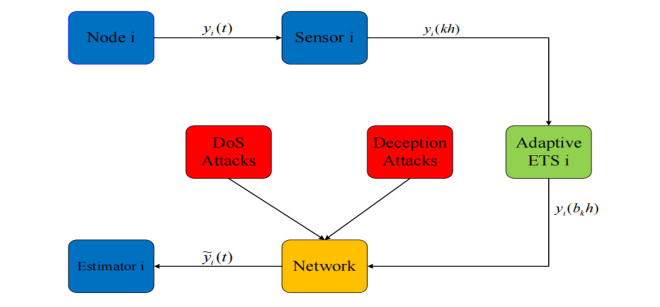
Citation: Afis Saliu, Khalida Inayat Noor, Saqib Hussain, Maslina Darus. Some results for the family of univalent functions related with Limaçon domain[J]. AIMS Mathematics, 2021, 6(4): 3410-3431. doi: 10.3934/math.2021204
[1] | Qiushi Wang, Hongwei Ren, Zhiping Peng . Event-triggered impulsive control for second-order nonlinear multi-agent systems under DoS attacks. AIMS Mathematics, 2024, 9(6): 13998-14011. doi: 10.3934/math.2024680 |
[2] | Chantapish Zamart, Thongchai Botmart, Wajaree Weera, Prem Junsawang . Finite-time decentralized event-triggered feedback control for generalized neural networks with mixed interval time-varying delays and cyber-attacks. AIMS Mathematics, 2023, 8(9): 22274-22300. doi: 10.3934/math.20231136 |
[3] | Jiaqi Liang, Zhanheng Chen, Zhiyong Yu, Haijun Jiang . Fixed-time consensus of second-order multi-agent systems based on event-triggered mechanism under DoS attacks. AIMS Mathematics, 2025, 10(1): 1501-1528. doi: 10.3934/math.2025070 |
[4] | Sheng-Ran Jia, Wen-Juan Lin . Adaptive event-triggered reachable set control for Markov jump cyber-physical systems with time-varying delays. AIMS Mathematics, 2024, 9(9): 25127-25144. doi: 10.3934/math.20241225 |
[5] | Kairui Chen, Yongping Du, Shuyan Xia . Adaptive state observer event-triggered consensus control for multi-agent systems with actuator failures. AIMS Mathematics, 2024, 9(9): 25752-25775. doi: 10.3934/math.20241258 |
[6] | Zhaohui Chen, Jie Tan, Yong He, Zhong Cao . Decentralized observer-based event-triggered control for an interconnected fractional-order system with stochastic Cyber-attacks. AIMS Mathematics, 2024, 9(1): 1861-1876. doi: 10.3934/math.2024091 |
[7] | Chengbo Yi, Rui Guo, Jiayi Cai, Xiaohu Yan . Pinning synchronization of dynamical neural networks with hybrid delays via event-triggered impulsive control. AIMS Mathematics, 2023, 8(10): 25060-25078. doi: 10.3934/math.20231279 |
[8] | Biwen Li, Guangyu Wang . Stability of stochastic nonlinear systems under aperiodically intermittent state quantization and event-triggered mechanism. AIMS Mathematics, 2025, 10(4): 10062-10092. doi: 10.3934/math.2025459 |
[9] | Yuanyuan Cheng, Yuan Li . A novel event-triggered constrained control for nonlinear discrete-time systems. AIMS Mathematics, 2023, 8(9): 20530-20545. doi: 10.3934/math.20231046 |
[10] | Saravanan Shanmugam, Mohamed Rhaima, Hamza Ghoudi . Exponential synchronization analysis for complex dynamical networks with hybrid delays and uncertainties under given control parameters. AIMS Mathematics, 2023, 8(12): 28976-29007. doi: 10.3934/math.20231484 |
In recent decades, the development of information technology has brought great portability to people's production and life [1]. CNs can be used to portray smart grids, intelligent transportation, social networks and neural systems, etc., and have been given significant research significance and wide application context by academia and industry [2]. It is well known that nodes and connection relationships are two key factors that constitute CNs [3]. For example, in a power system network, power plants are connected to each other by transmission lines, where power plants can be abstractly considered as nodes, and similarly, transmission lines between power plants can be considered as connection relations [4]. For a multi-robot system, each mobile robot is considered as a node in the network, and the mutual sensing and intercommunication between robots are seen as the connection relationship. When the number of controlled objects is large, the cooperation and formation maintenance for the multi-robot system can be studied with the help of the theory of CNs. Similarly, the above ideas are also applicable to the study of multi-UAS. It can be seen that the connection relations, nodes, and the interactions between connection relations and nodes constitute CNs [5]. From the perspective of control discipline, some works such as state estimation, synchronization and control, and topology identification of CNs are still widely studied [6,7,8]. In addition, many practical problems are inevitable in information transmission, such as channel redundancy, cyber-attacks, time delay, packet loss, and noise [9,10]. The authors in [7] studied the partial-nodes-based state estimation problem on estimating the entire network state using partial outputs of the available nodes. In this paper, the impact of cyber-attacks and channel redundancy on the state estimation for CNs is discussed.
