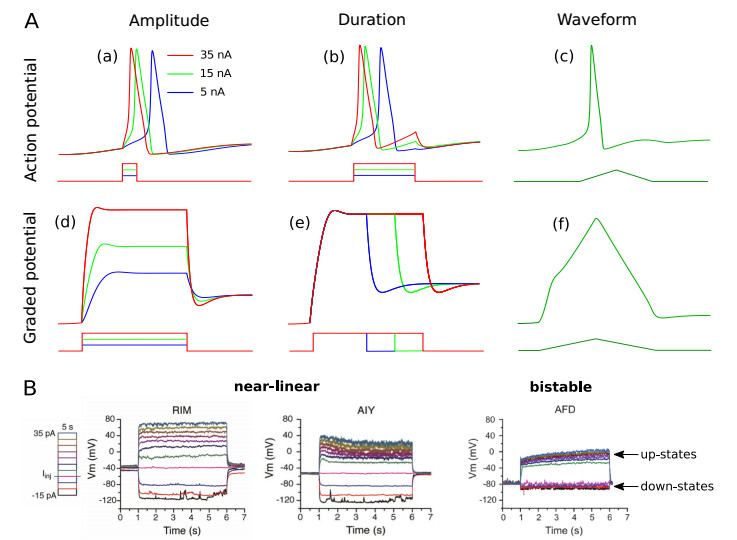
Citation: Takeyuki Nagasawa, Kohei Nakamura. Asymptotic analysis for non-local curvature flows for plane curves with a general rotation number[J]. Mathematics in Engineering, 2021, 3(6): 1-26. doi: 10.3934/mine.2021047
[1] | Yi Yang, Rongfeng Li, Xiangguang Dai, Haiqing Li, Changcheng Xiang . Exploring dynamic behavior and bifurcations in a Filippov neuronal system with a double-tangency singularity. AIMS Mathematics, 2024, 9(7): 18984-19014. doi: 10.3934/math.2024924 |
[2] | Qingyi Cui, Changjin Xu, Wei Ou, Yicheng Pang, Zixin Liu, Jianwei Shen, Muhammad Farman, Shabir Ahmad . Further study on Hopf bifurcation and hybrid control strategy in BAM neural networks concerning time delay. AIMS Mathematics, 2024, 9(5): 13265-13290. doi: 10.3934/math.2024647 |
[3] | Gaeithry Manoharam, Azleena Mohd Kassim, Suad Abdeen, Mohd Shareduwan Mohd Kasihmuddin, Nur 'Afifah Rusdi, Nurul Atiqah Romli, Nur Ezlin Zamri, Mohd. Asyraf Mansor . Special major 1, 3 satisfiability logic in discrete Hopfield neural networks. AIMS Mathematics, 2024, 9(5): 12090-12127. doi: 10.3934/math.2024591 |
[4] | Pablo Fernández-López, Patricio García Báez, Ylermi Cabrera-León, Aleš Procházka, Carmen Paz Suárez-Araujo . Modeling the implications of nitric oxide dynamics on information transmission: An automata networks approach. AIMS Mathematics, 2023, 8(12): 30142-30181. doi: 10.3934/math.20231541 |
[5] | Khalil Ur Rehman, Wasfi Shatanawi, Zead Mustafa . Artificial intelligence (AI) based neural networks for a magnetized surface subject to tangent hyperbolic fluid flow with multiple slip boundary conditions. AIMS Mathematics, 2024, 9(2): 4707-4728. doi: 10.3934/math.2024227 |
[6] | Nurshazneem Roslan, Saratha Sathasivam, Farah Liyana Azizan . Conditional random k satisfiability modeling for k = 1, 2 (CRAN2SAT) with non-monotonic Smish activation function in discrete Hopfield neural network. AIMS Mathematics, 2024, 9(2): 3911-3956. doi: 10.3934/math.2024193 |
[7] | Muhammad Aqmar Fiqhi Roslan, Nur Ezlin Zamri, Mohd. Asyraf Mansor, Mohd Shareduwan Mohd Kasihmuddin . Major 3 Satisfiability logic in Discrete Hopfield Neural Network integrated with multi-objective Election Algorithm. AIMS Mathematics, 2023, 8(9): 22447-22482. doi: 10.3934/math.20231145 |
[8] | Jianwei Jiao, Keqin Su . A new Sigma-Pi-Sigma neural network based on L1 and L2 regularization and applications. AIMS Mathematics, 2024, 9(3): 5995-6012. doi: 10.3934/math.2024293 |
[9] | Ying Xu, Xiaodi Li . The pattern dynamics of interneuronal networks with inhibitory synaptic coupling. AIMS Mathematics, 2025, 10(5): 10976-10993. doi: 10.3934/math.2025498 |
[10] | S. Kathiresan, Ardak Kashkynbayev, K. Janani, R. Rakkiyappan . Multi-stability analysis of fractional-order quaternion-valued neural networks with time delay. AIMS Mathematics, 2022, 7(3): 3603-3629. doi: 10.3934/math.2022199 |
Spiking neurons and spiking neural networks are the most widely studied cases in computational neuroscience due to their ubiquity in neural processes [1,2,3,4,5,6]. However, such neurons are not the only information processing component of the nervous system. Indeed, numerous nervous tissues in both vertebrate and invertebrate animals function normally without triggering spikes. Spiking neurons compress continuous inputs into digital signals for transmitting information via action potentials, while non-spiking neurons do not elicit ones but modulate analog signals (i.e. graded potential responses). Specifically, the amplitude and waveform of the action potentials are essentially invariant with respect to the amplitude, duration, and waveform of the stimulus, unlike graded potentials which are stimulus dependent (Figure 1.A) [7].
