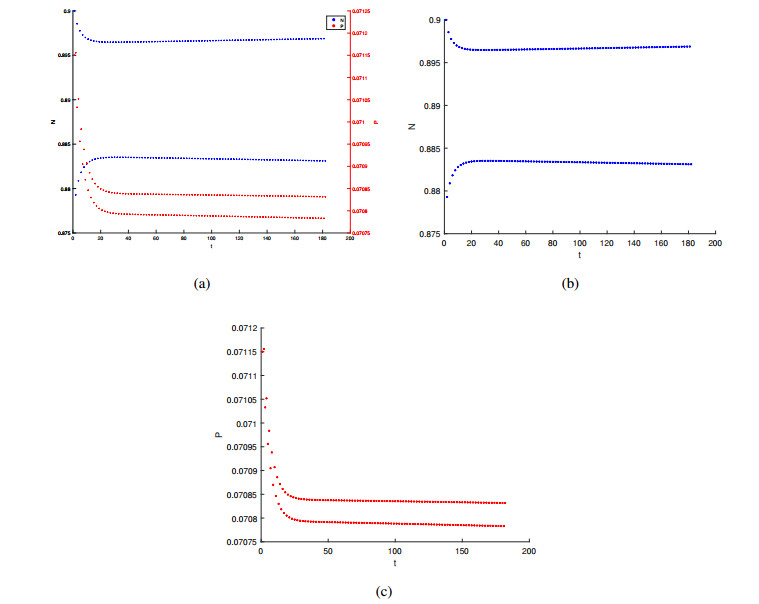
Undoubtedly, one of the most common machine learning challenges is multiclass classification. In light of this, a novel bio-inspired neural network (NN) has been developed to address multiclass classification-related issues. Given that weights and structure determination (WASD) NNs have been acknowledged to alleviate the disadvantages of conventional back-propagation NNs, such as slow training pace and trapping in a local minimum, we developed a bio-inspired WASD algorithm for multiclass classification problems (BWASDC) by using the metaheuristic beetle antennae search (BAS) algorithm to enhance the WASD algorithm's learning process. The BWASDC's effectiveness is then evaluated through applications in occupational classification systems. It is important to mention that systems of occupational classification serve as a fundamental indicator of occupational exposure. For this reason, they are highly significant in social science research. According to the findings of four occupational classification experiments, the BWASDC model outperformed some of the most modern classification models obtainable through MATLAB's classification learner app on all fronts.
Citation: Yu He, Xiaofan Dong, Theodore E. Simos, Spyridon D. Mourtas, Vasilios N. Katsikis, Dimitris Lagios, Panagiotis Zervas, Giannis Tzimas. A bio-inspired weights and structure determination neural network for multiclass classification: Applications in occupational classification systems[J]. AIMS Mathematics, 2024, 9(1): 2411-2434. doi: 10.3934/math.2024119
[1] | Abdelwahed Motwake, Aisha Hassan Abdalla Hashim, Marwa Obayya, Majdy M. Eltahir . Enhancing land cover classification in remote sensing imagery using an optimal deep learning model. AIMS Mathematics, 2024, 9(1): 140-159. doi: 10.3934/math.2024009 |
[2] | Alaa O. Khadidos . Advancements in remote sensing: Harnessing the power of artificial intelligence for scene image classification. AIMS Mathematics, 2024, 9(4): 10235-10254. doi: 10.3934/math.2024500 |
[3] | Hanan T. Halawani, Aisha M. Mashraqi, Yousef Asiri, Adwan A. Alanazi, Salem Alkhalaf, Gyanendra Prasad Joshi . Nature-Inspired Metaheuristic Algorithm with deep learning for Healthcare Data Analysis. AIMS Mathematics, 2024, 9(5): 12630-12649. doi: 10.3934/math.2024618 |
[4] | Maha M. Althobaiti, José Escorcia-Gutierrez . Weighted salp swarm algorithm with deep learning-powered cyber-threat detection for robust network security. AIMS Mathematics, 2024, 9(7): 17676-17695. doi: 10.3934/math.2024859 |
[5] | Jun Ma, Junjie Li, Jiachen Sun . A novel adaptive safe semi-supervised learning framework for pattern extraction and classification. AIMS Mathematics, 2024, 9(11): 31444-31469. doi: 10.3934/math.20241514 |
[6] | Manal Abdullah Alohali, Fuad Al-Mutiri, Kamal M. Othman, Ayman Yafoz, Raed Alsini, Ahmed S. Salama . An enhanced tunicate swarm algorithm with deep-learning based rice seedling classification for sustainable computing based smart agriculture. AIMS Mathematics, 2024, 9(4): 10185-10207. doi: 10.3934/math.2024498 |
[7] | Yuto Omae, Yusuke Sakai, Hirotaka Takahashi . Features gradient-based signals selection algorithm of linear complexity for convolutional neural networks. AIMS Mathematics, 2024, 9(1): 792-817. doi: 10.3934/math.2024041 |
[8] | Mashael Maashi, Mohammed Abdullah Al-Hagery, Mohammed Rizwanullah, Azza Elneil Osman . Deep convolutional neural network-based Leveraging Lion Swarm Optimizer for gesture recognition and classification. AIMS Mathematics, 2024, 9(4): 9380-9393. doi: 10.3934/math.2024457 |
[9] | Mohammed Aljebreen, Hanan Abdullah Mengash, Khalid Mahmood, Asma A. Alhashmi, Ahmed S. Salama . Enhancing cybersecurity in cloud-assisted Internet of Things environments: A unified approach using evolutionary algorithms and ensemble learning. AIMS Mathematics, 2024, 9(6): 15796-15818. doi: 10.3934/math.2024763 |
[10] | Mohammed Abdul Kader, Muhammad Ahsan Ullah, Md Saiful Islam, Fermín Ferriol Sánchez, Md Abdus Samad, Imran Ashraf . A real-time air-writing model to recognize Bengali characters. AIMS Mathematics, 2024, 9(3): 6668-6698. doi: 10.3934/math.2024325 |
Undoubtedly, one of the most common machine learning challenges is multiclass classification. In light of this, a novel bio-inspired neural network (NN) has been developed to address multiclass classification-related issues. Given that weights and structure determination (WASD) NNs have been acknowledged to alleviate the disadvantages of conventional back-propagation NNs, such as slow training pace and trapping in a local minimum, we developed a bio-inspired WASD algorithm for multiclass classification problems (BWASDC) by using the metaheuristic beetle antennae search (BAS) algorithm to enhance the WASD algorithm's learning process. The BWASDC's effectiveness is then evaluated through applications in occupational classification systems. It is important to mention that systems of occupational classification serve as a fundamental indicator of occupational exposure. For this reason, they are highly significant in social science research. According to the findings of four occupational classification experiments, the BWASDC model outperformed some of the most modern classification models obtainable through MATLAB's classification learner app on all fronts.
In biology, discrete models of interspecific relationships in populations without generation-level overlap are more generalizable than continuous ones, which has attracted many scholars in recent years (see [1,2,3,4,5,6,7,8,9,10,11,12]). Obviously, all these works indicate that discrete systems have more complex dynamic behavior. The predator-prey model is a class of classical biomathematical model that has been studied by different scholars in terms of the evolutionary patterns of populations over time [13,14] and the effects of different functional responses on the stability of populations [15,16].
