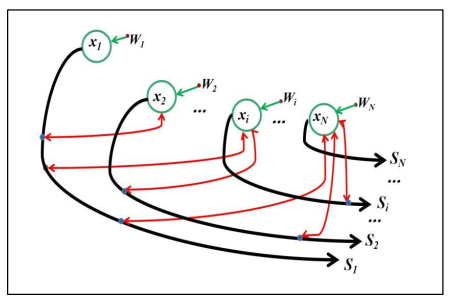
Within the swiftly evolving domain of neural networks, the discrete Hopfield-SAT model, endowed with logical rules and the ability to achieve global minima of SAT problems, has emerged as a novel prototype for SAT solvers, capturing significant scientific interest. However, this model shows substantial sensitivity to network size and logical complexity. As the number of neurons and logical complexity increase, the solution space rapidly contracts, leading to a marked decline in the model's problem-solving performance. This paper introduces a novel discrete Hopfield-SAT model, enhanced by Crow search-guided fuzzy clustering hybrid optimization, effectively addressing this challenge and significantly boosting solving speed. The proposed model unveils a significant insight: its uniquely designed cost function for initial assignments introduces a quantification mechanism that measures the degree of inconsistency within its logical rules. Utilizing this for clustering, the model utilizes a Crow search-guided fuzzy clustering hybrid optimization to filter potential solutions from initial assignments, substantially narrowing the search space and enhancing retrieval efficiency. Experiments were conducted with both simulated and real datasets for 2SAT problems. The results indicate that the proposed model significantly surpasses traditional discrete Hopfield-SAT models and those enhanced by genetic-guided fuzzy clustering optimization across key performance metrics: Global minima ratio, Hamming distance, CPU time, retrieval rate of stable state, and retrieval rate of global minima, particularly showing statistically significant improvements in solving speed. These advantages play a pivotal role in advancing the discrete Hopfield-SAT model towards becoming an exemplary SAT solver. Additionally, the model features exceptional parallel computing capabilities and possesses the potential to integrate with other logical rules. In the future, this optimized model holds promise as an effective tool for solving more complex SAT problems.
Citation: Caicai Feng, Saratha Sathasivam, Nurshazneem Roslan, Muraly Velavan. 2-SAT discrete Hopfield neural networks optimization via Crow search and fuzzy dynamical clustering approach[J]. AIMS Mathematics, 2024, 9(4): 9232-9266. doi: 10.3934/math.2024450
[1] | Xiaojun Xie, Saratha Sathasivam, Hong Ma . Modeling of 3 SAT discrete Hopfield neural network optimization using genetic algorithm optimized K-modes clustering. AIMS Mathematics, 2024, 9(10): 28100-28129. doi: 10.3934/math.20241363 |
[2] | Farah Liyana Azizan, Saratha Sathasivam, Nurshazneem Roslan, Ahmad Deedat Ibrahim . Logic mining with hybridized 3-satisfiability fuzzy logic and harmony search algorithm in Hopfield neural network for Covid-19 death cases. AIMS Mathematics, 2024, 9(2): 3150-3173. doi: 10.3934/math.2024153 |
[3] | Gaeithry Manoharam, Azleena Mohd Kassim, Suad Abdeen, Mohd Shareduwan Mohd Kasihmuddin, Nur 'Afifah Rusdi, Nurul Atiqah Romli, Nur Ezlin Zamri, Mohd. Asyraf Mansor . Special major 1, 3 satisfiability logic in discrete Hopfield neural networks. AIMS Mathematics, 2024, 9(5): 12090-12127. doi: 10.3934/math.2024591 |
[4] | Muhammad Aqmar Fiqhi Roslan, Nur Ezlin Zamri, Mohd. Asyraf Mansor, Mohd Shareduwan Mohd Kasihmuddin . Major 3 Satisfiability logic in Discrete Hopfield Neural Network integrated with multi-objective Election Algorithm. AIMS Mathematics, 2023, 8(9): 22447-22482. doi: 10.3934/math.20231145 |
[5] | Nur 'Afifah Rusdi, Nur Ezlin Zamri, Mohd Shareduwan Mohd Kasihmuddin, Nurul Atiqah Romli, Gaeithry Manoharam, Suad Abdeen, Mohd. Asyraf Mansor . Synergizing intelligence and knowledge discovery: Hybrid black hole algorithm for optimizing discrete Hopfield neural network with negative based systematic satisfiability. AIMS Mathematics, 2024, 9(11): 29820-29882. doi: 10.3934/math.20241444 |
[6] | Nurshazneem Roslan, Saratha Sathasivam, Farah Liyana Azizan . Conditional random k satisfiability modeling for k = 1, 2 (CRAN2SAT) with non-monotonic Smish activation function in discrete Hopfield neural network. AIMS Mathematics, 2024, 9(2): 3911-3956. doi: 10.3934/math.2024193 |
[7] | Bing Li, Yuan Ning, Yongkun Li . Besicovitch almost periodic solutions to Clifford-valued high-order Hopfield fuzzy neural networks with a $ D $ operator. AIMS Mathematics, 2025, 10(5): 12104-12134. doi: 10.3934/math.2025549 |
[8] | Xiaoyan Liu, Mohd Shareduwan Mohd Kasihmuddin, Nur Ezlin Zamri, Yunjie Chang, Suad Abdeen, Yuan Gao . Higher order Weighted Random k Satisfiability ($k = 1, 3$) in Discrete Hopfield Neural Network. AIMS Mathematics, 2025, 10(1): 159-194. doi: 10.3934/math.2025009 |
[9] | S. Neelakandan, Sathishkumar Veerappampalayam Easwaramoorthy, A. Chinnasamy, Jaehyuk Cho . Fuzzy adaptive learning control network (FALCN) for image clustering and content-based image retrieval on noisy dataset. AIMS Mathematics, 2023, 8(8): 18314-18338. doi: 10.3934/math.2023931 |
[10] | Jin Gao, Lihua Dai . Anti-periodic synchronization of quaternion-valued high-order Hopfield neural networks with delays. AIMS Mathematics, 2022, 7(8): 14051-14075. doi: 10.3934/math.2022775 |
Within the swiftly evolving domain of neural networks, the discrete Hopfield-SAT model, endowed with logical rules and the ability to achieve global minima of SAT problems, has emerged as a novel prototype for SAT solvers, capturing significant scientific interest. However, this model shows substantial sensitivity to network size and logical complexity. As the number of neurons and logical complexity increase, the solution space rapidly contracts, leading to a marked decline in the model's problem-solving performance. This paper introduces a novel discrete Hopfield-SAT model, enhanced by Crow search-guided fuzzy clustering hybrid optimization, effectively addressing this challenge and significantly boosting solving speed. The proposed model unveils a significant insight: its uniquely designed cost function for initial assignments introduces a quantification mechanism that measures the degree of inconsistency within its logical rules. Utilizing this for clustering, the model utilizes a Crow search-guided fuzzy clustering hybrid optimization to filter potential solutions from initial assignments, substantially narrowing the search space and enhancing retrieval efficiency. Experiments were conducted with both simulated and real datasets for 2SAT problems. The results indicate that the proposed model significantly surpasses traditional discrete Hopfield-SAT models and those enhanced by genetic-guided fuzzy clustering optimization across key performance metrics: Global minima ratio, Hamming distance, CPU time, retrieval rate of stable state, and retrieval rate of global minima, particularly showing statistically significant improvements in solving speed. These advantages play a pivotal role in advancing the discrete Hopfield-SAT model towards becoming an exemplary SAT solver. Additionally, the model features exceptional parallel computing capabilities and possesses the potential to integrate with other logical rules. In the future, this optimized model holds promise as an effective tool for solving more complex SAT problems.
The SAT problem is a classic NP-complete problem in computer science [1]. The fundamental objective of the SAT problem is to determine whether a given Boolean logic expression has a set of Boolean variable assignments that make the expression true (satisfiable). This problem holds significant importance in both theoretical computer science and practical applications, such as logic programming, circuit design, and artificial intelligence. Various methods exist to solve the SAT problem, including traditional exhaustive search methods, branch and bound, learning and backtracking techniques, specialized SAT solvers, and neural computational approaches. Among these, the application of discrete Hopfield Neural Networks (DHNN) for solving the SAT problem has garnered widespread attention as an emerging research direction.
Discrete Hopfield Neural Networks (DHNN) are a classical self-feedback neural network model [2]. DHNN acquires the weight matrix of the objective function using Hebbian learning and dynamically updates to a stable state based on the principle of energy minimization, which corresponds to potential solutions of the objective function. Due to its unique energy minimization characteristics, DHNN is often used as a tool for solving combinatorial optimization problems. The SAT problem is essentially a combinatorial optimization problem. In recent years, with breakthroughs in neural symbolic learning on neural networks and the discovery of the Wan Abdullah learning method, researchers have found a suitable way to embed the SAT problem as an objective function in discrete Hopfield networks and obtain solutions to the SAT problem.
In 1992, Wan Abdullah introduced a novel DHNN learning method that successfully embedded special logic programming as symbolic rules into discrete Hopfield neural networks [3]. This method, equivalent to traditional Hebbian learning, was formally named the Wan Abdullah learning method (WA method) in 2011 [4]. In WA method, logic rules of the SAT problem are learned in Conjunctive Normal Form (CNF) and stored in the form of an optimal weight matrix. Neurons in the neural network store the truth values of atomic propositions, and different combinations of neuron states express different logical clauses. A cost function corresponding to the CNF is constructed using logic inconsistency theory, and the optimal weight matrix representing the CNF is learned by comparing the cost function with the energy function. WA method embeds logic rules into DHNN, effectively storing the SAT problem as an objective function in DHNN. Building upon this, an Exhaustive search algorithm [5] is applied to retrieve the global minimum of the SAT problem. Starting with an initial assignment in the assignment space, neurons calculate local fields based on the optimal weight matrix and update to the state of minimum energy following the principle of energy minimization. This minimum energy state of the network corresponds to the global minimum of the SAT problem, which is also a consistent interpretation to the CNF [6]. This makes all clauses of the CNF true, rendering the CNF satisfied with its cost function of 0. The size of the assignment space is 2N, where N represents the number of variables in the CNF.
In 2014, Sathasivam et al. successfully embedded high-order Horn SAT into DHNN [6]. Different logic rules typically represent constraints or conditions of different types of real-world scenarios related to SAT. Motivated by this, researchers have continued to develop DHNN models with different types of logic rules for SAT problems, successfully obtaining the desired solutions. In 2017, Kasihmuddin et al. proposed DHNN for 2-SAT problems [5], and subsequently, Mansor et al. introduced DHNN for 3-SAT problems with higher-order logic rules [7]. Considering that not all combinatorial problems are inherently satisfiable, DHNN models based on maximum K-SAT logic rules were proposed in 2018 [8]. In 2020, Sathasivam proposed the DHNN-RAN-2-SAT model incorporating first and second-order logic rules [9]. In 2023, DHNN with 3-SAT fuzzy logic was introduced by Azizan and Sathasivam [10]. These DHNN-based models for SAT, collectively referred to as DHNN-SAT models or DHNN-SAT-WA models, have been applied to solve various constraint optimization problems [11,12,13,14,15], owing to the diversity of logic rules they encompass. However, as researchers have delved deeper into DHNN-SAT models, they have discovered that due to the inherent limitations of discrete Hopfield neural networks, the computational efficiency of DHNN-SAT models is not optimal for large-scale problems, and they tend to get trapped in local minima. To mitigate these issues, scholars have attempted to incorporate heuristic elements to enhance the accuracy of DHNN-SAT models in the optimization process [16,17,18,19,20]. Currently, these studies have shown promising results in achieving high global minimum value ratios, representing accuracy, in models with fewer neurons [21].