Many nervous tissues are mostly composed of non-spiking neurons such as vertebrate retina networks [8], the C. elegans nervous system [9], many interneurons in insects and crustaceans [10], and motorneurons in the Ascaris worm [11]. Retina has long been the object of attention through the modeling of its several major neuronal classes [12,13,14]. More recently, efforts to model the neurons of the C. elegans worm have been made [15,16], including the development of general optimization methods to characterize non-spiking neuron behaviors [17,18]. C. elegans is a well-known model organism in neuroscience due to the simplicity of its nervous system, only composed of 302 neurons and about 7000 synaptic connections [19,20,21]. Despite this simplicity, the C. elegans nervous system shares fundamental general neuronal principles with more sophisticated nervous systems, including the human one [22,23,24].
Two standard non-spiking neuron behaviors exist (Figure 1.B): (ⅰ) near-linear, with smooth depolarizations or hyperpolarizations from the resting potential, and (ⅱ) bistable, with nonlinear transitions between the resting potential and a depolarized potential. Therefore, a bistable cell spontaneously alternates between two membrane plateau potentials: a depolarized potential (up-state) and a relatively hyperpolarized potential (down-state). When the membrane potential is in the up-state (respectively down-state), the neuron is said to be ON (respectively OFF). It is worth noting that many spiking neurons also exhibit such a bistable behavior by triggering action potentials in the up-state, such as mitral cells in olfactory bulb [25], purkinje cells [26], or about 15% of the thalamocortical neurons [27].
Whole-brain calcium imaging of neuronal activity in C. elegans [28,29] reveals that several dozens of non-spiking neurons have biological emergent properties such as collective activity with correlated up- and down-states. The same type of collective behavior, with all-ON and all-OFF network states, has also been observed experimentally in a chemotaxis non-spiking neural network of C. elegans by monitoring the intracellular calcium dynamics of its neurons [30]. An important question is to determine whether these ON- and OFF-states of coupled neurons result from the intrinsic bistability of these cells, or whether they reflect bistable synaptic inputs [26]. Indeed, as an example, Purkinje cells exhibit intrinsic bistable dynamics [26], whereas up- and down-states in cortical pyramidal neurons are not intrinsic but induced by the synaptic activity [31].
The aim of this paper is to understand how emergent biological ON- and OFF-states in non-spiking neural networks can emerge from the intrinsic dynamics of neurons and their couplings. To do this, we use a recently developed simple model with dimensionless parameters that is able to reproduce the qualitative behavior of both types of non-spiking neurons: near-linear and bistable. First, we analyse mathematically the stationary points and the bifurcation dynamics of this model applied to the RIM, AIY and AFD neurons of C. elegans (Figure 1). In particular, RIM and AIY exhibit a near-linear behavior, while AFD displays a bistable one. On this basis, we then give mathematical conditions for determining whether the up- and down-states in non-spiking neural networks are the result of intrinsic bistability or bistable synaptic activity. Finally, using a canonical example of a network of two coupled neurons, we show that up- and down-states in non-spiking neural networks could be driven by bistable neurons which dictate their behaviors to their postsynaptic ones through bistable synaptic inputs. The generality of our study gives a rigorous framework for better understanding how the behavior of coupled non-spiking neurons depends on their intrinsic dynamics and their couplings. In other words, it allows to gain insight into how intrinsic dynamics and couplings of single non-spiking neurons impact neural network behaviors.
The remainder of this paper is organized as follows. Section 2 presents the new non-spiking phenomenological model recently developed in [32]. The analysis of its stationary points and its bifurcation dynamics is carried out in Section 3. Section 4 presents elements for modeling synaptic connections in non-spiking neural networks, with a particular focus on C. elegans. Then, we study both mathematically and numerically how the intrinsic dynamics of a simple presynaptic neuron impact the behavior of a postsynaptic neuron.
Non-spiking neurons are ubiquitous in many nervous tissues. For this reason, a recent simple model has been proposed in [32] as an alternative to conductance-based models (a.k.a. Hodgkin–Huxley type models), known to be computationally expensive and difficult to treat mathematically. In this section, we briefly present this simple non-spiking neuron model, derived from the bifurcations' study of conductance-based models (CBMs) of non-spiking neurons [17,34].
