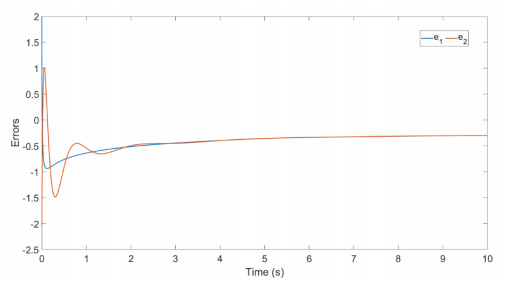
This paper aims to introduce the new concept of an α-θ-Geraghty type contraction mapping using CG-simulation in a metric-like space. Additionally, through this type of contraction, we establish fixed point results that generalize several known fixed point results in the literature. We provide some examples as an application that proves the credibility of our results.
Citation: Abdellah Taqbibt, M'hamed Elomari, Milica Savatović, Said Melliani, Stojan Radenović. Fixed point results for a new α-θ-Geraghty type contraction mapping in metric-like space via CG-simulation functions[J]. AIMS Mathematics, 2023, 8(12): 30313-30334. doi: 10.3934/math.20231548
[1] | Song Lunji . A High-Order Symmetric Interior Penalty Discontinuous Galerkin Scheme to Simulate Vortex Dominated Incompressible Fluid Flow. AIMS Mathematics, 2016, 1(1): 43-63. doi: 10.3934/Math.2016.1.43 |
[2] | Dong Pan, Huizhen Qu . Finite-time boundary synchronization of space-time discretized stochastic fuzzy genetic regulatory networks with time delays. AIMS Mathematics, 2025, 10(2): 2163-2190. doi: 10.3934/math.2025101 |
[3] | Ailing Zhu, Yixin Wang, Qiang Xu . A weak Galerkin finite element approximation of two-dimensional sub-diffusion equation with time-fractional derivative. AIMS Mathematics, 2020, 5(5): 4297-4310. doi: 10.3934/math.2020274 |
[4] | Tiantian Zhang, Wenwen Xu, Xindong Li, Yan Wang . Multipoint flux mixed finite element method for parabolic optimal control problems. AIMS Mathematics, 2022, 7(9): 17461-17474. doi: 10.3934/math.2022962 |
[5] | Zonghong Xiong, Wei Wei, Ying Zhou, Yue Wang, Yumei Liao . Optimal control for a phase field model of melting arising from inductive heating. AIMS Mathematics, 2022, 7(1): 121-142. doi: 10.3934/math.2022007 |
[6] | Şuayip Toprakseven, Seza Dinibutun . Error estimations of a weak Galerkin finite element method for a linear system of ℓ≥2 coupled singularly perturbed reaction-diffusion equations in the energy and balanced norms. AIMS Mathematics, 2023, 8(7): 15427-15465. doi: 10.3934/math.2023788 |
[7] | Essam R. El-Zahar, Ghaliah F. Al-Boqami, Haifa S. Al-Juaydi . Piecewise approximate analytical solutions of high-order reaction-diffusion singular perturbation problems with boundary and interior layers. AIMS Mathematics, 2024, 9(6): 15671-15698. doi: 10.3934/math.2024756 |
[8] | Zuliang Lu, Xiankui Wu, Fei Huang, Fei Cai, Chunjuan Hou, Yin Yang . Convergence and quasi-optimality based on an adaptive finite element method for the bilinear optimal control problem. AIMS Mathematics, 2021, 6(9): 9510-9535. doi: 10.3934/math.2021553 |
[9] | Junchi Ma, Lin Chen, Xinbo Cheng . Virtual element method for the Laplacian eigenvalue problem with Neumann boundary conditions. AIMS Mathematics, 2025, 10(4): 8203-8219. doi: 10.3934/math.2025377 |
[10] | Lingling Sun, Hai Bi, Yidu Yang . A posteriori error estimates of mixed discontinuous Galerkin method for a class of Stokes eigenvalue problems. AIMS Mathematics, 2023, 8(9): 21270-21297. doi: 10.3934/math.20231084 |
This paper aims to introduce the new concept of an α-θ-Geraghty type contraction mapping using CG-simulation in a metric-like space. Additionally, through this type of contraction, we establish fixed point results that generalize several known fixed point results in the literature. We provide some examples as an application that proves the credibility of our results.
In recent years, fractional calculus has gained considerable attention due to its enhanced capability to model and control complex dynamic systems more accurately than traditional integer-order derivatives. The Caputo fractional derivative has been extensively applied in fields that require modeling complex dynamic behaviors. For instance, in viscoelasticity, fractional derivatives capture the stress-strain relationship by incorporating time-dependent memory effects [1,2,3]. In biomedical applications, fractional calculus models physiological processes where past states influence current dynamics, as seen in cardiac tissue modeling and drug delivery systems [4]. The Caputo fractional derivative with respect to another function builds upon this utility by allowing systems to be defined in terms of an auxiliary function, which can be tailored to specific application requirements. This adaptability is crucial for applications where growth rates or system dynamics vary nonlinearly over time, such as in renewable energy management, where this approach enables enhanced stability in power grids under fluctuating loads [5]. These fields benefit from fractional derivatives due to their ability to incorporate historical data into system dynamics, enhancing accuracy in scenarios where memory effects are significant. Within control theory, the use of fractional derivatives has been proven advantageous in observer-based control designs. Previous studies have used Caputo derivatives to improve system performance by ensuring stability and error convergence under disturbances [6]. However, for complex nonlinear systems, obtaining accurate state information is crucial for ensuring stability, performance, and robustness in control. Observers serve this purpose by estimating the internal states of a system based on available outputs, which is especially important when direct measurements are impractical or noisy. Designing fractional-order observers for nonlinear systems provides a more adaptive and robust estimation framework, capturing the memory and hereditary effects inherent in fractional dynamics. This study aims to advance observer design by leveraging fractional-order calculus to improve estimation accuracy and stability in nonlinear systems.