For the predator-prey system, external factors such as food supply, climate change and population migration can affect predation and population reproduction [17,18]. However, the effects of changes in predators themselves are often overlooked, such as predator fear [19]. Frightened predators tend to eat less, which leads to reduced birth rates and increased mortality [19]. Therefore, predator fear, which cannot be quantitatively described, is more difficult to study.
Sasmal [20] studied a predator-prey model with Allee effect and reduced reproduction of prey due to cost of fear. They discussed the effects of time scale separation between prey and predator.
{dudt=ru(1−uk)(u−θ)11+fv−auv(0<θ<k)dvdt=aαuv−mv | (1.1) |
where u and v are the prey and predator population densities, respectively. r is the maximum growth rate of the population, k is the carrying capacity, and θ represents the Allee threshold (0<θ<k), below which the population becomes extinct. f refers to the level of fear, which is due to the anti-predator response of prey. a is the predation rate, α is the conversion efficiency of predator by consuming prey, and m represents the predator's natural mortality rate.
To simplify parameters, define N=uk, P=arkv, ϵ=aαr, θ=θk, m=maαk, f=rkfa, t=aαkt. Then, the system is given by
{dNdt=1ϵ[N(1−N)(N−θ)11+fP−NP]dPdt=NP−mP |
At present, there are some studies on predator-prey systems with Flip bifurcation and Hopf bifurcation [21] in continuous models, where, however, the chaotic properties have not been mentioned. For example, Khan studied a discrete-time Nicholson-Bailey host-parasitoid model and obtained the local dynamics and supercritical Neimark-Sacker bifurcation in 2017 [22]. In 2019, Li et al. investigated the discrete time predator-prey model undergoing Flip and Neimark Sacker bifurcation using the center manifold theorem and bifurcation theory [23]. In 2022, Yu et al. gave a description of the bifurcations in theory for a symmetrically coupled period-doubling system, including the Transcritical bifurcation, Pitchfork bifurcation, Flip bifurcation and Neimark-Sacker bifurcation [24]. In 2022, Chen et al. studied a discrete predator-prey system with Allee effect, which undergoes Fold bifurcation and Flip bifurcation. They obtained cascades of period-doubling bifurcation in orbits of period-2, 4, 8 and chaotic properties [25].
Based on the above analysis and discussion, we obtain the following discrete-time model:
{Nn+1=Nn+h{1ϵ[Nn(1−Nn)(Nn−θ)11+fPn−NnPn]}Pn+1=Pn+h[NnPn−mPn] | (1.2) |
where h>0 refers to the step length of (1.2), 0<θ<1.
In this paper, we study the existence and stability of four equilibria of model (1.2) and focus on local dynamics of the unique positive equilibrium point in Section 2. In Sections 3 and 4, we study Flip bifurcation and Hopf bifurcation when bifurcation parameter h varies in a small neighborhood of the unique positive equilibrium point. In Section 5, we study chaos scenarios of Flip bifurcation. Meanwhile, some numerical simulations about bifurcations are given by maximum Lyapunov exponent and phase diagrams to verify our results. Finally, brief conclusions are given in Section 6.
Initially, the existence and stability of the equilibrium point of model (1.2) are analyzed.
By calculating model (1.2), obviously, the trivial equilibrium point E1=(0,0) and boundary equilibria E2=(1,0), E3=(θ,0) are obtained. Since the negative equilibrium point has no practical meaning in biology, the unique positive internal equilibrium point E4=(m,−1+√1+4f(1−m)(m−θ)2f) is chosen.
The Jacobi matrix of the linear system of (1.2) at any equilibrium point (N∗,P∗) is given by:
J=(1+hϵ[−3N2+2(1+θ)N−θ1+fP−P]hϵ[(N3−(1+θ)N2+θN)f(1+fP)2−N]hP1+hN−mh)|(N∗,P∗) |
Now, we give some dynamical properties of four equilibria.
Theorem 2.1. For the trivial equilibrium E1, with eigenvalues λ1=1−hθϵ and λ2=1−hm.
(i) E1 is a sink if 0<h<min{2ϵθ,2m}, with eigenvalues |λ1|<1 and |λ2|<1.
(ii) E1 is a source if h>max{2ϵθ,2m}, with eigenvalues |λ1|>1 and |λ2|>1.
(iii) E1 is a saddle point if 2ϵθ<h<2m with eigenvalues |λ1|>1 and |λ2|<1, or 2m<h<2ϵθ with eigenvalues |λ1|<1 and |λ2|>1.
(iv) Flip bifurcation occurs at E1 if h=2ϵθ<2m, with eigenvalues λ1=−1 and |λ2|<1, or h=2m<2ϵθ, with eigenvalues λ2=−1 and |λ1|<1.
Theorem 2.2. For the boundary equilibrium E2, with eigenvalues λ1=1+hϵ(θ−1) and λ2=1+h(1−m).
(i) E2 is a sink if {m>10<h<min(2ϵ1−θ,2m−1), with eigenvalues |λ1|<1 and |λ2|<1.
(ii) E2 is a source if {m<1h>2ϵ1−θ or {m>1h>max{2ϵ1−θ,2m−1}, with eigenvalues |λ1|>1 and |λ2|>1.
(iii) E2 is a saddle point if {m>12ϵ1−θ<h<2m−1 or {m>12m−1<h<2ϵ1−θ with eigenvalues |λ1|>1 and |λ2|<1, or {m<1h<2ϵ1−θ with eigenvalues |λ1|<1 and |λ2|>1.
(iv) Flip bifurcation occurs at E2 if {m>1h=2ϵ1−θ<2m−1 with eigenvalues λ1=−1 and |λ2|<1, or h=2m−1<2ϵ1−θ with eigenvalues λ2=−1 and |λ1|<1.
(v) Transcritical bifurcation occurs at E2 if {m=1h<2ϵ1−θ, with eigenvalues λ2=1 and |λ1|<1.
Theorem 2.3. For the equilibrium E3, with eigenvalues λ1=1+hϵθ(1−θ) and λ2=1+h(θ−m).
(i) E3 is a source if θ>m or {θ<mh>2m−θ, with eigenvalues |λ1|>1 and |λ2|>1.
(ii) E3 is a saddle point if {θ<m0<h<2m−θ, with eigenvalues |λ1|>1 and |λ2|<1.
There is no situation as |λ1|<1, i.e., stability does not exist.
