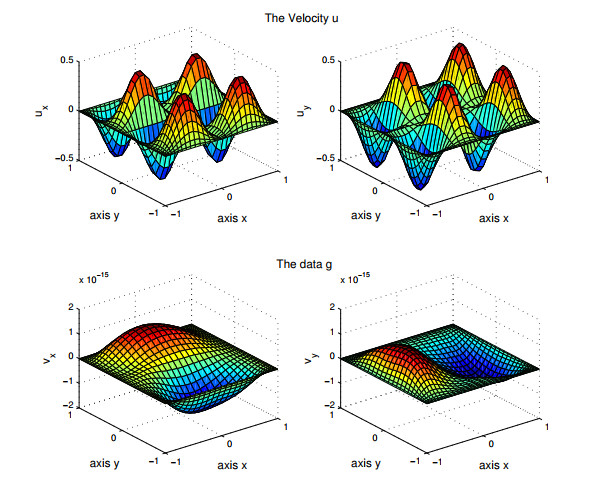
Various topical formulations comprise of methyl salicylate and thymol due to their analgesic and anti-inflammatory properties. The huge demand has led traditional medicines being susceptible to adulteration with synthetic drugs or their analogues to enhance their efficacy and to minimise the cost of obtaining the limited natural substance. The objectives of this study are to analyse a suitable analytical method for simultaneous determination of methyl salicylate and thymol in selected Malaysian traditional medicines using High Performance Liquid Chromatography (HPLC) and to screen the selected Malaysian traditional medicines for possible methyl salicylate and thymol adulteration using the analytical method. Most literature search showed the determination of methyl salicylate and thymol as an individual compound or in combination with other compounds instead of both being detected simultaneously using a single method. Methyl salicylate and thymol were separated at about 3.8 and 6.2 min, respectively at a flow rate of 1 mL/min on C8 column with methanol and water (65: 35) as the mobile phase, column temperature of 35 °C and detector wavelength of 230 nm within 9 minutes of run time. Method validation was conducted and this method was sensitive, linear, specific, precise and accurate. The validated method was applied for screening of methyl salicylate and thymol in 10 samples of liniment and ointment. Half of the samples were detected with methyl salicylate and none with thymol. Majority of the positive samples were unregistered traditional medicines. As quantitation of both compounds is achievable with this method, it will be beneficial in the regulatory and industrial settings. This will ensure the safety and quality of traditional medicines, thereby safeguarding consumer's health. Hence, the method can be adopted for routine quality control (QC) analysis of methyl salicylate and thymol in traditional medicines.
Citation: Muhammad Shahariz Mohamad Adzib, Zul Ilham. Simultaneous analytical determination of methyl salicylate and thymol in selected Malaysian traditional medicines[J]. AIMS Medical Science, 2020, 7(2): 43-56. doi: 10.3934/medsci.2020004
[1] | Huajin Li, Songbiao Zhang, Yue Deng, Huilin Wang . Research on the efficiency of agro-tourism integration in China: Based on the DEA cross-efficiency model. AIMS Mathematics, 2023, 8(10): 23164-23182. doi: 10.3934/math.20231178 |
[2] | Yanting Xu, Tinghui Li . Measurement and spatiotemporal patterns of China's digital economy efficiency. AIMS Mathematics, 2023, 8(12): 29307-29331. doi: 10.3934/math.20231500 |
[3] | Sharifeh Soofizadeh, Reza Fallahnejad . Evaluation of groups using cooperative game with fuzzy data envelopment analysis. AIMS Mathematics, 2023, 8(4): 8661-8679. doi: 10.3934/math.2023435 |
[4] | Qianwei Zhang, Zhihua Yang, Binwei Gui . Two-stage network data envelopment analysis production games. AIMS Mathematics, 2024, 9(2): 4925-4961. doi: 10.3934/math.2024240 |
[5] | Le Fu, Jingxuan Chen, Xuanchen Li, Chunfeng Suo . Novel information measures considering the closest crisp set on fuzzy multi-attribute decision making. AIMS Mathematics, 2025, 10(2): 2974-2997. doi: 10.3934/math.2025138 |
[6] | Victoria Herranz, Diego Napp, Carmen Perea . Weight-2 input sequences of 1/n convolutional codes from linear systems point of view. AIMS Mathematics, 2023, 8(1): 713-732. doi: 10.3934/math.2023034 |
[7] | Yunfeng Shi, Shu Lv, Kaibo Shi . A new parallel data geometry analysis algorithm to select training data for support vector machine. AIMS Mathematics, 2021, 6(12): 13931-13953. doi: 10.3934/math.2021806 |
[8] | Xiuxian Chen, Zhongyang Sun, Dan Zhu . Mean-variance investment and risk control strategies for a dynamic contagion process with diffusion. AIMS Mathematics, 2024, 9(11): 33062-33086. doi: 10.3934/math.20241580 |
[9] | Jamilu Adamu, Kanikar Muangchoo, Abbas Ja'afaru Badakaya, Jewaidu Rilwan . On pursuit-evasion differential game problem in a Hilbert space. AIMS Mathematics, 2020, 5(6): 7467-7479. doi: 10.3934/math.2020478 |
[10] | Muhammad Akram, Syed Muhammad Umer Shah, Mohammed M. Ali Al-Shamiri, S. A. Edalatpanah . Extended DEA method for solving multi-objective transportation problem with Fermatean fuzzy sets. AIMS Mathematics, 2023, 8(1): 924-961. doi: 10.3934/math.2023045 |
Various topical formulations comprise of methyl salicylate and thymol due to their analgesic and anti-inflammatory properties. The huge demand has led traditional medicines being susceptible to adulteration with synthetic drugs or their analogues to enhance their efficacy and to minimise the cost of obtaining the limited natural substance. The objectives of this study are to analyse a suitable analytical method for simultaneous determination of methyl salicylate and thymol in selected Malaysian traditional medicines using High Performance Liquid Chromatography (HPLC) and to screen the selected Malaysian traditional medicines for possible methyl salicylate and thymol adulteration using the analytical method. Most literature search showed the determination of methyl salicylate and thymol as an individual compound or in combination with other compounds instead of both being detected simultaneously using a single method. Methyl salicylate and thymol were separated at about 3.8 and 6.2 