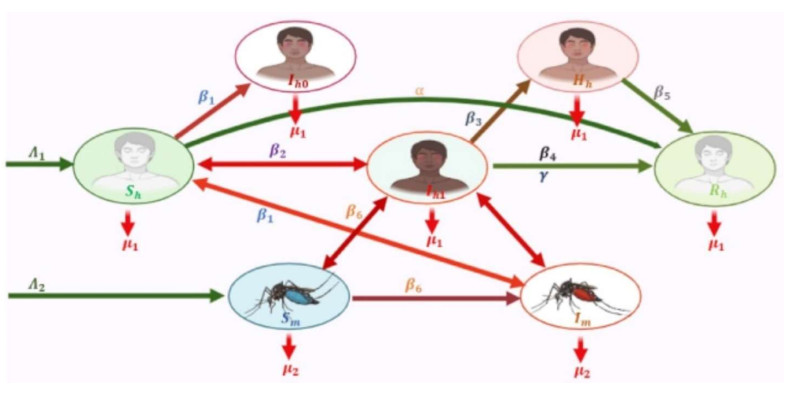
Dengue impacts 129 nations, threatens over 50% of the global population, and results in around 400 million illnesses annually. The purpose of this paper was to build the global stability and sensitivity analysis of a vector-host dengue mathematical model with compartments of symptomatic and hospitalized infected humans. Additionally, it aimed to assess the impact of the immunological response of vulnerable individuals, through the ingestion of natural foods, on the transmission of the disease. The solution's positivity and boundedness proved the model's mathematical well-posedness. To examine endemicity, the reproduction number was calculated using the next-generation technique. The Lyapunov function approach was employed to illustrate the model's global stability. Our mathematical discoveries were illustrated through numerical simulations of the dengue epidemic. The dynamical system sensitivity analysis suggests that the best way to control illness is to increase the immune system rate of susceptible hosts by consuming natural foods.
Citation: Turki D. Alharbi, Md Rifat Hasan. Global stability and sensitivity analysis of vector-host dengue mathematical model[J]. AIMS Mathematics, 2024, 9(11): 32797-32818. doi: 10.3934/math.20241569
[1] | Windarto, Muhammad Altaf Khan, Fatmawati . Parameter estimation and fractional derivatives of dengue transmission model. AIMS Mathematics, 2020, 5(3): 2758-2779. doi: 10.3934/math.2020178 |
[2] | Khaled A. Aldwoah, Mohammed A. Almalahi, Kamal Shah, Muath Awadalla, Ria H. Egami . Dynamics analysis of dengue fever model with harmonic mean type under fractal-fractional derivative. AIMS Mathematics, 2024, 9(6): 13894-13926. doi: 10.3934/math.2024676 |
[3] | Asma Hanif, Azhar Iqbal Kashif Butt . Atangana-Baleanu fractional dynamics of dengue fever with optimal control strategies. AIMS Mathematics, 2023, 8(7): 15499-15535. doi: 10.3934/math.2023791 |
[4] | Ahmed Alshehri, Miled El Hajji . Mathematical study for Zika virus transmission with general incidence rate. AIMS Mathematics, 2022, 7(4): 7117-7142. doi: 10.3934/math.2022397 |
[5] | Najat Almutairi, Sayed Saber, Hijaz Ahmad . The fractal-fractional Atangana-Baleanu operator for pneumonia disease: stability, statistical and numerical analyses. AIMS Mathematics, 2023, 8(12): 29382-29410. doi: 10.3934/math.20231504 |
[6] | Kottakkaran Sooppy Nisar, Aqeel Ahmad, Mustafa Inc, Muhammad Farman, Hadi Rezazadeh, Lanre Akinyemi, Muhammad Mannan Akram . Analysis of dengue transmission using fractional order scheme. AIMS Mathematics, 2022, 7(5): 8408-8429. doi: 10.3934/math.2022469 |
[7] | B. S. Alofi, S. A. Azoz . Stability of general pathogen dynamic models with two types of infectious transmission with immune impairment. AIMS Mathematics, 2021, 6(1): 114-140. doi: 10.3934/math.2021009 |
[8] | Saiwan Fatah, Arkan Mustafa, Shilan Amin . Predator and n-classes-of-prey model incorporating extended Holling type Ⅱ functional response for n different prey species. AIMS Mathematics, 2023, 8(3): 5779-5788. doi: 10.3934/math.2023291 |
[9] | Mamta Barik, Chetan Swarup, Teekam Singh, Sonali Habbi, Sudipa Chauhan . Dynamical analysis, optimal control and spatial pattern in an influenza model with adaptive immunity in two stratified population. AIMS Mathematics, 2022, 7(4): 4898-4935. doi: 10.3934/math.2022273 |
[10] | Muhammad Altaf Khan, Saif Ullah, Muhammad Farhan . The dynamics of Zika virus with Caputo fractional derivative. AIMS Mathematics, 2019, 4(1): 134-146. doi: 10.3934/Math.2019.1.134 |
Dengue impacts 129 nations, threatens over 50% of the global population, and results in around 400 million illnesses annually. The purpose of this paper was to build the global stability and sensitivity analysis of a vector-host dengue mathematical model with compartments of symptomatic and hospitalized infected humans. Additionally, it aimed to assess the impact of the immunological response of vulnerable individuals, through the ingestion of natural foods, on the transmission of the disease. The solution's positivity and boundedness proved the model's mathematical well-posedness. To examine endemicity, the reproduction number was calculated using the next-generation technique. The Lyapunov function approach was employed to illustrate the model's global stability. Our mathematical discoveries were illustrated through numerical simulations of the dengue epidemic. The dynamical system sensitivity analysis suggests that the best way to control illness is to increase the immune system rate of susceptible hosts by consuming natural foods.
Dengue fever, a highly transmissible illness, threatens more than two to three million individuals annually on a global scale [1,2]. Dengue, the second most lethal mosquito-borne illness after malaria, results in hundreds of fatalities and over 4 million infections each year [3]. More than 129 nations are home to it, and it can cause from mild to severe symptoms, especially in the tropics and subtropics [4,5]. About three quarters of all dengue cases are dengue fever (DF), the most prevalent type of disease. Five distinct serotypes of dengue virus define the viral infection known as dengue fever, which is transmitted by mosquitoes: DEN-1, DEN-2, DEN-3, DEN-4, and DEN-5. When mosquitoes bite an infected person, they take the virus and let it incubate for eight to ten days. Following a mosquito bite that carries an infection, patients often start symptoms for five to seven days after. DF symptoms include a high temperature, intense headaches, joints and muscles discomfort, and rashes [6,7].
People, insects, and the virus disseminate dengue in several environments. Due to space complexity, dengue transmission studies are complex [8]. The epidemic has many causes. Worldwide host and vector mobility boosted viral circulation, urban congestion encouraged multiple transmissions from a single infected vector, and vector control mechanisms were lost. Temperature, precipitation, and humidity affect vector development from egg viability to adult longevity and dispersal, among other dengue transmission factors [9]. Unplanned construction, high population density, and unstable trash collection promote mosquito breeding sites and dengue.
Dengue has no cure; thus, severe cases require hospitalization and supportive care [10]. A cheap, efficient vaccine is essential for universal control. Governments mostly use vector control and insect repellents to lower infection rates. Community education about dengue hazards is essential for prevention [11]. Media campaigns and community involvement may spread preventative knowledge. Community-government miscommunications can raise dengue risk, making ongoing community awareness crucial for successful interventions.