However, the DHNN-SAT model, as a hybrid network integrating discrete Hopfield neural networks and SAT problem rules, faces challenges not only due to the limitations of discrete Hopfield neural networks but also due to the logical complexity of SAT rules. As the logical complexity of SAT rules increases, interference between neurons and the redundancy of neurons also increase, leading to a drastic decrease in model computational accuracy and speed. Therefore, addressing how to enhance the solving capability, and improve the accuracy, and speed of DHNN-SAT models under the influence of both model size and logical complexity is an urgent task. Presently, the literature on this topic is notably scarce. In 2011, Sathasivam discussed the use of fuzzy logic to solve logic programs with lower logical complexity [22]. In this work, each clause of the CNF contained a positive literal, guaranteeing the existence of at least one satisfying solution. This article focuses on binary satisfiability problems (2-SAT) with more complex logical relations. In 2-SAT, each clause is composed of exactly two literals (Boolean variables or their negations), and the entire Boolean formula represents the conjunction (AND operation) of these clauses. 2-SAT, as a fundamental subset of Boolean satisfiability problems, holds profound significance in the fields of computational theory and algorithm design. Due to its simpler problem representation [23,24], it has gained favor among researchers in various domains [25,26,27,28]. In traditional DHNN-2SAT models, as the model size and logical complexity increase, numerous interferences and redundancies occur among neurons, leading to a rapid decrease in the number of global minima and more frequent oscillations. Moreover, the final convergence state of the model increasingly depends on its initial configuration, limiting the solution of the model to specific problems. As logical complexity continues to increase and enters the realm of hard-to-solve areas, 2-SAT problems shift from having many satisfying solutions to being hard to satisfy, resulting in poor solution accuracy and speed for the model.
On one hand, considering that, during the application of the traditional Exhaustive search algorithm to retrieve global minima, as the number of neurons and logical complexity increase, interference and redundancy affect the logical inconsistency of each CNF of initial assignments in the assignment space. The degree of logical inconsistency determines whether the CNF can successfully converge to satisfying solutions. Fortunately, the cost function benefits from its unique structure, which enables it to quantify the degree of initial assignment inconsistency of CNFs. Inspired by this, this paper employs fuzzy clustering techniques to cluster initial assignments in the assignment space, filtering out potential satisfying solutions. Compared to the Exhaustive search algorithm, this significantly reduces the search space, improving retrieval speed. On the other hand, considering that, in the context of CNFs, only global minima correspond to useful consistent explanations. Fuzzy clustering is essentially a local search technique and highly sensitive to the choice of cluster centers. To ensure that the model maintains excellent global optimization capability in hard-to-solve areas with a large number of neurons and high logical complexity, it is crucial to introduce a global optimization algorithm to guide the selection of optimal initial cluster centers for fuzzy clustering. Therefore, this paper selects the novel Crow Search Algorithm (CSA) to guide fuzzy clustering in the context of global optimization, proposing the DHNN-2SAT model with Crow search algorithm-guided fuzzy clustering (DHNN-2SAT-CSAFC model). To comprehensively assess the performance of the proposed DHNN-2SAT-CSAFC model, this paper incorporates another renowned global optimization algorithm, the Genetic Algorithm. This integration results in the creation of the DHNN-2SAT-GAFC model. In order to establish a robust benchmark for comparison, the traditional DHNN-2SAT-WA model, which employs the Exhaustive search algorithm, is included alongside the DHNN-2SAT-CSAFC model as a control group.
The remainder of this paper is organized as follows: Section 2 provides the foundational knowledge, including an introduction to DHNN models, the Wan Abdullah method, fuzzy C-means clustering, and the Crow search algorithm. In Section 3, the implementation and workflow of the DHNN-2SAT-CSAFC model, guided by the Crow search algorithm, are described in detail. In Section 4, a series of simulation experiments are conducted to solve target 2-SAT problems of different scales and logical complexities. The results are analyzed and compared among the proposed DHNN-2SAT-CSAFC model and two reference models: the traditional Exhaustive search algorithm-based DHNN-2SAT-WA model and the Genetic Algorithm-guided fuzzy clustering-based DHNN-2SAT-GAFC model, using five evaluation metrics. Finally, in Section 5, this work is summarized.
The Discrete Hopfield Neural Network (DHNN) is a recursive neural network renowned for its proficient associative memory functions. A key distinguishing feature of DHNN is the use of an energy function to gauge the stability of the network state. DHNN constitutes a monolayer network comprising N neurons, designated as {x1,x2,⋯,xN}. The state of each neuron is bipolar, conventionally denoted as Si∈{−1,1}. Neurons engage in bidirectional communication, transmitting their outputs to other neurons via synaptic connections while concurrently receiving input from other neurons, as shown in Figure 1. Synaptic weight Wij between neurons xi and xj undergo learning via the Hebbian rule.
Wij=∑(2Si−1)(2Sj−1). | (1) |
The synaptic weights are strictly symmetric. Each neuron maintains full connectivity without self-feedback, leading to Wjj=Wii=0.
The input of neuron xi at time t is referred to as the "local field, " denoted as
hi(t)=∑jWijSj(t)−Wi, | (2) |
where Si is the state of unit xi;Wij is the synaptic weight from xj to xi; and Wi is the predefined threshold for xi.
The activated neurons adhere to the well-known McCulloch-Pitts Updating Rule and update their states according to the formula (3) based on the local field's magnitude. influenced by minimal energy excitation, the network converges to a stable state. In this context, the activation function is typically chosen to be a binary sign function, consistent with the Discrete Hopfield Neural Network.
Si(t+1)=sign(hi(t))={1,hi(t)≥0,−1,hi(t)<0. | (3) |
The Lyapunov energy function is expressed as follows,
HP=−12∑i∑jWijSiSj−∑iWiSi. | (4) |
Because the output variation ΔHP can only be 0 or negative values, energy can only decrease or remain constant with each update during the network's dynamic evolution.
For each stable state, its Lyapunov energy H is computed, and it is determined whether this stable state is the global minimum based on whether the energy reaches the minimum energy. If the conditions specified in Eq (5) are met, then the stable state is considered a global minimum. Otherwise, it is deemed a local minimum.
|H−HminP|<σ. | (5) |
Here, HminP represents the Lyapunov minimum energy, and σ represents the user-defined tolerance value.
The Wan-Abdullah (WA) method leverages principles from logical programming theory to construct a cost function, providing a unique perspective on developing a novel learning approach for DHNN by uncovering logical relationships among neurons. Recognized as one of the earliest learning methods based on logical inconsistency for extracting synaptic weights [3], the WA method has been empirically demonstrated to be equivalent to Hebbian learning [4].
In the Wan Abdullah method, the rationality and effectiveness of representing various logical rules for the SAT problem in the form of Conjunctive Normal Form (CNF) clauses within the framework of neural symbol integration have been explicitly demonstrated in [29]. Consequently, various logical rules for the SAT problem are expressed in CNF and corresponding cost functions are constructed.
By comparing these cost functions with energy functions, optimal connection weights representing the logical rules are learned. Finally, these optimal weights are stored in the Discrete Hopfield Neural Network in the form of the best weight matrix.
The subsequent section elucidates the precise principles and sequential procedures entailed in acquiring synaptic weights via the utilization of the WA method.
Step 1. For the given 2SAT formula below, associate the Boolean variables Sα and Sβ with the binary neurons xα and xβ of the Hopfield neural network.
P=(Sα∨Sβ)∧(Sα∨¬Sβ)∧(¬Sα∨Sβ)∧(¬Sα∨¬Sβ)∧(ST∨SQ). | (6) |
Step 2. Apply De Morgan's law for negation of P,
¬P=(¬Sα∧¬Sβ)∨(¬Sα∧Sβ)∨(Sα∧¬Sβ)∨(Sα∧Sβ)∨(¬ST∧¬SQ). | (7) |
Step 3. Construct the cost function Ep for ¬P, where 12(1−SX) and 12(1+SX) represent the logical values of neurons X (X occurs) and ¬X (X does not occur) within ¬P. Here, if X is true, then SX equals 1, and if X is false, then SX equals -1. Utilize multiplication to denote conjunction connectors and addiction to represent disjunction connectors. Therefore, the cost function for Eq (6) is as follows:
Ep=12(1−Sα)12(1−Sβ)+12(1−Sα)12(1+Sβ)+12(1+Sα)12(1−Sβ) |
+12(1+Sα)12(1+Sβ)+12(1−ST)12(1−SQ). | (8) |
It is worth noting that in this specific construction method, the cost function Ep exhibits a notable feature: its value is directly correlated with the quantity of inconsistent clauses.
Step 4. Seek a consistent interpretation for P, which involves finding the combination of minimum values for ¬P's inconsistencies. The minimum value of Ep corresponds to the "most consistent" selection for S. Through a comparative analysis of cost function (8) and energy function (5), the specific synaptic weight values can be determined, as outlined in Table 1. The flowchart for the Wan Abdullah method can be found in Figure 2.
Synaptic Weights | Sα∨Sβ | Sα∨¬Sβ | ¬Sα∨Sβ | ¬Sα∨¬Sβ | ST∨SQ |
Wα | 1/4 | 1/4 | -1/4 | -1/4 | 0 |
Wβ | 1/4 | -1/4 | 1/4 | -1/4 | 0 |
Wαβ | -1/2 | 1/2 | 1/2 | -1/2 | 0 |
WT | 0 | 0 | 0 | 0 | 1/4 |
WQ | 0 | 0 | 0 | 0 | 1/4 |
WTQ | 0 | 0 | 0 | 0 | -1/2 |
The Fuzzy C-Means (FCM) clustering algorithm, initially introduced by Dunn [30] and subsequently advanced by Bezdek [31], stands as a significant cornerstone within the realm of fuzzy clustering methodologies. Fuzzy C-Means (FCM) leverages the principles of fuzzy mathematics to express the probability of each sample belonging to different clusters as values ranging from 0 to 1. This enables each sample to be associated with multiple distinct classes. The clustering process involves the minimization of an objective function.
Let X={x1,x2,⋯,xn} be the data set and X have to be partitioned into C clusters based on the features of the data set. Using distance as the metric for similarity evaluation, the membership degree of sample xj to class Xi is defined as
μji=1/∑Cl=1(djidjl)2m−1,m∈Z,m>1, | (9) |
μji∈[0,1] also needs to satisfy equations (10) and (11).
∑Ci=1μji=1,j=1,2,⋯,n, | (10) |
0<∑nj=1μji<n,i=1,2,⋯,C, | (11) |
m is the fuzzification parameter. It is used to dominate the influence of membership grade and therefore the centroids and reduces the noise sensitivity in the computation of the centroids.
The objective function of FCM is as follows
Jm(U,V)=∑nj∑Ci(μji)m(dji)2,m>1. | (12) |
The centroids are updated according to the following formula
vi(k+1)=∑nj=1[(μji)m⋅xj]∑nj=1(μji)m,i=1,2,⋯,C. | (13) |
Following the update of all centroids, it is imperative to recompute the fresh membership values. This iterative procedure persists until consecutive iterations converge to identical centroid positions or until the saturation of the objective function is attained. In culmination, guided by the computed membership values across all classes for each individual sample, the assignment of samples to their appropriate categories is achieved, thereby automating the process of sample data classification. Figure 3 depicts the flowchart for the fuzzy C-means clustering method.
The Crow search algorithm (CSA) is a metaheuristic optimization algorithm inspired by the intelligent behavior of Crows. It was introduced by Alireza Askarzadeh in 2016 [32] and achieves a balance between local and global search by controlling two key parameters: flight length and perception probability. Unlike many other well-known heuristic algorithms, CSA requires fewer parameter adjustments and is relatively easy to implement. Consequently, it has found widespread applications in diverse optimization problem domains, including power systems [33], healthcare [34], image processing [35], and chemical engineering [36], among others.
In this optimization scheme, each Crow serves as a representative of a potential solution to the problem under consideration. The assessment of these solutions hinges on their fitness, a measure dictated by the objective function. Assuming there are n Crows, each with its food stash hidden in an undisclosed location, we operate within a predetermined limit of maximum iterations, denoted as Tmax. Within the t-th iteration, the positions of Crow k and its best-remembered location are xkt and memkt, respectively. Crow k then embarks on a random pursuit of Crow j, in hopes of unveiling the hiding place of Crow j 's sustenance. Two distinct scenarios unfold in this endeavor:
(a) Crow j remains oblivious to the fact that Crow k is tracking it, thereby leading Crow k to the spot where food is concealed.
(b) Crow j, discerning Crow k 's tracking intent, endeavors to safeguard the location of its
sustenance by misleading Crow k, luring it to an alternate random spot within the search space.
Using AP to represent the perception probability of Crow j being tracked. The position update equation for Crow k manifests as follows:
xkt+1={xkt+rk×fl×(memjt−xkt),rk≥AP,randomposition,rk<AP. | (14) |
Here, rk∈(0,1) signifies the random probability associated with Crow k, while fl represents the flight length. An excessively modest flight length may entrap us within a local minimum, whereas an extended flight length may unveil a global minimum.
