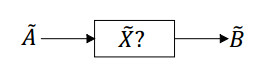
Based on the three-dimensional endocrine neuron model, a four-dimensional endocrine neuron model was constructed by introducing the magnetic flux variable and induced current according to the law of electromagnetic induction. Firstly, the codimension-one bifurcation and Interspike Intervals (ISIs) analysis were applied to study the bifurcation structure with respect to external stimuli and parameter k0, and two dynamical behaviors were found: period-adding and period-doubling bifurcation leading to chaos. Besides, Hopf bifurcation was specially discussed corresponding to the transformation of the state. Secondly, the different firing patterns such as regular bursting, subthreshold oscillations, fast spiking, mixed-mode oscillations (MMOs) etc. can be observed by changing the external stimuli and the induced current. The neuron model presented more firing activities under strong coupling strength. Finally, the codimension-two bifurcation analysis shown more details of bifurcation. At the same time, the Bogdanov-Takens bifurcation point was also analyzed and three bifurcation curves were derived.
Citation: Qixiang Wen, Shenquan Liu, Bo Lu. Firing patterns and bifurcation analysis of neurons under electromagnetic induction[J]. Electronic Research Archive, 2021, 29(5): 3205-3226. doi: 10.3934/era.2021034
[1] | Naiwen Wang . Solvability of the Sylvester equation AX−XB=C under left semi-tensor product. Mathematical Modelling and Control, 2022, 2(2): 81-89. doi: 10.3934/mmc.2022010 |
[2] | Jianhua Sun, Ying Li, Mingcui Zhang, Zhihong Liu, Anli Wei . A new method based on semi-tensor product of matrices for solving reduced biquaternion matrix equation l∑p=1ApXBp=C and its application in color image restoration. Mathematical Modelling and Control, 2023, 3(3): 218-232. doi: 10.3934/mmc.2023019 |
[3] | Wenxv Ding, Ying Li, Dong Wang, AnLi Wei . Constrainted least squares solution of Sylvester equation. Mathematical Modelling and Control, 2021, 1(2): 112-120. doi: 10.3934/mmc.2021009 |
[4] | Hongli Lyu, Yanan Lyu, Yongchao Gao, Heng Qian, Shan Du . MIMO fuzzy adaptive control systems based on fuzzy semi-tensor product. Mathematical Modelling and Control, 2023, 3(4): 316-330. doi: 10.3934/mmc.2023026 |
[5] | Xueling Fan, Ying Li, Wenxv Ding, Jianli Zhao . H-representation method for solving reduced biquaternion matrix equation. Mathematical Modelling and Control, 2022, 2(2): 65-74. doi: 10.3934/mmc.2022008 |
[6] | Daizhan Cheng, Zhengping Ji, Jun-e Feng, Shihua Fu, Jianli Zhao . Perfect hypercomplex algebras: Semi-tensor product approach. Mathematical Modelling and Control, 2021, 1(4): 177-187. doi: 10.3934/mmc.2021017 |
[7] | Yuyang Zhao, Yang Liu . Output controllability and observability of mix-valued logic control networks. Mathematical Modelling and Control, 2021, 1(3): 145-156. doi: 10.3934/mmc.2021013 |
[8] | Lei Wang, Xinyun Liu, Ting Li, Jiandong Zhu . Skew-symmetric games and symmetric-based decomposition of finite games. Mathematical Modelling and Control, 2022, 2(4): 257-267. doi: 10.3934/mmc.2022024 |
[9] | Daizhan Cheng, Ying Li, Jun-e Feng, Jianli Zhao . On numerical/non-numerical algebra: Semi-tensor product method. Mathematical Modelling and Control, 2021, 1(1): 1-11. doi: 10.3934/mmc.2021001 |
[10] | Weiwei Han, Zhipeng Zhang, Chengyi Xia . Modeling and analysis of networked finite state machine subject to random communication losses. Mathematical Modelling and Control, 2023, 3(1): 50-60. doi: 10.3934/mmc.2023005 |
Based on the three-dimensional endocrine neuron model, a four-dimensional endocrine neuron model was constructed by introducing the magnetic flux variable and induced current according to the law of electromagnetic induction. Firstly, the codimension-one bifurcation and Interspike Intervals (ISIs) analysis were applied to study the bifurcation structure with respect to external stimuli and parameter k0, and two dynamical behaviors were found: period-adding and period-doubling bifurcation leading to chaos. Besides, Hopf bifurcation was specially discussed corresponding to the transformation of the state. Secondly, the different firing patterns such as regular bursting, subthreshold oscillations, fast spiking, mixed-mode oscillations (MMOs) etc. can be observed by changing the external stimuli and the induced current. The neuron model presented more firing activities under strong coupling strength. Finally, the codimension-two bifurcation analysis shown more details of bifurcation. At the same time, the Bogdanov-Takens bifurcation point was also analyzed and three bifurcation curves were derived.
The concept of type-2 fuzzy sets (T2 FSs) was first proposed by Zadeh [1] and a detailed introduction was given in [2]. T2 FSs are an extension of the type-1 fuzzy set (T1 FS) and further considers the fuzziness of the fuzzy set. Since the definition of T2 FSs was proposed, most scholars have mainly studied the operations and properties of T2 FSs [3,4]. Until the 1990s, Prof. Mendel redefined T2 FSs and proposed the type-2 fuzzy logic system (T2 FLS) [5,6].
As an extension of T1 FSs, T2 FSs overcome the limitations of T1 FSs in dealing with the uncertainties of actual objects. However the definition of T2 FSs is complicated, and the corresponding graph must be a spatial graph. Due to the complexity of the expression of T2 FSs, Mendel introduces the definition of interval T2 FSs (IT2 FSs) as a special case of T2 FSs. The secondary membership grade of IT2 FSs is constant one, which is more simpler than T2 FSs. In general, IT2 fuzzy logic system (IT2 FLS) are used in most theories and applications [7,8].
In the field of fuzzy control, solving fuzzy relation equations (FREs) plays an important role in the design of fuzzy controller and fuzzy logic reasoning. Most algorithms for solving FREs can obtain some specific solutions, such as the minimum or maximum solution [9], or can describe the solution theoretically [10]. In the existing methods, most of them are used for solving type-1 fuzzy relation equations (T1 FREs), while few methods are used for solving interval type-2 fuzzy relation equations (IT2 FREs). The main work of this paper is to propose a new method to obtain the entire solution set of IT2 FREs.
On the other hand, Prof. Cheng proposed a new matrix product-semi-tensor product (STP) of matrices, which is the generalization of the conventional matrix product and retains almost all the main properties of the conventional matrix product. As a novel mathematical technique for handling logical operations, STP has been successfully applied to logical systems [11,12,13,14] and, based on this, a new algorithm for solving FREs has been devised. For example, in T1 FREs, the STP is used to solve fuzzy relation equalities and fuzzy relation inequalities [15,16,17,18]. In type-2 fuzzy relational equations (T2 FREs), only some simple algorithms have been proposed to study the solution of type-2 single-valued fuzzy relation equations and type-2 symmetry-valued fuzzy relation equations [19,20]. However, the ordinary STP cannot be used directly to solve IT2 FREs. Therefore, we extend the STP to interval matrices and propose the STP of interval matrices, then discuss the solutions of IT2 FREs.