Mathematical models of infectious illness are valuable tools for comprehending and forecasting the patterns of outbreaks, comprehending the advancement and development of diseases, and estimating the consequences of health interventions on people or populations [12,13,14]. The models generated by mathematics can be used to improve tactics for reducing disease spread and enhance public health decisions with epidemiological evidence. Numerous authors have widely employed mathematical modeling to inform public health initiatives aimed at controlling the transmission of dengue [15,16]. The mathematical modeling approach is quite demanding due to the intricate nature of the dengue transmission mechanism. Utilizing a more intricate model can enhance the accuracy of the modeling process, but it also presents challenges when attempting to derive analytical data and draw conclusions. Therefore, the researcher must construct a practical yet uncomplicated model based on realistic assumptions. Utilizing actual incidence data is essential for accurately adjusting the model's performance. Various methodologies can be employed to build the dengue transmission model, including differential equations, fractional differential equations, stochastic differential equations, and alternative techniques. Rao et al. [17] introduced a variational model for delayed impulsive epidemic models, tackling mathematical challenges in the reaction-diffusion model with a delayed impulse. Yang et al. [18] investigated the presence of positive periodic solutions to neutral-type integral differential equations inside an epidemic model, employing Mawhin's continuation theorem and the characteristics of neutral-type operators. Zhao et al. [19] explored the stability of impulsive stochastic competition models with time-varying delays, focusing on persistence, extinction, and practical exponential stability. Xiao et al. [20] provided an innovative methodology for analyzing the dynamics of rumors inside social networks, differentiating between enthusiasts and ordinary individuals. Hattaf [21] presented a novel definition of fractional derivatives that integrates singular and non-singular kernels, along with an associated fractional integral, augmenting their utility in computational biology. Hattaf [22] introduced a novel class of generalized differential and integral operators that generalize the definitions of fractal-fractional derivatives and integral operators employed to model complex dynamics in various disciplines.
Many modeling analysis of viral illnesses like dengue, chikungunya, and zika have been conducted in the literature. Naaly et al. [23] studied how vector management, treatment, and mass awareness affect dengue fever transmission dynamics. The authors in [24] discovered awareness and control strategies for dengue disease transmission. Researchers Aldila et al. [25] looked into the interaction between social awareness, the detection of cases, and the capability of hospitals in Jakarta to eliminate dengue. Li et al. [26] analyzed the effects of awareness initiatives on dengue and identified the optimal control approaches. Bonyah et al. [27] performed an analysis of the fractional stochastic modeling of dengue illness, specifically focusing on the perspective of social awareness. The authors [28] conducted a mathematical analysis to investigate how community ignorance affects dengue population dynamics. Sood et al. [29] conducted an investigation on a sophisticated healthcare system designed to forecast and avert dengue virus illness. Macalalag et al. [30] analyzed the dengue model's global stability incorporating awareness and temporal delays. A dengue disease model was used by Saha et al. [31] to study the host-vector dynamics using the optimal control technique. The authors of [32] conducted a study on forecasting dengue epidemic outbreaks by utilizing climate variation and Monte Carlo techniques. Bhuju et al. [33] examined the fuzzy SEIR-SEI dengue disease model's sensitivity and bifurcation analysis. Harshit [34] conducted a sensitivity study to examine dengue transmission. Based on the sensitivity analysis of the dengue epidemic using a modified saturation incidence rate, the authors of [35] invented an optimal control strategy. Guo et al. [36] performed a stability analysis and simulation of a delayed dengue transmission model incorporating logistic growth and a nonlinear incidence rate. Leandro et al. [37] investigated the spatial distribution of dengue transmission in a city with a high incidence of the disease in Brazil, demonstrating significant spatial organization in the local dynamics of dengue transmission. Hasan et al. [38] performed a sensitivity analysis of a dynamic dengue epidemic model incorporating vector-host interactions. Abidemi et al. [39] performed a Lyapunov stability study and implemented optimization strategies for a model that simulates the transmission of dengue disease. The author of [40] conducted a thorough analysis of effective control measures for managing dengue infection in East Java. A study on the mathematical evaluation of the impact of hospitalization in dengue intervention was carried out by Nawawi et al. [41]. Researchers Hamdan et al. [42] used a case study of hospitalized infected individuals in Malaysia to determine the impact of temperature on creating a deterministic dengue epidemic model. The study by Abidemi et al. [43] offered valuable insights into the dynamics of dengue, with a specific focus on the asymptomatic, isolated, and vigilant compartments. Jan et al. [44] conducted a study on the transmission dynamics of dengue by asymptomatic carriers and the effectiveness of control strategies. A study by Jose et al. [45] used mathematical models to look into how the Zika virus and Dengue fever spread in people who show symptoms of both diseases.
All of these studies are predicated on deterministic models; however, none have examined the consequences of the infection rate within the host or the symptomatically infected and hospitalized humans in question. Furthermore, the immune system's enhancement through the consumption of natural foods and awareness of symptomatic human infections were not taken into account. In light of the aforementioned, this work presents a novel mathematical model that accurately captures the interactions between dengue hosts and vectors. The model includes compartments for infected individuals, individuals with symptoms, and individuals requiring hospitalization. This paradigm will help us to better understand the reality of dengue transmission, and eradication.
The following parts of this work are arranged as follows: Our model is presented in Section 2, together with a biological justification of its parameters. Section 3 is devoted to analyzing the positivity as well as boundedness of the model. In addition, we analyze the equilibrium points and compute the basic reproduction number, represented as R0. Section 4 discusses the stability of the model. Section 5 focuses on sensitivity analysis to illustrate the influence of the proposed model on R0. In Section 6, we introduce a computational method for solving the model. Furthermore, this section presents the numerical findings and their accompanying explanations. Ultimately, the concluding portion of the study provides a concise summary of the main contributions of our work and identifies prospective areas for further research.
The compartmental models provide a thorough description of how the epidemic spreads and the various preventive measures that can be taken to stop it. In order to create the epidemic model, we utilize the compartmental modeling approach. The model consists of seven compartments which represent two distinct sub-populations: the human (host) population and the vector (mosquito) population. The total host population, can be divided into five distinct groups: Sh represents susceptible humans who are capable of contracting the disease, Ih0 signifies infected humans who are not capable of transmitting the disease to others, Ih1 represents symptomatically infected humans who are able to transmit the disease to others, Hh implies hospitalized infected humans, and Rh reflects the recovered population who have temporary immunity against dengue. Similarly, the vector population Nm of mosquitoes is divided into two groups: mosquitoes capable of being infected (susceptible, Sm) and mosquitoes that are infected by the dengue virus (infected, Im). Figure 1 shows a transmission diagram illustrating compartment interactions, while Table 1 describes the parameters.
Parameter | Descriptions |
Λ1 | Human population recruitment rates |
β1 | Infection transmission rate from vector to host |
α | Rate of immune system of susceptible host by the consumption of natural foods |
β2 | Infections rate within the host |
μ1 | Human population natural mortality |
β3 | Rate of symptomatic to hospitalized infected individuals |
β4 | Symptomatic infection recovery rate |
γ | Awareness rate among symptomatic infected people |
β5 | Hospitalized infected individual's recovery rate |
Λ2 | Vector population recruitment rates |
β6 | Infection transmission rate from human to vector |
μ2 | Vector population natural mortality |
In order to construct the model, we take into account the rate of immune system of the susceptible host achieved by the consumption of natural foods, denoted by α. β1 is the infection transmission rate from vector to host, and the infection rate within hosts is β2. Additionally, the human awareness of individuals who are symptomatically infected (γ) has protected them from dengue by employing mosquito-nets and mosquito -repellent sprays. Also, the hospitalized infected humans Hh are introduced here.
