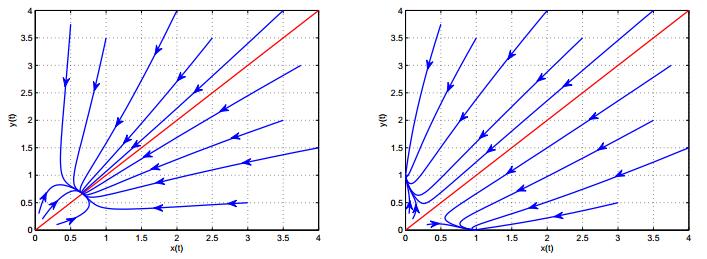
Citation: Junjing Xiong, Xiong Li, Hao Wang. The survival analysis of a stochastic Lotka-Volterra competition model with a coexistence equilibrium[J]. Mathematical Biosciences and Engineering, 2019, 16(4): 2717-2737. doi: 10.3934/mbe.2019135
[1] | Jinyu Wei, Bin Liu . Coexistence in a competition-diffusion-advection system with equal amount of total resources. Mathematical Biosciences and Engineering, 2021, 18(4): 3543-3558. doi: 10.3934/mbe.2021178 |
[2] | Azmy S. Ackleh, Shuhua Hu . Comparison between stochastic and deterministic selection-mutation models. Mathematical Biosciences and Engineering, 2007, 4(2): 133-157. doi: 10.3934/mbe.2007.4.133 |
[3] | Ling Xue, Sitong Chen, Xinmiao Rong . Dynamics of competition model between two plants based on stoichiometry. Mathematical Biosciences and Engineering, 2023, 20(10): 18888-18915. doi: 10.3934/mbe.2023836 |
[4] | Jinyu Wei, Bin Liu . Global dynamics of a Lotka-Volterra competition-diffusion-advection system for small diffusion rates in heterogenous environment. Mathematical Biosciences and Engineering, 2021, 18(1): 564-582. doi: 10.3934/mbe.2021031 |
[5] | Yuanshi Wang, Donald L. DeAngelis . A mutualism-parasitism system modeling host and parasite with mutualism at low density. Mathematical Biosciences and Engineering, 2012, 9(2): 431-444. doi: 10.3934/mbe.2012.9.431 |
[6] | Ali Mai, Guowei Sun, Lin Wang . The impacts of dispersal on the competition outcome of multi-patch competition models. Mathematical Biosciences and Engineering, 2019, 16(4): 2697-2716. doi: 10.3934/mbe.2019134 |
[7] | Paul Georgescu, Hong Zhang, Daniel Maxin . The global stability of coexisting equilibria for three models of mutualism. Mathematical Biosciences and Engineering, 2016, 13(1): 101-118. doi: 10.3934/mbe.2016.13.101 |
[8] | Huili Wei, Wenhe Li . Dynamical behaviors of a Lotka-Volterra competition system with the Ornstein-Uhlenbeck process. Mathematical Biosciences and Engineering, 2023, 20(5): 7882-7904. doi: 10.3934/mbe.2023341 |
[9] | Vaibhava Srivastava, Eric M. Takyi, Rana D. Parshad . The effect of "fear" on two species competition. Mathematical Biosciences and Engineering, 2023, 20(5): 8814-8855. doi: 10.3934/mbe.2023388 |
[10] | Azmy S. Ackleh, Keng Deng, Yixiang Wu . Competitive exclusion and coexistence in a two-strain pathogen model with diffusion. Mathematical Biosciences and Engineering, 2016, 13(1): 1-18. doi: 10.3934/mbe.2016.13.1 |
In Memory of Geoffrey J. Butler and Herbert I. Freedman
The classical two-species Lotka-Volterra competition model takes the form
{˙X(t)=X(t)(a1−b1X(t)−b12Y(t)),˙Y(t)=Y(t)(a2−b2Y(t)−b21X(t)), | (1.1) |
where all parameters are positive with clear biological meanings in literature. It has been one of the most popular models in ecology and its dynamic behaviors have been fully studied as summarized in [1]. In fact, only when b12b2<a1a2<b1b21 or b1b21<a1a2<b12b2, the unique positive equilibrium (X∗,Y∗) exists.
In reality, population dynamics are inevitably affected by environmental noises. Different kinds of random perturbations have been considered in literature, such as [2,3,4,5,6,7,8,9,10,11] and references therein. Persistence and extinction of a competitive system with linear random perturbations have been studied in [4,9].
In particular, a Lotka-Volterra competition system with nonlinear random perturbations
{dX(t)=X(t)(a1−b1X(t)−c1Y(t))dt+α1X2(t)dB1(t)+β1X(t)Y(t)dB2(t),dY(t)=Y(t)(a2−b2Y(t)−c2X(t))dt+α2Y2(t)dB3(t)+β2X(t)Y(t)dB2(t), | (1.2) |
was studied in [8], where Bi(t) (i=1,2,3) are three independent Brownian motions. The authors constructed two threshold values λi (i=1,2) that only depend on the coefficients of (1.2) by using the invariant probability densities of diffusions on the axes, and obtained that the signs of λi (i=1,2) determine if the two species could coexist or one species excludes the other, and additionally estimated the Lyapunov exponents.
In [12], a stochastic competition model with time-dependent delays
{dX(t)=X(t)[r1−a11X(t)−a12X(t−τ1(t))−a13Y(t−τ2(t))]dt+σ1X(t)(X(t)−X∗)dB1(t),dY(t)=Y(t)[r2−a21Y(t)−a22Y(t−τ3(t))−a23X(t−τ4(t))]dt+σ2Y(t)(Y(t)−Y∗)dB2(t), |
was considered, where the random perturbations were related to the coexistence equilibrium state (X∗,Y∗) of the corresponding deterministic system. Here the intrinsic rates ri (i=1,2) are subject to the environmental noises, and clearly (X∗,Y∗) is also the equilibrium of the above stochastic system. The sufficient conditions of the asymptotic stability of its positive equilibrium were obtained by using the method of Lyapunov function.
In [13], we studied the following stochastic system
{dX(t)=X(t)(a1−b1X(t)−b12Y(t))dt+σ1X(t)(Y(t)−Y∗)dB1(t),dY(t)=Y(t)(a2−b2Y(t)−b21X(t))dt+σ2Y(t)(X(t)−X∗)dB2(t), | (1.3) |
which was obtained by perturbing b12 and b21 in (1.1), and investigated the asymptotic stability of its positive equilibrium by constructing an appropriate Lyapunov function. However, due to the limitation of methods, the case of competitive exclusion had not been considered in [13], and we will investigate this case completely in this paper.
In fact, the two-species Lotka-Volterra competition model (1.1) can be simplified as
{˙X(t)=X(t)(1−X(t)−k1Y(t)),˙Y(t)=rY(t)(1−Y(t)−k2X(t)), | (1.4) |
where r is the ratio of the intrinsic rates of the two species, and k1,k2 represent the abilities of their competition between each other. Clearly, only when k1,k2<1 or k1,k2>1, the positive equilibrium exists, and the corresponding results are showed as follows:
(ⅰ) if k1,k2<1, all positive solutions (X(t),Y(t)) to (1.4) converge to the unique positive equilibrium (X∗,Y∗)=(1−k11−k1k2,1−k21−k1k2);
(ⅱ) if k1,k2>1, there is an unstable manifold (called the separatrix) splitting the interior of the positive quadrant R2,o+ into two regions. Solutions above the separatrix converge to (0,1), while solutions below the separatrix converge to (1,0).