In the rest of this paper, section two introduces the basic concepts of the STP of interval matrices. Section three mainly gives the relevant definitions of interval-valued logic and gives its matrix representation. Section four discusses the solvability of IT2 FREs and designs an algorithm to solve IT2 FREs. Section five explains the viability of the proposed algorithm with a numerical example. Section six gives a brief summary of the paper.
First, in order to express conveniently, we introduce some notations used throughout the paper.
●
I[0,1]:={[α_,¯α]|0≤α_≤¯α≤1}, |
where α_,¯α∈R. If α=[α_,α_](or α=[¯α,¯α]), this is a point interval and α degenerates into a real number.
●
I([0,1]m):={[A_,¯A]|A_≤¯A}. |
A_=(α_i)m and ¯A=(¯αi)m are two m-dimensional vectors and [a_i,¯ai]∈I[0,1], i=1⋯m.
●
I([0,1]m×n):={[A_,¯A]|A_≤¯A}. |
A_=(α_ij)m×n and ¯A=(¯αij)m×n are two m×n dimensional matrices and [α_ij,¯αij]∈I[0,1], i=1⋯m, j=1⋯n.
● δir : the ith column of unit matrix In.
● [δir,δjr] : a bounded closed interval, where δin represents its lower bound and δjn represents its upper bound, abbreviated as δr[i,j].
● Coli(M): the ith column of interval matrix M.
● Rowj(M): the jth row of interval matrix M.
Next, we define ∧,∨ and ¬ in I[0,1].
Definition 2.1. [21] (1) Let
α=[α_,¯α], β=[β_,¯β]∈I[0,1], |
then,
α∨β=[max(α_,β_),max(¯α,¯β)], | (2.1) |
α∧β=[min(α_,β_),min(¯α,¯β)]. | (2.2) |
(2) Let
A=[a_ij,¯aij]∈I([0,1]m×n), |
B=[b_jk,¯bjk]∈I([0,1]n×p), |
then their max-min composition operation is defined as
A∘B=C=[c_ik,¯cik]∈I([0,1]m×p), | (2.3) |
where
[c_ik,¯cik]=([a_i1,¯ai1]∧[b_1k,¯b1k])∨([a_i2,¯ai2]∧[b_2k,¯b2k]) ∨⋯∨([a_in,¯ain]∧[b_nk,¯bnk]), |
where i=1,⋯,m, j=1,⋯,k.
Definition 2.2. [22] Let
A=[a_ij,¯aij], |
B=[b_ij,¯bij]∈I([0,1]m×n), |
then the partial order ≥,≤ and = are defined as
(1) If a_ij≥b_ij,¯aij≥¯bij, we say A≥B.
(2) If a_ij≤b_ij,¯aij≤¯bij, we say A≤B.
(3) If a_ij=b_ij,¯aij=¯bij, we say A=B.
Property 2.1. [15] Let
A=[a_ij,¯aij], B=[b_ij,¯bij]∈I([0,1]m×n), |
C=[c_jk,¯cjk], D=[d_jk,¯djk]∈I([0,1]n×p). |
Assume A≤B and C≤D, then
A∘C≤B∘D. |
Definition 2.3. [22] (1) Let
α=[α_,¯α], β=[β_,¯β]∈I[0,1], |
then the four operations of intervals α and β are as follows.
1) Addition operation
α+β=[α_,¯α]+[β_,¯β]=[α_+β_,¯α+¯β]. | (2.4) |
2) Subtraction operation
α−β=[α_,¯α]−[β_,¯β]=[α_−¯β,¯α−β_]. | (2.5) |
3) Multiplication operation
α×β=[α_,¯α]×[β_,¯β]=[α_β_,¯α¯β]. | (2.6) |
4) Division operation
α/β=[α_,¯α]/[β_,¯β]=[min(α_/β_,α_/¯β,¯α/β_,¯α/¯β),max(α_/β_,α_/¯β,¯α/β_,¯α/¯β)]. | (2.7) |
Note that 0∉β=[β_,¯β].
(2) If
α=[α_,¯α]∈I[0,1], |
A=[a_ij,¯aij]m×n∈I([0,1]m×n), |
then the product of interval α and interval matrix A is
α×A:=([α_,¯α]×[a_ij,¯aij])m×n. | (2.8) |
(3) If
A=[a_ij,¯aij]m×n∈I([0,1]m×n), |
B=[b_jk,¯bjk]n×p∈I([0,1]n×p), |
then the product of interval matrices A and B is
A×B=C=[c_ik,¯cik]n×p=[[c_11,¯c11]⋯[c_1p,¯c1p]⋮⋱⋮[c_n1,¯cn1]⋯[c_np,¯cnp]], | (2.9) |
where
[c_ik,¯cik]=m∑j=1[a_ij,¯aij]×[b_jk,¯bjk]=[a_i1,¯ai1]×[b_1k,¯b1k]+[a_i2,¯ai2]×[b_2k,¯b2k]+⋯+[a_im,¯aim]×[b_mk,¯bmk]. |
Based on Definition 2.3, we give the relevant definition of the STP of interval matrices.
Definition 2.4. (1) If
A=[a_ij,¯aij]m×n∈I([0,1]m×n), |
B=[b_kl,¯bkl]p×q∈I([0,1]p×q), |
then the kronecker product of interval matrices A and B is
A⊗B=[a11×B⋯a1n×B⋮⋱⋮am1×B⋯amn×B]. | (2.10) |
(2) If
A=[a_ij,¯aij]m×n∈I([0,1]m×n), |
B=[b_kl,¯bkl]p×q∈I([0,1]p×q), |
then the STP of interval matrices A and B is
A⋉B=(A⊗Itn)×(B⊗Itp), | (2.11) |
where t=lcm(n,p) is the least common multiple of n and p.
(3) If
A=[a_ij,¯aij]m×n∈I([0,1]m×n), |
B=[b_kl,¯bkl]p×n∈I([0,1]p×n), |
then the khatri-rao product of interval matrices A and B is
A∗B=[Col1(A)⋉Col1(B) Col2(A)⋉Col2(B)⋯Coln(A)⋉Coln(B)]. | (2.12) |
Remark 2.1. In Definition 2.4, if n=p, then the STP of interval matrices degenerates to the ordinary interval matrix multiplication. Therefore, the STP of interval matrices is a generalization of interval matrices multiplication. In the context, the STP of interval matrices is ⋉, which is omitted by default.