Traditionally, the Caputo derivative has been used in its standard form, which depends on the time variable. A recent advance in fractional calculus is the Caputo fractional derivative with respect to another function, which generalizes the classical Caputo derivative by incorporating an additional function into its definition. This derivative offers a broader framework for modeling complex systems by allowing greater flexibility in selecting the governing function, making it suitable for systems with non-uniform time scales or nonlinear growth rates. Despite its theoretical potential, this derivative remains underexplored in control theory, particularly in observer design and stability analysis [7,8].
The Caputo fractional derivative is specifically chosen in this study due to several distinct advantages that make it well-suited for control applications, particularly in observer design for nonlinear systems. Here are the primary reasons for selecting the Caputo derivative:
● Compatibility with initial conditions: Unlike other fractional derivatives, such as the Riemann-Liouville derivative, the Caputo fractional derivative allows initial conditions to be specified in terms of classical integer-order derivatives. This compatibility simplifies the practical application of fractional calculus in control systems, where initial conditions are typically known and easier to set in classical terms.
● Memory effects: Fractional derivatives are known for their ability to model memory and hereditary properties of dynamic systems. The Caputo derivative, in particular, is advantageous in systems where past states impact current dynamics, such as in systems with memory, delays, or feedback loops. This property is especially useful in nonlinear systems where state history influences behavior, improving estimation accuracy in observer design.
● Flexibility with respect to another function**: This study leverages the Caputo fractional derivative with respect to another function, an extension that generalizes the Caputo derivative. This extension allows for even greater flexibility by enabling system dynamics to depend on an auxiliary function, making it applicable to systems with non-uniform time scales or nonlinear growth rates. This adaptability is important for real-world control systems that exhibit varying dynamics over time.
● Enhanced robustness for observer design**: The Caputo derivative's ability to capture fractional dynamics enhances robustness in the observer design process, particularly under disturbances or uncertainties. By incorporating fractional-order dynamics, observers can provide more accurate state estimations for nonlinear systems, which is crucial for achieving stability and control objectives.
The Caputo fractional derivative with respect to another function offers significant flexibility by allowing the derivative's evolution to depend on a secondary function rather than strictly the time variable. This feature is advantageous for modeling systems with non-uniform time scales or nonlinear growth rates, as it enables the model to mirror variable dynamics that would otherwise be challenging for standard fractional derivatives. For instance, in systems where changes do not progress uniformly—such as biological processes that vary with environmental conditions or economic models with fluctuating growth rates—this approach allows for a more accurate representation by selecting an auxiliary function that reflects these unique dynamics. This added adaptability enhances the derivative's capacity to capture complex behaviors in real-world systems, making it particularly useful in control and estimation applications for nonlinear and memory-dependent systems.
On the other hand, the concept of practical stability has become important in control theory to address the limitations of classical stability definitions. Classical stability requires system solutions to converge exactly to an equilibrium point. However, in many real-world scenarios, exact convergence is neither feasible nor necessary. Instead, it is sufficient for system solutions to remain within a small, bounded region around the equilibrium, especially in the presence of external disturbances or model uncertainties. Practical stability offers a more realistic approach to control by ensuring that system trajectories converge to a neighborhood of the desired point rather than requiring precise convergence [9,10,11,12]. This makes practical stability particularly suitable for applications such as robotics, where systems are subject to external forces, or in industrial control processes, where environmental disturbances are common. In this work, we extend the notion of practical stability to systems governed by the Caputo fractional derivative with respect to another function, providing a novel framework that enhances robustness in nonlinear systems.
Conversely, the one-side Lipschitz (OSL) condition has been extensively utilized in control theory to ensure the stability of nonlinear systems. The OSL condition facilitates the design of stable observers even in the presence of system nonlinearities [13,14,15,16,17]. In this paper, we extend the OSL condition to fractional-order systems by incorporating the Caputo fractional derivative with respect to another function. This extension is significant, as fractional-order systems exhibit different stability properties compared to their integer-order counterparts. Our extended OSL condition guarantees that the error between the actual state and the estimated state converges to a ball centered at the origin. This advance represents a substantial improvement in the design of fractional-order observers, providing a new stability criterion that is both more flexible and robust than existing methods.
Our proposed methodology, which integrates the Caputo fractional derivative with respect to another function, provides a more comprehensive and adaptable framework for controlling nonlinear systems. By extending the OSL condition and introducing practical stability criteria, we offer an innovative solution that is both resilient to disturbances and adaptable to diverse system dynamics. The theoretical approach presented in this paper, which applies Caputo fractional-order derivatives with respect to another function, represents a significant advancement in control theory with potential applications in various engineering fields. For instance, in robotics, this method can enhance stability and performance in dynamic environments, while in boiler turbine systems [22], it can be used to develop dynamic event-based tracking control strategies with guaranteed performance. Additionally, in energy management systems, our approach can optimize the performance of renewable energy sources, improving the stability and efficiency of the power grid. In biomedical engineering, it can ensure practical stability in drug delivery mechanisms and physiological control systems, maintaining desired therapeutic outcomes despite biological variability. Furthermore, in aerospace and automotive systems, the proposed methodologies can provide robust and precise control under uncertain conditions, enhancing safety and reliability. The implementation of these results into real-world applications is a future perspective, and we plan to collaborate with industry partners and researchers to develop practical implementations and validate the effectiveness of our theoretical findings in real-world settings.
