑖𝑡ℎ "i" 𝑎𝑚𝑖𝑛𝑜 𝑎𝑐𝑖𝑑𝑠
𝑖 : = 𝑁𝑢𝑚𝑏𝑒𝑟 𝑜𝑓 𝑎𝑚𝑖𝑛𝑜 𝑎𝑐𝑖𝑑𝑠 𝑡ℎ𝑎𝑡 𝑐𝑜𝑚𝑝𝑟𝑖𝑠𝑒 𝑡ℎ𝑒 𝑚𝑜𝑑𝑒𝑙𝑙𝑒𝑑 𝑷𝑻𝑮𝟒
𝐽 : = 𝑀𝑎𝑥𝑖𝑚𝑢𝑚 𝑛𝑢𝑚𝑏𝑒𝑟 𝑜𝑓 𝑐𝑎𝑛𝑜𝑛𝑖𝑐𝑎𝑙 𝑝𝑇𝐺4 𝑓𝑜𝑟 "𝑖" 𝑎𝑚𝑖𝑛𝑜 𝑎𝑐𝑖𝑑𝑠
A dataset that comprises experimentally validated G4-forming mRNA segments of several genes (n = 99) was downloaded (http://scottgroup.med.usherbrooke.ca/G4RNA/) and used to investigate the distribution of G4 [16]. Genes which possess non-redundant RNA (R) sub sequences in the PCS are translated in 6 reading frames using an online tool (http://web.expasy.org/translate). The peptides generated are classified as those: ⅰ) with one or more uninterrupted stretch of N-terminal amino acids of length ≥7 aa (~A), ⅱ) with an in-frame termination signal designated as 'STOP' (~B) and ⅲ) without any termination signal, i.e., absence of a 'STOP' in their sequence (~C). The translated peptides are classified as "VALID" ((B∩A)∪(C∩A)) and then queried for matches with pTG4ij(7≤i≤20,j∈N). The PERL scripts that are required to parse and process the resulting data files have been developed in house and the pseudocode for the same is presented as additional information (Pseudocode, PS1: Supplementary Text 1).
This is done by examining the occurrence of PTG4 in amino acid/protein sequences of disordered regions (IDRs) and full-length proteins with disordered regions (IDPs). DisProt 7.0 (http://disprot.org), is a database of experimentally validated and non-redundant sequences of IDRs and IDPs [38]. The sequences (|IDR| = 1445;|IDP| = 800) that comprise these are queried for occurrences of pTG4ij(7≤i≤20,j∈N) (Supplementary Texts 2 and 3). A preliminary partitioning schema divides these datasets into two distinct subsets, i.e., #pTG4ij≥1 (PT+≡PPOS⊂{IDR, IDP}; (Def.6)) and #pTG4ij = 0 (PT-≡PNEG⊂{IDR, IDP}; (Def. 7)). The extent of co-occurrence of one or more SLiMSw≡SL (w = {1, 2, 3}) with pTG4ij (SL±∈{PPOS, PNEG}) (Defs.8 and 9) is then evaluated to infer relevance of PTG4 to misfolding induced proteostasis. The distribution of overlapping/shared sequences of amino acids ((zn)n≥2∈(pTG4ij∩SLiMSw); zn∈Z; ) (Def.10), is examined in protein sequences from taxonomically diverse organisms with ScanProsite (https://prosite.expasy.org/scanprosite). The proof behind this rationale is presented:
(zn)n≥2 ∈(pTG4ij∩SLiMSw)=((zn)n≥2∈pTG4ij)∩((zn)n≥2∈SLiMSw)Let zn=z′n and zn=z′′n.Rewriting =((z′n)n≥2∈pTG4ij)∩((z′′n)n≥2∈SLiMSw)=((z′n)n≥2,(z′′n)n≥2)=pTG4ij×SLiMSw |
𝒁 : = 𝑆𝑒𝑡 𝑜𝑓 𝑎𝑚𝑖𝑛𝑜 𝑎𝑐𝑖𝑑𝑠 (𝑧𝑛 ∈ 𝒁)
𝑝𝑇𝐺4𝑖𝑗 : = 𝐶𝑎𝑛𝑜𝑛𝑖𝑐𝑎𝑙 𝑎𝑚𝑖𝑛𝑜 𝑎𝑐𝑖𝑑 𝑓𝑜𝑟𝑚 𝑜𝑓 𝑷𝑻𝑮𝟒
𝑺𝑳𝒊𝑴𝑺 : = 𝑆𝑒𝑡 𝑜𝑓 𝑠ℎ𝑜𝑟𝑡 𝑙𝑖𝑛𝑒𝑎𝑟 𝑚𝑜𝑡𝑖𝑓𝑠 (𝑆𝐿𝑖𝑀𝑆𝑤 ∈ 𝑺𝑳𝒊𝑴𝑺)
𝑖, 𝑗, 𝑛, 𝑤 : = 𝐼𝑛𝑑𝑖𝑐𝑒𝑠 𝑜𝑓 𝑚𝑒𝑚𝑏𝑒𝑟𝑠 𝑜𝑓 𝒁, 𝑷𝑻𝑮𝟒, 𝑺𝑳𝒊𝑴𝑺
The indices utilized by this study to establish relevance of matched instances of various motifs/co-motifs in the peptide/protein sequences of interest include the accuracy (A), precision (P), recall (R) and the p-value. A 2X2 table which represents the categorized data (2.1.4) is constructed and used to compute various bioinformatics indices. This is outlined as under:
![]() |
𝑇𝑁 ≔ 𝑇𝑟𝑢𝑒 𝑛𝑒𝑔𝑎𝑡𝑖𝑣𝑒 (|𝒔𝑵𝑬𝑮 ∩ 𝑷𝑵𝑬𝑮| = |𝒔𝑵𝑬𝑮|) ≡ 𝑆𝐿−𝑃𝑇− (𝐷𝑒𝑓. 11)
𝐹𝑃 ≔ 𝐹𝑎𝑙𝑠𝑒 𝑝𝑜𝑠𝑖𝑡𝑖𝑣𝑒 (|𝑺𝑵𝑬𝑮 ∩ 𝑷𝑷𝑶𝑺| = |𝑺𝑵𝑬𝑮|) ≡ 𝑆𝐿−𝑃𝑇+ (𝐷𝑒𝑓. 12)
𝐹𝑁 ≔ 𝐹𝑎𝑙𝑠𝑒 𝑛𝑒𝑔𝑎𝑡𝑖𝑣𝑒 (|𝒔𝑷𝑶𝑺 ∩ 𝑷𝑵𝑬𝑮| = |𝒔𝑷𝑶𝑺|) ≡ 𝑆𝐿+𝑃𝑇− (𝐷𝑒𝑓. 13)
𝑇𝑃 ≔ 𝑇𝑟𝑢𝑒 𝑝𝑜𝑠𝑖𝑡𝑖𝑣𝑒 (|𝑺𝑷𝑶𝑺 ∩ 𝑷𝑷𝑶𝑺| = |𝑺𝑷𝑶𝑺|) ≡ 𝑆𝐿+𝑃𝑇+ (𝐷𝑒𝑓. 14)
The equations may then be written as:
(A)=(TN+TP/TN+FP+FN+TP)X100 | (4) |
(P)=(TP/FP+TP)X100 | (5) |
(R)=(TP/FN+TP)X100 | (6) |
The p-values for these analyses are computed by comparing the frequency of occurrence of all pTG4ij in a test sequence (ϕpTG4ij) with the same in randomly-generated (v∈V) sequences of similar lengths (ϕpTG4vij), i.e., 7-50 aa (1≤v≤10000) and > 50 aa (1≤v≤100000) (Pseudocode, PS2: Supplementary Text 1):
p−value=ϕpTG4vij/ϕpTG4ij=(v=|V|∑v=1i=21∑i=7j=J∑j=1pTG4vij)/(i=21∑i=7j=J∑j=1pTG4ij)=(v=|V|∑v=1i=21∑i=7j=J∑j=1pTG4vij/i=21∑i=7j=J∑j=1pTG4ij) | (7) |
The frequency of occurrence of overlapping sequences of amino acids ((zn)n≥2∈(pTG4ij∩SLiMSw); zn∈Z) in pre-compiled and curated protein sequences (ϕ(zn)) across taxa is compared with randomly chosen sequences of comparable lengths (ϕ(vzn); n = 5000). These are used to estimate statistical significance, i.e., p-value = ϕ(vzn)/ϕ(zn) (8).
The data presented discusses implementation of a model of short intra-strand TG4 for various values of α and β, populates PTG4 and establishes the equivalence TG4~PTG4. Co-occurrence and homology studies between PTG4 and the SLiMS in IDRs/IDPs and generic protein sequences across taxa are used to infer probable mechanisms of TG4~PTG4 facilitated misfolding-induced proteostasis.
An association-competent codon not only takes into account the presence of a Guanine residue, but also gives weightage to its position (Figures 1–3, Table 1). This schema partitions standard vertebrate codons into those with a high- (Ranks 1-4;α > 0.0000) or low- (Rank 5;α = 0.0000) propensity to form a contiguous cluster of Guanine residues (Figures 3 and 4, Table 1). Whilst, 'GGG' (Rank 1;α = 2.12) can associate with ({GGG, GxG, xGG, GGx, Gxx, xxG}) bilaterally (δ = 6;Ω = 2), 'GxG' (Rank 2; α = 2.0066) can do so only with 'GGG' (δ = 1;Ω = 2). On the other hand, the codon subsets 'GGx' and 'xGG' (Rank 3;α = 1.0132) can form two clusters of contiguous Guanine residues with 'GGG' and 'xGG'/ 'GGx' unilaterally (δ = 2;Ω = 1). Similarly, the subsets 'xxG' or 'Gxx' (Rank 4;α = 1.0022), can form contiguous Guanines with a single occurrence of 'GGG' (δ = 1;Ω = 1) (Figures 3 and 4, Table 1). Conversely, codons with either a single occurrence of a central Guanine residue 'xGx' or no Guanine residues 'xxx' (Rank 5;α = 0.0000) are unable to form the 'GGGG' and are excluded from this study (Figures 3 and 4, Table 1).