In this paper, for simplicity we assume r=1 and consider the following stochastic system
{dX(t)=X(t)(1−X(t)−k1Y(t))dt+σ1X(t)(Y(t)−Y∗)dB1(t),dY(t)=Y(t)(1−Y(t)−k2X(t))dt+σ2Y(t)(X(t)−X∗)dB2(t), | (1.5) |
where (X∗,Y∗)=(1−k11−k1k2,1−k21−k1k2) is the coexistence (or positive) equilibrium of the corresponding deterministic system (1.4), and the inter-specific competition rates k1,k2 are subject to white noises. In reality, the stochasticity in inter-specific competition is more important than that in intra-specific competition. In fact, system (1.5) is a special case of system (1.3).
In this paper, we consider a Lotka-Volterra competition model with special stochastic terms related to its positive equilibrium, which implies that system (1.5) makes sense only for k1,k2<1 or k1,k2>1. Above all, (X∗,Y∗) is also the unique positive equilibrium of the stochastic differential equation (1.5), which is the main difference between our model and other stochastic models. Thus it is significant to investigate whether and how the existence of such environmental noises regulates the dynamic behaviors of the deterministic system.
We first pose an assumption (H0) about the restriction on σi (i=1,2), which guarantees that all solutions to system (1.5) remain positive and non-explosive in any finite time. Subsequently, we use the main ideas in [8] to consider the equations on the two axes and calculate two critical values λ1 and λ2 via these coefficients of (1.5) under another assumption (H1). We obtain that if they are both positive, the two species coexist, and moreover all solutions converge to the coexistence equilibrium (X∗,Y∗), and while they are both negative, the two species go extinct with positive probabilities and these two probability values add up to one. Moreover, if Y(t) (or X(t)) converges to zero, its Lyapunov exponent is exactly λ1 (or λ2), that is also the rate at which the corresponding species goes extinct.
Following the same logic in [8], we obtain quite different results due to the special stochastic terms, that is, (X∗,Y∗) is also the positive equilibrium of the stochastic system (1.5). In this paper, we just consider the stochastic system under the two cases of the corresponding deterministic system in which (X∗,Y∗) is globally asymptotically stable or the bistability occurs. Here the intensity of noises σi (i=1,2) cannot be too strong (the assumption (H0) is a restriction), otherwise the solutions to system (1.5) make no biological sense. Moreover, assumption (H1) guarantees the existence of λi (i=1,2), which have precise expressions. Different from that in [8], λ1λ2<0 should not happen according to many numerical trials, and we find that the dynamic behaviors of the deterministic system will not be destroyed if the random perturbations are relatively small, which will be discussed in the final part.
The rest of the paper is organized as follows. Some preliminaries and the main ideas are presented in Section 2, and the main results in this paper are listed in Sections 3. In Sections 4 and 5, the cases of coexistence and competitive exclusion are considered, respectively. Finally in Section 6, some discussions about this model and some numerical examples are provided to illustrate our mathematical results.
Let (Ω,F,{Ft}t≥0,P) be a complete filtered probability space with the filtration {Ft}t≥0 satisfying the usual condition, i.e. it is increasing and right continuous while F0 contains all P-null sets. Throughout this paper, we denote R2,o+={(x,y):x>0, y>0} and let Zz(t)=(Xz(t),Yz(t)) be the solution to system (1.5) with initial value z=(x,y).
First of all, we pose an assumption under which all solutions to system (1.5) are reasonable in biological sense, the similar proof can be found in [13] and we omit it here.
Lemma 2.1. Under the assumption (H0):σ1<√2, σ2<√2, there is a unique global positive solution (X(t),Y(t))T to system (1.5) on R2,o+ for any given initial value (X0,Y0)∈R2,o+ a.s..
Therefore the rest of this paper is carried out under this assumption and below we provide some important properties of the solutions to system (1.5). In fact, they are based on the results in Lemma 2.1 and the detailed proofs are similar to that in [2].
Corollary 2.2. For any ε>0, H>1, T>0, there is an ¯H=¯H(ε,H,T)>1 such that
P{¯H−1≤Xz(t)≤¯H, t∈[0,T]}≥1−ε, z∈[H−1,H]×[0,H], |
and
P{¯H−1≤Yz(t)≤¯H, t∈[0,T]}≥1−ε, z∈[0,H]×[H−1,H]. |
Now we consider the equations on the boundary. On the x-axis,
dφ(t)=φ(t)[1−φ(t)]dt−σ1Y∗φ(t)dB1(t). | (2.1) |
Let ξt=lnφ(t), where ξt is a function of t, then
dξt=[1−12σ21(Y∗)2−eξt]dt−σ1Y∗dB1(t). |
According to the method in [7], if σ1<√2Y∗, the diffusion (2.1) has a unique invariant probability measure π∗1 in (0,∞) with density
f∗1(ϕ)=c∗1ϕ2σ21(Y∗)2−2exp(−2σ21(Y∗)2ϕ), |
where c∗1 is the normalizing constant and
1c∗1=∫∞0u2σ21(Y∗)2−2exp(−2σ21(Y∗)2u)du. |
According to the Ergodic Theorem in [14,15], for any measurable function g(⋅):R+→R satisfying that ∫∞0|g(ϕ)|f∗1(ϕ)dϕ<∞, we have
P{limT→∞1T∫T0g(φx(t))dt=∫∞0g(ϕ)f∗1(ϕ)dϕ}=1, x>0, | (2.2) |
where φx(t) is the solution to (2.1) starting at x. In particular, for any p∈(−∞,3),
P{limT→∞1T∫T0φpx(t)dt=Qp:=∫∞0ϕpf∗1(ϕ)dϕ<∞}=1, x>0. | (2.3) |
In fact, Q1 and Q2 can be calculated directly by the expression of c∗1, that is,
Q1=∫∞0ϕ[c∗1ϕ2σ21(Y∗)2−2exp(−2σ21(Y∗)2ϕ)]dϕ=c∗1∫∞0ϕ2σ21(Y∗)2−1exp(−2σ21(Y∗)2ϕ)dϕ=c∗1(2σ21(Y∗)2−1)σ21(Y∗)22∫∞0ϕ2σ21(Y∗)2−2exp(−2σ21(Y∗)2ϕ)dϕ=1−12σ21(Y∗)2. |
Analogously, Q2=1−12σ21(Y∗)2. Define
λ1=∫∞0(1−12σ22(X∗)2−(k2−σ22X∗)ϕ−12σ22ϕ2)f∗1(ϕ)dϕ=1−12σ22(X∗)2−(k2−σ22X∗)Q1−12σ22Q2=1−12σ22(X∗)2−(k2−σ22X∗)(1−12σ21(Y∗)2)−12σ22(1−12σ21(Y∗)2)=1−12σ22(X∗)2−(k2−σ22X∗+12σ22)(1−12σ21(Y∗)2). | (2.4) |
Similarly, the diffusion equation on the y-axis is
dψ(t)=ψ(t)(1−ψ(t))dt−σ2X∗ψ(t)dB2(t), |
which has a unique invariant probability measure π∗2 in (0,∞) if σ2<√2X∗. Likewise, define
λ2=1−12σ21(Y∗)2−(k1−σ21Y∗+12σ21)(1−12σ22(X∗)2). |
Below we explain the definitions of λi (i=1,2) and how to use them. To investigate whether Yz(t) converges to zero or not, we consider the Lyapunov exponent of Yz(t) when Yz(t) is small for a sufficiently long time. Therefore, we consider the following equation
lnYz(T)T=lnyT+1T∫T0[1−12σ22(Xz(t)−X∗)2−Yz(t)−k2Xz(t)]dt +1T∫T0σ2(Xz(t)−X∗)dB2(t), | (2.5) |
which is derived from Itô's formula. Moreover, it can be rewritten as
lnYz(T)T=lnyT+1T∫T0[1−12σ22(X∗)2−Yz(t)−(k2−σ22X∗)Xz(t)−12σ22X2z(t)]dt +1T∫T0σ2(Xz(t)−X∗)dB2(t). | (2.6) |
When T is sufficiently large, on the right side of (2.6), the first term tends to zero, and the third term is also very small according to the strong law of large numbers for martingales. Intuitively, if Yz(t) is small in [0,T], then Xz(t) is close to φx(t). By the ergodicity, lnYz(T)T is close to λ1.