We subsequently compute the fitness value f(xkt+1) pertaining to the novel position. If the fitness value of this newfound location surpasses that of the previously remembered position, update the memory of Crow k.
memkt+1={xkt+1,f(xkt+1)<f(memkt)memkt,otherwise., | (15) |
The iteration terminates when the termination criterion is met, and the global optimal solution is output. Figure 4 illustrates the flowchart of the Crow search algorithm.
The conventional DHNN-2SAT-WA model utilizes an exhaustive search algorithm in the retrieval phase, tasked with updating, ensuring convergence, and evaluating each random initial assignment within the assignment space of size '2N'. Its goal is to search for consistent interpretations satisfying 2-SAT clauses. However, as the network's size and logical complexity increase, interference and redundancy between neurons intensify, leading to a rapid contraction of the solution space. More and more random initial assignments experience a staggering increase in their corresponding cost functions due to the growing number of inconsistent clauses. These initial assignments tend to exhibit significant oscillations during subsequent updates, making the model susceptible to local minima, prolonged convergence cycles, or even convergence failure.
Addressing these challenges posed by the traditional DHNN-2SAT-WA model, this paper introduces a novel solution: Crow Search-guided Fuzzy Clustering-based Hybrid Optimization DHNN-2SAT model, abbreviated as DHNN-2SAT-CSAFC. In the DHNN-2SAT-CSAFC model, the initial assignments within the assignment space are subjected to fuzzy clustering based on the cost function, as its size directly relates to the number of inconsistent clauses. This process identifies potential solutions, significantly reducing the search space and improving retrieval efficiency.
However, fuzzy clustering inherently represents a local search technique and is highly sensitive to cluster center selection. Concurrently, the 2-SAT problem mandates stringent quality criteria for solutions. In the context of CNF, only the global minimum corresponds to consistent interpretations. Therefore, incorporating the Crow Search method, one of the latest global optimization metaheuristics, to guide fuzzy clustering for optimal initial cluster center selection can substantially enhance global optimization capabilities. CSA has been proven to have a significant advantage in various optimization domains, particularly when integrated with FCM. References [37,38,39,40,41,42,43] highlight CSA's effectiveness in a variety of optimization fields, especially its integration with FCM [44,45,46].
The DHNN-2SAT-CSAFC model represents an innovative amalgamation of the DHNN-2SAT framework with the Crow Search Fuzzy Clustering Hybrid Optimization Algorithm. Its primary objective is convergent to a set of Boolean variable assignments that adhere to the CNF formula associated with the 2SAT problem. During the model's initialization phase, a fundamental mapping is established: each neuron within the Hopfield network is linked to a specific Boolean variable within the CNF. In practice, neurons serve as repositories for preserving the truth values of atomic variables, while synaptic weights serve as a representation of the intricate relationships that exist between variables and clauses.
Subsequent to initialization, the operational workflow of this model unfolds through two pivotal phases: the learning phase and the retrieval phase. During the learning phase, the acquisition of synaptic weight matrices is orchestrated through the utilization of the WA learning methodology. As the model progresses to the retrieval phase, activated neurons undertake iterative updates, adjusting their local fields and states in alignment with predefined update rules. This iterative process continues until the network converges to a stable equilibrium, during which the dynamic energy function undergoes a consistent monotonic descent.
Ultimately, the pivotal role of the energy function is in determining whether this stabilized equilibrium corresponds to the global minimum of the 2SAT problem. The identification of the global minimum signifies a coherent interpretation of the CNF. The specific implementation steps of the DHNN-2SAT-CSAFC model are outlined as follows:
Step 1. Model initialization.
For a given 2SAT problem, translate into the corresponding CNF formula, denoted as P. Let P contain n Boolean variables and m clauses. The initialization of various parameters within the optimization algorithm is also carried out.
Step 2. Neuron assignment.
Each Boolean variable is uniquely assigned a Hopfield neuron, with neurons used to store the truth values of individual atoms. Consequently, the Hopfield network is configured with n neurons {x1,x2,⋯,xn}, and their corresponding states at time t are denoted as {S1(t),S2(t),⋯,Sn(t)}.
Step 3. Learning phase.
During this phase, the model acquires synaptic weights using the WA method. The cost function EP for the negation of ¬P is derived, followed by the computation of synaptic weights through a comparison between the cost function EP and the energy function H.
Step 4. Retrieval phase.
A batch of M random initial assignments {P1,P2,⋯,PM} is generated during this phase, where Pi=Pi(S(t))=Pi(S1(t),S2(t),⋯,Sn(t)),1≤i≤M. These assignments may or may not constitute consistent interpretations of the CNF formula. The cost function EP={EP1,EP2,⋯,EPM} for these initial assignments is computed based on Eq (7).
Step 5. Fuzzy clustering with Crow search guidance.
Leveraging the cost function, a fuzzy clustering algorithm guided by Crow search is employed to cluster {P1,P2,⋯,PM}. Pre-select a subset of random initial assignments with favorable cost functions, denoted as Ak=Ak(S(t))=Ak(S1(t),S2(t),⋯,Sn(t)),k∈Z,t=0. Initially, the Crow search algorithm minimizes an objective function Jm identical to that of the fuzzy clustering algorithm, resulting in optimal centroid solutions Vbest={v1,v2,⋯,vC}. Subsequently, fuzzy clustering is carried out. Importantly, the minimization process of the objective function is guided by CSA, rather than an iterative steepest descent process.
Step 6. Local field calculation.
For each A_k(S(t))=A_k(S1(t),S2(t),⋯,Sn(t)),k=0,t=0, calculates its local field A_k(h(t))=A_k(h1(t),h2(t),⋯, hn(t)).
Step 7. State updating and stability determination.
Each neuron state within A_k(S1(t),S2(t),⋯,Sn(t)) is updated according to the McCulloch-Pitts Updating Rule, with Si(t+1)=sign(hi(t))={1,hi(t)≥0−1,hi(t)<0, until a stable state is reached. If, after five consecutive runs, the neuron states remain consistent (i.e., A_k(S(t))=A_k(S(t−1)) = A_k(S(t−2)) = A_k(S(t−3)) = A_k(S(t−4))), the final state A_k(S(t)) is defined as the stable state.
Step 8. Global minimum energy assessment
The stability state's energy HA_k is assessed using the Lyapunov energy criteria. If the following condition (5) is met |HA_k−HminP|<σ. Where HminP represents the global minimum energy, and σ is the tolerance value predetermined by the user, then the stability state A_k(S(t)) is recognized as the global minimum and is stored. Otherwise, if it does not meet this condition, it is considered a local minimum, and k=k+1, leading to a repeat of Step 6.
Step 9. Calculation of metrics
This final step involves calculating metrics such as the global minima ratio, Hamming distance, CPU time, retrieval rate of stable state, and retrieval rate of global minima. Figure 5 presents the flowchart of the DHNN-2SAT-CSAFC model.
To accurately assess the performance of the proposed DHNN-2SAT-CSAFC model and enhance the robustness of experimental results, this experiment encompasses two control group models: the traditional DHNN-2SAT-WA model, which employs the exhaustive search algorithm, and another model, DHNN-2SAT-GAFC, guided by genetic algorithms for fuzzy clustering-based hybrid optimization.
Considering the high precision requirements for SAT problem solutions and the significant impact of the chosen optimization algorithm on the success rate and efficiency of consistent interpretations' search, it was crucial to examine whether the selection of CSA as the global optimization algorithm to guide fuzzy clustering, was a prudent choice. Despite several studies [47,48,49,50,51] suggesting that CSA requires relatively few parameter adjustments compared to other metaheuristic algorithms such as Particle Swarm Optimization (PSO), Shark Algorithm (SA), Genetic Algorithm (GA), Weed Algorithm (WA), Grey Wolf Optimizer (GWO), Sine Cosine Algorithm (SCA), Bat Algorithm (BA), Firefly Algorithm (FA), Moth Flame Optimization (MFO), Whale Optimization Algorithm (WOA), Invasive Weed Optimization (IWO), and Electromagnetic Mechanism-based Algorithm (EM), which have demonstrated superior performance in specific application domains. However, adhering to rigorous scientific research principles, this experiment additionally introduces another control group model, DHNN-2SAT-GAFC, guided by genetic algorithms for fuzzy clustering-based hybrid optimization. In this DHNN-2SAT-GAFC model, another classic global optimization algorithm, Genetic Algorithm, was chosen to guide the fuzzy clustering process. Genetic Algorithm (GA), initially proposed by John Holland in 1975 [52], serves as an early heuristic algorithm embedded within the Fuzzy Clustering method (GAFC), and it has found wide applications across domains such as transportation and classification problems [53,54,55,56].
In this simulation experiment for solving the 2-SAT problem, we utilized MATLAB R2021b, running on a laptop equipped with the Windows 11 operating system, an AMD Ryzen R7-5800H processor, and 16GB of RAM. During the experiment, we optimized the relevant parameters, as detailed in Tables 2–4, through iterative adjustments based on trial and error. The logical complexity of a propositional formula is measured by the ratio of the number of clauses to the number of Boolean variables, known as the constraint rate coefficient or constraint density. The number of neurons ranged from 10 to 100 in increments of 10, while the constraint ratio coefficient varied from 0.1 to 2.0 in steps of 0.1. To mitigate statistical errors, we conducted 100 trials with different neuron combinations for each level of logical complexity, with each combination undergoing 250*NN experiments. Simulated datasets were employed to evaluate the performance of the DHNN-2SAT-WA, DHNN-2SAT-GAFC, and DHNN-2SAT-CSAFC models using five performance evaluation metrics: global minima ratio, Hamming distance, CPU time, retrieval rate of stable states, and retrieval rate of global minima.
Parameter | Parameter Value |
Number of neurons | Between 10 and 100, in steps of 10 |
Constraint density | Between 0.1 and 2.0, in steps of 0.1 |
CPU time threshold | 3 hours |
Tolerance of DHNN-2SAT | 0.001 |
Parameter | Parameter Value |
Population size | 50 |
Crossover rate | 0.9 |
Selection rate | 0.9 |
Fuzziness parameter | 2 |
Maximum number of iterations | 100 |
Mutation rate | 0.05 |
Number of clusters | 5 |
Convergence threshold | 0.001 |
Parameter | Parameter Value |
Population size | 50 |
Flight length | 0.2 |
Number of clusters | 5 |
Convergence threshold | 0.001 |
Maximum number of iterations | 100 |
Perceived probability | 0.1 |
Fuzziness parameter | 2 |
The Global Minima Ratio (GMR) is a metric defined as the ratio of global minima count to the total number of solutions [57]. For example, if the GMR value equals 0.9605, it signifies that out of 10,000 simulated results, 9,605 correspond to global minima, while the remaining 395 represent local minima solutions. GMR is a dependable measure of algorithm efficiency, with a value near 1 indicating model robustness [58].
Figures 6 and 7 illustrate the GMR results concerning diverse logical complexities and varying numbers of neurons. At lower logical complexities, all three models benefit from the robust global search capability of the discrete Hopfield neural network model with SAT. Every neuron converges toward the global optimal solution, yielding a GMR of 1.
However, as the constraint ratio coefficient escalates from 0.1 to 2.0 while maintaining a constant number of neurons, logical complexity surges. Different logical clauses start to interfere with each other due to sharing the same atomic elements [4]. Coupled with a significant amount of logical redundancy, this leads to a rapid reduction in the solution space, making it increasingly challenging for models to retrieve satisfying solutions. Consequently, the GMR also declines swiftly. Notably, even in such scenarios, the DHNN-2SAT-CSAFC and DHNN-2SAT-GAFC models, incorporating optimization methods, consistently demonstrate significantly higher GMR values than the conventional DHNN-2SAT-WA model.
According to Table 5, as the number of neurons increases from 10 to 100, the average GMR for the HNN-2SAT-CSAFC model is 1.173 times that of the HNN-2SAT-WA model and 1.053 times that of the HNN-2SAT-GAFC model. This can be attributed to the Crow search algorithm-guided fuzzy clustering, which preselects initial assignments with strong logical consistency, effectively directing the reduction of network energy.