min, respectively at a flow rate of 1 mL/min on C8 column with methanol and water (65: 35) as the mobile phase, column temperature of 35 °C and detector wavelength of 230 nm within 9 minutes of run time. Method validation was conducted and this method was sensitive, linear, specific, precise and accurate. The validated method was applied for screening of methyl salicylate and thymol in 10 samples of liniment and ointment. Half of the samples were detected with methyl salicylate and none with thymol. Majority of the positive samples were unregistered traditional medicines. As quantitation of both compounds is achievable with this method, it will be beneficial in the regulatory and industrial settings. This will ensure the safety and quality of traditional medicines, thereby safeguarding consumer's health. Hence, the method can be adopted for routine quality control (QC) analysis of methyl salicylate and thymol in traditional medicines.
Most physical or chemical phenomena are governed by partial differential equations that describe the evolution of the constituents of the problem under study. If all the parameters of the system are known (the geometry of the domain, the boundary and initial conditions, and the coefficients of the equations), the model to be solved is a direct problem. On the other hand, if certain parameters in the equation are unknown, these parameters can be determined from experimental data or from the values at the final time in an evolution problem. The identification of such a parameter in the partial differential equation represents an inverse problem.
When experimental measurements are made on the boundary to determine a coefficient in a partial differential equation, there is always measurement error, which can mean a very large error in identification. For this reason, most inverse problems are ill-posed (we refer the reader to [1], for further details on the results found and the methods developed).
Many papers in this area deal with elliptic problems, see for example [2,3,4,5]. On the other hand, the literature on applications governed by elliptic, parabolic and hyperbolic, linear and nonlinear systems is rather limited. Theoretical results for the latter case can be found, for example, in [6,7,8].
We are interested in studying the state-constrained optimal control problem of the steady-state Navier-Stokes equations. In this area, several applications have been proposed to solve optimal control problems [9,10,11,12]. The elliptic optimization problem was first discussed theoretically by Reyes and R. Griesse [13]. Research on numerical methods for optimal control of the Navier-Stokes equations has made significant advancements over the years. The initial approaches for optimal control of the Navier-Stokes equations employed classical optimization methods such as the conjugate gradient method, quasi-Newton method, or augmented Lagrangian method. These methods yielded promising results, but they were often limited by the nonlinearity of the Navier-Stokes equations and the presence of constraints. Then came the domain decomposition methods, which are used to divide the computational domain into smaller subdomains in order to solve the Navier-Stokes equations more efficiently. In the context of optimal control, these methods help reduce the problem size by partitioning the domain into regions that can be solved independently. This facilitates parallel computations and leads to faster results [14]. The adjoint-based optimization methods, on the other hand, are commonly used to solve optimal control problems. These methods leverage the principle of dynamic programming by calculating an adjoint variable that provides information about the system's sensitivity with respect to the control. This information is then used to adjust the control optimally. Adjoint-based optimization methods have been successfully applied to the Navier-Stokes equations, enabling the solution of complex optimal control problems [15,16]. Also worth mentioning are the methods based on genetic algorithms, which are optimization methods inspired by principles of natural selection. In the context of the Navier-Stokes equations, these methods have been used to solve optimal control problems by generating a population of potential solutions and evolving them over generations. Genetic algorithms have the advantage of being able to explore a larger search space, but they may require a large number of iterations to converge to an optimal solution. It should be noted that the comparison of different approaches will depend on the specific context of the optimal control problem for the Navier-Stokes equations. Each method has its own advantages and limitations, and the choice of method will depend on computational constraints, control objectives and available resources [17]. The approach presented in this paper is a powerful technique for solving this and many other such nonlinear problems. We have applied a new method to construct a new family of numerical schemes that convert the inverse problem into a direct problem, which helps us to solve numerical problems easily. We construct an algorithm that can solve this problem. We use spectral methods to find approximate solutions through the preconditioned GMRES method. The stability and convergence of the method are analyzed [18,19].
