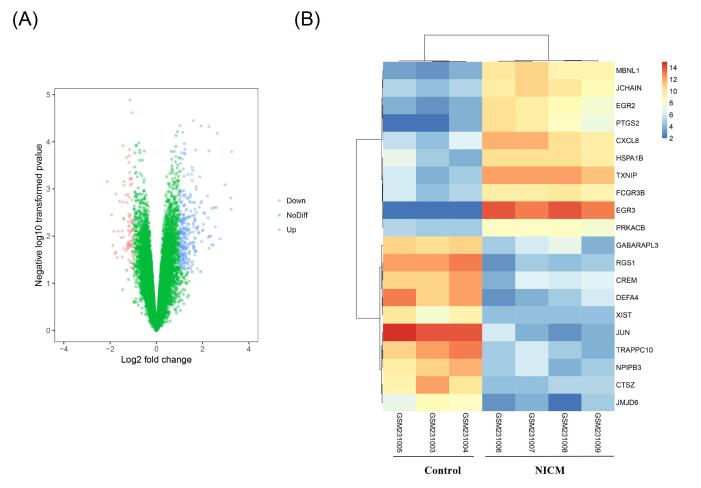
Citation: Ning Li, Jining Zhou. Screening and validating the immune-related gene expression signatures in peripheral blood mononuclear cells of nonischaemic cardiomyopathy[J]. Mathematical Biosciences and Engineering, 2020, 17(3): 2330-2347. doi: 10.3934/mbe.2020124
[1] | Qian Li, Minawaer Hujiaaihemaiti, Jie Wang, Md. Nazim Uddin, Ming-Yuan Li, Alidan Aierken, Yun Wu . Identifying key transcription factors and miRNAs coregulatory networks associated with immune infiltrations and drug interactions in idiopathic pulmonary arterial hypertension. Mathematical Biosciences and Engineering, 2023, 20(2): 4153-4177. doi: 10.3934/mbe.2023194 |
[2] | Sufang Wu, Hua He, Jingjing Huang, Shiyao Jiang, Xiyun Deng, Jun Huang, Yuanbing Chen, Yiqun Jiang . FMR1 is identified as an immune-related novel prognostic biomarker for renal clear cell carcinoma: A bioinformatics analysis of TAZ/YAP. Mathematical Biosciences and Engineering, 2022, 19(9): 9295-9320. doi: 10.3934/mbe.2022432 |
[3] | Wei Niu, Lianping Jiang . A seven-gene prognostic model related to immune checkpoint PD-1 revealing overall survival in patients with lung adenocarcinoma. Mathematical Biosciences and Engineering, 2021, 18(5): 6136-6154. doi: 10.3934/mbe.2021307 |
[4] | Jun Wang, Mingzhi Gong, Zhenggang Xiong, Yangyang Zhao, Deguo Xing . Immune-related prognostic genes signatures in the tumor microenvironment of sarcoma. Mathematical Biosciences and Engineering, 2021, 18(3): 2243-2257. doi: 10.3934/mbe.2021113 |
[5] | Chenyang Jiang, Weidong Jiang . AGTR1, PLTP, and SCG2 associated with immune genes and immune cell infiltration in calcific aortic valve stenosis: analysis from integrated bioinformatics and machine learning. Mathematical Biosciences and Engineering, 2022, 19(4): 3787-3802. doi: 10.3934/mbe.2022174 |
[6] | Kaiyu Shen, Shuaiyi Ke, Binyu Chen, Tiantian Zhang, Hongtai Wang, Jianhui Lv, Wencang Gao . Identification and validation of biomarkers for epithelial-mesenchymal transition-related cells to estimate the prognosis and immune microenvironment in primary gastric cancer by the integrated analysis of single-cell and bulk RNA sequencing data. Mathematical Biosciences and Engineering, 2023, 20(8): 13798-13823. doi: 10.3934/mbe.2023614 |
[7] | Jie Chen, Jinggui Chen, Bo Sun, Jianghong Wu, Chunyan Du . Integrative analysis of immune microenvironment-related CeRNA regulatory axis in gastric cancer. Mathematical Biosciences and Engineering, 2020, 17(4): 3953-3971. doi: 10.3934/mbe.2020219 |
[8] | Aiying Ying, Yueguang Zhao, Xiang Hu . Identification of biomarkers related to prostatic hyperplasia based on bioinformatics and machine learning. Mathematical Biosciences and Engineering, 2023, 20(7): 12024-12038. doi: 10.3934/mbe.2023534 |
[9] | Yong Luo, Xiaopeng Liu, Jingbo Lin, Weide Zhong, Qingbiao Chen . Development and validation of novel inflammatory response-related gene signature to predict prostate cancer recurrence and response to immune checkpoint therapy. Mathematical Biosciences and Engineering, 2022, 19(11): 11345-11366. doi: 10.3934/mbe.2022528 |
[10] | Xuesi Chen, Qijun Zhang, Qin Zhang . Predicting potential biomarkers and immune infiltration characteristics in heart failure. Mathematical Biosciences and Engineering, 2022, 19(9): 8671-8688. doi: 10.3934/mbe.2022402 |
NICM: nonischaemic cardiomyopathy; PBMCs: peripheral blood mononuclear cells; DEGs: differentially expressed genes; GO: gene ontology; KEGG: Kyoto Encyclopedia of Genes and Genomes; PPI: protein-protein interaction; HF: heart failure; IL-6:interleukin-6; IFN-γ: interferon-gamma; GEO: Gene Expression Omnibus; BP: biological process; MF: molecular function; CC: cellular component; NCBI: National Center for Biotechnology Information; CT: cycle threshold; IBD: inflammatory bowel disease
Heart failure (HF), a chronic cardiac syndrome, could give rise to a mean survival of 5 years once it is diagnosed, placing millions of individuals globally at risk of death at present, despite the vital progress that has been made in its diagnosis and treatment [1,2]. Nonischaemic cardiomyopathy (NICM) is one of the most common causes of HF [3]. At present, patients diagnosed with HF due to NICM, especially if conventional therapy shows no effect for them, require further diagnostic and therapeutic procedures involving endomyocardial biopsy. However, endomyocardial biopsy is invasive and thus may easily cause adverse complications [4]. Hence, it is of great necessity to establish a series of non-invasive approaches that could be operated by routine assays in the clinic.
Increasing evidence has demonstrated that dysregulated levels of circulating pro-inflammatory cytokines in peripheral blood mononuclear cells (PBMCs) from patients with NICM exhibit a strong relationship with the disease severity and/or prognosis [5]. Pressure and volume overload trigger profound alterations in cardiac extracellular matrix components, which eventually induce cardiac remodelling by affecting the removal of pro-inflammatory cytokines [6]. Pro-inflammatory monocytes and T-cell infiltration are also observed when myocardial dysfunction and cardiac remodelling occur [7]. These immune cells could mediate hypertension, cardiac hypertrophy and fibrosis by releasing certain cytokines, such as interleukin-6 (IL-6) and interferon-gamma (IFN-γ) [8,9]. PBMCs are a group of immune cells, mainly including T cells, B cells and monocytes/macrophages, the profiling of which has drawn particular attention in a number of diseases due to the convenience and noninvasiveness of PBMCs assessments in recent years [10,11].