Number of neurons | Geometric Mean | ||
DHNN-2SAT-GAFC / DHNN-2SAT-WA | DHNN-2SAT-CSAFC / DHNN-2SAT-WA | DHNN-2SAT-CSAFC / DHNN-2SAT-GAFC | |
10 | 1.087 | 1.101 | 1.012 |
20 | 1.091 | 1.106 | 1.013 |
30 | 1.104 | 1.132 | 1.025 |
40 | 1.107 | 1.139 | 1.029 |
50 | 1.117 | 1.169 | 1.046 |
60 | 1.169 | 1.245 | 1.065 |
70 | 1.171 | 1.266 | 1.082 |
80 | 1.132 | 1.225 | 1.082 |
90 | 1.142 | 1.286 | 1.126 |
100 | 1.019 | 1.081 | 1.061 |
10 ~ 100 | 1.113 | 1.173 | 1.053 |
Hamming distance serves as a metric to gauge the precision of model solutions by quantifying the disparity in bits between stable states and global minimum values. A Hamming distance nearing zero signifies a more precise convergence of stable states toward the global minimum.
Figures 8 and 9 illustrate that at lower constraint ratio coefficients, all three models, with varying numbers of neurons, exhibit Hamming distances that are essentially zero. When the number of neurons remains constant and the constraint ratio coefficient gradually increases to 2.0, the network's memory capacity diminishes, leading to a reduced solution space. Although the Hamming distance gradually increases under these conditions, it still maintains a relatively low level.
With the number of neurons expanding from 10 to 100, even though an increase in redundant and duplicated variables may introduce some interference to the weights, the connections among neurons become more intricate and closely interconnected. As a result, the models' learning capabilities not only remain unimpaired but also encourage a further convergence of final states toward the global minimum Hamming distance. Table 6 reveals that the average Hamming distance for the DHNN-2SAT-CSAFC model is 0.668 times that of the DHNN-2SAT-WA model and 0.898 times that of the DHNN-2SAT-GAFC model. This indicates that all three models perform exceptionally well in terms of precision when solving the 2-SAT problem. Notably, the HNN-2SAT-CSAFC model excels in conducting more precise global searches.
Number of neurons | Geometric Mean | ||
DHNN-2SAT-GAFC / DHNN-2SAT-WA | DHNN-2SAT-CSAFC / DHNN-2SAT-WA | DHNN-2SAT-CSAFC / DHNN-2SAT-GAFC | |
10 | 0.567 | 0.494 | 0.872 |
20 | 0.655 | 0.609 | 0.929 |
30 | 0.692 | 0.507 | 0.734 |
40 | 0.726 | 0.699 | 0.963 |
50 | 0.803 | 0.774 | 0.964 |
60 | 0.748 | 0.678 | 0.907 |
70 | 0.795 | 0.736 | 0.925 |
80 | 0.836 | 0.756 | 0.904 |
90 | 0.774 | 0.680 | 0.878 |
100 | 0.826 | 0.755 | 0.914 |
10 ~ 100 | 0.744 | 0.668 | 0.898 |
CPU time refers to the average time required for each model to achieve a global minimum value. Smaller average CPU times indicate higher computational efficiency for the models.
Figures 10 and 11 provide insights that when the number of neurons is relatively low, neither the DHNN-2SAT-CSAFC model nor the DHNN-2SAT-GAFC model exhibits a notable advantage. This is because utilizing the Crow search algorithm or Genetic Algorithm to guide the optimal centroids consumes time.
However, with an increase in the number of neurons and constraint rate coefficient, due to the fully connected nature of DHNN where each neuron is connected to every other neuron, the computational cost grows exponentially. The traditional HNN-2SAT-WA model, which employs the Exhaustive search algorithm, needs to retrieve randomly generated initial assignments from the assignment space within a given time threshold, incurring significant time costs. In contrast, the HNN-2SAT-CSAFC and HNN-2SAT-GAFC models benefit from the integration of different optimization algorithms, implementing directed filtering of random assignments within the assignment space, significantly reducing the search space and thus greatly decreasing computational time.
Furthermore, as shown in Table 7, when the number of neurons increases from 10 to 100, the computational speed of the HNN-2SAT-CSAFC model is 0.131 times that of the HNN-2SAT-WA model and 0.414 times that of the HNN-2SAT-GAFC model. Moreover, as the constraint ratio coefficient increases, the speed advantage of HNN-2SAT-CSAFC exhibits a noticeable linear growth trend. This indicates that within the same given time frame, the number of consistent interpretations obtained using the proposed HNN-2SAT-CSAFC model is 7.6334 times and 2.415 times that of the other two control models, respectively. Clearly, Crow Search-guided fuzzy clustering, due to its fewer parameters and simpler implementation, is more effective in reducing the computational complexity of the 2-SAT problem and accelerating global search compared to the other two models.
Number of neurons | Geometric Mean | ||
DHNN-2SAT-GAFC/ DHNN-2SAT-WA | DHNN-2SAT-CSAFC/ DHNN-2SAT-WA | DHNN-2SAT-CSAFC/ DHNN-2SAT-GAFC | |
10 | 2.220 | 0.447 | 0.201 |
20 | 0.570 | 0.233 | 0.410 |
30 | 0.446 | 0.164 | 0.367 |
40 | 0.251 | 0.129 | 0.513 |
50 | 0.572 | 0.117 | 0.205 |
60 | 0.391 | 0.096 | 0.245 |
70 | 0.117 | 0.089 | 0.763 |
80 | 0.209 | 0.092 | 0.441 |
90 | 0.095 | 0.080 | 0.840 |
100 | 0.175 | 0.113 | 0.647 |
10~100 | 0.316 | 0.131 | 0.414 |
With the increase in network capacity and logical complexity, the solution space contracts, making it increasingly difficult for models to discover the global minimum within specified timeframes. Especially when network capacity and logical complexity reach a certain threshold, the 2-SAT problem enters the region of difficulty in solving, where extensive search efforts during the retrieval phase often end in vain. At this point, the focus of model improvement is to maximize the success rate of locating the global minimum and even local minima within each retrieval task. To assess the success rate of model retrieval efforts, we introduce two novel evaluation criteria: the retrieval rate of stable states and the retrieval rate of the global minimum. Specifically, the retrieval rate of stable states is defined as the ratio of the number of stable states to the total number of retrievals, while the retrieval rate of the global minimum is defined as the ratio of the number of global minima to the total number of retrievals.
Figures 12 to 15 illustrate that both the DHNN-2SAT-CSAFC model and the DHNN-2SAT-GAFC model effectively leverage optimization algorithms to identify potential solutions, substantially enhancing the model's success rate. Data presented in Tables 8 and 9 indicate that as the number of neurons increases from 10 to 100, the retrieval rate of stable states and the retrieval rate of the global minimum for the DHNN-2SAT-CSAFC model are 2.423 and 2.726 times that of the DHNN-2SAT-WA model, respectively, and 1.105 and 1.157 times that of the DHNN-2SAT-GAFC model. When the search region enters the challenging zone, the retrieval rates of stable states and the retrieval rates of the global minimum experience a sharp decline, almost approaching zero. In such circumstances, the DHNN-2SAT-CSAFC model effectively boosts the retrieval rate of stable states and the retrieval rate of the global minimum to approximately four times that of the traditional model.
Number of neurons | Geometric Mean | ||
DHNN-2SAT-GAFC/ DHNN-2SAT-WA | DHNN-2SAT-CSAFC/ DHNN-2SAT-WA | DHNN-2SAT-CSAFC/ DHNN-2SAT-GAFC | |
10 | 1.576 | 1.614 | 1.024 |
20 | 1.831 | 1.901 | 1.038 |
30 | 1.869 | 2.001 | 1.071 |
40 | 2.070 | 2.214 | 1.070 |
50 | 2.167 | 2.386 | 1.101 |
60 | 2.401 | 2.676 | 1.115 |
70 | 2.426 | 2.661 | 1.097 |
80 | 2.486 | 2.973 | 1.196 |
90 | 2.562 | 2.879 | 1.124 |
100 | 2.718 | 3.314 | 1.219 |
10~100 | 2.192 | 2.423 | 1.105 |
Number of neurons | Geometric Mean | ||
DHNN-2SAT-GAFC / DHNN-2SAT-WA | DHNN-2SAT-CSAFC / DHNN-2SAT-WA | DHNN-2SAT-CSAFC / DHNN-2SAT-GAFC | |
10 | 1.662 | 1.717 | 1.033 |
20 | 1.890 | 1.978 | 1.046 |
30 | 1.902 | 2.088 | 1.098 |
40 | 2.141 | 2.322 | 1.085 |
50 | 2.255 | 2.770 | 1.228 |
60 | 2.610 | 3.064 | 1.174 |
70 | 2.633 | 2.963 | 1.125 |
80 | 2.851 | 3.456 | 1.212 |
90 | 2.907 | 3.431 | 1.180 |
100 | 3.055 | 4.314 | 1.412 |
10 ~ 100 | 2.357 | 2.726 | 1.157 |
In Chapter 4, we exhaustively demonstrated the significant advantages of the HNN-2SAT-CSAFC model over traditional HNN-2SAT-WA and HNN-2SAT-GAFC models in solving 2-SAT problems of varying logical complexities and model scales through the application on simulated datasets. The experimental results on the simulated datasets not only validated the effectiveness of the HNN-2SAT-CSAFC model but also laid a solid foundation for further research. However, to comprehensively evaluate the HNN-2SAT-CSAFC model's capability in addressing real-world application issues, we believe it is necessary to conduct further tests on real-world datasets. Chapter 5 aims to bridge this research gap by applying the HNN-2SAT-CSAFC model and traditional HNN-2SAT-WA model to specific 2-SAT problem instances, exploring their performance in solving practical problems.
Due to the absence of a renowned dataset exclusively containing 2-SAT problems, we utilized the JNH dataset from the DIMACS benchmark instances available on SATLIB (https://www. cs. ubc.ca/ ~hoos/SATLIB/benchm.html). This dataset comprises 16 satisfiable instances and 34 unsatisfiable instances, provided by John Hooker, generated through a hard generator, presenting random problems with certain challenging characteristics, such as the absence of single-literal clauses and hard density. To construct a representative set of 2-SAT instances, we selected 16 problem instances (jnhi−2SAT,1≤i≤16) that contain only clauses with two literals from these 16 satisfiable instances (jnhi,1≤i≤16). This ensured that our experimental dataset maintained a clear 2-SAT structure, consistent with real-world application problems, where each clause contains precisely two literals.
In the subsequent experiments, the traditional HNN-2SAT-WA model and the HNN-2SAT-CSAFC model proposed in this paper were applied to solve these 16 2-SAT instances. During the retrieval phase, the HNN-2SAT-WA model directly searches among 10,000 different combinations of neuron initial assignments, whereas the HNN-2SAT-CSAFC model employs Crow-guided fuzzy clustering to preprocess these 10,000 combinations of neuron initial assignments, picking out a subset of potential solutions for retrieval. This significantly narrows the actual search space and reduces computational efforts. To minimize statistical errors, each combination of neurons will undergo 100 repeated experiments to calculate an average value. Specific parameters are detailed in Tables 10 and 11. We will comprehensively document the performance of the HNN-2SAT-CSAFC model and the HNN-2SAT-WA model in solving these 16 2-SAT instances across the following five performance metrics: Global minima ratio, Hamming distance, CPU time, retrieval rate of stable states, and retrieval rate of global minima, as shown in Table 12. Through this experiment, we will objectively assess the performance advantage of the HNN-2SAT-CSAFC model over the traditional HNN-2SAT-WA model in solving practical application problems, thereby providing valuable insights for future research and applications.