In practical engineering, the network bandwidth available to a system is usually limited [11,12]. Due to the limitation of network resources, channel redundancy, data congestion, network-induced delay, and packet loss inevitably occur when the components within the system perform network access and information transmission [13,14]. To mitigate the impact of these problems on system performance, various data transmission mechanisms have been proposed, such as event-triggered schemes (ETSs), communication protocol scheduling, and codec strategies. Currently, ETS is a common data transmission scheduling strategy in networked systems [15,16]. The core idea of the scheme is to determine in real time whether the current system state satisfies the triggering conditions to control the task execution on demand and meet the system performance [17]. On this basis, data transmission strategies such as distributed ETS, AETS, self-triggered scheme, and dynamic ETS have been proposed one after another [18,19,20,21]. By introducing the AETS, a fuzzy dynamic output feedback controller is designed for the nonlinear system with actuator failure and packet loss [20]. In [21], the authors propose a co-design method for filter and distributed AETS for nonlinear interconnected systems.
ETSs bring great convenience to networked systems, but open networks are vulnerable to malicious attackers, which poses a potential security risk to networked systems [22,23]. In networked systems, network attacks appear in various ways and affect or even destroy system performance, such as DoS attacks and deception attacks [24]. DoS attacks: attackers can block the transmission of information in the communication network between sensors and controllers (estimators), resulting in no available data [25]. Deception attack: attackers can tamper with the available data received by the controller (estimator) from the sensor, thus corrupting the integrity of the data [26]. In recent years, the security of networked systems has attracted widespread attention from the community, for example, on May 11, 2021, Belgium's public sector Internet service provider Belnet was subjected to a massive distributed DoS attack, which took all internal systems of the Belgian government and public-facing websites offline and forced many government websites and services in Belgium offline; on August 1, 2021, Italy The local vaccination appointment system was forced to shut down due to the cyber-attacks. In [10], the authors investigated the state estimation problem for a class of uncertain complex networks with partial node failures resistant to deception attacks. A co-design approach for dynamic ETS and observer-based PID controller against deception attacks has been presented in [27]. In [28], the authors studied the problem of state estimation for cyberphysical systems constrained by communication resources, DoS attacks, and sensor saturation. The authors in [29] presented event-triggered control countermeasures for the multiple cyber-attacks that can occur in cyberphysical systems. Since large-scale CNs have a large number of nodes with intricate connections, their states are usually unmeasurable and only partial information about the network nodes can be obtained through the output of the communication channel [30]. Due to the limitation of network bandwidth and the threat of cyber-attacks, only part of the node information is generally measurable [31]. In order to solve the node state agnosticism, the design of state estimator for CNs is necessary. In this paper, we use two sets of Bernoulli distribution variables and arbitrary functions related to the system state to characterize randomly occurring DoS attacks and deception attacks.
Inspired by the above mentioned work, it can be seen that although event-triggered state estimation for CNs has been extensively studied, relevant research on CNs with multiple attack scenarios is very limited. Second, we also discuss how to efficiently conserve network resources under the condition of limited communication channel capacity. The above two points are the two motivations that motivate the completion of this paper.
Based on the above discussion, we focus on the design of event-triggered state estimators for CNs with malicious attacks. In addition, an AETS is introduced to avoid sensors from sending unnecessary packets to the remote estimator for the purpose of saving network bandwidth. Then, based on the derived sufficient conditions for system stability, a feasible approach for co-design is proposed. Finally, a numerical example verifies the effectiveness of the proposed method. The main contributions of this paper are as follows.
● In order to reflect the real network environment, multiple scenarios of possible malicious attacks in the transmission channel are considered. By introducing Bernoulli distribution variables and an arbitrary function based on the system state, a mathematical model of the deception attacks is developed. Then the action sequence of the DoS attacks is portrayed by introducing another set of Bernoulli variables. The so-called hybrid network attack is modeled.
● We incorporate the plant to be studied, the state estimator, the network resource scheduling policy, and the aforementioned attack model into a unified architecture and describe them through an augmented system.
● We derive sufficient conditions to ensure the asymptotic stability of the above system (Theorem I). Then we give a co-design method for computing the estimation gain of the estimator and the weight matrix of the AETS (Theorem II).
Notations: Rn×m means n×m real matrix; Rn denotes n-dimensional Euclidean space; A>0(A≥0) implies positive definite (positive semi-definite) symmetric matrix; E{A} represents mathematical expectation of random variable A; colN{Xi} and diagN{Yi} stand for the block-column matrix col{X1,X2,…,XN} and the block-diagonal matrix col{Y1,Y2,…,YN}, respectively; ⊗ denotes Kronecker product.
Consider the following class of CNs:
{˙xi(t)=Dixi(t)+g(xi(t))+N∑j=1aijΓ,xj(t)+Eiwi(t),yi(t)=Cixi(t),zi(t)=Mixi(t),xi(t)=ϕi(θ),θ∈(−∞,0],i=1,2,…,N, | (2.1) |
where xi(t), yi(t), and zi(t) denote, respectively, the state vector, the measurement output, and the output of the i-th node. wi(t)∈L2[0,∞) denotes the disturbance noise. ϕ is the initial conditions. g(⋅) is the the nonlinear vector-valued function. Γ=diag{γ1,γ2,…,γn} is an inner-coupling matrix. A=(aij)N×N is thecoupled configuration matrix with aij>0(i≠j) but not all zero. As usual, the diagonal element is described by aii=−∑Nj=1,j≠iaij. Ci,Di,Ei, and Mi are the known matrices.