Bioinformatic analysis and gene expression profiling analysis could help us screen molecular markers between patients and healthy individuals, thereby providing novel insights into diseases at multiple levels ranging from the alterations of copy number at the genome level to gene expression at the transcriptome level and even epigenetic alterations [12]. In this study, we sought to determine the differentially expressed genes (DEGs) in PBMCs between patients with NICM and healthy controls based on the gene expression profiles from GSE9128, which was downloaded from the Gene Expression Omnibus (GEO, http://www.ncbi.nlm.nih.gov/geo/). Meanwhile, we analysed gene ontology (GO) involving biological process (BP), molecular function (MF), cellular component (CC), and the KEGG pathways of these DEGs. Furthermore, we constructed a protein-protein interaction (PPI) network of these DEGs and identified the top 15 hub genes with a high degree of connectivity. Finally, we detected the mRNA levels of the top 5 upregulated and downregulated DEGs in PBMCs from a mouse model induced by isoproterenol.
Gene expression profiles from the GSE9128 dataset were downloaded from the National Center for Biotechnology Information (NCBI) GEO database, which is free and publicly available [13]. The dataset, based on the GPL96 platform [HG-U133A] Affymetrix Human Genome U133A Array, contains samples of PBMCs from 4 NICM patients and 3 normal individuals. Meanwhile, the Series Matrix File of GSE9128 from the GEO database in PubMed was also downloaded. The peripheral blood samples in this dataset were from Caucasian patients with NICM and healthy controls. Chronic HF was classified according to New York Heart Association (NYHA) functional class Ⅲ/Ⅳ as lasting for more than 6 months; the left ventricular ejection fraction (LVEF) of these patients was less than 36%. All of these NICM patients had no history of angiographically abnormal coronary arteries and myocardial infarction. Gene expression profiling was performed by Affymetrix microarrays.
We screened the DEGs between NICM and healthy samples using GEO2R (http://www.ncbi.nlm.nih.gov/geo/geo2r), an interactive analysis tool for the GEO database based on the R language. We modified the DEGs as differentially expressed with a logFC < 1 (upregulated genes) or logFC > 1 (downregulated genes) according to the criteria described in the references ADDIN EN.CITE [1,2]. Meanwhile, a P value < 0.05 was regarded as significantly different, aiming to reduce the false positive rate. Finally, after downloading the relatively raw TXT data, we employed visual hierarchical cluster analysis to display the volcano plot and heat map of these DEGs in PBMCs between the NICM and healthy samples using ImageGP (http://www.ehbio.com/ImageGP/index.php/Home/Index/index.html).
Gene ontology (GO) analysis is a common framework that can annotate genes and gene products involving functions of cellular components (CC), biological pathways (BP) and molecular function (MF) [14]. The Kyoto Encyclopedia of Genes and Genomes (KEGG) is a collection of databases that help to handle genomes, biological pathways, diseases, chemical substances and drugs [15]. In our study, GO and KEGG analysis was performed by the Database for Annotation, Visualization and Integrated Discovery (DAVID, http://david.ncifcrf.gov) (version 6.7), which is an online biological function database integrating considerable biological data and analysis tools [16]. P < 0.05 was regarded as the cut-off criterion for significant difference. After the key BP, MF, CC and KEGG pathways of these DEGs were determined using DAVID, a scatterplot was generated by ImageGP according to the results of the GO and KEGG pathway analyses.
The Search Tool for the Retrieval of Interacting Genes (STRING) is an online tool to assess and integrate protein-protein interaction (PPI) information, containing physical and functional associations [17]. The PPI network can then identify the core hub genes and key gene modules between patients and healthy individuals. To find the potential correlations of the DEGs, we used STRING to map the PPI network of these DEGs. Subsequently, we used the Cytoscape software platform on the basis of the PPI associations to select 15 hub genes according to their ranked order of connectivity degree. Moreover, the Molecular Complex Detection (MCODE) app was also utilized to screen the typical modules of the PPI network in Cytoscape in line with degree cutoff = 2, k-core = 2, node score cutoff = 0.2 and max depth = 100.
All animal experimental procedures in this study were approved by the Animal Care and Use Committee of Renmin Hospital of Wuhan University and were performed in accordance with the Care and Use of Laboratory Animals published by the US National Institute of Health (Revised 2011) (approval number: WDRX-2017K012). C57/B6 male mice (8-10 weeks old) weighing 25.1 ± 1.6 g were obtained from the Institute of Laboratory Animal Science of the Chinese Academy of Medical Sciences (Beijing, China). The animals were randomly divided into two groups (20 per group) for treatment, the control group and the NICM group. For the mouse model of NICM, isoprenaline (dissolved in sterile saline) was injected (60 mg/kg/day) intraperitoneally once daily for 2 consecutive weeks [18]. Success for HF model creation was assessed by serial echocardiography demonstrating dilation, hypertrophy, and ventricular dysfunction [19].
Blood samples were collected by intracardiac puncture (3–5 mL per animal). As described previously [20], the PBMCs of the mice were isolated from whole blood by density gradient centrifugation with Ficoll-Paque Plus reagent and suspended in QIAzol Lysis Reagent (Qiagen, Dusseldorf, Germany). Subsequently, the layer with the PBMCs was removed and washed twice with saline (0.9%), and the recovered cells were used for total RNA extraction.
Total RNA was isolated using a TRIzol (Invitrogen, Carlsbad, CA, USA) assay, the concentrations and purities of which were quantified using an ultraviolet spectrophotometer. The RNA was then reverse transcribed according to the previous description [21]. The expression levels of the top 5 upregulated genes and the top 5 downregulated genes in PBMCs were normalized to GAPDH. Relative mRNA expression levels were analysed by the 2-∆∆ cycle threshold (CT) method. Apart from detecting the mRNA levels of the top 5 upregulated genes and the top 5 downregulated genes in the control group and NICM group, we also measured the mRNA levels of these genes in the isolated different cell types, the T cells (CD4+ and CD8+), B cells (CD20+) and monocytes (CD14+), from the obtained PBMCs of the mice by positive selection (Anti-PE MicroBeads UltraPure, Miltenyi Biotec, Teterow, Germany).
The obtained data are presented as the mean ± SD (standard deviation) and were assessed by a two-tailed Student's t-test. P < 0 05 was considered statistically significant.
In the present study, a comparison of 3 healthy samples with 4 NICM samples was executed by employing the GEO2R online analysis tool based on a P value < 0.05 and logFC ≤ −1 or logFC ≥ 1 criteria. After analysing the GSE9128 microarray, a total of 371 DEGs were selected, consisting of 288 upregulated DEGs and 83 downregulated DEGs. The expression proportion of these DEGs is displayed in the volcano plot (Figure 1A). The expression levels of the top 10 upregulated and top 10 downregulated DEGs are displayed in a heat map to visualize the results (Figure 1B). Among these 371 DEGs, the top 5 upregulated DEGs were EGR3, MBNL1, PTGS2, EGR2 and TXNIP, while the top 5 downregulated DEGs were GABARAPL3, NPIPB3, RGS1, CREM and JUN. The biological functions and gene titles of these 10 DEGs are presented in Table 1.