While our work builds upon established concepts in fractional calculus and control theory, it introduces several novel contributions that advance the field. We have clearly delineated these contributions to highlight the scientific value of our research:
1. Novel application of Caputo derivatives with respect to another function: This paper is the first to apply the Caputo fractional derivative with respect to another function in the context of control theory. This generalization of the classical Caputo derivative allows for greater flexibility in modeling systems with non-uniform time scales and nonlinear growth rates, which has not been thoroughly explored in previous works.
2. Introduction of practical stability for nonlinear systems: We extend the concept of practical stability to systems governed by the Caputo fractional derivative with respect to another function. This extension provides a more realistic approach to stability analysis, ensuring that system solutions remain within a bounded region around the equilibrium point, which is crucial for real-world applications.
3. Extension of the one-sided Lipschitz (OSL) condition: Our work extends the OSL condition to fractional-order systems by incorporating the Caputo fractional derivative with respect to another function. This new stability criterion facilitates the design of stable observers and controllers for nonlinear systems, enhancing their robustness and adaptability.
4. Development of observer and controller design methodologies: We propose novel observer and controller design methodologies for fractional-order systems that satisfy the extended OSL condition. These methodologies provide a comprehensive framework for ensuring practical stability and robust performance in the presence of disturbances and model uncertainties.
5. Validation through numerical examples: The theoretical advancements are substantiated through detailed numerical examples that simulate real-world scenarios. These examples demonstrate the effectiveness of the proposed observer and controller designs, providing practical insights and validating the theoretical findings.
The remainder of this paper is organized as follows: Section 2 provides the necessary preliminaries and foundational concepts. In Section 3, we delve into the observer design for Lipschitz fractional-order systems. Section 4 extends the discussion to observer design for systems satisfying the OSL and quasi-OSL conditions. Section 5 focuses on the implementation of observer-based control strategies. Finally, Section 6 presents illustrative examples to validate the effectiveness of the proposed methodologies.
In this part, some preliminaries are presented [7,8].
Definition 1. Let δ∈C1[t0,b].The fractional integral w.r.t. δ of a function z is expressed by:
Ir,δt0,tz(t)=1Γ(r)t∫t0(δ(t)−δ(s))r−1δ'(s)z(s)ds,fort∈(t0,b], | (1) |
where z is a locally integrable function and r>0 is the order of the integral.
Definition 2. Let δ∈C1[t0,b]. The CDF w.r.t. δ of a function z of order 0<r<1 is given by:
CDr,δt0,tz(t)=1Γ(1−r)t∫t0(δ(t)−δ(s))−rz'(s)ds,fort0∈(1,b], | (2) |
where z is an absolutely continuous function.
Lemma 1. Let R be a matrix in a manner that R=RT>0. Then,
CDr,δt0,tzTRz(t)≤2zT(t)RCDr,δt0,tz(t), | (3) |
where 0<r<1.
Definition 3. The following Eα(z) function:
Eα(z)=∑∞k=0zkΓ(1+kα)andEα,β(z)=∑∞k=0zkΓ(β+kα),α>0,β>0. | (4) |
Named: "the Mittag-Leffler (ML) functions".
Consider the system:
CDr,δt0,tz(t)=ψ(z,t) for t≥1,rϵ(0,1]. | (5) |
Definition 4. Assume that m is a locally Lipschitz function. System (5) is said to be practically stable if ∃μ,γ>0 and r≥0 in a manner that ∀z(·) of (5), the inequality
‖z(t)‖≤(m(‖(z(1)‖)Eα(−μ(δ(t)−δ(t0))α))γ+r,t≥t0 |
is verified, with m(s)≥0,m(0)=0.
In our case study, the nonlinear system using Caputo derivatives with respect to another function is given by:
CDr,δt0,tz(t)=Az+Bu+f(z,u)+Bε(t),t≥t0, |
y=Cz, | (6) |
with fϵC(Rn×Rm,Rn), zϵRn, uϵRm, yϵRq, ε:[1,∞)→Rm, AϵRn×n, BϵRn×m, CϵRq×n. We have f(0,u)=0.
Remark 2.1. The motivation for considering system (6) stems from the need to address the complexities inherent in nonlinear dynamic systems, particularly those exhibiting non-uniform time scales and nonlinear growth rates. Traditional integer-order models often fall short of accurately capturing the dynamics of such systems, especially when historical influences play a significant role. System (6) leverages the Caputo fractional derivative with respect to another function, which provides a more flexible and comprehensive framework for modeling and control. This derivative allows for the incorporation of an additional function into the derivative's definition, enabling the modeling of systems with varying time scales and growth rates more effectively. The specific form of system (6) is chosen to illustrate the practical applicability of the proposed observer and controller design methodologies. By incorporating the Caputo fractional derivative with respect to another function, we can extend the classical stability and observer design techniques to a broader class of systems. This extension is crucial for applications in fields such as robotics, autonomous systems, and industrial process control, where robustness and adaptability to disturbances are paramount.
Assumption 1. ε is a continuous function that satisfies
∥ε(t)∥≤Cε(t),t≥t0, |
where ε is an unknown disturbance and δε is a continuous function in a manner that:
gl(t)=t∫t0(δ(t)−δ(s))r−1δ'(s)Er,r(−l(δ(t)−δ(s))α)Cε2(s)ds |
is a bounded function for some l > 0.
In this part, we present the findings pertaining to the observer design for systems where the nonlinear component satisfies the Lipschitz condition.
