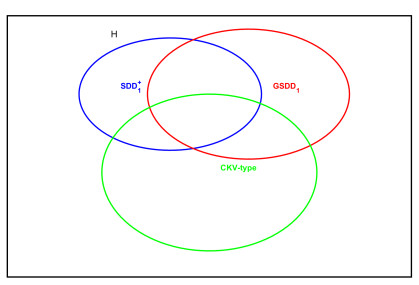
We introduce a policy model coupled with the susceptible–infected- recovered (SIR) epidemic model to study interactions between policy-making and the dynamics of epidemics. We considered both single-region policies as well as game-theoretic models involving interactions among several regions and hierarchical interactions among policy-makers modeled as multi-layer games. We assumed that the policy functions are piece-wise constant with a minimum time interval for each policy stage, considering that policies cannot change frequently in time or be easily followed. The optimal policy was obtained by minimizing a cost function that consists of an implementation cost, an impact cost, and, in the case of multi-layer games, a non-compliance cost. We show, in a case study of COVID-19 in France, that when the cost function is reduced to the impact cost and parameterized as the final epidemic size, the solution approximates that of the optimal control in Bliman et al, (2021) for a sufficiently small minimum policy time interval. For a larger time interval, however, the optimal policy is a step down function, quite different from the step up structure typically deployed during the COVID-19 pandemic. In addition, we present a counterfactual study of how the pandemic would have evolved if herd immunity was reached during the second wave in the county of Los Angeles, California. Finally, we study a case of three interacting counties with and without a governing state.
Citation: Xia Li, Andrea L. Bertozzi, P. Jeffrey Brantingham, Yevgeniy Vorobeychik. Optimal policy for control of epidemics with constrained time intervals and region-based interactions[J]. Networks and Heterogeneous Media, 2024, 19(2): 867-886. doi: 10.3934/nhm.2024039
[1] | Joseph L. Shomberg . Well-posedness and global attractors for a non-isothermal viscous relaxationof nonlocal Cahn-Hilliard equations. AIMS Mathematics, 2016, 1(2): 102-136. doi: 10.3934/Math.2016.2.102 |
[2] | Jianxia He, Ming Li . Existence of global solution to 3D density-dependent incompressible Navier-Stokes equations. AIMS Mathematics, 2024, 9(3): 7728-7750. doi: 10.3934/math.2024375 |
[3] | Geetika Saini, B. N. Hanumagowda, S. V. K. Varma, Jasgurpreet Singh Chohan, Nehad Ali Shah, Yongseok Jeon . Impact of couple stress and variable viscosity on heat transfer and flow between two parallel plates in conducting field. AIMS Mathematics, 2023, 8(7): 16773-16789. doi: 10.3934/math.2023858 |
[4] | Adel M. Al-Mahdi, Maher Noor, Mohammed M. Al-Gharabli, Baowei Feng, Abdelaziz Soufyane . Stability analysis for a Rao-Nakra sandwich beam equation with time-varying weights and frictional dampings. AIMS Mathematics, 2024, 9(5): 12570-12587. doi: 10.3934/math.2024615 |
[5] | Zayd Hajjej . A suspension bridges with a fractional time delay: Asymptotic behavior and Blow-up in finite time. AIMS Mathematics, 2024, 9(8): 22022-22040. doi: 10.3934/math.20241070 |
[6] | S. R. Mishra, Subhajit Panda, Mansoor Alshehri, Nehad Ali Shah, Jae Dong Chung . Sensitivity analysis on optimizing heat transfer rate in hybrid nanofluid flow over a permeable surface for the power law heat flux model: Response surface methodology with ANOVA test. AIMS Mathematics, 2024, 9(5): 12700-12725. doi: 10.3934/math.2024621 |
[7] | Zhongying Liu, Yang Liu, Yiqi Jiang . Global solvability of 3D non-isothermal incompressible nematic liquid crystal flows. AIMS Mathematics, 2022, 7(7): 12536-12565. doi: 10.3934/math.2022695 |
[8] | Mingyu Zhang . On the Cauchy problem of 3D nonhomogeneous micropolar fluids with density-dependent viscosity. AIMS Mathematics, 2024, 9(9): 23313-23330. doi: 10.3934/math.20241133 |
[9] | Takumi Washio, Akihiro Fujii, Toshiaki Hisada . On random force correction for large time steps in semi-implicitly discretized overdamped Langevin equations. AIMS Mathematics, 2024, 9(8): 20793-20810. doi: 10.3934/math.20241011 |
[10] | Said Mesloub, Hassan Altayeb Gadain, Lotfi Kasmi . On the well posedness of a mathematical model for a singular nonlinear fractional pseudo-hyperbolic system with nonlocal boundary conditions and frictional damping terms. AIMS Mathematics, 2024, 9(2): 2964-2992. doi: 10.3934/math.2024146 |
We introduce a policy model coupled with the susceptible–infected- recovered (SIR) epidemic model to study interactions between policy-making and the dynamics of epidemics. We considered both single-region policies as well as game-theoretic models involving interactions among several regions and hierarchical interactions among policy-makers modeled as multi-layer games. We assumed that the policy functions are piece-wise constant with a minimum time interval for each policy stage, considering that policies cannot change frequently in time or be easily followed. The optimal policy was obtained by minimizing a cost function that consists of an implementation cost, an impact cost, and, in the case of multi-layer games, a non-compliance cost. We show, in a case study of COVID-19 in France, that when the cost function is reduced to the impact cost and parameterized as the final epidemic size, the solution approximates that of the optimal control in Bliman et al, (2021) for a sufficiently small minimum policy time interval. For a larger time interval, however, the optimal policy is a step down function, quite different from the step up structure typically deployed during the COVID-19 pandemic. In addition, we present a counterfactual study of how the pandemic would have evolved if herd immunity was reached during the second wave in the county of Los Angeles, California. Finally, we study a case of three interacting counties with and without a governing state.