DEGs | Gene title | Gene symbol | LogFC | Biological function | ||||
Up-regulated | early growth response 3 | EGR3 | 3.25296217 | Regulating systemic autoimmunity | ||||
muscleblind like splicing regulator 1 | MBNL1 | 3.22538031 | Regulating pre-mRNA alternative splicing | |||||
prostaglandin-endoperoxide synthase 2 | PTGS2 | 3.21406038 | Catalyzings the conversion of arachidonic acid to prostaglandins; Immune response suppression | |||||
early growth response 2 | EGR2 | 2.96759548 | Intrinsic negative regulator of DC immunogenicity; Mmonocyte/macrophage cell fate determination | |||||
thioredoxin interacting protein | TXNIP | 2.76192581 | Linking oxidative stress to inflammasome activation | |||||
Down-regulated | GABA type A receptor associated protein like 3 pseudogene | GABARAPL3 | −1.78817386 | Inhibiting inflammasome | ||||
nuclear pore complex interacting protein family member B3 | NPIPB3 | −1.82835573 | Modulating Type I interferon | |||||
regulator of G-protein signaling 1 | RGS1 | −1.87910047 | Regulating chemokine signalling | |||||
cAMP responsive element modulator | CREM | −1.93814192 | Suppresseing IL-2 and TCRζ chain gene transcription | |||||
Jun proto-oncogene, AP-1 transcription factor subunit | JUN | −2.12183042 | Modulating inflammation |
To gain a more extensive and in-depth knowledge of the selected DEGs, we used DAVID to analyse significantly enriched GO functions. By analysing GO enrichment of all upregulated and downregulated DEGs via DAVID, we discovered that the upregulated DEGs in BP were principally enriched in inflammatory response, immune response, response to lipopolysaccharide, cellular response to mechanical stimulus and negative regulation of translation, while the downregulated DEGs were enriched in circadian regulation of gene expression, negative regulation of sequence-specific DNA binding transcription factor activity, innate immune response, cellular response to hydrogen peroxide and negative regulation of I-kappaB kinase/NF-kappaB signaling. For CC, the upregulated DEGs were mainly enriched in extracellular exosome, nucleus, membrane and nucleoplasm and cytosol, while the downregulated DEGs were enriched in nucleus, nucleoplasm, centrosome and transcription factor complex. MF analysis revealed that the upregulated DEGs were mainly responsible for ATP binding, poly(A) RNA binding, chromatin binding, nucleotide binding and GTP binding. By contrast, the downregulated DEGs may function in protein binding, mitochondrial uncoupling and cytokine activity. The details of the GO analysis are presented in Figure 2 A–C and Table 2.
Expression | Category | Term | Count | PValue |
Up-regulated | GOTERM_BP_DIRECT | GO:0006954~inflammatory response | 9 | 0.008 |
GOTERM_BP_DIRECT | GO:0006955~immune response | 8 | 0.012 | |
GOTERM_BP_DIRECT | GO:0032496~response to lipopolysaccharide | 7 | 0.001 | |
GOTERM_BP_DIRECT | GO:0071260~cellular response to mechanical stimulus | 6 | 2.66E-04 | |
GOTERM_BP_DIRECT | GO:0017148~negative regulation of translation | 5 | 0.001 | |
GOTERM_CC_DIRECT | GO:0070062~extracellular exosome | 51 | 1.41E-04 | |
GOTERM_CC_DIRECT | GO:0005634~nucleus | 49 | 0.011 | |
GOTERM_CC_DIRECT | GO:0016020~membrane | 27 | 5.87E-05 | |
GOTERM_CC_DIRECT | GO:0005654~nucleoplasm | 27 | 0.021 | |
GOTERM_CC_DIRECT | GO:0005829~cytosol | 19 | 0.049 | |
GOTERM_MF_DIRECT | GO:0005524~ATP binding | 25 | 0.039 | |
GOTERM_MF_DIRECT | GO:0044822~poly(A) RNA binding | 23 | 0.006 | |
GOTERM_MF_DIRECT | GO:0003682~chromatin binding | 11 | 0.008 | |
GOTERM_MF_DIRECT | GO:0000166~nucleotide binding | 10 | 0.016 | |
GOTERM_MF_DIRECT | GO:0005525~GTP binding | 10 | 0.044 | |
Down-regulated | GOTERM_BP_DIRECT | GO:0032922~circadian regulation of gene expression | 3 | 0.008 |
GOTERM_BP_DIRECT | GO:0043433~negative regulation of sequence-specific DNA binding transcription factor activity | 3 | 0.011 | |
GOTERM_BP_DIRECT | GO:0045087~innate immune response | 3 | 0.001 | |
GOTERM_BP_DIRECT | GO:0070301~cellular response to hydrogen peroxide | 2 | 0.003 | |
GOTERM_BP_DIRECT | GO:0043124~negative regulation of I-kappaB kinase/NF-kappaB signaling | 2 | ≤0.001 | |
GOTERM_CC_DIRECT | GO:0005634~nucleus | 14 | 0.032 | |
GOTERM_CC_DIRECT | GO:0005654~nucleoplasm | 13 | 7.15E-04 | |
GOTERM_CC_DIRECT | GO:0005813~centrosome | 5 | 0.019 | |
GOTERM_CC_DIRECT | GO:0005667~transcription factor complex | 3 | 0.007 | |
GOTERM_MF_DIRECT | GO:0005515~protein binding | 58 | 0.003 | |
GOTERM_MF_DIRECT | GO:0008083~mitochondrial uncoupling | 5 | 0.031 | |
GOTERM_MF_DIRECT | GO:0005125~cytokine activity | 5 | 0.032 | |
GO: Gene Ontology. |
To obtain more integrated information regarding the crucial pathways of these selected DEGs, KEGG pathways were also analysed via DAVID, the result of which is presented in Table 3 and Figure 2D. The downregulated DEGs were enriched in protein processing in the endoplasmic reticulum, legionellosis, NF-kappa B signaling pathway, NOD-like receptor signaling pathway, estrogen signaling pathway while the upregulated DEGs were enriched in inflammatory bowel disease (IBD), TGF-beta signaling pathway, neurotrophin signaling pathway and Epstein-Barr virus infection.
Category | Term | Count | % | P-Value | Genes |
Down-regulated | ptr04141:Protein processing in endoplasmic reticulum | 11 | 0.030 | 5.49E-04 | SEC23A, HSP90AB1, HSP90B1, HSP90AA1, UBE2J1, MAN1A1, EDEM3, UBE2D1, SAR1B, TRAM1, SSR1 |
ptr05134:Legionellosis | 7 | 0.020 | 5.25E-04 | CXCL2, CXCL8, IL1B, TLR4, HSPD1, CASP1, SAR1B | |
ptr04064:NF-kappa B signaling pathway | 7 | 0.020 | 0.004304358 | PTGS2, BCL2A1, CXCL8, IL1B, TLR4, TAB2, ATM | |
ptr04621:NOD-like receptor signaling pathway | 6 | 0.017 | 0.002195688 | HSP90AB1, HSP90AA1, CXCL8, IL1B, CASP1, TAB2 | |
ptr04915:Estrogen signaling pathway | 6 | 0.017 | 0.023154474 | HSP90AB1, HSP90B1, HSP90AA1, FKBP5, CREB1, PRKACB | |
Up-regulated | cjc05321:Inflammatory bowel disease (IBD) | 3 | 0.030 | 0.000636646 | JUN, SMAD3, RORA |
cjc04350:TGF-beta signaling pathway | 3 | 0.030 | 0.03910693 | SMAD7, ID1, SMAD3 | |
cjc04722:Neurotrophin signaling pathway | 3 | 0.030 | 0.000546883 | NFKBIE, JUN, MAPKAPK2 | |
cjc05169:Epstein-Barr virus infection | 3 | 0.030 | 0.000983148 | NFKBIE, JUN, TNFAIP3 | |
KEGG: Kyoto Encyclopedia of Genes and Genomes; FDR: False Discovery Rate. |
Furthermore, 15 hub genes identified from the Cytoscape software, which occupy the core position in the PPI network, were found in accordance with a high degree of connectivity (Table 4 & Figure 3A). We built the PPI network from the top 15 hub genes via the information from the STRING protein query of public databases. The top 15 hub genes, possessing a high degree of connectivity in NICM, are as follows: JUN, MYC, HSP90AA1, PCNA, CREB1, IL1B, IL8, SMARCA2, TLR4, RB1, RANBP2, EGR1, PTGS2, ENO1 and XPO1. Among the top 15 hub genes, only one gene (JUN) was significantly downregulated in the PBMCs from the patients with NICM, while the others were significantly increased in the PBMCs. Intriguingly, JUN is not only the DEG with the highest connectivity but is also the downregulated DEG with the highest fold change. Additionally, the top 15 hub genes could interact with 149 DEGs directly. These hub genes could also interact with each other and exhibited a strong correlation. For example, JUN could directly interact with IL8, RB1, EGR1, PTGS2, IL1B, SMARCA2, CREB1, HSP90AA1, TLR4 and MYC, while CREB1 interacts with IL1B, PTGS2, JUN, SMARCA2, RB1, MYC, XPO1, TLR4 and HSP90AA1. Moreover, we used the MCODE plug-in to detect the highest modules in the PPI network. We identified the top 2 modules of the network; Module 1 (Figure 3B) was associated with immune response and protein processing in the endoplasmic reticulum, while Module 2 (Figure 3C) was associated with the signaling pathway and cellular response to inflammation. Collectively, the PPI network suggested that the top 15 hub genes, especially JUN and CREB, may play essential roles in the immune response of NICM.
