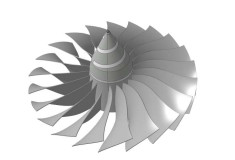
This paper proposes a near-field shape neural network (NSNN) to determine the shape of a sound-soft cavity based on a single source and several measurements placed on a curve inside the cavity. The NSNN employs the near-field measurements as input, and the output is the shape parameters of the cavity. The self-attention mechanism is employed to obtain the feature information of the near-field data, as well as the correlations among them. The weights and biases of the NSNN are updated through the gradient descent algorithm, which minimizes the error of the reconstructed shape of the cavity. We prove that the loss function sequence related to the weights is a monotonically bounded non-negative sequence, which indicates the convergence of the NSNN. Numerical experiments show that the shape of the cavity can be effectively reconstructed with the NSNN.
Citation: Weishi Yin, Jiawei Ge, Pinchao Meng, Fuheng Qu. A neural network method for the inverse scattering problem of impenetrable cavities[J]. Electronic Research Archive, 2020, 28(2): 1123-1142. doi: 10.3934/era.2020062
[1] | Ahmed A. Elgibaly, Mohamed Ghareeb, Said Kamel, Mohamed El-Sayed El-Bassiouny . Prediction of gas-lift performance using neural network analysis. AIMS Energy, 2021, 9(2): 355-378. doi: 10.3934/energy.2021019 |
[2] | Abdulrahman Th. Mohammad, Wisam A. M. Al-Shohani . Numerical and experimental investigation for analyzing the temperature influence on the performance of photovoltaic module. AIMS Energy, 2022, 10(5): 1026-1045. doi: 10.3934/energy.2022047 |
[3] | Nelson Batista, Rui Melicio, Victor Mendes . Darrieus-type vertical axis rotary-wings with a new design approach grounded in double-multiple streamtube performance prediction model. AIMS Energy, 2018, 6(5): 673-694. doi: 10.3934/energy.2018.5.673 |
[4] | Muluken Biadgelegn Wollele, Abdulkadir Aman Hassen . Design and experimental investigation of solar cooker with thermal energy storage. AIMS Energy, 2019, 7(6): 957-970. doi: 10.3934/energy.2019.6.957 |
[5] | Sri Kurniati, Sudirman Syam, Arifin Sanusi . Numerical investigation and improvement of the aerodynamic performance of a modified elliptical-bladed Savonius-style wind turbine. AIMS Energy, 2023, 11(6): 1211-1230. doi: 10.3934/energy.2023055 |
[6] | Joanna McFarlane, Jason Richard Bell, David K. Felde, Robert A. Joseph III, A. Lou Qualls, Samuel Paul Weaver . Performance and Thermal Stability of a Polyaromatic Hydrocarbon in a Simulated Concentrating Solar Power Loop. AIMS Energy, 2014, 2(1): 41-70. doi: 10.3934/energy.2014.1.41 |
[7] | Muzaffar Ali, Hafiz.M. Ali, Waqar Moazzam, M. Babar Saeed . Performance enhancement of PV cells through micro-channel cooling. AIMS Energy, 2015, 3(4): 699-710. doi: 10.3934/energy.2015.4.699 |
[8] | Honnurvali Mohamed Shaik, Adnan Kabbani, Abdul Manan Sheikh, Keng Goh, Naren Gupta, Tariq Umar . Measurement and validation of polysilicon photovoltaic module degradation rates over five years of field exposure in Oman. AIMS Energy, 2021, 9(6): 1192-1212. doi: 10.3934/energy.2021055 |
[9] | M. Boussaid, A. Belghachi, K. Agroui, N.Djarfour . Mathematical models of photovoltaic modules degradation in desert environment. AIMS Energy, 2019, 7(2): 127-140. doi: 10.3934/energy.2019.2.127 |
[10] | Saad S. Alrwashdeh . Investigation of the effect of the injection pressure on the direct-ignition diesel engine performance. AIMS Energy, 2022, 10(2): 340-355. doi: 10.3934/energy.2022018 |
This paper proposes a near-field shape neural network (NSNN) to determine the shape of a sound-soft cavity based on a single source and several measurements placed on a curve inside the cavity. The NSNN employs the near-field measurements as input, and the output is the shape parameters of the cavity. The self-attention mechanism is employed to obtain the feature information of the near-field data, as well as the correlations among them. The weights and biases of the NSNN are updated through the gradient descent algorithm, which minimizes the error of the reconstructed shape of the cavity. We prove that the loss function sequence related to the weights is a monotonically bounded non-negative sequence, which indicates the convergence of the NSNN. Numerical experiments show that the shape of the cavity can be effectively reconstructed with the NSNN.