Assumption 2. The pair (A, C) is observable and ∃θ>0 in a manner that:
−θS−ATS−SA+CTC=0, | (7) |
with ST=S>0.
Definition 5. f(x,u) is said to be a Lipschitz function, indicating that ∃m>0 in a manner that ∀z1,z2∈Rn,u∈Rm:
‖f(z1,u)−f(z2,u)‖≤m‖z1−z2‖, | (8) |
where r is the Lipschitz constant and f is a continuous function.
Based on the system outlined in Eq (5), we suggest the following observer based on Caputo derivatives with respect to another function to estimate the state:
CDr,δt0,tˆz(t)=Aˆz+Bu+f(ˆz,u)−βS−1CT(Cˆz−y),t≥t0, | (9) |
with β≥1.
Theorem 3.1. If condition (8) is verified and Assumption 2 is fulfilled, and if Assumption 1 is met for
l=λmin(θS+(2β−1)CTC)−2mλmax(S)−η>0, | (10) |
with η>0, consequently, the origin of the error system between the actual state of the system described in Eq (5) and the estimated states produced by the observer in Eq (9) is practically stable.
Proof. One supposes
e=ˆz−z. |
Then,
CDr,δt0,te(t)=Aˆz+Bu+f(ˆz,u)−βS−1CT(Cˆz−y)−Az−Bu−f(z,u)−Bε(t) |
=(A−βS−1CTC)e+Δf−Bε(t), |
where Δf=f(ˆz,u)−f(z,u). One supposes:
V(e)=eTSe. |
Based on Lemma 1 and substituting the error dynamics into the Lyapunov function derivative, we get:
CDr,δt0,tV(e)≤2eT(t)SCDαt0,te(t),∀t≥t0,≤[eT(A−βS−1CTC)T+ΔfT]Se+eTS[(A−βS−1CTC)e+Δf]−2eTSBε(t)≤eT[(A−βS−1CTC)TS+S(A−βS−1CTC)]e+2eTSΔf−2eTSBε(t)≤eT(ATS−2βCTC+SA)e+2eTSΔf−2eTSBε(t). |
We have
CDr,δt0,tV(e)≤−θeTSe+(1−2β)eTCTCe+2eTSΔf−2eTSBε(t). |
And so
CDr,δt0,tV(e)≤−θeTSe+(1−2β)eTCTCe+2‖e‖‖S‖‖Δf‖+2‖S‖‖B‖‖ε(t)‖‖e‖. |
Using the Lipschitz condition, we have
CDr,δt0,tV(e)≤−θeTSe+(1−2β)eTCTCe+2m‖e‖2‖S‖+2‖S‖‖B‖‖ε(t)‖‖e‖. |
Using Assumption 1, we deduce that
CDr,δt0,tV(e)≤−θeTSe+(1−2β)eTCTCe+2m‖e‖2‖S‖+2Cε(t)‖S‖‖B‖‖e‖. |
Let μ(t)=Cε(t)∥S∥∥B∥. Using the fact that
2μ(t)∥e∥≤μ2(t)η+η∥e∥2. |
Then using (8), one can have
CDr,δt0,tV(e)≤−λmin(θS+(2β−1)CTC)‖e‖2+2mλmax(S)‖e‖2+μ2(t)η+η∥e∥2. |
For practical stability, we require: λmin(θS+(2β−1)CTC)−2mλmax(S)−η>0.
Thus, if (10) is fulfilled, we have CDr,δt0,tV(e)≤−l‖e‖2+μ2(t)η.
Analogous to the demonstration of [18, Proposition 3.1], the result of Theorem 3.1 is reached.
Below is the observer-based Caputo derivatives with respect to another function design algorithm for Lipschitz systems:
Input: System matrices A,B,C disturbance function ε(t)
● Step 1: Check the Lipschitz condition, ensure f(z,u) satisfies the Lipschitz constant m.
● Step 2: Solve the matrix inequality in Theorem 3.1 to find S.
● Step 3: Design the observer using Eq (4) with feedback gain based on β.
Output: Estimated state ˆz.
In this section, we present the findings pertaining to the observer design for systems where the nonlinear component satisfies the one-sided Lipschitz (OSL) condition and the quasi-one-sided Lipschitz (quasi-OSL) condition.
Definition 6. f(z,u) is said to be OSL in Rn, that is to say ∀z,ˆz∈Rnandu∈Rm, the following inequality holds:
⟨Sf(z,u)−Sf(ˆz,u),z−ˆz⟩≤v‖z−ˆz‖2, | (11) |
where v is the OSL constant.
Theorem 4.1. If Assumption 1 is verified and if condition (11) is satisfied and if
l=λmin(θS+(2β−1)CTC)−2v−η>0, | (12) |
consequently, the origin of the error system between the actual state of the system described in Eq (1) and the estimated states produced by the observer in Eq (4) is practically stable.