Theorem 2.4. For the internal equilibrium E4,
(i) E4 is a sink if
{1+hϵ[m(1+θ−2m)1+√1+4f(1−m)(m−θ)]+h2ϵ[m(1−m)(m−θ)√1+4f(1−m)(m−θ)[1+√1+4f(1−m)(m−θ)]2]>0m(1−m)(m−θ)√1+4f(1−m)(m−θ)>01+θ−2m1+√1+4f(1−m)(m−θ)+h[2(1−m)(m−θ)√1+4f(1−m)(m−θ)[1+√1+4f(1−m)(m−θ)]2]<0 |
(ii) Transcritical bifurcation occurs at E4 if
{m=1 or m=θ−2ϵ<h[2m(1+θ−2m)1+√1+4f(1−m)(m−θ)]<0. |
(iii) Flip bifurcation occurs at E4 if
{1+hϵ[m(1+θ−2m)1+√1+4f(1−m)(m−θ)]+h2ϵ[m(1−m)(m−θ)√1+4f(1−m)(m−θ)[1+√1+4f(1−m)(m−θ)]2]=0−4<hϵ[m(1+θ−2m)1+√1+4f(1−m)(m−θ)]<−2. |
(iv) Hopf bifurcation occurs at E4 if
{h=(2m−θ−1)[1+√1+4f(1−m)(m−θ)]2(1−m)(m−θ)√1+4f(1−m)(m−θ)−4<hϵ[m(1+θ−2m)1+√1+4f(1−m)(m−θ)]<0. |
Proof. The Jacobi matrix of (1.2) at point E4=(N0,P0) is
J∣(N0,P0)=(1+hϵ[2m(1+θ−2m)1+√1+4f(1−m)(m−θ)] hϵ[−2m+√1+4f(1−m)(m−θ)1+√1+4f(1−m)(m−θ)]h(−1+√1+4f(1−m)(m−θ))2f 1) | (2.1) |
where n=1+√1+4f(1−m)(m−θ).
Meanwhile, the characteristic polynomial of J∣(N0,P0) is given by:
f(λ)=λ2−(A+1)λ+(A+BC)=0 | (2.2) |
where
A=1+hϵ[2m(1+θ−2m)1+√1+4f(1−m)(m−θ)], |
B=hϵ[−2m+√1+4f(1−m)(m−θ)1+√1+4f(1−m)(m−θ)],C=h(−1+√1+4f(1−m)(m−θ))2f. |
Then, eigenvalues of J∣(N0,P0) are λ1=A+1+√Δ2 and λ2=A+1−√Δ2, where Δ=(A−1)2−4BC.
(i) (N0,P0) is a sink, if |λ1|<1 and |λ2|<1. From the Jury Criterion [26], we obtain {1+(A+1)+(A+BC)>01−(A+1)+(A+BC)>01−(A+BC)>0.
(ii) If Δ>0, λ1=1 and |λ2|<1 or λ2=1 and |λ1|<1, then we have {BC=0|A|<1.
(iii) If Δ>0, λ1=−1 and |λ2|<1 or λ2=−1 and |λ1|<1, then we have {2A+BC+2=0|A+2|<1.
(iv) If Δ<0, |λ1|=|λ2|=1, which is a pair of conjugate complex roots, then we have {A+BC=10<|A+1|<2.
When λ1 and λ2 is a pair of conjugate complex roots, we assume λ1=a+bi and λ2=a−bi (a2+b2=1 b≠0), i.e., 0<|a|<1. Since (λ−λ1)(λ−λ2)=λ2−(λ1+λ2)λ+λ1λ2=0, obviously we have λ1+λ2=A+1=2a and λ1λ2=A+BC=1.
All of the expressions A,B,C can be brought into equations mentioned above to obtain the corresponding conclusions.
In this section, we discuss how system (1.2) undergoes Flip bifurcation around its internal equilibrium E4=(m,−1+√1+4f(1−m)(m−θ)2f) when h is chosen as bifurcation parameter. The necessary conditions for Flip bifurcation to occur is given by the following curve:
U={(m,n,θ,f,ϵ)∈R5+:h=h∗=2f(2m−1−θ)+2√f2(1+θ−2m)2+1m(n−1)(n−2)ϵfnm(n−1)(n−2) ,|D|<1} |
where D=hϵ(m(1+θ−2m)1+√1+4f(1−m)(m−θ))+3 and n=1+√1+4f(1−m)(m−θ).
The Jacobi matrix of the linear system of (1.2) at the equilibrium point (N0,P0) is given by:
J∣(N0,P0)=(1+hϵ[2m(1+θ−2m)n] hϵ[−2m(n−1)n]h(n−2)2f 1) | (3.1) |
where n=1+√1+4f(1−m)(m−θ).
In order to obtain the bifurcation properties, we consider translations ¯Nn+1=Nn+1−N0, ¯Pn+1=Pn+1−P0 for shifting (N0,P0) to the origin. Then, the model (1.2) is given by
{¯Nn+1=¯Nn+h{1ϵ[(¯Nn+N0)(1−¯Nn−N0)(¯Nn+N0−θ)11+f(¯Pn+P0)−(¯Nn+N0)(¯Pn+P0)]}¯Pn+1=¯Pn+h[(¯Nn+N0)(¯Pn+P0)−m(¯Pn+P0)] | (3.2) |
Using Taylor expansion at the point E4(N0,P0) yields the following expression :
(¯Nn+1¯Pn+1)=J∣(N0,P0)(¯Nn¯Pn)+h(1ϵψ1(¯Nn,¯Pn)ψ2(¯Nn,¯Pn)) | (3.3) |
where
ψ1(¯Nn,¯Pn)=b1¯Nn2+b2¯Nn¯Pn+b3¯Pn2+b4¯Nn3+b5¯Nn2¯Pn+b6¯Nn¯Pn2+b7¯Pn3+O((|¯Nn|+|¯Pn|)4),
ψ2(¯Nn,¯Pn)=¯Nn¯Pn,
b1=−3N0+1+θ1+fP0, b2=[3N20−2(1+θ)N0+θ]f(1+fP0)2−1, b3=[−N30+(1+θ)N20−θN0]f2(1+fP0)3, b4=−11+fP0, b5=(3N0−1−θ)f(1+fP0)2,
b6=[3N20−2(1+θ)N0+θ]f2(1+fP0)3, b7=[N30−(1+θ)N20+θN0]f3(1+fP0)4.
From the characteristic polynomial (2.2), we obtain f(−1)=h2ϵ[m(n−1)(n−2)fn]+hϵ[4m(1+θ−2m)n]+4.
Since step size h>0, the bifurcation parameter is chosen as
h∗=2f(2m−1−θ)+2√f2(1+θ−2m)2+1m(n−1)(n−2)ϵfnm(n−1)(n−2) |
where parameter m satisfies 1+θ2<m<1.
Consider parameter h with a small perturbation δ, i.e., h=h∗+δ, |δ|≪1, and the system (3.3) becomes
(¯Nn+1¯Pn+1)=(1+(h∗+δ)ϵ[2m(1+θ−2m)n](h∗+δ)ϵ[−2m(n−1)n](h∗+δ)(n−2)2f1)(¯Nn¯Pn)+(h∗+δ)(1ϵψ1(¯Nn,¯Pn)ψ2(¯Nn,¯Pn)) | (3.4) |
The characteristic polynomial of (3.4) is given by
g(λ)=λ2−[2+h∗+δϵ[2m(1+θ−2m)n]]λ+1+h∗+δϵ[2m(1+θ−2m)n]+(h∗+δ)2ϵ[m(n−1)(n−2)fn] |
The transversal condition at (N0,P0) is
dg(λ)dδ∣λ=−1,δ=0=−4−2mh∗(1+θ−2m)ϵn |
If dg(λ)dδ∣λ=−1,δ=0≠0, then Flip bifurcation will occur at E4.
To facilitate discussion, define A=(1+h∗ϵ[2m(1+θ−2m)n]h∗ϵ[−2m(n−1)n]h∗(n−2)2f1).