Example 2.1. Given the interval matrices A and B,
A=[[0.2,0.4][0.4,0.5][0.6,1.0][0.8,0.9]], B=[[0,1][0.2,0.3][0.4,0.6][0.6,0.7][0.8,0.9][1,1][0.7,0.9][0.3,0.4]]. |
The kronecker product of the interval matrix A and the unit interval matrix I2 is
A⊗I2=[[0.2,0.4][0,0][0.4,0.5][0,0][0,0][0.2,0.4][0,0][0.4,0.5][0.6,1.0][0,0][0.8,0.9][0,0][0,0][0.6,1.0][0,0][0.8,0.9]]. |
The STP of interval matrices A and B is
A⋉BΔ=(A⊗I2)×B=[[0.16,0.70][0.28,0.47][0.32,1.54][0.60,0.93][0.44,0.81][0.32,0.60][1.04,1.71][0.84,1.36]]. |
The khatri-rao product of interval matrices A and B is
A∗B=[Col1(A)×Col1(B)Col2(A)×Col2(B)]=[[0.00,0.40][0.04,0.12][0.08,0.24][0.12,0.28][0.32,0.45][0.40,0.50][0.28,0.45][0.12,0.20][0.00,1.00][0.12,0.30][0.24,0.60][0.36,0.70][0.64,0.81][0.80,0.90][0.56,0.81][0.24,0.32]]T. |
According to the definition of STP of interval matrices, we can get the following properties.
Property 2.2. (1) Let A,B∈I([0,1]m×n),C∈I([0,1]p×q), then
(A+B)⋉C=A⋉C+B⋉C,C⋉(A+B)=C⋉A+C⋉B. | (2.13) |
(2) Let A∈I([0,1]m×n),B∈I([0,1]p×q) and C∈I([0,1]r×s), then
(A⋉B)⋉C=A⋉(B⋉C). | (2.14) |
(3) Let A∈I([0,1]m×n), C∈I([0,1]s) and R∈I([0,1]s) are column and row interval vectors, respectively, then
C⋉A=(Is⊗A)⋉C,R⋉A=(A⊗Is)⋉R. | (2.15) |
Let the interval type-2 fuzzy relation ˜R∈F(V×W), where the domain V={v1,v2,⋯,vn} and W={w1,w2,⋯,wp}, then the matrix form of interval type-2 fuzzy relation ˜R can be defined as
M˜R=[f˜R(v1,w1)μ˜R(v1,w1)⋯f˜R(v1,wp)μ˜R(v1,wp)⋮⋱⋮f˜R(vn,w1)μ˜R(vn,w1)⋯f˜R(vn,wp)μ˜R(vn,wp)]. | (2.16) |
μ˜R(vi,wk) and f˜R(vi,wk) represent the primary membership grade and secondary membership grade of IT2 FSs, respectively. For primary membership grade, it is composed of upper membership grade and lower membership grade; that is,
μ˜R(vi,wk)=[μ_˜R(vi,wk),¯μ˜R(vi,wk)]. |
The secondary membership grade of IT2 FSs equals one; that is, f˜R(vi,wk)=1, then the matrix form of interval type-2 fuzzy relation ˜R can be further described as
M˜R=[1[μ_˜R(v1,w1),¯μ˜R(v1,w1)]⋯1[μ_˜R(v1,ws),¯μ˜R(v1,wp)]⋮⋱⋮1[μ_˜R(vn,w1),¯μ˜R(vn,w1)]⋯1[μ_˜R(vn,ws),¯μ˜R(vn,wp)]]. | (2.17) |
Two common types of FREs exist in practical application [20]. One type is that the fuzzy relation is unknown, which is commonly used for designing fuzzy controllers. The other type is that the fuzzy input is unknown, which is commonly used for diagnosing diseases based on the symptom similarity. In terms of the aforementioned situations, it can be assumed that there are similar two types of IT2 FREs, as shown in Figures 1 and 2.
Type 1: assume ˜A∈F(U×V),˜B∈F(U×W). We seek an interval type-2 fuzzy relation ˜X∈F(V×W) such that it satisfies
˜A∘˜X=˜B. | (2.18) |
Type 2: assume ˜R∈F(V×W),˜B∈F(U×W). We seek an interval type-2 fuzzy input ˜X∈F(U×V) such that it satisfies
˜X∘˜R=˜B. | (2.19) |
Remark 2.2. Take a transpose of both sides of (2.19) to get ˜RT∘˜XT=˜BT. (2.19) is equivalent to (2.18), so we only need to consider the solvability of (2.18).
Definition 3.1. (1)
If={[α_,¯α]|0≤α_≤¯α≤1} |
is called the domain of interval-valued fuzzy logic, and the interval-valued fuzzy logic variable is P∈If. When α=[0,0] (orα=[1,1]), α degenerates into a classical logic variable.
(2)
Ik={[α_1,¯α1],[α_2,¯α2],⋯,[α_k,¯αk]}, [α_i,¯αi]∈If, |
i=1,⋯,k, then Ik is called the domain of k-valued interval-valued fuzzy logic.
(3) Mapping
f:Ik×Ik×⋯×Ik⏟r→Ik |
is called r-ary k-valued interval-valued logic function.
If
Ik={[α_1,¯α1],[α_2,¯α2],⋯,[α_k,¯αk]}, |
put the different upper and lower bounds of all interval-valued fuzzy logic variables in Ik into the ordered set Θ. If Θ does not contain zero and one, it needs to add zero or one:
Θ={ap|p=1,⋯,s;0≤a1<a2<⋯<as≤1}. |
In order to facilitate matrix calculation, each variable in Ik is represented as an interval vector. If α_i=am (1≤m≤s,m∈Z+) and ¯αi=an (1≤n≤s,n∈Z+), then the lower bound α_i can be represented by vector δms and the upper bound ¯αi can be represented by vector δns. Therefore,
[α_i,¯αi]∼[δms,δns]=δs[m,n]. |
Similar to the proof of theorem in paper [23], we can obtain Theorem 3.1.
Theorem 3.1. f is a r-ary k-valued interval-valued logic function, then there exists a unique structural matrix Mf, whose algebraic form is
f(x1,x2,⋯,xr)=Mf⋉ri=1[x_i,¯xi]. | (3.1) |
Remark 3.1. Structure matrix is also a special interval matrix that can be used to replace ∧,∨ and ¬ for algebraic operations.
In the following, we give the structure matrix of ∧,∨ and ¬.