In a networked system, the instant information in the communication network is usually transmitted in an equal-period transmission scheme, which can cause unnecessary waste of limited network bandwidth. The event-based transmission strategy allows the communication channel not to be occupied all the time, thus reducing the network burden and achieving the goal of saving network resources. However, an open network environment also exposes the system to potential security risks, and for this reason, the impact of cyber attacks on the system is further discussed. The main work of this paper is to discuss a co-design approach of state estimators and AETS for a class of complex networks suffering from hybrid cyber attacks. Then, we incorporate the above-mentioned complex network model with AETS, hybrid cyber attack model and state estimator into a unified framework as shown in Figure 1. In the rest of this section, AETS, hybrid cyber attack modeling, and system modeling are discussed respectively. The details are as follows:
With the booming development of network technology, the ETS has been very effective in scheduling network resources. Under the ETS, the successful transmission of sampled data needs to satisfy a triggering condition:
(yi(bkh)−yi(bkh+lh))TΩi(yi(bkh)−yi(bkh+lh))−ϱyTi(bkh)Ωiyi(bkh)>0. | (2.2) |
Based on the traditional ETS, a new ETS with variable threshold is proposed to save more network resources. Under the AETS, the threshold in the triggering condition can be described as:
˙ϱ(t)=1ϱ(t)(1ϱ(t)−ϖ)eTki(t)Ωieki(t), | (2.3) |
where 0<ϱ(0)≤1, ϖ>0, and eki(t)=yi(bkh)−yi(bkh+lh).
Define that τk=tk−bkh and ℓk=bk+1−bk−1, where τk is network-induced delay, and τk∈[τm,τM]. According to tk<tk+1, the interval [tk,tk+1) can be divided as ⋃ℓkl=0ℑl, where
ℑn={[tk+lh,tk+(l+1)h),l=0,1,…,ℓk−1,[tk+lh,tk+1),l=ℓk. | (2.4) |
By setting d(t)=t−(bkh+lh), we have
d(t)={t−bkh,t∈ℑ0,t−bkh−h,t∈ℑ1,⋮⋮,t−bkh−ℓkh,t∈ℑℓk, | (2.5) |
eki(t)={0,t∈ℑ0,yi(bkh)−yi(bkh+h),t∈ℑ1,⋮⋮,yi(bkh)−yi(bkh+ℓkh),t∈ℑℓk. | (2.6) |
From (2.1)–(2.6), the actual measurement output under AETM can be expressed as:
ˉyi(t)=yi(t−d(t))+eki(t), | (2.7) |
where 0<τm≤d(t)≤h+τM=dM.
Remark 1. In the ith sensor-to-estimator channel, the ith trigger determines whether the current sampled data of the ith sensor corresponding to the node needs to be sent, i.e., whether the current sampled data in sensor i satisfies the ith adaptive event-triggered condition (2.2). The above scheme is introduced to ensure that the information transmission efficiency can be effectively improved under the limitation of network bandwidth.
The AETS greatly facilitate the transfer of data between components in a networked system. However, the actual network network environment is complex and volatile, and there may be potential security threats. From the defender's standpoint, the attack type and attack moment cannot be determined. For this reason, both deception attacks and DoS attacks are considered, both of which are the most common attack behaviors in network. Also, two sets of Bernoulli distributed variables are introduced to characterize the moment of occurrence of the two attack behaviors. Denote that F=f(yi(t−η(t))). The hybrid attack model can be expressed as follows:
˜yi(t)=(1−βi(t))[(1−αi(t))ˉyi(t)+αi(t)F], | (2.8) |
where, αi(t) and βi(t) are BDVs, and satisfy the following statistical properties:
Pr{αi(t)=1}=ˉαi,Pr{αi(t)=0}=1−ˉαi,E{αi(t)}=ˉαi,E{αi(t)−ˉαi}=0,E{(αi(t)−ˉαi)2}=ˉαi(1−ˉαi)=δ2α.Pr{βi(t)=1}=ˉβi,Pr{βi(t)=0}=1−ˉβi,E{βi(t)}=ˉβi,E{βi(t)−ˉβi}=0,E{(βi(t)−ˉβi)2}=ˉβi(1−ˉβi)=δ2β. | (2.9) |
Remark 2. Equation (2.8) is a hybrid-driven cyber-attack behavior, i.e., the deception attacks and the DoS attacks both switch with each other at different frequencies in the communication channel. The BDVs α(t) and β(t) describe the occurrence sequence of deception attacks and DoS attacks, respectively. For example, if β(t)=1, the communication network is under DoS attacks, which means that all transmission data is blocked. If α(t)=1, the communication network suffers from under deception attack, which means that the real transmission data is replaced by the deception attack signal.
Remark 3. For the hybrid attack model, a nonlinear function with time delay η(t) on the interval (0,ηM] is introduced to express the deception attack signal F, and satisfies the following assumption:
||f(yi(t−η(t)))||2≤||Fiyi(t−η(t))||2, | (2.10) |
where F is a known matrix.