Gene | Degree of connectivity | P value | Log FC |
JUN | 47 | ≤0.001 | -2.121 |
MYC | 44 | ≤0.001 | 1.932 |
HSP90AA1 | 40 | 0.040 | 1.155 |
PCNA | 35 | 0.004 | 1.649 |
CREB1 | 33 | 0.006 | 1.617 |
IL1B | 33 | 0.009 | 1.351 |
IL8 | 33 | ≤0.001 | 1.241 |
SMARCA2 | 30 | 0.002 | 1.131 |
TLR4 | 30 | 0.008 | 1.821 |
RB1 | 28 | 0.003 | 1.176 |
RANBP2 | 28 | 0.002 | 1.855 |
EGR1 | 24 | 0.003 | 1.721 |
PTGS2 | 24 | 0.001 | 3.214 |
ENO1 | 23 | 0.013 | 1.953 |
XPO1 | 22 | 0.015 | 1.067 |
Top 15 hub genes with higher degree of connectivity |
To ensure the credibility of the GSE9128 microarray and obtain further credible analysis, we re-identified the top 5 upregulated genes and the top 5 downregulated genes via qPCR in a mouse model induced by isoprenaline. The results from PCR (Figure 4) demonstrated that the mRNA expression levels of EGR3, MBNL1, PTGS2, EGR2 and TXNIP in PBMCs from mice with NICM were significantly higher than those in control mice (P < 0.05), while the mRNA expression levels of GABARAPL3, NPIPB3, RGS1, CREM and JUN in the NICM group were statistically lower than those in the control group (P < 0.05).
Furthermore, we detected the mRNA levels of the top 5 upregulated and top 5 downregulated genes in the subtypes of the PBMCs. To our knowledge, the main subtypes of PBMCs consists of T cells, B cells and monocytes. Therefore, we principally explored the mRNA levels of these 10 genes in CD4+ T cells, CD8+ T cells, CD14+ monocytes and CD20+ B cells (Figure 5). EGR3 was mainly expressed in T cells in mice with NICM. MBNL, PTGS2 and EGR2 were consistently higher in monocytes; however, their expression was significantly higher in mice with NICM. TXNIP was mainly expressed in CD4+ T cells, CD8+ T cells and CD20+ B cells, the expression of which was significantly increased in the presence of NICM. Regarding the downregulated genes, we found that JUN was highly expressed in T cells in normal subjects, while its level was reduced in T cells but increased in B cells in mice with NICM. CREM was principally expressed in T cells, while its level was decreased in T cells in mice with NICM. RGS1 was primarily expressed in B cells in normal mice. The level of NPIPB3 was higher in T cells and monocytes of normal mice compared with the mice with NICM. The mRNA levels of GABARAPL3 in the four types of immune cells were consistently higher in normal mice than in mice with NICM.
Patients with left ventricular dysfunction resulting from NICM possess an annual rate of death from any cause of approximately 7 percent when they received standard medical therapy for HF [22]. The aetiology of NICM is complicated and multifactorial, and at present, it is recognized that NICM is usually an integrated pathologic change implicated with genetic factors, mechanical stress, metabolic disorders, and infectious (mainly viral) causes, as well as toxicity-related causes [23]. The potential pathophysiological mechanisms underlying these alterations in NICM remain incompletely explored, and it is believed that the interplay among altered sarcomeric and cytoskeletal proteins, autoimmune mechanisms, postinfection immune mechanisms, direct pathogen damage, and free oxygen radical species are associated with the development and progression of NICM [24]. In particular, immune mechanisms in NICM have received increasing attention and have been unveiled in recent years. Some therapeutic strategies have also been proposed to indirectly modulate the inflammatory and immune status of patients with NICM. However, to date, our knowledge of immune mediators and mechanisms in NICM is still too superficial. Hence, some key immune-related diagnostic biomarkers and therapeutic targets in PBMCs should be verified as early as possible.
In this study, we first performed a comprehensive investigation on the expression profile of PBMCs obtained from patients with NICM and healthy individuals. Our study included 3 normal individuals and 4 patients with NICM from the GSE9128 GEO database. In our analysis, a total of 371 DEGs (accounting for 2.1 % of all detected genes) were found: 288 upregulated genes and 83 downregulated genes. Among the 371 DEGs, we identified the top 5 upregulated (EGR3, MBNL1, PTGS2, EGR2 and TXNIP) and top 5 downregulated (GABARAPL3, NPIPB3, RGS1, CREM and JUN) genes between healthy individuals and patients with NICM. Furthermore, we detected their mRNA levels in PBMCs in a mouse model induced by isoprenaline, and the results based on our experiments validated the bioinformatics analysis. Thus, we hypothesize that these 10 DEGs are expected to be promising biomarkers for the diagnosis of NICM. Meanwhile, based on a PPI network, we selected 15 hub genes with high connectivity, which all occupied the core node of the network, indicating that these hub genes may exert pivotal influence in the immune response of NICM. Furthermore, by annotating and analysing all DEGs in the PBMCs, we found that immune response, inflammatory response, extracellular exosome and cell differentiation were enriched in the progression of NICM. In fact, most of the DEGs, especially the top 5 upregulated/downregulated genes and the top 15 hub genes, perform vital immunoregulatory functions.
EGR3 is a transcription factor of the Cys2His2-type zinc finger transcription factor family that can inhibit IL-2 and interferon (IFN)-γ secretion in T cells and upregulate the level of the E3 ligase Cbl-b, which is important for the modulation of T cell energy and tolerance [25]. In a NICM model induced by transverse aortic constriction (TAC), IL-2 was significantly downregulated, and pretreatment with IL-2 was effective in the selective induction of regulatory T cells (Tregs) and in alleviating the development of cardiac remodelling through decreasing cardiac systemic inflammation [26]. On the other hand, the frequency of T helper (Th) 17 cells, which are derived from naive Th cells, was significantly elevated in patients with HF [27]. Meanwhile, EGR3 could trigger a Th17 response by enhancing the development of γδ T cells, suggesting that EGR3 may mediate the activation of Th17 cells and induce HF [28]. In our study, EGR3 was significantly increased in T cells of patients and mice with NICM. Thus, we speculate that EGR3 may suppress the secretion of IL2 by inhibiting Tregs and promoting Th17 cells, thereby deteriorating cardiac injury and dysfunction.