The nonsingular H-matrix and its subclass play an important role in a lot of fields of science such as computational mathematics, mathematical physics, and control theory, see [1,2,3,4]. Meanwhile, the infinity norm bounds of the inverse for nonsingular H-matrices can be used in convergence analysis of matrix splitting and matrix multi-splitting iterative methods for solving large sparse systems of linear equations [5], as well as bounding errors of linear complementarity problems [6,7]. In recent years, many scholars have developed a deep research interest in the infinity norm of the inverse for special nonsingular H-matrices, such as GSDD1 matrices [8], CKV-type matrices [9], S-SDDS matrices [10], S-Nekrasov matrices [11], S-SDD matrices [12], and so on, which depends only on the entries of the matrix.
In this paper, we prove that M is a nonsingular H-matrix by constructing a scaling matrix D for SDD+1 matrices such that MD is a strictly diagonally dominant matrix. The use of the scaling matrix is important for some applications, for example, the infinity norm bound of the inverse [13], eigenvalue localization [3], and error bounds of the linear complementarity problem [14]. We consider the infinity norm bound of the inverse for the SDD+1 matrix by multiplying the scaling matrix, then we use the result to discuss the error bounds of the linear complementarity problem.
For a positive integer n≥2, let N denote the set {1,2,…,n} and Cn×n(Rn×n) denote the set of all complex (real) matrices. Successively, we review some special subclasses of nonsingular H-matrices and related lemmas.
Definition 1. [5] A matrix M=(mij)∈Cn×n is called a strictly diagonally dominant (SDD) matrix if
|mii|>ri(M),∀i∈N, | (1.1) |
where ri(M)=n∑j=1,j≠i|mij|.
Various generalizations of SDD matrices have been introduced and studied in the literatures, see [7,15,16,17,18].
Definition 2. [8] A matrix M=(mij)∈Cn×n is called a generalized SDD1 (GSDD1) matrix if
{ri(M)−pN2i(M)>0,i∈N2,(ri(M)−pN2i(M))(|mjj|−pN1j(M))>pN1i(M)pN2j(M),i∈N2,j∈N1, | (1.2) |
where N1={i∈N|0<|mii|≤ri(M)}, N2={i∈N||mii|>ri(M)}, pN2i(M)=∑j∈N2∖{i}|mij|rj(M)|mjj|, pN1i(M)=∑j∈N1∖{i}|mij|,i∈N.
Definition 3. [9] A matrix M=(mij)∈Cn×n, with n⩾2, is called a CKV-type matrix if for all i∈N the set S⋆i(M) is not empty, where
S⋆i(M)={S∈∑(i):|mii|>rsi(M),andforallj∈¯S(|mii|−rSi(M))(|mjj|−r¯Sj(M))>r¯Si(M)rSj(M)}, |
with ∑(i)={S⊊N:i∈S} and rSi(M):=∑j∈S∖{i}|mij|.
Lemma 1. [8] Let M=(mij)∈Cn×n be a GSDD1 matrix. Then
‖M−1‖∞≤max{ε,maxi∈N2ri(M)|mii|}min{mini∈N2ϕi,mini∈N1ψi}, | (1.3) |
where
ϕi=ri(M)−∑j∈N2∖{i}|mij|rj(M)|mjj|−∑j∈N1|mij|ε,i∈N2, | (1.4) |
ψi=|mii|ε−∑j∈N1∖{i}|mij|ε−∑j∈N2|mij|rj(M)|mjj|,i∈N1, | (1.5) |
and
ε∈{maxi∈N1pN2i(M)|mii|−pN1i(M),minj∈N2rj(M)−pN2j(M)pN1j(M)}. | (1.6) |
Lemma 2. [8] Suppose that M=(mij)∈Rn×n is a GSDD1 matrix with positive diagonal entries, and D=diag(di) with di∈[0,1]. Then
maxd∈[0,1]n‖(I−D+DM)−1‖∞≤max{max{ε,maxi∈N2ri(M)|mii|}min{mini∈N2ϕi,mini∈N1ψi},max{ε,maxi∈N2ri(M)|mii|}min{ε,mini∈N2ri(M)|mii|}}, | (1.7) |
where ϕi,ψi,andε are shown in (1.4)–(1.6), respectively.