The model is governed by the differential equations that are shown in the following system:
dShdt=Λ1−β1ShIm−β2ShIh1−αSh−μ1Sh,dIh0dt=β1ShIm−μ1Ih0,dIh1dt=β2ShIh1−β3Ih1−β4Ih1−γIh1−μ1Ih1,dHhdt=β3Ih1−β5Hh−μ1Hh,dRhdt=β4Ih1+β5Hh+αSh+γIh1−μ1Rh,dSmdt=Λ2−β6SmIh1−μ2Sm,dImdt=β6SmIh1−μ2Im, | (2.1) |
With initial host population Sh(0)≥0,Ih0(0)≥0,Ih1(0)≥0,Hh(0)≥0,andRh(0)≥0, and the vector population Sm(0)≥0,andIm(0)≥0.
A fundamental need for an epidemiological model is that its solutions must exhibit both non-negativity and boundedness. Therefore, it is essential to demonstrate that all variables remain positive for every instance where t is greater than zero.
Theorem 3.1. The system (2.1)'s feasible region stated as
χ={Sh(t),Ih0(t),Ih1(t),Hh(t),Rh(t),Sm(t),Im(t)ϵR+7:Nh(t)≤Λ1μ1,Nm(t)≤Λ2μ2} |
is positively invariant along with the initial condition defined by R+7.
Proof. One way to express system (1) is :
dYdt=K(Y)+Z, | (3.1) |
Y=(Sh,Ih0,Ih1,Hh,Rh,Sm,Im)t, |
K=(−k1000000k2−μ100000k30−k4000000k5−k6000k70k8k9−μ10000000−k10000000k11−μ2), |
where, k1=β1Im+β2Ih1+α+μ1, k2=β1ShIm, k3=β3Ih1, k4=β3+β4+γ+μ1, k5=β3Ih1, k6=β5+μ1, k7=α, k8=β4+γ, k9=β5, k10=β6Ih1+μ2, k11=β4Ih1.
And
Z=(Λ1,0,0,0,0,Λ2,0)t. |
In this case, any entry of the matrix K(Y) off-diagonal is non-negative. Therefore, the matrix is referred to as the Metzler matrix. Furthermore, vector Z possesses a positive character. Consequently, it may be deduced that the system (3.1) is always positively invariant in the region R+7.
Setting each system of model (2.1) to zero, results in the production of the dengue-free equilibrium (DFE). Additionally, the DFE has no infections or recovery procedures in place. Therefore, the DFE of the dengue model is provided by
E0=(S0h,I0h0,I0h1,H0h,R0h,S0m,I0m)=(Λ1μ1+α,0,0,0,0,Λ2μ2,0). |
The basic reproduction number is a critical threshold in mathematical epidemiology research since it helps predict the possibility of disease transmission. Utilizing the next-generation matrix to calculate the system (1)'s R0 as
F=(00β1S0h0β2S0h00β6S0m0),V=(μ1000β3+β4+μ1+γ000μ2). |
The next-generation matrix FV−1 generates R0 as
R0=β2Λ1(μ1+α)(β3+β4+μ1+γ). |
The endemic equilibrium of system (2.1) is
E1=(S∗h,I∗h0,I∗h1,H∗h,R∗h,S∗m,I∗m) |
where,
S∗h=β3+β4+μ1β2,I∗h0=β1β6Λ2(β3+β4+μ1+γ)I∗h1μ1μ2(β6+μ2), |
I∗h1=(μ1+α)μ2(β5+μ1)(β6+μ2)(R0−1)β1β6Λ2+β2μ2(β6+μ2),H∗h=β3I∗h1β5+μ1, |
R∗h=β2(β4+γ)(β5+μ1)+β2β3β5)I∗h1+α(β5+μ1)(β3+β4+μ1)μ1β2(β5+μ1), |
S∗m=Λ2β6I∗h1+μ2,I∗m=Λ2β6I∗h1μ2(β6+μ2). |
The endemic equilibria exist if R0>1.
Theorem 4.1.1. System (2.1)'s DFE (E0) is locally asymptotically stable if R0<1 and unstable if R0>1.
Proof. The Jacobian matrix at the dengue-free equilibrium point (E0) is
J(E0)=(−(μ1+α)0−β2S0h000−β1S0h0−μ10000β1S0h00β2S0h−(β3+β4+μ1+γ)000000β3−β5−μ1000α0β4+γβ5−μ10000−β6S0m00−μ2000β6S0m000−μ2). |
The eigenvalues for the matrix J(E0) are −μ1(multiplicity 2), −μ2(multiplicity 2), −(μ1+α), −(β5+μ1), and β2S0h−(β3+β4+μ1+γ). An obvious negative sign appears in the first six eigenvalues. As this is the case, the DFE E0 is locally asymptotically stable if
β2S0h−(β3+β4+μ1+γ)<0, |
β2S0h<(β3+β4+μ1+γ), |
β2S0h(β3+β4+μ1+γ)<1, |
β2Λ1(μ1+α)(β3+β4+μ1+γ)<1, |
R0<1. |
So, E0 is locally asymptotically stable if R0<1, otherwise; it is unstable.
Theorem 4.1.2. System (2.1)'s E1 is locally asymptotically stable if R0>1.
Proof. The Jacobian matrix at the endemic equilibrium point (E1) is
J(E1)=(−ϕ10−β2S∗h000−β1S∗hβ1I∗m−μ10000β1S∗hβ2I∗h10−ϕ2000000β3−β5−μ100000β4+γβ5−μ10000−ϕ300−ϕ4−μ2000ϕ300ϕ4−μ2), |
where, ϕ1=β1I∗m+β2I∗h1+α+μ1, ϕ2=β3+β4+γ+μ1−β2S∗h, ϕ3=β6S∗m, ϕ4=β16I∗h1.
Four eigenvalues of the above matrix are −μ1, −μ1, -μ2, −(β5+μ1), and the other roots may be found by using the cubic equation
λ3+ϵ1λ2+ϵ2λ+ϵ3=0, | (4.1) |
where,
ϵ1=ϕ1+ϕ2+ϕ4+μ2, |
ϵ2=β22I∗h1S∗h+ϕ1μ2+ϕ2μ2+ϕ1ϕ2μ2+ϕ1ϕ2+ϕ1ϕ4+ϕ2ϕ4, |
ϵ3=ϕ1ϕ2ϕ4+μ2β22I∗h1S∗h+ϕ3β1β2I∗h1S∗h. |
Here, ϵ1>0, ϵ3>0, ϵ1ϵ2−ϵ3>0.