Excessive inflammation and monocyte/macrophage activation are featured in cardiac remodelling associated with NICM [29]. However, the roles of monocytes/macrophages in cardiac remodelling and HF remain controversial. For example, inhibition of macrophages in hypertensive rats resulted in an acceleration of cardiomyopathy, which was evidenced by left ventricle systolic dysfunction [30]. Other studies have provided primary evidence that the localization of macrophages may contribute to inflammation, fibrosis and cardiac injury induced by TAC [31,32]. RGS1 was reported to inactivate G-protein signalling and suppress the response to continuous chemokine stimulation. A recent study reported that in non-activated monocytes, RGS1 was low, the expression of which was immediately upregulated upon the activation of monocytes by pro-inflammatory signals during the recruitment phase. Subsequently, monocytes began to differentiate into macrophages, resulting in the increased expression of RGS1. The increased RGS1 further terminated chemokine signalling, eventually reducing the capacity for inflammatory cell migration and increasing the accumulation of macrophages [33]. In our study, we found that RGS1 was highly expressed in monocytes in the control group and that the mRNA level of RGS1 was significantly decreased in patients and mice with NICM, which hinted to us that RGS1 may exert a protective role in NICM by blocking the differentiation of monocytes/macrophages. In fact, another top 5 upregulated gene in our study, EGR2 was also uncovered to regulate the differentiation of monocytes into macrophages by modulating the expression of colony-stimulating factor-1 (CSF-1). In the inflammatory response, the hub gene (TLR4) has been reported to be significantly activated in monocytes and macrophages in atherosclerosis [34]. Collectively, our study suggested that the DEGs we screened participated in the differentiation of monocytes into macrophages in the development of NICM from the perspective of bioinformatics analysis.
Another finding in our study was that the inflammatory response played an essential role in the development of NICM [35]. Although a vast number of studies have proven the biological status of inflammation in NICM, few studies have provided evidence using bioinformatics analysis. The GO and KEGG pathway analysis in our study revealed that many inflammatory pathways in patients with NICM were activated, including the NF-kappa B signaling pathway, mitochondrial uncoupling, Toll-like receptor signaling, NOD-like receptor signaling pathway and TGF-beta signaling pathway. Additionally, many of the screened DEGs and hub genes were also associated with inflammation, including TXNIP, GABARAPL3, CREM, JUN, PCNA, TLR4, IL8, IL1B and HSP90AA1. To our knowledge, activation of the immune system is not independent of inflammation in the progression of HF. In chronic HF, activating the immune system can usually contribute to the activation of the complement system, secretion of pro-inflammatory cytokines, and production and release of autoantibodies, indicating that immune-inflammatory activation in patients with NICM may occur synchronously but not in isolation [36].
Taken together, using a series of bioinformatics analyses, we performed an integrated and novel illustration of mRNA expression profiles to identify DEGs expressed in PBMCs, which may play critical roles in the occurrence and development in patients with NICM. Genes and pathways implicated in inflammation and the immune response were significantly altered in the PBMCs of NICM. These findings will greatly contribute to unveiling the molecular mechanisms of NICM and promoting a non-invasive diagnosis. To allow these biomarkers and targets to be used more routinely in the clinic, further investigations into these genes in PBMCs should be performed.
All procedures performed in study involving human participants were in accordance with the ethical standards of the institutional and/or national research committee and with the 1964 Helsinki declaration and its later amendments or comparable ethical standards. All applicable international, national, and/or institutional guidelines for the care and use of animals were followed.
This work was supported by grants from the National Natural Science Foundation of China (No. 81470516, 81530012, 81300070, and 81770399), the Key Project of the National Natural Science Foundation (No.81530012) and Fundamental Research Funds for the Central Universities of China (2042018kf0121).
The data used to support the findings of this study are available from the corresponding author upon request.
ZJ conceived the experiments. LN conducted the experiments. LN analyzed the data. ZJ wrote the paper.
The authors declare that they have no competing interests.
[1] | M. Jessup, T. H. Marwick, P. Ponikowski, A. A. Voors, C. W. Yancy, 2016 ESC and ACC/AHA/HFSA heart failure guideline update—what is new and why is it important?, Nat. Rev. Cardiol., 13 (2016), 623-628. |
[2] | S. V. Konstantinides, G. Meyer, C. Becattini, H. Bueno, G. J. Geersing, V. P. Harjola, et al., 2019 ESC Guidelines for the diagnosis and management of acute pulmonary embolism developed in collaboration with the European Respiratory Society (ERS), Eur. Heart J., 27 (2019), 2129-2200. |
[3] | F. Ali-Ahmed, F. Dalgaard, S. M. Al-Khatib, Sudden cardiac death in patients with myocarditis: Evaluation, risk stratification, and management, Am. Heart J., 220 (2019), 29-40. |
[4] | C. Wojciechowska, J. Wodniecki, R. Wojnicz, E. Romuk, W. Jachec, A. Tomasik, et al., Neopterin and beta-2 microglobulin relations to immunity and inflammatory status in nonischemic dilated cardiomyopathy patients, Mediators Inflamm., 2014 (2014), 585067. |
[5] | Y. Zhang, J. Bauersachs, H. F. Langer, Immune mechanisms in heart failure,. Eur. J. Heart Fail, 19 (2017), 1379-1389. |