Definition 4. [19] Matrix M=(mij)∈Cn×n is called an SDD1 matrix if
|mii|>ri′(M),foreachi∈N1, | (1.8) |
where
ri′(M)=∑j∈N1∖{i}|mij|+∑j∈N2∖{i}|mij|rj(M)|mjj|, |
N1={i∈N|0<|mii|≤ri(M)},N2={i∈N||mii|>ri(M)}. |
The rest of this paper is organized as follows: In Section 2, we propose a new subclass of nonsingular H-matrices referred to SDD+1 matrices, discuss some of the properties of it, and consider the relationships among subclasses of nonsingular H-matrices by numerical examples, including SDD1 matrices, GSDD1 matrices, and CKV-type matrices. At the same time, a scaling matrix D is constructed to verify that the matrix M is a nonsingular H-matrix. In Section 3, two methods are utilized to derive two different upper bounds of infinity norm for the inverse of the matrix (one with parameter and one without parameter), and numerical examples are used to show the validity of the results. In Section 4, two error bounds of the linear complementarity problems for SDD+1 matrices are given by using the scaling matrix D, and numerical examples are used to illustrate the effectiveness of the obtained results. Finally, a summary of the paper is given in Section. 5.
For the sake of the following description, some symbols are first explained:
N=N1∪N2,N1=N(1)1∪N(1)2≠∅,ri(M)≠0,N1={i∈N|0<|mii|≤ri(M)},N2={i∈N||mii|>ri(M)}, | (2.1) |
N(1)1={i∈N1|0<|mii|≤ri′(M)},N(1)2={i∈N1||mii|>ri′(M)}, | (2.2) |
ri′(M)=∑j∈N1∖{i}|mij|+∑j∈N2|mij|rj(M)|mjj|,i∈N1. | (2.3) |
By the definitions of N(1)1 and N(1)2, for N1=N(1)1∪N(1)2, ri′(M) in Definition 4 can be rewritten as
ri′(M)=∑j∈N1∖{i}|mij|+∑j∈N2∖{i}|mij|rj(M)|mjj|=∑j∈N(1)1∖{i}|mij|+∑j∈N(1)2∖{i}|mij|+∑j∈N2∖{i}|mij|rj(M)|mjj|. | (2.4) |
According to (2.4), it is easy to get the following equivalent form for SDD1 matrices.
A matrix M is called an SDD1 matrix, if
{|mii|>∑j∈N(1)1∖{i}|mij|+∑j∈N(1)2|mij|+∑j∈N2rj(M)|mjj||mij|,i∈N(1)1,|mii|>∑j∈N(1)1|mij|+∑j∈N(1)2∖{i}|mij|+∑j∈N2rj(M)|mjj||mij|,i∈N(1)2. | (2.5) |
By scaling conditions (2.5), we introduce a new class of matrices. As we will see, these matrices belong to a new subclass of nonsingular H-matrices.
Definition 5. A matrix M=(mij)∈Cn×n is called an SDD+1 matrix if
{|mii|>Fi(M)=∑j∈N(1)1∖{i}|mij|+∑j∈N(1)2|mij|r′j(M)|mjj|+∑j∈N2|mij|rj(M)|mjj|,i∈N(1)1,|mii|>F′i(M)=∑j∈N(1)2∖{i}|mij|+∑j∈N2|mij|,i∈N(1)2, | (2.6) |
where N1,N2,N(1)1,N(1)2,andri′(M) are defined by (2.1)–(2.3), respectively.
Proposition 1. If M=(mij)∈Cn×n is an SDD+1 matrix and N(1)1≠∅, then ∑j∈N(1)2|mij|+∑j∈N2|mij|≠0 for i∈N(1)1.
Proof. Assume that there exists i∈N(1)1 such that ∑j∈N(1)2|mij|+∑j∈N2|mij|=0. We find that
|mii|>∑j∈N(1)1∖{i}|mij|=∑j∈N(1)1∖{i}|mij|+∑j∈N(1)2∖{i}|mij|+∑j∈N2|mij|rj(M)|mjj|=∑j∈N(1)1∖{i}|mij|+∑j∈N2|mij|rj(M)|mjj|=r′i(M), |
which contradicts i∈N(1)1. The proof is completed.
Proposition 2. If M=(mij)∈Cn×n is an SDD+1 matrix with N(1)2=∅, then M is also an SDD1 matrix.
Proof. From Definition 5, we have
|mii|>∑j∈N(1)1∖{i}|mij|+∑j∈N2|mij|rj(M)|mjj|,∀i∈N(1)1. |
Because of N1=N(1)1, it holds that
|mii|>∑j∈N1∖{i}|mij|+∑j∈N2|mij|rj(M)|mjj|=ri′(M),∀i∈N1. |
The proof is completed.
Example 1. Consider the following matrix:
M1=(8−52339624−290−31210). |
In fact, N1={1,2} and N2={3,4}. Through calculations, we obtain that
r1(M1)=10,r2(M1)=11,r3(M1)=6,r4(M1)=6, |
r1′(M1)=|m12|+|m13|r3(M1)|m33|+|m14|r4(M1)|m44|≈8.1333, |
r2′(M1)=|m21|+|m23|r3(M1)|m33|+|m24|r4(M1)|m44|=8.2000. |
Because of |m11|=8<8.1333=r1′(M1), M1 is not an SDD1 matrix.
Since
r′1(M1)≈8.1333>|m11|=8, |
r′2(M1)=8.2000<|m22|=9, |
then N(1)1={1}, N(1)2={2}. As
|m11|=8>|m12|r′2(M1)|m22|+|m13|r3(M1)|m33|+|m14|r4(M1)|m44|≈7.6889, |
|m22|=9>|m23|+|m24|=8, |
M1 is an SDD+1 matrix by Definition 5.