Rank | Codon set, Cardinality | Codon | γ | θ | δ | Ω | α=γ.θ.δ+Ω | aa |
1 | GGG, 1 | GGG | 0.02 | 1.00 | 6 | 2 | 2.1200 | Gly |
2 | GxG, 3 | GUG | 0.02 | 0.33 | 1 | 2 | 2.0066 | Val |
GCG | 0.02 | 0.33 | 1 | 2 | 2.0066 | Ala | ||
GAG | 0.02 | 0.33 | 1 | 2 | 2.0066 | Glu | ||
3 | xGG, 3 | UGG | 0.02 | 0.33 | 2 | 1 | 1.0132 | Trp |
CGG | 0.02 | 0.33 | 2 | 1 | 1.0132 | Arg | ||
AGG | 0.02 | 0.33 | 2 | 1 | 1.0132 | Arg | ||
3 | GGx, 3 | GGU | 0.02 | 0.33 | 2 | 1 | 1.0132 | Gly |
GGC | 0.02 | 0.33 | 2 | 1 | 1.0132 | Gly | ||
GGA | 0.02 | 0.33 | 2 | 1 | 1.0132 | Gly | ||
4 | xxG, 9 | UUG | 0.02 | 0.11 | 1 | 1 | 1.0022 | Leu |
UCG | 0.02 | 0.11 | 1 | 1 | 1.0022 | Ser | ||
UAG | 0.02 | 0.11 | 1 | 1 | 1.0022 | Ter | ||
CUG | 0.02 | 0.11 | 1 | 1 | 1.0022 | Leu | ||
CCG | 0.02 | 0.11 | 1 | 1 | 1.0022 | Pro | ||
CAG | 0.02 | 0.11 | 1 | 1 | 1.0022 | Gln | ||
AUG | 0.02 | 0.11 | 1 | 1 | 1.0022 | Met | ||
ACG | 0.02 | 0.11 | 1 | 1 | 1.0022 | Thr | ||
AAG | 0.02 | 0.11 | 1 | 1 | 1.0022 | Lys | ||
4 | Gxx, 9 | GUU | 0.02 | 0.11 | 1 | 1 | 1.0022 | Val |
GCU | 0.02 | 0.11 | 1 | 1 | 1.0022 | Ala | ||
GAU | 0.02 | 0.11 | 1 | 1 | 1.0022 | Asp | ||
GUC | 0.02 | 0.11 | 1 | 1 | 1.0022 | Val | ||
GCC | 0.02 | 0.11 | 1 | 1 | 1.0022 | Ala | ||
GAC | 0.02 | 0.11 | 1 | 1 | 1.0022 | Asp | ||
GUA | 0.02 | 0.11 | 1 | 1 | 1.0022 | Val | ||
GCA | 0.02 | 0.11 | 1 | 1 | 1.0022 | Ala | ||
GAA | 0.02 | 0.11 | 1 | 1 | 1.0022 | Glu | ||
5 | xGx, 9 | UGU | 0.02 | 0.11 | 0 | 0 | 0.0000 | Cys |
UGC | 0.02 | 0.11 | 0 | 0 | 0.0000 | Cys | ||
UGA | 0.02 | 0.11 | 0 | 0 | 0.0000 | Ter | ||
CGU | 0.02 | 0.11 | 0 | 0 | 0.0000 | Arg | ||
CGC | 0.02 | 0.11 | 0 | 0 | 0.0000 | Arg | ||
CGA | 0.02 | 0.11 | 0 | 0 | 0.0000 | Arg | ||
AGU | 0.02 | 0.11 | 0 | 0 | 0.0000 | Ser | ||
AGC | 0.02 | 0.11 | 0 | 0 | 0.0000 | Ser | ||
AGA | 0.02 | 0.11 | 0 | 0 | 0.0000 | Arg | ||
5 | xxx, 27 | UUU | 0.02 | 0.04 | 0 | 0 | 0.0000 | Phe |
UCU | 0.02 | 0.04 | 0 | 0 | 0.0000 | Ser | ||
UAU | 0.02 | 0.04 | 0 | 0 | 0.0000 | Tyr | ||
UUC | 0.02 | 0.04 | 0 | 0 | 0.0000 | Phe | ||
UCC | 0.02 | 0.04 | 0 | 0 | 0.0000 | Ser | ||
UUA | 0.02 | 0.04 | 0 | 0 | 0.0000 | Leu | ||
UCA | 0.02 | 0.04 | 0 | 0 | 0.0000 | Ser | ||
UAA | 0.02 | 0.04 | 0 | 0 | 0.0000 | Ter | ||
CUU | 0.02 | 0.04 | 0 | 0 | 0.0000 | Leu | ||
CCU | 0.02 | 0.04 | 0 | 0 | 0.0000 | Pro | ||
CAU | 0.02 | 0.04 | 0 | 0 | 0.0000 | His | ||
CUC | 0.02 | 0.04 | 0 | 0 | 0.0000 | Leu | ||
CCC | 0.02 | 0.04 | 0 | 0 | 0.0000 | Pro | ||
CAC | 0.02 | 0.04 | 0 | 0 | 0.0000 | His | ||
CUA | 0.02 | 0.04 | 0 | 0 | 0.0000 | Leu | ||
CCA | 0.02 | 0.04 | 0 | 0 | 0.0000 | Pro | ||
CAA | 0.02 | 0.04 | 0 | 0 | 0.0000 | Gln | ||
AUU | 0.02 | 0.04 | 0 | 0 | 0.0000 | Ile | ||
ACU | 0.02 | 0.04 | 0 | 0 | 0.0000 | Thr | ||
AAU | 0.02 | 0.04 | 0 | 0 | 0.0000 | Asn | ||
AUC | 0.02 | 0.04 | 0 | 0 | 0.0000 | Ile | ||
ACC | 0.02 | 0.04 | 0 | 0 | 0.0000 | Thr | ||
AAC | 0.02 | 0.04 | 0 | 0 | 0.0000 | Asn | ||
AUA | 0.02 | 0.04 | 0 | 0 | 0.0000 | Ile | ||
ACA | 0.02 | 0.04 | 0 | 0 | 0.0000 | Thr | ||
AAA | 0.02 | 0.04 | 0 | 0 | 0.0000 | Lys |
Abbreviations
𝛾: General probability of a codon (𝛾 = 1/64 ≅ 0.02)
𝜃: Probability of codon within a group (𝜃 = {0.04, 0.33, 0.11, 1.00})
𝛿: Number of distinct codon sets that could complete 'GGGG' (𝛿 = {0, 1, 2, 6})
Ω: Number of adjacent positions that contain 𝛿 (Ω = {0, 1, 2})
𝛼: Threshold for selecting codons that may favour G-quadruplex formation
x: Codon specific generic ribonucleotide {𝐴, 𝐺, 𝑈, 𝐶}
aa: Amino acid
Ter: Stop codons {𝑈𝐴𝐺, 𝑈𝐺𝐴, 𝑈𝐴𝐴}
An estimate of the possible combinations of the simplest peptide (∑i=7∑j=Jj=1pTG4ij = 8.00E+03;GlyzGlyzGlyzGly; J = (20)3; i = lengthp(TG4ij) = 7 aa; z∈Z) (Figures 3 and 4, Table 2). This justifies usage of PTG4 (pTG4ij∈PTG4) as a generic representation of the putative peptidome encoded by the TG4 (PTG4). Approximately ~12% (n = 11) of in silico translated amino acid sequences from exon-derived TG4 possesses one of more "STOP" signals and include ESR1, longer RNA variants of PRNP (85 nt) and BCL2 (29 n t, 33 nt, 34 nt) (Table 3; Table 1, Supplementary Text 2). With the exceptions of KCNH2/ZNF669 and the shorter variants of PRNP (14 nt, 15 nt, 20 nt, 24 nt), "VALID" sub sequences are found for BACE1, BCL2, ESR1, PRNP (long) and TERF2 (Table 3; Tables 1A and 1C). Interestingly, all the genes considered possessed at least one occurrence of PTG4 (P = 100%, n = 6) (Table 3; Table 1B). This finding, despite the small sample size is proof-of-principal that the TG4 can be mapped to definite peptide sequences, i.e., TG4~PTG4. Since this can occur only after a ribosomal read through of the G4 containing mRNA, it raises the intriguing possibility that PTG4 whence part of a larger protein may increase its propensity to undergo misfolding. This notion is investigated in non-redundant sequences of IDRs (PTG4~10%, n = 145;0.00≤p-value≤0.20) and IDPs (PTG4~34%, n = 269;0.00≤p-value < 0.5) (Table 4; Tables 2 and 3).
aa | CODamino | gCOD+amino | β | |
Group 1 (n=7) | Ala | 4 | 4 | 1.00 |
Val | 4 | 4 | 1.00 | |
Asp | 2 | 2 | 1.00 | |
Glu | 2 | 2 | 1.00 | |
Trp | 1 | 1 | 1.00 | |
Met | 1 | 1 | 1.00 | |
Gly | 4 | 4 | 1.00 | |
Group 2 (n=7) | Leu | 6 | 2 | 0.3333 |
Gln | 2 | 1 | 0.5 | |
Arg | 6 | 2 | 0.3333 | |
Lys | 2 | 1 | 0.5 | |
Ser | 6 | 1 | 0.1667 | |
Thr | 4 | 1 | 0.25 | |
Pro | 4 | 1 | 0.25 | |
Group 3 (n=6) | Cys | 2 | 0 | 0.00 |
Asn | 2 | 0 | 0.00 | |
Ile | 3 | 0 | 0.00 | |
His | 2 | 0 | 0.00 | |
Phe | 2 | 0 | 0.00 | |
Tyr | 2 | 0 | 0.00 |
Abbreviations
gCOD+amino : Guanine-containing optimal codons excluding STOP (UAG) (𝛼 > 0.000)
COD−amino : Non-optimal codon excluding STOP (UGA, UAA) (𝛼 = 0.000)
𝑪𝑶𝑫𝒂𝒎𝒊𝒏𝒐 = gCOD+amino + COD−amino : All codons for an amino acid
GENE | NAME | G4 (nt) | Ex | STOP(n=11) | VALID(n=59) | |PTG4| |
BACE1 | Beta-secretase 1 | 33 | 3 | n=0 | n=6 | n=2 |
BCL2 | B-cell lymphoma 2 | 33 | 2 | n=1 | n=6 | n=1 |
23 | n=0 | n=6 | ||||
28 | n=0 | n=6 | ||||
29 | n=1 | n=5 | ||||
34 | n=1 | n=5 | ||||
33 | 3 | n=2 | n=5 | |||
ESR1 | Estrogen receptor alpha (ERα) | 36 | 4 | n=1 | n=5 | n=2 |
KCNH2 | Potassium Voltage-Gated Channel sub family H | 18 | 12 | n=0 | n=0 | NA |
ZNF669 | Member 2 Zinc Finger Protein 669 |
1 | ||||
PRNP | Prion protein | 14 | 2 | n=0 | n=0 | n=1 |
15 | n=0 | n=0 | ||||
20 | n=0 | n=0 | ||||
24 | n=0 | n=6 | ||||
85 | n=6 | n=3 | ||||
TERF2 | Telomeric repeat-binding factor 2 | 55 | 1 | n=0 | n=6 | n=1 |
Disordered regions (IDRs; n=1445;0.00≤p-value < 0.05) | |||||||||||||||||
SL-PT- | SL-PT+ | SL+PT- | SL+PT+ | R1T | R2T | C1T | C2T | A (%) | P (%) | R (%) | |||||||
SLiMS1 | 1078 | 64 | 58 | 9 | 1142 | 67 | 1136 | 73 | 89.90 | 12.32 | 13.43 | ||||||
SLiMS2 | 749 | 18 | 121 | 29 | 767 | 150 | 870 | 47 | 84.84 | 61.70 | 19.33 | ||||||
SLiMS3 | 1212 | 108 | 34 | 9 | 1320 | 43 | 1246 | 117 | 89.58 | 7.69 | 20.93 | ||||||
Proteins with disordered segments (IDPs; n=800;0.00≤p-value < 0.05) | |||||||||||||||||
SL-PT- | SL-PT+ | SL+PT- | SL+PT+ | R1T | R2T | C1T | C2T | A (%) | P (%) | R (%) | |||||||
SLiMS1 | 86 | 12 | 28 | 18 | 98 | 46 | 114 | 30 | 72.22 | 60.00 | 39.10 | ||||||
SLiMS2 | 1 | 1 | 96 | 66 | 2 | 162 | 97 | 67 | 40.85 | 98.50 | 40.74 | ||||||
SLiMS3 | 250 | 57 | 26 | 25 | 307 | 51 | 276 | 82 | 76.81 | 30.48 | 49.01 |
Abbreviations
𝐼𝐷𝑅𝑠: Intrinsically disordered regions
𝐼𝐷𝑃s: Intrinsically disordered proteins
𝑧: Any amino acid
𝑆𝐿𝑖𝑀𝑆1: [𝑆𝑇]𝑃𝑧𝑅
𝑆𝐿𝑖𝑀𝑆2: [𝐸𝐷]𝑧𝑧[𝐷𝐸][𝐴𝐺𝑆]
𝑆𝐿𝑖𝑀𝑆3: [𝐾𝑅]𝑧𝑃𝑧𝑧𝑃
𝑆𝐿−𝑃𝑇−: |𝑺𝑵𝑬𝑮 ∩ 𝑷𝑵𝑬𝑮|
𝑆𝐿−𝑃𝑇+: |𝑺𝑵𝑬𝑮 ∩ 𝑷𝑷𝑶𝑺|
𝑆𝐿+𝑃𝑇−: |𝑺𝑷𝑶𝑺 ∩ 𝑷𝑵𝑬𝑮|
𝑆𝐿+𝑃𝑇+: |𝑺𝑷𝑶𝑺 ∩ 𝑷𝑷𝑶𝑺|
𝑅1𝑇: 𝑆𝐿−𝑃𝑇− + 𝑆𝐿−𝑃𝑇+
𝑅2𝑇: 𝑆𝐿+𝑃𝑇− + 𝑆𝐿+𝑃𝑇+
𝐶1𝑇: 𝑆𝐿−𝑃𝑇−+ 𝑆𝐿+𝑃𝑇−
𝐶2𝑇: 𝑆𝐿−𝑃𝑇+ + 𝑆𝐿+𝑃𝑇+
𝐴: Accuracy
𝑃: Precision
𝑅: Recall
The amino acids that comprise the peptide members of PTG4 and the short linear motifs (g1, g2 vs SLiMS) are well conserved. The co-occurrence of PTG4 with SLiMS in the IDRs (A~85-89%;0.00 < p-value≤0.05) suggests that this association is non-trivial and may favor all purported mechanisms of misfolding (hyperphosphorylation, proteolytic cleavage, complex formation) (Table 4; Tables 2 and 3). However, the higher precision of PTG4 with the proteolytic-SLiMS suggests that this may predominate (Table 4; Tables 2 and 3). The data with the IDPs suggests a similar predilection for proteolytic cleavage (A~40-77%;P~99%;0.00 < p-value < 0.05, although hyperphosphorylation (P~60%;0.00 < p-value < 0.05) and complex-promotion (P~30%;0.00 < p-value < 0.05) may constitute viable alternatives to the genesis of misfolding (Table 4; Tables 2 and 3). The presence of overlapping sequences of amino acids between PTG4 and the SLiMS when examined in protein sequences from taxonomically diverse organisms is degenerate for SLiMS1 (number of matches = 6251) and SLiMS3 (number of matches = 1480) (Table 5; Table 4). In contrast, the corresponding data for SLiMS2 (number ofmatches = 3759;0.00 < p-value < 0.05) is statistically significant (Table 5). The taxonomic spread includes archaea (n = 150), bacteria (n = 1735), viruses (n = 84), green land plants (n = 199), fungi (n = 182), eukaryotic invertebrates (n = 43) and vertebrates (n = 700) (Table 4).