If the eigenvalue of A goes for λ=−1, the corresponding eigenvector is given as:
T1=(h∗ϵ[−2m(n−1)n]−2−h∗ϵ[2m(1+θ−2m)n]) |
If the eigenvalue of A goes for λ=λ2, the corresponding eigenvector is given as:
T2=(h∗ϵ[−2m(n−1)n]λ2−1−h∗ϵ[2m(1+θ−2m)n]) |
Then, we have the invertible matrix
T=(T1,T2)=(h∗ϵ[−2m(n−1)n]h∗ϵ[−2m(n−1)n]−2−h∗ϵ[2m(1+θ−2m)n] λ2−1−h∗ϵ[2m(1+θ−2m)n]) |
Using translation (xnyn)=T−1(¯Nn¯Pn), system (3.4) turns into
(xn+1yn+1)=(−100λ2)(xnyn)+(f1(¯Nn,¯Pn,δ)g1(¯Nn,¯Pn,δ)) | (3.5) |
where
f1(¯Nn,¯Pn,δ)=a11¯Nnδ+a12¯Pnδ+b11¯Nn2+b12¯Nn¯Pn+b13¯Pn2+b14¯Nn3+b15¯Nn2¯Pn+b16¯Nn¯Pn2+b17¯Pn3+c11¯Nn2δ+c12¯Nn¯Pnδ+c13¯Pn2δ+c14¯Nn3δ+c15¯Nn2¯Pnδ+c16¯Nn¯Pn2δ+c17¯Pn3δ |
g1(¯Nn,¯Pn,δ)=a21¯Nnδ+a22¯Pnδ+b21¯Nn2+b22¯Nn¯Pn+b23¯Pn2+b24¯Nn3+b25¯Nn2¯Pn+b26¯Nn¯Pn2+b27¯Pn3+c21¯Nn2δ+c22¯Nn¯Pnδ+c23¯Pn2δ+c24¯Nn3δ+c25¯Nn2¯Pnδ+c26¯Nn¯Pn2δ+c27¯Pn3δ |
a11=1h∗(λ2+1)[2m−1−θn−1](λ2−1−h∗ϵ[2m(1+θ−2m)n])−n−22f(λ2+1)a12=1h∗(λ2+1)(λ2−1−h∗ϵ[2m(1+θ−2m)n])a21=1h∗(λ2+1)[2m−1−θn−1](2+h∗ϵ[2m(1+θ−2m)n])+n−22f(λ2+1)a22=1h∗(λ2+1)(2+h∗ϵ[2m(1+θ−2m)n])b11=1λ2+1[n−2m(n−1)](λ2−1−h∗ϵ[2m(1+θ−2m)n])b1b12=1λ2+1[n−2m(n−1)](λ2−1−h∗ϵ[2m(1+θ−2m)n])b2−h∗λ2+1b13=1λ2+1[n−2m(n−1)](λ2−1−h∗ϵ[2m(1+θ−2m)n])b3⋮b17=1λ2+1[n−2m(n−1)](λ2−1−h∗ϵ[2m(1+θ−2m)n])b7cij=h∗bij(i=1,2 ; j=1,2,⋯,7) |
Using translation (¯Nn+1¯Pn+1)=T(xn+1yn+1), system (3.5) becomes
(xn+1yn+1)=(−xnλ2yn)+(f2(xn,yn,δ)g2(xn,yn,δ)) | (3.6) |
where
f2(xn,yn,δ)=d11xnδ+d12ynδ+e11x2n+e12xnyn+e13y2n+e14x3n+e15x2nyn+e16xny2n+e17y3n+f11x2nδ+f12xnynδ+f13y2nδ+f14x3nδ+f15x2nynδ+f16xny2nδ+f17y3nδ |
g2(xn,yn,δ)=d21xnδ+d22ynδ+e21x2n+e22xnyn+e23y2n+e24x3n+e25x2nyn+e26xny2n+e27y3n+f21x2nδ+f22xnynδ+f23y2nδ+f24x3nδ+f25x2nynδ+f26xny2nδ+f27y3nδ |
k11=k12=h∗ϵ[−2m(n−1)n], k21=−2−h∗ϵ[2m(1+θ−2m)n], k22=λ2−1−h∗ϵ[2m(1+θ−2m)n]
d11=a11k11+a12k21,d12=a11k12+a12k22,e11=b11k211+b12k11k21+b13k221,e12=2b11k11k12+b12(k11k22+k12k21)+2b13k21k22,e13=b11k212+b12k12k22+b13k222,e14=b14k311+b15k211k21+b16k11k221+b17k321,e15=3b14k211k12+b15(k211k22+2k11k12k21)+b16(2k11k21k22+k12k221)+3b17k221k22,e16=3b14k11k212+b15(2k11k12k22+k212k21)+b16(k11k222+2k12k21k22)+3b17k21k222,e17=b14k312+b15k212k22+b16k12k222+b17k322,d21=a21k11+a22k21,d22=a21k12+a22k22,e21=b21k211+b22k11k21+b23k221,e22=2b21k11k12+b22(k11k22+k12k21)+2b23k21k22,e23=b21k212+b22k12k22+b23k222,e24=b24k311+b25k211k21+b26k11k221+b27k321,e25=3b24k211k12+b25(k211k22+2k11k12k21)+b26(2k11k21k22+k12k221)+3b27k221k22,e26=3b24k11k212+b25(2k11k12k22+k212k21)+b26(k11k222+2k12k21k22)+3b27k21k222,e27=b24k312+b25k212k22+b26k12k222+b27k322,fij=heij(i=1,2 ; j=1,2,⋯,7). |
Next, by using the center manifold theorem and normal form theories, the direction of Flip bifurcation at E4 is given.
α1=(∂2f∂˜xnδ+12∂f∂δ∂2f∂˜x2n)∣(0,0),α2=(16∂3f∂˜x3n+(12∂2f∂˜x2n)2)∣(0,0), |
where the coefficients of α1 and α2 are derived from (3.6).
Theorem 3.1. If α1≠0, α2≠0, then the system undergoes Flip bifurcation at the immobile point (N0,P0), and if α2>0(<0), then the two-cycle point is stable (unstable).
For sufficiently small neighborhoods of the parameter δ=0, there exists a central manifold at (0,0):
Wc(0,0)={(˜x,˜y):˜yn+1=m1˜x2n+1+m2˜xn+1δ} | (3.7) |
Substituting (3.7) into (3.6), the solution is given as
m1=e211−λ2=b21k211+b22k11k21+b23k2211−λ2,m2=−d211+λ2=−(a21k11+a22k21) 1+λ2 |
Restricting the system of equations on Wc(0,0), the results are as follows.
˜xn+1=−˜xn+l1˜x2n+l2˜xnδ+l3˜x2nδ+l4˜xnδ2+l5˜x3n+O((|˜xn|+|δ|)4) |
where l1=e11=b11k211+b12k11k21+b13k221, l2=d11=a11k11+a12k21, l3=d12m1+f11+e12m2, l4=d12m2, l5=e12m1+e14.
According to the normal form theories related to bifurcation analysis, we require the following quantity at (x,y,δ)=(0,0,0).
α1=(∂2f∂˜xnδ+12∂f∂δ∂2f∂˜x2n)∣(0,0)=l2+l3,α2=(16∂3f∂˜x3n+(12∂2f∂˜x2n)2)∣(0,0)=l5+l21 |
For numerical simulations, we choose the following parameters to prove our theoretical discussion. The unique positive equilibrium point (N0,P0)≈(0.89,0.0708765) of the model can be obtained by giving the parameters h=0.1, e=0.023933614, θ=0.2, f=1, m=0.89. By choosing the initial value of (0.9,0.07115), the result can be obtained by numerical simulation as Figure 1.