Example 2. Consider the following matrix:
M2=(1548−77−51−216). |
In fact, N1={2} and N2={1,3}. By calculations, we get
r1(M2)=12,r2(M2)=12,r3(M2)=3, |
r2′(M2)=0+|m21|r1(M2)|m11|+|m23|r3(M2)|m33|=6.5375. |
Because of |m22|=7>6.5375=r2′(M2), M2 is an SDD1 matrix.
According to
r′2(M2)=6.5375<|m22|=7, |
we know that N(1)2={2}. In addition,
|m22|=7<0+|m21|+|m23|=12, |
and M2 is not an SDD+1 matrix.
As shown in Examples 1 and 2 and Proposition 2, it can be seen that SDD+1 matrices and SDD1 matrices have an intersecting relationship:
{SDD1}⊈{SDD+1} and {SDD+1}⊈{SDD1}. |
The following examples will demonstrate the relationships between SDD+1 matrices and other subclasses of nonsingular H-matrices.
Example 3. Consider the following matrix:
M3=(401−2120104.14620−2334804−620230−42040). |
In fact, N1={2,3} and N2={1,4,5}. Through calculations, we get that
r1(M3)=6,r2(M3)=14.1,r3(M3)=34,r4(M3)=12,r5(M3)=36, |
r′2(M3)=|m23|+|m21|r1(M3)|m11|+|m24|r4(M3)|m44|+|m25|r5(M3)|m55|=11.9>|m22|, |
r′3(M3)=|m32|+|m31|r1(M3)|m11|+|m34|r4(M3)|m44|+|m35|r5(M3)|m55|=14.6<|m33|, |
and N(1)1={2}, N(1)2={3}. Because of
|m22|>|m23|r′3(M3)|m33|+|m21|r1(M3)|m11|+|m24|r4(M3)|m44|+|m25|r5(M3)|m55|≈9.61, |
|m33|=33>0+|m31|+|m34|+|m35|=32. |
So, M3 is an SDD+1 matrix. However, since |m22|=10<11.9=r′2(M3), then M3 is not an SDD1 matrix. And we have
pN11(M3)=3,pN12(M3)=4.1,pN13(M3)=2,pN14(M3)=10,pN15(M3)=6, |
pN21(M3)=2.4,pN22(M3)=7.8,pN23(M3)=12.6,pN24(M3)=1.8,pN25(M3)=4.5. |
Note that, taking i=1,j=2, we have that
(r1(M3)−pN21(M3))(|m22|−pN12(M3))=21.24<pN11(M3)pN22(M3)=23.4. |
So, M3 is not a GSDD1 matrix. Moreover, it can be verified that M3 is not a CKV-type matrix.
Example 4. Consider the following matrix:
M4=(−10.4000.5100−12.11−0.51−20.311). |
It is easy to check that M4 is a CKV-type matrix and a GSDD1 matrix, but not an SDD+1 matrix.
Example 5. Consider the following matrix:
M5=(30.78666.755.256362.2533.78361.54.532.256633.036665.2530.752.25628.531.52.254.54.50.755.251.50.7529.28662.255.254.51.50.755.2535.2835.255.2534.5636.7530.033.754.50.753.757.55.250.750.7529.28). |
We know that M5 is not only an SDD+1 matrix, but also CKV-type matrix and GSDD1 matrix.
According to Examples 3–5, we find that SDD+1 matrices have intersecting relationships with the CKV-type matrices and GSDD1 matrices, as shown in the Figure 1 below. In this paper, we take N1≠∅ and ri(M)≠0, so we will not discuss the relationships among SDD matrices, SDD+1 matrices, and GSDD1 matrices.
Example 6. Consider the tri-diagonal matrix M∈Rn×n arising from the finite difference method for free boundary problems [8], where
M6=(b+αsin(1n)c0⋯0ab+αsin(2n)c⋯0⋱⋱⋱0⋯ab+αsin(n−1n)c0⋯0ab+αsin(1)). |
Take n=12000, a=5.5888, b=16.5150, c=10.9311, and α=14.3417. It is easy to verify that M6 is an SDD+1 matrix, but not an SDD matrix, a GSDD1 matrix, an SDD1 matrix, nor a CKV-type matrix.
As is shown in [19] and [8], SDD1 matrix and GSDD1 matrix are both nonsingular H-matrices, and there exists an explicit construction of the diagonal matrix D, whose diagonal entries are all positive, such that MD is an SDD matrix. In the following, we construct a positive diagonal matrix D involved with a parameter that scales an SDD+1 matrix to transform it into an SDD matrix.
Theorem 1. Let M=(mij)∈Cn×n be an SDD+1 matrix. Then, there exists a diagonal matrix D=diag(d1,d2,⋯,dn) with
di={1,i∈N(1)1,ε+r′i(M)|mii|,i∈N(1)2,ε+ri(M)|mii|,i∈N2, | (2.7) |
where
0<ε<mini∈N(1)1pi, | (2.8) |
and for all i∈N(1)1, we have
pi=|mii|−∑j∈N(1)1∖{i}|mij|−∑j∈N(1)2|mij|r′j(M)|mjj|−∑j∈N2|mij|rj(M)|mjj|∑j∈N(1)2|mij|+∑j∈N2|mij|, | (2.9) |
such that MD is an SDD matrix.