[6] | W. Chen, N. G. Frangogiannis, Fibroblasts in post-infarction inflammation and cardiac repair, Biochim. Biophys Acta, 1822 (2013), 945-953. |
[7] | T. Shioi, A. Matsumori, Y. Kihara, M. Inoko, K. Ono, Y. Iwanaga, et al., Increased expression of interleukin-1 beta and monocyte chemotactic and activating factor/monocyte chemoattractant protein-1 in the hypertrophied and failing heart with pressure overload, Circ. Res., 18 (1997), 664-671. |
[8] | B. Coles, C. A. Fielding, S. Rose-John, J. Scheller, S. A. Jones, V. B. O'Donnell, Classic interleukin-6 receptor signaling and interleukin-6 trans-signaling differentially control angiotensin Ⅱ-dependent hypertension, cardiac signal transducer and activator of transcription-3 activation, and vascular hypertrophy in vivo, Am. J. Pathol., 171 (2007), 315-325. |
[9] |
M. Afanasyeva, D. Georgakopoulos, D. F. Belardi, D. Bedja, D. Fairweather, Y. Wang, et al., Impaired up-regulation of CD25 on CD4+ T cells in IFN-gamma knockout mice is associated with progression of myocarditis to heart failure, Proc. Natl. Acad. Sci. U S A, 102 (2005), 180-185. doi: 10.1073/pnas.0408241102
![]() |
[10] | S. D'Amore, M. Vacca, M. Cariello, G. Graziano, A. D'Orazio, R. Salvia, et al., Genes and miRNA expression signatures in peripheral blood mononuclear cells in healthy subjects and patients with metabolic syndrome after acute intake of extra virgin olive oil, Biochim. Biophys Acta, 1861, (2016), 1671-1680. |
[11] | G. Serino, F. Sallustio, C. Curci, S. N. Cox, F. Pesce, G. De Palma, et al., Role of let-7b in the regulation of N-acetylgalactosaminyltransferase 2 in IgA nephropathy, Nephrol. Dial. Transplant, 30 (2015), 1132-1139. |
[12] | N. Li, L. Li, Y. S. Chen, The identification of core gene expression signature in Hepatocellular Carcinoma, Oxid. Med. Cell Longev., 2018 (2018), 3478305. |
[13] | L. Zhang, Y. Yang, L. Cheng, Y. Cheng, H. H. Zhou, Z. R. Tan, Identification of common genes refers to colorectal carcinogenesis with paired cancer and noncancer samples, Dis. Markers, 2018 (2018), 3452739. |
[14] | P. Gaudet, N. Skunca, J. C. Hu, C. Dessimoz, Primer on the gene ontology, Methods Mol. Biol., 1446 (2017), 25-37. |
[15] | M Kanehisa, The KEGG database, Novartis Found Symp., 247 (2002), 91-101; discussion 101-103, 119-128, 244-152. |
[16] | D. W. Huang, B. T. Sherman, Q. Tan, J. R. Collins, W. G. Alvord, J. Roayaei, et al., The DAVID gene functional classification tool: A novel biological module-centric algorithm to functionally analyze large gene lists, Genome Biol., 8 (2007), R183. |
[17] |
D. Szklarczyk, A. Franceschini, S. Wyder, K. Forslund, D. Heller, J. Huerta-Cepas, et al., STRING v10: Protein-protein interaction networks, integrated over the tree of life, Nucleic Acids Res., 43 (2015), D447-452. doi: 10.1093/nar/gku1003
![]() |
[18] | J. Zhang, M. Huang, S. Shen, X. Wu, X. Wu, P. Lv, et al., Qiliqiangxin attenuates isoproterenol-induced cardiac remodeling in mice, Am. J. Transl. Res., 9 (2017), 5585-5593. |
[19] | S. C. Chang, S. Ren, C. D. Rau, J. J. Wan, Isoproterenol-induced heart failure mouse model using osmotic pump implantation, Methods Mol. Biol.,1816 (2018), 207-220. |
[20] | S. Desjardins, E. Belkai, D. Crete, L. Cordonnier, J. M. Scherrmann, F. Noble, et al., Effects of chronic morphine and morphine withdrawal on gene expression in rat peripheral blood mononuclear cells, Neuropharmacology, 55 (2008), 1347-1354. |
[21] | N. Hamdani, C. Franssen, A. Lourenco, I. Falcao-Pires, D. Fontoura, S. Leite, et al., Myocardial titin hypophosphorylation importantly contributes to heart failure with preserved ejection fraction in a rat metabolic risk model, Circ. Heart Fail, 6 (2013),1239-1249. |
[22] | A. Kadish, A. Dyer, J. P. Daubert, R. Quigg, N. A. Estes, K. P. Anderson, et al., Prophylactic defibrillator implantation in patients with nonischemic dilated cardiomyopathy, N Engl. J. Med., 350 (2004), 2151-2158. |
[23] | P. Richardson, W. McKenna, M. Bristow, B. Maisch, B. Mautner, J. O'Connell, et al., Report of the 1995 World Health Organization/International Society and Federation of Cardiology Task Force on the Definition and Classification of cardiomyopathies, Circulation, 93 (1996), 841-842. |
[24] | G. Poglajen, G. Zemljic, S. Frljak, A. Cerar, V. Androcec, M. Sever, et al., Stem cell therapy in patients with chronic nonischemic heart failure, Stem Cells Int., 2018, (2018), 6487812. |
[25] | S. Sumitomo, K. Fujio, T. Okamura, K. Yamamoto, Egr2 and Egr3 are the unique regulators for systemic autoimmunity, Jakstat, 2 (2013), e23952. |
[26] | H. Wang, L. Hou, D. Kwak, J. Fassett, X. Xu, A. Chen, et al., Increasing regulatory T cells with Interleukin-2 and Interleukin-2 antibody complexes attenuates lung inflammation and heart failure progression, Hypertension, 68 (2016), 114-122. |
[27] | N. Li, H. Bian, J. Zhang, X. Li, X. Ji, Y Zhang, The Th17/Treg imbalance exists in patients with heart failure with normal ejection fraction and heart failure with reduced ejection fraction, Clin. Chim. Acta, 411 (2010), 1963-1968. |
[28] | R. M. Parkinson, S. L. Collins, M. R. Horton, J. D. Powell, Egr3 induces a Th17 response by promoting the development of gammadelta T cells, PLoS One, 9 (2014), e87265. |
[29] | N. Glezeva, S. Horgan, J. A. Baugh, Monocyte and macrophage subsets along the continuum to heart failure: Misguided heroes or targetable villains?, J. Mol. Cell Cardiol., 89 (2015), 136-145. |
[30] | H. R. Zandbergen, U. C. Sharma, S. Gupta, J. W. Verjans, S. van den Borne, S. Pokharel, et al., Macrophage depletion in hypertensive rats accelerates development of cardiomyopathy, J. Cardiovasc. Pharmacol. Ther., 14 (2009), 68-75. |
[31] | R. K. Braun, J. M. Koch, T. A. Hacker, D. Pegelow, J. Kim, A. N. Raval, et al., Cardiopulmonary and histological characterization of an acute rat lung injury model demonstrating safety of mesenchymal stromal cell infusion, Cytotherapy, 18 (2016), 536-545. |