It is obvious from the diagrams that variables N and P appear as two-point solutions with a period for a given parameter condition and gradually stabilize near the equilibrium point, which verifies Flip bifurcation occurs at this point.
In this section, we still choose h as the bifurcation parameter to discuss Hopf bifurcation around its positive equilibrium (N0,P0). The necessary conditions for Hopf bifurcation to occur is given by the following curve:
S={(m,n,θ,f,ϵ)∈R5+:h=h∗1=(2m−1−θ)[1+√1+4f(1−m)(m−θ)]2(1−m)(m−θ)√1+4f(1−m)(m−θ), |E|<2} |
where E=hϵ(m(1+θ−2m)1+√1+4f(1−m)(m−θ))+2.
For emergence of Hopf bifurcation around positive equilibrium (N0,P0), two roots of the characteristic polynomial (2.2) must be complex conjugate with unit modulus. Therefore, it is easy to obtain the bifurcation parameter h∗1=(2m−θ−1)(1+√1+4f(1−m)(m−θ))2(1−m)(m−θ)√1+4f(1−m)(m−θ)>0, i.e., 0<m<θ or 1+θ2<m<1.
We still consider parameter h with a small perturbation δ, and then characteristic equation (2.2) can be rewritten as:
λ2+p(δ)λ+q(δ)=0, |
where
p(δ)=−[2+(h∗1+δ)ϵ[2m(1+θ−2m)n]], |
q(δ)=1+(h∗1+δ)ϵ[2m(1+θ−2m)n]+(h∗1+δ)2ϵ[m(n−1)(n−2)fn] |
The roots of the characteristic equation of J∣(N0,P0) are
λ1=p(δ)+i√4q(δ)−p(δ)22 , λ2=p(δ)−i√4q(δ)−p(δ)22 |
Also,
|λ1,2|=√q(δ),d|λ1,2|dδ∣δ=0=[1−4f(1+θ−2m)2ϵn(n−1)(n−2)+2(2m−1−θ)ϵmn]−12(1+θ−2m)(m−2)ϵn |
where d|λ1,2|dδ∣δ=0>0 if and only if (1+θ−2m)(m−2)ϵn>0.
If p(0)≠0,1, we have −4f(2m−1−θ)2ϵn(n−1)(n−2)≠2,3, which means λn1,2≠1 n=1,2,3,4.
The transversal condition at (N0,P0) is given by
d|λ1|2dδ∣δ=0=(λ1dλ2dδ+λ2dλ1dδ)∣δ=0=m(1+θ−2m)ϵn[3+2mh∗1(1+θ−2m)ϵn]+mh∗1(n−1)(n−2)ϵfn |
If 1+θ2<m<1, we have d|λ1|2dδ∣δ=0>0. Then, Hopf bifurcation will occur at (N0,P0).
Now, let
α=1+h∗1ϵ[m(1+θ−2m)n],β=h∗1√m(n−1)(n−2)ϵfn−m2(1+θ−2m)2ϵ2n2 |
The invertible matrix T is given by
T=( hϵ[−2m(n−1)n]0 α−1−hϵ[2m(1+θ−2m)n]−β ) |
Using the following translation
(¯Nn+1¯Pn+1)=T(¯xn+1¯yn+1) |
Then, the Eq (3.4) transforms to
(¯xn+1¯yn+1)=(α−ββα)(ˉxnˉyn)+(f(ˉxn,ˉyn)g(ˉxn,ˉyn)) |
where
f(ˉxn,ˉyn)=l11ˉx2n+l12ˉxnˉyn+l13ˉy2n+l14ˉx3n+l15ˉxn2ˉyn+l16ˉxnˉy2n+l17ˉy3n,g(ˉxn,ˉyn)=l21ˉx2n+l22ˉxnˉyn+l23ˉy2n+l24ˉx3n+l25ˉxn2ˉyn+l26ˉxnˉy2n+l27ˉy3n,l11=b1α3+b2α2β+αβ2+b3αβ2,l12=−2b1α2β+b2α3+βα2−b2αβ2−β3+2b3α2β,l13=b1αβ2−b2α2β−αβ2+b3α3,l14=b4α4+b5α3β+b6α2β2+b7αβ3,l15=−3b4α3β+b5α4−2b5α2β2+2b6α3β−b6αβ3+3b7α2β2,l16=3b4α2β2+b5αβ3−2b5α3β+b6α4−2b6α2β2+3b7α3β,l17=−b4αβ3+b5α2β2−b6α3β+b7α4,l21=b1α2β+b2αβ2−α2β+b3β3,l22=−2b1αβ2+b2α2β−b2β3−α3+αβ2+2b3αβ2,l23=b1β3−b2αβ2+α2β+b3α2β,l24=b4α3β+b5α2β2+b6αβ3+b7β4,l25=−3b4α2β2+b5α3β−2b5αβ3+2b6α2β2−b6β4+3b7αβ3,l26=3b4αβ3+b5β4−2b5α2β2+b6α3β−2b6αβ3+3b7α2β2,l27=−b4β4+b5αβ3−b6α2β2+b7α3β |
Next, by using the center manifold theorem and normal form theories, the direction of Hopf bifurcation at E4 is given. According to the normal form theories related to bifurcation analysis, we require the following quantity at (x,y,δ)=(0,0,0).
L=−Re[(1−2λ)ˉλ21−λξ11ξ20]−12|ξ11|2−|ξ02|2+Re(ˉλξ21) |
where
ξ20=18[fˉxˉx+fˉyˉy+2gˉxˉy+i(gˉxˉx−gˉyˉy−2fˉxˉy)],ξ11=14[fˉxˉx+fˉyˉy+i(gˉxˉx+gˉyˉy)],ξ02=18[fˉxˉx−fˉyˉy+2gˉxˉy+i(gˉxˉx−gˉxˉy+2fˉxˉy)],ξ21=116[fˉxˉxˉx+fˉxˉyˉy+gˉxˉxˉy+gˉyˉyˉy+i(gˉxˉxˉx+gˉxˉyˉy−fˉxˉxˉy−fˉyˉyˉy)],fˉxˉx=2l11 , fˉxˉy=l12 , fˉxˉxˉx=6l14 , fˉxˉxˉy=2l15 , fˉxˉyˉy=2l16 , fˉyˉy=2l13 , fˉyˉyˉy=6l17,gˉxˉx=2l21 , gˉxˉy=l22 , gˉxˉxˉx=6l24 , gˉxˉxˉy=2l25 , gˉxˉyˉy=2l26 , gˉyˉy=2l23 , gˉyˉyˉy=6l27 . |
Theorem 4.1. If the above conditions hold, and L≠0, the Hopf bifurcation occurs at the point (N0,P0). When δ>0, if L<0, then it attracts at that point; when δ<0, if L>0, then it repels at that point.
To verify whether the condition is correct, we select the following parameters for numerical simulations and obtain the following conclusions. By selecting the first set of parameters h≈0.062163, e=1, θ=0.1, f=1, m=0.554, the unique positive equilibrium point (N0,P0)≈(0.554,0.004932) of the model can be obtained. By choosing the initial value K1=(0.5,0.005), the following results can be obtained by numerical simulation.