For this
ϵ1=ϕ1+ϕ2+ϕ4+μ2=β1I∗m+β2I∗h1+α+μ1+β3+β4+γ+μ1−β2S∗h+β6I∗h1+μ2=Λ2β1β6(μ1+α)μ2(β5+μ1)(β6+μ2)(R0−1)μ2(β6+μ2)β1β6Λ2+β2μ2(β6+μ2)+β2(μ1+α)μ2(β5+μ1)(β6+μ2)(R0−1)β1β6Λ2+β2μ2(β6+μ2)+β6(μ1+α)μ2(β5+μ1)(β6+μ2)(R0−1)β1β6Λ2+β2μ2(β6+μ2)+μ1+α+γ+μ2>0, |
if R0>1.
Hence, the Routh-Hurwitz criterion ϵ1>0, ϵ3>0, ϵ1ϵ2−ϵ3>0 ensues the local asymptotically stability for R0>1.
Lemma 4.2.1. The region ω1={Xϵω:Sh≤S0h,Sm≤S0m} is a positively invariant for the model (1), where X={Sh,Ih0,Ih1,Hh,Rh,Sm,Im}.
Proof. Using the model's first equation, we obtain
dShdt=Λ1−β1ShIm−β2ShIh1−αSh−μ1Sh≤Λ1−αSh−μ1Sh≤(μ1+α)(Λ1(μ1+α)−Sh)≤(μ1+α)(S0h−Sh). |
Implying that, Sh≤S0h−(S0h−Sh(0))e−(μ1+α)t. Thus Sh(t)≤S0h for all t≥0.
Using the model's fifth equation, we obtain
dSmdt=Λ2−β6SmIh1−μ2Sm≤Λ2−μ2Sm≤μ2(Λ2μ2−Sm). |
Implying that, Sm≤S0m−(S0m−Sm(0))e−μ2t. Thus Sm(t)≤S0m for all t≥0.
In summary, we conclude that the ω1 is positively invariant.
Theorem 4.2.1. The vector-host model may be expressed generally as
dϰ1dt=F(ϰ1,ϰ2),dϰ2dt=G(ϰ1,ϰ2),G(ϰ1,0)=0. | (4.2) |
Where, ϰ1=(Sh,Rh,Sm)t,andϰ2=(Ih0,Ih,Hh,Im)t represent the individuals who are infected and those who are not. Then, the DFE is now represented by the following:
E0=(ϰ01,0)=(Λ1(μ1+α),μ1(μ1+α)αΛ1,Λ2μ2,0,0,0,0). |
The following conditions must be met in order for E0 to maintain global asymptomatic stability:
H1:dϰ1dt=F(ϰ01,0),ϰ01 is global asymptomatic stable, H2: ˆG(ϰ1,ϰ2)=Aϰ2−G(ϰ1,ϰ2),ˆG(ϰ1,ϰ2)≥0, where A=Dϰ2F(ϰ01,0) is Metzler matrix.
Theorem 4.2.2. If the criteria of Eq (4.2) are met and R0<1, E0=(ϰ01,0) is a global asymptomatic stable.
Proof. Applying the theorem (4.1.2)
F(ϰ01,0)=Λ1−αSh−μ1Sh,ˆG(ϰ1,ϰ2)=Aϰ2−G(ϰ1,ϰ2). |
Where,
A=[−μ100β1S0h0β2S0h−β3+β4+γ+μ1000β3−(β5+μ1)00β6S0m0−μ2]. |
Now,
ˆG(ϰ1,ϰ2)=Aϰ2−G(ϰ1,ϰ2)=[β1Im(S0h−Sh)β2Ih1(S0h−Sh)0β6Ih1(S0m−Sm)]=[ˆG1ˆG20ˆG3]. |
Clearly, ˆG1≥0,ˆG2≥0andˆG3≥0, since Sh≤S0h,Sm≤S0m. Therefore, ˆG(ϰ1,ϰ2)≥0.
Also, A is Metzler matrix. Hence, the dengue-free equilibrium E0 is global asymptotical stable.
Theorem 4.2.3. System (2.1)'s E1 is globally asymptotically stable if R0>1.
Proof. The form's Lyapunov function in
W(t)=12(Sh−S∗h)2+12(Ih0−I∗h0)2+12(Ih1−I∗h1)2+12(Hh−H∗h)2+12(Rh−R∗h)2+12(Sm−S∗m)2+12(Im−I∗m)2. |
Differentiating with respect to time t, we get:
W'(t)=(Sh−S∗h)S'h+(Ih0−I∗h0)I'h0+(Ih1−I∗h1)I'h1+(Hh−H∗h)H'h+(Rh−R∗h)R'h+(Sm−S∗m)S'm+(Im−I∗m)I'm=(Sh−S∗h)(Λ1−β1ShIm−β2ShIh1−αSh−μ1Sh)+(Ih0−I∗h0)(β1ShIm−μ1Ih0)+(Ih1−I∗h1)(β2ShIh1−β3Ih1−β4Ih1−γIh1−μ1Ih1)+(Hh−H∗h)(β3Ih1−β5Hh−μ1Hh)+(Rh−R∗h)(β4Ih1+β5Hh+αSh+γIh1−μ1Rh)+(Sm−S∗m)(Λ2−β6SmIh1−μ2Sm)+(Im−I∗m)(β6SmIh1−μ2Im). |
Using the equilibrium conditions Λ1=μ1S∗h+μ1I∗h0+μ1I∗h1+μ1H∗h+μ1R∗h and Λ2=μ2S∗m+μ2I∗m into the above equation
W'(t)=(Sh−S∗h)(μ1S∗h+μ1I∗h0+μ1I∗h1+μ1H∗h+μ1R∗h)−(Sh−S∗h)(β1ShIm+β2ShIh1+αSh+μ1Sh)+(Ih0−I∗h0)(β1ShIm−μ1Ih0)+(Ih1−I∗h1)(β2ShIh1−β3Ih1−β4Ih1−γIh1−μ1Ih1)+(Hh−H∗h)(β3Ih1−β5Hh−μ1Hh)+(Rh−R∗h)(β4Ih1+β5Hh+αSh+γIh1−μ1Rh)+(Sm−S∗m)(μ2S∗m+μ2I∗m−β6SmIh1−μ2Sm)+(Im−I∗m)(β6SmIh1−μ2Im)=−μ1(Sh−S∗h)2+μ1I∗h0(Sh−S∗h)+μ1I∗h1(Sh−S∗h)−β2ShI∗h1(Sh−S∗h)+μ1H∗h(Sh−S∗h)+μ1R∗h(Sh−S∗h)−β1ShIm(Sh−S∗h)−αSh(Sh−S∗h)+β1ShIm(Ih0−I∗h0)−μ1Ih0(Ih0−I∗h0)+β2ShIh1(Ih1−I∗h1)−γIh1(Ih1−I∗h1)−(β3+β4)Ih1(Ih1−I∗h1)−μ1Ih1(Ih1−I∗h1)+β3Ih1(Hh−H∗h)−μ1Hh(Hh−H∗h)+β4Ih1(Rh−R∗h)+β5Hh(Rh−R∗h)+αSh(Rh−R∗h)+γIh1(Rh−R∗h)−μ1Rh(Rh−R∗h)−μ2(Sm−S∗m)2+μ2I∗m(Sm−S∗m)−β6SmIh1(Sm−S∗m)+β6SmIh1(Im−I∗m)−μ2Sm(Im−I∗m)=−μ1(Sh−S∗h)2−μ1{Ih0(Ih0−I∗h0)−I∗h0(Sh−S∗h)}−β2Sh{I∗h1(Sh−S∗h)−Ih1(Ih1−I∗h1)}−μ1{Ih1(Ih1−I∗h1)−I∗h1(Sh−S∗h)}−μ1{Hh(Hh−H∗h)−H∗h(Sh−S∗h)}−αSh(Sh−S∗h−Rh+R∗h)−γIh1(Ih1−I∗h1−Rh+R∗h)−β1ShIm(Sh−S∗h−Ih0+I∗h0)−β3Ih1(Ih1−I∗h1−Hh+H∗h)−β4Ih1(Ih1−I∗h1−Rh+R∗h)−β5Hh(Hh−H∗h−Rh+R∗h)−μ1{Rh(Rh−R∗h)−R∗h(Sh−S∗h)}−μ2(Sm−S∗m)2−μ2{(Im−I∗m)Sm−I∗m(Sm−S∗m)}−β6SmIh1(Sm−S∗m−Im+I∗m). |
Now, W'(t)≤0and W'(t)=0 for
Sh=S∗h,Ih0=I∗h0,Ih1=I∗h1,Hh=H∗h,Rh=R∗h,Sm=S∗m,,Im=I∗m. |
So, the largest invariance set is the singleton set {E1}. Therefore, using the principle of LaSalle's invariance, the endemic equilibrium E1 is globally asymptotically stable.