Under AETM and hybrid attacks, the estimator on the node i is given as follows:
{ˆxi(t)=Diˆxi(t)+g(ˆxi(t))+N∑j=1aijΓˆxj(t)+Li(˜yi(t)−ˆyi(t)),ˆyi(t)=Ciˆxi(t),ˆzi(t)=Miˆxi(t), | (2.11) |
where ˆx∈Rn is the estimated state on the node i, ˆzi(t)∈Rm is the estimate of zi(t), and Li is the estimator gain to be designed.
Define
ei(t)=xi(t)−ˆxi(t), |
and
˜zi(t)=zi(t)−ˆzi(t). |
For simplicity, we denote that
e(t)=colN{ei(t)},ed(t)=colN{ei(t−d(t))},x(t)=colN{xi(k)},xd(t)=colN{xi(t−d(t))},˜x(t)=colN{˜xi(t)},w(t)=colN{wi(t)},z(t)=colN{zi(t)},C|D|E|L|M|Ω=diagN{Ci|Di|Ei|Li|Mi|Ωi},α(t)|β(t)=diagN{αi(t)|βi(t)},ˉα|ˉβ=diagN{ˉαi|ˉβi},ek(t)=colN{eki(t)},f(y(t−η(t)))=colN{f(yi(t−η(t)))}. |
Then, by combining (2.1)–(2.11) and utilizing Kronecker product, we have
{˙e(t)=(D+A⊗Γ−LC)e(t)+g(e(t))+LCx(t)+Ew(t)−{(1−β(t))(1−α(t))LC[x(t−d(t))+ek(t)]+(1−β(t))α(t)LF},˜z(t)=Me(t). | (2.12) |
Denote ˜x(t)=[x(t)e(t)], and the augmented system can be obtained from (2.1) and (2.11) as follows:
{˙˜x(t)=D˜x(t)+g(˜x(t))+Ew(t)−(1−β(t))(1−α(t)){Λ1˜x(t−d(t))+Λ2ek(t)}−(1−β(t))α(t)Λ3F.˜z(t)=˜M˜x(t). | (2.13) |
where
D=[D+A⊗Γ0LCD+A⊗Γ−LC],E=[EE],˜M=[0M],Λ1=[00LC0],Λ2=[0LC],Λ3=[0L],H=[I0], |
system (2.13) can be written as:
˙˜x(t)=Π1+(α(t)−ˉα)Π2+(β(t)−ˉβ)Π3+(α(t)−ˉα)(β(t)−ˉβ)Π4, | (2.14) |
where
Π1=D˜x(t)+g(˜x(t))+Eω(t)−(1−ˉβ)(1−ˉα){Λ1˜x(t−d(t))+Λ2ek(t)}−(1−ˉβ)ˉαΛ3F,Π2=(1−ˉβ)[Λ1˜x(t−d(t))+Λ2ek(t)−Λ3F],Π3=(1−ˉα)[Λ1˜x(t−d(t))+Λ2ek(t)]+ˉαΛ3F,Π4=−Λ1˜x(t−d(t))−Λ2ek(t)+Λ3F. |
Assumption 1. [24] For positive diagonal maxtrix U=diag{μ1,μ2,⋯,μn}, the following inequality holds:
[˜x(t)g(˜x(t))]T[−UG1∗G2U−U][˜x(t)g(˜x(t))]≥0, | (2.15) |
where
G1=diag{ν−1ν+1,ν−2ν+2,⋯,ν−nν+n},G2=diag{ν−1+ν+12,ν−2+ν+22,⋯,ν−n+ν+n2}. |
Lemma 1. [24] For any matrices R1|2>0, positive scalars dM|ηM, and d(t)|η(t)∈[0,dM|ηM], if there exist matrices N1|2∈Rn×n such that [R1|2∗N1|2R1|2]>0, the following inequality holds:
−dM|ηM∫tt−dM|ηM˙˜xT(s)R1|2˙˜x(s)ds≤ΞT[−R1|2∗∗R1|2−N1|2−2R1|2+N1|2+NT1|2∗N1|2R1|2−N1|2−R1|2]Ξ. | (2.16) |
where Ξ=[˜x(t)˜x(t−d(t)|η(t))˜x(t−dM|ηM)].
Lemma 2. [11] For any positive-definite matrices R,Z and scalar ϵ, the following inequality holds.
−ZR−1Z≤ϵ2R−2ϵZ. | (2.17) |
This section is concerned with the design problem for adaptive event-triggered state estimators such that the augmented dynamics (2.14) of the CNs (2.1) is asymptotically stable.