Figure 2 reflects that variable N gradually tends to oscillate steadily at the equilibrium point N=0.554 with the increase of the number of iterations n, while variable P also gradually oscillates steadily with the increase of the number of iterations N, but the equilibrium point is attracted to P≈0.157.
By choosing the second set of parameters h≈1.120901, e=1, θ=0.61, f=1, m=0.823 the only internal equilibrium point (N0,P0)≈(0.823,0.020442) of the model can be obtained. Choosing the initial value K2=(0.8,0.02), the following results can be obtained by numerical simulation.
Figure 3 shows that as the number of iterations n increases, eventually, the variable P will also be attracted to stabilize at P≈0.035.
In this section, chaotic cases at bifurcating points are analyzed using numerical simulations. We give maximum Lyapunov exponents and phase diagrams for different perturbation parameters to prove our results. The chaos theory analysis of Flip bifurcation and Hopf bifurcation is given as follows.
Definition 5.1. [27] The formula for the maximum Lyapunov index is given by
λ=limn→∞1nn−1∑n=0ln|df(xn,μ)dx| |
Theorem 5.1. [27] If λ<0, the neighboring points eventually come together and merge into a single point, which corresponds to stable immobile points and periodic motion. If λ>0, the neighboring points eventually separate, which corresponds to local instability of the orbit generating chaotic situations.
In the following, we choose two different equilibria to consider the Flip bifurcation with chaotic cases. Initially, we select the parameters h=0.95, e≈0.1841359, θ=0.5, f=1, m=0.961, and obviously the model comes to
{Nn+1=Nn+0.95{10.1841359[Nn(1−Nn)(Nn−0.5)11+Pn−NnPn]}Pn+1=Pn+0.95[NnPn−0.961Pn] |
where the internal equilibrium point is (N1,P1)≈(0.961,0.01807).
After selecting the perturbation δ∈(0,0.2) and analyzing the trend of N with δ by numerical simulation, we obtain the chaotic bifurcating cases (see Figure 4).
Next, choosing the parameters h=0.95, e≈0.184136, θ=0.5, f=1, m=0.9601, we obtain the model
{Nn+1=Nn+0.95{10.184136[Nn(1−Nn)(Nn−0.5)11+Pn−NnPn]}Pn+1=Pn+0.95[NnPn−0.9601Pn] |
Obviously, the internal equilibrium point (N2,P2)≈(0.9601,0.0180734). We still choose the perturbation δ∈(0,0.2) to analyze the trend of N with δ. The chaotic bifurcating case is obtained (see Figure 5). By calculating the bifurcating diagrams and the maximum Lyapunov exponent for two bifurcating points with small differences, it can be shown that the small change of the initial value near the equilibrium point has a great influence on the stability of the initial state. Although they are different perturbed bifurcating cases, the two cases tend to go the same during the process of δ gradually increasing.
In the following, we choose two different equilibria to consider Hopf bifurcation with chaotic cases. By picking the first set of parameters h≈0.062163, e=1, θ=0.1, f=1, m=0.554, the unique internal equilibrium point (N3,P3)≈(0.554,0.004932) of the model can be obtained.
After choosing perturbation δ∈(0,0.5), we obtain phase diagrams (see Figure 6).
The analysis mentioned above shows that with the perturbation δ increasing, the asymptotic stability of variable N gradually becomes weaker, and the range of periodic solutions gradually becomes larger, but the convergence speed comes faster increases (see Figure 6).
By choosing the second set of parameters h≈1.120901, e=1, θ=0.61, f=1, m=0.823, we can obtain the unique internal equilibrium point (N4,P4)≈(0.823,0.020442) of the model.
After choosing perturbation δ∈(−1,1), we obtain phase diagrams (see Figure 7).
Our work deals with the study of local dynamical properties of a predator-prey model with discrete time (1.2), Flip bifurcation and Hopf bifurcation associated with the periodic solution, as well as their chaotic cases. We prove that the system (1.2) has four unique equilibria. In addition, their stability and instability conditions are given, which mark the final equilibrium state of both groups as fear, climate and other factors change. We focus on the unique positive equilibrium point E4=(m,−1+√1+4f(1−m)(m−θ)2f), with stability condition:
{1+hϵ[m(1+θ−2m)1+√1+4f(1−m)(m−θ)]+h2ϵ[m(1−m)(m−θ)√1+4f(1−m)(m−θ)[1+√1+4f(1−m)(m−θ)]2]>0 m(1−m)(m−θ)√1+4f(1−m)(m−θ)>01+θ−2m1+√1+4f(1−m)(m−θ)+h[2(1−m)(m−θ)√1+4f(1−m)(m−θ)[1+√1+4f(1−m)(m−θ)]2]<0 |
Significantly, after analyzing the formation conditions of Flip bifurcation and Hopf bifurcation at (N0,P0), we give their chaotic scenarios from both theoretical and numerical simulations. Moreover, it is found that Flip bifurcation is more prone to chaos scenarios, and Hopf bifurcation is closely related to periodic solutions.
Biologically, the occurrence of Flip bifurcation implies the number of predators and prey will alternate around a value instead of converging to a fixed value, eventually. The corresponding chaotic scenario suggests that small perturbations in the variable parameters will eventually have a significant impact on the population, ultimately leading to chaos. The appearance of Hopf bifurcation points indicates that the equilibrium point of the system loses its attraction and eventually produces a closed orbit, which implies the existence of periodic oscillations of predators and prey. Therefore, we can precisely change the biological density of predators and prey to achieve the desired goal by regulating the number of bifurcation parameters h, for the fact that the chaotic scenario does not exist. All the conclusions illustrate the effect of fear on populations. Finally, the accuracy of the theory is demonstrated using the maximum Lyapunov exponent and phase diagrams.
In future work, the model can be investigated using different discrete methods. In addition, to obtain specific biological properties, new bifurcation parameters can be selected for the study to obtain the desired conclusions.
The authors declare that they have no competing interests.