Aeroengine is an important equipment to propel the aircraft forward and its total thrust is the sum of the thrust generated by the core engine and the turbine fan. The air flow state between adjacent blades of the turbine fan determines the thrust value of aeroengine. Since the experiments cost a lot, numerical simulations become an essential part of the blade geometry design and optimization [5,10,2,12,7]. Nevertheless, many difficulties arise in numerical simulation such as nonlinearity, high Reynolds number, complex three-dimensional (3D) geometrical domains, and boundary layer effect (see, e.g., [9]). In order to alleviate these difficulties, we will use the dimension splitting method to simulate the airflow state of aeroengine blade fan.
Based on the article [6], we establish a semi-geodesic coordinate system (called R-coordinate system), whose two basis vectors are on the manifold, and the other is along the hub circle. Thus, Navier-Stokes equations (NSEs) in the R-coordinate system can be rewritten as a set of membrane operator equations on the blade surface, and the bending operator equations along the hub circle [8]. By using Euler central difference scheme to approximate the third variable, the 3D NSEs become a series of two-dimensional (2D) equations with three variables. After successively iterations, the approximate solution to the NSEs can be obtained. Obviously, the significant feature of this new method is this method only solves the 2D problem in each sub-domain. In addition, it can alleviate the boundary layer effect by approaching adjacent surfaces, and the parameterized surface provides convenience for blade design and optimization.
The purpose of this work is to introduce our proposed method to simulate the flow state of the channel of aeroengine turbine fan. A lot of work has been carried out in the field of viscous flow and its applications in aeroengine turbine [16,15,4,11,14,13,3]. In this paper, a toy model is designed to give a comparison between our novel method and traditional methods. It turns out the new method shows a good performance for the toy model. Then we apply the proposed method to simulate the flow state of aeroengine turbine fan.
The present paper is built up as follows. In Section 2, some essential differential geometry knowledge is briefly introduced, then the R-coordinate system is established. Meanwhile, the NSEs' new form, splitting method and the variational form are formulated in Sections 3. Furthermore, we derive the finite element form in Section 4. Section 5 presents the numerical results, which contain the comparison of the new method and traditional methods, and the simulation results of aeroengine turbine fan.