Proof. The proof of Theorem 4.1 employs a methodology akin to that used for Theorem 3.1. It is assumed that:
e=ˆz−z. |
So,
CDr,δt0,te(t)=(A−βS−1CTC)e+Δf−Bε(t). |
Consider V(e)=eTSe. Based on Lemma 1, we define the Lyapunov function:
V(e)=eTSe. |
Substituting the error dynamics into the Lyapunov function derivative, we get:
CDr,δt0,tV(e)≤2eT(t)SCDαt0,te(t),∀t≥t0,≤[eT(A−βS−1CTC)T+ΔfT]Se+eTS[(A−βS−1CTC)e+Δf]−2eTSBε(t)≤eT[(A−βS−1CTC)TS+S(A−βS−1CTC)]e+2eTSΔf−2eTSBε(t)≤eT(ATS−2βCTC+SA)e+2eTSΔf−2eTSBε(t). |
Now using (7), we have
CDr,δt0,tV(e)≤−θeTSe+(1−2β)eTCTCe+2eTSΔf−2eTSBε(t). |
So using Assumption 1, we deduce that
CDr,δt0,tV(e)≤−θeTSe+(1−2β)eTCTCe+2eTSΔf+2Cε(t)‖S‖‖B‖‖e‖. |
Let μ(t)=Cε(t)∥S∥∥B∥. Using the fact that
2μ(t)∥e∥≤μ2(t)η+η∥e∥2. |
Then, using Assumption (11), one can have
CDr,δt0,tV(e)≤−λmin(θS+(2β−1)CTC)‖e‖2+2v‖e‖2+μ2(t)η+η∥e∥2. |
For practical stability, we require: λmin(θS+(2β−1)CTC)−2v−η>0.
So, if (12) is fulfilled, we have CDr,δt0,tV(e)≤−l‖e‖2+μ2(t)η.
So, the origin of the error system between the actual state of the system described in Eq (6) and the estimated states produced by the observer in Eq (9) is practically stable.
We now introduce a quasi-OSL condition:
Definition 7. f(z,u) is said to be a quasi-OSL in Rn with an OSL constant matrix M in a manner that MT=M, i.e.,
⟨Sf(z,u)−Sf(ˆz,u),z−ˆz⟩≤(z−ˆz)TM(z−ˆz),∀z,ˆz∈Rn,u∈Rm. | (13) |
Theorem 4.2. If condition (8) and Assumption 1 are verified and if
l=λmin(θS+(2β−1)CTC)−2λmax(M)−η>0. | (14) |
So, the origin of the error system between the actual state of the system described in Eq (5) and the estimated states produced by the observer in Eq (9) is practically stable.
Proof. We assume V(e)=eTSe with e=ˆz−z, we get:
CDr,δt0,tV(e)≤−θeTSe+(1−2β)eTCTCe+2eTSΔf−2eTSBε(t). |
Then using (13), one can have
CDr,δt0,tV(e)≤−λmin(θS+(2β−1)CTC)‖e‖2+2eTMe+2Cε(t)‖S‖‖B‖‖e‖ |
≤−[λmin(θS+(2β−1)CTC)−2λmax(M)−η]‖e‖2+μ2(t)η. |
Then, the origin of the error system between the actual state of the system described in Eq (5) and the estimated states produced by the observer in Eq (9) is practically stable.
In this section, we introduce the separation principle technique for observer-based control of system (5).
Assumption 2. We assume that (A, B) is stabilizable. ∃K in a manner thatRe(λ(A+BK))<0, and a function named Lyapunov function can be expressed as zTPz, where P=PT>0, in a manner that
P(A+BK)+(A+BK)TP=−Q,Q>0. | (15) |
We introduce the following result.
Lemma 2. One supposes that conditions 2 and 3 are fulfilled, and m verifies the following inequality:
−l=−λmin(Q)+2mλmax(P)+η<0. | (16) |
Consequently, the control law u(z)=Kz ensures that the origin of the system described in Eq (5) is practically stable.
Proof. Solving (15) for P>0. One supposes Q=QT>0 and u(z)=Kz. One defines V1(z)=zTPz. One obtains:
CDr,δt0,tV1(z)≤−zTQz+2zTPf(z,Kz)+2zTPBε(t). |
Based on Assumption (15), condition 1 and recognizing that f(0,u)=0,
CDr,δt0,tV1(x)≤[−λmin(Q)+2mλmax(P)]‖z‖2+2Cε(t)‖P‖‖B‖‖z‖. |
Let μ(t)=Cε(t)∥P∥∥B∥. Using the fact that
2μ(t)∥z∥≤μ2(t)η+η∥z∥2. |
Yields,
CDr,δt0,tV1(z)≤[−λmin(Q)+2mλmax(P)]‖z‖2+μ2(t)η+η∥z∥2, |
CDr,δt0,tV1(z)≤[−λmin(Q)+2mλmax(P)+η]‖z‖2+μ2(t)η. |
So if (16) is verified, the result of Lemma 2 is reached.
Below is the practical controller design algorithm-based Caputo derivatives with respect to another function.
Input: System matrices A,B
● Step 1: Solve the Lyapunov Eq (15) to find P and K.
● Step 2: Apply the control law u(z)=Kz to stabilize the system.
● Step 3: Verify the stability conditions (Lemma 2).
Output: State of the system z.
Now, consider Q=I with u(ˆx)=Kˆx.
Theorem 5.1. If the condition (7) is verified and if conditions (8) and (15) are verified for
l=λmin(θS+(2β−1)CTC)−2mλmax(S)−η>0, |
and if
m<12inf(1λmax(P),λmin(θS+(2β−1)CTC)−ηλmax(S)),β≥1, | (17) |
then the system
CDr,δt0,tˆz(t)=Aˆz+BKˆz+f(ˆz,Kˆz)−βS−1CTCe,t≥t0, |
CDr,δt0,te(t)=(A−βS−1CTC)e+f(ˆz,Kˆz)−f(ˆz−e,Kˆz)−Bε(t),t≥t0, | (18) |
is practically stable.
Proof. One supposes V1(ˆx)=ˆzTPˆz and V2(e)=eTSe.