Let V represent the membrane potential of a neuron. The neuron 1-D model takes the general form
τdVdt=−f(V)+I | (2.1) |
where I describes an injection current, τ the constant time for which V reaches its equilibrium value V∗, and V↦f(V) a cubic function which reads as
f(V)=aV3+bV2+cV+d. | (2.2) |
The parameters a, b, c, and d are dimensionless. These are estimated to reproduce the steady-state current of the neuron, noted I∞, shown to determine the neuro-computational features of non-spiking neurons [17]. The biological meaning of the steady-state current is the following: in typical voltage-clamp experiments, the membrane potential is clamped at several values VH (H for hold) for which the resulting currents are measured (Figure 2.A, left). Asymptotic values (t→∞) of those currents, depending only on VH, are called steady-state currents (Figure 2.A, right). As a representative example, the experimental steady-state currents of the RIM, AIY and AFD neurons are represented in Figure 9. The stationary points V∗ of the model (2.1) satisfy
f(V∗)=I |
so that the function V↦f(V), in particular its shape, determines the neuro-computational features of the non-spiking model (2.1). Indeed, two typical shapes of the curve f exist, monotonic and N-shaped (Figure 2.B), each involving two fundamental different behaviors of the neuron:
● As shown in Figure 2.B (left), a model with a monotonic function f has only one equilibrium for any value of I. Such a model displays a near-linear behavior characterized by smooth depolarizations or hyperpolarizations from the resting potential, such as the RIM and AIY neurons (Figure 1.B, left).
● As shown in Figure 2.B (right), a model with a N-shaped function f has one, two, or three equilibria depending on the value of I. Such a model displays a bistable behavior characterized by voltage jumps between the resting potential and a depolarized potential, such as the AFD neuron (Figure 1.B, right).
In a previous work [32], the parameters of the model (2.1) were estimated using the differential evolution algorithm [35] to fit the experimental steady-state current of the RIM, AIY and AFD neurons displayed in Figure 9. Further, we reproduced the mean of the steady-state current (Figure 3.A) in order to capture the experimental trial-to-trial variability of non-spiking neuron responses, which is known to be larger than that of spiking neurons [36]. Finally, Figure 3.B shows the resulting voltage dynamics of the model against an example of experimental voltage traces. Again, the model is not intended to reproduce the specific shown traces, but to capture the trial-to-trial variability of the neuron responses. As explained in [32], although the model slightly diverges from the experimental voltage traces, it preserves the main qualitative features of the neuron dynamics. Indeed, the RIM model is depolarized or hyperpolarized in a smooth manner under current clamp, while the AIY model is more sensitive to hyperpolarization than depolarization inputs with transition point around −30 mV, as in the experimental membrane potential behaviors. Likewise, the voltage jump of the AFD model to its up-state occurs for the same values of current injections as in the biological neuron.
This section aims at studying the phenomenological non-spiking model (2.1) presented in Section 2. The first part analyses the stationary points of the model, while the second studies its bifurcation dynamics. Moreover, we apply the model with the parameters of the RIM, AIY and AFD neurons (Table 2), representative of known types of behaviors of non-spiking cells: near-linear and bistable. All numerical simulations of the models presented in this article are performed with the function ode of the Scilab software. As a first step, some biological hypotheses are formulated and used throughout the manuscript based on experimental studies.
Biological hypotheses throughout the manuscript. Biological cells have limited tolerance to electrical stimulation. This is even more true for the C. elegans neurons due to their small size. Beyond a certain threshold, the stimulus bursts the cell. Therefore, we define the range of the neuron functioning, noted I, as follows:
I∈I:=[m;M] | (3.1) |
where m=−15 pA and M=35 pA as in [33].
Let a, b, c and d be the parameters of the model (2.1). In the following, let A and B be defined by
{A:=27a2B:=54da2+54a(2b327a3−bc3a2) | (3.2) |
so that
˜I:=B2A | (3.3) |
Moreover, let p and q be defined by
{p:=−b23a2+caq(I):=2b327a3−bc3a2+d−Ia | (3.4) |
and I1 and I2 as follows:
{I1:=B+√B2−4AC2AI2:=B−√B2−4AC2A | (3.5) |
where
C=27(2b327a3−bc3a2)2+54da(2b327a3−bc3a2)+27d2a2+4p3 | (3.6) |
Then, the following proposition holds.
Proposition 3.1. Let
Δ(I):=4p3+27q2(I) | (3.7) |
where p and q are defined in (3.4). Moreover, let ˜I be defined by (3.3), and I1 and I2 be defined by (3.5). Then:
1. if Δ(˜I)>0, the model (2.1) has a unique stationary point V∗(I) for all I∈I given by
V∗(I)=3√−q(I)2+√q2(I)4+p327+3√−q(I)2−√q2(I)4+p327 | (3.8) |
2. if Δ(˜I)≤0, then:
● for all I∈]m;I1[∪]I2;M[, the model (2.1) has a unique stationary point V∗(I) given in (3.8).
● for I=I1 and I=I2, the model (2.1) has two stationary points V1∗(I) and V2∗(I) given by
{V1∗(I)=23√−q(I)2V2∗(I)=−3√−q(I)2 | (3.9) |
● for all I∈]I1;I2[, the model (2.1) has three stationary points V1∗(I), V2∗(I) and V3∗(I) given by
{V1∗(I)=√−4p3sin(ρ3)V2∗(I)=√−4p3sin(2π+ρ3)V3∗(I)=√−4p3sin(4π+ρ3) | (3.10) |
where ρ=arcsin(√−27q2(I)4p3).