To mitigate and manage dengue outbreaks, a sensitivity analysis is performed to pinpoint the factors that exert the most significant influence on the transmission and dissemination of dengue within the population, as outlined in the model (2.1), employing the same methodology as previous studies [46,47,48]. The normalized forward sensitivity index of R0 relates to the system (2.1) parameter ϑ, which is signified by ζϑR0=∂R0∂ϑ.ϑR0.
Table 2 and Figure 2 display the dengue fever model's sensitivity index in relation to R0. When an index has a positive sign, R0 increases; when it has a negative sign, R0 declines. If the other parameters are maintained constantly and the negative indices (α,μ1,γ,β3,β4) are increased, the value of R0 falls, suggesting a reduction in the rate of disease transmission. Conversely, if all other parameters remain constant and the indices with positive values (Λ1,β2) are raised, R0 will also rise, resulting in a higher rate of disease transmission among humans. Furthermore, the sensitivity index shows that the infectious rate within the host has the highest positive value (+1), suggesting that changes in the host's infectious rate will affect R0. In addition, the negative sensitivity index of -0.99324 for the rate of immune system of susceptible host by consumption of natural foods (α) indicates that a rise in α leads to a reduction in R0 and vice versa. So, the best control approach to keep diseases under control is to increase the immune system rate of susceptible hosts by eating natural foods.
Parameter | Sensitivity index |
Λ1 | +1.0 |
β2 | +1.0 |
α | -0.993245927 |
μ1 | -0.0084993573 |
γ | -0.3573103612 |
β3 | -0.2793059925 |
β4 | -0.3616383535 |
Figure 3–6 display the R0 of the proposed system as it varies with different input parameters. Visualizing these parameters' effect on the outcomes of R0 is the primary goal of this endeavor. Dengue fever in the population decreases as the rate of the immune system in the susceptible host grows due to the consumption of natural foods (α), while the rate of infection within the host (β2) remains constant (refer to Figure 3). It is indeed possible to take R0 to a satisfactory level of R0<1 by enhancing the immune system of susceptible hosts by consuming natural foods, with an α value of approximately 0.42.
Figures 4–6 demonstrate that while the infectious disease rate (β2) stays constant within the host, there is noticeable variation in the awareness rate among symptomatic infected humans (γ), the rate of symptomatic to hospitalized infected (β3), and symptomatic infection recovery rate (β4). The results indicate that γ and β3 have small effects on R0, but the symptomatic infection recovery rate has a larger impact on R0(Figure 6).
To demonstrate the findings of this study, the model's numerical simulations are performed using the parameter values specified in Table 3. The simulation visually represents the quantities of susceptible, infected, symptomatic infected, and hospitalized infected carriers within the host population, as well as the quantities of susceptible and infected people within the vector population.
Parameter | Values | Reference |
Λ1 | .9999 | [49] |
β1 | .8500 | Assumed |
β2 | .6294 | [50] |
α | .2520 | Assumed |
μ1 | .003468 | [51] |
β3 | .555 | [52] |
β4 | .7186 | [53] |
γ | .111 | [54] |
β5 | .0062 | Assumed |
Λ2 | .00034 | [55] |
β6 | .009 | [56] |
μ2 | .000244 | Assumed |
Figure 7 shows the dynamic behavior of an epidemic model over 150 days, illustrating the impact of fluctuations in the parameter α: the rate of the immune system of susceptible hosts by consumption of natural foods. Figure 7 shows the susceptible human (Sh), symptomatically infected human (Ih1), recovered human (Rh), and total infected population (Ih0+Ih1+Hh) under three conditions: the original α(green), a 10% increase in α(red), and a 10% decrease in α(blue). The susceptible human (Sh) experiences rapid decline and stabilization, with α influencing the initial peak and pace of stabilization (Figure 7(a)). The symptomatically infected human (Ih1) shows a dramatic initial surge followed by a steady reduction, with greater peaks and faster falls found with increased α (Figure 7(b)). Increased α leads to a fast increase in the recovered human (Rh) in Figure 7(c), before settling at higher values. In Figure 7(d), the overall infected human follows a similar trend of rapid rise and stabilization, with higher and faster stabilization found with a 10% increase in α levels. Increasing α intensifies and shortens the epidemic, whereas decreasing α leads to a less severe but longer epidemic course.
Figure 8 displays four graphs illustrating the impacts of altering the parameter β3 on an epidemic model over a span of 150 days. The figure illustrates the fluctuations in the susceptible human population (Sh), hospitalized infected human population (Hh), infected vector population (Im), and the total population (Sh+Ih0+Ih+Hh) across three scenarios: the initial β3(green), a 10% rise in β3(red), and a 10% drop in β3(blue). The population of individuals susceptible to the infection (Sh) initially drops and then reaches a stable state. This is due to a decrease in the transmission rate β3, which results in a smaller peak in infections and a faster population stability. The population of infected individuals who are hospitalized (Hh) exhibits a notable peak followed by a steady fall, which is caused by a reduced β3 resulting in a more significant peak and longer duration of hospitalization. The population of infected vectors (Im), diminishes with time, with a reduced β3 leading to a more significant initial value and a slower rate of decline. The aggregate population (Sh+Ih0+Ih+Hh) experiences a significant increase followed by a period of stability, with negligible influence from changes in β3 on the overall pattern. Overall, a reduction in β3 exacerbates and lengthens the epidemic, while an augmentation in β3 alleviates its severity and duration.
Figure 9 illustrates the impact of changing the parameter β5 on the number of hospitalized infected and recovered individuals over a period of time, measured in days. The initial β5(green) of the hospitalized infected exhibits a fast ascent, reaching its maximum around day 40, and subsequently undergoes a slow decrease (Figure 9(a)). An increment of 10% in the value of β5(red) leads to a decrease in the highest point and a slightly swifter decrease. A reduction of 10% in the value of β5 (blue) results in an increased peak and a longer duration of hospitalization. In Figure 9(b), the population of the original β5(green) strain has rapid growth, reaching its highest point around day 50, and then remains stable. A 10% augmentation in the value of β5(red) leads to a marginally elevated peak and a faster attainment of stability. A reduction of 10% in the value of β5(blue) results in a marginally diminished highest point and a delayed attainment of stability. In general, raising the value of β5 has the tendency to decrease the maximum number of patients requiring hospitalization and speed up the process of recovery, leading to a less severe outbreak.