[1] |
E. Felten, M. Raj, R. Seamans, Occupational, industry, and geographic exposure to artificial intelligence: A novel dataset and its potential uses, Strategic. Manage. J., 42 (2021), 2195–2217. https://doi.org/10.1002/smj.3286 doi: 10.1002/smj.3286
![]() |
[2] | E. W. Felten, M. Raj, R. Seamans, The occupational impact of artificial intelligence: Labor, skills, and polarization, NYU Stern School of Business, 2019. https://doi.org/10.2139/ssrn.3368605 |
[3] |
B. Bigdeli, P. Pahlavani, H. A. Amirkolaee, An ensemble deep learning method as data fusion system for remote sensing multisensor classification, Appl. Soft Comput., 110 (2021), 107563. https://doi.org/10.1016/j.asoc.2021.107563 doi: 10.1016/j.asoc.2021.107563
![]() |
[4] |
R. J. S. Raj, S. J. Shobana, I. V. Pustokhina, D. A. Pustokhin, D. Gupta, K. Shankar, Optimal feature selection-based medical image classification using deep learning model in internet of medical things, IEEE Access, 8 (2020), 58006–58017. https://doi.org/10.1109/ACCESS.2020.2981337 doi: 10.1109/ACCESS.2020.2981337
![]() |
[5] |
T. E. Simos, V. N. Katsikis, S. D. Mourtas, A multi-input with multi-function activated weights and structure determination neuronet for classification problems and applications in firm fraud and loan approval, Appl. Soft Comput., 127 (2022), 109351. https://doi.org/10.1016/j.asoc.2022.109351 doi: 10.1016/j.asoc.2022.109351
![]() |
[6] | G. Varelas, D. Lagios, S. Ntouroukis, P. Zervas, K. Parsons, G. Tzimas, Employing natural language processing techniques for online job vacancies classification, in Artificial Intelligence Applications and Innovations. AIAI 2022 IFIP WG 12.5 International Workshops. AIAI 2022 (eds. I. Maglogiannis, L. Iliadis, J. Macintyre and P. Cortez), vol. 652 of IFIP Advances in Information and Communication Technology, Springer, Cham, 2022,333–344. |
[7] |
A. Rácz, D. Bajusz, K. Héberger, Effect of dataset size and train/test split ratios in QSAR/QSPR multiclass classification, Molecules, 26 (2021), 1111. https://doi.org/10.3390/molecules26041111 doi: 10.3390/molecules26041111
![]() |
[8] |
R. Venkatesan, M. J. Er, A novel progressive learning technique for multi-class classification, Neurocomputing, 207 (2016), 310–321. https://doi.org/10.1016/j.neucom.2016.05.006 doi: 10.1016/j.neucom.2016.05.006
![]() |
[9] | T. E. Simos, V. N. Katsikis, S. D. Mourtas, P. S. Stanimirović, Unique non-negative definite solution of the time-varying algebraic {R}iccati equations with applications to stabilization of LTV systems, Math. Comput. Simul., 202 (2022), 164–180. |
[10] | S. D. Mourtas, V. N. Katsikis, C. Kasimis, Feedback control systems stabilization using a bio-inspired neural network, EAI Endorsed Trans. AI Robot, 1 (2022), 1–13. |
[11] |
N. Premalatha, A. V. Arasu, Prediction of solar radiation for solar systems by using ANN models with different back propagation algorithms, J. Appl. Res. Technol., 14 (2016), 206–214. https://doi.org/10.1016/j.jart.2016.05.001 doi: 10.1016/j.jart.2016.05.001
![]() |
[12] | C. Huang, X. Jia, Z. Zhang, A modified back propagation artificial neural network model based on genetic algorithm to predict the flow behavior of 5754 aluminum alloy, Materials, 11 (2018), 855. |
[13] |
L. Chen, Z. Huang, Y. Li, N. Zeng, M. Liu, A. Peng, et al., Weight and structure determination neural network aided with double pseudoinversion for diagnosis of flat foot, IEEE Access, 7 (2019), 33001–33008. https://doi.org/10.1109/ACCESS.2019.2903634 doi: 10.1109/ACCESS.2019.2903634
![]() |
[14] |
T. E. Simos, V. N. Katsikis, S. D. Mourtas, A fuzzy WASD neuronet with application in breast cancer prediction, Neural Comput. Appl., 34 (2021), 3019–3031. https://doi.org/10.1007/s00521-021-06572-9 doi: 10.1007/s00521-021-06572-9
![]() |
[15] |
M. R. Daliri, A hybrid automatic system for the diagnosis of lung cancer based on genetic algorithm and fuzzy extreme learning machines, J. Medical Syst., 36 (2012), 1001–1005. https://doi.org/10.1007/s10916-011-9806-y doi: 10.1007/s10916-011-9806-y
![]() |
[16] | S. Gayathri, A. K. Krishna, V. P. Gopi, P. Palanisamy, Automated binary and multiclass classification of diabetic retinopathy using Haralick and multiresolution features, IEEE Access, 8 (2020), 57497–57504. https://doi.org/10.1109/ACCESS.2020.2979753 |
[17] |
S. D. Mourtas, V. N. Katsikis, Exploiting the Black-Litterman framework through error-correction neural networks, Neurocomputing, 498 (2022), 43–58. https://doi.org/10.1016/j.neucom.2022.05.036 doi: 10.1016/j.neucom.2022.05.036
![]() |
[18] |
S. D. Mourtas, V. N. Katsikis, E. Drakonakis, S. Kotsios, Stabilization of stochastic exchange rate dynamics under central bank intervention using neuronets, Int. J. Inf. Technol. Decis., 22 (2023), 855–883. https://doi.org/10.1142/s0219622022500560 doi: 10.1142/s0219622022500560
![]() |
[19] |
S. D. Mourtas, A weights direct determination neuronet for time-series with applications in the industrial indices of the federal reserve bank of St. Louis, J. Forecast., 14 (2022), 1512–1524. https://doi.org/10.1002/for.2874 doi: 10.1002/for.2874
![]() |
[20] |
T. E. Simos, V. N. Katsikis, S. D. Mourtas, Multi-input bio-inspired weights and structure determination neuronet with applications in European Central Bank publications, Math. Comput. Simul., 193 (2022), 451–465. https://doi.org/10.1016/j.matcom.2021.11.007 doi: 10.1016/j.matcom.2021.11.007
![]() |
[21] |
R. Boselli, M. Cesarini, S. Marrara, F. Mercorio, M. Mezzanzanica, G. Pasi, et al., WoLMIS: A labor market intelligence system for classifying web job vacancies, J. Intell. Inf. Syst., 51 (2018), 477–502. https://doi.org/10.1007/s10844-017-0488-x doi: 10.1007/s10844-017-0488-x
![]() |
[22] |
P. G. Lovaglio, M. Cesarini, F. Mercorio, M. Mezzanzanica, Skills in demand for ICT and statistical occupations: Evidence from web-based job vacancies, Stat. Anal. Data Min., 11 (2018), 78–91. https://doi.org/10.1002/sam.11372 doi: 10.1002/sam.11372
![]() |
[23] |
E. Heinesen, S. Imai, S. Maruyama, Employment, job skills and occupational mobility of cancer survivors, J. Health Econ., 58 (2018), 151–175. https://doi.org/10.1016/j.jhealeco.2018.01.006 doi: 10.1016/j.jhealeco.2018.01.006
![]() |
[24] |
F. Groes, P. Kircher, I. Manovskii, The U-shapes of occupational mobility, Rev. Econ. Stud., 82 (2015), 659–692. https://doi.org/10.1093/restud/rdu037 doi: 10.1093/restud/rdu037
![]() |
[25] |
M. Khalis, B. Charbotel, E. Fort, V. Chajes, H. Charaka, K. E. Rhazi, Occupation and female breast cancer: A case-control study in Morocco, Rev. Epidemiol. Sante Publique, 66 (2018), S302. https://doi.org/10.1016/j.respe.2018.05.172 doi: 10.1016/j.respe.2018.05.172