One supposes the function
V(ˆz,e)=ςV1(ˆz)+V2(e), |
where μ>0. Then, we have
CDr,δt0,tV(ˆz,e)=ςCDr,δt0,tV1(ˆz)+CDr,δt0,tV2(e). |
From Lemma 1, it follows that
CDr,δt0,tV1(ˆz)≤ˆzT[P(A+BK)+(A+BK)TP]ˆz+2ˆzTPf(ˆz,Kˆz)−2βˆzTPS−1CTCe. |
Now, we have:
CDr,δt0,tV1(ˆz)≤−‖ˆz‖2+2ˆzTPf(ˆz,Kˆz)+2βλmax(P)‖ˆz‖‖S−1‖‖CTC‖‖e‖, |
knowing that f(0,u)=0 and using condition (8), So
CDr,δt0,tV(ˆz,e)≤ς[−1+2mλmax(P)]‖ˆz‖2+2ςβλmax(P)‖S−1‖‖CTC‖‖e‖‖ˆz‖+CDr,δt0,tV2(e). |
If (17) satisfied, we present in the proof of theorem that
CDr,δt0,tV2(e)≤−l‖e‖2+μ2(t)η. |
So, one has
CDr,δt0,tV(ˆz,e)≤−ςa‖ˆz‖2+2ςb‖e‖‖ˆz‖−l‖e‖2+μ2(t)η, |
where
a=1−2mλmax(P)>0, |
b=βλmax(P)‖S−1‖‖CTC‖>0. |
Thus, if ς is chosen in a manner that ς<al/b2, the result of Theorem 5.1 is reached.
The examples selected for this study aim to validate the theoretical contributions in the design of the observer and controller for nonlinear fractional-order systems with respect to another function. The purpose is to showcase the effectiveness of the proposed methods through simulation. Each example highlights a particular aspect of the theoretical findings:
● Example 1 illustrates the relevance of the observer design as outlined in Theorem 3.1, highlighting the practical stability of the system error.
● Examples 2 and 3 expand upon the methodology to incorporate an observer-based controller design, demonstrating the efficacy of the integrated observer and controller in maintaining the practical stability of the system.
The choice of these examples ensures that theoretical findings are effectively validated and that the simulations provided are clear.
The parameters for the systems, such as the matrices A, B, and C, as well as the initial conditions for the state variables z and ˆz, were chosen based on typical values used in control theory literature and previous studies [14]. These parameters were selected to represent realistic scenarios and to ensure that the systems exhibit the desired dynamic behavior for testing the proposed methodologies. The simulations were conducted using MATLAB and Simulink, which provide robust functions for solving differential equations and visualizing the results. The Caputo fractional derivative with respect to another function was implemented using a discretization approach that approximates the continuous-time dynamics. The simulation environment was configured to accurately model the fractional-order systems and to ensure the reliability of the results. While we have provided initial validation through numerical examples, a comprehensive sensitivity analysis is identified as a future perspective. This analysis will require further mathematical development to rigorously prove the robustness of the proposed observer and controller designs under varying parameters. We plan to address this in future work to provide a deeper understanding of the system's behavior and stability.
The implementation of these results into real-world applications is a future perspective. We plan to collaborate with industry partners and researchers to develop practical implementations and validate the effectiveness of our theoretical findings in real-world settings. For examples of these applications, see [19,20,21]. The control of boiler turbine systems is a complex task due to the nonlinear dynamics and the presence of disturbances [22]. Our approach can be applied to develop dynamic event-based tracking control strategies for boiler turbine systems, ensuring guaranteed performance. By incorporating the Caputo fractional derivative with respect to another function, we can design controllers that maintain the desired performance despite variations in operating conditions and external disturbances.
Example 1. We consider the following system based on Caputo derivatives with respect to another function:
CDr,δt0,tz1(t)=−7z2+u+0.02cos(z2)+1t2+4,t≥0, |
CDr,δt0,tz2(t)=z1+0.03cos(z1),t≥0, | (19) |
where
z=(z1,z2)T∈R2 and δ(t)=t4+t. |
By solving (2), we find:
S=[5.7161005.7161]=ST>0, |
with θ=5.2.
One chooses β=1 and u=1.
The Lipschitz constant m equals to 0.03.
All conditions of Theorem 3.1 are satisfied. By setting z10=4,z20=−4 and ˆz10=−2,ˆz20=2, we have the following simulation results.
These evolutions, displayed in Figure 1, indisputably demonstrate the practical stability of all signals, therefore confirming the theoretical findings of Theorem 3.1.
Example 2. We consider the following system based on Caputo derivatives with respect to another function:
CDr,δt0,tz1(t)=3z2+u+0.1sin(z2)+t2t2+1,t≥1, |
CDr,δt0,tz2(t)=z1+0.1sin(z1),t≥1, | (20) |
where z=(z1,z2)T∈R2 and δ(t)=tlog(t).
Using Lemma 2, u(z)=−4z2−z1 practically stabilizes the linear part of system (18). One chooses Q=I and one solves (15), One obtains:
P=[1.000.500.501.50]=PT>0. |
One has r=0.111<λmin(Q)2λmax(P)≃0.2769.
By solving (7), we find:
S=[0.1660−0.0811−0.08110.0695]=ST>0, |
with θ=7.
(17) holds because m=0.1<λmin(θS+CTC)2λmax(S)≃0.736, where β=1. So, u(ˆz)=−4ˆz2−ˆz1 practically stabilizes system (18), which confirms the result of Theorem 5.1
By setting z10=−2,z20=−1 and ˆz10=2,ˆz20=1, Figures 2 and 3 illustrate the evolution of the error curves e1 and e2 for α=0.5. These evolutions indisputably demonstrate the practical stability of all signals, therefore confirming theoretical findings.