The flow of an in-compressible viscous fluid in a domain Ω of R2 is characterized by two variables velocity u and pressure p, given functions f=(fx,fy) in (L2(Ω))2 and a control force g which is the optimization variable.
The problem posed in this paper is to find a solution pair (u,g) solving the functional J defined by.
J(g)=12∫Ω|u(g)−ud|2dx+α2∫Ω|g|2dx, | (Jg) |
where u is the solution to the problem
−νΔu+(u.∇)u+∇p=f+g in Ω,divu=0 in Ω,u=0 on Γ=∂Ω, | (Pg) |
where ud and f are the data and ν is the viscosity. Our work is based on a simple computation of the gradient J which leads to the coupled problem which is the main subject of this study. This paper is organized as follows:
∙ In Section 2, we introduce the optimal control problem under constraints (Pg) [20]. We also prove the existence of a global optimal solution for the optimal control problem (S) [21].
∙ In Section 3, after linearization, we study the existence and uniqueness of weak solutions of (Pn). We prove the convergence of un solutions of (Pn) to the u solution of (Pg). We then derive an optimal system of equations from which the optimal solution can be computed.
∙ In Section 4, We propose a numerical algorithm for solving coupled systems of equations, where the numerical solution is generated by spectral methods [22,23].
Let Ω be a bounded domain of R2 with Lipschitz-continuous boundary Γ. Additionally, V={v∈X,divv=0}, where X=H10(Ω)2={v∈H1(Ω)2;v|∂Ω=0}.
We set M=L20(Ω)={v∈L2(Ω);∫Ωvdx=0}, Y=X×M and W=V×M.
Remark 1. The symbol E↪G denotes the continuous and dense embedding of E into G.
The symbol E⇀G denotes the weak convergence of E to G.
The symbol E→G denotes the strong convergence of E to G.
In this section, we are concerned with the following state-constrained optimal control problem. Find (u∗,g∗)∈W×L2(Ω)2 which solves
minJ(g)=12∫Ω|u(g)−ud|2dx+γ2∫Ω|g|2dx,such that |
νΔu+(u.∇)u+∇p=f+g in Ω,divu=0 in Ω,u=0 on Γ,u∈C, | (S) |
where the state u is sought in the space W=H2(Ω)2∩V.
● C is a closed convex subset of C0(Ω)={ω∈C(ˉΩ);ω|Γ=0}, the space of continuous functions on Ω vanishing on Γ.
● g is a distributed control function.
● The function ud∈L2(Ω)2 denotes the desired state.
● The parameter γ>0 stands for the control cost coefficient.
State constraints are relevant in practical applications to suppress backward flow in channels. Next, we derive necessary optimal conditions for (S).
We have two types of constraint sets C. The first one is
C1={v∈C0;ya(x)≤v(x)≤yb(x),∀x∈˜Ω⊂Ω}, |
which covers point-wise constraints on each component of the velocity vector field, i.e., v(x)≤yb(x) gives vi(x)≤yb,i(x) for i=1,...,d, on a sub-domain ˜Ω⊂Ω.
The set of feasible solutions is defined as:
Tad={(u,g)∈W×L2(Ω)2;u satisfies the state equation in (Pg) andu∈C}. | (2.1) |
The weak formulation of the first and third equations of (Pg) is defined as follows Find u∈V knowing that
∫Ω∇u∇vdx+∫Ω((u.∇)u)vdx=∫Ω(f+g)vdx∀v∈V. | (2.2) |
Before we study the problem of optimal control we start with the following proposition.
Proposition 2. [13] Let Ω be a bounded domain of R2 of class C2 and f and g∈L2(Ω)2. Then every solution of (2.2) satisfies u∈H2(Ω)2 and p∈L20(Ω)∩H1(Ω) for the corresponding pressure. Moreover, there exists a constant c(ν,Ω)>0 such that
∥u∥H2(Ω)2+∥∇p∥L2(Ω)2≤c(1+∥f∥3L2(Ω)2+∥g∥3L2(Ω)2). | (2.3) |
Theorem 3. If Tad is non-empty, then there exists a global optimal solution for the optimal control problem (S).