Parameter | Parameter Value |
Number of initial assignments | 10000 |
CPU time threshold | 4h |
Tolerance of DHNN-2SAT | 0.001 |
Parameter | Parameter Value | Parameter | Parameter Value |
Number of initial assignments | 10000 | Population size | 50 |
Flight length | 0.2 | maximum number of iterations | 100 |
Number of clusters | 4 | perceived probability | 0.1 |
Convergence threshold | 0.001 | Fuzziness parameter | 2 |
Instances of 2-SAT | Global Minima Ratio | Hamming Distance | CPU Time (s) | Retrieval Rate of Stable State (%) | Retrieval Rate of Global Minima (%) | |||||
HNN-2SAT-WA | HNN-2SAT-CSAFC | HNN-2SAT-WA | HNN-2SAT-CSAFC | HNN-2SAT-WA | HNN-2SAT-CSAFC | HNN-2SAT-WA | HNN-2SAT-CSAFC | HNN-2SAT-WA | HNN-2SAT-CSAFC | |
Jnh1-2SAT | 0.6667 | 0.7308 | 0.0061 | 0.0049 | 591.7 | 69.8 | 0.0750 | 0.2600 | 0.0500 | 0.1900 |
Jnh2-2SAT | 0.8335 | 0.8889 | 0.0030 | 0.0018 | 1490.9 | 28.2 | 0.0300 | 0.1333 | 0.025 | 0.1185 |
Jnh3-2SAT | 0.1667 | 0.2593 | 0.0230 | 0.0190 | 1491.0 | 24.4 | 0.1500 | 0.5703 | 0.025 | 0.1477 |
Jnh4-2SAT | 0.6250 | 0.6364 | 0.0047 | 0.0080 | 595.2 | 20.6 | 0.1000 | 0.4797 | 0.0625 | 0.3053 |
Jnh5-2SAT | 1 | 1 | 0 | 0 | 42.2 | 4.1 | 0.6000 | 1.2420 | 0.6000 | 1.2420 |
Jnh6-2SAT | 0.5455 | 0.5600 | 0.0062 | 0.0060 | 385.7 | 33.4 | 0.1375 | 0.4523 | 0.075 | 0.2533 |
Jnh7-2SAT | 0.3800 | 0.4444 | 0.0224 | 0.0096 | 1300.5 | 30.0 | 0.1625 | 0.2595 | 0.0625 | 0.1153 |
Jnh8-2SAT | 0.9908 | 0.9915 | 0.0001 | 0.0001 | 13.4 | 5.5 | 1.3625 | 3.4544 | 1.3500 | 3.4250 |
Jnh9-2SAT | 0.4100 | 0.4348 | 0.0217 | 0.0116 | 346.3 | 53.0 | 0.1500 | 0.3966 | 0.0625 | 0.1724 |
Jnh10-2SAT | 0.9286 | 0.9454 | 0.0014 | 0.0013 | 139.0 | 9.0 | 0.1750 | 0.6342 | 0.1625 | 0.5996 |
Jnh11-2SAT | 0.7000 | 0.7083 | 0.0039 | 0.0033 | 478.1 | 37.1 | 0.1250 | 0.3453 | 0.0875 | 0.2446 |
Jnh12-2SAT | 1 | 1 | 0 | 0 | 330.3 | 13.0 | 0.0875 | 0.2737 | 0.0875 | 0.2737 |
Jnh13-2SAT | 1 | 1 | 0 | 0 | 54.4 | 5.7 | 0.6500 | 1.1886 | 0.6500 | 1.1886 |
Jnh14-2SAT | 0.7143 | 0.7778 | 0.0034 | 0.0034 | 2179.9 | 45.7 | 0.0875 | 0.1042 | 0.0625 | 0.0810 |
Jnh15-2SAT | 0.1325 | 0.2571 | 0.0188 | 0.0153 | 3198.2 | 46.6 | 0.1625 | 0.8070 | 0.0125 | 0.2075 |
Jnh16-2SAT | 0.1250 | 0.1852 | 0.0200 | 0.0198 | 3393.1 | 145.7 | 0.1000 | 0.3508 | 0.0125 | 0.0650 |
As depicted in Figures 16 to 20, the DHNN-2SAT-CSAFC model outperforms the DHNN-2SAT-WA model across all five metrics. Notably, a significant advantage of the DHNN-2SAT-CSAFC model is observed in terms of CPU time. CPU time is a crucial factor in measuring algorithm efficiency, as it directly reflects the average time required by the model to obtain a satisfactory solution for a 2SAT instance. Given the early stages of the HNN-SAT model as an emerging SAT processor and the current context of precious computing resources, reducing the CPU time needed for algorithm operation not only saves valuable computing resources but also enhances the feasibility and appeal of the algorithm in practical applications. Therefore, we decided to conduct a more in-depth statistical analysis on the CPU time metric.
As shown in Tables 13 and 14, with the significance level set at 0.05, the p-values of the Jarque-Bera test for the DHNN-2SAT-WA model and the DHNN-2SAT-CSAFC model are 0.0406 and 0.0401, respectively, both of which are less than 0.05. Thus, the CPU times for both models do not follow a normal distribution. Further analysis using the Wilcoxon signed-rank test reveals a significant difference in the median CPU times between the two models. This indicates that the DHNN-SAT-Dy-Ev model proposed in this paper has a significant improvement over the traditional DHNN-2SAT-WA model in terms of CPU time, highlighting its enhanced performance and potential for practical application.
Null Hypothesis (H0) | CPU time follows a normal distribution. | ||
Alternative Hypothesis (Ha) | CPU time does not follow a normal distribution. | ||
P | HNN-2SAT-WA | 0.0406 | Reject the null hypothesis, indicating that CPU time does not follow a normal distribution. |
HNN-2SAT-CSAFC | 0.0401 | Reject the null hypothesis, indicating that CPU time does not follow a normal distribution. |
Null Hypothesis (H0) | There is no significant difference in the median CPU times between the two models. | |
Alternative Hypothesis (Ha) | There is a significant difference in the median CPU times between the two models. | |
P | 0.0004 | Reject the null hypothesis, indicating that there is a significant difference in the median CPU times. |
The HNN-SAT model, developed in recent years as a prototype of emerging SAT solvers, focuses on a crucial research direction: improving the model to achieve faster solving speeds and higher precision in addressing SAT problems. The previous studies predominantly focused on the impact of model scale. This study comprehensively investigates both the scale of the model and its logical complexity, along with their impacts on HNN-2SAT. With the model scale and logical complexity gradually increasing, HNN-2SAT evolves from having multiple satisfiable solutions to becoming challenging to solve, or even unsolvable.
Grounded on the discovery that "the cost function can serve as a quantification tool for the degree of inconsistency in logical rules, " this establishes a basis for fuzzy clustering. The proposed HNN-2SAT-CSAFC model integrates the advantages of CSA and fuzzy clustering, effectively mitigating this issue. Compared to the traditional HNN-2SAT-WA and another heuristic-integrated model, HNN-2SAT-GAFC, the HNN-2SAT-CSAFC model features fewer parameters, simpler implementation, and leverages a Crow search-guided fuzzy clustering hybrid optimization method for batch processing of search objects. This approach pre-selects potential solutions, narrows the search space, and significantly enhances computational speed. Additionally, two new evaluation criteria are defined, namely the retrieval rate of stable state and retrieval rate of global minima, effectively assessing the model's success rate.
In Chapter 4, we employ a simulation dataset of 2 SAT problems to experiment with our solution model. Within the simulated environment for solving a series of 2SAT problems with gradually increasing model scale and logical complexity, we meticulously observe the differing patterns exhibited by the HNN-2SAT-WA, HNN-2SAT-GAFC, and HNN-2SAT-CSAFC solution models across five metrics: global minima ratio, Hamming distance, CPU time, retrieval rate of stable state, and retrieval rate of global minima. The experimental results on the simulation dataset not only validate the effectiveness of the HNN-2SAT-CSAFC model but also lay a solid foundation for further research.
In this simulation experiment, as the constraint rate coefficient increased from 0.1 to 2.0 and the number of neurons grew from 10 to 100, the HNN-2SAT-CSAFC model consistently achieved the best performance in terms of global minima ratio, Hamming distance, CPU time, retrieval rate of stable state, and retrieval rate of global minima. Compared to the HNN-2SAT-WA and HNN-2SAT-GAFC models, the HNN-2SAT-CSAFC model exhibited superior results, with an average GMR being 1.173 times and 1.053 times higher, an average Hamming distance being 0.668 times and 0.898 times lower, an average CPU time being 0.131 times and 0.414 times less, a number of consistent interpretations obtained within the same time frame being 7.6334 times and 2.415 times greater, a stable state retrieval rate being 2.423 times and 1.105 times higher, and a global minimum retrieval rate being 2.726 times and 1.157 times greater, respectively. This signifies that the HNN-2SAT-CSAFC model not only enhances the quality of solutions in terms of accuracy and precision but also increases the quantity of solutions retrieved, even in regions where finding solutions is challenging. Notably, the HNN-2SAT-CSAFC model demonstrates a notable improvement in computational efficiency. Furthermore, the model's advantages across five evaluation metrics are expected to exhibit a linear growth trend with increasing network size and logical complexity. Evidently, the HNN-2SAT-CSAFC model is better suited for handling large-scale, highly logically complex 2-SAT problems.
In Chapter 5, the application of the solving models to 2SAT problem instances from real datasets was explored, demonstrating the superior capability of the proposed HNN-2SAT-CSAFC model over traditional models in addressing practical application issues. This is the first time in the field where the DHNN-SAT model has been applied to solve real dataset instances. Experimental results indicate that the HNN-2SAT-CSAFC model outperforms the DHNN-2SAT-WA model across five evaluation metrics. Notably, it exhibits statistically significant improvements in CPU time. This signifies that the DHNN-2SAT-CSAFC model is more efficient in solving practical SAT problems.
Although there is room for improvement in the scale and speed of solving compared to other SAT-solving tools, the HNN-2SAT-CSAFC model offers advantages such as parallel computing, ease of integration with other logical rules, and the absence of preprocessing for duplicates and redundancies. Looking ahead, with continued exploration and refinement, this model holds great potential to evolve into an outstanding SAT-solving tool.
The authors declare that no Artificial Intelligence (AI) tools were utilized in the drafting of this article.
The authors are deeply thankful to the editor and the reviewers for their valuable suggestions to improve the quality of this manuscript. The authors extend their appreciation for the financial support from the Mathematical Modeling and Intelligent Computing Research Center (GKY-2022CQJG-1) by the Guangdong University of Science and Technology, as well as the Fundamental Research Grant (FRGS) by the Ministry of Higher Education Malaysia (MOHE), FRGS/1/2022/STG06/USM/02/11.
All authors declare no conflicts of interest in this paper.