In order to study the relationship between the two values λi (i=1,2) and the survival situation of the two species, the assumption
(H1):σ1<√2Y∗, σ2<√2X∗ |
should be satisfied, because it guarantees the existence of λ1 and λ2. Moreover, the following assumption
(H2):σ1≤√k1Y∗, σ2≤√k2X∗ |
is essential in the latter sections.
Recall that (X∗,Y∗)=(1−k11−k1k2,1−k21−k1k2), then X∗,Y∗<1 whether k1,k2<1 or k1,k2>1. Obviously, assumption (H1) implies assumption (H0), which implies that λi (i=1,2) can exist with assumption (H0). Interestingly, we find that assumption (H1) also implies assumption (H2), but there is no relationship between assumptions (H0) and (H2).
From now on, we always assume that assumptions (H0) and (H2) are satisfied. In addition, we use the definitions of stochastic coexistence and competitive exclusion in [8] as follows.
Definition 3.1 The populations of two species modeled by (1.5) are said to stochastically coexist if for any ε>0, there is an M=M(ε)>1 such that
lim inft→∞P{M−1≤X(t), Y(t)≤M}≥1−ε. |
The competitive exclusion is said to take place almost surely if
P{limt→∞X(t)=0 or limt→∞Y(t)=0}=1. |
Below we present our main results of the coexistence and the competitive exclusion of the two species in system (1.5), the detailed proofs are provided in the next two sections, respectively.
Theorem 3.2. Under assumptions (H0) and (H2), if λ1>0 and λ2>0, then the two species coexist. Moreover, all solutions converge to the positive equilibrium (X∗,Y∗).
Theorem 3.3. Under assumptions (H0) and (H2), if λ1<0 and λ2<0, then X(t) or Y(t) goes extinct. For any z∈R2,o+, we have
P{limt→∞lnXz(t)t=λ2}=pz, P{limt→∞lnYz(t)t=λ1}=qz, |
where pz>0, qz>0 and pz+qz=1. Moreover, if Yz(t) converges to zero, then the distribution of Xz(t) converges weakly to π∗1, and the other case is analogous.
Remark 3.4 We remark that since λ1 and λ2 can be rewritten as
λ1=λ1(σ1,σ2)=(1−k2)−σ22[12(X∗)2+(12−X∗)(1−12σ21(Y∗)2)], |
λ2=λ2(σ1,σ2)=(1−k1)−σ21[12(Y∗)2+(12−Y∗)(1−12σ22(X∗)2)], |
then when σi (i=1,2) are relatively small, k1,k2<1 leads to λ1,λ2>0, while k1,k2>1 leads to λ1,λ2<0. Therefore, relatively small random perturbations will not destroy the dynamic behaviors of the deterministic system.
In this section, we consider the stochastic coexistence of system (1.5) and prove Theorem 3.2. Define the stopping time
τσz=inf{t≥0:Yz(t)≥σ}. |
Lemma 4.1. For any T>1, ε>0, σ>0, there is a δ=δ(T,ε,σ)>0 such that
P{τσz≥T}≥1−ε, z∈(0,∞)×(0,δ]. |
Proof. By the exponential martingale inequality, we have P(Ωz1)≥1−ε, where
Ωz1={∫t0σ2(Xz(s)−X∗)dB2(s)<ln1ε+12∫t0σ22(Xz(s)−X∗)2ds, t≥0}. |
In view of (2.5), for any ω∈Ωz1 we have
lnYz(t)<lny+ln1ε+∫t0ds=lny+ln1ε+t, t≥0. |
By choosing δ=σεe−T, moreover if y<δ, then Yz(t)<σ for any t<T and any ω∈Ωz1.
Lemma 4.2. For any H>1, T>1, ε>0, ν>0, there is a σ>0 such that for all z∈[H−1,H]×(0,σ],
P{|φx(t)−Xz(t)|<ν, 0≤t≤T∧τσz}≥1−ε. |
Proof. According to Corollary 2.2, there is an ¯H=¯H(H,T,ε) sufficiently large such that
P{(φx(t))∨(Xz(t))≤¯H, t≤T}≥1−ε2, z∈[H−1,H]×(0,1]. |
It follows from Itô's formula that
d(φx(t)−Xz(t))=[(φx(t)−Xz(t))−(φ2x(t)−X2z(t))+k1Xz(t)Yz(t)]dt −[σ1Xz(t)Yz(t)+σ1Y∗(φx(t)−Xz(t))]dB1(t), |
which leads to
|φx(t)−Xz(t)|≤∫t0|φx(u)−Xz(u)|du+∫t0|φ2x(u)−X2z(u)|du+k1∫t0Xz(u)Yz(u)du +σ1|∫t0Xz(u)Yz(u)dB1(u)|+σ1Y∗|∫t0(φx(u)−Xz(u))dB1(u)|. |
Together with the elementary inequality (n∑i=1ai)2≤2nn∑i=1a2i, we have
Esups≤t(φx(s∧ξz)−Xz(s∧ξz))2 ≤32E∫t∧ξz0|φx(u)−Xz(u)|2du+32k21E∫t∧ξz0X2z(u)Y2z(u)du +32E(∫t∧ξz0|φx(u)−Xz(u)|(φx(u)+Xz(u))du)2 +32σ21Esups≤t|∫s∧ξz0Xz(u)Yz(u)dB1(u)|2+32σ21Esups≤t|∫s∧ξz0(φx(u)−Xz(u))dB1(u)|2, | (4.1) |
where ξz:=τσz∧inf{u:(φx(u))∨(Xz(u))≥¯H}.
For any t∈[0,T], it can be estimated that
Esups≤t|∫s∧ξz0Xz(u)Yz(u)dB1(u)|2≤4E|∫t∧ξz0Xz(u)Yz(u)dB1(u)|2≤4E∫t∧ξz0X2z(u)Y2z(u)du, | (4.2) |
Esups≤t|∫s∧ξz0(φx(u)−Xz(u))dB1(u)|2≤4E∫t∧ξz0|φx(u)−Xz(u)|2du, | (4.3) |
E∫t∧ξz0X2z(u)Y2z(u)du≤¯H2σ2T, | (4.4) |
where (4.2) and (4.3) follow from Martingale inequality. By Hölder's inequality,
E(∫t∧ξz0|φx(u)−Xz(u)|(φx(u)+Xz(u))du)2≤4¯H2TE∫t∧ξz0|φx(u)−Xz(u)|2du. | (4.5) |
Applying (4.2), (4.3), (4.4) and (4.5) to (4.1), we obtain that
Esups≤t(φx(s∧ξz)−Xz(s∧ξz))2≤¯m(σ2+E∫t∧ξz0|φx(u)−Xz(u)|2du)≤¯m(σ2+∫t0Esups≤u(φx(s∧ξz)−Xz(s∧ξz))2du), t∈[0,T] |
for some ¯m=¯m(¯H,T)>0. Then it follows from Gronwall's inequality that
Esups≤T(φx(s∧ξz)−Xz(s∧ξz))2≤¯mσ2exp(¯mT). |
Hence
P{sups≤T(φx(s∧ξz)−Xz(s∧ξz))2≥ν2}≤¯mσ2e¯mTν2<ε2 |
for sufficiently small σ. Equivalently,
P{sups≤T|φx(s∧ξz)−Xz(s∧ξz)|<ν}≥1−ε2. | (4.6) |
In fact,
P{s∧ξz=s∧τσz, ∀s∈[0,T]}≥P{sups≤T{(φx(s))∨(φx(s))}≤¯H}≥1−ε2. | (4.7) |
Applying (4.7) to (4.6), we have
P{sups≤T|φx(s∧τσz)−Xz(s∧τσz)|<ν}≥1−ε, |
which yields the desired result.