In this section, we establish a new coordinate system according to the geometric shape of the blade and give the relationship between the new coordinate system and the rectangular coordinate system and the cylindrical coordinate system. To express concisely and clearly, we let Greek letters
Compared with the size of the aircraft engine fan, the thickness of the fan blade can be neglected. Thus the blade surface
ℜ(x)=rer+rΘ(r,z)eθ+zk. | (2.1) |
Here
The channel
ℜ(x;ξ)=rer+rθeθ+zk, | (2.2) |
where
Let
x1=z,x2=r,ξ=ε−1(θ−Θ(x)), |
it is clear that the Jacobian matrix
(r,θ,z)→(x1,x2,ξ):x1=z,x2=r,ξ=ε−1(θ−Θ(x)). | (2.3) |
In the R-coordinate system, the fixed region
Since the new coordinate is established, these basis vectors become a bridge to communicate different coordinate systems. Let
{er=cosθi+sinθj,eθ=−sinθi+cosθj,i=cosθer−sinθeθ,j=sinθer+cosθeθ. |
The basis vectors of the R-coordinate system are denoted as
{eα=∂αℜ=∂αxi+∂αyj+∂αzk,α=1,2,e3=∂∂ξ(ℜ)=∂x∂ξi+∂y∂ξj+∂z∂ξk, | (2.4) |
where
{x:=x(x1,x2,ξ)=rcosθ=x2cos(εξ+Θ(x1,x2)),y:=y(x1,x2,ξ)=rsinθ=x2sin(εξ+Θ(x1,x2)),z:=z(x1,x2,ξ)=x1. |
By straightforward calculation, the relationship between base vectors is as follows
{e1=x2Θ1eθ+k=−x2sinθΘ1i+x2cosθΘ1j+k,e2=Θ2x2eθ+er=(cosθ−x2sinθΘ2)i+(sinθ+x2cosθΘ2)j,e3=x2εeθ=−εx2sinθi+εx2cosθj,er=e2−ε−1Θ2e3,eθ=(εx2)−1e3,k=e1−ε−1Θ1e3,i=cosθe2−(ε−1cosθΘ2+(εx2)−1sinθ)e3,j=sinθe2+((εx2)−1cosθ−ε−1Θ2sinθ)e3, | (2.5) |
where
The metric tensor
aαβ:=eαeβ=δαβ+r2ΘαΘβ, |
and it is easy to see that
a=det(aαβ)=1+r2|∇Θ|2>0, | (2.6) |
where
Similarly, the covariant and contravariant components of metric tensor are
gij=eiej,gij=eiej. |
By calculation, they can be expressed as (cf.[5])
{gαβ=aαβ,g3β=gβ3=εr2Θβ,g33=ε2r2,gαβ=δαβ,g3β=gβ3=−ε−1Θβ,g33=(εr)−2a. | (2.7) |
As described in our previous article [6], for given
In this section, we derive the flows state governed by the incompressible rotational NSEs through employing differential operators in the R-coordinate system. Because of the lighter mass of air, its body force is neglected and the rotational NSEs can be expressed as
{∂u∂t−νΔu+(u∇)u+2ω×u+∇p=−ω×(ω×r),divu=0. | (3.1) |
For a time-dependent problem, we usually discretize the problem in time and solve the static problem at each time step, then the time-dependent problem is reduced to a static problem at each time step. If we consider an implicit time discretization (e.g., the backward Euler method) to equation (3.1) with time-step size
{u−νκΔu+κ(u∇)u+2κω×u+κ∇p=−κω×(ω×r)+fu,divu=0, | (3.2) |