This study focuses on developing and examining a nonlinear mathematical model that represents the dynamics of dengue. The model considers different compartments for infected people, individuals with symptoms, and individuals requiring hospitalization. We took a look at the suggested model's fundamental characteristics. The model equilibria were used to produce the control reproduction number, the most essential mathematical quantity with major public health relevance. The results of the model's stability tests were presented. The application of the Jacobian matrix method reveals that the local asymptotic stability of the disease-free steady-state is disrupted. Numerical simulation using several parameter configurations demonstrated the evolution of epidemics, the dynamics of the system, and validated theoretical findings. The numerical simulations illustrate the impact of parameters such as the rate of immune system of susceptible hosts by consuming natural foods, the rate of symptomatic infection to hospitalized infected humans, and the recovery rate of hospitalized infected humans. We also conducted a sensitivity study to evaluate the impact of model parameters on the dynamics and management of the disease. We examined the impact of various parameter rates in order to determine the extent to which this variance influences the epidemic trajectory. The research findings indicate that raising the rate of α has the most significant impact on reducing the reproduction number, with no other parameter having a comparable effect on reducing infection. An investigation of this nature can provide crucial information for policymakers and health experts who may be faced with the harsh reality of infectious diseases.
All authors contributed equal significant contributions to the study's concept, design, methodology, validation, and analysis. All authors have read and approved the final version of the manuscript for publication.
The authors declare that the research was conducted in the absence of any conflict of interest.
[1] |
O. C. Akinsulie, I. Idris, Global re-emergence of dengue fever: The need for a rapid response and surveillance, The Microbe, 4 (2024), 100107. https://doi.org/10.1016/j.microb.2024.100107 doi: 10.1016/j.microb.2024.100107
![]() |
[2] |
R. K. Mohapatra, P. Bhattacharjee, D. N. Desai, V. Kandi, A. K. Sarangi, S. Mishra, et al., Global health concern on the rising dengue and chikungunya cases in the American regions: countermeasures and preparedness, Health Sci. Rep., 7 (2024), e1831. https://doi.org/10.1002/hsr2.1831 doi: 10.1002/hsr2.1831
![]() |
[3] |
L. AL-Eitan, M. Alnemri, H. Ali, M. Alkhawaldeh, A. Mihyar, Mosquito-borne diseases: Assessing risk and strategies to control their spread in the Middle East, J Biosaf. Biosecur., 6 (2024), 1–12. https://doi.org/10.1016/j.jobb.2023.12.003 doi: 10.1016/j.jobb.2023.12.003
![]() |
[4] |
J. Intirach, C. Shu, X. Lv, S. Gao, N. Sutthanont, T. Chen, et al., Human parasitic infections of the class Adenophorea: global epidemiology, pathogenesis, prevention and control, Infect. Dis. Poverty, 13 (2024), 48. https://doi.org/10.1186/s40249-024-01216-1 doi: 10.1186/s40249-024-01216-1
![]() |
[5] |
K. Hushmandi, S. H. Saadat, S. Bokaie, H. Akbarein, S. Daneshi, M. Najafi, et al., An overview of dengue fever, Arch. Adv. Biosci., 15 (2024), 1–24. https://doi.org/10.22037/aab.v15i1.44108 doi: 10.22037/aab.v15i1.44108
![]() |
[6] | S. Kumar, D. Kaushik, S. K. Sharma, Autoimmune disorders: Types, symptoms, and risk factors, In: Artificial intelligence and autoimmune diseases, Singapore: Springer, 2024. https://doi.org/10.1007/978-981-99-9029-0_1 |
[7] | D. Vilar-Compte, P. Cornejo-Juárez, E. S. Rojas, Chapter 7-Fever and rash, A Rational Approach to Clinical Infectious Diseases, 2022, 1–26. https://doi.org/10.1016/b978-0-323-69578-7.00007-7 |
[8] |
J. Lourenço, W. Tennant, N. R. Faria, A. Walker, S. Gupta, M. Recker, Challenges in dengue research: A computational perspective, Evol. Appl., 11 (2018), 516–533. https://doi.org/10.1111/eva.12554 doi: 10.1111/eva.12554
![]() |
[9] |
P. Prasad, S. K. Gupta, K. K. Mahto, G. Kumar, A. Rani, I. Velan, et al., Influence of climatic factors on the life stages of Aedes mosquitoes and vectorial transmission: A review, J. Vector Borne Dis., 61 (2024), 158–166. https://doi.org/10.4103/jvbd.jvbd_42_24 doi: 10.4103/jvbd.jvbd_42_24
![]() |
[10] |
M. J. Pajor, B. Long, S. Y. Liang, Dengue: A focused review for the emergency clinician, Am. J. Emerg. Med., 82 (2024), 82–87. https://doi.org/10.1016/j.ajem.2024.05.022 doi: 10.1016/j.ajem.2024.05.022
![]() |
[11] |
C. Naing, W. Y. Ren, C. Y. Man, K. P. Fern, Q. Chua, C. N. Ning, et al., Awareness of dengue and practice of dengue control among the semi-urban community: A cross sectional survey, J. Community Health, 36 (2011), 1044–1049. https://doi.org/10.1007/s10900-011-9407-1 doi: 10.1007/s10900-011-9407-1
![]() |
[12] |
C. I. Siettos, L. Russo, Mathematical modeling of infectious disease dynamics, Virulence, 4 (2013), 295–306. https://doi.org/10.4161/viru.24041 doi: 10.4161/viru.24041
![]() |
[13] |
M. R. Hasan, A. A. Alsaiari, B. Z. Fakhurji, M. H. R. Molla, A. H. Asseri, M. A. A. Sumon, et al., Application of mathematical modeling and computational tools in the modern drug design and development process, Molecules, 27 (2022), 4169. https://doi.org/10.3390/molecules27134169 doi: 10.3390/molecules27134169
![]() |
[14] |
A. S. Mata, S. M. P. Dourado, Mathematical modeling applied to epidemics: an overview, Sao Paulo J. Math. Sci., 15 (2021), 1025–1044. https://doi.org/10.1007/s40863-021-00268-7 doi: 10.1007/s40863-021-00268-7
![]() |
[15] |
S. A. Carvalho, S. O. da Silva, I. da C. Charret, Mathematical modeling of dengue epidemic: control methods and vaccination strategies, Theory Biosci., 138 (2019), 223–239. https://doi.org/10.1007/s12064-019-00273-7 doi: 10.1007/s12064-019-00273-7
![]() |
[16] | M. R. Hasan, A. Hobiny, A. Alshehri, Dynamic vector-host dengue epidemic model with vector control and sensitivity analysis, Adv. Dyn. Syst. Appl., 18 (2023), 1–21. |
[17] |