Let
Ik={[α_1,¯α1],[α_2,¯α2],⋯,[α_k,¯αk]}. |
The ordered set Θ generated by Ik contains s different elements. To simply represent the structure matrix of ∧,∨ and ¬, we introduce a set of s-dimensional vectors
Uv=(1 2⋯v−1v⋯v⏟s−v+1),Vv=(v⋯v⏟v v+1 v+2⋯s), v=1,⋯,s. |
(1) The structure matrix of ∨:
Msd=[M_sd,¯Msd], M_sd=¯Msd=δs[U1U2⋯Us]. |
When s = 3, we have
M3d=δ3[[1,1][1,1][1,1][1,1][2,2] [2,2][1,1][2,2][3,3]]. |
(2) The structure matrix of ∧:
Msc=[M_sc,¯Msc], M_sc=¯Msc=δs[U1U2⋯Us]. |
When s = 3, we have
M3c=δ3[[1,1][2,2][3,3][2,2][2,2] [3,3][3,3][3,3][3,3]]. |
Definition 4.1. [20] In (2.17), the matrix constructed by the primary membership grade μ˜R(vj,wk) is called primary fuzzy matrix of interval type-2 fuzzy relation, denoted as ˜Rμ(μ˜R(vj,wk)) and abbreviated as ˜Rμ:
˜Rμ=[[μ_˜R(v1,w1),¯μ˜R(v1,w1)]⋯[μ_˜R(v1,wp),¯μ˜R(v1,wp)]⋮⋱⋮[μ_˜R(vn,w1),¯μ˜R(vn,w1)]⋯[μ_˜R(vn,wp),¯μ˜R(vn,wp)]]. |
Similarly, in (2.17), the matrix constructed by the secondary membership grade f˜R(vj,wk) is called secondary fuzzy matrix of interval type-2 fuzzy relation, denoted as ˜Rf(f˜R(vj,wk)) and abbreviated as ˜Rf:
˜Rf=[1⋯1⋮⋱⋮1⋯1]. |
Clearly, (2.18) is composed of the primary fuzzy matrix equation and secondary fuzzy matrix equation.
Definition 4.2. The IT2 FRE (2.18) can be divided into two parts: primary fuzzy matrix equation and secondary fuzzy matrix equation.
(1) The primary fuzzy matrix equation is
˜Aμ∘˜Xμ=˜Bμ, | (4.1) |
where ˜Aμ∈I([0,1]m×n), ˜Bμ∈I([0,1]m×p), ˜Xμ∈I([0,1]n×p) and ˜Xμ is unknown.
If
˜Xμ=[X_μ,¯Xμ]∈I([0,1]n×p) |
satisfies (4.1), then we call that ˜Xμ is the solution of (4.1). X_μ, ¯Xμ are lower and upper bound matrices of ˜Xμ, respectively.
If
˜Hμ=[H_μ,¯Hμ] |
is a solution of (4.1), and for any solution ˜Xμ of (4.1), there is ˜Xμ≤˜Hμ, then ˜Hμ is called the maximum solution of (4.1).
If
˜Jμ=[J_μ,¯Jμ] |
is a solution of (4.1), and for any solution ˜Xμ of (4.1), there is ˜Xμ≥˜Jμ, then ˜Jμ is called the minimal solution of (4.1).
If
˜Qμ=[Q_μ,¯Qμ] |
is a solution of (4.1), and for any solution ˜Xμ of (4.1), as long as ˜Xμ≤˜Qμ is satisfied, there is ˜Xμ=˜Qμ, then ˜Qμ is called the minimum solution of (4.1).
(2) The secondary fuzzy matrix equation is
˜Af∘˜Xf=˜Bf, | (4.2) |
where ˜Af∈Mm×n, ˜Bf∈Mm×p, ˜Xf∈Mn×p and ˜Xf is unknown.
The matrix ˜Xf satisfying (4.2) is called the solution of this equation. In (4.2), the elements of ˜Af and ˜Bf are all one, then the elements of ˜Xf are all one.
The primary fuzzy matrix Eq (4.1) is equivalent to
{A_μ∘X_μ=B_μ,¯Aμ∘¯Xμ=¯Bμ,X_μ≤¯Xμ. | (4.3) |
The conditions for the establishment of (4.3) are relatively difficult, so we first need to determine whether (4.1) has solutions.
Lemma 4.1. [24] Let
A=(aij)m×n, B=(bik)m×p. |
The T1 FRE A∘X=B has solutions if, and only if, ATαB is a solution of this equation and ATαB is the maximum solution of this equation. The α composition operation between fuzzy matrices is
ATαB=∧ni=1(aji)α(bik), |
where (aki)α(bij)={bij,aki>bij,1,aki≤bij.
Theorem 4.1. If the primary fuzzy matrix Eq (4.1) has solutions then
˜Hμ=[h_ik,¯hik]n×p={[H_μ,¯Hμ], ∀ h_ik≤¯hik,[H_μ′,¯Hμ], ∃ h_ik>¯hik. | (4.4) |
is a solution of this equation and ˜Hμ is the maximum solution of this equation.
In (4.4),
H_=A_TαB_=(h_ik)n×p, ¯H=¯AT¯αB=(¯hik)n×p, |
when
∀ h_ik≤¯hik, H_=(h_ik)n×p, ¯H=(¯hik)n×p. |
When ∃ h_ik>¯hik, we replace all elements of H_ that do not satisfy h_ik≤¯hik with ¯hik; thus, generating a new lower bound matrix H_μ′.
Proof. The primary fuzzy matrix Eq (4.1) has solutions, then T1 FREs
A_μ∘X_μ=B_μand¯Aμ∘¯Xμ=¯Bμ |
must have solutions. Lemma 4.1 implies that H_μ and ¯Hμ are solutions of T1 FREs
A_μ∘X_μ=B_μand¯Aμ∘¯Xμ=¯Bμ, |
respectively. H_μ and ¯Hμ must exist in either of the following two cases.
(1) For ∀ h_ij≤¯hij, we known that H_μ≤¯Hμ. H_μ and ¯Hμ are solutions of T1 FREs
A_μ∘X_μ=B_μand¯Aμ∘¯Xμ=¯Bμ, |
respectively. Hence,
˜Hμ=[H_μ,¯Hμ] |
satisfies (4.3) and ˜Hμ is a solution of the primary fuzzy matrix equation.
From Lemma 4.1, it follows that H_μ and ¯Hμ are maximum solutions of T1 FREs
A_μ∘X_μ=B_μand¯Aμ∘¯Xμ=¯Bμ, |
respectively. Clearly, X_μ≤H_μ and ¯Xμ≤¯Hμ, so
˜Hμ=[H_μ,¯Hμ] |
is the maximum solution of the primary fuzzy matrix equation.
(2) For ∃ h_ij>¯hij, we know that the newly generated matrix is H_μ′ and the matrix satisfies
˜Aμ∘H_μ′=˜BμandH_μ′≤¯Hμ. |
According to
˜Aμ∘H_μ′=˜Bμ, |
H_μ′ is a solution of T1 FRE
A_μ∘X_μ=B_μ. |
From Lemma 4.1, it follows that ¯Hμ is a solution of T1 FRE ¯Aμ∘¯Xμ=¯Bμ, respectively. Hence,
˜Hμ=[H_μ′,¯Hμ] |
satisfies (4.3) and ˜Hμ is a solution of the primary fuzzy matrix equation.
From Lemma 4.1, it is known that H_μ and ¯Hμ are, respectively, maximum solutions of T1 FREs
A_μ∘X_μ=B_μand¯Aμ∘¯Xμ=¯Bμ. |
According to the requirement that X_μ≤¯Xμ, we construct a new matrix H_μ′ based on H_μ. Clearly, X_μ≤H_μ′ and ¯Xμ≤¯Hμ, so
˜Hμ=[H_μ′,¯Hμ] |
is the maximum solution of the primary fuzzy matrix equation.