Example 3. One supposes the following system based on Caputo derivatives with respect to another function:
CDr,δt0,tz1(t)=2z2+(17)√z12+z22+tt2+1,t≥1, |
CDr,δt0,tz2(t)=z1+u+(17)sin(z1),t≥0, | (21) |
with z=(z1,z2)T∈R2 and δ(t)=t2+t.
We can present system (21) as CDr,δt0,tx(t)=Ax+Bu+f(x,u)+Bε(t), where:
A=[0210],B=[01],f(z,u)=17[√z12+z22sin(z1)]andε(t)=tt2+1. |
Using Lemma 2, u(z)=−4z2−z1 practically stabilizes the linear part of system (21). One chooses Q=I and one solves (15), we have :
P=[1.1250.2510.2511]=PT>0. |
One has r=1/7≃0.14145<λmin(Q)2λmax(P)≃0.378.
By solving (7), we find:
S=[0.2327−0.105−0.1050.0808]=ST>0, |
with θ=5.2.
(17) holds because m≃0.14<λmin(θS+CTC)2λmax(S)≃0.4669, where β=1. So, u(ˆz)=−3ˆz1−ˆz2 practically stabilizes system (18), which confirm the result of Theorem 5.1
By setting z10=2,z20=−2 and ˆz10=−2,ˆz20=2, Figures 4 and 5 illustrate the evolution of the error curves e1 and e2 for α=0.5. These numbers clearly show that all signals are practically stable, supporting the theoretical results.
In conclusion, this work pioneers the application of the CDF with respect to another function in control theory, expanding the capabilities of the CH derivative. By introducing practical stability for nonlinear systems governed by this derivative, the study guarantees that system solutions approach and remain within a bounded region near the origin. Additionally, extending the OSL condition to fractional-order systems provides a solid foundation for observer design and observer-based control, boosting the adaptability and efficiency of control techniques. The theoretical advancements are substantiated through numerical examples, demonstrating the practical applicability and potential of the proposed framework in advancing control theory. Building on this framework, future research can address several key challenges and avenues for improvement. One area of interest lies in the analytical and computational challenges associated with fractional-order systems governed by Caputo derivatives with respect to other functions. Developing more efficient computational methods for these systems will be essential for implementing these theories in real-time applications, where computational complexity could impact performance. Additionally, exploring different classes of auxiliary functions in the Caputo derivative framework could expand the applicability of our methods, allowing for a finer tuning of system responses across various applications. Expanding these theories to a wider range of systems and scenarios is another promising direction. For instance, applying our observer-based control framework in the fields of renewable energy, autonomous vehicles, and biomedical systems could yield substantial insights. In renewable energy, real-time stability control of power grids could be achieved by adapting our framework to handle time-varying loads and nonlinearities in grid dynamics. Similarly, the field of robotics, particularly in autonomous navigation, could benefit from robust, memory-inclusive observer designs that handle unpredictable environmental disturbances. Lastly, conducting a sensitivity analysis on the stability of fractional-order systems under varying parameters is crucial for understanding the robustness of our approach in diverse practical settings. Further work in this direction will allow us to develop adaptive control strategies that can respond dynamically to parameter shifts and disturbances. We aim to collaborate with industry and research partners to implement and validate our framework in these complex real-world settings, refining the theoretical foundations to ensure reliability and performance.
Kareem Alanazi: Conceptualization, methodology, software, writing-original draft preparation; Omar Naifar: Supervision, validation, writing-review & editing, project administration; Raouf Fakhfakh: Formal analysis, investigation; Abdellatif Ben Makhlouf: Resources, visualization, writing-review & editing. All authors have read and approved the final version of the manuscript for publication.
The authors declare they have not used Artificial Intelligence (AI) tools in the creation of this article.
This research is funded by the Deanship of Graduate Studies and Scientific Research at Jouf University through the Fast-Track Research Funding Program.
The authors declare no conflicts of interest regarding this manuscript.