Proof. By (2.6), we have
|mii|−∑j∈N(1)1∖{i}|mij|−∑j∈N(1)2|mij|r′j(M)|mjj|−∑j∈N2|mij|rj(M)|mjj|>0. | (2.10) |
From Proposition 1, for all i∈N(1)1, it is easy to know that
pi=|mii|−∑j∈N(1)1∖{i}|mij|−∑j∈N(1)2|mij|r′j(M)|mjj|−∑j∈N2|mij|rj(M)|mjj|∑j∈N(1)2|mij|+∑j∈N2|mij|>0. | (2.11) |
Immediately, there exists a positive number ε such that
0<ε<mini∈N(1)1pi. | (2.12) |
Now, we construct a diagonal matrix D=diag(d1,d2,⋯,dn) with
di={1,i∈N(1)1,ε+r′i(M)|mii|,i∈N(1)2,ε+ri(M)|mii|,i∈N2, | (2.13) |
where ε is given by (2.12). It is easy to find that all the elements in the diagonal matrix D are positive. Next, we will prove that MD is strictly diagonally dominant.
Case 1. For each i∈N(1)1, it is not difficult to find that |(MD)ii|=|mii|. By (2.11) and (2.13), we have
ri(MD)=∑j∈N(1)1∖{i}dj|mij|+∑j∈N(1)2dj|mij|+∑j∈N2dj|mij|=∑j∈N(1)1∖{i}|mij|+∑j∈N(1)2(ε+r′j(M)|mjj|)|mij|+∑j∈N2(ε+rj(M)|mjj|)|mij|=∑j∈N(1)1∖{i}|mij|+∑j∈N(1)2|mij|r′j(M)|mjj|+∑j∈N2|mij|rj(M)|mjj|+ε(∑j∈N(1)2|mij|+∑j∈N2|mij|)<|mii|=|(MD)ii|. |
Case 2. For each i∈N(1)2, we obtain
|(MD)ii|=|mii|(ε+r′i(M)|mii|)=ε|mii|+r′i(M). | (2.14) |
From (2.3), (2.13), and (2.14), we derive that
ri(MD)=∑j∈N(1)1|mij|+∑j∈N(1)2∖{i}|mij|r′j(M)|mjj|+∑j∈N2|mij|rj(M)|mjj|+ε(∑j∈N(1)2∖{i}|mij|+∑j∈N2|mij|)≤r′i(M)+ε(∑j∈N(1)2∖{i}|mij|+∑j∈N2|mij|)<r′i(M)+ε|mii|=|(MD)ii|. |
The first inequality holds because of |mii|>r′i(M) for any i∈N(1)2, and
r′i(M)=∑j∈N1∖{i}|mij|+∑j∈N2|mij|rj(M)|mjj|=∑j∈N(1)1|mij|+∑j∈N(1)2∖{i}|mij|+∑j∈N2|mij|rj(M)|mjj|≥∑j∈N(1)1|mij|+∑j∈N(1)2∖{i}|mij|r′j(M)|mjj|+∑j∈N2|mij|rj(M)|mjj|. |
Case 3. For each , we have
(2.15) |
Meanwhile, for each , it is easy to get
(2.16) |
and
(2.17) |
From (2.13), (2.15), and (2.16), it can be deduced that
So, for . Thus, is an matrix. The proof is completed.
It is well known that the -matrix is nonsingular if there exists a diagonal matrix such that is an matrix (see [1,19]). Therefore, from Theorem 1, matrices are nonsingular -matrices.
Corollary 1. Let be an matrix. Then, is also an -matrix. If, in addition, has positive diagonal entries, then .
Proof. We see from Theorem 1 that there is a positive diagonal matrix such that is an matrix (cf. (M35) of Theorem 2.3 of Chapter 6 of [1]). Thus, is a nonsingular -matrix. Since the diagonal entries of and are positive, has positive diagonal entries. From the fact that is an matrix, it is well known that , which means .
In this section, we start to consider two infinity norm bounds of the inverse of matrices. Before that, some notations are defined:
(3.1) |
(3.2) |
(3.3) |
Next, let us review an important result proposed by Varah (1975).
Theorem 2. [20] If is an matrix, then
(3.4) |
Theorem 2 can be used to bound the infinity norm of the inverse of an matrix. This theorem together with the scaling matrix allows us to gain the following Theorem 3.
Theorem 3. Let be an matrix. Then,
(3.5) |
where , are defined in (2.8), (2.9), and (3.1)–(3.3), respectively.
Proof. By Theorem 1, there exists a positive diagonal matrix such that is an matrix, where is defined as (2.13). Hence, we have the following result:
(3.6) |
and
where is given by (2.8). Note that is an matrix, by Theorem 2, we have
However, there are three scenarios to solve . For , we get
For , we have
For , we obtain
Hence, according to (3.6) we have
The proof is completed.
It is noted that the upper bound in Theorem 3 is related to the interval values of the parameter. Next, another upper bound of is given, which depends only on the elements in the matrix.
Theorem 4. Let be an matrix. Then,
(3.7) |
where and are shown in (2.6).