where
Assume that
Γijk=ei⋅ejk,∇kui=∂ui∂xk+Γikmum,∗Γαβσ=eα⋅eβλ,∗∇βuα=∂uα∂xβ+∗Γαβσuσ, |
where
Lemma 3.1. [6] By denoting
1). The Laplace operator
Δui=˜Δui+2g3γ∗∇γ∂ui∂ξ+g33∂2ui∂ξ2+Li3γ∂uγ∂ξ+Li33∂u3∂ξ+Liσγ∗∇σuγ+Li0γuγ+δi3L3σ3∗∇σu3, | (3.3) |
where
{Lα3γ=−(εr)−1(Θ2δαγ+2aδ2αΘγ)−˜ΔΘδαγ,L33γ=−2(εr)−2(r2ΘβΘβγ−r−1δ2γ),Lα33=−2rδ2α,L333=−(εr)−1(r˜ΔΘ+Θ2),Lασγ=2rδ2αΘσΘγ−(r|∇Θ|2−r−1)δ2σδαγ,L3σγ=2(εr)−1(δ2σΘγ+rΘγσ)Lα0γ={Θγ[(a+1)Θ2+r˜ΔΘ]−r−2δ2γ+rΘβΘβγ}δ2α,L30γ=(εr)−1(3Θ2γ+2(r2Θ2αΘα+rΘ22)Θγ+)rΘααγ+r−2Θ2δ2γ,L3σ3=(3r−1−r|∇Θ|2)δ2σ, | (3.4) |
and
2). Its related items coriolis force and centrifugal force satisfy, respectively,
C=2κω×u=κCijujei, | (3.5) |
fic=−κεijkωj(→ω×r)k=κεijkgjmωmεklnωlrn=κεijkgjmωmεklnωlgn2r, | (3.6) |
where
3). The pressure gradient is
∇p=[gαβ∂p∂xβ+gα3∂p∂ξg3β∂p∂xβ+g33∂p∂ξ]=[∂p∂xα−ε−1Θα∂p∂ξ−ε−1Θβ∂p∂xβ+(rε)−2a∂p∂ξ]. |
4). The nonlinear term in equation (3.1) is
B(u,u)=κ(u∇)u=κBi(u)ei=κ[uβ∗∇βuα+u3∂uα∂ξ+nαkmukumuβ∗∇βu3+u3∂u3∂ξ+n3kmukum], | (3.7) |
where
{nαλσ=0,nα3β=nαβ3=−rεδ2αΘβ,nα33=−rε2δ2α,n333=rεΘ2,n3λσ=(rε)−1(a2λΘσ+δ2σΘλ)+ε−1Θλσ,n33β=n3β3=r−1a2β. |
5). The mass conservation formula can be rewritten as
div u=∂uα∂xα+∂u3∂ξ+u2r,∗divu=∂uα∂xα−rΘ2Θσuσ. | (3.8) |
By Lemma 3.1, the incompressible rotational NSEs in the R-coordinate system can be rewritten as
{ui+κ{uβ∗∇βui+u3∂ui∂ξ+nikmukum+Cijuj−ν[˜Δui+2g3γ∗∇γ∂ui∂ξ+g33∂2ui∂ξ2+Li3γ∂uγ∂ξ+Li33∂u3∂ξ+Liσγ∗∇σuγ+Li0γuγ+δi3L3σ3∗∇σu3]+giβ∂p∂xβ+gi3∂p∂ξ}=Fi,∂uβ∂xβ+∂u3∂ξ+u2r=0, | (3.9) |
where
Fi=fic+fiu. |
And its channel region and boundaries are
{Ω={(x1,x2,ξ)|(x1,x2)∈D,−1≤ξ≤1,},∂Ω=Γin∪Γout∪ℑ+∪ℑ−∪Γt∪Γb. | (3.10) |
The initial and boundary value conditions are
u|t=0=uo,u|ℑ+∪ℑ−=0,u|Γin=uin. | (3.11) |
In this section, the finite-element-difference method will be presented. The first step is to divide the interval
[−1,+1]=∪N−1k=0[ξk,ξk+1],ξk=−1+kτ,k=0,1,⋯N−1. |
Then the domain
∂w∂ξ≃wk+1−wk−12τ,∂2w∂ξ2≃wk+1−2wk+wk−1τ2. | (3.12) |
where
[w]+k:=wk+1+wk−1τ2,[w]−k:=wk+1−wk−12τ. | (3.13) |
Plugging (3.13) into (3.9), we obtain
{ui+κ{uβ∗∇βui+u3[ui]−k+nikmukum−ν[˜Δui+2g3γ∗∇γ[ui]−k+g33([ui]+k−2uiτ2)+Li3γ[uγ]−k+Li33[u3]−k+Liσγ∗∇σuγ+Li0γuγ+δi3L3σ3∗∇σu3]+giβ∂p∂xβ+gi3[p]−k+Cijuj}=˜Fik,∂uα∂xα+[u3]−k+u2r=0, | (3.14) |
where
˜Fik:=1τ∫ξk+1ξkFi. | (3.15) |
And its boundary conditions are
uk|γ0=0,uk|γin=uin,∂D=γ0∪γin∪γout, | (3.16) |
where
Introduce the Hilbert space
V(D)={u∈H1(D)×H1(D)×H1(D),u=0|γ0∪γin}, |
then its inner product and norms are given, respectively, by
(w,v)D=∫Ωaijwivj√adx,aij={aαβ=aαβ,aα3=a3α=0,a33=1},|w|21,D=∑α∑j‖∂αwj‖20,D,‖w‖20,D=∑j‖wj‖20,D,‖w‖21,D=|w|21,D+‖w‖20,D. |
Without the ambiguity, the index `D' is often omitted.