SLiMS | Sample | (zn)n≥2∈pTG4ij∩SLiMSw (p-value) | |
SLiMS1=[ST]PzR | Pz | PG (n=1) | PG (Degenerate) |
SLiMS2=[ED]zzD[AGS] | z[DE] | G[DE] (n=2) | [WGRVAELMKQSTP][AG][DE]z2EG[VADE](p-value=0.00069) |
[DE]z | [DE]G (n=1) | ||
zz[DE] | [LMKQSTP]G[DE] (n=14) [VAE]G[DE] (n=6) [WGR][AG][DE] (n=6) |
||
[DE]zz[DE] | [VAE]G[DE]zzEG[VADE] (n=28) [WGR][AG][DE]zzEG[VADE] (n=24) [WGR][AG][DE]zzEG (n=6) GEzzEG[VADE] (n=4) GEzzEG (n=1) |
||
SLiMS3=[KR]zPzzP | Pzz | PG[VADE] (n=4) | PGV (Degenerate) PGA (Degenerate) PGD (Degenerate) PGE (Degenerate) |
Abbreviations
𝑝𝑇𝐺4𝑖𝑗: Members of putative peptidome (𝑝𝑇𝐺4𝑖𝑗 ∈ 𝑷𝑻𝑮𝟒)
𝑆𝐿𝑖𝑀𝑆𝑤: Short linear motifs (𝑆𝐿𝑖𝑀𝑆𝑤 ∈ 𝑺𝑳𝒊𝑴𝑺)
𝑧𝑛: Shared sequence(s) of amino acids between 𝑷𝑻𝑮𝟒 and 𝑺𝑳𝒊𝑴𝑺
𝑖, 𝑗, 𝑤, 𝑛: Indices to characterize members of 𝑷𝑻𝑮𝟒, 𝑺𝑳𝒊𝑴𝑺, 𝒁
The significant association and homology between PTG4 and the SLiMS along with the equivalence data (PTG4~TG4) suggest that TG4 may influence proteostasis in a multitude of ways (Tables 1–5; Tables 1–4, Supplementary Text 2–4).
The short TG4 modeled in this study has an average loop length (h~2 Mer) which may contribute to thermodynamic stability by restricting the mobility of the participating strands (1) [8,9,10,11]. The physical presence of TG4 will result in a stalled ribosome and translation which is prolonged, inefficient and incomplete [31,32,33]. Interestingly, this analysis also includes UAG (Amber; α > 0.0000), which when present in-frame will prematurely terminate translation and result in a truncated protein (Table 1) [39]. Whilst nonsense-mediated mRNA decay may be triggered if the stop codon is within ±50 Mer of the exon-junction complex (EJC), a read-through may occur nonetheless. The resulting protein sequences may be modified which in tandem with one or more occurrences of PTG4 and/or SLiMS would predispose the same to agammaegate and result in a proteopathy [39,40].
Whilst the preponderance of Glycine (Gly) might impart heightened flexibility and limit the formation of stabilizing secondary structural elements in the hypothetical protein, Proline (Pro) confers rigidity and may retard proper folding. There is also remarkable conservation between the amino acids that comprise PTG4 and the SLiMS. These include the complex-promoting hydrophobic (Ala, Val, Met, Trp) and ionic (Asp, Glu, Lys, Arg) residues, along with nucleophile-favoring Serine and Threonine (Figures 3 and 4, Tables 2–5). Whilst, the former may favor agammaegation by non-covalent interactions, the latter may promote phosphorylation-mediated charge imbalance and thence misfolding. Interestingly, the loops of G4 whence modeled by Adenine-containing codons (Axx) are translated to Lysine (K), Arginine (R), Serine (S), Threonine (T) and Isoleucine (I); all of which may also promote misfolding (Figures 3 and 4, Tables 2-5) [8,9,10,11,34,35]. The distribution of PTG4 amongst physiologically relevant proteins further suggests that the peptide-mediated misfolding may influence/regulate signal transduction, cytoskeleton organization, metabolism, synaptic transmission and transcription/translation (Table 6; Table 5).
Cellular function | Disordered regions of proteins | |
1. | Signal transduction | DP00274, DP00224, DP00141, DP00332, DP01063, DP00506, DP00418, DP00341, DP00435, DP00613, DP00463, DP00954, DP00959, DP01104, DP00611, DP00519, DP00086, DP00707, DP00712 |
2. | Endocytosis | DP01073, DP01065, DP01066, DP00225 |
3. | Calcium-calmodulin | DP00092, DP00132, DP00561, DP00118, DP00253 |
4. | Myofibril assembly | DP01090 |
5. | Cytoskeleton | DP01056 DP00240, DP01022, DP00169, DP00716, DP00717, DP01100, DP00122 |
6. | Nuclear pore | DP01075, DP01077, DP01079 |
7. | Phototransduction | DP00768, DP00347 |
8. | Targeting | DP00893, DP00609, DP00610, DP01058 |
9. | Transcription | DP00062, DP00177, DP00633, DP00348, DP00786, DP00049, DP00231, DP00873, DP00720, DP00217, DP00081 |
10. | Translation | DP00082, DP00164, DP00229 DP00949, DP00134 |
11. | Synaptic transmission | DP00943 |
12. | Supercoiling | DP00076 |
13. | Binding | DP00539, DP00854, DP01052, DP00659, DP00656 |
14. | Peptide bond formation | DP00944 |
15. | Enzymes | DP00557, DP00032, DP00095, DP00337, DP00379, DP00787, DP00427, DP00429 |
16. | Bacterial/parasitic virulence | |
Secreted toxins | DP00345, DP00591 | |
Cytoadherence | DP00025, DP00065, DP01096 | |
17. | Viral infectivity | |
Cyclophilin interaction | DP00615, DP01031 | |
Chaperones | DP00699, DP00700, DP00674 | |
Capsid assembly | DP00133, DP00876 | |
Membrane fusion | DP01043 | |
Latency | DP01060 | |
18. | Unknown | DP00119 |
Note: DP≔DisProt ID
The distribution of overlapping/shared amino acids in protein sequences of non-vertebrates suggests that PTG4 is either completely degenerate with the SLiMS or present in proportions that is statistically significant (Tables 5 and 6; Tables 4 and 5). These data imply that motif-mimicry too, might constitute a probable cause (tropism, oncogenic potential, virulence) of infection/infestation-mediated acute/chronic proteopathies [34,35,41,42]. The contribution(s) of misfolding to the pathogenesis of secondary proteopathies is however, debatable. Whilst, there is evidence that mislocalization of proteins can precipitate misfolding, mimicry itself may result in exonuclease-mediated proteolytic cleavage and thence trigger an infective proteopathy [43,44]. Additionally, the presence of sequences of amino acids such as Proline and Threonine in viral or fungal proteins may be responsible for creating and/or maintaining a milieu conducive to the genesis of infective/transmissible proteopathies, viz., a high charge density and imbalance of electrostatic interactions [43,44].
The coexistence of potentially translatable G-quadruplexes (TG4) with unfolded ribonucleotides in the PCS of an mRNA transcript may have important consequences for protein homeostasis. Here, I have investigated the contribution of a short intra-strand translatable G-quadruplex and its associated peptidome (TG4~PTG4) to the genesis of misfolding-induced proteostasis. The co-occurrence, homology and distribution of overlapping/shared amino acids of PTG4 with the SLiMS suggests that this may occur by truncation, complex formation, increased charge density and/or accelerated degradation. An additional mechanism that is also supported is motif-mimicry by pathogens which may trigger the development of infective proteopathies. The putative peptidome (~7–20 aa) that corresponds to the short translatable G-quadruplex delineated by this investigation may be utilized as novel markers of both the primary and secondary proteopathies.
SK outlined and designed the study, designed and conceptualized the algorithm(s) and formulae for prediction, wrote mathematical proofs to establish rigor, collated the data, constructed the models, formulated the filters, carried out the computational analysis, wrote all necessary code and the manuscript.
The author declares no conflict of interest.
[1] | Ohio Department of Development (ODD) Population and Housing: Ohio's population; Ohio Development Services Agency, 2010. Available from: https://development.ohio.gov/reports/reports_pop_proj_map.htm. |
[2] |
Tong STY, Sun Y, Ranatunga T, et al. (2012) Predicting plausible impacts of sets of climate and land use change scenarios on water resources. Appl Geogr 32: 477–489. doi: 10.1016/j.apgeog.2011.06.014
![]() |
[3] | Debrewer LM, Rowe GL, Reutter DC, et al. Environmental setting and effects on water quality in the Great and Little Miami River basins, Ohio and Indiana: Water-Resources Investigations Report, 2000. Available from: https://pubs.usgs.gov/wri/1999/4201/report.pdf. |
[4] | OEPA biological and water quality study of the Upper Little Miami River. Clark, Clinton, Greene, Madison, Montgomery, and Warren Counties, Ohio. OHIO EPA Technical Report: Division of Surface Water, 2011. Available from: https://epa.ohio.gov/Portals/35/documents/LMR_Upper_Basin_2011_TSD.pdf. |
[5] | Pitt R, Bozeman M. Water quality and biological effects of urban runoff in Coyote Creek, U.S. Ohio Environmental Protection Agency, 1980. Available from: https://nepis.epa.gov/Exe/ZyPURL.cgi?Dockey=30000C3M.TXT. |
[6] |
Tong STY (1990) The hydrologic effects of urban land use: A case study of the little Miami River Basin. Landsc and Urban Plan 19: 99–105. doi: 10.1016/0169-2046(90)90037-3
![]() |
[7] |
Turner RE, Rabalais NN (2004) Suspended sediment, C, N, P, and Si yields from the Mississippi River Basin. Hydrobiologia 511: 79–89. doi: 10.1023/B:HYDR.0000014031.12067.1a
![]() |
[8] | Rowe GL, Reutter DC, Runkle DL, et al. Water quality in the Great and Little Miami River Basins, Ohio and Indiana, 1999–2001, USGS, 2004. Available from: https://pubs.er.usgs.gov/publication/cir1229. |
[9] |
Kaushal SS, Groffman PM, Band LE, et al. (2008) Interaction between urbanization and climate variability amplifies watershed nitrate export in Maryland. Environ Sci Technol 42: 5872–5878. doi: 10.1021/es800264f
![]() |
[10] | OEPA Nutrient Mass Balance Study for Ohio's Major Rivers, Ohio Environmental Protection Agency, Division of Surface Water Modeling, Assessment and TMDL Section, 2016. Available from: https://epa.ohio.gov/Portals/35/documents/Nutrient%20Mass%20Balance%20Study%202018_F inal.pdf. |
[11] |
Yu S, Yu GB, Liu Y, et al. (2012) Urbanization impairs surface water quality: Eutrophication and metal stress in the Grand Canal of China. River Res Appl 28: 1135–1148. doi: 10.1002/rra.1501
![]() |
[12] | EPA National Nonpoint Source Program-a catalyst for water quality improvements, 2017. Available from: https://www.epa.gov/sites/production/files/2016-10/documents/nps_program_highlights_report-508.pdf. |
[13] | MCD Lower Great Miami River Nutrient Management Project, 2017. Available from: https://www.mcdwater.org/wp-content/uploads/2017/06/2017-LGMR_Report-FINAL_02-28-17_Compressed_Graphics.pdf. |
[14] |
Naramngam S, Tong STY (2013) Environmental and economic implications of various conservative agricultural practices in the Upper Little Miami River basin. Agric Water Manag 119: 65–79. doi: 10.1016/j.agwat.2012.12.008
![]() |
[15] |
Paul MJ, Meyer JL (2001) Streams in the urban landscape. Annu Rev Ecol Syst 32: 333–365. doi: 10.1146/annurev.ecolsys.32.081501.114040
![]() |
[16] | Center for Watershed Protection, Impacts of impervious cover on aquatic ecosystems, Watershed Protection Research Monograph No. 1. Center for Watershed Protection, 2003. Available from: https://clear.uconn.edu/projects/TMDL/library/papers/Schueler_2003.pdf. |