Proof. The stationary points of the model (2.1) are given by solving
aV3+bV2+cV+d−I=0 | (3.11) |
Let us denote V=X−α/3 with α=b/a. Then, the equation (3.11) can be rewritten as
X3+pX+q=0 | (3.12) |
where p and q are defined in (3.4). The number of roots of the third-degree polynomial (3.12) depends on the sign of the function Δ defined in (3.7) which is its discriminant. Moreover, Δ is a convex parabola, so that the number of stationary points of the model (2.1) is determined by the sign of the minimum of the function Δ. We can rewrite Δ in the following form:
Δ(I)=AI2−BI+C | (3.13) |
where A and B are defined in (3.2), and C is defined in (3.6). Then, we have
Δ′(I)=2AI−B=0⟺I=B2A |
so that the minimum of the function Δ is reached in ˜I=B/2A. From the Cardan-Tartaglia method, if Δ(˜I)>0 then the model (2.1) displays a unique stationary point given by the equation (3.8). Moreover, if Δ(˜I)≤0, then the model (2.1) has one, two, or three stationary points depending on the value of I. The critical values of I at which the number of stationary points changes are those satisfying Δ(I)=0. These are given by I1 and I2 defined in (3.5). Therefore, for all I<I1 and I>I2, Δ(I)>0 so that we have only one stationary point given by (3.8); for I=I1 or I=I2, Δ(I)=0 so that we have two stationary points given by (3.9); for all I1<I<I2, Δ(I)<0 so that we have three stationary points given by (3.10).
Biological interpretation. If Δ(˜I)>0, then the model (2.1) displays a near-linear behavior defined by smooth depolarizations or hyperpolarizations from the resting potential (Figure 1.B, left). If Δ(˜I)<0, then the model (2.1) exhibits a bistable behavior defined by a nonlinear transition between the resting potential and a depolarized potential (Figure 1.B, right). In this case, I1 and I2 defined in (3.5) are the injection current thresholds from which the neuron jumps to its down- and up-state, respectively.
Application to the RIM, AIY and AFD neurons. Here we determine the number and the expression of the stationary points of the three neurons under study (RIM, AIY and AFD). To do this, we compute Δ(˜I), defined in (3.7), with the parameters a, b, c and d given in Table 2, determined in a previous work [32] to reproduce the qualitative behavior of these neurons. The result for each ones is summarized in Table 1 and shown in Figure 4.
Neuron | RIM | AIY | AFD |
Δ(˜I) | 6.18e11 | 6.27e10 | -1.57e07 |
We observe that Δ(˜I)>0 for the RIM and AIY neurons so that the following corollary holds from Proposition 3.1.
Corollary 3.1 (RIM and AIY neurons). Model (2.1) endowed with parameters values relative to the RIM and AIY neurons has a unique stationary point V∗ given in (3.8).
Moreover, Δ(˜I)<0 for the AFD neuron. We compute I1 and I2 defined in (3.5) and we find I1=2.62 and I2=3.12. Then, we have the following corollary:
Corollary 3.2 (AFD neuron). Let I1=2.62 and I2=3.12. Then, model (2.1) endowed with parameters values relative to the AFD neuron displays one, two, or three stationary points depending on the value of I:
● for all I∈]m;I1[∪]I2;M[, AFD has a unique stationary point V∗(I) given in (3.8);
● for I=I1 and I=I2, AFD has two stationary points V1∗(I) and V2∗(I) given in (3.9);
● for all I∈]I1;I2[, AFD has three stationary points V1∗(I), V2∗(I) and V3∗(I) given in (3.10).
Stability of stationary points. Consider I such as F(V∗(I),I)=0 where F is the right-hand side of the model (2.1). The first-order derivative of the function (V,I)↦F(V,I) with respect to V is given by
∂F(V,I)∂V=−3aV2−2bV−c | (3.14) |
Then, for each neuron, we compute from Cardan-Tartaglia formula the equilibria V∗(I) for each I∈I by 0.01pA, as well as the value of the function (3.14) in V∗(I) to determine its nature. As shown in Figure 5, the sign of the function (3.14) is always negative for RIM and AIY, showing that their unique equilibrium point is always stable. Regarding AFD, it depends on the value of I. For any I<I1 and I>I2, the unique equilibrium point is stable. For I=I1 and I=I2, we have one stable and one non-hyperbolic equilibrium point. For any I∈]I1;I2[, we have two stable equilibria and one unstable equilibrium point.
The analysis of the stationary points carried out in the previous section shows the existence of two non-hyperbolic equilibrium points for bistable dynamics of the model (2.1). The following proposition gives information on the dynamics of the model (2.1) near these points.
Proposition 3.2. Let V∗(I∗) be a non-hyperbolic equilibrium point of the model (2.1) such as
3aV∗+b≠0. |
Then, a saddle-node bifurcation occurs at I=I∗.