[32] | H. Kai, F. Kuwahara, K. Tokuda, T. Imaizumi, Diastolic dysfunction in hypertensive hearts: Roles of perivascular inflammation and reactive myocardial fibrosis, Hypertens Res., 28 (2005), 483-490. |
[33] | J. Patel, E. McNeill, G. Douglas, A. B. Hale, J. de Bono, R. Lee, et al., RGS1 regulates myeloid cell accumulation in atherosclerosis and aortic aneurysm rupture through altered chemokine signalling, Nat. Commun., 6 (2015), 6614. |
[34] | L. Chavez-Sanchez, A. Madrid-Miller, K. Chavez-Rueda, M. V. Legorreta-Haquet, E. Tesoro-Cruz, F. Blanco-Favela, Activation of TLR2 and TLR4 by minimally modified low-density lipoprotein in human macrophages and monocytes triggers the inflammatory response, Hum. Immunol., 71 (2010), 737-744. |
[35] | L. Gullestad, P. Aukrust, Review of trials in chronic heart failure showing broad-spectrum anti-inflammatory approaches, Am. J. Cardiol., 95 (2005), 17C-23C; discussion 38C-40C. |
[36] | P. Alvarez, A. Briasoulis, Immune modulation in heart failure: The promise of novel biologics. |
1. | Ke-Ying Fang, Wen-Chao Cao, Tian-Ao Xie, Jie Lv, Jia-Xin Chen, Xun-Jie Cao, Zhong-Wei Li, Shu-Ting Deng, Xu-Guang Guo, Exploration and validation of related hub gene expression during SARS-CoV-2 infection of human bronchial organoids, 2021, 15, 1479-7364, 10.1186/s40246-021-00316-5 | |
2. | Min Lei, Dongdong Zhang, Yixin Sun, Cong Zou, Yue Wang, Yongjun Hong, Yanchao Jiao, Chengfu Cai, Web‐based transcriptome analysis determines a sixteen‐gene signature and associated drugs on hearing loss patients: A bioinformatics approach, 2021, 35, 0887-8013, 10.1002/jcla.24065 | |
3. | Hua Yao, Yue Zhou, Tingting Li, Yao Li, Fan Li, Geng Zhang, Xin Fu, Yan Kang, Qin Wu, Bioinformatic identification and experiment validation revealed that ACTG1 is a promising prognostic signature and therapeutic target for sepsis, 2023, 114, 1938-3673, 325, 10.1093/jleuko/qiad072 |
DEGs | Gene title | Gene symbol | LogFC | Biological function | ||||
Up-regulated | early growth response 3 | EGR3 | 3.25296217 | Regulating systemic autoimmunity | ||||
muscleblind like splicing regulator 1 | MBNL1 | 3.22538031 | Regulating pre-mRNA alternative splicing | |||||
prostaglandin-endoperoxide synthase 2 | PTGS2 | 3.21406038 | Catalyzings the conversion of arachidonic acid to prostaglandins; Immune response suppression | |||||
early growth response 2 | EGR2 | 2.96759548 | Intrinsic negative regulator of DC immunogenicity; Mmonocyte/macrophage cell fate determination | |||||
thioredoxin interacting protein | TXNIP | 2.76192581 | Linking oxidative stress to inflammasome activation | |||||
Down-regulated | GABA type A receptor associated protein like 3 pseudogene | GABARAPL3 | −1.78817386 | Inhibiting inflammasome | ||||
nuclear pore complex interacting protein family member B3 | NPIPB3 | −1.82835573 | Modulating Type I interferon | |||||
regulator of G-protein signaling 1 | RGS1 | −1.87910047 | Regulating chemokine signalling | |||||
cAMP responsive element modulator | CREM | −1.93814192 | Suppresseing IL-2 and TCRζ chain gene transcription | |||||
Jun proto-oncogene, AP-1 transcription factor subunit | JUN | −2.12183042 | Modulating inflammation |
Expression | Category | Term | Count | PValue |
Up-regulated | GOTERM_BP_DIRECT | GO:0006954~inflammatory response | 9 | 0.008 |
GOTERM_BP_DIRECT | GO:0006955~immune response | 8 | 0.012 | |
GOTERM_BP_DIRECT | GO:0032496~response to lipopolysaccharide | 7 | 0.001 | |
GOTERM_BP_DIRECT | GO:0071260~cellular response to mechanical stimulus | 6 | 2.66E-04 | |
GOTERM_BP_DIRECT | GO:0017148~negative regulation of translation | 5 | 0.001 | |
GOTERM_CC_DIRECT | GO:0070062~extracellular exosome | 51 | 1.41E-04 | |
GOTERM_CC_DIRECT | GO:0005634~nucleus | 49 | 0.011 | |
GOTERM_CC_DIRECT | GO:0016020~membrane | 27 | 5.87E-05 | |
GOTERM_CC_DIRECT | GO:0005654~nucleoplasm | 27 | 0.021 | |
GOTERM_CC_DIRECT | GO:0005829~cytosol | 19 | 0.049 | |
GOTERM_MF_DIRECT | GO:0005524~ATP binding | 25 | 0.039 | |
GOTERM_MF_DIRECT | GO:0044822~poly(A) RNA binding | 23 | 0.006 | |
GOTERM_MF_DIRECT | GO:0003682~chromatin binding | 11 | 0.008 | |
GOTERM_MF_DIRECT | GO:0000166~nucleotide binding | 10 | 0.016 | |
GOTERM_MF_DIRECT | GO:0005525~GTP binding | 10 | 0.044 | |
Down-regulated | GOTERM_BP_DIRECT | GO:0032922~circadian regulation of gene expression | 3 | 0.008 |
GOTERM_BP_DIRECT | GO:0043433~negative regulation of sequence-specific DNA binding transcription factor activity | 3 | 0.011 | |
GOTERM_BP_DIRECT | GO:0045087~innate immune response | 3 | 0.001 | |
GOTERM_BP_DIRECT | GO:0070301~cellular response to hydrogen peroxide | 2 | 0.003 | |
GOTERM_BP_DIRECT | GO:0043124~negative regulation of I-kappaB kinase/NF-kappaB signaling | 2 | ≤0.001 | |
GOTERM_CC_DIRECT | GO:0005634~nucleus | 14 | 0.032 | |
GOTERM_CC_DIRECT | GO:0005654~nucleoplasm | 13 | 7.15E-04 | |
GOTERM_CC_DIRECT | GO:0005813~centrosome | 5 | 0.019 | |
GOTERM_CC_DIRECT | GO:0005667~transcription factor complex | 3 | 0.007 | |
GOTERM_MF_DIRECT | GO:0005515~protein binding | 58 | 0.003 | |
GOTERM_MF_DIRECT | GO:0008083~mitochondrial uncoupling | 5 | 0.031 | |
GOTERM_MF_DIRECT | GO:0005125~cytokine activity | 5 | 0.032 | |
GO: Gene Ontology. |
Category | Term | Count | % | P-Value | Genes |
Down-regulated | ptr04141:Protein processing in endoplasmic reticulum | 11 | 0.030 | 5.49E-04 | SEC23A, HSP90AB1, HSP90B1, HSP90AA1, UBE2J1, MAN1A1, EDEM3, UBE2D1, SAR1B, TRAM1, SSR1 |
ptr05134:Legionellosis | 7 | 0.020 | 5.25E-04 | CXCL2, CXCL8, IL1B, TLR4, HSPD1, CASP1, SAR1B | |
ptr04064:NF-kappa B signaling pathway | 7 | 0.020 | 0.004304358 | PTGS2, BCL2A1, CXCL8, IL1B, TLR4, TAB2, ATM | |
ptr04621:NOD-like receptor signaling pathway | 6 | 0.017 | 0.002195688 | HSP90AB1, HSP90AA1, CXCL8, IL1B, CASP1, TAB2 | |
ptr04915:Estrogen signaling pathway | 6 | 0.017 | 0.023154474 | HSP90AB1, HSP90B1, HSP90AA1, FKBP5, CREB1, PRKACB | |