[17] | OEPA Ohio Nutrient Reduction Strategy, Ohio Environmental Protection Agency, 2013. Available from: https://epa.ohio.gov/Portals/35/wqs/ONRS_final_jun13.pdf. |
[18] |
Townsend AR, Howarth RW, Bazzaz FA, et al. (2003) Human health effects of a changing global nitrogen cycle. Front Ecol Environ 1: 240–246. doi: 10.1890/1540-9295(2003)001[0240:HHEOAC]2.0.CO;2
![]() |
[19] | Newcomber G, House J, Ho L, et al. Management strategies for cyanobacteria (blue-green algae) and their toxins: A guide for water utilities. Water Quality Research Australia Limited, 2009. Available from: https://www.researchgate.net/profile/Lionel_Ho/publication/242740698_Management_Strategie s_for_Cyanobacteria_Blue-Green_Algae_A_Guide_for_Water_Utilities/links/02e7e52d62273e8f70000000/Management-Strategies-for-Cyanobacteria-Blue-Green-Algae-A-Guide-for-Water-Utilities.pdf. |
[20] |
Graham J, Loftin K, Meyer M, et al. (2010) Cyanotoxin mixtures and taste-and-odor compounds in cyanobacterial blooms from the Midwestern United States. Environ Sc. Technol 44: 7361–7368. doi: 10.1021/es1008938
![]() |
[21] | MCD nitrogen and phosphorus concentrations and loads in the Great Miami River Watershed, Ohio 2005 – 2011, 2012. Available from: https://www.mcdwater.org/wp-content/uploads/PDFs/2012NutrientMonitoringReport_Final.pdf. |
[22] | OEPA biological and water quality study of the Middle and Lower Great Miami River and selected tributaries, 1995-Volume I, Ohio Environmental Protection Agency, 1997. Available from: https://epa.ohio.gov/portals/35/documents/lmgmr95.pdf. |
[23] | Smith JH. Miami River algae draws local attention, 2011. Available from: https://www.daytondailynews.com/news/local/miami-river-algae-draws-local- attention/Rh715SpRPOMoJsF6qMyghK. |
[24] | NPS Nonpoint Source Management Plan Update, Ohio Environmental Protection Agency, 2018. Available from: https://www.epa.ohio.gov/portals/35/nps/nps_mgmt_plan.pdf. |
[25] | OEPA Integrated Water Quality Monitoring and Assessment Report, Ohio Environmental Protection Agency, 2018. Available from: https://www.epa.ohio.gov/Portals/35/tmdl/2018intreport/2018IR_Final.pdf. |
[26] | Takle ES, Xia M, Guan F, et al. (2006) Upper Mississippi River basin modeling system part 4: climate change impacts on flow and water quality, In: Coastal Hydrology and Processes, Water Resources Publications, 135–142. |
[27] | Luo Y, Ficklin DL, Liu X, et al. (2013) Assessment of climate change impacts on hydrology and water quality with a watershed modeling approach. Sci Total Environ 450–451: 72–82. |
[28] |
Ekness P, Randhir TO (2015) Effect of climate and land cover changes on watershed runoff: A multivariate assessment for storm water management. J Geophys Res-Geosciences 120: 1785–1796. doi: 10.1002/2015JG002981
![]() |
[29] |
Rahman K, da Silva AG, Tejeda EM (2015) An independent and combined effect analysis of land use and climate change in the upper Rhone River watershed, Switzerland. Appl Geogr 63: 264–272. doi: 10.1016/j.apgeog.2015.06.021
![]() |
[30] |
Li Z, Fang H (2017) Modeling the impact of climate change on watershed discharge and sediment yield in the black soil region, northeastern China. Geomorphology 293: 255–271. doi: 10.1016/j.geomorph.2017.06.005
![]() |
[31] |
Barnett TP, Adam JC, Lettenmaier DP (2005) Potential impacts of a warming climate on water availability in snow-dominated regions. Nature 438: 303–309. doi: 10.1038/nature04141
![]() |
[32] |
Wang GQ, Zhang JY, Xuan YQ (2013) Simulating the Impact of Climate Change on Runoff in a Typical River Catchment of the Loess Plateau, China. J Hydrometeor 14: 1553–1561. doi: 10.1175/JHM-D-12-081.1
![]() |
[33] |
Barranco LM, Álvarez-Rodríguez J, Olivera F (2014) Assessment of the expected runoff change in Spain using climate simulations. J Hydrol Eng 19: 1481–1490. doi: 10.1061/(ASCE)HE.1943-5584.0000920
![]() |
[34] |
Demaria EMC, Roundy JK, Wi S (2016) The effects of climate change on seasonal snowpack and the hydrology of the Northeastern and Upper Midwest United States. J Climate 29: 6527–6541. doi: 10.1175/JCLI-D-15-0632.1
![]() |
[35] | Wagena MB, Collick AS, Ross AC (2018) Impact of climate change and climate anomalies on hydrologic and biogeochemical processes in an agricultural catchment of the Chesapeake Bay watershed, USA. Sci Total Environ 637–638: 1443–1454. |
[36] |
Gleick PH (1989) Climate change, hydrology, and water resources. Rev Geophys 27: 329–344. doi: 10.1029/RG027i003p00329
![]() |
[37] |
Dyer F, ElSawah S, Croke B, et al. (2014) The effects of climate change on ecologically-relevant flow regime and water quality attributes. Stoch Environ Res Risk Assess 28: 67–82. doi: 10.1007/s00477-013-0744-8
![]() |
[38] |
Ferrer J, Pérez-Martín MA, Jiménez S, et al. (2012) GIS-based models for water quantity and quality assessment in the Júcar River Basin, Spain, including climate change effects. Sci Total Environ 440: 42–59. doi: 10.1016/j.scitotenv.2012.08.032
![]() |
[39] | Van Liew M, Feng S, Pathak T (2013) Assessing climate change impacts on water balance, runoff, and water quality at the field scale for four locations in the heartland. Transactions of the ASABE 56: 833–900. |
[40] |
Butterbach-Bahl K, Dannenmann M (2011) Denitrification and associated soil N2O emissions due to agricultural activities in a changing climate. Curr Opin Env Sust 3: 389–395. doi: 10.1016/j.cosust.2011.08.004
![]() |
[41] | OEPA Ohio Nutrient Reduction Strategy, Ohio Environmental Protection Agency, 2016. Available from: https://epa.ohio.gov/Portals/35/wqs/ONRS_addendum.pdf. |
[42] | OEPA Ohio water resource inventory, executive summary: Summary, conclusions, and recommendations Division of Surface Water and Monitoring Assessment Section, Ohio Environmental Protection Agency, 1996. Available from: https://www.epa.ohio.gov/portals/35/documents/exsumm96.pdf. |
[43] |
Tong STY, Liu AJ, Goodrich JA (2007) Climate change impacts on nutrient and sediment loads in a Midwestern agricultural watershed. J Environ Inform 9: 18–28. doi: 10.3808/jei.200700084
![]() |
[44] | Rowe GL, Baker NT. National Water-Quality Assessment Program, Great and Little Miami River Basins, 1997. Available from: https://pubs.water.usgs.gov/circ1229. |
[45] | Cross WP, Drainage areas of Ohio streams, supplement to Gazetteer of Ohio streams; Ohio Water Plan Inventory Report: Ohio Department of Natural Resources, 1967, 61. |
[46] | OEPA East Fork Little Miami River comprehensive water quality report, Little Miami River Basin, Clinton, Highland, Brown, and Clermont Counties: Ohio Environmental Protection Agency, 1985. Available from: http://msdgc.org/downloads/initiatives/water_quality/2012_lmr_biological_water_quality_study. pdf. |
[47] | Rankin ET, Yoder CO, Mishne D. Ohio water resources inventory executive summary-Summary, conclusions and recommendation: Ohio Environmental Protection Agency, 1996, 75 p. Available from: https://www.epa.ohio.gov/portals/35/documents/exsumm96.pdf. |
[48] | Miami Conservancy District Flood protection dams: Miami Conservancy District, 2018. Available from: http://www.conservancy.com/dams.asp#dams. |
[49] | National Research Council Alternative agriculture, National Academy Press, 1989. Available from: https://www.nap.edu/catalog/1208/alternative-agriculture. |
[50] |
Dickey E, Shelton DP, Jasa P, et al. (1985) Soil erosion from tillage systems used in soybean and corn residues. T ASAE 28: 1124–1130. doi: 10.13031/2013.32399
![]() |
[51] | Walters D, Jasa P. Conservation tillage in the United States: An overview, 2018. Available from: http://agecon.okstate.edu/isct/labranza/walters/conservation.doc. |
[52] |
Tebrügge F, Düring R-A (1999) Reducing tillage intensity - a review of results from a long-term study in Germany. Soil Tillage Res 53: 15–28. doi: 10.1016/S0167-1987(99)00073-2
![]() |
[53] |
Armand R, Bockstaller C, Auzet A-V, et al. (2009) Runoff generation related to intra-field soil surface characteristics variability: Application to conservation tillage context. Soil Tillage Res 102: 27–37. doi: 10.1016/j.still.2008.07.009
![]() |
[54] |
Borie F, Rubio R, Ruanet JL, et al. (2006) Effects of tillage systems on soil characteristics, glomalin and mycorrhizal propagules in a Chilean Ultisol. Soil Tillage Res 88: 253–261. doi: 10.1016/j.still.2005.06.004
![]() |
[55] |
Rocha EO, Calijuri ML, Santiago AF, et al. (2012) The contribution of conservation practices in reducing runoff, soil loss, and transport of nutrients at the watershed level. Water Resour Manage 26: 3831–3852. doi: 10.1007/s11269-012-0106-1
![]() |
[56] |
Jarecki MK, Lal R (2003) Crop management for soil carbon sequestration. Crit Rev Plant Sci 22: 471–502. doi: 10.1080/713608318
![]() |
[57] | Mangalassery S, Sjögersten S, Sparkes DL, et al. (2014) To what extent can zero tillage lead to a reduction in greenhouse gas emissions from temperate soils? Sci Rep 4: 4586. |
[58] | Baker NT. Tillage practices in the conterminous United States, 1989–2004-Datasets aggregated by watershed, USGS, 2011, 22 p. Available from: https://pubs.usgs.gov/ds/ds573/pdf/dataseries573final.pdf. |
[59] |
Cox WJ, Zobel RW, van Es HM, et al. (1990) Growth development and yield of maize under three tillage systems in the northeastern U.S.A. Soil Tillage Res 18: 295–310. doi: 10.1016/0167-1987(90)90067-N
![]() |
[60] |
Ruffo ML, Bollero GA (2003) Modeling rye and hairy vetch residue decomposition as a function of degree-days and decomposition-days. Agron J 95: 900–907. doi: 10.2134/agronj2003.9000
![]() |
[61] |
Findlater PA, Carter DJ (1990) A model to predict the effects of prostrate ground cover on wind erosion. Aust J Soil Res 28: 609–622. doi: 10.1071/SR9900609
![]() |
[62] | Staver KW, Brinsfield RB (1998) Using cereal grain winter cover crops to reduce groundwater nitrate contamination in the mid-Atlantic coastal plain. J Soil Water Conserv 53: 230–240. |
[63] |
Whish JPM, Price L, Castor PA (2009) Do spring cover crops rob water and so reduce wheat yields in the northern grain zone of eastern Australia? Crop Pasture Sci 60: 517–525. doi: 10.1071/CP08397
![]() |
[64] | Fageria NK, Baligar VC, Bailey BA (2018) Role of cover crops in improving soil and row crop productivity. Commun Soil Sci Plant Anal 36: 19–20. |
[65] | Hoorman JJ. Using cover crops to convert to no-till, 2018. Available from: https://ohioline.osu.edu/factsheet/SAG-11. |