Proof. By the well-known fact (see[21,22]) that
(3.8) |
for some , we have
(3.9) |
Assume that there is a unique such that , then
When , let (), and (). Then we have
Which implies that
For , let . It follows that
Hence, we obtain that
For , we get
and
which implies that
To sum up, we obtain that
The proof is completed.
Next, some numerical examples are given to illustrate the superiority of our results.
Example 7. Consider the following matrix:
It is easy to verify that is an matrix. However, we know that is not an matrix, a matrix, an matrix and a -type matrix. By the bound in Theorem 3, we have
When , we get
The range of parameter values in Theorem 3 is not empty set, and its optimal solution can be illustrated through examples. In Example 7, the range of values for the error bound and its optimal solution can be seen from Figure 2. The bound for Example 7 is (8.1633,100), and the optimal solution for Example 7 is 8.1633.
However, according to Theorem 4, we obtain
Through this example, it can be found that the bound of Theorem 4 is better than Theorem 3 in some cases.
Example 8. Consider the following matrix:
Here, , , , , , , , , , , , and . It is easy to verify that is an matrix. However, we can get that is not an matrix, a matrix, an matrix, and a -type matrix. By the bound in Theorem 3, we have
When , we have
If , we have
Taking , then it is easy to calculate
By the bound in Theorem 4, we have
Example 9. Consider the following matrix:
It is easy to verify that the matrix is a matrix and an matrix. When is a matrix, it can be calculated according to Lemma 1 that
According to Figure 3, if we take , we can obtain an optimal bound, namely
When is an matrix, it can be calculated according to Theorem 3. We obtain
According to Figure 3, if we take , we can obtain an optimal bound, namely
However, according to Theorem 4, we get
Example 10. Consider the following matrix:
It is easy to verify that the matrix is a -type matrix and an matrix. When is a -type matrix, it can be calculated according to Theorem 21 in [9]
When is an matrix, take according to Theorem 3. We obtain an optimal bound, namely
When is an matrix, it can be calculated according to Theorem 4. We can obtain
From Examples 9 and 10, it is easy to know that the bound in Theorem 3 and Theorem 4 in our paper is better than available results in some cases.
The -matrix refers to a matrix in which all principal minors are positive [19], and it is widely used in optimization problems in economics, engineering, and other fields. In fact, the linear complementarity problem in the field of optimization has a unique solution if and only if the correlation matrix is a -matrix, so the -matrix has attracted extensive attention, see [23,24,25]. As we all know, the linear complementarity problem of matrix , denoted by , is to find a vector such that
(4.1) |
or to prove that no such vector exists, where and . One of the essential problems in is to estimate
where , , , . It is well known that when is a -matrix, there is a unique solution to linear complementarity problems.
In [2], Chen et al. gave the following error bound for ,
(4.2) |
where is the solution of , , and the min operator denotes the componentwise minimum of two vectors. However, for P-matrices that do not have a specific structure and have a large order, it is very difficult to calculate the error bound of . Nevertheless, the above problem is greatly alleviated when the proposed matrix has a specific structure [7,16,26,27,28].
It is well known that a nonsingular -matrix with positive diagonal entries is a -matrix. In [29], when the matrix is a nonsingular -matrix with positive diagonal entries, and there is a diagonal matrix so that is an matrix, the authors propose a method to solve the error bounds of the linear complementarity problem of the matrix . Now let us review it together.
Theorem 5. [29] Assume that is an -matrix with positive diagonal entries. Let , , for all , be a diagonal matrix such that is strictly diagonally dominant by rows. For any , let . Then,
(4.3) |
Next, the error bound of the linear complementarity problem of matrices is given by using the positive diagonal matrix in Theorem 1.
Theorem 6. Suppose that is an matrix with positive diagonal entries, and for any , . Then,
(4.4) |
where , are defined in (2.8), (2.9), and (3.1)–(3.3), respectively.
Proof. Since is an matrix with positive diagonal elements, the existence of a positive diagonal matrix such that is a strictly diagonal dominance matrix can be seen. For , we can get
By Theorem 5, for , we get
For , we have
For , we have
To sum up, it can be seen that
According to Theorems 1 and 5, it can be obtained that
The proof is completed.
It is noted that the error bound of Theorem 6 is related to the interval of the parameter .
Lemma 3. [16] Letting and , for any ,
(4.5) |
Theorem 7. Let be an matrix. Then, is also an matrix, where with , .
Proof. Since , then
By Lemma 3, for any , we have
In addition, , that is, for each .
For any , we have
So, , that is, for each . Therefore, is an matrix.
Next, another upper bound about is given, which depends on the result in Theorem 4.
Theorem 8. Assume that is an matrix with positive diagonal entries, and , with . Then,
(4.6) |
where are given by (2.1), (2.2), and (2.6), respectively.
Proof. Because is an matrix, according to Theorem 7, is also an matrix, where
By (3.7), we can obtain that
According to Theorem 7, for , let
we have
For , we get
For , we can obtain that
To sum up, it holds that
The proof is completed.
The following examples show that the bound (4.6) in Theorem 8 is better than the bound (4.4) in some conditions.