R. Rao, Z. Lin, X. Ai, J. Wu, Synchronization of epidemic systems with Neumann boundary value under delayed impulse, Mathematics, 10 (2022), 2064. https://doi.org/10.3390/math10122064 doi: 10.3390/math10122064
![]() |
[18] |
Q. Yang, X. Wang, X. Cheng, B. Du, Y. Zhao, Positive periodic solution for neutral-type integral differential equation arising in epidemic model, Mathematics, 11 (2023), 2701. https://doi.org/10.3390/math11122701 doi: 10.3390/math11122701
![]() |
[19] |
Y. Zhao, H. Lin, X. Qiao, Y. Zhao, H. Lin, X. Qiao, Persistence, extinction and practical exponential stability of impulsive stochastic competition models with varying delays, AIMS Math., 8 (2023), 22643–22661. https://doi.org/10.3934/math.20231152 doi: 10.3934/math.20231152
![]() |
[20] |
H. Xiao, Z. Li, Y. Zhang, H. Lin, Y. Zhao, A dual rumor spreading model with consideration of fans versus ordinary people, Mathematics, 11 (2023), 2958. https://doi.org/10.3390/math11132958 doi: 10.3390/math11132958
![]() |
[21] |
K. Hattaf, A new mixed fractional derivative with applications in computational biology, Computation, 12 (2024), 7. https://doi.org/10.3390/computation12010007 doi: 10.3390/computation12010007
![]() |
[22] |
K. Hattaf, A new class of generalized fractal and fractal-fractional derivatives with non-singular kernels, Fractal Fract., 7 (2023), 395. https://doi.org/10.3390/fractalfract7050395 doi: 10.3390/fractalfract7050395
![]() |
[23] |
B. Z. Naaly, T. Marijani, A. Isdory, J. Z. Ndendya, Mathematical modeling of the effects of vector control, treatment and mass awareness on the transmission dynamics of dengue fever, Comput. Method. Prog. Bio. Update, 6 (2024), 100159. https://doi.org/10.1016/j.cmpbup.2024.100159 doi: 10.1016/j.cmpbup.2024.100159
![]() |
[24] | A. Jana, S. K. Roy, M. H. A. Biswas, Transmission dynamics of dengue disease, awareness and control strategies, Int. J. Model. Simul., 2024. https://doi.org/10.1080/02286203.2024.2334979 |
[25] |
D. Aldila, M. Z. Ndii, N. Anggriani, Windarto, H. Tasman, B. D. Handari, Impact of social awareness, case detection, and hospital capacity on dengue eradication in Jakarta: A mathematical model approach, Alex. Eng. J., 64 (2023), 691–707. https://doi.org/10.1016/j.aej.2022.11.032 doi: 10.1016/j.aej.2022.11.032
![]() |
[26] |
J. Li, H. Wan, M. Sun, Modeling the impact of awareness programs on the transmission dynamics of dengue and optimal control, Int. J. Biomath., 16 (2023), 2250072. https://doi.org/10.1142/s1793524522500723 doi: 10.1142/s1793524522500723
![]() |
[27] |
E. Bonyah, Y. Yuan, S. Mangal, Fractional stochastic modelling of dengue fever: The social awareness perspective, Sci. Afr., 22 (2023), e01966. https://doi.org/10.1016/j.sciaf.2023.e01966 doi: 10.1016/j.sciaf.2023.e01966
![]() |
[28] |
D. Aldila, C. Aulia Puspadani, R. Rusin, Mathematical analysis of the impact of community ignorance on the population dynamics of dengue, Fron.t Appl. Math. Stat., 9 (2023), 1094971. https://doi.org/10.3389/fams.2023.1094971 doi: 10.3389/fams.2023.1094971
![]() |
[29] |
S. K. Sood, V. Sood, I. Mahajan, Sahil, An intelligent healthcare system for predicting and preventing dengue virus infection, Computing, 105 (2023), 617–655. https://doi.org/10.1007/s00607-020-00877-8/figures/9 doi: 10.1007/s00607-020-00877-8/figures/9
![]() |
[30] | J. M. R. Macalalag, T. R. Y. Teng, E. P. de Lara-Tuprio, Global stability analysis of dengue model with awareness, In: Towards intelligent systems modeling and simulation, Cham: Springer, 2022. https://doi.org/10.1007/978-3-030-79606-8_14 |
[31] |
S. Saha, G. Samanta, Analysis of a host-vector dynamics of a dengue disease model with optimal vector control strategy, Math. Comput. Simul., 195 (2022), 31–55. https://doi.org/10.1016/j.matcom.2021.12.021 doi: 10.1016/j.matcom.2021.12.021
![]() |
[32] |
T. K. Martheswaran, H. Hamdi, A. Al-Barty, A. A. Zaid, B. Das, Prediction of dengue fever outbreaks using climate variability and Markov chain Monte Carlo techniques in a stochastic susceptible-infected-removed model, Sci. Rep., 12 (2022, 5459. https://doi.org/10.1038/s41598-022-09489-y doi: 10.1038/s41598-022-09489-y
![]() |
[33] |
G. Bhuju, G. R. Phaijoo, D. B. Gurung, Sensitivity and bifurcation analysis of fuzzy SEIR-SEI dengue disease model, J. Math., 2022 (2022), 1927434. https://doi.org/10.1155/2022/1927434 doi: 10.1155/2022/1927434
![]() |
[34] |
Harshit, P. Harjule, Mathematical modelling and analysis of dengue transmission dynamics, Procedia Comput. Sci., 235 (2024), 539–548. https://doi.org/10.1016/j.procs.2024.04.053 doi: 10.1016/j.procs.2024.04.053
![]() |
[35] | S. Vaidya, Dr. A. Saraf, An optimal control with sensitivity analysis of dengue endemic using modified saturated incidence rate. https://doi.org/10.2139/ssrn.4664459 |
[36] |
F. Guo, X. Tian, Stability analysis and simulation of a delayed dengue transmission model with logistic growth and nonlinear incidence rate, Int. J. Bifurcat. Chaos, 34 (2024), 2450028. https://doi.org/10.1142/s0218127424500287 doi: 10.1142/s0218127424500287
![]() |
[37] |
A. S. Leandro, W. A. Chiba de Castro, M. V. Garey, R. Maciel-de-Freitas, Spatial analysis of dengue transmission in an endemic city in Brazil reveals high spatial structuring on local dengue transmission dynamics, Sci. Rep., 14 (2024), 8930. https://doi.org/10.1038/s41598-024-59537-y doi: 10.1038/s41598-024-59537-y
![]() |
[38] |
Md. R. Hasan, A. Hobiny, A. Alshehri, Sensitivity analysis of vector-host dynamic dengue epidemic model, Commun. Math. Appl., 14 (2023), 1001–1017. https://doi.org/10.26713/cma.v14i2.2119 doi: 10.26713/cma.v14i2.2119
![]() |
[39] |
A. Abidemi, J. Ackora-Prah, H.O. Fatoyinbo, J. K. K. Asamoah, Lyapunov stability analysis and optimization measures for a dengue disease transmission model, Phy. A, 602 (2022), 127646. https://doi.org/10.1016/j.physa.2022.127646 doi: 10.1016/j.physa.2022.127646
![]() |
[40] |
M. A. Khan, Fatmawati, Dengue infection modeling and its optimal control analysis in East Java, Indonesia, Heliyon, 7 (2021), e06023. https://doi.org/10.1016/j.heliyon.2021.e06023 doi: 10.1016/j.heliyon.2021.e06023
![]() |
[41] |