[66] |
Dabney SM, Delgado JA, Reeves DW (2001) Using winter cover crops to improve soil and water quality. Commun Soil Sci Plant Anal 32: 1221–1250. doi: 10.1081/CSS-100104110
![]() |
[67] | SARE- CTIC Report of the 2013–2014 Cover Crop Survey. Joint publication of the Conservation Technology Information Center and the North Central Region Sustainable Agriculture Research and Education Program.; Conservation Technology Information Center: West Lafayette, Indiana, 2014. Available from: https://www.sare.org/Learning-Center/From-the-Field/North-Central-SARE-From-the-Field/2013-14-Cover-Crops-Survey-Analysis. |
[68] |
Creamer NG, Baldwin KR (2000) An evaluation of summer cover crops for use in vegetable production systems in North Carolina. HortScience 35: 600–603. doi: 10.21273/HORTSCI.35.4.600
![]() |
[69] | SARE- CTIC Cover Crop survey report. Conservation Technology Information Center. 2014. Report of the 2013-14 Cover Crop Survey. Joint publication of the Conservation Technology Information Center and the North Central Region Sustainable Agriculture Research and Education Program; Conservation Technology Information Center: West Lafayette, Indiana, 2015. Available from: https://www.sare.org/Learning-Center/From-the-Field/North-Central-SARE-From-the-Field/2016-Cover-Crop-Survey-Analysis. |
[70] | Nokes S, Ward A. Surface water quality best management practices summary guide: Columbus, The Ohio State University Extension Factsheet, 1997. Available from: http://epa.ohio.gov/dsw/storm/technical_guidance. |
[71] |
Gorham T, Jia Y, Shum CK, et al. (2017) Ten-year survey of cyanobacterial blooms in Ohio's waterbodies using satellite remote sensing. Harmful Algae 66: 13–19. doi: 10.1016/j.hal.2017.04.013
![]() |
[72] | Goolsby DA, Battaglin WA, Lawrence GB, et al. Flux and sources of nutrients in the Mississippi-Atchafalaya River Basin: Topic 3 Report for the Integrated Assessment on Hypoxia in the Gulf of Mexico, 1999. Available from: http://www.cop.noaa.gov/pubs/das/das17.pdf. |
[73] | Baker DB, Richards RP, Kramer JW. Point source-nonpoint source trading: applicability to stream TMDLs in Ohio. In Proceedings of the Proceedings – Innovations in Reducing Nonpoint Source Pollution, 2006, 328–337. |
[74] |
Scavia D, Justic D, Bierman VJ (2004) Reducing hypoxia in the Gulf of Mexico: Advice from three models. Estuaries 27: 419–425. doi: 10.1007/BF02803534
![]() |
[75] |
Smith RA, Schwarz GE, Alexander RB (1997) Regional interpretation of water-quality monitoring data. Water Resour Res 33: 2781–2798. doi: 10.1029/97WR02171
![]() |
[76] | Battaglin WA, Goolsby DA. Spatial data in geographic information system format on agricultural chemical use, land use, and cropping practices in the United States, 1995. Available from: https://pubs.usgs.gov/wri/1994/4176/report.pdf. |
[77] |
Rabalais NN, Turner RE, Díaz RJ, et al. (2009) Global change and eutrophication of coastal waters. ICES J Mar Sci 66: 1528–1537. doi: 10.1093/icesjms/fsp047
![]() |
[78] | Midwest Biodiversity Institute Biological and water quality assessment of the Great Miami River and tributaries 2013 Hamilton County, Ohio, 2014, 121. Available from: https://midwestbiodiversityinst.org/reports/biological-and-water-quality-study-of-the-little-miami-river-and-tributaries-2012-hamilton-county-ohio/LMR%202012%20MSDGC%20FINAL%20REPORT%2020130930.pdf. |
[79] | OEPA Integrated Water Quality Monitoring and Assessment Report: Ohio Environmental Protection Agency, 2002. Available from: https://www.epa.ohio.gov/portals/35/tmdl/2002IntReport/Ohio2002IntegratedReport_100102.pdf. |
[80] | MCD Water Data Report, Great Miami River Watershed, Ohio, 2015. Available from: https://www.mcdwater.org/wp-content/uploads/PDFs/2015-Water-Data-Report-FINAL-reduced.pdf. |
[81] | Hayhoe K, Edmonds J, Kopp RE, et al. (2017) Climate models, scenarios, and projections: Climate Science Special Report: Fourth National Climate Assessment; U.S. Global Change Research Program, 133–160. |
[82] | IPCC Climate Change 2014: Synthesis Report. Contribution of Working Groups I, II and III to the Fifth Assessment Report of the Intergovernmental Panel on Climate Change: Geneva, Switzerland,. Available from: https://www.ipcc.ch/report/ar5/syr. |
[83] | IPCC Contribution of Working Groups I, II and III to the Fourth Assessment Report of the Intergovernmental Panel on Climate Change Core Writing Team; Geneva, Switzerland, 2007. Available from: https://www.ipcc.ch/report/ar4/syr. |
[84] |
Meehl GA, Tebaldi C (2004) More intense, more frequent, and longer lasting heat waves in the 21st century. Science 305: 994–997. doi: 10.1126/science.1098704
![]() |
[85] |
Rosenzweig C, Major DC, Demong K, et al. (2007) Managing climate change risks in New York City's water system: assessment and adaptation planning. Mitig Adapt Strat Glob Change 12: 1391–1409. doi: 10.1007/s11027-006-9070-5
![]() |
[86] | Karl TR, Melillo JM, Peterson TC. Global climate change impacts in the United States, Cambridge University Press: UK, 2009. Available from: http://www.nrc.gov/docs/ML1006/ML100601201.pdf. |
[87] | Lawrimore JH, Menne MJ, Gleason BE, et al. (2011) An overview of the Global Historical Climatology Network monthly mean temperature data set, version 3. JGR Atmospheres 2011, 116. |
[88] |
Vörösmarty CJ, McIntyre PB, Gessner MO, et al. (2010) Global threats to human water security and river biodiversity. Nature 467: 555–561. doi: 10.1038/nature09440
![]() |
[89] | Lettenmaier DP, Milly PCD (2009) Land waters and sea level. Nature Geoscience 2009, 2, 3. |
[90] | Hatfield JL, Prueger JH (2004) Impacts of changing precipitation patterns on water quality. J Soil Water Conserv 59: 51–58. |
[91] |
Prowse TD, Beltaos S, Gardner JT, et al. (2006) Climate change, flow regulation and land-use effects on the hydrology of the Peace-Athabasca-Slave System; Findings from the Northern Rivers Ecosystem Initiative. Environ Monit Assess 113: 167–197. doi: 10.1007/s10661-005-9080-x
![]() |
[92] |
Zhang A, Zhang C, Fu G, et al. (2012) Assessments of impacts of climate change and human activities on runoff with SWAT for the Huifa River Basin, Northeast China. Water Resour Manage 26: 2199–2217. doi: 10.1007/s11269-012-0010-8
![]() |
[93] |
Ye L, Grimm NB (2013) Modelling potential impacts of climate change on water and nitrate export from a mid-sized, semiarid watershed in the US Southwest. Climatic Change 120: 419–431. doi: 10.1007/s10584-013-0827-z
![]() |
[94] |
Zahabiyoun B, Goodarzi MR, Bavani ARM, et al. (2013) Assessment of climate change impact on the Gharesou River Basin using SWAT hydrological model. CLEAN – Soil, Air, Water 41: 601–609. doi: 10.1002/clen.201100652
![]() |
[95] | Backlund,P, Janetos A Schimel D. The effects of climate change on agriculture, land resources, water resources, and biodiversity in the United States. Synthesis and Assessment Product 4.3. Washington, DC: U.S. Environmental Protection Agency, Climate Change Science Program, 2008, 240. |
[96] |
Peterson TC, Heim RR, Hirsch R, et al. (2013) Monitoring and understanding changes in heat waves, cold waves, floods, and droughts in the United States: State of knowledge. Bull Amer Meteor Soc 94: 821–834. doi: 10.1175/BAMS-D-12-00066.1
![]() |
[97] |
Dudula J, Randhir TO (2016) Modeling the influence of climate change on watershed systems: Adaptation through targeted practices. J Hydrol 541: 703–713. doi: 10.1016/j.jhydrol.2016.07.020
![]() |
[98] |
Davis MB, Shaw RG (2001) Range shifts and adaptive responses to quaternary climate change. Science 292: 673–679. doi: 10.1126/science.292.5517.673
![]() |
[99] |
Feeley KJ, Silman MR (2010) Land-use and climate change effects on population size and extinction risk of Andean plants. Glob Chang Biol 16: 3215–3222. doi: 10.1111/j.1365-2486.2010.02197.x
![]() |
[100] |
Parmesan C (2006) Ecological and evolutionary responses to recent climate change. Annu Rev Ecol Evol Syst 37: 637–669. doi: 10.1146/annurev.ecolsys.37.091305.110100
![]() |
[101] |
Poloczanska ES, Brown CJ, Sydeman WJ, et al. (2013) Global imprint of climate change on marine life. Nature Clim Chang 3: 919–925. doi: 10.1038/nclimate1958
![]() |
[102] |
Runion GB (2003) Climate change and plant pathosystems: Future disease prevention starts here. New Phytol 159: 531–533. doi: 10.1046/j.1469-8137.2003.00868.x
![]() |
[103] |
Smith DL, Almaraz JJ (2004) Climate change and crop production: contributions, impacts, and adaptations. Can J Plant Pathol 26: 253–266. doi: 10.1080/07060660409507142
![]() |
[104] |
Ashmore M, Toet S, Emberson L (2006) Ozone – a significant threat to future world food production? New Phytol 170: 201–204. doi: 10.1111/j.1469-8137.2006.01709.x
![]() |
[105] |
Alexander JM, Diez JM, Levine JM (2015) Novel competitors shape species' responses to climate change. Nature 525: 515–518. doi: 10.1038/nature14952
![]() |
[106] | Duku C, Zwart SJ, Hein L (2018) Impacts of climate change on cropping patterns in a tropical, sub-humid watershed. PLOS ONE 13, e0192642. |
[107] |
Aguilera R, Marcé R, Sabater S (2015) Detection and attribution of global change effects on river nutrient dynamics in a large Mediterranean basin. Biogeosciences 12: 4085–4098. doi: 10.5194/bg-12-4085-2015
![]() |
[108] |
Cousino LK, Becker RH, Zmijewski KA (2015) Modeling the effects of climate change on water, sediment, and nutrient yields from the Maumee River watershed. J Hydrol Reg Stud 4: 762–775. doi: 10.1016/j.ejrh.2015.06.017
![]() |
[109] | Cordovil C, Cruz S, Brito A, et al. (2018) A simplified nitrogen assessment in Tagus River Basin: A management focused review. Water 10, 406. |
[110] |
Easterling DR (2002) Recent changes in frost days and the frost-free season in the united states. Bull Amer Meteor Soc 83: 1327–1332. doi: 10.1175/1520-0477-83.9.1327
![]() |
[111] |
Easterling DR, Evans JL, Groisman PY, et al. (2000) Observed variability and trends in extreme climate events: A brief review. Bull Amer Meteor Soc 81: 417–426. doi: 10.1175/1520-0477(2000)081<0417:OVATIE>2.3.CO;2
![]() |
[112] | Williamson S, Lakhey S, Karetnikov D, et al. Economic impacts of climate change on Ohio; Center for Integrative Environmental Research, University of Maryland: College Park, Maryland, USA, 2008, 20. |
[113] |
Kundzewicz ZW, Mata LJ, Arnell NW, et al. (2008) The implications of projected climate change for freshwater resources and their management. Hydrolog Sci J 53: 3–10. doi: 10.1623/hysj.53.1.3