[1] | S. A. Cook, The complexity of theorem-proving procedures, In: Logic, automata, and computational complexity: The works of Stephen A. Cook, 2023,143–152. https://doi.org/10.1145/3588287.3588297 |
[2] |
J. J. Hopfield, Neural networks and physical systems with emergent collective computational abilities, P. Natl. Acad. Sci., 79 (1982), 2554–2558. https://doi.org/10.1073/pnas.79.8.2554 doi: 10.1073/pnas.79.8.2554
![]() |
[3] |
W. A. T. W. Abdullah, Logic programming on a neural network, Int. J. Intell. Syst., 7 (1992), 513–519. https://doi.org/10.1002/int.4550070604 doi: 10.1002/int.4550070604
![]() |
[4] |
S. Sathasivam, W. A. T. W. Abdullah, Logic mining in neural network: reverse analysis method, Computing, 91 (2011), 119–133. https://doi.org/10.1007/s00607-010-0117-9 doi: 10.1007/s00607-010-0117-9
![]() |
[5] | M. S. M. Kasihmuddin, M. A. Mansor, S. Sathasivam, Hybrid genetic algorithm in the Hopfield network for logic satisfiability problem, Pertanika J. Sci. Technol., 25 (2017), 139–152. |
[6] |
S. Sathasivam, N. P. Fen, M. Velavan, Reverse analysis in higher order Hopfield network for higher order horn clauses, Appl. Math. Sci., 8 (2014), 601–612. https://doi.org/10.12988/ams.2014.310565 doi: 10.12988/ams.2014.310565
![]() |
[7] | M. A. Mansor, M. S. M. Kasihmuddin, S. Sathasivam, Artificial immune system paradigm in the Hopfield network for 3-satisfiability problem, Pertanika J. Sci. Technol., 25 (2017), 1173–1188. |
[8] | M. S. M. Kasihmuddin, M. A. Mansor, S. Sathasivam, Discrete Hopfield neural network in restricted maximum k-satisfiability logic programming, Sains Malays., 47 (2018), 1327–1335. https://dx.doi.org/10.17576/jsm-2018-4706-30 |
[9] |
S. Sathasivam, M. A. Mansor, A. I. M. Ismail, S. Z. M. Jamaludin, M. S. M. Kasihmuddin, M. Mamat, Novel random k satisfiability for k≤ 2 in Hopfield neural network, Sains Malays., 49 (2020), 2847–2857. https://doi.org/10.17576/jsm-2020-4911-23 doi: 10.17576/jsm-2020-4911-23
![]() |
[10] |
F. L. Azizan, S. Sathasivam, Randomised alpha-cut fuzzy logic hybrid model in Solving 3-satisfiability Hopfield neural network, Malays. J. Fundam. Appl. Sci., 19 (2023), 43–55. https://doi.org/10.11113/mjfas.v19n1.2697 doi: 10.11113/mjfas.v19n1.2697
![]() |
[11] |
S. A. Alzaeemi, S. Sathasivam, Examining the forecasting movement of palm oil price using RBFNN-2SATRA metaheuristic algorithms for logic mining, IEEE Access, 9 (2021), 22542–22557. https://doi.org/10.1109/ACCESS.2021.3054816 doi: 10.1109/ACCESS.2021.3054816
![]() |
[12] |
M. A. Mansor, M. S. M. Kasihmuddin, S. Sathasivam, VLSI circuit configuration using satisfiability logic in Hopfield network, Int. J. Intell. Syst. Appl., 8 (2016), 22. https://doi.org/10.5815/ijisa.2016.09.03 doi: 10.5815/ijisa.2016.09.03
![]() |
[13] |
M. A. Mansor, M. S. M. Kasihmuddin, S. Sathasivam, Enhanced Hopfield network for pattern satisfiability optimization, Int. J. Intell. Syst. Appl., 8 (2016), 27. https://doi.org/10.5815/ijisa.2016.11.04 doi: 10.5815/ijisa.2016.11.04
![]() |
[14] |
M. S. M. Kasihmuddin, M. A. Mansor, S. Sathasivam, Bezier curves satisfiability model in enhanced Hopfield network, Int. J. Intell. Syst. Appl., 8 (2016), 9. https://doi.org/10.5815/ijisa.2016.12.02 doi: 10.5815/ijisa.2016.12.02
![]() |
[15] |
M. S. M. Kasihmuddin, M. A. Mansor, S. Sathasivam, Students' performance via satisfiability reverse analysis method with Hopfield neural network, AIP Conf Proc., 2184 (2019), 060035. https://doi.org/10.1063/1.5136467 doi: 10.1063/1.5136467
![]() |
[16] |
L. C. Kho, M. S. M. Kasihmuddin, M. A. Mansor, S. Sathasivam, 2 Satisfiability logical rule by using ant colony optimization in Hopfield neural network, AIP Conf. Proc., 2184 (2019), 060009. https://doi.org/10.1063/1.5136441 doi: 10.1063/1.5136441
![]() |
[17] | M. A. Mansor, M. S. M. Kasihmuddin, S. Z. M. Jamaluddin, S. Sathasivam, Pattern 2 satisfiability snalysis via hybrid artificial bee colony algorithm as a learning algorithm, Commun. Comput. Appl. Math., 2 (2020), 12–18. |
[18] |
S. Sathasivam, M. A. Mansor, M. S. M. Kasihmuddin, H. Abubakar, Election algorithm for random k satisfiability in the Hopfield neural network, Processes, 8 (2020), 568. https://doi.org/10.3390/pr8050568 doi: 10.3390/pr8050568
![]() |
[19] |
M. A. Mansor, M. S. M. Kasihmuddin, S. Sathasivam, Grey wolf optimization algorithm with discrete Hopfield neural network for 3 Satisfiability analysis, J. Phys.: Conf. Ser., 1821 (2021), 012038. https://doi.org/10.1088/1742-6596/1821/1/012038 doi: 10.1088/1742-6596/1821/1/012038
![]() |
[20] |
F. L. Azizan, S. Sathasivam, M. K. M Ali, N. Roslan, C. Feng, Hybridised network of fuzzy logic and a genetic algorithm in solving 3-satisfiability Hopfield neural networks, Axioms, 12 (2023), 250. https://doi.org/10.3390/axioms12030250 doi: 10.3390/axioms12030250
![]() |
[21] |
M. S. Mohd Kasihmuddin, N. S. Abdul Halim, S. Z. Mohd Jamaludin, M. Mansor, A. Alway, N. E. Zamri, et al., Logic mining approach: Shoppers' purchasing data extraction via evolutionary algorithm, J. Inf. Commun. Technol., 22 (2023), 309–335. https://doi.org/10.32890/jict2023.22.3.1 doi: 10.32890/jict2023.22.3.1
![]() |
[22] | S. Sathasivam, Applying fuzzy logic in neuro symbolic integration, World Appl. Sci. J., 17 (2012), 79–86. |
[23] |
B. Bollobás, C. Borgs, J. T. Chayes, J. H. Kim, D. B. Wilson, The scaling window of the 2-SAT transition, Random Struct. Algor., 18 (2001), 201–256. https://doi.org/10.1002/rsa.1006 doi: 10.1002/rsa.1006
![]() |
[24] | M. Fürer, S. P. Kasiviswanathan, Algorithms for counting 2-SAT solutions and colorings with applications, In: Algorithmic aspects in information and management, 2007. https://doi.org/10.1007/978-3-540-72870-2_5 |
[25] | M. Formann, F. Wagner, A packing problem with applications to lettering of maps, In: Proceedings of the seventh annual symposium on computational geometry, 1991. |
[26] |
S. Ramnath, Dynamic digraph connectivity hastens minimum sum-of-diameters clustering, SIAM J. Discrete Math., 18 (2004), 272–286. https://doi.org/10.1137/S0895480102396099 doi: 10.1137/S0895480102396099
![]() |
[27] |
R. Miyashiro, T. Matsui, A polynomial-time algorithm to find an equitable home-away assignment, Oper. Res. Lett., 33 (2005), 235–241. https://doi.org/10.1016/j.orl.2004.06.004 doi: 10.1016/j.orl.2004.06.004
![]() |
[28] |
K. J. Batenburg, W. A. Kosters, Solving Nonograms by combining relaxations, Pattern Recogn., 42 (2009), 1672–1683. https://doi.org/10.1016/j.patcog.2008.12.003 doi: 10.1016/j.patcog.2008.12.003
![]() |
[29] | S. Sathasivam, Clauses representation comparison in neuro-symbolic integration, Proceedings of the World Congress on Engineering, 2010. |
[30] |
J. C. Dunn, A fuzzy relative of the ISODATA process and its use in detecting compact well-separated clusters, J. Cyb., 3 (1973), 32–57. https://doi.org/10.1080/01969727308546046 doi: 10.1080/01969727308546046
![]() |
[31] |
J. C. Bezdek, R. Ehrlich, W. Full, FCM: The fuzzy c-means clustering algorithm, Comput. Geosci., 10 (1984), 191–203. https://doi.org/10.1016/0098-3004(84)90020-7 doi: 10.1016/0098-3004(84)90020-7
![]() |
[32] |
A. Askarzadeh, A novel metaheuristic method for solving constrained engineering optimization problems: Crow search algorithm, Comput. Struct., 169 (2016), 1–12. https://doi.org/10.1016/j.compstruc.2016.03.001 doi: 10.1016/j.compstruc.2016.03.001
![]() |
[33] | A. Saha, A. Bhattacharya, P. Das, A. K. Chakraborty, Crow search algorithm for solving optimal power flow problem, In: 2017 Second International Conference on Electrical, Computer and Communication Technologies (ICECCT), 2017. https://doi.org/10.1109/ICECCT.2017.8118028 |
[34] | A. Lenin Fred, S. N. Kumar, P. Padmanaban, B. Gulyas, H. Ajay Kumar, Fuzzy-Crow search optimization for medical image segmentation, In: Applications of hybrid metaheuristic algorithms for image processing, 2020. https://doi.org/10.1007/978-3-030-40977-7_18 |
[35] |
P. Upadhyay, J. K. Chhabra, Kapur's entropy based optimal multilevel image segmentation using Crow search algorithm, Appl. Soft Comput., 97 (2020), 105522. https://doi.org/10.1016/j.asoc.2019.105522 doi: 10.1016/j.asoc.2019.105522
![]() |
[36] |
G. Y. Abdallh, Z. Y. Algamal, A QSAR classification model of skin sensitization potential based on improving binary Crow search algorithm, Electron. J. Appl. Stat., 13 (2020), 86–95. https://doi.org/10.1285/i20705948v13n1p86 doi: 10.1285/i20705948v13n1p86
![]() |
[37] |
S. Arora, H. Singh, M. Sharma, S. Sharma, P. Anand, A new hybrid algorithm based on grey wolf optimization and Crow search algorithm for unconstrained function optimization and feature selection, IEEE Access, 7 (2019), 26343–26361. https://doi.org/10.1109/ACCESS.2019.2897325 doi: 10.1109/ACCESS.2019.2897325
![]() |
[38] | F. Davoodkhani, S. Arabi Nowdeh, A. Y. Abdelaziz, S. Mansoori, S. Nasri, M. Alijani, A new hybrid method based on gray wolf optimizer-Crow search algorithm for maximum power point tracking of photovoltaic energy system, In: Modern maximum power point tracking techniques for photovoltaic energy systems, 2020. https://doi.org/10.1007/978-3-030-05578-3_16 |