In summary, ˜Hμ is a solution of the primary fuzzy matrix Eq (4.1) and is the maximum solution of this equation.
If the primary fuzzy matrix Eq (4.1) has solutions, the next step is to explore how to construct parameter set solutions I∗(˜Xμ) and I∗(˜Xμ) of this equation.
First, take all the elements in ˜Aμand ˜Bμ and place the different upper and lower bounds of these elements in the the ordered set Θ:
Θ={ξi|i=1,⋯,r;0=ξ1<ξ2<⋯<ξr=1}. |
Construct an ordered interval-valued set Ψ by the ordered set Θ, defined as
Ψ={[ξ1,ξ1],[ξ1,ξ2],⋯,[ξ1,ξr];[ξ2,ξ2],[ξ2,ξ3],⋯,[ξ2,ξr];⋯;[ξr,ξr]}. |
Next, according to the order interval-valued set Ψ, we define two mappings necessary to construct the parameter set solution I∗(˜Xμ) and I∗(˜Xμ) of primary fuzzy matrix Eq (4.1).
Definition 4.3. Assuming x∈If, [ξi,ξj]∈Ψ.
(1) I∗: [x_,¯x]→Ψ is
I∗(x)=I∗([x_,¯x])=max{[ξi,ξj]∈Ψ|ξi≤x_,ξj≤¯x}. | (4.5) |
(2) I∗: [x_,¯x]→Ψ is
I∗(x)=I∗([x_,¯x])=min{[ξi,ξj]∈Ψ|ξi≥x_,ξj≥¯x}. | (4.6) |
Note that 1) When x_=ξi∈Ξ, ¯x=ξj∈Ξ,
I∗(x)=I∗(x)=[ξi,ξj]. |
2) When x_∉Ξ, ¯x=ξj∈Ξ, there exists a unique i such that ξi<x<ξi+1, then
I∗(x)=[ξi,ξj],I∗(x)=[ξi+1,ξj]. |
3) When x_=ξi∈Ξ, ¯x∉Ξ, there exists a unique j such that ξj<x<ξj+1, then
I∗(x)=[ξi,ξj],I∗(x)=[ξi,ξj+1]. |
4) When x_∉Ξ, ¯x∉Ξ, there exists a unique i and j such that ξi<x<ξi+1, ξj<x<ξj+1, then
I∗(x)=[ξi,ξj],I∗(x)=[ξi+1,ξj+1]. |
By Definition 4.3, it is not difficult to derive the following properties.
Property 4.1. Let
˜Aμ=[a_ij,¯aij]∈I([0,1]m×n), |
˜Bμ=[b_ik,¯bik]∈I([0,1]m×p), |
then,
(1) I∗(aij)=I∗(aij)=aij;I∗(bik)=I∗(bik)=bik.
(2) I∗(˜Aμ)=I∗(˜Aμ=˜Aμ;I∗(˜Bμ)=I∗(˜Bμ)=˜Bμ.
(3) I∗(˜Aμ∘˜Xμ)=I∗(˜Bμ)=˜Bμ;I∗(˜Aμ∘˜Xμ)=I∗(˜Bμ)=˜Bμ.
(4) ˜Xμ≤I∗(˜Xμ),I∗(˜Xμ)≤˜Xμ.
Property 4.2. Let x,y∈If, xi,yi∈If, i=1,⋯,n, then
(1) I∗(x)∨I∗(y)=I∗(x∨y); I∗(x)∨I∗(y)=I∗(x∨y).
(2) I∗(x)∧I∗(y)=I∗(x∧y); I∗(x)∧I∗(y)=I∗(x∧y).
(3) n∨i=1[I∗(xi)∧I∗(yi)]=I∗[n∨i=1(xi∧yi)].
(4) n∨i=1[I∗(xi)∧I∗(yi)]=I∗[n∨i=1(xi∧yi)].
Property 4.3. Let
˜Aμ=[a_ij,¯aij]∈I([0,1]m×n), |
˜Xμ=[x_jk,¯xjk]∈I([0,1]n×p), |
then,
(1) I∗(˜Aμ∘˜Xμ)=I∗(˜Aμ)∘I∗(˜Xμ).
(2) I∗(˜Aμ∘˜Xμ)=I∗(˜Aμ)∘I∗(˜Xμ).
Theorem 4.2. ˜Xμ is a solution of the primary fuzzy matrix Eq (4.1) if, and only if, I∗(˜Xμ) is a solution of the primary fuzzy matrix equation.
Proof. (Necessity) Assuming that ˜Xμ is a solution of the primary fuzzy matrix equation, it is clear that ˜Aμ∘˜Xμ=˜Bμ. By Property 4.1, it follows that
I∗(˜Aμ∘˜Xμ)=I∗(˜Bμ)=˜Bμ. | (4.7) |
According to the Property 4.3, we know that
I∗(˜Aμ∘˜Xμ)=I∗(˜Aμ)∘I∗(˜Xμ). |
From (4.7) we have
I∗(˜Aμ)∘I∗(˜Xμ)=˜Bμ. | (4.8) |
By the Property 4.1, it is not difficult to obtain I∗(˜Aμ)=˜Aμ. From (4.8) we have
˜Aμ∘I∗(˜Xμ)=˜Bμ. | (4.9) |
Formula (4.9) shows that I∗(˜Xμ) is a solution of the primary fuzzy matrix equation.
(Sufficiency) Assuming that I∗(˜Xμ) is a solution of the primary fuzzy matrix equation, it is clear that ˜Aμ∘I∗(˜Xμ)=˜Bμ. By Property 4.1, it follows that
˜Xμ≤I∗(˜Xμ). | (4.10) |
Using Property 2.1, we can get
˜Bμ≤˜Aμ∘˜Xμ≤˜Aμ∘I∗(˜Xμ). | (4.11) |
Formula (4.11) shows that ˜Xμ is a solution of the primary fuzzy matrix equation.
Therefore, the conclusion is correct.
Similarly, ˜Xμ is a solution of the primary fuzzy matrix Eq (4.1) if, and only if, I∗(˜Xμ) is a solution of the primary fuzzy matrix equation.
By Theorem 4.2, we can obtain the following corollary.
Corollary 4.1. (1) The interval matrix ˜Hμ is the maximum solution of primary fuzzy matrix Eq (4.1) if, and only if, I∗(˜Hμ) is the maximum solution of this equation.
(2) The interval matrix ˜Jμ is the minimum solution of primary fuzzy matrix Eq (4.1) if, and only if, I∗(˜Jμ) is the minimum solution of this equation.
(3) The interval matrix ˜Qμ is the minimal solution of primary fuzzy matrix Eq (4.1) if, and only if, I∗(˜Qμ) is the minimal solution of this equation.