Example 11. Let us consider the matrix in Example 6. According to Theorem 6, by calculation, we obtain
Taking , then
In addition, from Theorem 8, we get
Example 12. Let us consider the matrix in Example 9. Since is a matrix, then, by Lemma 2, we get
From Figure 4, when , the optimal bound can be obtained as follows:
Moreover, is an matrix, and by Theorem 6, we get
From Figure 4, when , the optimal bound can be obtained as follows:
However, according to Theorem 8, we obtain that
Example 13. Consider the following matrix:
Obviously, and . By calculations, we know that the matrix is a -type matrix with positive diagonal entries, and thus is a -type -matrix. It is easy to verify that the matrix is an matrix. By bound (4.4) in Theorem 6, we get
By the bound (4.6) in Theorem 8, we get
while by Theorem 3.1 in [18], it holds that
From Examples 12 and 13, it is obvious to know that the bounds in Theorems 6 and 8 in our paper is better than available results in some cases.
In this paper, a new subclass of nonsingular -matrices, matrices, have been introduced. Some properties of matrices are discussed, and the relationships among matrices and matrices, matrices, and -type matrices are analyzed through numerical examples. A scaling matrix is used to transform the matrix into a strictly diagonal dominant matrix, which proves the non-singularity of matrices. Two upper bounds of the infinity norm of the inverse matrix are deduced by two methods. On this basis, two error bounds of the linear complementarity problem are given. Numerical examples show the validity of the obtained results.
Lanlan Liu: Conceptualization, Methodology, Supervision, Validation, Writing-review; Yuxue Zhu: Investigation, Writing-original draft, Conceptualization, Writing-review and editing; Feng Wang: Formal analysis, Validation, Conceptualization, Writing-review; Yuanjie Geng: Conceptualization, Validation, Editing, Visualization. All authors have read and approved the final version of the manuscript for publication.
The authors declare they have not used Artificial Intelligence (AI) tools in the creation of this article.
This research is supported by Guizhou Minzu University Science and Technology Projects (GZMUZK[2023]YB10), the Natural Science Research Project of Department of Education of Guizhou Province (QJJ2022015), the Talent Growth Project of Education Department of Guizhou Province (2018143), and the Research Foundation of Guizhou Minzu University (2019YB08).
The authors declare no conflicts of interest.
[1] | LA County Department of Public Health, COVID-19: Keeping Los Angeles safe. Los Angeles Mayor's Office, 2020. Available from: https://coronavirus.lacity.gov/. |
[2] |
P. A. Bliman, M. Duprez, How best can finite-time social distancing reduce epidemic final size? J. Theor. Biol., 511 (2021), 110557. https://doi.org/10.1016/j.jtbi.2020.110557 doi: 10.1016/j.jtbi.2020.110557
![]() |
[3] |
P. A. Bliman, M. Duprez, Y. Privat, N. Vauchelet, Optimal immunity control and final size minimization by social distancing for the SIR epidemic model, J Optim Theory Appl, 189 (2021), 408–436. https://doi.org/10.1007/s10957-021-01830-1 doi: 10.1007/s10957-021-01830-1
![]() |
[4] | S. Blower, K. Koelle, J. Mills, Health policy modeling: Epidemic control, HIV vaccines, and risky behavior, Quantitative evaluation of HIV prevention programs, Yale University Press, New Haven, 2008,260–289. |
[5] |
M. C. J. Bootsma, N. M. Ferguson, The effect of public health measures on the 1918 influenza pandemic in U.S. cities, Proc. Natl. Acad. Sci., 104 (2007), 7588–7593. https://doi.org/10.1073/pnas.0611071104 doi: 10.1073/pnas.0611071104
![]() |
[6] |
D. S. Burke, J. M. Epstein, D. A. Cummings, J. I. Parker, K. C. Cline, R. M. Singa, et al., Individual-based computational modeling of smallpox epidemic control strategies, Acad Emerg Med, 13 (2006), 1142–1149. https://doi.org/10.1197/j.aem.2006.07.017 doi: 10.1197/j.aem.2006.07.017
![]() |
[7] | Centers for Disease Control and Prevention, CDC Museum COVID-19 Timeline. Centers for Disease Control and Prevention, 2022. Available from: https://www.cdc.gov/museum/timeline/covid19.html. |
[8] | Centers for Disease Control and Prevention, U.S. State and Territorial Stay-AtHome Orders: March 15, 2020–August 15, 2021 by County by Day. Environmental Public Health Tracking, 2022. Available from: https://data.cdc.gov/Policy-Surveillance/U-S-State-and-Territorial-Stay-At-Home-Orders-Marc/y2iy-8irm. |
[9] | Department of Public Health, Los Angeles, Department of Public Health, Los Angeles, 2022. Available from: http://publichealth.lacounty.gov/. |
[10] |