J. Nawawi, D. Aldila, Mathematical assessment on the effect of hospitalization in dengue intervention, AIP Conf. Proc., 2242 (2020), 030002. https://doi.org/10.1063/5.0012142 doi: 10.1063/5.0012142
![]() |
[42] |
N. 'Izzati Hamdan, A. Kilicman, The development of a deterministic dengue epidemic model with the influence of temperature: A case study in Malaysia, Appl. Math. Model., 90 (2021), 547–567. https://doi.org/10.1016/j.apm.2020.08.069 doi: 10.1016/j.apm.2020.08.069
![]() |
[43] |
A. Abidemi, O. J. Peter, Host-vector dynamics of dengue with asymptomatic, isolation and vigilant compartments: insights from modelling, Eur. Phys. J. Plus., 138 (2023), 199. https://doi.org/10.1140/epjp/s13360-023-03823-7 doi: 10.1140/epjp/s13360-023-03823-7
![]() |
[44] |
R. Jan, M. A. Khan, J. F. Gómez-Aguilar, Asymptomatic carriers in transmission dynamics of dengue with control interventions, Optim. Contr. Appl. Met., 41 (2020), 430–447. https://doi.org/10.1002/oca.2551 doi: 10.1002/oca.2551
![]() |
[45] |
S. A. Jose, R. Raja, B. I. Omede, R. P. Agarwal, J. Alzabut, J. Cao, et al., Mathematical modeling on co-infection: transmission dynamics of Zika virus and Dengue fever, Nonlinear Dyn., 111 (2023), 4879–4914. https://doi.org/10.1007/s11071-022-08063-5 doi: 10.1007/s11071-022-08063-5
![]() |
[46] |
R. P. Kumar, G. S. Mahapatra, P. K. Santra, Dynamical analysis of SARS-CoV-2-Dengue co-infection mathematical model with optimum control and sensitivity analyses, Nonlinear Anal. Real, 80 (2024), 104175. https://doi.org/10.1016/j.nonrwa.2024.104175 doi: 10.1016/j.nonrwa.2024.104175
![]() |
[47] |
H. R. Pandey, G. R. Phaijoo, D. B. Gurung, Dengue dynamics in Nepal: A Caputo fractional model with optimal control strategies, Heliyon, 10 (2024), e33822. https://doi.org/10.1016/j.heliyon.2024.e33822 doi: 10.1016/j.heliyon.2024.e33822
![]() |
[48] |
M. Asaduzzaman, A. Kilicman, Abdulla-Al-Mamun, M. D. Hossain, Analysis of a novel conformable fractional order ASIR dengue transmission model in the perspective of Bangladesh, Math. Models Comput. Simul., 16 (2024), 431–456. https://doi.org/10.1134/S2070048224700157 doi: 10.1134/S2070048224700157
![]() |
[49] |
A. Dwivedi, R. Keval, Analysis for transmission of dengue disease with different class of human population, Epidemiol. Method., 10 (2021), 20200046. https://doi.org/10.1515/em-2020-0046 doi: 10.1515/em-2020-0046
![]() |
[50] |
L. Pimpi, S. W. Indratno, J. W. Puspita, E. Cahyono, Stochastic and deterministic dynamic model of dengue transmission based on dengue incidence data and climate factors in Bandung city, Commun. Biomath. Sci., 5 (2022), 78–89. https://doi.org/10.5614/cbms.2022.5.1.5 doi: 10.5614/cbms.2022.5.1.5
![]() |
[51] |
P. Mutsuddy, S. Tahmina Jhora, A. K. M. Shamsuzzaman, S. M. G. Kaisar, M. N. A. Khan, S. Dhiman, Dengue situation in Bangladesh: An epidemiological shift in terms of morbidity and mortality, Canadian J. Infect. Dis. Med. Microbiol., 2019 (2019), 3516284. https://doi.org/10.1155/2019/3516284 doi: 10.1155/2019/3516284
![]() |
[52] |
A. Chamnan, P. Pongsumpun, I. M. Tang, N. Wongvanich, Local and global stability analysis of dengue disease with vaccination and optimal control, Symmetry, 13 (2021), 1917. https://doi.org/10.3390/sym13101917 doi: 10.3390/sym13101917
![]() |
[53] |
H. S. Rodrigues, M. T. T. Monteiro, D. F. M. Torres, Sensitivity analysis in a dengue epidemiological model, Conference Papers in Mathematics, 2013 (2013), 721406. https://doi.org/10.1155/2013/721406 doi: 10.1155/2013/721406
![]() |
[54] |
M. R. Hasan, A. Hobiny, A. Alshehri, Analysis of vector-host SEIR-SEI dengue epidemiological model, Int. J. Anal. Appl., 20 (2022), 57. https://doi.org/10.28924/2291-8639-20-2022-57 doi: 10.28924/2291-8639-20-2022-57
![]() |
[55] |
A. Omame, M. E. Isah, M. Abbas, An optimal control model for COVID‐19, zika, dengue, and chikungunya co‐dynamics with reinfection, Optim. Control Appl. Method., 44 (2022), 170–204. https://doi.org/10.1002/oca.2936 doi: 10.1002/oca.2936
![]() |
[56] | A. Dwivedi, V. Baniya, R. Keval, Application of optimal controls on dengue dynamics-A mathematical study, In: Mathematical modelling and computational intelligence techniques, Springer, 2021. https://doi.org/10.1007/978-981-16-6018-4_1 |
Parameter | Descriptions |
Λ1 | Human population recruitment rates |
β1 | Infection transmission rate from vector to host |
α | Rate of immune system of susceptible host by the consumption of natural foods |
β2 | Infections rate within the host |
μ1 | Human population natural mortality |
β3 | Rate of symptomatic to hospitalized infected individuals |
β4 | Symptomatic infection recovery rate |
γ | Awareness rate among symptomatic infected people |
β5 | Hospitalized infected individual's recovery rate |
Λ2 | Vector population recruitment rates |
β6 | Infection transmission rate from human to vector |
μ2 | Vector population natural mortality |
Parameter | Sensitivity index |
Λ1 | +1.0 |
β2 | +1.0 |
α | -0.993245927 |
μ1 | -0.0084993573 |
γ | -0.3573103612 |
β3 | -0.2793059925 |
β4 | -0.3616383535 |
Parameter | Descriptions |
Λ1 | Human population recruitment rates |
β1 | Infection transmission rate from vector to host |
α | Rate of immune system of susceptible host by the consumption of natural foods |
β2 | Infections rate within the host |
μ1 | Human population natural mortality |
β3 | Rate of symptomatic to hospitalized infected individuals |
β4 | Symptomatic infection recovery rate |
γ | Awareness rate among symptomatic infected people |
β5 | Hospitalized infected individual's recovery rate |
Λ2 | Vector population recruitment rates |
β6 | Infection transmission rate from human to vector |
μ2 | Vector population natural mortality |
Parameter | Sensitivity index |
Λ1 | +1.0 |
β2 | +1.0 |
α | -0.993245927 |
μ1 | -0.0084993573 |
γ | -0.3573103612 |
β3 | -0.2793059925 |
β4 | -0.3616383535 |
Parameter | Values | Reference |
Λ1 | .9999 | [49] |
β1 | .8500 | Assumed |
β2 | .6294 | [50] |
α | .2520 | Assumed |
μ1 | .003468 | [51] |
β3 | .555 | [52] |
β4 | .7186 | [53] |
γ | .111 | [54] |
β5 | .0062 | Assumed |
Λ2 | .00034 | [55] |
β6 | .009 | [56] |
μ2 | .000244 | Assumed |