Moreover, the normal form of the model (2.1) near the stationary point V∗(I∗) is given by
{dηdt=μ−η2if3aV∗+b>0dηdt=μ+η2if3aV∗+b<0 | (3.15) |
where
μ=|3aV∗+b|(I−I∗) |
Proof. For the convenience of readability, we set τ=1.
● We carry out the following change of variables:
{y=V−V∗p=I−I∗ |
The model (2.1) in the new coordinates becomes
dydt=−a(y+V∗)3−b(y+V∗)2−c(y+V∗)−d+p+I∗=−ay3−3aV∗y2−3aV2∗y−aV3∗−by2−2bV∗y−bV2∗−cy−cV∗−d+p+I∗:=g(y,p) | (3.16) |
Therefore, (0,0) is a non-hyperbolic stationary point of the model (3.16).
● The third order term in y is negligible in 0 compared to the second order and first order terms in y. Then, we obtain
dydt=−3aV∗y2−3aV2∗y−aV3∗−by2−2bV∗y−bV2∗−cy−cV∗−d+p+I∗=(−3aV∗−b)y2+(−3aV2∗−2bV∗−c)y−aV3∗−bV2∗−cV∗−d+I∗+p | (3.17) |
Given
−3aV2∗−2bV∗−c=∂g∂y(0,0)=0 |
and
−aV3∗−bV2∗−cV∗−d+I∗=g(0,0)=0 |
the Eq (3.17) becomes
dydt=(−3aV∗−b)y2+p |
By assumption, −3aV∗−b≠0, so that
{dydt=p−(3aV∗+b)y2 if 3aV∗+b>0dydt=p+|3aV∗+b|y2 if 3aV∗+b<0 | (3.18) |
● Finally, we rescale the Eqs (3.18) by considering the change of variable
η=|3aV∗+b|y |
so that
{dηdt=μ−η2 si 3aV∗+b>0dηdt=μ+η2 si 3aV∗+b<0 |
where
μ=|3aV∗+b|p |
Application to the AFD neuron. In the previous section, we highlighted two non-hyperbolic equilibrium points V∗(I1) and V∗(I2) in the case of the AFD neuron (see Figure 5 as an illustration), where I1 and I2 are still defined in (3.5). Indeed,
∂F(V∗(I1),I1)∂V=0 and ∂F(V∗(I2),I2)∂V=0 |
where F is given in (3.14). From Proposition 3.2, the dynamics of the model (2.1) near the non-hyperbolic equilibrium V∗(I1) is given by the following normal form:
dηdt=μ−η2 | (3.19) |
whereas near V∗(I2), the normal form reads as
dηdt=μ+η2. | (3.20) |
Finally, we obtain the bifurcation diagram displayed in Figure 6.
Experimental measurements of the non-spiking neuronal activity in freely C. elegans worms show that ON and OFF network states underlie physiological behaviors [28,29,30]. This section aims at understanding how such correlated up- and down-states of the membrane potential of the neurons can emerge from the intrinsic neuron dynamics and their couplings. To that end, we first present the C. elegans synaptic connections and their modeling, before determining mathematical conditions informing whether the ON- and OFF-states result from the intrinsic neuron dynamics or the synaptic activity.
Anatomical reconstruction of the C. elegans nervous system reveals both chemical and electrical synaptic connections [37]. Synaptic transmission at chemical synapses occurs through the release of neurotransmitters similar to those in more complex vertebrates, such as GABA [38], glutamate [39], acetylcholine [40], and some amines (serotonine, dopamine, etc.) [41]. Regarding C. elegans gap junctions [42], i.e. electrical synapses, numerous works demonstrate their functional equivalence to mammalian gap junctions (we refer to [43] for a recent review). Chemical and eletrical synapses often coexist in same target neurons.
From a general viewpoint, neurons can transmit information through various modes of neurotransmitter release. In the case of C. elegans, experimental biological studies show that neurons release neurotransmitters in a graded manner [44,45,46,47,48]. In addition, there is evidence of a graded release in Ascaris lumbricoides [49,50], another extensively studied nematode related to C. elegans. More precisely, the graded synaptic connection is defined as follows:
Definition 4.1 (Graded synaptic connection [45]). Graded synaptic connection is one in which action potentials are required neither for transmitter release nor for postsynaptic signalling, and in which incremental changes in membrane potential in the soma of the presynaptic neuron cause incremental changes in membrane potential in the soma of the postsynaptic neuron.
Graded release of neurotransmitters in C. elegans has been firstly modeled in [51] based on data from Ascaris lumbricoides [49,50] adapted to the particular case of C. elegans neurons. In the following, we will refine some parameter values based on recent experimental evidences from C. elegans.
Each instance of the models composing the network follow the general equation
τidVidt=−f(Vi)−N∑j=1(Isynij+Igapij)+Iexti | (4.1) |
with
f(Vi)=aiV3i+biV2i+ciVi+di |
where:
● Vi denotes the membrane potential of a neuron i;
● τi, ai, bi, ci, and di are the parameters of the neuron i;
● N is the number of neurons in the network;
● Iexti is any injected current by the experimenter, modeling a sensory input;
● Isynij is the synaptic current flowing into neuron i from neuron j accross a single chemical synapse;
● Igapij the current flowing across a single gap junction between neuron j and neuron i.