Theorem 1. For given constants dM,ηM,ˉα,ˉβ,ϖ, estimator gain L, and weighting matrix Ω, the augmented dynamics (2.14) is asymptotically stable if there exist matrices P>0,Qi>0,Ri>0, and Ni, such that the following inequalities are satisfied for i=1,2:
Θ=[Σ∗∗∗∗Ψ1R∗∗∗∗Ψ20R∗∗∗Ψ300R∗∗Ψ4000R∗]<0, | (3.1) |
[R1∗N1R1]>0, | (3.2) |
[R2∗N2R2]>0, | (3.3) |
where
Σ=[(1.1)=DTP+PD+Q1+Q2−UG1−R1−R2+˜MT˜M(2.1)=−(1−ˉα)(1−ˉβ)ΛT1P+R1−N1(2.2)=HTCTΩCH−2R1+N1+NT1(3.1)=N1,(3.2)=R1−N1,(3.3)=−R1−Q1(4.1)=R2−N2,(4.4)=−2R2+N2+NT2+HTCTFTFCH(5.1)=N2,(5.4)=R2−N2,(5.5)=−Q2−R2(6.1)=−(1−ˉβ)(1−ˉα)ΛT2P,(6.6)=−ϖΩ(7.1)=−(1−ˉβ)ˉαΛT3P,(7.7)=−I(8.1)=P+G2U,(8.8)=−U(9.1)=EP,(9.9)=−γ2],Ψj=[dMΓjηMΓj],Γ1=[PD−(1−ˉβ)(1−ˉα)PΛ1000−(1−ˉβ)(1−ˉα)PΛ2−(1−ˉβ)(1−ˉα)PΛ3PPE],Γ2=[0δα(1−ˉβ)PΛ1000δα(1−ˉβ)PΛ2−δα(1−ˉβ)PΛ300],Γ3=[0δβ(1−ˉα)PΛ1000δβ(1−ˉα)PΛ2δαδβPΛ300],Γ4=[0−δαδβPΛ1000−δαδβPΛ2δαδβPΛ300],R∗=diag{−PR−11P,−PR−12P}. |
Proof. Contruct an Lyapunov functional candidate:
V(x(t),t)=3∑i=1Vi(x(t),t)+V4(t), | (3.4) |
where
V1(x(t),t)=˜xT(t)P˜x(t),V2(x(t),t)=∫tt−dM˜xT(s)Q1˜x(s)ds+∫tt−ηM˜xT(s)Q2˜x(s)ds,V3(x(t),t)=dM∫0−dM∫tt+θ˙˜xT(s)R1˙˜x(s)dsdθ+ηM∫0−ηM∫tt+θ˙˜xT(s)R2˙˜x(s)dsdθ,V4(t)=12ϱ2(t), |
The derivative and mathematical expectation of (3.4) can be calculated as:
E{˙V(x(t),t)}=sym{˜xT(t)PΠ1}+˜xT(t)(Q1+Q2)˜x(t)−˜xT(t−dM)Q1˜x(t−dM)−˜xT(t−ηM)Q2˜x(t−ηM)+ΠT1ΞΠ1+δ2αΠT2ΞΠ2+δ2βΠT3ΞΠ3+δ2αδ2βΠT4ΞΠ4+1ϱ(t)eTk(t)Ωek(t)−ϖeTk(t)Ωek(t)−dM∫tt−dM˙˜xT(s)R1˙˜x(s)ds−ηM∫tt−ηM˙˜xT(s)R2˙˜x(s)ds, | (3.5) |
where Ξ=d2MR1+η2MR2.
Then, the following inequality can be obtained from Remark 3:
˜xT(t−η(t))HTCTFTFCH˜x(t−η(t))−FTF≥0. | (3.6) |
Combining (3.5) and (3.6), using Lemma 1 to estimate one cross term in (3.5), taking the adaptive triggering condition (2.2) and (2.3) to bound the term 1ϱ(t)eTk(t)Ωek(t) in (3.5), and adding (2.15) in Assumption 1 to the right-hand side of (3.5), one obtains
E{˙V(x(t),t)}+˜zT(t)˜z(t)−γ2wT(t)w(t)≤ξT(t)Σξ(t)+ΠT1ΞΠ1+δ2αΠT2ΞΠ2+δ2βΠT3ΞΠ3+δ2αδ2βΠT4ΞΠ4, | (3.7) |
where ξ(t)={˜x(t),˜x(t−d(t)),˜x(t−dM),˜x(t−η(t)),˜x(t−ηM),ek(t),F,g(˜x(t)),w(t)}.
Utilizing Schur complement, we can obtain that (3.7) is equivalent to (3.1)–(3.3). When w(t)=0, it can be seen that the system (2.14) is asymptotically stable from the LMIs (3.1)–(3.3). This completes the proof.
So far, the stability analysis problem of augmented systems for state estimation about complex network has been solved. Based on Theorem 1, we can easily obtain the estimator gains. Details are as follows.