E. Dong, H. Du, L. Gardner, An interactive web-based dashboard to track COVID-19 in real time, Lancet Infect. Dis., 20 (2020), 533–534. https://doi.org/10.1016/S1473-3099(20)30120-1 doi: 10.1016/S1473-3099(20)30120-1
![]() |
[11] | D. Fudenberg, D. K. Levine, The Theory of Learning in Games, Cambridge: The MIT Press, 1998. |
[12] |
X. Geng, G. G. Katul, F. Gerges, E. Bou-Zeid, H. Nassif, M. C. Boufadel, A kernel-modulated SIR model for COVID-19 contagious spread from county to continent, Proc. Natl. Acad. Sci., 118 (2021), e2023321118. https://doi.org/10.1073/pnas.2023321118 doi: 10.1073/pnas.2023321118
![]() |
[13] |
R. F. Grais, J. H. Ellis, G. E. Glass, Assessing the impact of airline travel on the geographic spread of pandemic influenza, Eur. J. Epidemiol., 18 (2003), 1065–1072. https://doi.org/10.1023/A:1026140019146 doi: 10.1023/A:1026140019146
![]() |
[14] | F. Jia, A. Mate, Z. Li, S. Jabbari, M. Chakraborty, M. Tambe, et al., A game-theoretic approach for hierarchical policy-making, arXiv: 2102.10646, [Preprint], (2012) [cited 2024 September 02]. Available from: http://161.97.88.200/studii/Studiu%20vaccin%20sars%20cov2/studii%20efecte%20adverse%20vaccin/2102.10646.pdf. |
[15] |
M. J. Keeling, P. Rohani, Estimating spatial coupling in epidemiological systems: a mechanistic approach, Ecol. Lett., 5 (2002), 20–29. https://doi.org/10.1046/j.1461-0248.2002.00268.x doi: 10.1046/j.1461-0248.2002.00268.x
![]() |
[16] | I. Z. Kiss, J. C. Miller, P. Simon, Mathematics of Epidemics on Networks: from Exact to Approximate Models, Schweiz: Springer International Publishing, 2017. |
[17] |
P. Kunwar, O. Markovichenko, M. Chyba, Y. Mileyko, A. Koniges, T. Lee, A study of computational and conceptual complexities of compartment and agent based models, Netw. Heterog. Media, 17 (2022), 359–384. https://doi.org/10.3934/nhm.2022011 doi: 10.3934/nhm.2022011
![]() |
[18] |
T. H. Lee, B. Do, L. Dantzinger, J. Holmes, M. Chyba, S. Hankins, et al., Mitigation planning and policies informed by COVID-19 modeling: A framework and case study of the state of Hawaii, Int. J. Environ. Res. Public Health, 19 (2022), 6119. https://doi.org/10.3390/ijerph19106119 doi: 10.3390/ijerph19106119
![]() |
[19] | R. G. Lin II, L.A. county now requires residents to wear face coverings. Los Angeles Times, 2020. Available from: https://www.latimes.com/california/story/2020-04-16/l-a-county-now-requires-residents-to-wear-face-coverings-here-are-the-details. |
[20] | B. Macdonald, P. Shakarian, N. Howard, G. Moores, Spreaders in the network SIR model: an empirical study, arXiv: 1208.4269, [Preprint], (2012) [cited 2024 August 02]. Available from: https://arXiv.org/abs/1208.4269. |
[21] |
A. L. Pitt, K. Humphreys, M. L. Brandeau, Modeling health benefits and harms of public policy responses to the us opioid epidemic, Am. J. Public Health, 108 (2018), 1394–1400. https://doi.org/10.2105/AJPH.2018.304590 doi: 10.2105/AJPH.2018.304590
![]() |
[22] |
S. Ruan, W. Wang, S. A. Levin, The effect of global travel on the spread of SARS, Math Biosci Eng, 3 (2006), 205–218. https://doi.org/10.3934/mbe.2006.3.205 doi: 10.3934/mbe.2006.3.205
![]() |
[23] | R. Tarjan, Depth-first search and linear graph algorithms, 12th Annual Symposium on Switching and Automata Theory (swat 1971), IEEE Computer Society, 1971,114–121. |
[24] |
R. Thompson, C. Gilligan, N. Cunniffe, Will an outbreak exceed available resources for control? Estimating the risk from invading pathogens using practical definitions of a severe epidemic, J R Soc Interface, 17 (2020), 20200690. https://doi.org/10.1098/rsif.2020.0690 doi: 10.1098/rsif.2020.0690
![]() |
[25] |
S. Thurner, P. Klimek, R. Hanel, A network-based explanation of why most COVID-19 infection curves are linear, Proc. Natl. Acad. Sci., 117 (2020), 22684–22689. https://doi.org/10.1073/pnas.2010398117 doi: 10.1073/pnas.2010398117
![]() |
[26] | Wikipedia, California COVID-19 Timeline. Available from: https://en.wikipedia.org/wiki/Timeline_of_the_COVID-19_pandemic_in_California. |
[27] | Wikipedia, COVID-19 pandemic in France. Available from: https://en.wikipedia.org/wiki/COVID-19_pandemic_in_France#Timeline_of_measures. |
[28] |
B. Yuan, H. Li, A. L. Bertozzi, P. J. Brantingham and M. A. Porter, Multivariate spatiotemporal Hawkes processes and network reconstruction, SIAM J. Math. Data Sci., 1 (2019), 356–382. https://doi.org/10.1137/18M1226993 doi: 10.1137/18M1226993
![]() |
1. | Xianting Wang, Yun-guang Lu, Richard De la cruz, Guoqiao You, Global Solutions to a Hydrodynamic Model for Semiconductors with Velocity Relaxation, 2023, 43, 0252-9602, 975, 10.1007/s10473-023-0226-0 |