Gap junctions are modeled as ohhmic resistances, while channels of chemical synapses are gated in the postsynaptic membrane with inward current. Their respective expressions are therefore given by
Igapij=ggapij(Vi−Vj) |
Isynij=gij(t)(Vi−Eij) |
with:
● Eij the reversal potential for the synaptic conductance, set to 0mV for excitatory synapses and −48mV for inhibitory synapses [51];
● Vj a presynaptic membrane potential;
● ggapij the constant conductance of the gap junction. Recent experimental works [52,53] directly recorded trans-junctional currents between C. elegans neurons, from AVA to VA5, giving a parameter value to about 0.4nS. Therefore, ggapij will be set to 0.4;
● gij the synaptic conductance of the postsynaptic membrane which is gated according to presynaptic potential Vj of a sigmoidal manner. Therefore, t↦gij(t) is described by the equation
dgijdt=gij∞(Vj)−gijτij |
where τij is the synaptic constant time and V↦gij∞(V) is a sigmoidal function relating pre- and postsynaptic membrane potential. In the C. elegans worm, τij≪1, so that the activation of synaptic conductances can be considered as instantaneous [51]. Therefore, the synaptic conductances depend only on the presynaptic membrane potential Vj, i.e.
gij(t)=gij∞(Vj) |
Finally, the expression of gij∞ is given by
gij∞(Vj)=¯gij1+exp(Vij1/2−VjVslope) |
where ¯gij is the maximal postsynaptic conductance, Vij1/2 satisfies gij∞(Vij1/2)=¯gij/2, and Vslope the slope factor where smaller values of Vslope lead to a sharper gij∞.
In the following section, we carry out a mathematical analysis of the dynamics of coupled neurons based on the formalism described in this section.
Let N be the number of neurons in the network, and i∈{1,…,N} a given neuron. Based on the previous section, the dynamics of the membrane potential of the neuron i is given by
τidVidt=−f(Vi)−N∑j=1gij∞(Vj)(Vi−Eij)⏟Isynij−N∑j=1ggapij(Vi−Vj)⏟Igapij+Iexti | (4.2) |
where
gij∞(Vj)=¯gij1+exp(Vij1/2−VjVslope) |
and
f(Vi)=aiV3i+biV2i+ciVi+di. |
Therefore, the system (4.2) can be written as follows:
τidVidt=−aiV3i−biV2i−Ci(Vj)Vi−Di(Vj,Iexti) | (4.3) |
where
{Ci(Vj)=ci+N∑j=1ggapij+N∑j=1gij∞(Vj)Di(Vj,Iexti)=di−N∑j=1gij∞(Vj)Eij−N∑j=1ggapijVj−Iexti |
Stationary point analysis of the coupled models. From (4.3), the stationary points of the membrane potential of the neuron i verify
aiV3i+biV2i+Ci(Vj)Vi+Di(Vj,Iexti)=0 | (4.4) |
where Vj and Iexti are considered as parameters of the system of the neuron i. Then, based on the previous section, the discriminant of the third-degree polynomial (4.4) is given by
Δi(Vj,Iexti)=4pi(Vj)3+27q2i(Vj,Iexti) | (4.5) |
where
{pi(Vj):=−b2i3a2i+Ci(Vj)aiqi(Vj,Iexti):=2b3i27a3i−biCi(Vj)3a2i+Di(Vj,Iexti)ai | (4.6) |
where Ci and Di are defined in (4.3). Then, the following proposition holds.
Proposition 4.1. Let Δi be defined in (4.5), pi and qi be defined in (4.6), and Vj and Iexti be the inputs considered as parameters. Then:
● if Δi>0, the membrane potential of the neuron i has a unique stationary point V∗i(Vj,Iexti) given by
V∗i(Vj,Iexti)=3√−qi(Vj,Iexti)2+√q2i(Vj,Iexti)4+p3i(Vj)27+3√−qi(Vj,Iexti)2−√q2i(Vj,Iexti)4+p3i(Vj)27 |
● if Δi=0, the membrane potential of the neuron i exhibits two stationary points V1∗i(Vj,Iexti) and V2∗i(Vj,Iexti) which reads as
{V1∗i(Vj,Iexti)=23√−qi(Vj,Iexti)2V2∗i(Vj,Iexti)=−3√−qi(Vj,Iexti)2 |
● if Δi<0, the membrane potential of the neuron i has three stationary points V1∗i(Vj,Iexti), V2∗i(Vj,Iexti) and V3∗i(Vj,Iexti) given by
{V1∗i(Vj,Iexti)=√−4pi(Vj)3sin(ρi(Vj,Iexti)3)V2∗i(Vj,Iexti)=√−4pi(Vj)3sin(2π+ρi(Vj,Iexti)3)V3∗i(Vj,Iexti)=√−4pi(Vj)3sin(4π+ρi(Vj,Iexti)3) |
where ρi(Vj,Iexti)=arcsin(√−27q2i(Vj,Iexti)4p3i(Vj)).