Lemma 4.3. For any H>1, T>0, ε>0, there is an ˆM=ˆM(ε,H,T)>0 such that
P{|∫T0σ2(Xz(t)−X∗)dB2(t)|≤ˆMε√T}≥1−ε. |
Proof. Since
E|∫T0σ2(Xz(t)−X∗)dB2(t)|2=E∫T0σ22(Xz(t)−X∗)2dt, |
together with Corollary 2.2, for ¯H=¯H(ε,H,T)>1, we have
E∫T0σ22(Xz(t)−X∗)2dt≤σ22(¯H+X∗)2T. |
Then
P{|∫T0σ2(Xz(t)−X∗)dB2(t)|≥ˆMε√T}≤σ22(¯H+X∗)2TεˆM2T. |
By choosing ˆM>σ2(¯H+X∗), we have
P{|∫T0σ2(Xz(t)−X∗)dB2(t)|≥ˆMε√T}<ε. |
Thus the above result is proved.
Proposition 4.4. Assume that λ1>0, then for any ε>0, H>1, there are T=T(ε,H)>0 and δ0=δ0(ε,H)>0 satisfying that for any z∈[H−1,H]×(0,δ0], P(ˆΩz)>1−4ε, where
ˆΩz={lnYz(t)−lny≥λ15T}. |
Proof. By the definition of λ1, for sufficiently small ν,
∫∞0(1−12σ22(X∗)2−(k2−σ22X∗)(ϕ+ν)−12σ22(ϕ+ν)2)f∗1(ϕ)dϕ≥4λ15. |
Let ˆM be in Lemma 4.3 and by the ergodicity of φx(t) [see (2.2)], there is a T=T(ε,H)>25ˆM2ε2λ21 such that
P{1T∫T0(1−12σ22(X∗)2−(k2−σ22X∗)(φH(t)+ν)−12σ22(φH(t)+ν)2)dt≥3λ15}≥1−ε. |
In fact, φx(t)<φH(t) a.s. for all x∈(H−1,H). Otherwise there is a t0∈(0,+∞) such that φx(t0)=φH(t0) because of the continuity of φ(t), which contradicts the uniqueness of solutions. Hence, according to assumption (H2), we have P(Ωz2)≥1−ε, where
Ωz2={∫T0(1−12σ22(X∗)2−(k2−σ22X∗)(φx(t)+ν)−12σ22(φx(t)+ν)2)dt≥3λ15T}. |
By Lemma 4.2, there is a σ=σ(ε,H)>0 such that σ<λ15 and P(Ωz3)≥1−ε, where
Ωz3={|φx(t)−Xz(t)|<ν, 0≤t≤T∧τσz}. |
According to Lemma 4.1, for definite σ, there is a δ0=δ0(ε,H)>0 satisfying that for all z∈[H−1,H]×(0,δ0], P(Ωz4)≥1−ε, where Ωz4={τσz≥T}. Since T>25ˆM2ε2λ21, it follows from Lemma 4.3 that P(Ωz5)≥1−ε, where
Ωz5={|∫T0σ2(Xz(t)−X∗)dB2(t)|≤λ15T}. |
Hence, for any z∈[H−1,H]×(0,δ0], ω∈ˆΩz=∩5i=2Ωzi, we obtain
lnYz(T)−lny≥∫T0(1−12σ22(X∗)2−(k2−σ22X∗)Xz(t)−12σ22X2z(t))dt −∫T0Yz(t)dt−|∫T0σ2(Xz(t)−X∗)dB2(t)|≥∫T0(1−12σ22(X∗)2−(k2−σ22X∗)(φx(t)+ν) −12σ22(φx(t)+ν)2)dt−λ15T−λ15T≥λ15T. |
The proof is completed by noting that P(ˆΩz)=P(∩5i=2Ωzi)>1−4ε.
Proposition 4.5. Suppose that λ1>0, then for any ρ>0, there is a T=T(ρ)>0 and a δ2=δ2(ρ)>0 such that
lim supn→∞1nn−1∑k=0P{Yz(kT)≤δ2}≤ρ, z∈R2,o+. |
The above result indicates that if λ1>0, then Y(t) will not go extinct in finite time. The similar proof can be found in [8], thus we omit the details here.
Proof of Theorem 3.2. Similar to that in [8], for any ε>0 and z∈R2,o+, there is a T=T(ε)>0 and a sufficiently large M, such that
lim infn→∞1nT∫nT0P{M−1≤Xz(t), Yz(t)≤M}dt≥1−ε. |
Then
lim inft→∞1t∫t0P{M−1≤X(s), Y(s)≤M}dt≥1−ε, |
which implies the existence of an invariant probability measure μ∗ and indicates that the two species coexist. Moreover, (X∗,Y∗) is also the unique positive equilibrium of the stochastic system (1.5), it has invariant Dirac measure, thus μ∗=δ(X∗,Y∗), which implies that all solutions converge to (X∗,Y∗) and remain unchanged afterwards.
In this section, we consider the competitive exclusion of system (1.5). If one species goes extinct, we estimate the corresponding Lyapunov exponent of the population, and analyze the behaviors of the other one. For example, if Yz(t) converges to zero, we can obtain the difference between Xz(t) and φx(t) by estimating |lnφx(t)−lnXz(t)| for t≥0.
Lemma 5.1. For any H>1, T>1, ε>0, γ>0, there is a ˜σ>0 such that for any z∈[H−1,H]×(0,˜σ],
P{|lnφx(t)−lnXz(t)|<γ, 0≤t≤T∧τ˜σz}≥1−ε. |
Proof. In view of Corollary 2.2, there is an ¯H=¯H(ε,H,T)>1 sufficiently large such that for any z∈[H−1,H]×[0,H],
P{¯H−1≤φx(t), Xz(t)≤¯H, t≤T}≥1−ε2. |
It follows from ¯H−1≤φx(t), Xz(t)≤¯H that
¯H−1|φx(t)−Xz(t)|≤|lnφx(t)−lnXz(t)|≤¯H|φx(t)−Xz(t)|. |
Thus the desired result is obtained from Lemma 4.2.