![]() |
[26] |
I. N. Generalao, Measuring the telework potential of jobs: Evidence from the international standard classification of occupations, Philipp. Rev. Econ., 58 (2021), 92–127. https://doi.org/10.37907/5erp1202jd doi: 10.37907/5erp1202jd
![]() |
[27] | C. Züll, The coding of occupations, GESIS Survey Guidelines, Mannheim, Germany: GESIS – Leibniz Institute for the Social Sciences. |
[28] |
S. B. Choi, J. H. Yoon, W. Lee, The modified international standard classification of occupations defined by the clustering of occupational characteristics in the Korean working conditions survey, Ind. Health, 58 (2020), 132–141. https://doi.org/10.2486/indhealth.2018-0169 doi: 10.2486/indhealth.2018-0169
![]() |
[29] | D. T. Marc, P. Dua, S. H. Fenton, K. Lalani, K. Butler-Henderson, The Health Information Workforce, chapter Occupational Classifications in the Health Information Disciplines, 71–78, Health Informatics. Springer, Cham., 2021. |
[30] | J. Rounds, P. I. Armstrong, H. Y. Liao, D. Rivkin, P. Lewis, Second generation occupational value profiles for the O* NET system: Summary, Raleigh, NC: National Center for O* NET Development, 2008. |
[31] |
M. P. Wilmot, D. S. Ones, Occupational characteristics moderate personality–performance relations in major occupational groups, J. Vocat. Behav., 131 (2021), 103655. https://doi.org/10.1016/j.jvb.2021.103655 doi: 10.1016/j.jvb.2021.103655
![]() |
[32] | M. Zhang, Estimation of differential occupational risk of COVID-19 by comparing risk factors with case data by occupational group, Am. J. Ind. Med., 64 (2021), 39–47. |
[33] | W. Uter, Kanerva's Occupational Dermatology, chapter Classification of occupations, Springer, Berlin, Heidelberg, 2012. |
[34] | E. Faia, S. Laffitte, M. Mayer, G. Ottaviano, On the employment consequences of automation and offshoring: A labor market sorting view, in Robots and AI, Routledge, 2021, 82–122. |
[35] |
A. S. Ioshisaqui, R. Attux, I. Luna, Analysis of occupational profiles in the Brazilian workforce based on non-negative matrix factorization, Big Data Res., 29 (2022), 100333. https://doi.org/10.1016/j.bdr.2022.100333 doi: 10.1016/j.bdr.2022.100333
![]() |
[36] |
P. Egana-delSol, G. Cruz, A. Micco, COVID-19 and automation in a developing economy: Evidence from Chile, Technol. Forecast. Soc. Change, 176 (2022), 121373. https://doi.org/10.1016/j.techfore.2021.121373 doi: 10.1016/j.techfore.2021.121373
![]() |
[37] |
R. Sebastian, Explaining job polarisation in Spain from a task perspective, SERIEs, 9 (2018), 215–248. https://doi.org/10.1007/s13209-018-0177-1 doi: 10.1007/s13209-018-0177-1
![]() |
[38] | Y. Zhang, D. Chen, C. Ye, Deep Neural Networks: WASD Neuronet Models, Algorithms, and Applications, CRC Press: Boca Raton, FL, USA, 2019. |
[39] | X. Jiang, S. Li, BAS: Beetle antennae search algorithm for optimization problems, arXiv preprint, abs/1710.10724, 2017. Available from: http://arXiv.org/abs/1710.10724 |
[40] |
T. E. Simos, S. D. Mourtas, V. N. Katsikis, Time-varying Black-Litterman portfolio optimization using a bio-inspired approach and neuronets, Appl. Soft Comput., 112 (2021), 107767. https://doi.org/10.1016/j.asoc.2021.107767 doi: 10.1016/j.asoc.2021.107767
![]() |
[41] |
Y. Cheng, C. Li, S. Li, Z. Li, Motion planning of redundant manipulator with variable joint velocity limit based on beetle antennae search algorithm, IEEE Access, 8 (2020), 138788–138799. https://doi.org/10.1109/ACCESS.2020.3012564 doi: 10.1109/ACCESS.2020.3012564
![]() |
[42] | X. Li, H. Jiang, M. Niu, R. Wang, An enhanced selective ensemble deep learning method for rolling bearing fault diagnosis with beetle antennae search algorithm, Mech. Syst. Signal Process., 142 (2020), 106752. |
[43] | X. Li, Z. Zang, F. Shen, Y. Sun, Task offloading scheme based on improved contract net protocol and beetle antennae search algorithm in fog computing networks, Mobile Netw. Appl. 25 (2020), 2517–2526. https://doi.org/10.1109/ACCESS.2020.3012564 |
[44] |
Y. Fan, J. Shao, G. Sun, Optimized PID controller based on beetle antennae search algorithm for electro-hydraulic position servo control system, Sensors, 19 (2019), 2727. https://doi.org/10.3390/s19122727 doi: 10.3390/s19122727
![]() |
[45] |
S. D. Mourtas, V. N. Katsikis, V-shaped BAS: Applications on large portfolios selection problem, Comput. Econ., 60 (2022), 1353–1373. https://doi.org/10.1007/s10614-021-10184-9 doi: 10.1007/s10614-021-10184-9
![]() |
[46] |
V. N. Katsikis, S. D. Mourtas, Diversification of time-varying tangency portfolio under nonlinear constraints through semi-integer beetle antennae search algorithm, Appl. Math., 1 (2021), 63–73. https://doi.org/10.3390/appliedmath1010005 doi: 10.3390/appliedmath1010005
![]() |
[47] | P. Kim, MATLAB Deep Learning: With Machine Learning, Neural Networks and Artificial Intelligence, Apress: Berkeley, CA, USA, 2017. |
[48] | Transformer models for MATLAB, 2023. Available from: https://github.com/matlab-deep-learning/transformer-models |
[49] | Y. Zhang, X. Yu, L. Xiao, W. Li, Z. Fan, W. Zhang, Weights and structure determination of articial neuronets, in Self-Organization: Theories and Methods, New York, NY, USA: Nova Science, 2013. |
[50] | Z. Zhu, Z. Zhang, W. Man, X. Tong, J. Qiu, F. Li, A new beetle antennae search algorithm for multi-objective energy management in microgrid, in Proc. 13th IEEE Conf. Industrial Electronics and Applications (ICIEA), 2018, 1599–1603. |
[51] |
Q. Wu, X. Shen, Y. Jin, Z. Chen, S. Li, A. H. Khan, et al., Intelligent beetle antennae search for UAV sensing and avoidance of obstacles, Sensors, 19 (2019), 1758. https://doi.org/10.3390/s19081758 doi: 10.3390/s19081758
![]() |
[52] |
X. Xu, K. Deng, B. Shen, A beetle antennae search algorithm based on Lévy flights and adaptive strategy, Syst. Sci. Control. Eng., 8 (2020), 35–47. https://doi.org/10.1080/21642583.2019.1708829 doi: 10.1080/21642583.2019.1708829
![]() |
[53] | M. Davis, L. Iancu, Unicode text segmentation, Unicode Standard Annex, 29 (2018), 65. https://doi.org/10.4324/9780429955600-9 |
[54] | A. K. Gupta, Numerical methods using MATLAB, MATLAB solutions series, Apress: Berkeley, CA, USA, New York, NY, 2014. |
[55] |
A. Tharwat, Classification assessment methods, Appl. Comput. Inform., 17 (2020), 168–192. https://doi.org/10.1016/j.aci.2018.08.003 doi: 10.1016/j.aci.2018.08.003
![]() |
[56] |
M. L. McHugh, Interrater reliability: the kappa statistic, Biochem. Med., 22 (2012), 276–282. https://doi.org/10.11613/bm.2012.031 doi: 10.11613/bm.2012.031
![]() |