Proof. Since the problem has at least one feasible pair, and J is bounded by zero, we can take the minimization sequence (uk,gk) in Tad. We obtain
γ2‖gk‖2≤J(uk,gk)<∞, |
which implies that {gk} is uniformly bounded in L2(Ω)2. Then we may extract a weakly convergent sub-sequence, also denoted by {gk}, such that
gk⇀g∗∈L2(Ω)2. | (2.4) |
Using 2.3, it follows that the sequence {uk} is also uniformly bounded in W and, consequently, we may extract a weakly convergent sub-sequence, also denoted by {uk} such that
uk⇀u∗∈W. | (2.5) |
In order to proof that (u∗,g∗) is a solution of the Navier-Stokes equations, the only problem is to pass to the limit in the nonlinear form ∫Ω(uk.∇uk)vdx. Due to the compact embedding W↪V and the continuity of ∫Ω(uk.∇uk)vdx, it follows that
∫Ω(uk.∇uk)vdx→∫Ω(u∗.∇u∗)vdx. | (2.6) |
Consequently, taking into account the linearity and continuity of all terms involved, the limit (u∗,g∗) satisfies the state equations.
Since C is convex and closed, it is weakly closed, so uk⇀u∗ in W and the embedding H2(Ω)∩(H10(Ω))↪C0(Ω) implies that u∗∈C. Taking into consideration that J(g) is weakly lower semi-continuous, the result follows via [13].
To solve (Pg), we construct a sequence of linear problems. Starting from an arbitrary u0∈X, we consider the iterative scheme
−νΔun+(un−1⋅∇)un+∇pn=f+gnin Ω,divun=0in Ω,u=0on Γ,un∈C. | (Pn) |
The variational formulation of (Pn) is
Find (un,pn) ∈ Y such that
a0(un,v)+an(un,v)+b(pn,v)=⟨f+gn,v⟩∀v∈V,b(q,un)=0∀q∈M. | (PVn) |
The bilinear forms a0, an and b are given by ∀p∈M and v∈X
a0(u,v)=ν∫Ω∇u∇vdx,an(u,v)=∫Ω(un−1⋅∇u)vdx,b(p,v)=∫Ω∇pvdx=−∫Ωpdivvdx, | (3.1) |
with f∈H−1(Ω).
Using Green's Theorem and divv=0, we have b(p,v)=0. Then, we associate with the problem (PVn), the following problem
Findun∈Vsuch that,a0(un,v)+an(un,v)=⟨f+gn,v⟩∀v∈V. | (PVn) |
For each n and for f,gn∈L2(Ω)2, the problem (PVn) has a unique solution un∈V [13].
The sequence (un)n∈N satisfies the following inequality:
ν2‖un‖2X≤a(un,un)=L(un)=∫Ω(f+gn)undx. |
Using the continuity of the linear form L(.) and Schwarz's inequality, we obtain the following inequality
‖un‖X≤2ν‖f+gn‖L2(Ω)2∀n≥1, | (3.2) |
which implies that the sequence (un)n∈N is bounded in X=(H10(Ω))2. Hence, there is a subsequence that converges weakly to ϕ in X on the one hand. However, on the other hand, it converges strongly in L2(Ω)2.
Lemma 4. If u0∈H2(Ω)2∩H10(Ω)2, f∈L2(Ω)2, and gn∈L2(Ω)2, then
limn→∞‖∇un−∇u‖L2(Ω)2=0. |
Proof. If u0∈H2(Ω)2∩H10(Ω)2, f∈L2(Ω)2 and gn∈L2(Ω)2, then a regularity theorem gives: un and ϕ are in H2(Ω)2∩H10(Ω)2. Furthermore,
ν2‖∇un−∇ϕ‖L2(Ω)2≤a0(un−ϕ,un−ϕ), | (3.3) |
with
a0(un−ϕ,un−ϕ)=a0(un−ϕ,un)−a0(un−ϕ,ϕ), | (3.4) |
and
a0(un−ϕ,un)−a0(un−ϕ,ϕ)=ν∫Ω∇un∇(un−ϕ)dx−ν∫Ω∇ϕ∇(un−ϕ)dx, | (3.5) |
and
|a0(un−ϕ,un)−a0(un−ϕ,ϕ)|≤ν|∫ΩΔun(un−ϕ)dx|+ν|∫ΩΔϕ(un−ϕ)dx|≤ν‖Δun‖L2(Ω)2‖un−ϕ‖L2(Ω)2+ν‖Δϕ‖L2(Ω)2‖un−ϕ‖L2(Ω)2≤ν‖un−ϕ‖L2(Ω)2(‖Δun‖L2(Ω)2+‖Δϕ‖L2(Ω)2). |
Using (3.3) we obtain
ν2‖∇un−∇ϕ‖2L2(Ω)2≤ν‖un−ϕ‖L2(Ω)2(‖Δun‖L2(Ω)2+‖Δϕ‖L2(Ω)2. |
Then we increase the regularity of u using the method of singular perturbation, we conclude via [11] that un is bounded in H20(Ω), then we extract a sequence still denoted by un, which converges weakly to u in H20(Ω) since the injection of H20(Ω) into H10(Ω) is compact, there is a subsequence still denoted by un which converges strongly to u in H10(Ω), we prove via [11]
limn→∞‖∇un−∇ϕ‖L2(Ω)2=0. | (3.6) |
We need this result.