Proposition 5.2. Assume that λ1<0. For any H>1, ε>0, γ>0, and λ∈(0,−λ1), there is a ˜δ>0 such that for any z∈[H−1,H]×[0,˜δ],
P({lim supt→∞lnYz(t)t≤−λ}∩{|lnφx(t)−lnXz(t)|≤γ, t≥0})≥1−4ε. |
Proof. From the definition of λ1, we have
∫∞0(12σ22(X∗)2+(k2−σ22X∗)ϕ+12σ22ϕ2)f∗1(ϕ)dϕ=1−λ1<∞. |
For any λ∈(0,−λ1), let d=−λ1−λ4, then by the continuity of integration there are η1,η2,η3∈(0,1) sufficiently small such that
∫∞η1(12σ22(X∗)2(1−η3)+(k2−σ22X∗)(ϕ−η1)+12σ22(1−η3)(ϕ−η1)2)f∗1(ϕ)dϕ ≥1−λ1−d=1+λ+3d, |
∫∞η−12(12σ22(X∗)2(1−η3)+(k2−σ22X∗)(ϕ−η1)+12σ22(1−η3)(ϕ−η1)2)f∗1(ϕ)dϕ≤d. |
By the ergodicity in (2.2), there is a T1=T1(ε,H) such that with a probability greater than 1−ε we have
1t∫t01{φH−1(s)≥η1}(12σ22(X∗)2(1−η3)+(k2−σ22X∗)(φH−1(s)−η1) +12σ22(1−η3)(φH−1(s)−η1)2)ds≥1+λ+2d, t≥T1, | (5.1) |
1t∫t01{φH(s)≥η−12}(12σ22(X∗)2(1−η3)+(k2−σ22X∗)(φH−1(s)−η1) +12σ22(1−η3)(φH−1(s)−η1)2)ds≤2d, t≥T1. | (5.2) |
The uniqueness of solutions implies that for any x∈[H−1,H], φH−1(s)≤φx(s)≤φH(s) a.s. s≥0. According to assumption (H2), with a probability greater than 1−ε, we have that φx(t) also satisfies (5.1) and (5.2). Then for any t≥T1, P(Ωz6)≥1−ε, where
Ωz6={a2−1t∫t01{η1≤φx(t)≤η−12}(12σ22(X∗)2(1−η3)+(k2−σ22X∗)(φx(s)−η1) +12σ22(1−η3)(φx(s)−η1)2)ds≤−λ}. |
In fact, x≥(ϕ−η1)1{η1≤ϕ≤η−12} if |lnϕ−lnx|≤η1η2. Define the stopping time
ϑz=inf{t>0:|lnφx(t)−lnXz(t)|≥γ0:=γ∧(η1η2)}. |
Consequently, for any ω∈Ωz6∩{ϑz≥T1} and t∈[T1,ϑz], we have
1t∫t0(1−12σ22(X∗)2(1−η3)−(k2−σ22X∗)Xz(s)−12σ22(1−η3)X2z(s))ds≤−λ. | (5.3) |
Recall that
lnYz(t)=lny+∫t0(a2−12σ22(X∗)2−b2Yz(s)−(b21−σ22X∗)Xz(s)−12σ22X2z(s))ds +∫t0σ2(Xz(s)−X∗)dB2(s). | (5.4) |
It follows from exponential martingale inequality that
P{∫t0σ2(Xz(s)−X∗)dB2(s)>1η3ln1ε+η32∫t0σ22(Xz(s)−X∗)2ds}≤ε, |
which implies that P(Ωz7)≥1−ε, where
Ωz7={∫t0σ2(Xz(s)−X∗)dB2(s)≤1η3ln1ε+η32∫t0(σ22(X∗)2+σ22X2z(s))ds}. |
From (5.3) and (5.4), for any ω∈Ωz6∩Ωz7∩{ϑz≥T1}, we have
lnYz(t)≤lny+1η3ln1ε−λt, t∈[T1,ϑz]. | (5.5) |
In particular, if y≤1, putting ˜m1=exp(1η3ln1ε)=exp(η−13)ε, then
Yz(t)≤˜m1exp(−λt), t∈[T1,ϑz], ω∈Ωz6∩Ωz7∩{ϑz≥T1}. | (5.6) |
Next we estimate |lnφx(t)−lnXz(t)| for a larger time interval. It follows from Itô's formula that
d(lnφx(t)−lnXz(t))2=f(φx(t),Xz(t),Yz(t))dt+g(φx(t),Xz(t),Yz(t))dB1(t), |
where
f(ϕ,x,y):=−2(ϕ−x)(lnϕ−lnx)+[2(k1−σ21Y∗)y+3σ21y2](lnϕ−lnx) ≤[2(k1−σ21Y∗)y+3σ21y2]|lnϕ−lnx|, |
and
g(ϕ,x,y):=−2σ1y(lnϕ−lnx)≤2σ1y|lnϕ−lnx|, |
which implies that
d(lnϕ−lnx)2≤[2(k1−σ21Y∗)y+3σ21y2]|lnϕ−lnx|dt+2σ1y|lnϕ−lnx|dB1(t). | (5.7) |
Set U=|lnϕ−lnx|2, then (5.7) becomes
dU≤[2(k1−σ21Y∗)y+3σ21y2]√Udt+2σ1y√UdB1(t). |
Let V=√U, it follows from Itô's formula that
dV≤[(k1−σ21Y∗)y+32σ21y2−12σ21y21V]dt+σ1ydB1(t). |
In fact, V=|lnϕ−lnx|, thus
d|lnφx(t)−lnXz(t)|≤[(k1−σ21Y∗)Yz(t)+32σ21Y2z(t)]dt+σ1Yz(t)dB1(t). |
By exponential martingale inequality, P(Ωz8)≥1−ε, where
Ωz8={∫t0σ1Yz(s)dB1(s)≤γ02+1γ0ln1ε∫t0σ21Y2z(s)ds, t≥0}. |
Thus for any ω∈Ωz8, we have
|lnφx(t)−lnXz(t)|≤γ02+∫t0[(k1−σ21Y∗)Yz(s)+˜m2Y2z(s)]ds, | (5.8) |
where ˜m2=σ21(32+1γ0ln1ε).
By assumption (H_{2}) , there is a T_{2} = T_{2}(\varepsilon, H)\geq T_{1} sufficiently large such that
\begin{equation} \left( k_{1}-\sigma_{1}^{2}Y^{\ast}\right)\int_{T_{2}}^{t}{\tilde{m}_{1}e^{-\lambda s}ds}+\tilde{m}_{2}\int_{T_{2}}^{t}{\tilde{m}_{1}^{2}e^{-2\lambda s}ds} \lt \frac{\gamma_{0}}{4}, \ t\geq T_{2} \end{equation} | (5.9) |
and a \tilde{\sigma} = \tilde{\sigma}(\varepsilon, H) < 1 sufficiently small such that
\begin{equation} \Big[ \left( k_{1}-\sigma_{1}^{2}Y^{\ast}\right)\tilde{\sigma}+\tilde{m}_{2}\tilde{\sigma}^{2}\Big]T_{2}\leq \frac{\gamma_{0}}{4}. \end{equation} | (5.10) |
In view of Lemma 4.1 and Lemma 5.1, there is a \tilde{\delta} = \tilde{\delta}(\varepsilon, H) so small that
\begin{equation} \ln \tilde{\delta}+\frac{1}{\eta_{3}}\ln \frac{1}{\varepsilon}-\lambda T_{2} \lt \ln \tilde{\sigma}, \end{equation} | (5.11) |
and
\begin{equation*} \mathbb{P}(\Omega_{9}^{z})\geq 1-\varepsilon, \ z\in [H^{-1}, H]\times(0, \tilde{\delta}], \ \text{where}\ \Omega_{9}^{z} = \big\{ \zeta_{z}: = \vartheta_{z}\wedge \tau_{z}^{\tilde{\sigma}}\geq T_{2}\big\}. \end{equation*} |
Set \tilde{\Omega}^{z} = \cap_{i = 6}^{9}\Omega_{i}^{z} , then \mathbb{P}(\tilde{\Omega}^{z})\geq 1-4\varepsilon . For any \omega\in \tilde{\Omega}^{z}, \ t\geq T_{2} , by using (5.6), (5.9) and (5.10) we obtain
\begin{equation*} \begin{aligned} & \int_{0}^{t\wedge\zeta_{z}}{\Big[ \left( k_{1}-\sigma_{1}^{2}Y^{\ast}\right)Y_{z}(s)+\tilde{m}_{2}Y_{z}^{2}(s)\Big]ds}\\ &\ \ \ \leq \Big[\left( k_{1}-\sigma_{1}^{2}Y^{\ast}\right)\tilde{\sigma}+\tilde{m}_{2}\tilde{\sigma}^{2}\Big]T_{2} +\int_{T_{2}}^{t\wedge\zeta_{z}}{\Big[ \left( k_{1}-\sigma_{1}^{2}Y^{\ast}\right)Y_{z}(s)+ \tilde{m}_{2}Y_{z}^{2}(s)\Big]ds}\\ & \ \ \ \leq \frac{\gamma_{0}}{4}+\int_{T_{2}}^{t\wedge\zeta_{z}}{\Big[\left( k_{1}-\sigma_{1}^{2}Y^{\ast}\right)\tilde{m}_{1}e^{-\lambda s}+ \tilde{m}_{2}\tilde{m}_{1}^{2}e^{-2\lambda s}\Big]ds}\\ &\ \ \ \lt \frac{\gamma_{0}}{4}+\frac{\gamma_{0}}{4} = \frac{\gamma_{0}}{2}. \end{aligned} \end{equation*} |