![]() |
[114] |
Milly PCD, Dunne KA, Vecchia AV (2005) Global pattern of trends in streamflow and water availability in a changing climate. Nature 438: 347–350. doi: 10.1038/nature04312
![]() |
[115] |
Wu S-Y (2010) Potential impact of climate change on flooding in the Upper Great Miami River Watershed, Ohio, USA: a simulation-based approach. Hydrolog Sci J 55: 1251–1263. doi: 10.1080/02626667.2010.529814
![]() |
[116] | Jha M, Pan Z, Takle ES, et al. (2004) Impacts of climate change on streamflow in the Upper Mississippi River Basin: A regional climate model perspective. JGR Atmospheres 2004, 109. |
[117] |
Pathak P, Kalra A, Ahmad S (2017) Temperature and precipitation changes in the Midwestern United States: implications for water management. Int J Water Resour Dev 33: 1003–1019. doi: 10.1080/07900627.2016.1238343
![]() |
[118] |
Milly PCD, Dunne KA (2001) Trends in evaporation and surface cooling in the Mississippi River Basin. Geophys Res Lett 28: 1219–1222. doi: 10.1029/2000GL012321
![]() |
[119] | WorldClim - Global Climate Data, Free climate data for ecological modeling and GIS. Available from: http://www.worldclim.org. |
[120] |
Gent PR, Danabasoglu G, Donner LJ, et al. (2011) The Community Climate System Model Version 4. J Climate 24: 4973–4991. doi: 10.1175/2011JCLI4083.1
![]() |
[121] |
Watanabe M, Suzuki T, O'ishi R, et al. (2010) Improved climate simulation by MIROC5: Mean States, Variability, and Climate Sensitivity. J Climate 23: 6312–6335. doi: 10.1175/2010JCLI3679.1
![]() |
[122] |
Collins WJ, Bellouin N, Doutriaux-Boucher M, et al. (2011) Development and evaluation of an Earth-System model – HadGEM2. Geosci Model Dev 4: 1051–1075. doi: 10.5194/gmd-4-1051-2011
![]() |
[123] | Taylor KE, Stouffer RJ, Meehl GA (2011) An overview of CMIP5 and the experiment design. Bull Amer Meteor Soc 93: 485–498. |
[124] | Sheffield J, Barrett AP, Colle B, et al. (2013) North American climate in CMIP5 experiments. Part I: Evaluation of historical simulations of continental and regional climatology. J Climate 26: 9209–9245. |
[125] |
van Vuuren DP, Edmonds J, Kainuma M, et al. (2011) The representative concentration pathways: an overview. Climatic Change 109: 5. doi: 10.1007/s10584-011-0148-z
![]() |
[126] |
Knutti R, Sedláček J (2013) Robustness and uncertainties in the new CMIP5 climate model projections. Nat Clim Chang 3: 369–373. doi: 10.1038/nclimate1716
![]() |
[127] |
Zwiers FW, Kharin VV (1998) Changes in the extremes of the climate simulated by CCC GCM2 under CO2 doubling. J Climate 11: 2200–2222. doi: 10.1175/1520-0442(1998)011<2200:CITEOT>2.0.CO;2
![]() |
[128] |
Kharin VV, Zwiers FW (2000). Changes in the extremes in an ensemble of transient climate simulations with a coupled atmosphere–ocean GCM. J Climate 13: 3760–3788. doi: 10.1175/1520-0442(2000)013<3760:CITEIA>2.0.CO;2
![]() |
[129] | Cubash U, Meehl GA. Projections of future climate change. In Climate Change 2001: The Scientific Basis.; Contribution of Working Group 1 to the Third IPCC Scientific Assessment; Cambridge University Press: UK, 2001: 524–582. |
[130] |
Semenov V, Bengtsson L (2002) Secular trends in daily precipitation characteristics: greenhouse gas simulation with a coupled AOGCM. Clim Dyn 19: 123–140. doi: 10.1007/s00382-001-0218-4
![]() |
[131] | Murdoch PS, Baron JS, Miller TL (2000) Potential effects of climate change on surface water quality in North America 1. JAWRA 36: 347–366. |
[132] |
Srinivasan R, Arnold JG, Jones CA (1998) Hydrologic modelling of the United States with the Soil and Water Assessment Tool. Int J Water Resour Dev 14: 315–325. doi: 10.1080/07900629849231
![]() |
[133] | Arnold JG, Williams JR, Srinivasan R, et al. SWAT-Soil and Water Assessment Tool User Manual, 2018. Available from: https://swat.tamu.edu/media/69296/swat-io-documentation-2012.pdf. |
[134] |
Pease LM, Oduor P, Padmanabhan G (2010) Estimating sediment, nitrogen, and phosphorous loads from the Pipestem Creek watershed, North Dakota, using AnnAGNPS. Comput Geosci 36: 282–291. doi: 10.1016/j.cageo.2009.07.004
![]() |
[135] | Borah DK, Xia R, Bera M. DWSM−A dynamic watershed simulation model for studying agricultural nonpoint−source pollution. Paper number 012028, ASAE Annual Meeting St. Joseph, Michigan, 2001. |
[136] | Bicknell BR, Imhoff JC, Kittle JL, et al. Hydrological Simulation Program--Fortran, User's manual for version 11: U.S. Environmental Protection Agency; U.S. Environmental Protection Agency: National Exposure Research Laboratory, 1997, 755. |
[137] |
Singh VP, Frevert DK (2002) Mathematical models of large watershed hydrology. J Hydrol Eng 7: 270–292. doi: 10.1061/(ASCE)1084-0699(2002)7:4(270)
![]() |
[138] |
Shen Z, Liao Q, Hong Q, Gong Y (2012) An overview of research on agricultural non-point source pollution modelling in China. Sep Pur Technol 84: 104–111. doi: 10.1016/j.seppur.2011.01.018
![]() |
[139] | Weller DE, Baker ME (2014) Cropland riparian buffers throughout Chesapeake Bay Watershed: Spatial patterns and effects on nitrate loads delivered to streams. JAWRA 50: 696–712. |
[140] |
Sharifi A, Lang MW, McCarty GW, et al. (2016) Improving model prediction reliability through enhanced representation of wetland soil processes and constrained model auto calibration – A paired watershed study. J Hydrol 541: 1088–1103. doi: 10.1016/j.jhydrol.2016.08.022
![]() |
[141] |
Borah DK, Bera M (2003) Watershed-scale hydrologic and nonpoint-source pollution models: Review of mathematical bases. T ASAE 46: 1553–1566. doi: 10.13031/2013.15644
![]() |
[142] |
Robson BJ (2014) State of the art in modelling of phosphorus in aquatic systems: Review, criticisms and commentary. Environ Modell Softw 61: 339–359. doi: 10.1016/j.envsoft.2014.01.012
![]() |
[143] |
Rinke A, Dethloff K, Cassano JJ, et al. (2006) Evaluation of an ensemble of Arctic regional climate models: spatiotemporal fields during the SHEBA year. Clim Dyn 26: 459–472. doi: 10.1007/s00382-005-0095-3
![]() |
[144] |
Mohammed IN, Bomblies A, Wemple BC (2015) The use of CMIP5 data to simulate climate change impacts on flow regime within the Lake Champlain Basin. J Hydrol Reg Stud 3: 160–186. doi: 10.1016/j.ejrh.2015.01.002
![]() |
[145] |
Nour MH, Smith DW, El-Din MG, et al. (2008) Effect of watershed subdivision on water-phase phosphorus modelling: An artificial neural network modelling application. J Environ Eng Sci 7: 95–108. doi: 10.1139/S08-043
![]() |
[146] |
Kim RJ, Loucks DP, Stedinger JR (2012) Artificial neural network models of watershed nutrient loading. Water Resour Manage 26: 2781–2797. doi: 10.1007/s11269-012-0045-x
![]() |
[147] | Tang X, Xia M, Guan F, et al. (2016) Spatial distribution of soil nitrogen, phosphorus and potassium stocks in Moso bamboo forests in subtropical China. Forests 7, 267. |
[148] |
Mohanty S, Jha MK, Kumar A, et al. (2010) Artificial neural network modeling for groundwater level forecasting in a river island of eastern India. Water Resour Manage 24: 1845–1865. doi: 10.1007/s11269-009-9527-x
![]() |
[149] |
Kalin L, Isik S, Schoonover JE, et al. (2010) Predicting water quality in unmonitored watersheds using artificial neural networks. J Environ Qual 39: 1429–1440. doi: 10.2134/jeq2009.0441
![]() |
[150] |
Fogelman S, Blumenstein M, Zhao H (2006) Estimation of chemical oxygen demand by ultraviolet spectroscopic profiling and artificial neural networks. Neural Comput Applic 15: 197–203. doi: 10.1007/s00521-005-0015-9
![]() |
[151] |
Dogan E, Sengorur B, Koklu R (2009) Modeling biological oxygen demand of the Melen River in Turkey using an artificial neural network technique. J Environ Manag 90: 1229–1235. doi: 10.1016/j.jenvman.2008.06.004
![]() |
[152] |
Iliadis LS, Maris F (2007) An artificial neural network model for mountainous water-resources management: The case of Cyprus mountainous watersheds. Environ Modell Softw 22: 1066–1072. doi: 10.1016/j.envsoft.2006.05.026
![]() |
[153] |
Noori N, Kalin L (2016) Coupling SWAT and ANN models for enhanced daily streamflow prediction. J Hydrol 533: 141–151. doi: 10.1016/j.jhydrol.2015.11.050
![]() |
[154] |
Okkan U, Serbes ZA (2012) Rainfall–runoff modeling using least squares support vector machines. Environmetrics 23: 549–564. doi: 10.1002/env.2154
![]() |
[155] |
Huang S, Chang J, Huang Q, Chen Y (2014) Monthly streamflow prediction using modified EMD-based support vector machine. J Hydrol 511: 764–775. doi: 10.1016/j.jhydrol.2014.01.062
![]() |
[156] | Jajarmizadeh M, Harun S, Salarpour M. An assessment of a proposed hybrid neural network for daily flow prediction in arid climate, 2014. Available from: https://www.hindawi.com/journals/mse/2014/635018. |
[157] | Gassman P, Reyes M, Green C, Arnold J (2007) The Soil and Water Assessment Tool: Historical development, applications, and future research directions. T ASABE 7: 1211–1250. |
[158] | Niraula R, Kalin L, Wang R, et al. (2012) Determining nutrient and sediment critical source areas with SWAT model: effect of lumped calibration. T ASABE 55: 137–147. |
[159] | White M, Gambone M, Yen H, et al. (2015) Regional blue and green water balances and use by selected crops in the U.S. JAWRA 51: 1626–1642. |
[160] |
Wang R, Bowling LC, Cherkauer KA (2016) Estimation of the effects of climate variability on crop yield in the Midwest USA. Agric For Meteorol 216: 141–156. doi: 10.1016/j.agrformet.2015.10.001
![]() |
[161] | Yen H, White MJ, Arnold JG, et al. (2016) Western Lake Erie Basin: Soft-data-constrained, NHDPlus resolution watershed modeling and exploration of applicable conservation scenarios. Sci Total Environ 569–570: 1265–1281. |
[162] |
Arnold JG, Fohner N (2005) Current capabilities and research opportunities in applied watershed modeling. Hydrol Process 19: 563–572. doi: 10.1002/hyp.5611
![]() |
[163] | Santhi C, Arnold JG, Williams JR, et al. (2001) Validation of the Swat Model on a Large Rwer Basin with Point and Nonpoint Sources. JAWRA 37: 1169–1188. |
[164] |
Bosch DD, Sheridan JM, Batten HL, et al. (2004) Evaluation of the SWAT model on a coastal plain agricultural watershed. T ASAE 47: 1493–1506. doi: 10.13031/2013.17629
![]() |
[165] |
Saleh A, Du B (2004) Evaluation of SWAT and HSPF within BASINS program for the Upper North Bosque River watershed in central Texas. T ASAE 47: 1039–1049. doi: 10.13031/2013.16577
![]() |
[166] |