Biological interpretation. Beyond informing us about the stationary points of a coupled neuron, Proposition 4.1 tells us about how the coupling impacts the instrinsic dynamics of the coupled neurons. Indeed, if Δi>0, then the neuron i governed by the equation (4.2) displays a near-linear intrinsic dynamics, while it exhibits an intrinsic bistable one if Δi<0.
Application on a canonical example of two coupled neurons. Here we implement a non-spiking neural network of two coupled neurons through a gap junction and an excitatory chemical synapse (Figure 7.A). We consider the AFD bistable neuron as the presynaptic sensory neuron, noted A, and the RIM near-linear neuron as the postsynaptic neuron, noted R. It is worth noting that the behavior of the AFD and RIM neurons represents the two qualitative types of non-spiking behavior (bistable and near-linear), and are representative of two major quantitative classes of C. elegans neurons [33].
The mathematical expression of the network is given by
{τAdVAdt=−aAV3A−bAV2A−cAVA−dA+IextAτRdVRdt=−aRV3R−bRV2R−cRVR−dR−gRA∞(VA)(VR−ERA)−ggapRA(VR−VA) |
The dynamics of the membrane potential VR of the RIM neuron shows up- and down-states when coupled with a bistable presynaptic neuron (Figure 7.B, left), as previously reported in [32]. These up- and down-states are apparent in the bimodal distribution of the membrane potential (Figure 7.B, right), computed using the distplot function of the Seaborn library in Python. This is in agreement with its bistable intracellular calcium dynamics when exposed to odor stimuli [30]. This shows that the connectivity strongly affects the behavior of RIM: the membrane potential of a near-linear neuron can have bimodal dynamics when coupled with a bistable neuron. The question that then arises is whether these up- and down-states result from the intrinsic bistability of the cell, or whether they reflect bistable synaptic inputs. In other words, we want to understand whether the connectivity has modified the intrinsic nature of the RIM neuron, from a near-linear type to a bistable type. To address this issue, we use the Proposition 4.1. As a first step, the equation on the postsynaptic RIM neuron is rewritten in the form (4.3):
τRdVRdt=−aRV3R−bRV2R−CR(VA)⏞(cR+gRA∞(VA)+ggapRA)VR−(dR−gRA∞(VA)ERA−ggapRAVA)⏟DR(VA,0) | (4.7) |
As the chemical synapse is excitatory, we have ERA=0 so that
{CR(VA)=cR+gRA∞(VA)+ggapRADR(VA,0)=dR−ggapRAVA |
Therefore, we plot in Figure 8.A the discriminant ΔR given in (4.5). We observe that ΔR>0 for any value of the presynaptic membrane potential VA. According to Proposition 4.1, the RIM postsynaptic neuron exhibits a unique stationnary point. This allows to conclude that the nature of the intrinsic dynamics of this coupled neuron remains near-linear despite the bimodality of its membrane potential. Finally, Figure 8.B shows the bistable dynamics of the synaptic activity which confers a bimodal membrane potential dynamics to the RIM neuron.
The same analysis has been conducted with the AIY neuron as the postsynaptic neuron, and AFD as the presynaptic neuron. As can be seen in Figure 10, the same results are obtained: the apparent bimodality of the AIY voltages is not the result of an intrinsic change in its nature, but of bistable synaptic inputs.
Experimental observations through calcium imaging in freely moving animals (e.g. in the C. elegans worm) show that non-spiking neural networks exhibit ON- and OFF-states. This paper aimed at understanding how such states could emerge from the intrinsic dynamics of neurons and their couplings. To that end, we used a recently developed simple model of non-spiking neurons from which a mathematical analysis of its dynamics were carried out. In particular, we determined explicit expressions of the stationary points of the model depending on its type, near-linear or bistable. In addition, the bifurcation dynamics of the model were studied, with the determination of its normal form. Then, we determined mathematical conditions for determining whether the ON- and OFF-states in non-spiking neural networks result from the intrinsic neuron dynamics or the synaptic activity. On this basis, we highlighted that the bimodality of the membrane potential of coupled neurons could be a consequence of bistable synaptic inputs, and not of intrinsically bistable neuron dynamics contrary to what one might have expected. Further, we suggested that up- and down-states in non-spiking neural networks could be driven by bistable neurons which dictate their behaviors to their postsynaptic neurons. Such a configuration, with bistable neurons sending electrical signals to near-linear (and bistable) postsynaptic neurons, is ubiquitous in non-spiking neural networks. Indeed, many sensory, inter- and motorneurons in C. elegans are bistable such as ASER [9], RMD [54], AWC [55], ASH [56], AFD [33], AWA [33], AIA [57] and numerous non identified neurons [9]. In the same way, the bipolar [58] and horizontal [59] cells of the retina are bistable and send eletrical signals to ganglion and amacrine cells respectively [8]. Therefore, our study gives a rigorous framework for better understanding how the behavior of coupled non-spiking neurons depends on their intrinsic dynamics and their couplings. In particular, it allows to gain insight into how intrinsic dynamics and couplings of single non-spiking neurons impact neural network behaviors.