Therefore, together with (5.8), we have
\begin{equation*} \big|\ln \varphi_{x}(t\wedge\zeta_{z})-\ln X_{z}(t\wedge\zeta_{z})\big| \lt \gamma_{0}. \end{equation*} |
As a result, in \tilde{\Omega}^{z} , t\wedge \zeta_{z} < \vartheta_{z} for any t\geq T_{2} , which implies that \tilde{\Omega}^{z}\subset \{\zeta_{z}\leq \vartheta_{z}\} . Notice that \zeta_{z} = \vartheta_{z}\wedge \tau_{z}^{\tilde{\sigma}} , then \tilde{\Omega}^{z}\subset \{\tau_{z}^{\tilde{\sigma}}\leq \vartheta_{z}\} . For any z\in [H^{-1}, H]\times(0, \tilde{\delta}] and \omega\in \tilde{\Omega}^{z} , it follows from (5.5) and (5.11) that
\begin{equation*} \ln Y_{z}(t\wedge \tau_{z}^{\tilde{\sigma}})\leq \ln y+\frac{1}{\eta_{3}}\ln \frac{1}{\varepsilon}-\lambda (t\wedge \tau_{z}^{\tilde{\sigma}}) \lt \ln \tilde{\sigma}, \ t\geq T_{2}, \end{equation*} |
which means that t\wedge \tau_{z}^{\tilde{\sigma}} < \tau_{z}^{\tilde{\sigma}} for any t\geq T_{2} , z\in [H^{-1}, H]\times(0, \tilde{\delta}] and \omega\in \tilde{\Omega}^{z} . In other word, \tau_{z}^{\tilde{\sigma}} = \vartheta_{z} = \infty .
Hence, for any z\in [H^{-1}, H]\times(0, \tilde{\delta}] ,
\begin{equation*} \begin{aligned} \mathbb{P}\left( \left\{ \limsup\limits_{t\to \infty} \frac{\ln Y_{z}(t)}{t}\leq -\lambda \right\} \cap \left\{\big|\ln \varphi_{x}(t)-\ln X_{z}(t)\big| \leq \gamma, \ t\geq 0 \right\} \right)\geq \mathbb{P}(\tilde{\Omega}^{z})\geq 1-4\varepsilon, \end{aligned} \end{equation*} |
which completes the proof.
Proposition 5.3. Assume that \lambda_{1} < 0 . For any H > 1, \ \varepsilon > 0 , there is a \overline{\delta} > 0 such that for any z\in [H^{-1}, H]\times(0, \overline{\delta}] ,
\begin{equation*} \mathbb{P}\left(\left\{\lim\limits_{t\to \infty}\frac{1}{t}\int_{0}^{t}{X_{z}(s)ds} = Q_{1}\right\} \cap \left\{\lim\limits_{t\to \infty}\frac{1}{t}\int_{0}^{t}{X_{z}^{2}(s)ds} = Q_{2}\right\}\right)\geq 1-\varepsilon. \end{equation*} |
Proof. For any \epsilon > 0 , let \overline{\eta}_{1}, \overline{\eta}_{2}\in (0, 1) be sufficiently small such that
\begin{equation*} \int_{\overline{\eta}_{1}}^{\overline{\eta}_{2}^{-1}}{(\phi-\overline{\eta}_{1})f_{1}^{\ast}(\phi)d\phi}\geq Q_{1}-\frac{\epsilon}{1\vee b_{1}}. \end{equation*} |
According to Proposition 5.2, there is a \overline{\delta} > 0 such that for any z\in [H^{-1}, H]\times(0, \overline{\delta}] , \mathbb{P}(\overline{\Omega}_{1}^{z})\geq 1-\varepsilon , where
\begin{equation*} \overline{\Omega}_{1}^{z} = \left\{ \lim\limits_{t\to \infty}Y_{z}(t) = 0 \right\}\cap \Big\{ \big| \ln \varphi_{x}(t)-\ln X_{z}(t)\big|\leq \overline{\eta}_{1}\overline{\eta}_{2}, \ t\geq 0 \Big\}. \end{equation*} |
Similar to (5.3), for \omega \in \overline{\Omega}_{1}^{z} , we have
\begin{equation} \liminf\limits_{t\to \infty}\frac{1}{t}\int_{0}^{t}{X_{z}(s)ds}\geq Q_{1}-\frac{\epsilon}{1\vee b_{1}}. \end{equation} | (5.12) |
On the other hand, it follows from Itô's formula that
\begin{equation*} \begin{aligned} \frac{\ln X_{z}(t)}{t}& = \frac{\ln x}{t}+1-\frac{1}{2}\sigma_{1}^{2}(Y^{\ast})^{2}+\frac{1}{t}\int_{0}^{t}{\sigma_{1}\big( Y_{z}(s)-Y^{\ast}\big)dB_{1}(s)}\\ & \ \ \ -\frac{1}{t}\int_{0}^{t}{\bigg[ X_{z}(s)+\left( k_{1}-\sigma_{1}^{2}Y^{\ast}\right)Y_{z}(s)+\frac{1}{2}\sigma_{1}^{2}Y_{z}^{2}(s)\bigg]ds} \end{aligned} \end{equation*} |
and
\begin{equation*} \frac{\ln \varphi_{x}(t)}{t} = \frac{\ln x}{t}+1-\frac{1}{2}\sigma_{1}^{2}(Y^{\ast})^{2}-\frac{1}{t}\int_{0}^{t}{\sigma_{1}Y^{\ast}dB_{1}(s)} -\frac{1}{t}\int_{0}^{t}{\varphi_{x}(s)ds}. \end{equation*} |
By the ergodicity of \varphi_{x}(t) and the strong law of large numbers for martingales, we have
\begin{equation*} \lim\limits_{t\to \infty}\left[\frac{1}{t}\int_{0}^{t}{\sigma_{1}Y^{\ast}dB_{1}(s)}+\frac{1}{t}\int_{0}^{t}{ \varphi_{x}(s)ds}\right] = Q_{1} = 1-\frac{1}{2}\sigma_{1}^{2}(Y^{\ast})^{2}\ \ a.s. \end{equation*} |
which implies that
\begin{equation*} \lim\limits_{t\to \infty}\frac{\ln \varphi_{x}(t)}{t} = 0\ \ a.s.. \end{equation*} |
Notice that for any \omega \in \overline{\Omega}_{1}^{z} , \big|\ln \varphi_{x}(t)-\ln X_{z}(t)\big|\leq \overline{\eta}_{1}\overline{\eta}_{2} , then \ln X_{z}(t)\geq \ln \varphi_{x}(t)-\overline{\eta}_{1}\overline{\eta}_{2} . Therefore
\begin{equation*} \liminf\limits_{t\to \infty}\frac{\ln X_{z}(t)}{t}\geq 0\ \ a.s.\ \text{for}\ \omega \in \overline{\Omega}_{1}^{z}. \end{equation*} |
Moreover, Y_{z}(t) converges to zero in \overline{\Omega}_{1}^{z} , then
\begin{equation*} \limsup\limits_{t \to \infty}\frac{1}{t}\int_{0}^{t}{X_{z}(s)ds}\leq 1-\frac{\sigma_{1}^{2}}{2} = Q_{1}\ \ a.s.\ \text{for}\ \omega\in \overline{\Omega}_{1}^{z}. \end{equation*} |
Together with (5.12), for \omega \in \overline{\Omega}_{1}^{z} , we have
\begin{equation} \lim\limits_{t\to \infty}\frac{1}{t}\int_{0}^{t}{X_{z}(s)ds} = Q_{1} = \lim\limits_{t\to \infty}\frac{1}{t}\int_{0}^{t}{\varphi_{x}(s)ds}. \end{equation} | (5.13) |