If the primary fuzzy matrix Eq (4.1) has solutions, we next explore how to obtain parameter set solutions of this equation. By Theorem 4.2, the ordered interval-valued set Ψ is sufficient to inscribe the entire parameter set solutions of the primary fuzzy matrix equation.
First, the primary fuzzy matrix equation can be rewritten to
˜Aμ∘Colk(˜Xμ)=Colk(˜Bμ), | (4.12) |
where k=1,⋯,p.
In (4.12), the kth equality is equivalent to
([a_i1,¯ai1]∧[x_1k,¯x1k])∨([a_i2,¯ai2]∧[x_2k,¯x2k])∨⋯∨([a_in,¯ain]∧[x_nk,¯xnk])=[b_ik,¯bik], | (4.13) |
where i=1,⋯,m.
Second, the logical form of the primary fuzzy matrix equation is converted to algebraic form.
For simplicity of presentation, let
aij=[a_ij,¯aij],xjk=[x_jk,¯xjk], j=1,⋯,n. |
With the help of Theorem 3.1, the left hand side (LHS) of (4.13) can be expressed in algebraic form:
LHS=(Msd)n−1[(Mscai1x1k)(Mscai2x2k) ⋯(Mscainxnk)], | (4.14) |
where i=1,⋯,m.
By Property 2.2, we know that
x1k(Mscai2x2k)=(Is⊗Mscai2)x1kx2k. | (4.15) |
According to (4.15), (4.14) is simplified to
LHS=(Msd)n−1[(Mscai1)(Is⊗Mscai2)x1kx2k(Mscai3x3k)⋯(Mscainxnk)]. | (4.16) |
From Property 2.2, it follows that
x1kx2k(Mscai3x3k)=(Is2⊗Mscai3)x1kx2kx3k. | (4.17) |
According to (4.17), (4.16) is further simplified to
LHS=(Msd)n−1[(Mscai1)(Is⊗Mscai2)(Is2⊗Mscai2)x1kx2kx3k⋯(Mscainxnk)]. | (4.18) |
Repeating the process of (4.15)–(4.18), (4.14) is finally expressed as
LHS=(Msd)n−1[(Mscai1)(Is⊗Mscai2)(Is2⊗Mscai2)⋯(Isn−1⊗Mscain)⋉nj=1xjk]=(Msd)n−1[(Msc[a_i1,¯ai1])(Is⊗Msc[a_i2,¯ai2])⋯(Isn−1⊗Msc[a_in,¯ain])]⋉nj=1[x_jk,¯xjk]:=Li[x_k,¯xk], | (4.19) |
where i=1,⋯,m, and
Li=(Msd)n−1Msc[a_i1,¯ai1](Is⊗Msc[a_i2,¯ai2])⋯(Isn−1⊗Msc[ain,ain])⋉nj=1[x_jk,¯xjk],[x_k,¯xk]=⋉nj=1[x_jk,¯xjk], |
then (4.19) can be simplified to
Li[x_k,¯xk]=[b_ik,¯bik], | (4.20) |
where i=1,⋯,m.
Equation (4.20) is equivalent to
L[x_k,¯xk]=[b_k,¯bk], | (4.21) |
where
L=L1∗L2∗⋯∗Lm,[b_k,¯bk]=⋉mi=1[b_ik,¯bik], |
where "*" denotes the khatri-rao product of interval matrices.
According to the above procedure, the value of the kth row of ˜Xμ can be determined. Let k=1,2,⋯,p, and we can obtain the parameter set solutions of the primary fuzzy matrix equation.
A specific algorithm for solving all solutions of IT2 FRE (2.18) is given in the following.
Algorithm 4.1. The following steps are used to solve the solution set of IT2 FRE (2.18).
Step. 1. Decompose IT2 FRE (2.18) to construct the primary fuzzy matrix Eq (4.1).
Step. 2. Use Theorem 4.1 to determine if there are solutions to the primary fuzzy matrix equation. If the primary fuzzy matrix equation has solutions, then proceed as follows; otherwise, IT2 FRE (equ:IT2 FRE(a)) has no solution.
Step. 3. Construct an ordered set Θ from ˜Aμ and ˜Bμ
Θ={ξi|i=1,⋯,r;0=ξ1<ξ2<⋯<ξr=1}. |
We specify
ξi∼δir,ξj∼δjr,[ξi,ξj]=δr[i,j]. |
The elements in ˜Aμ and ˜Bμ can be represented as vectors to facilitate algebraic operations.
Step. 4. Construct (4.12) and convert it into the form of (4.21) to solve for the parameter set solutions of Colk(˜Xμ).
Step. 5. Let k=1,2,⋯,p, and we can get all parameter set solutions of (˜Xμ). Determine the maximum and minimum (or minimal) solutions of the primary fuzzy matrix equation.
Step. 6. Finally, based on the solution set of the primary fuzzy matrix equation and secondary fuzzy matrix equation, the solution set ˜X of IT2 FRE is constructed.
Consider the following IT2 FRE,
˜X∘˜R=˜B, | (5.1) |
where
˜X=[1[x_11,¯x11]1[x_12,¯x12]1[x_21,¯x21]1[x_22,¯x22]], ˜R=[1[0.3,0.7]1[0.2,0.3]1[0.1,0.5]1[0.5,0.7]],˜B=[1[0.1,0.5]1[0.1,0.3]1[0.2,0.5]1[0.5,0.7]]. |
First, taking a transpose on both sides of (5.1), we get
˜RT∘˜XT=˜BT. | (5.2) |
By decomposing IT2 FRE (5.2), we can obtain the primary fuzzy matrix equation and the secondary fuzzy matrix equation. From Definition 4.1, we only need to solve the primary fuzzy matrix equation to obtain the solution set of IT2 FRE. The primary fuzzy matrix equation of (5.2) can be expressed as
˜RμT∘˜XμT=˜BμT, | (5.3) |
where
˜RTμ=[[0.3,0.7][0.1,0.5[0.2,0.3][0.5,0.7]], ˜XTμ=[[x_11,¯x11][x_21,¯x21][x_12,¯x12][x_22,¯x22]],˜BTμ=[[0.1,0.5][0.2,0.5][0.1,0.3][0.5,0.7]]. |
Next, use Theorem 4.1 to determine if (5.3) has solutions.
H_μ=(A_μT)Tα(B_μT)=[0.30.10.20.5]Tα[0.10.20.10.5]=[0.10.20.11],¯Hμ=(¯AμT)Tα(¯BμT)=[0.70.50.30.7]Tα[0.50.50.30.7]=[0.50.50.31],˜Hμ=[[0.1,0.5][0.2,0.5][0.1,0.3][1,1]],˜RμT∘˜Hμ=[[0.1,0.5][0.2,0.5][0.1,0.3][0.5,0.7]]=˜BμT. |
According to the above calculation, H_μ≤¯Hμ, ˜Hμ is a solution of (5.3) and ˜Hμ is the maximum solution of this equation.
Let
Col1(˜XTμ)=[[x_11,¯x11] [x_21,¯x21]]T, |
which needs to satisfy the following logical equation.