Theorem 2. For given constants dM,ηM,ˉα,ˉβ,ϖ, the estimator (2.11) for the system (2.14) can be designed if there exist matrices P=diag{P1,P2}>0,Qi>0,Ri>0, and Ni, such that the following inequalities are satisfied for i=1,2:
ˆΘ=[ˆΣ∗∗∗∗ˆΨ1ˆR∗∗∗∗ˆΨ20ˆR∗∗∗ˆΨ300ˆR∗∗ˆΨ4000ˆR∗]<0, | (3.8) |
(3.2) and (3.3), where
ˆΣ=[(1.1)=Λ4+ΛT4+Q1+Q2−UG1−R1−R2+˜MT˜M(2.1)=−(1−ˉα)(1−ˉβ)ΛT5+R1−N1(2.2)=HTCTΩCH−2R1+N1+NT1(3.1)=N1,(3.2)=R1−N1,(3.3)=−R1−Q1(4.1)=R2−N2,(4.4)=−2R2+N2+NT2+HTCTFTFCH(5.1)=N2,(5.4)=R2−N2,(5.5)=−Q2−R2(6.1)=−(1−ˉβ)(1−ˉα)ΛT6,(6.6)=−ϖΩ(7.1)=−(1−ˉβ)ˉαΛT7,(7.7)=−I(8.1)=P+G2U,(8.8)=−U(9.1)=EP,(9.9)=−γ2],ˆΨj=[dMˆΓjηMˆΓj],ˆΓ1=[Λ4−(1−ˉβ)(1−ˉα)Λ5000−(1−ˉβ)(1−ˉα)Λ6−(1−ˉβ)(1−ˉα)Λ7PPE],ˆΓ2=[0δα(1−ˉβ)Λ5000δα(1−ˉβ)Λ6−δα(1−ˉβ)Λ700],ˆΓ3=[0δβ(1−ˉα)Λ5000δβ(1−ˉα)Λ6δαδβΛ700],ˆΓ4=[0−δαδβΛ5000−δαδβΛ6δαδβΛ700],ˆR∗=diag{ε2R1−2εP,ε2R2−2εP},Λ4=[P1D+P1A⊗Γ0XCP2D+P2A⊗Γ−XC],Λ5=[00XC0],Λ6=[0XC],Λ7=[0X]. |
In addition, the estimator gains can be obtained by Li=P−12iXi(i=1,2,…,N).
Proof. By applying Lemma 2, replacing −PR1P in (3.1) by εR1−2εP, a new ˆR∗ is obtained as:
ˆR∗=diag{ε2R1−2εP,ε2R2−2εP}. |
Noticing that X=P2L, (3.8) can be obtained. This completes the proof.
In this section, we provide numerical simulations to demonstrate the validity of the theoretical approach. We take a complex network with three nodes as an example.
Example 1. The system parameters are given as follows:
D1=D2=D3=[−100−1],Γ=[0.5000.5],aij={−2,i≠j1,i=j,E1=[0.30.3],E2=[0.50.5],E3=[0.40.4],C1=[0.40.5],C2=[−0.40.5],C3=[0.4−0.5],M1=M2=M3=[0.30.7],g(xi(t))=[0.5xi1(t)−tanh(0.2xi1(t))+0.2xi2(t),0.95xi2(t)−tanh(0.75xi1(t))]. |
The deception attack signal is chosen as
f(xi(t))=[−tanh(−0.3xi2(t))−tanh(−0.3xi1(t))]. |
Moreover, other parameters are selected by
dM=0.1,ηM=0.1,ˉα=0.2,ˉβ=0.2,ϖ=7,γ=4,ε=1,F=diagN{0.3,0.3},G1=diagN{0,0},G2=diagN{0.02,0.02}. |
By utilizing the MATLAB LMI Toolbox, the feasible solutions can be obtained based on the constraints (3.8), (3.2) and (3.3) in Theorem 2.
X1=[11.478411.2668],X2=[0.11312.8645],X3=[0.1590−2.6171].P21=103×[2.5019−2.4698−2.46982.5020],P22=103×[2.5087−2.4629−2.46292.5088],P23=103×[2.5087−2.4628−2.46282.5089],Ω1=52.0965,Ω2=839.7490,Ω2=950.6389. |
Further, the estimator gains can be designed as:
L1=[0.35380.3538],L2=[0.03220.0327],L3=[−0.0264−0.0270]. |
Given the initial state x1(0)=[1−1]T, x2(0)=[10]T, x3(0)=[0−1]T, ˆxi(0)=[00]T, and ϱi(0)=0.25(i=1,2,3), numerical simulations further verify the validity of our theoretical approach. Figures 2–4 show the state responses for node i. Figures 2–7 plot the output estimation error for node i. Figures 8 and 9 depict the occurring instants of deception attacks and DoS attacks, respectively. Figures 10–12 present the release instants and intervals under AETS for node i. In addition, the release instants and intervals of ETS are plotted in Figures 13–15, respectively. The average period, maximum release interval, and transmission rate by Theorem 2 and reported in [24] are listed in Table 1, which the transmission rate indicates thetransmitteddatathesampleddata×100%. Obviously, the AETS can obtain a larger release interval than the traditional ETS, and have a lower data delivery rate.
sampling period h=0.1s | average period | maximum release interval | transmission rate |
AETS in node 1 | 0.4000 | 4.4 | 25% |
AETS in node 2 | 1.4285 | 4.3 | 7% |
AETS in node 3 | 1.4285 | 3.5 | 7% |
ETS [24] in node 1 | 0.3571 | 3.0 | 28% |
ETS [24] in node 2 | 1.2500 | 4.0 | 8% |
ETS [24] in node 3 | 1.2500 | 3.2 | 8% |
The issue of event-triggered state estimation has been studied for CNs under hybrid cyber-attacks in this paper. AETS has been introduced to alleviate the network congestion problem. With the help of two sets of BDVs and an arbitrary function related to the system state, a mathematical model of the hybrid cyber-attacks has been established to portray randomly occurring DoS attacks and deception attacks. Then, CNs, AETS, hybrid cyber-attacks, and state estimators have been incorporated into a unified architecture. As a result, an augmented system has been presented. Furthermore, based on Lyapunov stability theory and LMIs, sufficient conditions to ensure the asymptotic stability of the augmented system have been derived, and the corresponding state estimator has been designed. Finally, the effectiveness of the theoretical method has been demonstrated by numerical examples and simulations. In the future, security state estimation problems for a class of CNs suffered by underlying attacks will be studied.