Tripathi MP, Panda RK, Raghuwanshi NS (2005) Development of effective management plan for critical subwatersheds using SWAT model. Hydrol Process 19: 809–826. doi: 10.1002/hyp.5618
![]() |
[167] | Grunwald S, Qi C (2006) Gis-Based Water Quality Modeling in the Sandusky Watershed, Ohio, USA. JAWRA 42: 957–973. |
[168] | Setegn SG, Srinivasan R, Dargahi B (2008) Hydrological Modelling in the Lake Tana Basin, Ethiopia Using SWAT Model. TOHJ, 2: 49–62. |
[169] | SWAT Literature Database SWAT Literature Database for Peer-Reviewed Journal Articles Available from: https://www.card.iastate.edu/swat_articles. |
[170] |
Tuo Y, Chiogna G, Disse M, et al. (2015) A multi-criteria model selection protocol for practical applications to nutrient transport at the catchment scale. Water 7: 2851–2880. doi: 10.3390/w7062851
![]() |
[171] |
Krysanova V, Hattermann F, Huang S, et al. (2015) Modelling climate and land-use change impacts with SWIM: lessons learnt from multiple applications. Hydrol Sci J 60: 606–635. doi: 10.1080/02626667.2014.925560
![]() |
[172] |
Čerkasova N, Umgiesser G, Ertürk A (2018) Development of a hydrology and water quality model for a large transboundary river watershed to investigate the impacts of climate change – A SWAT application. Ecol Eng 124: 99–115. doi: 10.1016/j.ecoleng.2018.09.025
![]() |
[173] | Cho J, Oh C, Choi J, et al. (2018) Climate change impacts on agricultural non-point source pollution with consideration of uncertainty in CMIP5. Irrig Drain 65: 209–220. |
[174] |
Yang X, Warren R, He Y, et al. (2018) Impacts of climate change on TN load and its control in a River Basin with complex pollution sources. Sci Total Environ 615: 1155–1163. doi: 10.1016/j.scitotenv.2017.09.288
![]() |
[175] |
Lee S, Yeo I-Y, Sadeghi AM, et al. (2018) Comparative analyses of hydrological responses of two adjacent watersheds to climate variability and change using the SWAT model. Hydrol Earth Syst Sci 22: 689–708. doi: 10.5194/hess-22-689-2018
![]() |
[176] |
Jha MK, Gassman PW, Panagopoulos Y (2015) Regional changes in nitrate loadings in the Upper Mississippi River Basin under predicted mid-century climate. Reg Environ Change 15: 449–460. doi: 10.1007/s10113-013-0539-y
![]() |
[177] |
Chiew FHS, McMahon TA (1993) Detection of trend or change in annual flow of Australian rivers. Int J Clim 13: 643–653. doi: 10.1002/joc.3370130605
![]() |
[178] |
Gellens D, Roulin E (1998) Streamflow response of Belgian catchments to IPCC climate change scenarios. J Hydrol 210: 242–258. doi: 10.1016/S0022-1694(98)00192-9
![]() |
[179] | Jha M, Gassman PW, Secchi S, et al. (2004) Effect of watershed subdivision on Swat flow, sediment, and nutrient predictions. JAWRA 40: 811–825. |
[180] |
Glavan M, Ceglar A, Pintar M (2015) Assessing the impacts of climate change on water quantity and quality modelling in small Slovenian Mediterranean catchment – lesson for policy and decision makers. Hydrol Process 29: 3124–3144. doi: 10.1002/hyp.10429
![]() |
[181] |
Kroeze C, Seitzinger SP (1998) Nitrogen inputs to rivers, estuaries and continental shelves and related nitrous oxide emissions in 1990 and 2050: a global model. Nutr Cycl Agroecosys 52: 195–212. doi: 10.1023/A:1009780608708
![]() |
[182] |
Seitzinger SP, Kroeze C, Bouwman AF, et al. (2002) Global patterns of dissolved inorganic and particulate nitrogen inputs to coastal systems: Recent conditions and future projections. Estuaries 25: 640–655. doi: 10.1007/BF02804897
![]() |
[183] |
Galloway JN, Dentener FJ, Capone DG, et al. (2004) Nitrogen cycles: Past, present, and future. Biogeochemistry 70: 153–226. doi: 10.1007/s10533-004-0370-0
![]() |
[184] | Bouwman AF, Drecht GV, Knoop JM, et al. (2005) Exploring changes in river nitrogen export to the world's oceans. Global Biogeochem Cy 19: GB1002. |
[185] |
Tilman D, Fargione J, Wolff B, et al. (2001) Forecasting agriculturally driven global environmental change. Science 292: 281–284. doi: 10.1126/science.1057544
![]() |
[186] | Fisher R (2013) Cost estimates of phosphorus removal at wastewater treatment plants.; Ohio Environmental Protection Agency, p. 50. |
[187] |
Kronvang B, Bechmann M, Pedersen ML, et al. (2003) Phosphorus dynamics and export in streams draining micro-catchments: Development of empirical models. J Plant Nutr Soil Sci 166: 469–474. doi: 10.1002/jpln.200321164
![]() |
[188] |
Osmond D, Meals D, Hoag D, et al. (2012). Improving conservation practices programming to protect water quality in agricultural watersheds: Lessons learned from the National Institute of Food and Agriculture–Conservation Effects Assessment Project. J Soil Water Conserv 67: 122–127. doi: 10.2489/jswc.67.2.122
![]() |
[189] | Gburek WJ, Drungil CC, Srinivasan MS, et al. (2002) Variable-source-area controls on phosphorus transport: Bridging the gap between research and design. J Soil Water Conserv 57: 534–543. |
[190] |
Gitau MW, Veith TL, Gburek WJ (2004) Farm-level optimization of BMP placement for cost-effective pollution reduction. T ASAE 47: 1923–1931. doi: 10.13031/2013.17805
![]() |
[191] | Arabi M, Govindaraju RS, Hantush MM (2006) Cost‐effective allocation of watershed management practices using a genetic algorithm - Arabi. Water Resouce Res 42: W10429. |
[192] | Caswell M, Fuglie K, Ingram C, et al. Adoption of agricultural production practices. Economic Research Service, Agricultural Economic Report No. 792, USDA, 2001. |
[193] |
Gabriel M, Knightes C, Cooter E, et al. (2018) Modeling the combined effects of changing land cover, climate, and atmospheric deposition on nitrogen transport in the Neuse River Basin. J Hydrol Reg Stud 18: 68–79. doi: 10.1016/j.ejrh.2018.05.004
![]() |
[194] |
Holman IP, Rounsevell MDA, Shackley S, et al. (2005) A regional, multi-sectoral and integrated assessment of the impacts of climate and socio-economic change in the UK. Climatic Change 71: 9–41. doi: 10.1007/s10584-005-5927-y
![]() |
[195] |
Dowd BM, Press D, Huertos ML (2008) Agricultural nonpoint source water pollution policy: The case of California's Central Coast. Agr Ecosyst Environ 128: 151–161. doi: 10.1016/j.agee.2008.05.014
![]() |
1. | Siddhartha Kundu, Modeling ligand-macromolecular interactions as eigenvalue-based transition-state dissociation constants may offer insights into biochemical function of the resulting complexes, 2022, 19, 1551-0018, 13252, 10.3934/mbe.2022620 | |
2. | Siddhartha Kundu, ProTG4: A Web Server to Approximate the Sequence of a Generic Protein From an in Silico Library of Translatable G-Quadruplex (TG4)-Mapped Peptides, 2021, 15, 1177-9322, 117793222110458, 10.1177/11779322211045878 |
Rank | Codon set, Cardinality | Codon | γ | θ | δ | Ω | α=γ.θ.δ+Ω | aa |
1 | GGG, 1 | GGG | 0.02 | 1.00 | 6 | 2 | 2.1200 | Gly |
2 | GxG, 3 | GUG | 0.02 | 0.33 | 1 | 2 | 2.0066 | Val |
GCG | 0.02 | 0.33 | 1 | 2 | 2.0066 | Ala | ||
GAG | 0.02 | 0.33 | 1 | 2 | 2.0066 | Glu | ||
3 | xGG, 3 | UGG | 0.02 | 0.33 | 2 | 1 | 1.0132 | Trp |
CGG | 0.02 | 0.33 | 2 | 1 | 1.0132 | Arg | ||
AGG | 0.02 | 0.33 | 2 | 1 | 1.0132 | Arg | ||
3 | GGx, 3 | GGU | 0.02 | 0.33 | 2 | 1 | 1.0132 | Gly |
GGC | 0.02 | 0.33 | 2 | 1 | 1.0132 | Gly | ||
GGA | 0.02 | 0.33 | 2 | 1 | 1.0132 | Gly | ||
4 | xxG, 9 | UUG | 0.02 | 0.11 | 1 | 1 | 1.0022 | Leu |
UCG | 0.02 | 0.11 | 1 | 1 | 1.0022 | Ser | ||
UAG | 0.02 | 0.11 | 1 | 1 | 1.0022 | Ter | ||
CUG | 0.02 | 0.11 | 1 | 1 | 1.0022 | Leu | ||
CCG | 0.02 | 0.11 | 1 | 1 | 1.0022 | Pro | ||
CAG | 0.02 | 0.11 | 1 | 1 | 1.0022 | Gln | ||
AUG | 0.02 | 0.11 | 1 | 1 | 1.0022 | Met | ||
ACG | 0.02 | 0.11 | 1 | 1 | 1.0022 | Thr | ||
AAG | 0.02 | 0.11 | 1 | 1 | 1.0022 | Lys | ||
4 | Gxx, 9 | GUU | 0.02 | 0.11 | 1 | 1 | 1.0022 | Val |
GCU | 0.02 | 0.11 | 1 | 1 | 1.0022 | Ala | ||
GAU | 0.02 | 0.11 | 1 | 1 | 1.0022 | Asp | ||
GUC | 0.02 | 0.11 | 1 | 1 | 1.0022 | Val | ||
GCC | 0.02 | 0.11 | 1 | 1 | 1.0022 | Ala | ||
GAC | 0.02 | 0.11 | 1 | 1 | 1.0022 | Asp | ||
GUA | 0.02 | 0.11 | 1 | 1 | 1.0022 | Val | ||
GCA | 0.02 | 0.11 | 1 | 1 | 1.0022 | Ala | ||
GAA | 0.02 | 0.11 | 1 | 1 | 1.0022 | Glu | ||
5 | xGx, 9 | UGU | 0.02 | 0.11 | 0 | 0 | 0.0000 | Cys |
UGC | 0.02 | 0.11 | 0 | 0 | 0.0000 | Cys | ||
UGA | 0.02 | 0.11 | 0 | 0 | 0.0000 | Ter | ||
CGU | 0.02 | 0.11 | 0 | 0 | 0.0000 | Arg | ||
CGC | 0.02 | 0.11 | 0 | 0 | 0.0000 | Arg | ||
CGA | 0.02 | 0.11 | 0 | 0 | 0.0000 | Arg | ||
AGU | 0.02 | 0.11 | 0 | 0 | 0.0000 | Ser | ||
AGC | 0.02 | 0.11 | 0 | 0 | 0.0000 | Ser | ||
AGA | 0.02 | 0.11 | 0 | 0 | 0.0000 | Arg | ||
5 | xxx, 27 | UUU | 0.02 | 0.04 | 0 | 0 | 0.0000 | Phe |
UCU | 0.02 | 0.04 | 0 | 0 | 0.0000 | Ser | ||
UAU | 0.02 | 0.04 | 0 | 0 | 0.0000 | Tyr | ||
UUC | 0.02 | 0.04 | 0 | 0 | 0.0000 | Phe | ||
UCC | 0.02 | 0.04 | 0 | 0 | 0.0000 | Ser | ||
UUA | 0.02 | 0.04 | 0 | 0 | 0.0000 | Leu | ||
UCA | 0.02 | 0.04 | 0 | 0 | 0.0000 | Ser | ||
UAA | 0.02 | 0.04 | 0 | 0 | 0.0000 | Ter | ||
CUU | 0.02 | 0.04 | 0 | 0 | 0.0000 | Leu | ||
CCU | 0.02 | 0.04 | 0 | 0 | 0.0000 | Pro | ||
CAU | 0.02 | 0.04 | 0 | 0 | 0.0000 | His | ||
CUC | 0.02 | 0.04 | 0 | 0 | 0.0000 | Leu | ||
CCC | 0.02 | 0.04 | 0 | 0 | 0.0000 | Pro | ||
CAC | 0.02 | 0.04 | 0 | 0 | 0.0000 | His | ||
CUA | 0.02 | 0.04 | 0 | 0 | 0.0000 | Leu | ||
CCA | 0.02 | 0.04 | 0 | 0 | 0.0000 | Pro | ||
CAA | 0.02 | 0.04 | 0 | 0 | 0.0000 | Gln | ||
AUU | 0.02 | 0.04 | 0 | 0 | 0.0000 | Ile | ||
ACU | 0.02 | 0.04 | 0 | 0 | 0.0000 | Thr | ||
AAU | 0.02 | 0.04 | 0 | 0 | 0.0000 | Asn | ||
AUC | 0.02 | 0.04 | 0 | 0 | 0.0000 | Ile | ||
ACC | 0.02 | 0.04 | 0 | 0 | 0.0000 | Thr | ||
AAC | 0.02 | 0.04 | 0 | 0 | 0.0000 | Asn | ||
AUA | 0.02 | 0.04 | 0 | 0 | 0.0000 | Ile | ||
ACA | 0.02 | 0.04 | 0 | 0 | 0.0000 | Thr | ||
AAA | 0.02 | 0.04 | 0 | 0 | 0.0000 | Lys |
aa | CODamino | gCOD+amino | β | |
Group 1 (n=7) | Ala | 4 | 4 | 1.00 |
Val | 4 | 4 | 1.00 | |
Asp | 2 | 2 | 1.00 | |
Glu | 2 | 2 | 1.00 | |
Trp | 1 | 1 | 1.00 | |
Met | 1 | 1 | 1.00 | |
Gly | 4 | 4 | 1.00 | |
Group 2 (n=7) | Leu | 6 | 2 | 0.3333 |
Gln | 2 | 1 | 0.5 | |
Arg | 6 | 2 | 0.3333 | |
Lys | 2 | 1 | 0.5 | |
Ser | 6 | 1 | 0.1667 | |
Thr | 4 | 1 | 0.25 | |
Pro | 4 | 1 | 0.25 | |
Group 3 (n=6) | Cys | 2 | 0 | 0.00 |
Asn | 2 | 0 | 0.00 | |
Ile | 3 | 0 | 0.00 | |
His | 2 | 0 | 0.00 | |
Phe | 2 | 0 | 0.00 | |
Tyr | 2 | 0 | 0.00 |
GENE | NAME | G4 (nt) | Ex | STOP(n=11) | VALID(n=59) | |PTG4| |
BACE1 | Beta-secretase 1 | 33 | 3 | n=0 | n=6 | n=2 |
BCL2 | B-cell lymphoma 2 | 33 | 2 | n=1 | n=6 | n=1 |
23 | n=0 | n=6 | ||||
28 | n=0 | n=6 | ||||
29 | n=1 | n=5 | ||||
34 | n=1 | n=5 | ||||