Lemma 5. 1) limn→∞a0(un,v)=a0(ϕ,v).
2) limn→∞an(un,v)=a∞(ϕ,v)=∫Ω(ϕ⋅∇)ϕ⋅vdx.
Proof. On the one hand, we have,
1)|a0(un,v)−a0(ϕ,v)|≤∫Ω|∇un−∇ϕ|⋅∇vdx≤‖∇un−∇ϕ‖L2(Ω)2‖∇v‖L2(Ω)2. | (3.7) |
Using Lemma 4, we obtain the result.
2) On the other hand, we have
|an(un,v)−a∞(ϕ,v)|=∫Ω{(un−1.∇)un−(ϕ.∇)ϕ}vdx. | (3.8) |
Setting
(un−1.∇)un−(ϕ.∇)ϕ=((un−1−ϕ).∇)un+(ϕ.∇)(un−ϕ). | (3.9) |
By using the continuity of the bi-linear form an(un,v), it gives the following
|an(un,v)−a∞(ϕ,v)|≤C(‖un−1−ϕ‖X‖un‖X+‖ϕ‖X‖un−ϕ‖X)‖v‖X. | (3.10) |
Theorem 6. The sequence (un)n∈N of solutions of problem (Pn) converges to the solution Φ of problem (Pg).
Proof. It follows from Lemma 5 that
limn→∞a0(un,v)+an(un,v)=a0(ϕ,v)+a∞(ϕ,v). |
The problem (PVn) gives
a0(un,v)+an(un,v)=⟨f+gn,v⟩∀v∈V, | (3.11) |
and we have γ2‖gn‖2≤J(vn,gn)<∞, which implies that gn is uniformly bounded in (L2(Ω))2. Hence, we can extract a weakly convergent sub-sequence, denoted by gn, such that gn⇀˘g∈(L2(Ω))2.
Then, using Lemma 5, we obtain
a0(ϕ,v)+a∞(ϕ,v)=⟨f+˘g,v⟩∀v∈V. |
Here we used Rham's Theorem. Let Ω be a bounded regular domain of R2 and L be a continuous linear form on H10(Ω)2, then the linear form L vanishes on V if and only if there exists a unique function φ∈L2(Ω)/R such that
∀v∈H10(Ω)2,L(v)=∫Ωφdivvdx. |
Now let L(v)=a0(ϕ,v)+a∞(ϕ,v)−⟨f+˘g,v⟩, therefore the form L(v)=0 for all v∈V, then Rham' theorem implies the existence of a unique function p∈L2(Ω)/R such that
a0(ϕ,v)+a∞(ϕ,v)−⟨f+˘g,v⟩=∫Ωpdivvdx∀v∈X, | (3.12) |
which gives
ν∫Ω∇ϕ∇vdx+∫Ω(ϕ⋅∇)ϕvdx−∫Ωpdivvdx=∫Ω(f+˘g)vdx∀v∈X, | (3.13) |
∫Ω(−νΔϕ+(ϕ⋅∇)ϕ+∇p−(f+˘g))vdx=0∀v∈X, | (3.14) |
then in D′(Ω):
−νΔϕ+(ϕ.∇)ϕ+∇p−(f+˘g)=0. | (3.15) |
Since ϕ∈V and (ϕ,˘g) satisfies Eqs (1) and (2) of (Pg), we conclude that ϕ is a solution of (Pg) and the result follows.
Consider the problem (Sn), defined as follows
minJ(gn),(un,gn)∈Uad,where (un,gn) solves (Pn), | (Sn) |
where we define the functional
J(gn)=12∫Ω|un(gn)−ud|2dx+c∫Ω|gn|2dx. |
The set of admissible solutions is defined as follows:
Uad={(un,gn)∈W×L2(Ω)2;unsatisfies the state equation in(Pn)andu∈C}. |
The method to calculate the gradient is defined by
limϵ→0J(gn+εw)−J(gn)ε=(J'(gn),w)=∫J'(gn)wdx. | (3.16) |
By linearity, un(gn+εw)=un(gn)+ε~un(w) with
−νΔ˜un(w)+(un−1.∇)~un+∇˜qn(w)=w in Ω,div ~un=0in Ω,~un=0on Γ. | (3.17) |
Otherwise, ˜un(w)=((un (gn))′,w).