Recall that
\begin{equation*} \begin{aligned} d\big(\varphi_{x}(t)- X_{z}(t)\big)& = \Big[\big(\varphi_{x}(t)- X_{z}(t)\big)-\big(\varphi_{x}^{2}(t)- X_{z}^{2}(t)\big)+k_{1}X_{z}(t)Y_{z}(t)\Big]dt\\ &\ \ \ -\Big[\sigma_{1}X_{z}(t)Y_{z}(t)+\sigma_{1}Y^{\ast}\big(\varphi_{x}(t)- X_{z}(t)\big)\Big]dB_{1}(t), \end{aligned} \end{equation*} |
which implies that
\begin{equation*} \begin{aligned} \frac{1}{t}\big(\varphi_{x}(t)-X_{z}(t)\big)& = \frac{1}{t}\int_{0}^{t}{\big(\varphi_{x}(s)-X_{z}(s)\big)ds} +\frac{1}{t}\int_{0}^{t}{k_{1}X_{z}(s)Y_{z}(s)ds} -\frac{1}{t}\int_{0}^{t}{\big(\varphi_{x}^{2}(s)-X_{z}^{2}(s)\big)ds}\\ &\ \ \ -\frac{1}{t}\int_{0}^{t}{\sigma_{1}X_{z}(s)Y_{z}(s)dB_{1}(s)} -\frac{1}{t}\int_{0}^{t}{\sigma_{1}Y^{\ast}\big(\varphi_{x}(s)-X_{z}(s)\big)dB_{1}(s)}. \end{aligned} \end{equation*} |
Let t\to \infty , then it follows from (2.3) and (5.13) that
\begin{equation*} \lim\limits_{t\to \infty}\frac{1}{t}\int_{0}^{t}{X_{z}^{2}(s)ds} = Q_{2}. \end{equation*} |
Hence
\begin{equation*} \mathbb{P}\left(\left\{\lim\limits_{t\to \infty}\frac{1}{t}\int_{0}^{t}{X_{z}(s)ds} = Q_{1}\right\} \cap \left\{\lim\limits_{t\to \infty}\frac{1}{t}\int_{0}^{t}{X_{z}^{2}(s)ds} = Q_{2}\right\}\right)\geq \mathbb{P}(\overline{\Omega}_{1}^{z})\geq 1-\varepsilon. \end{equation*} |
Proof of Theorem 3.3. Similar to that in [8], if \lambda_{1} < 0, \ \lambda_{2} < 0 , then for any \varepsilon > 0 and z\in \mathbb{R}_{+}^{2, o} we obtain
\begin{equation*} \mathbb{P}\left\{ \lim\limits_{t\to \infty}X_{z}(t) = 0 \ \text{or}\ \lim\limits_{t\to \infty}Y_{z}(t) = 0 \right\}\geq 1-\varepsilon. \end{equation*} |
Since \varepsilon is taken arbitrarily, and according to Proposition 5.2, we claim p_{z}+q_{z} = 1 , where
\begin{equation*} \mathbb{P}\left\{\lim\limits_{t \to \infty}\frac{\ln X_{z}(t)}{t} = \lambda_{2}\right\} = p_{z} \gt 0, \ \mathbb{P}\left\{\lim\limits_{t \to \infty}\frac{\ln Y_{z}(t)}{t} = \lambda_{1}\right\} = q_{z} \gt 0. \end{equation*} |
If for some z\in \mathbb{R}_{+}^{2, o} , Y_{z}(t) converges to zero, by Propositions 5.2 and 5.3, we obtain that \big|X_{z}(t)-\varphi_{x}(t)\big| is sufficiently small and the distribution of X_{z}(t) converges weakly to \pi_{1}^{\ast} . More details can be found in [8], thus we omit here.
In this paper, we consider a stochastic Lotka-Volterra competition model (1.5) and analyze the cases of coexistence and competitive exclusion, respectively. Firstly, the model makes sense under the assumption that the positive equilibrium (X^{\ast}, Y^{\ast}) of the corresponding deterministic system exists, and it is obtained by perturbing inter-specific competition rates b_{12}, b_{21} , therefore this model is reasonable mathematically. Secondly, (X^{\ast}, Y^{\ast}) is also the coexistence equilibrium of the stochastic system (1.5), which implies that there is an invariant Dirac measure, and which is the biggest difference between our model and other stochastic competitive models. Therefore, the survival analysis for model (1.5) is significative.
Recall that only if k_{1}, k_{2} < 1 or k_{1}, k_{2} > 1 , the unique positive (coexistence) equilibrium (X^{\ast}, Y^{\ast}) of system (1.4) exists, which guarantees the rationality of the stochastic system (1.5). Therefore, we consider system (1.5) in the above two cases.
Firstly, we provide an example to demonstrate the mathematical results of the deterministic system (1.4) by choosing many different initial values.
Example 1. Consider the deterministic system (1.4) with r = 1 and parameters k_{1} = k_{2} = 0.5 and k_{1} = k_{2} = 2 , respectively. Many solutions are obtained by choosing different initial points from either side of the diagonal line. Moreover, the same set of initial points are used in both cases, which is more convenient for comparison. In the former case, (X^{\ast}, Y^{\ast}) = (\frac{2}{3}, \frac{2}{3}) , and it can be seen from the left panel of Figure 1 that all solutions converge to (X^{\ast}, Y^{\ast}) . In the latter case, (X^{\ast}, Y^{\ast}) = (\frac{1}{3}, \frac{1}{3}) , and solutions with different initial values converge to (1, 0) or (0, 1) , which can be seen clearly from the right panel of Figure 1.
In this paper, we construct two values \lambda_{1}, \lambda_{2} that can be directly calculated via those coefficients of system (1.5), and obtain that if they are both positive, all solutions to system (1.5) converge to the unique positive (coexistence) equilibrium (X^{\ast}, Y^{\ast}) , and if they are both negative, X(t) or Y(t) goes extinct. Below we present two examples to illustrate the main mathematical results in this paper by choosing appropriate parameters, and obtain corresponding figures according to the method in [16]. In addition, we just consider the two cases k_{1}, k_{2} < 1 and k_{1}, k_{2} > 1 , and choose some relatively small values for \sigma_{1}, \sigma_{2} such that assumptions (H_{0}) and (H_{2}) both hold.