33 | 3 | n=2 | n=5 | |||
ESR1 | Estrogen receptor alpha (ERα) | 36 | 4 | n=1 | n=5 | n=2 |
KCNH2 | Potassium Voltage-Gated Channel sub family H | 18 | 12 | n=0 | n=0 | NA |
ZNF669 | Member 2 Zinc Finger Protein 669 |
1 | ||||
PRNP | Prion protein | 14 | 2 | n=0 | n=0 | n=1 |
15 | n=0 | n=0 | ||||
20 | n=0 | n=0 | ||||
24 | n=0 | n=6 | ||||
85 | n=6 | n=3 | ||||
TERF2 | Telomeric repeat-binding factor 2 | 55 | 1 | n=0 | n=6 | n=1 |
Disordered regions (IDRs; n=1445;0.00≤p-value < 0.05) | |||||||||||||||||
SL-PT- | SL-PT+ | SL+PT- | SL+PT+ | R1T | R2T | C1T | C2T | A (%) | P (%) | R (%) | |||||||
SLiMS1 | 1078 | 64 | 58 | 9 | 1142 | 67 | 1136 | 73 | 89.90 | 12.32 | 13.43 | ||||||
SLiMS2 | 749 | 18 | 121 | 29 | 767 | 150 | 870 | 47 | 84.84 | 61.70 | 19.33 | ||||||
SLiMS3 | 1212 | 108 | 34 | 9 | 1320 | 43 | 1246 | 117 | 89.58 | 7.69 | 20.93 | ||||||
Proteins with disordered segments (IDPs; n=800;0.00≤p-value < 0.05) | |||||||||||||||||
SL-PT- | SL-PT+ | SL+PT- | SL+PT+ | R1T | R2T | C1T | C2T | A (%) | P (%) | R (%) | |||||||
SLiMS1 | 86 | 12 | 28 | 18 | 98 | 46 | 114 | 30 | 72.22 | 60.00 | 39.10 | ||||||
SLiMS2 | 1 | 1 | 96 | 66 | 2 | 162 | 97 | 67 | 40.85 | 98.50 | 40.74 | ||||||
SLiMS3 | 250 | 57 | 26 | 25 | 307 | 51 | 276 | 82 | 76.81 | 30.48 | 49.01 |
SLiMS | Sample | (zn)n≥2∈pTG4ij∩SLiMSw (p-value) | |
SLiMS1=[ST]PzR | Pz | PG (n=1) | PG (Degenerate) |
SLiMS2=[ED]zzD[AGS] | z[DE] | G[DE] (n=2) | [WGRVAELMKQSTP][AG][DE]z2EG[VADE](p-value=0.00069) |
[DE]z | [DE]G (n=1) | ||
zz[DE] | [LMKQSTP]G[DE] (n=14) [VAE]G[DE] (n=6) [WGR][AG][DE] (n=6) |
||
[DE]zz[DE] | [VAE]G[DE]zzEG[VADE] (n=28) [WGR][AG][DE]zzEG[VADE] (n=24) [WGR][AG][DE]zzEG (n=6) GEzzEG[VADE] (n=4) GEzzEG (n=1) |
||
SLiMS3=[KR]zPzzP | Pzz | PG[VADE] (n=4) | PGV (Degenerate) PGA (Degenerate) PGD (Degenerate) PGE (Degenerate) |
Cellular function | Disordered regions of proteins | |
1. | Signal transduction | DP00274, DP00224, DP00141, DP00332, DP01063, DP00506, DP00418, DP00341, DP00435, DP00613, DP00463, DP00954, DP00959, DP01104, DP00611, DP00519, DP00086, DP00707, DP00712 |
2. | Endocytosis | DP01073, DP01065, DP01066, DP00225 |
3. | Calcium-calmodulin | DP00092, DP00132, DP00561, DP00118, DP00253 |
4. | Myofibril assembly | DP01090 |
5. | Cytoskeleton | DP01056 DP00240, DP01022, DP00169, DP00716, DP00717, DP01100, DP00122 |
6. | Nuclear pore | DP01075, DP01077, DP01079 |
7. | Phototransduction | DP00768, DP00347 |
8. | Targeting | DP00893, DP00609, DP00610, DP01058 |
9. | Transcription | DP00062, DP00177, DP00633, DP00348, DP00786, DP00049, DP00231, DP00873, DP00720, DP00217, DP00081 |
10. | Translation | DP00082, DP00164, DP00229 DP00949, DP00134 |
11. | Synaptic transmission | DP00943 |
12. | Supercoiling | DP00076 |
13. | Binding | DP00539, DP00854, DP01052, DP00659, DP00656 |
14. | Peptide bond formation | DP00944 |
15. | Enzymes | DP00557, DP00032, DP00095, DP00337, DP00379, DP00787, DP00427, DP00429 |
16. | Bacterial/parasitic virulence | |
Secreted toxins | DP00345, DP00591 | |
Cytoadherence | DP00025, DP00065, DP01096 | |
17. | Viral infectivity | |
Cyclophilin interaction | DP00615, DP01031 | |
Chaperones | DP00699, DP00700, DP00674 | |
Capsid assembly | DP00133, DP00876 | |
Membrane fusion | DP01043 | |
Latency | DP01060 | |
18. | Unknown | DP00119 |
Rank | Codon set, Cardinality | Codon | γ | θ | δ | Ω | α=γ.θ.δ+Ω | aa |
1 | GGG, 1 | GGG | 0.02 | 1.00 | 6 | 2 | 2.1200 | Gly |
2 | GxG, 3 | GUG | 0.02 | 0.33 | 1 | 2 | 2.0066 | Val |
GCG | 0.02 | 0.33 | 1 | 2 | 2.0066 | Ala | ||
GAG | 0.02 | 0.33 | 1 | 2 | 2.0066 | Glu | ||
3 | xGG, 3 | UGG | 0.02 | 0.33 | 2 | 1 | 1.0132 | Trp |
CGG | 0.02 | 0.33 | 2 | 1 | 1.0132 | Arg | ||
AGG | 0.02 | 0.33 | 2 | 1 | 1.0132 | Arg | ||
3 | GGx, 3 | GGU | 0.02 | 0.33 | 2 | 1 | 1.0132 | Gly |
GGC | 0.02 | 0.33 | 2 | 1 | 1.0132 | Gly | ||
GGA | 0.02 | 0.33 | 2 | 1 | 1.0132 | Gly | ||
4 | xxG, 9 | UUG | 0.02 | 0.11 | 1 | 1 | 1.0022 | Leu |
UCG | 0.02 | 0.11 | 1 | 1 | 1.0022 | Ser | ||
UAG | 0.02 | 0.11 | 1 | 1 | 1.0022 | Ter | ||
CUG | 0.02 | 0.11 | 1 | 1 | 1.0022 | Leu | ||
CCG | 0.02 | 0.11 | 1 | 1 | 1.0022 | Pro | ||
CAG | 0.02 | 0.11 | 1 | 1 | 1.0022 | Gln | ||
AUG | 0.02 | 0.11 | 1 | 1 | 1.0022 | Met | ||
ACG | 0.02 | 0.11 | 1 | 1 | 1.0022 | Thr | ||
AAG | 0.02 | 0.11 | 1 | 1 | 1.0022 | Lys | ||
4 | Gxx, 9 | GUU | 0.02 | 0.11 | 1 | 1 | 1.0022 | Val |
GCU | 0.02 | 0.11 | 1 | 1 | 1.0022 | Ala | ||
GAU | 0.02 | 0.11 | 1 | 1 | 1.0022 | Asp | ||
GUC | 0.02 | 0.11 | 1 | 1 | 1.0022 | Val | ||
GCC | 0.02 | 0.11 | 1 | 1 | 1.0022 | Ala | ||
GAC | 0.02 | 0.11 | 1 | 1 | 1.0022 | Asp | ||
GUA | 0.02 | 0.11 | 1 | 1 | 1.0022 | Val | ||
GCA | 0.02 | 0.11 | 1 | 1 | 1.0022 | Ala | ||
GAA | 0.02 | 0.11 | 1 | 1 | 1.0022 | Glu | ||
5 | xGx, 9 | UGU | 0.02 | 0.11 | 0 | 0 | 0.0000 | Cys |
UGC | 0.02 | 0.11 | 0 | 0 | 0.0000 | Cys | ||
UGA | 0.02 | 0.11 | 0 | 0 | 0.0000 | Ter | ||
CGU | 0.02 | 0.11 | 0 | 0 | 0.0000 | Arg | ||
CGC | 0.02 | 0.11 | 0 | 0 | 0.0000 | Arg | ||
CGA | 0.02 | 0.11 | 0 | 0 | 0.0000 | Arg | ||
AGU | 0.02 | 0.11 | 0 | 0 | 0.0000 | Ser | ||
AGC | 0.02 | 0.11 | 0 | 0 | 0.0000 | Ser | ||
AGA | 0.02 | 0.11 | 0 | 0 | 0.0000 | Arg | ||
5 | xxx, 27 | UUU | 0.02 | 0.04 | 0 | 0 | 0.0000 | Phe |
UCU | 0.02 | 0.04 | 0 | 0 | 0.0000 | Ser | ||
UAU | 0.02 | 0.04 | 0 | 0 | 0.0000 | Tyr | ||
UUC | 0.02 | 0.04 | 0 | 0 | 0.0000 | Phe | ||
UCC | 0.02 | 0.04 | 0 | 0 | 0.0000 | Ser | ||
UUA | 0.02 | 0.04 | 0 | 0 | 0.0000 | Leu | ||
UCA | 0.02 | 0.04 | 0 | 0 | 0.0000 | Ser | ||
UAA | 0.02 | 0.04 | 0 | 0 | 0.0000 | Ter | ||
CUU | 0.02 | 0.04 | 0 | 0 | 0.0000 | Leu | ||
CCU | 0.02 | 0.04 | 0 | 0 | 0.0000 | Pro | ||
CAU | 0.02 | 0.04 | 0 | 0 | 0.0000 | His | ||
CUC | 0.02 | 0.04 | 0 | 0 | 0.0000 | Leu | ||
CCC | 0.02 | 0.04 | 0 | 0 | 0.0000 | Pro | ||
CAC | 0.02 | 0.04 | 0 | 0 | 0.0000 | His | ||
CUA | 0.02 | 0.04 | 0 | 0 | 0.0000 | Leu | ||
CCA | 0.02 | 0.04 | 0 | 0 | 0.0000 | Pro | ||
CAA | 0.02 | 0.04 | 0 | 0 | 0.0000 | Gln | ||
AUU | 0.02 | 0.04 | 0 | 0 | 0.0000 | Ile | ||
ACU | 0.02 | 0.04 | 0 | 0 | 0.0000 | Thr | ||
AAU | 0.02 | 0.04 | 0 | 0 | 0.0000 | Asn | ||
AUC | 0.02 | 0.04 | 0 | 0 | 0.0000 | Ile | ||
ACC | 0.02 | 0.04 | 0 | 0 | 0.0000 | Thr | ||
AAC | 0.02 | 0.04 | 0 | 0 | 0.0000 | Asn | ||
AUA | 0.02 | 0.04 | 0 | 0 | 0.0000 | Ile | ||
ACA | 0.02 | 0.04 | 0 | 0 | 0.0000 | Thr | ||
AAA | 0.02 | 0.04 | 0 | 0 | 0.0000 | Lys |
aa | CODamino | gCOD+amino | β | |
Group 1 (n=7) | Ala | 4 | 4 | 1.00 |
Val | 4 | 4 | 1.00 | |
Asp | 2 | 2 | 1.00 | |
Glu | 2 | 2 | 1.00 | |
Trp | 1 | 1 | 1.00 | |
Met | 1 | 1 | 1.00 | |
Gly | 4 | 4 | 1.00 | |
Group 2 (n=7) | Leu | 6 | 2 | 0.3333 |
Gln | 2 | 1 | 0.5 | |
Arg | 6 | 2 | 0.3333 | |
Lys | 2 | 1 | 0.5 | |
Ser | 6 | 1 | 0.1667 | |
Thr | 4 | 1 | 0.25 | |
Pro | 4 | 1 | 0.25 | |
Group 3 (n=6) | Cys | 2 | 0 | 0.00 |
Asn | 2 | 0 | 0.00 | |
Ile | 3 | 0 | 0.00 | |
His | 2 | 0 | 0.00 | |
Phe | 2 | 0 | 0.00 | |
Tyr | 2 | 0 | 0.00 |
GENE | NAME | G4 (nt) | Ex | STOP(n=11) | VALID(n=59) | |PTG4| |
BACE1 | Beta-secretase 1 | 33 | 3 | n=0 | n=6 | n=2 |
BCL2 | B-cell lymphoma 2 | 33 | 2 | n=1 | n=6 | n=1 |
23 | n=0 | n=6 | ||||
28 | n=0 | n=6 | ||||
29 | n=1 | n=5 | ||||
34 | n=1 | n=5 | ||||
33 | 3 | n=2 | n=5 | |||
ESR1 | Estrogen receptor alpha (ERα) | 36 | 4 | n=1 | n=5 | n=2 |
KCNH2 | Potassium Voltage-Gated Channel sub family H | 18 | 12 | n=0 | n=0 | NA |
ZNF669 | Member 2 Zinc Finger Protein 669 |
1 | ||||
PRNP | Prion protein | 14 | 2 | n=0 | n=0 | n=1 |
15 | n=0 | n=0 | ||||
20 | n=0 | n=0 | ||||
24 | n=0 | n=6 | ||||
85 | n=6 | n=3 | ||||
TERF2 | Telomeric repeat-binding factor 2 | 55 | 1 | n=0 | n=6 | n=1 |
Disordered regions (IDRs; n=1445;0.00≤p-value < 0.05) | |||||||||||||||||
SL-PT- | SL-PT+ | SL+PT- | SL+PT+ | R1T | R2T | C1T | C2T | A (%) | P (%) | R (%) | |||||||
SLiMS1 | 1078 | 64 | 58 | 9 | 1142 | 67 | 1136 | 73 | 89.90 | 12.32 | 13.43 | ||||||
SLiMS2 | 749 | 18 | 121 | 29 | 767 | 150 | 870 | 47 | 84.84 | 61.70 | 19.33 | ||||||
SLiMS3 | 1212 | 108 | 34 | 9 | 1320 | 43 | 1246 | 117 | 89.58 | 7.69 | 20.93 | ||||||
Proteins with disordered segments (IDPs; n=800;0.00≤p-value < 0.05) | |||||||||||||||||
SL-PT- | SL-PT+ | SL+PT- | SL+PT+ | R1T | R2T | C1T | C2T | A (%) | P (%) | R (%) | |||||||
SLiMS1 | 86 | 12 | 28 | 18 | 98 | 46 | 114 | 30 | 72.22 | 60.00 | 39.10 | ||||||
SLiMS2 | 1 | 1 | 96 | 66 | 2 | 162 | 97 | 67 | 40.85 | 98.50 | 40.74 | ||||||
SLiMS3 | 250 | 57 | 26 | 25 | 307 | 51 | 276 | 82 | 76.81 | 30.48 | 49.01 |
SLiMS | Sample | (zn)n≥2∈pTG4ij∩SLiMSw (p-value) | |
SLiMS1=[ST]PzR | Pz | PG (n=1) | PG (Degenerate) |
SLiMS2=[ED]zzD[AGS] | z[DE] | G[DE] (n=2) | [WGRVAELMKQSTP][AG][DE]z2EG[VADE](p-value=0.00069) |
[DE]z | [DE]G (n=1) | ||
zz[DE] | [LMKQSTP]G[DE] (n=14) [VAE]G[DE] (n=6) [WGR][AG][DE] (n=6) |
||
[DE]zz[DE] | [VAE]G[DE]zzEG[VADE] (n=28) [WGR][AG][DE]zzEG[VADE] (n=24) [WGR][AG][DE]zzEG (n=6) GEzzEG[VADE] (n=4) GEzzEG (n=1) |
||
SLiMS3=[KR]zPzzP | Pzz | PG[VADE] (n=4) | PGV (Degenerate) PGA (Degenerate) PGD (Degenerate) PGE (Degenerate) |
Cellular function | Disordered regions of proteins | |
1. | Signal transduction | DP00274, DP00224, DP00141, DP00332, DP01063, DP00506, DP00418, DP00341, DP00435, DP00613, DP00463, DP00954, DP00959, DP01104, DP00611, DP00519, DP00086, DP00707, DP00712 |
2. | Endocytosis | DP01073, DP01065, DP01066, DP00225 |
3. | Calcium-calmodulin | DP00092, DP00132, DP00561, DP00118, DP00253 |
4. | Myofibril assembly | DP01090 |
5. | Cytoskeleton | DP01056 DP00240, DP01022, DP00169, DP00716, DP00717, DP01100, DP00122 |
6. | Nuclear pore | DP01075, DP01077, DP01079 |
7. | Phototransduction | DP00768, DP00347 |
8. | Targeting | DP00893, DP00609, DP00610, DP01058 |
9. | Transcription | DP00062, DP00177, DP00633, DP00348, DP00786, DP00049, DP00231, DP00873, DP00720, DP00217, DP00081 |
10. | Translation | DP00082, DP00164, DP00229 DP00949, DP00134 |
11. | Synaptic transmission | DP00943 |
12. | Supercoiling | DP00076 |
13. | Binding | DP00539, DP00854, DP01052, DP00659, DP00656 |
14. | Peptide bond formation | DP00944 |
15. | Enzymes | DP00557, DP00032, DP00095, DP00337, DP00379, DP00787, DP00427, DP00429 |
16. | Bacterial/parasitic virulence | |
Secreted toxins | DP00345, DP00591 | |
Cytoadherence | DP00025, DP00065, DP01096 | |
17. | Viral infectivity | |
Cyclophilin interaction | DP00615, DP01031 | |
Chaperones | DP00699, DP00700, DP00674 | |
Capsid assembly | DP00133, DP00876 | |
Membrane fusion | DP01043 | |
Latency | DP01060 | |
18. | Unknown | DP00119 |