{([0.3,0.7]∧[x_11,¯x11])∨([0.1,0.5]∧[x_21,¯x21])=[0.1,0.5],([0.2,0.3]∧[x_11,¯x11])∨([0.5,0.7]∧[x_21,¯x21])=[0.1,0.3]. | (5.4) |
However, solving (5.4) directly is relatively difficult, so it needs to be converted into algebraic form.
Construct the ordered set Θ based on ˜Aμ and ˜Bμ:
Θ={0,0.1,0.2,0.3,0.5,1}. |
Represent the elements in Θ as vectors
1∼δ17; 0.7∼δ27; 0.5∼δ37; 0.3∼δ47;0.2∼δ57; 0.1∼δ67; 0∼δ77. |
Convert (5.4) into an algebraic equation
{M7d(M7cδ7[4,2][x_11,¯x11])(M7cδ7[6,3][x_21,¯x21])=δ7[6,3],M7d(M7cδ7[5,4][x_11,¯x11])(M7cδ7[3,2][x_21,¯x21])=δ7[6,4]. |
Let
[x_1,¯x1]=[x_11,¯x11]⋉[x_21,¯x21], |
which is equivalent to
{M7dM7cδ7[4,2](I7⊗M7cδ7[6,3])[x_1,¯x1]=δ7[6,3],M7dM7cδ7[5,4](I7⊗M7cδ7[3,2])[x_1,¯x1]=δ7[6,4]. |
Let
L1=M7dM7c[δ47,δ27](I7⊗M7c[δ67,δ37]), |
L2=M7dM7c[δ57,δ47](I7⊗M7c[δ37,δ27]). |
This leads to
L⋉[x_1,¯x1]=[b_1,¯b1]. | (5.5) |
The MATLAB program provided in the literature [15] is improved so that it can calculate the STP of the interval matrix. (5.5) is calculated as
L=L1∗L2=δ49[[24,9][24,9][24,10][25,11][26,11][26,11][26,11][24,9][24,9][24,10][25,11][26,11][26,11][26,11][24,16][24,16][24,17][25,18][26,18][26,18][26,18][24,16][24,16][24,17][25,25][26,25][26,25][26,25][31,16][31,16][31,17][32,25][33,33][33,33][33,33][38,16][38,16][38,17][39,25][40,33][41,41][41,41][38,16][38,16][38,17][39,25][40,33][41,41][49,49]],[b_1,¯b1]=[δ67,δ37]⋉[δ67,δ47]=[δ4149,δ1849]. |
Solving for (5.5), we get
[x_1,¯x1]=[δi49,δj49], |
where i=41,42,48, j=18,19,20,21.
From the values of [x_1,¯x1], there are 3×4=12 parameter set solutions for Col1(˜XTμ), two of which do not satisfy x_i1≤¯xi1(i=1,2); then
(˜XTμ)11=δ7[[6,3][6,4]]T∼[[0.1,0.5][0.1,0.3]]T;(˜XTμ)21=δ7[[6,3][6,5]]T∼[[0.1,0.5][0.1,0.2]]T;(˜XTμ)31=δ7[[6,3][6,6]]T∼[[0.1,0.5][0.1,0.1]]T;(˜XTμ)41=δ7[[6,3][7,4]]T∼[[0.1,0.5][0,0.3]]T;(˜XTμ)51=δ7[[6,3][7,5]]T∼[[0.1,0.5][0,0.2]]T;(˜XTμ)61=δ7[[6,3][7,6]]T∼[[0.1,0.5][0,0.1]]T;(˜XTμ)71=δ7[[6,3][7,7]]T∼[[0.1,0.5][0,0.0]]T;(˜XTμ)81=δ7[[7,3][6,4]]T∼[[0,0.5][0.1,0.3]]T;(˜XTμ)91=δ7[[7,3][6,5]]T∼[[0,0.5][0.1,0.2]]T;(˜XTμ)101=δ7[[7,3][6,6]]T∼[[0,0.5][0.1,0.1]]T. |
Assuming
Col2(˜XTμ)=[[x_12,¯x12] [x_22,¯x22]]T, |
we have
L⋉[x_2,¯x2]=[b_2,¯b2], | (5.6) |
where the value of L has been obtained in (5.5),
[b_2,¯b2]=δ7[5,3]⋉δ7[3,2]=δ49[31,16]. |
Solving for (5.6), we get
[x_2,¯x2]=[δi49,δj49], |
where i=29,30,31, j=15,16,22,23,29,30,36.
Depending on the value of [x_2,¯x2], it follows that Col2(˜XTμ) has 3×7=21 parameter set solutions, six of which do not satisfy x_i2≤¯xi2(i=1,2); then
(˜XTμ)12=δ7[[5,3][1,1]]T∼[[0.2,0.5][1,1]]T;(˜XTμ)22=δ7[[5,4][1,1]]T∼[[0.2,0.3][1,1]]T;(˜XTμ)32=δ7[[5,5][1,1]]T∼[[0.2,0.2][1,1]]T;(˜XTμ)42=δ7[[5,3][2,1]]T∼[[0.2,0.5][0.7,1]]T;(˜XTμ)52=δ7[[5,3][2,2]]T∼[[0.2,0.5][0.7,0.7]]T;(˜XTμ)62=δ7[[5,4][2,1]]T∼[[0.2,0.3][0.7,1]]T;(˜XTμ)72=δ7[[5,4][2,2]]T∼[[0.2,0.3][0.7,0.7]]T;(˜XTμ)82=δ7[[5,5][2,1]]T∼[[0.2,0.2][0.7,1]]T;(˜XTμ)92=δ7[[5,5][2,2]]T∼[[0.2,0.2][0.7,0.7]]T;(˜XTμ)102=δ7[[5,3][3,1]]T∼[[0.2,0.5][0.5,1]]T;(˜XTμ)112=δ7[[5,3][3,2]]T∼[[0.2,0.5][0.5,0.7]]T;(˜XTμ)122=δ7[[5,4][3,1]]T∼[[0.2,0.3][0.5,1]]T;(˜XTμ)132=δ7[[5,4][3,2]]T∼[[0.2,0.3][0.5,0.7]]T;(˜XTμ)142=δ7[[5,5][3,1]]T∼[[0.2,0.2][0.5,1]]T;(˜XTμ)152=δ7[[5,5][3,2]]T∼[[0.2,0.2][0.5,0.7]]T. |
In summary, we can conclude that:
(1) The primary fuzzy matrix Eq (5.3) has a total of 10×15=150 parameter set solutions.
(2) The maximum solution of this equation is
˜Hμ=[(˜XTμ)11,(˜XTμ)12]=[[0.1,0.5][0.2,0.5][0.1,0.3][1,1]]. |
(3) The equation has no minimum solution and only two minimal solutions,
(˜Qμ)1=[(XTμ)71,(XTμ)152]=[[0.1,0.5][0.2,0.2][0,0][0.5,0.7]],(˜Qμ)2=[(XTμ)101,(XTμ)152]=[[0,0.5][0.2,0.2][0.1,0.1][0.5,0.7]]. |
(4) Based on the maximum and minimal solutions of the primary fuzzy matrix equation, we can work out all the parameter set solutions of the primary fuzzy matrix equation.