Up-regulated | cjc05321:Inflammatory bowel disease (IBD) | 3 | 0.030 | 0.000636646 | JUN, SMAD3, RORA |
cjc04350:TGF-beta signaling pathway | 3 | 0.030 | 0.03910693 | SMAD7, ID1, SMAD3 | |
cjc04722:Neurotrophin signaling pathway | 3 | 0.030 | 0.000546883 | NFKBIE, JUN, MAPKAPK2 | |
cjc05169:Epstein-Barr virus infection | 3 | 0.030 | 0.000983148 | NFKBIE, JUN, TNFAIP3 | |
KEGG: Kyoto Encyclopedia of Genes and Genomes; FDR: False Discovery Rate. |
Gene | Degree of connectivity | P value | Log FC |
JUN | 47 | ≤0.001 | -2.121 |
MYC | 44 | ≤0.001 | 1.932 |
HSP90AA1 | 40 | 0.040 | 1.155 |
PCNA | 35 | 0.004 | 1.649 |
CREB1 | 33 | 0.006 | 1.617 |
IL1B | 33 | 0.009 | 1.351 |
IL8 | 33 | ≤0.001 | 1.241 |
SMARCA2 | 30 | 0.002 | 1.131 |
TLR4 | 30 | 0.008 | 1.821 |
RB1 | 28 | 0.003 | 1.176 |
RANBP2 | 28 | 0.002 | 1.855 |
EGR1 | 24 | 0.003 | 1.721 |
PTGS2 | 24 | 0.001 | 3.214 |
ENO1 | 23 | 0.013 | 1.953 |
XPO1 | 22 | 0.015 | 1.067 |
Top 15 hub genes with higher degree of connectivity |
DEGs | Gene title | Gene symbol | LogFC | Biological function | ||||
Up-regulated | early growth response 3 | EGR3 | 3.25296217 | Regulating systemic autoimmunity | ||||
muscleblind like splicing regulator 1 | MBNL1 | 3.22538031 | Regulating pre-mRNA alternative splicing | |||||
prostaglandin-endoperoxide synthase 2 | PTGS2 | 3.21406038 | Catalyzings the conversion of arachidonic acid to prostaglandins; Immune response suppression | |||||
early growth response 2 | EGR2 | 2.96759548 | Intrinsic negative regulator of DC immunogenicity; Mmonocyte/macrophage cell fate determination | |||||
thioredoxin interacting protein | TXNIP | 2.76192581 | Linking oxidative stress to inflammasome activation | |||||
Down-regulated | GABA type A receptor associated protein like 3 pseudogene | GABARAPL3 | −1.78817386 | Inhibiting inflammasome | ||||
nuclear pore complex interacting protein family member B3 | NPIPB3 | −1.82835573 | Modulating Type I interferon | |||||
regulator of G-protein signaling 1 | RGS1 | −1.87910047 | Regulating chemokine signalling | |||||
cAMP responsive element modulator | CREM | −1.93814192 | Suppresseing IL-2 and TCRζ chain gene transcription | |||||
Jun proto-oncogene, AP-1 transcription factor subunit | JUN | −2.12183042 | Modulating inflammation |
Expression | Category | Term | Count | PValue |
Up-regulated | GOTERM_BP_DIRECT | GO:0006954~inflammatory response | 9 | 0.008 |
GOTERM_BP_DIRECT | GO:0006955~immune response | 8 | 0.012 | |
GOTERM_BP_DIRECT | GO:0032496~response to lipopolysaccharide | 7 | 0.001 | |
GOTERM_BP_DIRECT | GO:0071260~cellular response to mechanical stimulus | 6 | 2.66E-04 | |
GOTERM_BP_DIRECT | GO:0017148~negative regulation of translation | 5 | 0.001 | |
GOTERM_CC_DIRECT | GO:0070062~extracellular exosome | 51 | 1.41E-04 | |
GOTERM_CC_DIRECT | GO:0005634~nucleus | 49 | 0.011 | |
GOTERM_CC_DIRECT | GO:0016020~membrane | 27 | 5.87E-05 | |
GOTERM_CC_DIRECT | GO:0005654~nucleoplasm | 27 | 0.021 | |
GOTERM_CC_DIRECT | GO:0005829~cytosol | 19 | 0.049 | |
GOTERM_MF_DIRECT | GO:0005524~ATP binding | 25 | 0.039 | |
GOTERM_MF_DIRECT | GO:0044822~poly(A) RNA binding | 23 | 0.006 | |
GOTERM_MF_DIRECT | GO:0003682~chromatin binding | 11 | 0.008 | |
GOTERM_MF_DIRECT | GO:0000166~nucleotide binding | 10 | 0.016 | |
GOTERM_MF_DIRECT | GO:0005525~GTP binding | 10 | 0.044 | |
Down-regulated | GOTERM_BP_DIRECT | GO:0032922~circadian regulation of gene expression | 3 | 0.008 |
GOTERM_BP_DIRECT | GO:0043433~negative regulation of sequence-specific DNA binding transcription factor activity | 3 | 0.011 | |
GOTERM_BP_DIRECT | GO:0045087~innate immune response | 3 | 0.001 | |
GOTERM_BP_DIRECT | GO:0070301~cellular response to hydrogen peroxide | 2 | 0.003 | |
GOTERM_BP_DIRECT | GO:0043124~negative regulation of I-kappaB kinase/NF-kappaB signaling | 2 | ≤0.001 | |
GOTERM_CC_DIRECT | GO:0005634~nucleus | 14 | 0.032 | |
GOTERM_CC_DIRECT | GO:0005654~nucleoplasm | 13 | 7.15E-04 | |
GOTERM_CC_DIRECT | GO:0005813~centrosome | 5 | 0.019 | |
GOTERM_CC_DIRECT | GO:0005667~transcription factor complex | 3 | 0.007 | |
GOTERM_MF_DIRECT | GO:0005515~protein binding | 58 | 0.003 | |
GOTERM_MF_DIRECT | GO:0008083~mitochondrial uncoupling | 5 | 0.031 | |
GOTERM_MF_DIRECT | GO:0005125~cytokine activity | 5 | 0.032 | |
GO: Gene Ontology. |
Category | Term | Count | % | P-Value | Genes |
Down-regulated | ptr04141:Protein processing in endoplasmic reticulum | 11 | 0.030 | 5.49E-04 | SEC23A, HSP90AB1, HSP90B1, HSP90AA1, UBE2J1, MAN1A1, EDEM3, UBE2D1, SAR1B, TRAM1, SSR1 |
ptr05134:Legionellosis | 7 | 0.020 | 5.25E-04 | CXCL2, CXCL8, IL1B, TLR4, HSPD1, CASP1, SAR1B | |
ptr04064:NF-kappa B signaling pathway | 7 | 0.020 | 0.004304358 | PTGS2, BCL2A1, CXCL8, IL1B, TLR4, TAB2, ATM | |
ptr04621:NOD-like receptor signaling pathway | 6 | 0.017 | 0.002195688 | HSP90AB1, HSP90AA1, CXCL8, IL1B, CASP1, TAB2 | |
ptr04915:Estrogen signaling pathway | 6 | 0.017 | 0.023154474 | HSP90AB1, HSP90B1, HSP90AA1, FKBP5, CREB1, PRKACB | |
Up-regulated | cjc05321:Inflammatory bowel disease (IBD) | 3 | 0.030 | 0.000636646 | JUN, SMAD3, RORA |
cjc04350:TGF-beta signaling pathway | 3 | 0.030 | 0.03910693 | SMAD7, ID1, SMAD3 | |
cjc04722:Neurotrophin signaling pathway | 3 | 0.030 | 0.000546883 | NFKBIE, JUN, MAPKAPK2 | |
cjc05169:Epstein-Barr virus infection | 3 | 0.030 | 0.000983148 | NFKBIE, JUN, TNFAIP3 | |
KEGG: Kyoto Encyclopedia of Genes and Genomes; FDR: False Discovery Rate. |
Gene | Degree of connectivity | P value | Log FC |
JUN | 47 | ≤0.001 | -2.121 |
MYC | 44 | ≤0.001 | 1.932 |
HSP90AA1 | 40 | 0.040 | 1.155 |
PCNA | 35 | 0.004 | 1.649 |
CREB1 | 33 | 0.006 | 1.617 |
IL1B | 33 | 0.009 | 1.351 |
IL8 | 33 | ≤0.001 | 1.241 |
SMARCA2 | 30 | 0.002 | 1.131 |
TLR4 | 30 | 0.008 | 1.821 |
RB1 | 28 | 0.003 | 1.176 |
RANBP2 | 28 | 0.002 | 1.855 |
EGR1 | 24 | 0.003 | 1.721 |
PTGS2 | 24 | 0.001 | 3.214 |
ENO1 | 23 | 0.013 | 1.953 |
XPO1 | 22 | 0.015 | 1.067 |
Top 15 hub genes with higher degree of connectivity |