As J(gn) is quadratic, we obtain
∫J'(gn)wdx=∫((un(gn)−ud).~un(w)+cgn.w)dx. |
To simplify the expression of the gradient, we use the following system where p is defined as the unique solution in H10(Ω)
−νΔpn−(un−1.∇)pn+∇ηn=un−udin Ω,∇ pn=0in Ω,pn=0on Γ. | (3.18) |
Multiplying the first equation of (3.17) by pn and the first equation of (3.18) by ˜un(w) and integrate by parts, we obtain
ν∫Ω∇pn.∇˜undx+∫Ω((un−1.∇)˜un)pndx+∫Ω˜qn ∇ pn=∫Ωwpndx. |
ν∫Ω∇~un.∇pndx−∫Ω((un−1.∇)pn)˜undx+∫Ωηn ∇.˜un=∫Ω(un−ud).˜undx. |
As
∫Ω((un−1.∇)˜un)pndx=−∫Ω((un−1.∇)pn)˜undx. |
Indeed
∫Ω((un−1.∇)˜un)pndx=∑i∑j∫Ωun−1i∂˜unj∂xipnjdx |
=−∑i∑j∫Ω˜unj∂(un−1ipnj)∂xidx |
=−∑i∑j∫Ω˜unj∂un−1i∂xipnjdx−∑i∑j∫Ω˜un−1j∂pnj∂xiun−1idx |
−∫Ω(˜un.pn)∇2un−1dx−∫Ω((un−1.∇)pn)˜undx |
=−∫Ω((un−1.∇)pn)˜undx |
Hence,
∫Ωwpndx=∫Ω(un−ud).˜undx. |
Consequently
∫J'(gn)wdx=∫Ω(pn+cgn).wdx. |
So J'(g)=pn+cgn=0, implies pn=−cgn and Δpn=−cΔgn, we then obtain the two systems defined as follows
−νΔun+(un−1.∇)un+divqn=f+gnin Ωdivun=0in Ωun=0on Γ, | (3.19) |
cνΔgn+c(un−1.∇)gn+∇ηn=un−udin Ω,div gn=0in Ω,gn=0on Γ. | (3.20) |
We now consider the variational formulation related to both problems (3.19) and (3.20).
Find (un,qn,gn,ηn) in V×M×V×M such as:
∀v∈V,ν∫Ω∇un∇vdx+∫Ω((un−1⋅∇)un)vdx−∫Ωqndivvdx−∫Ωv⋅gndx=⟨f,v⟩Ω,∀ϕ∈M,−∫Ωϕdivundx=0,∀S∈V,cν∫Ω∇gn∇Sdx−c∫Ω((un−1⋅∇)gn)Sdx+∫ΩηndivSdx+∫ΩS⋅undx=⟨ud,S⟩Ω,∀φ∈M,∫Ωφdivgndx=0. | (3.21) |
where ⟨⟩Ω represents the duality product between H−1(Ω) and H10(Ω). The following result is a consequence of the density of D(Ω) in L2(Ω) and H10(Ω).
Proposition 7. The problem S is equivalent to the problem (3.21) in the sense that for all triples (u,p,g) in H10(Ω)2×L20(Ω)×L2(Ω)2 is a solution of the system (S) in the distribution sense if and only if it is a solution of the problem (3.21).
We are now interested in the discretization of problem (PVn) in the case where Ω=]−1,1[2.
In dimension d=2, for any integers n,m≥0, we define Pl,m(Ω) as the the space of polynomials on R.
We denote by Pl,m(Ω) the space of the restrictions of functions on Ω of the set Pl,m of degree ≤l respectively x and ≤m y respectively.
In dimension d=2, denoting by PN(Ω) the space of restrictions to ]−11[2 of polynomials with degree ≤N. The space P0N(Ω) which approximates H10(Ω) is the space of polynomials of PN(Ω) vanishing at ∓1.
Setting ξ0=−1 and ξN=1, we introduce the N−1 nodes ξj, 1≤j≤N−1, and the N+1 weights ρj, 0≤j≤N, of the Gauss-Lobatto quadrature formula. We recall that the following equality holds
∫1−1ϕ(ζ)dζ=N∑j=0ϕ(ξj)ρj. | (3.22) |
We also recall ([24], form. (13.20)) the following property, which is useful in what follows.
∀φN∈PN(−1,1)‖φN‖2L2(]−11[)≤N∑j=0φ2N(ζ)ρj≤3‖φN‖2L2(]−11[). | (3.23) |
Relying on this formula, we introduce the discrete product, defined for continuous functions u and v by
(u,v)N={N∑i=0N∑j=0u(ξi,ξj)v(ξi,ξj)ρiρj,si d = 2. | (3.24) |
It follows from (3.23) that this is a scalar product on PN(Ω).
Finally, let IN denote the Lagrange interpolation operator at the nodes ξi,0≤i≤N, with values in PN(Ω).