[39] | A. B. Pratiwi, A hybrid cat swarm optimization-Crow search algorithm for vehicle routing problem with time windows, In: 2017 2nd international conferences on information technology, information systems and electrical engineering (ICITISEE), 2017. https://doi.org/10.1109/ICITISEE.2017.8285529 |
[40] |
H. M. Farh, A. M. Al-Shaalan, A. M. Eltamaly, A. A. Al-Shamma'A, A novel Crow search algorithm auto-drive PSO for optimal allocation and sizing of renewable distributed generation, IEEE Access, 8 (2020), 27807–27820. https://doi.org/10.1109/ACCESS.2020.2968462 doi: 10.1109/ACCESS.2020.2968462
![]() |
[41] |
K. Gaddala, P. S. Raju, Merging lion with Crow search algorithm for optimal location and sizing of UPQC in distribution network, J. Control Autom. Electr. Syst., 31 (2020), 377–392. https://doi.org/10.1007/s40313-020-00564-1 doi: 10.1007/s40313-020-00564-1
![]() |
[42] |
R. Ganeshan, P. Rodrigues, Crow-AFL: Crow based adaptive fractional lion optimization approach for the intrusion detection, Wireless Pers. Commun., 111 (2020), 2065–2089. https://doi.org/10.1007/s11277-019-06972-0 doi: 10.1007/s11277-019-06972-0
![]() |
[43] |
D. K. Shende, S. S. Sonavane, Crow Whale-ETR: Crow Whale optimization algorithm for energy and trust aware multicast routing in WSN for IoT applications, Wireless Netw., 26 (2020), 4011–4029. https://doi.org/10.1007/s11276-020-02299-y doi: 10.1007/s11276-020-02299-y
![]() |
[44] |
A. M. Anter, A. E. Hassenian, D. Oliva, An improved fast fuzzy c-means using Crow search optimization algorithm for crop identification in agricultural, Expert Syst. Appl., 118 (2019), 340–354. https://doi.org/10.1016/j.eswa.2018.10.009 doi: 10.1016/j.eswa.2018.10.009
![]() |
[45] |
A. M. Anter, M. Ali, Feature selection strategy based on hybrid Crow search optimization algorithm integrated with chaos theory and fuzzy c-means algorithm for medical diagnosis problems, Soft Comput., 24 (2020), 1565–1584. https://doi.org/10.1007/s00500-019-03988-3 doi: 10.1007/s00500-019-03988-3
![]() |
[46] | L. O. Hall, J. C. Bezdek, S. Boggavarpu, A. Bensaid, Genetic fuzzy clustering, In: Proceedings of the first international joint conference of the north American fuzzy information processing society biannual conference, 1994,411–415. https://doi.org/10.1109/IJCF.1994.375077 |
[47] |
N. Siswanto, A. N. Adianto, H. A. Prawira, A. Rusdiansyah, A Crow search algorithm for aircraft maintenance check problem and continuous airworthiness maintenance program, JSMI, 3 (2019), 10115–10123. https://doi.org/10.30656/jsmi.v3i2.1794 doi: 10.30656/jsmi.v3i2.1794
![]() |
[48] |
D. Gupta, S. Sundaram, J. J. Rodrigues, A. Khanna, An improved fault detection Crow search algorithm for wireless sensor network, Int. J. Commun. Syst., 36 (2023), e4136. https://doi.org/10.1002/dac.4136 doi: 10.1002/dac.4136
![]() |
[49] |
J. Mandala, M. C. Rao, Privacy preservation of data using Crow search with adaptive awareness probability, J. Inf. Secur. Appl., 44 (2019), 157–169. https://doi.org/10.1016/j.jisa.2018.12.005 doi: 10.1016/j.jisa.2018.12.005
![]() |
[50] |
R. Dash, S. Samal, R. Dash, R. Rautray, An integrated TOPSIS Crow search based classifier ensemble: In application to stock index price movement prediction, Appl. Soft Comput., 85 (2019), 105784. https://doi.org/10.1016/j.asoc.2019.105784 doi: 10.1016/j.asoc.2019.105784
![]() |
[51] |
A. G. Hussien, M. Amin, M. Wang, G. Liang, A. Alsanad, A.Gumaei, et al., Crow search algorithm: Theory, recent advances, and applications, IEEE Access, 8 (2020), 173548–173565. https://doi.org/10.1109/ACCESS.2020.3024108 doi: 10.1109/ACCESS.2020.3024108
![]() |
[52] | J. H. Holland, Adaptation in natural and artificial systems: An introductory analysis with applications to biology, control, and artificial intelligence, MIT Press, 1992. |
[53] |
M. Alata, M. Molhim, A. Ramini, Optimizing of fuzzy c-means clustering algorithm using GA, Int. J. Comput. Inf. Eng., 2 (2008), 670–675. https://doi.org/10.5281/zenodo.1081049 doi: 10.5281/zenodo.1081049
![]() |
[54] |
Y. Ding, X. Fu, Kernel-based fuzzy c-means clustering algorithm based on genetic algorithm, Neurocomputing, 188 (2016), 233–238. https://doi.org/10.1016/j.neucom.2015.01.106 doi: 10.1016/j.neucom.2015.01.106
![]() |
[55] |
X. Wang, H. Wang, Driving behavior clustering for hazardous material transportation based on genetic fuzzy C-means algorithm, IEEE Access, 8 (2020), 11289–11296. https://doi.org/10.1109/ACCESS.2020.2964648 doi: 10.1109/ACCESS.2020.2964648
![]() |
[56] |
X. Cui, E. C. Yan, Fuzzy c-means cluster analysis based on variable length string genetic algorithm for the grouping of rock discontinuity sets, KSCE J. Civ. Eng., 24 (2020), 3237–3246. https://doi.org/10.1007/s12205-020-2188-2 doi: 10.1007/s12205-020-2188-2
![]() |
[57] | S. Sathasivam, Upgrading logic programming in Hopfield network, Sains Malays., 39 (2010), 115–128. |
[58] |
M. Velavan, Z. R. bin Yahya, M. N. bin Abdul Halif, S. Sathasivam, Mean field theory in doing logic programming using Hopfield network, Mod. Appl. Sci., 10 (2016), 154. https://doi.org/10.5539/mas.v10n1p154 doi: 10.5539/mas.v10n1p154
![]() |
1. | Abdullah Almogahed, Hairulnizam Mahdin, Yeong Hyeon Gu, Mohammed A. Al-Masni, Shehab Abdulhabib Alzaeemi, Mazni Omar, Abdulwadood Alawadhi, Samera Obaid Barraood, Abdul Rehman Gilal, Adnan Ameen Bakather, Multi-Classification Refactoring Framework Using Hopfield Neural Network for Sustainable Software Development, 2025, 13, 2169-3536, 31785, 10.1109/ACCESS.2025.3542087 |
Synaptic Weights | Sα∨Sβ | Sα∨¬Sβ | ¬Sα∨Sβ | ¬Sα∨¬Sβ | ST∨SQ |
Wα | 1/4 | 1/4 | -1/4 | -1/4 | 0 |
Wβ | 1/4 | -1/4 | 1/4 | -1/4 | 0 |
Wαβ | -1/2 | 1/2 | 1/2 | -1/2 | 0 |
WT | 0 | 0 | 0 | 0 | 1/4 |
WQ | 0 | 0 | 0 | 0 | 1/4 |
WTQ | 0 | 0 | 0 | 0 | -1/2 |
Parameter | Parameter Value |
Number of neurons | Between 10 and 100, in steps of 10 |
Constraint density | Between 0.1 and 2.0, in steps of 0.1 |
CPU time threshold | 3 hours |
Tolerance of DHNN-2SAT | 0.001 |
Parameter | Parameter Value |
Population size | 50 |
Crossover rate | 0.9 |
Selection rate | 0.9 |
Fuzziness parameter | 2 |
Maximum number of iterations | 100 |
Mutation rate | 0.05 |
Number of clusters | 5 |
Convergence threshold | 0.001 |
Parameter | Parameter Value |
Population size | 50 |
Flight length | 0.2 |
Number of clusters | 5 |
Convergence threshold | 0.001 |
Maximum number of iterations | 100 |
Perceived probability | 0.1 |
Fuzziness parameter | 2 |
Number of neurons | Geometric Mean | ||
DHNN-2SAT-GAFC / DHNN-2SAT-WA | DHNN-2SAT-CSAFC / DHNN-2SAT-WA | DHNN-2SAT-CSAFC / DHNN-2SAT-GAFC | |
10 | 1.087 | 1.101 | 1.012 |
20 | 1.091 | 1.106 | 1.013 |
30 | 1.104 | 1.132 | 1.025 |
40 | 1.107 | 1.139 | 1.029 |
50 | 1.117 | 1.169 | 1.046 |
60 | 1.169 | 1.245 | 1.065 |
70 | 1.171 | 1.266 | 1.082 |
80 | 1.132 | 1.225 | 1.082 |
90 | 1.142 | 1.286 | 1.126 |
100 | 1.019 | 1.081 | 1.061 |
10 ~ 100 | 1.113 | 1.173 | 1.053 |
Number of neurons | Geometric Mean | ||
DHNN-2SAT-GAFC / DHNN-2SAT-WA | DHNN-2SAT-CSAFC / DHNN-2SAT-WA | DHNN-2SAT-CSAFC / DHNN-2SAT-GAFC | |
10 | 0.567 | 0.494 | 0.872 |
20 | 0.655 | 0.609 | 0.929 |
30 | 0.692 | 0.507 | 0.734 |
40 | 0.726 | 0.699 | 0.963 |
50 | 0.803 | 0.774 | 0.964 |
60 | 0.748 | 0.678 | 0.907 |
70 | 0.795 | 0.736 | 0.925 |
80 | 0.836 | 0.756 | 0.904 |
90 | 0.774 | 0.680 | 0.878 |
100 | 0.826 | 0.755 | 0.914 |
10 ~ 100 | 0.744 | 0.668 | 0.898 |
Number of neurons | Geometric Mean | ||
DHNN-2SAT-GAFC/ DHNN-2SAT-WA | DHNN-2SAT-CSAFC/ DHNN-2SAT-WA | DHNN-2SAT-CSAFC/ DHNN-2SAT-GAFC | |
10 | 2.220 | 0.447 | 0.201 |
20 | 0.570 | 0.233 | 0.410 |
30 | 0.446 | 0.164 | 0.367 |
40 | 0.251 | 0.129 | 0.513 |
50 | 0.572 | 0.117 | 0.205 |
60 | 0.391 | 0.096 | 0.245 |
70 | 0.117 | 0.089 | 0.763 |
80 | 0.209 | 0.092 | 0.441 |
90 | 0.095 | 0.080 | 0.840 |
100 | 0.175 | 0.113 | 0.647 |
10~100 | 0.316 | 0.131 | 0.414 |
Number of neurons | Geometric Mean | ||
DHNN-2SAT-GAFC/ DHNN-2SAT-WA | DHNN-2SAT-CSAFC/ DHNN-2SAT-WA | DHNN-2SAT-CSAFC/ DHNN-2SAT-GAFC | |
10 | 1.576 | 1.614 | 1.024 |
20 | 1.831 | 1.901 | 1.038 |
30 | 1.869 | 2.001 | 1.071 |
40 | 2.070 | 2.214 | 1.070 |
50 | 2.167 | 2.386 | 1.101 |
60 | 2.401 | 2.676 | 1.115 |
70 | 2.426 | 2.661 | 1.097 |
80 | 2.486 | 2.973 | 1.196 |
90 | 2.562 | 2.879 | 1.124 |
100 | 2.718 | 3.314 | 1.219 |
10~100 | 2.192 | 2.423 | 1.105 |
Number of neurons | Geometric Mean | ||
DHNN-2SAT-GAFC / DHNN-2SAT-WA | DHNN-2SAT-CSAFC / DHNN-2SAT-WA | DHNN-2SAT-CSAFC / DHNN-2SAT-GAFC | |
10 | 1.662 | 1.717 | 1.033 |
20 | 1.890 | 1.978 | 1.046 |
30 | 1.902 | 2.088 | 1.098 |
40 | 2.141 | 2.322 | 1.085 |
50 | 2.255 | 2.770 | 1.228 |
60 | 2.610 | 3.064 | 1.174 |
70 | 2.633 | 2.963 | 1.125 |
80 | 2.851 | 3.456 | 1.212 |
90 | 2.907 | 3.431 | 1.180 |
100 | 3.055 | 4.314 | 1.412 |
10 ~ 100 | 2.357 | 2.726 | 1.157 |
Parameter | Parameter Value |
Number of initial assignments | 10000 |
CPU time threshold | 4h |
Tolerance of DHNN-2SAT | 0.001 |
Parameter | Parameter Value | Parameter | Parameter Value |