The authors declare that there is no conflict of interest regarding the publication of this paper.
[1] | R. M. Ali, V. Ravichandran, N. Seenivasagan, Coefficient bounds for p-valent functions, Appl. Math. Comput., 187 (2007), 35–46. |
[2] | K. O. Babalola, On H3(1) Hankel determinant for some classes of univalent functions, Inequality Theory and Applications, 6 (2010), 1–7. |
[3] |
N. E. Cho, V. Kumar, S. S. Kumar, V. Ravichandran, Radius problems for starlike functions associated with the sine function, B. Iran. Math. Soc., 45 (2019), 213–232. doi: 10.1007/s41980-018-0127-5
![]() |
[4] |
M. Darus, I. Faisal, Some subclasses of analytic functions of complex order defined by new differential operator, Tamkang J. Math., 43 (2012), 223–242. doi: 10.5556/j.tkjm.43.2012.740
![]() |
[5] |
M. Darus, S. Hussain, M. Raza, J. Sokół, On a subclass of starlike functions, Results Math., 73 (2018), 22. doi: 10.1007/s00025-018-0771-3
![]() |
[6] |
R. M. El-Ashwash, M. K. Aouf, M. Darus, Differential subordination results for analytic functions, Asian-Eur. J. Math., 6 (2013), 1350044. doi: 10.1142/S1793557113500447
![]() |
[7] | A. W. Goodman, Univalent functions, Vols. I and II, Polygonal Publishing House, Washinton, New Jersey, 1983. |
[8] |
A. Goodman, On uniformly convex functions, Ann. Polon. Math., 56 (1991), 87–92. doi: 10.4064/ap-56-1-87-92
![]() |
[9] | S. Hussain, A. Rasheed, M. A. Zaighum, M. Darus, A Subclass of Analytic Functions Related to k-Uniformly Convex and Starlike Functions, J. Funct. Spaces, 2017 (2017), 9010964. |
[10] |
S. Hussain, S. Khan, M. A. Zaighum, M. Darus, Certain subclass of analytic functions related with conic domains and associated with Salagean q-differential operator, AIMS Math., 2 (2017), 622–634. doi: 10.3934/Math.2017.4.622
![]() |
[11] | I. S. Jack, Functions starlike and convex of order α, J. Lond. Math. Soc., 2 (1971), 469–474. |
[12] |
W. Janowski, Some extremal problems for certain families of analytic functions I, Ann. Polon. Math., 28 (1973), 297–326. doi: 10.4064/ap-28-3-297-326
![]() |
[13] |
S. Kanas, A. Wisniowska, Conic regions and k-uniform convexity, J. Comput. Appl. Math., 105 (1999), 327–336. doi: 10.1016/S0377-0427(99)00018-7
![]() |
[14] | S. Kanas, A. Wisniowska, Conic domains and starlike functions, Rev. Roumaine Math. Pures Appl., 45 (2000), 647–658. |
[15] |
S. Kanas, V. S. Masih, A. Ebadian, Relations of a planar domains bounded by hyperbolas with families of holomorphic functions, J. Inequal. Appl., 2019 (2019), 246. doi: 10.1186/s13660-019-2190-8
![]() |
[16] | S. Kanas, V. S. Masih, A. Ebadian, Coefficients problems for families of holomorphic functions related to hyperbola, ⌢Math. Slovaca, 70 (2020), 605–616. |
[17] | W. Ma, D. Minda, A unified treatment of some special classes of univalent functions. In: Proceedings of the Conference on Complex Analysis (Tianjin, 1992), 157–169, Conf. Proc. Lecture Notes Anal., I, Int. Press, Cambridge, MA. |
[18] |
V. S. Masih, S. Kanas, Subclasses of Starlike and Convex Functions Associated with the Limaçon Domain, Symmetry, 12 (2020), 942. doi: 10.3390/sym12060942
![]() |
[19] | S. S. Miller, P. T. Mocanu, Differential Subordinations, Theory and Applications, Series of Monographs and Textbooks in Pure and Applied Mathematics, vol. 225, Marcel Dekker Inc., New York/Basel, 2000. |
[20] |
G. Murugusundaramoorthy, T. Bulboaca, Hankel Determinants for New Subclasses of Analytic Functions Related to a Shell Shaped Region, Mathematics, 8 (2020), 1041. doi: 10.3390/math8061041
![]() |
[21] | Z. Nehari, Conformal mapping, McGraw-Hill, New York, 1952. |
[22] | J. W. Noonan, D. K. Thomas, On the Hankel determinants of areally mean p-valent functions, Proc. Lond. Math. Soc., 3 (1972), 503–524. |
[23] |
K. I. Noor, S. N. Malik, On coefficient inequalities of functions associated with conic domains, Comput. Math. Appl., 62 (2011), 2209–2217. doi: 10.1016/j.camwa.2011.07.006
![]() |
[24] | R. K. Raina, J. Sokól, On coefficient estimates for a certain class of starlike functions, Hacet. J. Math. Stat., 44 (2015), 1427–1433. |