To approximate L20(Ω), we consider the space
MN=L20(Ω)∩PN−2(Ω). | (3.25) |
The space that approximates H10(Ω) is
XN=(P0N(Ω))2. | (3.26) |
We now assume that the functions f and gn are continuous on ¯Ω. Thus, the discrete problem is constructed from (PVn) by using the Galerkin method combined with numerical integration and is defined as follows
Find (unN,pnN)∈XN×MN such that
∀vN∈XN,(ν∇unN,∇vN)N+((un−1N.∇)unN,vN)N−(∇vN,pnN)N=(fN+gnN,vN)N∀qN∈MN,−(∇unN,qN)=0 | ((PVn)N) |
where fN=INf.
The existence and uniqueness of the solution (unN,pnN) is proved in [25], see also Brezzi approach and Rappaz Raviart for more details [26]. Thus, the discrete problem deduced from (3.21) is
Find(unN,qnN,gnN,ηnN)∈XN×MN×XN×MNsuch as
∀vN∈XN, (ν∇unN,∇vN)N+((un−1N.∇)unN,vN)N−(∇vN,qN)N−(gnN,vN)N=(fN,vN)N∀ϕN∈MN, −(∇unN,ϕN)N=0∀SN∈XN, cν(∇gnN,∇SN)N−c((un−1N.∇)gnN,SN)N+(divSN,qN)N+(unN,SN)N=(udN,SN)N∀φN∈MN, −(∇gnN,φN)N=0, | (3.27) |
where INud=udN.
Proposition 8. The problem (3.27) has a unique solution (unN,qnN,gnN,ηnN) in XN×MN×XN×MN.
In this part, we will implement some tests illustrating the effectiveness of the proposed algorithm. We choose MATLAB as the programming tool for the numerical simulations.
The matrix system deduced from (3.21) and (3.27) has the following form
(νA+C)un+Bq−Idgn=fBTun=0c(νA−C)gn+Bηn+Idun=udBTgn=0, |
Which can be represented as follows
((νA+C)B−Id0BT000Id0c(νA−C)−B00BT0)(unqngnηn)=(f0ud0). |
Therefore we obtain a linear system with the form EX=F, where E is a non-symmetric invertible matrix. This linear system is solved by a preconditioned GMRES method. To simplify we assume that c=ν=1. In the first and second tests the pair (ud,p) is a solution of problem Pg with g=0. Theoretically, the solution u must be equal to ud and g must be zero in this case. Moreover, this case is among the rare cases where the pair (u,g) can be provided and J(g) must be zero.
First test: Let ud=(0.5sin(πx)2sin(2πy)−0.5sin(2πx)sin(πy)2), an analytic function which vanishes on the edge ∂Ω and p=x+y. In Figure 1, we present the graphs of the solutions u and g for N=32. Note that J(g) reaches 10−12 when N=15 (N is the a number of nodes in the spectral discretization of the problem).
Second test: We now choose ud=(y(1−x2)72(1−y2)52−x(1−x2)52(1−y2)72), a singular function that vanishes on the edge ∂Ω and p=y.cos(πx). In Figure 2, we present the graphs of solutions u and g (for N = 32). In Table 1, we illustrate the variation of J(g) with respect to the value of N.
N | 10 | 14 | 18 | 24 | 32 | 36 | 40 |
J(g) | 1.10−8 | 4.10−10 | 3.10−11 | 2.10−12 | 9.10−14 | 3.10−14 | 10−16 |
In both validation tests, u converges to ud and g converges to 0.
The convergence of J(g) is perfectly shown in the first case because of the choice of the function ud which is an analytic function. However in the second case ud is a singular function. In this case, J(g) reached a good convergence for N=40.
In the third test, the solution (u,g) is unknown. We solve the problem (3.27) with ud=(y(1−x2)2(1−y2)−x(1−x2)(1−y2)2) and f=(fx,fy) where fx=fy=103xy2.
Figure 3 presents the solutions u and g. In Table 2, we give the approximate values of J(g) as a function of the parameter N. This case shows that the algorithm converges. Without knowing the real solution, we note that J(g) converges to a particular number every time N increases.
J(g) | N |
27.305410002457329 | 10 |
27.312223356317649 | 14 |
27.312231333141991 | 18 |
27.312231829834353 | 24 |
27.312231825367355 | 32 |
27.312231824107311 | 36 |
27.312231823788572 | 40 |
To better estimate this convergence, Figure 4 presents the difference between two successive values of J(g) in the function of the average of two associated values of N.
The aim of this paper is to develop a numerical method that solves an optimal control problem by transforming it into a coupled problem. We have tried to simplify the theoretical part as much as possible, and we have even preferred not to add the part related to the discretization of the method since it risks becoming too long. We have considered two examples to illustrate the efficiency of the proposed algorithm. The results given show a good convergence of the algorithm, in particular, a high degree of convergence of J(g) (thanks to the spectral method, which is known for its precision) as a function of the variation of N. We can also use this method for other types of similar nonlinear problems. However, it should be noted that one must always be careful when linearizing the nonlinear term, for example, in our case, if we take the term (un+1.∇un) un instead of (un.∇)un or (un.∇)un+1, the algorithm does not converge.