Example 2. Consider system (1.5) with parameters k_{1} = 0.4, \ k_{2} = 0.5 , then k_{1}, k_{2} < 1 and (X^{\ast}, Y^{\ast}) = (0.75, 0.625) . Let \sigma_{1} = \sigma_{2} = 0.5 , it can be easily verified that assumptions (H_{0}) and (H_{2}) both hold. Moreover, by direct calculation, \lambda_{1} = 0.51 > 0, \ \lambda_{2} = 0.61 > 0 . According to Theorem 3.2, the two species coexist, moreover the solution converges to (X^{\ast}, Y^{\ast}) and keeps on it afterwards, which can be seen clearly from Figure 2.
Example 3. Consider system (1.5) with parameters k_{1} = 2, \ k_{2} = 3 , then k_{1}, k_{2} > 1 and (X^{\ast}, Y^{\ast}) = (0.2, 0.4) . Let \sigma_{1} = \sigma_{2} = 0.5 , clearly assumptions (H_{0}) and (H_{2}) are both satisfied, and \lambda_{1} = -2.02 < 0, \ \lambda_{2} = -1.03 < 0 . By Theorem 3.3, X(t) or Y(t) goes extinct, and below two trials with initial values z_{1} = (1.2, 0.2) and z_{2} = (0.2, 1.2) are provided, respectively. Here the two initial values are chosen symmetrically, which makes it easier to be compared. See Figure 3 and Figure 4.
From Figure 3 we can see that Y_{z_{1}}(t) converges to zero and \lim\limits_{t\to \infty}\frac{\ln Y_{z_{1}}(t)}{t} = \lambda_{1} , and X_{z_{1}}(t) keeps floating up and down around one. Similarly, Figure 4 shows that X_{z_{2}}(t) converges to zero and \lim\limits_{t\to \infty}\frac{\ln X_{z_{2}}(t)}{t} = \lambda_{2} , and Y_{z_{2}}(t) keeps floating up and down around one.
After many trials, we find that if \sigma_{i}\ (i = 1, 2) satisfy assumptions (H_{0}) and (H_{2}) , k_{1}, k_{2} < 1 will lead to \lambda_{1}, \lambda_{2} > 0 , while k_{1}, k_{2} > 1 leads to \lambda_{1}, \lambda_{2} < 0 . In other words, in either case, we cannot find appropriate \sigma_{1}, \sigma_{2} satisfying these two assumptions such that \lambda_{1}\lambda_{2} < 0 .
According to the results in [13], if the noise intensities \sigma_{1}, \sigma_{2} satisfy assumption (H_{0}) and
\begin{equation*} k_{1}k_{2} \lt \left(\frac{k_{2}}{k_{1}}-\frac{1}{2}\sigma_{2}^{2}Y^{\ast}\right) \left(\frac{k_{1}}{k_{2}}-\frac{1}{2}\sigma_{1}^{2}X^{\ast}\right), \end{equation*} |
then the positive (coexistence) equilibrium (X^{\ast}, Y^{\ast}) of the stochastic system (1.5) is globally asymptotically stable. In this paper, similar results are obtained if \lambda_{1}, \lambda_{2} > 0 . The two kinds of conditions must have some connections although there is no obvious relationship between their expressions.
Especially, if \sigma_{1} = \sigma_{2} = 0 , system (1.5) actually becomes the deterministic system (1.4), and \lambda_{1}^{0} = 1-k_{2}, \ \lambda_{2}^{0} = 1-k_{1} . As claimed before, if k_{1}, k_{2} < 1 , then \lambda_{1}^{0}, \lambda_{2}^{0} > 0 , and the positive (coexistence) equilibrium (X^{\ast}, Y^{\ast}) is globally asymptotically stable; if k_{1}, k_{2} > 1 , then \lambda_{1}^{0}, \lambda_{2}^{0} < 0 , and the case of bistability occurs. It implies that our mathematical results for the stochastic system (1.5) can go back to that of the corresponding deterministic system.
According to the above statements, we obtain that if the random perturbations are relatively weak, the dynamic behaviors of the stochastic system (1.5) are similar to those of the corresponding deterministic system (1.4). However, the conjecture that there is no appropriate \sigma_{1}, \sigma_{2} satisfying assumptions (H_{0}) and (H_{2}) such that \lambda_{1}\lambda_{2} < 0 has not been rigorously proved mathematically, and that will be our future work.
This work was partially supported by an NSFC (11571041) and partially supported by an NSERC grant (RGPIN-2015-04581).
The authors declare that they have no competing interests.
[1] | J. D. Murray, Mathematical Biology, Springer-Verlag, Berlin, Heidelberg, 2002. |
[2] | X. Mao, S. Sabais and E. Renshaw, Asymptotic behavior of stochastic Lotka-Volterra model, J. Math. Anal. Appl., 287 (2003), 141–156. |
[3] | N. H. Du and V. H. Sam, Dynamics of a stochastic Lotka-Volterra model perturbed by white noise, J. Math. Anal. Appl., 324 (2006), 82–97. |
[4] | D. Jiang, C. Ji, X. Li, et al., Analysis of autonomous Lotka-Volterra competition systems with random perturbation, J. Math. Anal. Appl., 390 (2012), 582–595. |
[5] | M. Liu and K. Wang, Population dynamical behavior of Lotka-Volterra cooperative systems with random perturbations, Discrete Contin. Dyn. Syst., 33 (2013), 2495–2522. |
[6] | R. Rudnicki, Long-time behaviour of a stochastic prey-predator model, Stochastic Process. Appl., 108 (2003), 93–107. |
[7] | D. H. Nguyen, N. H. Du and T. V. Ton, Asymptotic behavior of predator-prey systems perturbed by white noise, Acta Appl. Math., 115 (2011), 351–370. |
[8] | D. H. Nguyen and G. Yin, Coexistence and exclusion of stochastic competitive Lotka-Volterra models, J. Differ. Equations, 262 (2017), 1192–1225. |
[9] | M. Liu, K. Wang and Q. Wu, Survival analysis of stochastic competitive models in a polluted environment and stochastic competitive exclusion principle, Bull. Math. Biol., 73 (2011), 1969– 2012. |
[10] | X. Mao, Stationary distribution of stochastic population systems, Syst. Control Lett., 60 (2011), 398–405. |
[11] | A. Bahar and X. Mao, Stochastic delay Lotka-Volterra model, J. Math. Anal. Appl., 292 (2004), 364–380. |
[12] | Q. Liu, The effects of time-dependent delays on global stability of stochastic Lotka-Volterra competitive model, Physica A., 420 (2015), 108–115. |
[13] | J. J. Xiong, X. Li and H.Wang, Global asymptotic stability of a Lotka-Volterra competition model with stochasticity in inter-specific competition, Appl. Math. Letters., 89 (2019), 58–63. |
[14] | A. V. Skorokhod, Asymptotic Methods in the Theory of Stochastic Differential Equations, American Mathematical Society, Providence, 1989. |
[15] | L. R. Bellet, Ergodic properties of Markov processes, in Open Quantum System II, Springer, Berlin, Heidelberg, (2006), 1–39. |
[16] | D. J. Higham, An algorithmic introduction to numerical simulation of stochastic differential equations, SIAM Rev., 43 (2001), 525–546. |
1. | Elena Kozlova, Viktoria Sergunova, Ekaterina Sherstyukova, Andrey Grechko, Snezhanna Lyapunova, Vladimir Inozemtsev, Aleksandr Kozlov, Olga Gudkova, Aleksandr Chernysh, Mechanochemical Synergism of Reactive Oxygen Species Influences on RBC Membrane, 2023, 24, 1422-0067, 5952, 10.3390/ijms24065952 | |
2. | Harindri Chaudhary, Mohammad Sajid, Santosh Kaushik, Ali Allahem, Stability analysis of chaotic generalized Lotka-Volterra system via active compound difference anti-synchronization method, 2023, 20, 1551-0018, 9410, 10.3934/mbe.2023413 |