(˜XTμ)1=[[0.1,0.5][0.2,0.2≤¯x12≤0.5][0≤x_21≤0.1,0≤¯x21≤0.3][0.5≤x_22≤1,0.7≤¯x22≤1]],(˜XTμ)2=[[0≤x_11≤0.1,0.5][0.2,0.2≤¯x12≤0.5][0.1,0.1≤¯x21≤0.3][0.5≤x_22≤1,0.7≤¯x22≤1]]. |
(5) The solution set of IT2 FRE is
(˜XTμ)1=[1[0.1,0.5]1[0≤x_21≤0.1,0≤¯x21≤0.3]1[0.2,0.2≤¯x12≤0.5]1[0.5≤x_22≤1,0.7≤¯x22≤1]],(˜XTμ)2=[1[0≤x_11≤0.1,0.5]1[0.1,0.1≤¯x21≤0.3]1[0.2,0.2≤¯x12≤0.5]1[0.5≤x_22≤1,0.7≤¯x22≤1]]. |
This paper focused on the solution of IT2 FRE ˜A∘˜X=˜B. First, the STP of interval matrices and its properties were introduced, and the matrix representation of the interval-valued logic was given. Then, the IT2 FRE was considered as the primary fuzzy matrix equation and secondary fuzzy matrix equation. The solution of secondary fuzzy matrix is known, so only the primary fuzzy matrix equation needs to be solved. Moreover, the solvability of the primary matrix equation was studied, and a specific algorithm for solving IT2 FREs based on the STP of interval matrices was given. Finally, a numerical example was given to verify the effectiveness of the proposed method.
The authors declare they have not used Artificial Intelligence (AI) tools in the creation of this article.
This work was supported in part by the Research Fund for the Taishan Scholar Project of Shandong Province of China under Grant tstp20221103, and in part by the National Natural Science Foundation of China under Grant 62273201.
The authors declare that there are no conflicts of interest in this paper.
[1] |
Optical magnetic detection of single-neuron action potentials using quantum defects in diamond. Proc. Natl. Acad. Sci. U.S.A. (2016) 113: 14133-14138. ![]() |
[2] |
Multi-timescale systems and fast-slow analysis. Math. Biol. (2017) 287: 105-121. ![]() |
[3] |
Analysis of the Takens-Bogdanov bifurcation on m-parameterized vector fields. Internat. J. Bifur. Chaos Appl. Sci. Engrg. (2010) 20: 995-1005. ![]() |
[4] |
Dynamics of neurons in the pre-B![]() |
[5] |
Two-parameter bifurcation analysis of firing activities in the Chay neuronal model. Neurocomputing (2008) 72: 341-351. ![]() |
[6] |
H. Gu, Experimental observation of transition from chaotic bursting to chaotic spiking in a neural pacemaker, Chaos, 23 (2013), 023126. doi: 10.1063/1.4810932
![]() |
[7] |
F. Han, Z. Wang, Y. Du, X. Sun and B. Zhang, Robust synchronization of bursting Hodgkin-Huxley neuronal systems coupled by delayed chemical synapses, Int. J. Non. Linear. Mech, 70 (2015), 105-111. doi: 10.1016/j. ijnonlinmec. 2014.10.010
![]() |
[8] |
Neural excitability, spiking and bursting. Internat. J. Bifur. Chaos Appl. Sci. Engrg. (2000) 10: 1171-1266. ![]() |
[9] |
M. S. Kafraj, F. Parastesh and S. Jafari, Firing patterns of an improved Izhikevich neuron model under the effect of electromagnetic induction and noise, Chaos, Solitons Fractals, 137 (2020), 109782, 11 pp. doi: 10.1016/j. chaos. 2020.109782
![]() |
[10] | Y. A. Kuznetsov, Elements of Applied Bifurcation Theory, 3nd edition, Springer-Verlag, New York, 2004. |
[11] |
J. Li, Y. Wu, M. Du and W. Liu, Dynamic behavior in firing rhythm transitions of neurons under electromagnetic radiation, Acta Phys. Sin, 64 (2015), 030503. doi: 10.7498/aps. 64.030503
![]() |
[12] |
Bistability of cerebellar Purkinje cells modulated by sensory stimulation. Nature Neurosci. (2005) 8: 202-211. ![]() |
[13] |
B. Lu, S. Liu, X. Liu, X. Jiang and X. Jang, Bifurcation and spike adding transition in Chay-Keizer model, Internat. J. Bifur. Chaos Appl. Sci. Engrg., 26 (2016), 1650090, 13 pp. doi: 10.1142/S0218127416500905
![]() |
[14] |
Multiple modes of electrical activities in a new neuron model under electromagnetic radiation. Neurocomputing (2016) 205: 375-381. ![]() |
[15] |
Bifurcation analysis and diverse firing activities of a modified excitable neuron model. Cognitive Neurodynamics (2019) 13: 393-407. ![]() |
[16] |
Pattern formation in diffusive excitable systems under magnetic flow effects. Phys. Lett. A (2017) 381: 2264-2271. ![]() |
[17] |
Glucose modulates ![]() |
[18] |
J. Rinzel, Bursting oscillations in an excitable membrane model, Ordinary Partial Differ. Equations, Springer Berlin Heidelberg, Berlin, Heidelberg 1151 (1985), 304-316. doi: 10.1007/BFb0074739
![]() |
[19] |
Wild oscillations in a nonlinear neuron model with resets: (I) bursting, spike-adding and chaos. Discrete. Contin. Dyn. Syst. Ser. B (2017) 22: 3967-4002. ![]() |
[20] |
Real-time detection of stimulus response in cultured neurons by high-intensity intermediate-frequency magnetic field exposure. Integr. Biol (2018) 10: 442-449. ![]() |
[21] |
A. Saito, K. Wada, Y. Suzuki and S. Nakasono, The response of the neuronal activity in the somatosensory cortex after high-intensity intermediate-frequency magnetic field exposure to the spinal cord in rats under anesthesia and waking states, Brain Res., 1747 (2020), 147063. doi: 10.1016/j. brainres. 2020.147063
![]() |
[22] |
From plateau to pseudo-plateau bursting: Making the transition. Bull. Math. Biol. (2011) 73: 1292-1311. ![]() |
[23] |
Full system bifurcation analysis of endocrine bursting models. J. Theor. Biol. (2010) 264: 1133-1146. ![]() |
[24] |
Genesis of bursting oscillations in the Hindmarsh-Rose model and homoclinicity to a chaotic saddle. Phys. D (1993) 62: 263-274. ![]() |
[25] |
Mixed-mode oscillations and bifurcation analysis in a pituitary model. Nonlinear Dynam. (2018) 94: 807-826. ![]() |