Number of initial assignments | 10000 | Population size | 50 |
Flight length | 0.2 | maximum number of iterations | 100 |
Number of clusters | 4 | perceived probability | 0.1 |
Convergence threshold | 0.001 | Fuzziness parameter | 2 |
Instances of 2-SAT | Global Minima Ratio | Hamming Distance | CPU Time (s) | Retrieval Rate of Stable State (%) | Retrieval Rate of Global Minima (%) | |||||
HNN-2SAT-WA | HNN-2SAT-CSAFC | HNN-2SAT-WA | HNN-2SAT-CSAFC | HNN-2SAT-WA | HNN-2SAT-CSAFC | HNN-2SAT-WA | HNN-2SAT-CSAFC | HNN-2SAT-WA | HNN-2SAT-CSAFC | |
Jnh1-2SAT | 0.6667 | 0.7308 | 0.0061 | 0.0049 | 591.7 | 69.8 | 0.0750 | 0.2600 | 0.0500 | 0.1900 |
Jnh2-2SAT | 0.8335 | 0.8889 | 0.0030 | 0.0018 | 1490.9 | 28.2 | 0.0300 | 0.1333 | 0.025 | 0.1185 |
Jnh3-2SAT | 0.1667 | 0.2593 | 0.0230 | 0.0190 | 1491.0 | 24.4 | 0.1500 | 0.5703 | 0.025 | 0.1477 |
Jnh4-2SAT | 0.6250 | 0.6364 | 0.0047 | 0.0080 | 595.2 | 20.6 | 0.1000 | 0.4797 | 0.0625 | 0.3053 |
Jnh5-2SAT | 1 | 1 | 0 | 0 | 42.2 | 4.1 | 0.6000 | 1.2420 | 0.6000 | 1.2420 |
Jnh6-2SAT | 0.5455 | 0.5600 | 0.0062 | 0.0060 | 385.7 | 33.4 | 0.1375 | 0.4523 | 0.075 | 0.2533 |
Jnh7-2SAT | 0.3800 | 0.4444 | 0.0224 | 0.0096 | 1300.5 | 30.0 | 0.1625 | 0.2595 | 0.0625 | 0.1153 |
Jnh8-2SAT | 0.9908 | 0.9915 | 0.0001 | 0.0001 | 13.4 | 5.5 | 1.3625 | 3.4544 | 1.3500 | 3.4250 |
Jnh9-2SAT | 0.4100 | 0.4348 | 0.0217 | 0.0116 | 346.3 | 53.0 | 0.1500 | 0.3966 | 0.0625 | 0.1724 |
Jnh10-2SAT | 0.9286 | 0.9454 | 0.0014 | 0.0013 | 139.0 | 9.0 | 0.1750 | 0.6342 | 0.1625 | 0.5996 |
Jnh11-2SAT | 0.7000 | 0.7083 | 0.0039 | 0.0033 | 478.1 | 37.1 | 0.1250 | 0.3453 | 0.0875 | 0.2446 |
Jnh12-2SAT | 1 | 1 | 0 | 0 | 330.3 | 13.0 | 0.0875 | 0.2737 | 0.0875 | 0.2737 |
Jnh13-2SAT | 1 | 1 | 0 | 0 | 54.4 | 5.7 | 0.6500 | 1.1886 | 0.6500 | 1.1886 |
Jnh14-2SAT | 0.7143 | 0.7778 | 0.0034 | 0.0034 | 2179.9 | 45.7 | 0.0875 | 0.1042 | 0.0625 | 0.0810 |
Jnh15-2SAT | 0.1325 | 0.2571 | 0.0188 | 0.0153 | 3198.2 | 46.6 | 0.1625 | 0.8070 | 0.0125 | 0.2075 |
Jnh16-2SAT | 0.1250 | 0.1852 | 0.0200 | 0.0198 | 3393.1 | 145.7 | 0.1000 | 0.3508 | 0.0125 | 0.0650 |
Null Hypothesis (H0) | CPU time follows a normal distribution. | ||
Alternative Hypothesis (Ha) | CPU time does not follow a normal distribution. | ||
P | HNN-2SAT-WA | 0.0406 | Reject the null hypothesis, indicating that CPU time does not follow a normal distribution. |
HNN-2SAT-CSAFC | 0.0401 | Reject the null hypothesis, indicating that CPU time does not follow a normal distribution. |
Null Hypothesis (H0) | There is no significant difference in the median CPU times between the two models. | |
Alternative Hypothesis (Ha) | There is a significant difference in the median CPU times between the two models. | |
P | 0.0004 | Reject the null hypothesis, indicating that there is a significant difference in the median CPU times. |
Synaptic Weights | Sα∨Sβ | Sα∨¬Sβ | ¬Sα∨Sβ | ¬Sα∨¬Sβ | ST∨SQ |
Wα | 1/4 | 1/4 | -1/4 | -1/4 | 0 |
Wβ | 1/4 | -1/4 | 1/4 | -1/4 | 0 |
Wαβ | -1/2 | 1/2 | 1/2 | -1/2 | 0 |
WT | 0 | 0 | 0 | 0 | 1/4 |
WQ | 0 | 0 | 0 | 0 | 1/4 |
WTQ | 0 | 0 | 0 | 0 | -1/2 |
Parameter | Parameter Value |
Number of neurons | Between 10 and 100, in steps of 10 |
Constraint density | Between 0.1 and 2.0, in steps of 0.1 |
CPU time threshold | 3 hours |
Tolerance of DHNN-2SAT | 0.001 |
Parameter | Parameter Value |
Population size | 50 |
Crossover rate | 0.9 |
Selection rate | 0.9 |
Fuzziness parameter | 2 |
Maximum number of iterations | 100 |
Mutation rate | 0.05 |
Number of clusters | 5 |
Convergence threshold | 0.001 |
Parameter | Parameter Value |
Population size | 50 |
Flight length | 0.2 |
Number of clusters | 5 |
Convergence threshold | 0.001 |
Maximum number of iterations | 100 |
Perceived probability | 0.1 |
Fuzziness parameter | 2 |
Number of neurons | Geometric Mean | ||
DHNN-2SAT-GAFC / DHNN-2SAT-WA | DHNN-2SAT-CSAFC / DHNN-2SAT-WA | DHNN-2SAT-CSAFC / DHNN-2SAT-GAFC | |
10 | 1.087 | 1.101 | 1.012 |
20 | 1.091 | 1.106 | 1.013 |
30 | 1.104 | 1.132 | 1.025 |
40 | 1.107 | 1.139 | 1.029 |
50 | 1.117 | 1.169 | 1.046 |
60 | 1.169 | 1.245 | 1.065 |
70 | 1.171 | 1.266 | 1.082 |
80 | 1.132 | 1.225 | 1.082 |
90 | 1.142 | 1.286 | 1.126 |
100 | 1.019 | 1.081 | 1.061 |
10 ~ 100 | 1.113 | 1.173 | 1.053 |
Number of neurons | Geometric Mean | ||
DHNN-2SAT-GAFC / DHNN-2SAT-WA | DHNN-2SAT-CSAFC / DHNN-2SAT-WA | DHNN-2SAT-CSAFC / DHNN-2SAT-GAFC | |
10 | 0.567 | 0.494 | 0.872 |
20 | 0.655 | 0.609 | 0.929 |
30 | 0.692 | 0.507 | 0.734 |
40 | 0.726 | 0.699 | 0.963 |
50 | 0.803 | 0.774 | 0.964 |
60 | 0.748 | 0.678 | 0.907 |
70 | 0.795 | 0.736 | 0.925 |
80 | 0.836 | 0.756 | 0.904 |
90 | 0.774 | 0.680 | 0.878 |
100 | 0.826 | 0.755 | 0.914 |
10 ~ 100 | 0.744 | 0.668 | 0.898 |
Number of neurons | Geometric Mean | ||
DHNN-2SAT-GAFC/ DHNN-2SAT-WA | DHNN-2SAT-CSAFC/ DHNN-2SAT-WA | DHNN-2SAT-CSAFC/ DHNN-2SAT-GAFC | |
10 | 2.220 | 0.447 | 0.201 |
20 | 0.570 | 0.233 | 0.410 |
30 | 0.446 | 0.164 | 0.367 |
40 | 0.251 | 0.129 | 0.513 |
50 | 0.572 | 0.117 | 0.205 |
60 | 0.391 | 0.096 | 0.245 |
70 | 0.117 | 0.089 | 0.763 |
80 | 0.209 | 0.092 | 0.441 |
90 | 0.095 | 0.080 | 0.840 |
100 | 0.175 | 0.113 | 0.647 |
10~100 | 0.316 | 0.131 | 0.414 |
Number of neurons | Geometric Mean | ||
DHNN-2SAT-GAFC/ DHNN-2SAT-WA | DHNN-2SAT-CSAFC/ DHNN-2SAT-WA | DHNN-2SAT-CSAFC/ DHNN-2SAT-GAFC | |
10 | 1.576 | 1.614 | 1.024 |
20 | 1.831 | 1.901 | 1.038 |
30 | 1.869 | 2.001 | 1.071 |
40 | 2.070 | 2.214 | 1.070 |
50 | 2.167 | 2.386 | 1.101 |
60 | 2.401 | 2.676 | 1.115 |
70 | 2.426 | 2.661 | 1.097 |
80 | 2.486 | 2.973 | 1.196 |
90 | 2.562 | 2.879 | 1.124 |
100 | 2.718 | 3.314 | 1.219 |
10~100 | 2.192 | 2.423 | 1.105 |
Number of neurons | Geometric Mean | ||
DHNN-2SAT-GAFC / DHNN-2SAT-WA | DHNN-2SAT-CSAFC / DHNN-2SAT-WA | DHNN-2SAT-CSAFC / DHNN-2SAT-GAFC | |
10 | 1.662 | 1.717 | 1.033 |
20 | 1.890 | 1.978 | 1.046 |
30 | 1.902 | 2.088 | 1.098 |
40 | 2.141 | 2.322 | 1.085 |
50 | 2.255 | 2.770 | 1.228 |
60 | 2.610 | 3.064 | 1.174 |
70 | 2.633 | 2.963 | 1.125 |
80 | 2.851 | 3.456 | 1.212 |
90 | 2.907 | 3.431 | 1.180 |
100 | 3.055 | 4.314 | 1.412 |
10 ~ 100 | 2.357 | 2.726 | 1.157 |
Parameter | Parameter Value |
Number of initial assignments | 10000 |
CPU time threshold | 4h |
Tolerance of DHNN-2SAT | 0.001 |
Parameter | Parameter Value | Parameter | Parameter Value |
Number of initial assignments | 10000 | Population size | 50 |
Flight length | 0.2 | maximum number of iterations | 100 |
Number of clusters | 4 | perceived probability | 0.1 |
Convergence threshold | 0.001 | Fuzziness parameter | 2 |
Instances of 2-SAT | Global Minima Ratio | Hamming Distance | CPU Time (s) | Retrieval Rate of Stable State (%) | Retrieval Rate of Global Minima (%) | |||||
HNN-2SAT-WA | HNN-2SAT-CSAFC | HNN-2SAT-WA | HNN-2SAT-CSAFC | HNN-2SAT-WA | HNN-2SAT-CSAFC | HNN-2SAT-WA | HNN-2SAT-CSAFC | HNN-2SAT-WA | HNN-2SAT-CSAFC | |
Jnh1-2SAT | 0.6667 | 0.7308 | 0.0061 | 0.0049 | 591.7 | 69.8 | 0.0750 | 0.2600 | 0.0500 | 0.1900 |
Jnh2-2SAT | 0.8335 | 0.8889 | 0.0030 | 0.0018 | 1490.9 | 28.2 | 0.0300 | 0.1333 | 0.025 | 0.1185 |
Jnh3-2SAT | 0.1667 | 0.2593 | 0.0230 | 0.0190 | 1491.0 | 24.4 | 0.1500 | 0.5703 | 0.025 | 0.1477 |
Jnh4-2SAT | 0.6250 | 0.6364 | 0.0047 | 0.0080 | 595.2 | 20.6 | 0.1000 | 0.4797 | 0.0625 | 0.3053 |
Jnh5-2SAT | 1 | 1 | 0 | 0 | 42.2 | 4.1 | 0.6000 | 1.2420 | 0.6000 | 1.2420 |
Jnh6-2SAT | 0.5455 | 0.5600 | 0.0062 | 0.0060 | 385.7 | 33.4 | 0.1375 | 0.4523 | 0.075 | 0.2533 |
Jnh7-2SAT | 0.3800 | 0.4444 | 0.0224 | 0.0096 | 1300.5 | 30.0 | 0.1625 | 0.2595 | 0.0625 | 0.1153 |
Jnh8-2SAT | 0.9908 | 0.9915 | 0.0001 | 0.0001 | 13.4 | 5.5 | 1.3625 | 3.4544 | 1.3500 | 3.4250 |
Jnh9-2SAT | 0.4100 | 0.4348 | 0.0217 | 0.0116 | 346.3 | 53.0 | 0.1500 | 0.3966 | 0.0625 | 0.1724 |
Jnh10-2SAT | 0.9286 | 0.9454 | 0.0014 | 0.0013 | 139.0 | 9.0 | 0.1750 | 0.6342 | 0.1625 | 0.5996 |
Jnh11-2SAT | 0.7000 | 0.7083 | 0.0039 | 0.0033 | 478.1 | 37.1 | 0.1250 | 0.3453 | 0.0875 | 0.2446 |
Jnh12-2SAT | 1 | 1 | 0 | 0 | 330.3 | 13.0 | 0.0875 | 0.2737 | 0.0875 | 0.2737 |
Jnh13-2SAT | 1 | 1 | 0 | 0 | 54.4 | 5.7 | 0.6500 | 1.1886 | 0.6500 | 1.1886 |
Jnh14-2SAT | 0.7143 | 0.7778 | 0.0034 | 0.0034 | 2179.9 | 45.7 | 0.0875 | 0.1042 | 0.0625 | 0.0810 |
Jnh15-2SAT | 0.1325 | 0.2571 | 0.0188 | 0.0153 | 3198.2 | 46.6 | 0.1625 | 0.8070 | 0.0125 | 0.2075 |
Jnh16-2SAT | 0.1250 | 0.1852 | 0.0200 | 0.0198 | 3393.1 | 145.7 | 0.1000 | 0.3508 | 0.0125 | 0.0650 |
Null Hypothesis (H0) | CPU time follows a normal distribution. | ||
Alternative Hypothesis (Ha) | CPU time does not follow a normal distribution. | ||
P | HNN-2SAT-WA | 0.0406 | Reject the null hypothesis, indicating that CPU time does not follow a normal distribution. |
HNN-2SAT-CSAFC | 0.0401 | Reject the null hypothesis, indicating that CPU time does not follow a normal distribution. |
Null Hypothesis (H0) | There is no significant difference in the median CPU times between the two models. | |
Alternative Hypothesis (Ha) | There is a significant difference in the median CPU times between the two models. | |
P | 0.0004 | Reject the null hypothesis, indicating that there is a significant difference in the median CPU times. |