On the importance of distinguishing between bimodality and bistability. Here we stress the importance of distinguishing between the terms bistable and bimodal. Bistability results from the intrinsic properties of the neuron, whereas a bimodal distribution of the neuron's voltages does not necessarily. A bistable neuron inherently displays a bimodal distribution of its voltages, but a neuron can exhibit a bimodality of its voltages when it is coupled without being intrinsically bistable. Indeed, we showed in this paper that the RIM near-linear coupled neuron can display a bimodal distribution of its voltages without being transformed into a bistable neuron.
The implications on the network signal processing could be radically different whether the bimodality of the RIM voltages are induced by intrinsic bistability or not. Indeed, a modification of the near-linear type of RIM into a bistable type would endow it with new computational characteristics. In this regard, bistable neurons enhance the short-term memory capabilities of excitatory recurrent networks [60], stabilize the persistent neural activity observed in working memory systems [61], and reduce the effects of noise and distraction stimuli [57,62]. Therefore, if we want to understand the way nervous systems work to produce specific behaviors, we then must understand how synaptic activities influence the dynamics of neurons and their intrinsic nature. We argue that the current paper, through Proposition 4.1, makes this possible in the case of non-spiking networks.
Perspective. In this paper, we determined mathematical conditions to study the influence of synaptic activities on the neuron intrinsic dynamics. We applied them to canonical examples of two coupled neurons, representative of widespread configurations in non-spiking networks. However, an application on larger scale networks could be implemented in the future, for example on C. elegans networks for which we have the fully mapped connectome (i.e. the wiring diagram of its nervous system) [21,37,63]. In particular, a biological network of four pair of neurons displaying correlated up- and down-states has been well-studied in a biological viewpoint [30]. This network underlies a chemotaxis behavior, characterized by C. elegans reversal (reorientation) responses to odors. Modeling this network could be of significant importance to deal with many issues. For instance, a currently open question is the specific contribution of the gap junctions vs. chemical synapses in the emergence of the up- and down-states [30].
We thank the reviewer for their valuable comments on the manuscript, as well as Thibaut Démare, Laetitia Raison-Aubry and Sophie Bavard.
The authors declare no competing interests.
![]() |
a | b | c | d | τ (10−1s) |
RIM | 0.000024 | 0.0036 | 0.31 | 7.22 | 0.042 |
AIY | 0.000044 | 0.0093 | 0.773 | 20.38 | 0.04 |
AFD | 0.00033 | 0.048 | 2.31 | 38.99 | 0.06 |
[1] | B. Andrews, J. McCoy, G. Wheeler, V. M. Wheeler, Closed ideal planar curves, arXiv: 1810.06154. |
[2] | B. Chow, P. Lu, L. Ni, Hamilton's Ricci flow, New York: American Mathematical Society, 2006. |
[3] | M. Gage, On an area-preserving evolution equation for plane curves, In: Nonlinear problems in geometry, Providence: American Mathematical Society, 1986, 51–62. |
[4] |
J. W. Hagood, B. S. Thomson, Recovering a function from a Dini derivative, Am. Math. Mon., 113 (2006), 34–46. doi: 10.1080/00029890.2006.11920276
![]() |
[5] |
L. Jiang, S. Pan, On a non-local curve evolution problem in the plane, Commun. Anal. Geom., 16 (2008), 1–26. doi: 10.4310/CAG.2008.v16.n1.a1
![]() |
[6] |
L. Ma, A. Zhu, On a length preserving curve flow, Monatsh. Math., 165 (2012), 57–78. doi: 10.1007/s00605-011-0302-8
![]() |
[7] | T. Nagasawa, K. Nakamura, Interpolation inequalities between the deviation of curvature and the isoperimetric ratio with applications to geometric flows, Adv. Differential Equ., 24 (2019), 581–608. |
[8] | K. Nakamura, An application of interpolation inequalities between the deviation of curvature and the isoperimetric ratio to the length-preserving flow, Discrete Contin. Dyn. S, doi: 10.3934/dcdss.2020385. |
[9] |
X. L. Wang, L. H. Kong, Area-preserving evolution of nonsimple symmetric plane curves, J. Evol. Equ., 14 (2014), 387–401. doi: 10.1007/s00028-014-0219-5
![]() |
[10] | G. Wheeler, Convergence for global curve diffusion flows, arXiv: 2004.08494. |
1. | Martina Nicoletti, Letizia Chiodo, Alessandro Loppini, Qiang Liu, Viola Folli, Giancarlo Ruocco, Simonetta Filippi, Gennady S. Cymbalyuk, Biophysical modeling of the whole-cell dynamics of C. elegans motor and interneurons families, 2024, 19, 1932-6203, e0298105, 10.1371/journal.pone.0298105 |
Neuron | RIM | AIY | AFD |
Δ(˜I) | 6.18e11 | 6.27e10 | -1.57e07 |
![]() |
a | b | c | d | τ (10−1s) |
RIM | 0.000024 | 0.0036 | 0.31 | 7.22 | 0.042 |
AIY | 0.000044 | 0.0093 | 0.773 | 20.38 | 0.04 |
AFD | 0.00033 | 0.048 | 2.31 | 38.99 | 0.06 |