[1] |
H. Aydi, A. Felhi, E. Karapinar, S. Sahmim, A Nadler-type fixed point theorem in dislocated spaces and applications, Miskolc Math. Notes, 19 (2018), 111–124. http://dx.doi.org/10.18514/MMN.2018.1652 doi: 10.18514/MMN.2018.1652
![]() |
[2] |
S. Chandok, Some fixed point theorems for (α,θ)-admissible Geraghty type contractive mappings and related results, Math. Sci., 9 (2015), 127–135. https://doi.org/10.1007/s40096-015-0159-4 doi: 10.1007/s40096-015-0159-4
![]() |
[3] | A. A. Harandi, Metric-like spaces, partial metric spaces and fixed points, Fixed Point Theory A. 2012 (2012), 1–10. https://doi.org/10.1186/1687-1812-2012-204 |
[4] | A. H. Ansari, Note on ϕ-ψ-contractive type mappings and related fixed point, In: The 2nd Regional Conference on Math. Appl. PNU, 11 (2014), 377–380. |
[5] | P. Kumam, D. Gopal, L. Budha, A new fixed point theorem under Suzuki type Z-contraction mappings, J. Math. Anal., 8 (2017), 113–119. |
[6] | M. Geraghty, On contractive mappings, Proc. Amer. Math. Soc., 40 (1973), 604–608. |
[7] |
A. Taqbibt, M. Chaib, M. Elomari, S. Melliani, Fixed point theorem for a new SF-GF-contraction mappings in metric space with supportive applications, Filomat, 37 (2023), 7953–7969. https://doi.org/10.2298/FIL2323953T doi: 10.2298/FIL2323953T
![]() |
[8] |
F. Khojasteh, S. Shukla, S. Radenović, A new approach to the study of fixed point theorems via simulation functions, Filomat, 26 (2015), 1189–1194. https://doi.org/10.2298/FIL1506189K doi: 10.2298/FIL1506189K
![]() |
[9] |
M. Olgun, O. Bicer, T. Alyildiz, A new aspect to Picard operators with simulation functions, Turkish J. Math., 40 (2016), 832–837. https://doi.org/10.3906/mat-1505-26 doi: 10.3906/mat-1505-26
![]() |
[10] |
A. Rold, E. Karapinar, C. Rold, J. Martinez, Coincidence point theorems on metric spaces via simulation function, J. Comput. Appl. Math., 275 (2015), 345–355. https://doi.org/10.1016/j.cam.2014.07.011 doi: 10.1016/j.cam.2014.07.011
![]() |
[11] | S. Chandok, A. Chanda, L. K. Dey, M. Pavlović, S. Radenović, Simulation functions and Geraghty type results, Bol. Soc. Paran. Mat., 39 (2021), 35–50. https://doi.org/10.5269/bspm.40499 |
[12] |
E. Karapinar, Fixed points results via simulation functions, Filomat, 30 (2016), 2343–2350. https://doi.org/10.2298/FIL1608343K doi: 10.2298/FIL1608343K
![]() |
[13] |
A. Taqbibt, M. Elomari, S. Melliani, Nonlocal semilinear ϕ-Caputo fractional evolution equation with a measure of noncompactness in Banach space, Filomat, 37 (2023), 6877–6890. https://doi.org/10.2298/FIL2320877T doi: 10.2298/FIL2320877T
![]() |
[14] |
S. Etemad, M. M. Matar, M. A. Ragusa, S. Rezapour, Tripled fixed points and existence study to a tripled impulsive fractional differential system via measures of noncompactness, Mathematics, 10 (2021), 25. https://doi.org/10.3390/math10010025 doi: 10.3390/math10010025
![]() |
[15] |
H. Alsamir, M. S. Noorani, W. Shatanawi, H. Aydi, H. Akhadkulov, H. Qawaqneh, et al., Fixed point results in metric-like spaces via σ-simulation functions, Eur. J. Pure Appl. Math., 12 (2019), 88–100. https://doi.org/10.29020/nybg.ejpam.v12i1.3331 doi: 10.29020/nybg.ejpam.v12i1.3331
![]() |
[16] |
S. Abbas, M. Benchohra, S. Krim, Initial value problems for caputo-fabrizio implicit fractional differntial equations in b-metrice spaces, Bull. Transilv. Univ. Bras., 63 (2021), 1–12. https://doi.org/10.31926/but.mif.2021.1.63.1.1 doi: 10.31926/but.mif.2021.1.63.1.1
![]() |
[17] |
B. Samet, C. Vetro, P. Vetro, Fixed point for α-ψ-contractive type mappings, Nonlinear Anal., 75 (2012), 2145–2165. https://doi.org/10.1016/j.na.2011.10.014 doi: 10.1016/j.na.2011.10.014
![]() |
[18] |
E. Karapinar, P. Kumam, P. Salimi, On α-ψ-Meri-Keeler contractive mappings, Fixed Point Theory A., 2013 (2013), 1–12. https://doi.org/10.1186/1687-1812-2013-94 doi: 10.1186/1687-1812-2013-94
![]() |
[19] |
S. Radenović, F. Vetro, J. Vujaković, An alternative and easy approach to fixed point results via simulation functions, Demonstr. Math., 50 (2017), 223–230. https://doi.org/10.1515/dema-2017-0022 doi: 10.1515/dema-2017-0022
![]() |
[20] |
H. Faraji, D. Savić, S. Radenović, Fixed point theorems for Geraghty contraction type mappings in b-metric spaces and applications, Axioms, 8 (2019), 34. https://doi.org/10.3390/axioms8010034 doi: 10.3390/axioms8010034
![]() |
[21] | X. L. Liu, A. H. Ansari, S. Chandok, S. Radenović, On some results in metric spaces using auxiliary simulation functions via new functions, J. Comput. Anal. Appl., 24 (2018), 1103–1114. |
[22] | N. Chefnaj, A. Taqbibt, K. Hilal, S. Melliani, A. Kajouni, Boundary value problems for differential equations involving the generalized Caputo-Fabrizio fractional derivative in λ-metric space, Turk. J. Sci., 8 (2023), 24–36. |
[23] | A. S. Anjum, C. Aage, Common fixed point theorem in F-metric spaces, J. Adv. Math. Stud., 15 (2022), 357–365. |
[24] |
A. Taqbibt, M. Chaib, M. Elomari, S. Melliani, Fixed point results for a new multivalued Geraghty type contraction via CG-simulation functions, Filomat, 37 (2023), 9709–9727. https://doi.org/10.2298/FIL2328709T doi: 10.2298/FIL2328709T
![]() |
[25] | G. S. Saluja, Some common fixed point theorems on S-metric spaces using simulation function, J. Adv. Math. Stud., 15 (2022), 288–302. |
1. | Divay Garg, Kamana Porwal, Unified discontinuous Galerkin finite element methods for second order Dirichlet boundary control problem, 2023, 185, 01689274, 336, 10.1016/j.apnum.2022.12.001 | |
2. | Kumar Rajeev Ranjan, S. Gowrisankar, Error analysis of discontinuous Galerkin methods on layer adapted meshes for two dimensional turning point problem, 2024, 70, 1598-5865, 2453, 10.1007/s12190-024-02054-y |