[25] | R. K. Raina, J. Sokól, On a class of analytic functions governed by subordination, Acta Univ. Sapientiae Math., 11 (2019), 144–155. |
[26] | A. Rasheed, S. Hussain, M. A. Zaighum, M. Darus, Class of analytic function related with uniformly convex and Janowski's functions, J. Funct. Spaces, 2018 (2018), 4679857. |
[27] | V. Ravichandran, F. Rønning, T. N. Shanmugam, Radius of convexity and radius of starlikeness for some classes of analytic functions, Complex Var. Elliptic Equ., 33 (1997), 265–280. |
[28] |
M. Raza, K. I. Noor, Subclass of Bazilevic functions of complex order, AIMS Math., 5 (2020), 2448–2460. doi: 10.3934/math.2020162
![]() |
[29] | W. Rogosinski, On the coefficients of subordinate functions, Proc. Lond. Math. Soc., 2 (1945), 48–82. |
[30] |
F. Rønning, Uniformly convex functions and a corresponding class of starlike functions, Proc. Amer. Math. Soc., 118 (1993), 189–196. doi: 10.1090/S0002-9939-1993-1128729-7
![]() |
[31] | A. Saliu, On Generalized k-Uniformly Close-to-Convex Functions of Janowski Type, International Journal of Analysis and Applications, 17 (2019), 958–973. |
[32] | A. Saliu, K. I. Noor, On Janowski Close-to-Convex Functions Associated with Conic Regions, International Journal of Analysis and Applications, 18 (2020), 614–623. |
[33] | A. Saliu, K. I. Noor, S. Hussain, M. Darus, On Quantum Differential Subordination Related with Certain Family of Analytic Functions, J. Math., 2020 (2020), 6675732. |
[34] | A. Saliu, K. I. Noor, On subclasses of functions with boundary and radius rotations associated with crescent domains, Bull. Korean Math. Soc., 57 (2020), 1529–1539. |
[35] |
P. Sharma, R. K. Raina, J. Sokól, Certain Ma-Minda type classes of analytic functions associated with the crescent-shaped region, Anal. Math. Phys., 9 (2019), 1887–1903. doi: 10.1007/s13324-019-00285-y
![]() |
[36] | S. Siregar, M. Darus, Certain conditions for starlikeness of analytic functions of Koebe type, Int. J. Math. Math. Sci., 2011 (2011), 679704. |
[37] |
H. M. Srivastava, K. Bilal, K. Nazar, Q. Z. Ahmad, Coefficient inequalities for q-starlike functions associated with the Janowski functions, Hokkaido Math. J., 48 (2019), 407–425. doi: 10.14492/hokmj/1562810517
![]() |
[38] |
N. Tuneski, M. Darus, E. Gelova, Simple sufficient conditions for bounded turning, Rend. Semin. Mat. Univ. Padova, 132 (2014), 231–238. doi: 10.4171/RSMUP/132-11
![]() |
[39] |
Y. Yunus, S. A. Halim, A. B. Akbarally, Subclass of starlike functions associated with a limaçon, AIP Conference Proceedings, 1974 (2018), 030023. doi: 10.1063/1.5041667
![]() |
1. | Na Lin, Dongyan Chen, Jun Hu, Chaoqing Jia, Hui Yu, Partial‐nodes‐based state estimation for state‐saturated complex networks with random mixed couplings under dynamic event‐triggered protocol, 2023, 0890-6327, 10.1002/acs.3568 | |
2. | Xun Wang, Engang Tian, Bin Wei, Jinliang Liu, Novel attack‐defense framework for nonlinear complex networks: An important‐data‐based method, 2023, 33, 1049-8923, 2861, 10.1002/rnc.6551 | |
3. | Cai Chen, Bowen Yue, Chaoqing Jia, Resolution‐Dependent State Estimation for a Class of Nonlinear Coupled Complex Networks With Stochastic Communication and Correlated Noises, 2024, 0890-6327, 10.1002/acs.3914 | |
4. | M. Mubeen Tajudeen, M. Syed Ali, Grienggrai Rajchakit, Anuwat Jirawattanapanit, Bandana Priya, Extended dissipative performance for a complex dynamical networks based on adaptive event‐triggered filter method subject to hybrid attacks, 2024, 38, 0890-6327, 415, 10.1002/acs.3707 | |
5. | Li Liu, Yinfang Song, Hong Yu, Gang Zhang, Almost sure exponential synchronization of multilayer complex networks with Markovian switching via aperiodically intermittent discrete observa- tion noise, 2024, 9, 2473-6988, 28828, 10.3934/math.20241399 |
sampling period h=0.1s | average period | maximum release interval | transmission rate |
AETS in node 1 | 0.4000 | 4.4 | 25% |
AETS in node 2 | 1.4285 | 4.3 | 7% |
AETS in node 3 | 1.4285 | 3.5 | 7% |
ETS [24] in node 1 | 0.3571 | 3.0 | 28% |
ETS [24] in node 2 | 1.2500 | 4.0 | 8% |
ETS [24] in node 3 | 1.2500 | 3.2 | 8% |