The authors declare they have not used Artificial Intelligence (AI) tools in the creation of this article.
We would like to thank you for following the instructions above very closely in advance. It will definitely save us lot of time and expedite the process of your paper's publication.
The authors declare no conflict of interest.
[1] | Anderson A, Mcconville A, Fanthorpe L, et al. (2017) Salicylate poisoning potential of topical pain relief agents: from age old remedies to engineered smart patches. Medicines 4: 1-13. |
[2] | Bachute MT, Shanbhag SV (2016) Analytical method development for simultaneous estimation of methyl salicylate, methol, thymol and camphor in an ointment and its validation by Gas Chromatography. Int J Chem Pharm Anal 3: 1-11. |
[3] | Nagoor Meeran MF, Javed H, Taee HA, et al. (2017) Pharmacological properties and molecular mechanisms of thymol: Prospects for its therapeutic potential and pharmaceutical development. Front Pharmacol 8: 1-34. |
[4] | Abubakar BM, Salleh FM, Omar MS, et al. (2018) Assessing product adulteration of Eurycoma longifolia (Tongkat Ali) herbal medicinal product using DNA barcoding and HPLC analysis. Pharm Biol 56: 1-10. |
[5] | Haneef J, Shaharyar M, Husain A, et al. (2013) Analytical methods for the detection of undeclared synthetic drugs in traditional herbal medicines as adulterants. Drug Test Anal 5: 607-613. |
[6] | (2005) International Council for Harmonisation of Technical Requirements for Pharmaceuticals for Human Use, ICH Topic Q2 (R1) Validation of Analytical Procedures: Text and Methodology. International Council for Harmonisation of Technical Requirements for Pharmaceuticals for Human Use Available from: https://database.ich.org/sites/default/files/Q2_R1__Guideline.pdf. |
[7] | Shabir GA (2005) Step-by-Step analytical methods validation and protocol in the quality system compliance industry. J Valid Technol 10: 314-324. |
[8] | Shabir GA, Lough WJ, Arain SA, et al. (2007) Evaluation and application of best practice in analytical method validation. J Liq Chromatogr Relat Technol 30: 311-333. |
[9] | Shabir GA, Bradshaw TK (2011) Development and validation of a Liquid Chromatography method for the determination of methyl salicylate in a medicated cream formulation. Turk J Pharm Sci 8: 117-126. |
[10] | (2013) AOAC International, AOAC Guidelines for Single Laboratory Validation of Chemical Methods for Dietary Supplements and Botanicals. AOAC International Available from: http://members.aoac.org/aoac_prod_imis/AOAC_Docs/StandardsDevelopment/SLV_Guidelines_Dietary_Supplements.pdf. |
[11] | (2017) MyHEALTH, Adulteration in Traditional Medicine Products. Ministry of Health MY Available from: http://www.myhealth.gov.my/en/adulteration-traditional-medicine-products/. |
[12] | Posadzki P, Watson L, Ernst E (2012) Contamination and adulteration of herbal medicinal products (HMPs): An overview of systematic reviews. Eur J Clin Pharmacol 69: 295-307. |
[13] | (2019) National Pharmaceutical Regulatory Agency, Cancellation of Registered Complementary and Alternative Product. National Pharmaceutical Regulatory Agency Available from: https://www.npra.gov.my/index.php/en/consumers/safety-information/cancellation-of-registered-complementary-alternative-product.html. |
[14] | (2016) National Pharmaceutical Regulatory Division, Drug Registration Guidance Document (DRGD). National Pharmaceutical Regulatory Division Available from: https://www.npra.gov.my/index.php/en/drug-registration-guidance-documents-drgd-e-book.html. |
[15] | Zakaria M, Vijayasekaran, Ilham Z, et al. (2014) Anti inflammatory activity of Calophyllum inophyllum fruit extracts. Procedia Chem 13: 218-220. |
[16] | Chang X, Sun P, Ma Y, et al. (2020) A new method for determination of thymol and carvacrol in Thymi herba by Ultraperformance Convergence Chromatography (UPC2). Molecules 25: 1-11. |
![]() |
![]() |
1. | Yuanxin Peng, Zhuo Chen, Jay Lee, Dynamic Convergence of Green Total Factor Productivity in Chinese Cities, 2020, 12, 2071-1050, 4883, 10.3390/su12124883 |
N | 10 | 14 | 18 | 24 | 32 | 36 | 40 |
J(g) | 1.10−8 | 4.10−10 | 3.10−11 | 2.10−12 | 9.10−14 | 3.10−14 | 10−16 |
J(g) | N |
27.305410002457329 | 10 |
27.312223356317649 | 14 |
27.312231333141991 | 18 |
27.312231829834353 | 24 |
27.312231825367355 | 32 |
27.312231824107311 | 36 |
27.312231823788572 | 40 |