For clarity and simplicity, we denote
{Li(k):=−κν(˜Δuik+Liσγ∗∇σuγk+Li0γuγk−2g33τ−2uik+δi3L3σ3∗∇σu3k)+uik,Bi(k):=κ(uβk∗∇βuik+nilmulkumk),Ci(k):=κCimumk,Si:=−κν(2g3γ∗∇γ[ui]−k+g33[ui]+k+Li3γ[uγ]−k+Li33[u3]−k)+κu3k[ui]−k,Pi:=κgi3[p]−k. | (3.17) |
Meanwhile, we denote
{Lα(k)+Bα(k)+Cα(k)+κgαβ∂pk∂xβ=ˆFαk,L3(k)+B3(k)+C3(k)+κg3β∂pk∂xβ=ˆF3k,∂uα∂xα+[u3]−k+u2r=0. | (3.18) |
Thus, the variational problem corresponding to the boundary value problem of the NSEs is as follows:
{Seek uk∈L∞(0,T;V(D))+uin, pk∈L2(D),k=0,1,2,⋯,N−1,s.t.a(uk,v)+(C(uk),v)+(L(uk),v)+b(uk,uk,v)−(pk,m(v))=(ˆFk,v)−<h,v>γout,∀v∈V(D),(∂uαk∂xα+u2kr,q)=([u3]−k,q),∀q∈L2(D), | (3.19) |
where the linear, bilinear and trilinear forms are given, respectively,
{a(uk,v)=νκ(aij∂λuik,∂λvj)+νκ(ˆaijuik,vj)+(aijui,vj),ˆaij=aijr−2aα2τ,(C(uk),v)=κ(C∗i,vi),C∗β=aαβCαmumk,C∗3=C3mumk,(L(uk),v)=νκ(L∗i,vi),L∗β=∂λaαβ∂λuαk−aαβ(Lασν∗∇σuνk+Lα0νuνk),L∗3=−(L3σλ∗∇σuλk+L3σ3∗∇σu3k+L30σuσk),b(uk,uk,v)=(κaijBi(k),vj),(ˆFk,v)=(aijˆFik,vj),<h,v>γout=−∫γoutκμaij∂λuikvjnλdl+∫γoutκpk(aαλvα−εΘβv3)nλdl,m(v)=κ(∂αvα−εΘβ∂βv3−ε˜ΔΘv3). | (3.20) |
In this section, we apply the Taylor-Hood elements, i.e.,
Vh:={vh∈C0(ˉΩ);vh|K∈P2(K),∀K∈Th},Mh:={ph∈C0(ˉΩ);ph|K∈P1(K),∀K∈Th}. | (4.1) |
The product space
{Seek wh∈Vh, ph∈Mh s.t.a(wh,vh)+(C(wh),vh)+(L(wh),vh)+b(wh,wh,vh)−(ph,m(vh))=(ˆFk,vh)−<h,vh>γout,∀vh∈Vh,(∂wαh∂xα+w2hr,qh)=−([w3h]−k,qh),∀qh∈Mh. | (4.2) |
Suppose the finite element basis functions are denoted as
φi(x),i=1,2,⋯,NG1, ϕi(x), i=1,2,⋯NG2, |
where
wmh=NG1∑i=1Ximφi(x),ph=NG2∑i=1Piϕi(x),m=1,2,3,vkh=NG1∑i=1Yikφi(x),qh=NG2∑i=1Qiϕi(x),k=1,2,3. | (4.3) |
Assume solution vector
Wm={X1m,X2m,⋯XNG1m}T,W={W1,W2,W3}T,Vk={Y1k,Y2k,⋯YNG1k}T,V={V1,V2,V3}T,P={P1,⋯,PNG2}T,Q={Q1,⋯,QNG2}T. | (4.4) |
Substitute (4.4) into (4.2), we obtain the result on 2D membrane operator:
{a(wh,vh)=KαβijXiαYjβ,(L(wh),vh)=LτβijXiτYjβ,(C(wh,ω),vh)=CτβijXiτYjβ+C3βijXi3Yjβ,A0(wh,vh)=a(wh,vh)+(L(wh),vh)+(C(wh,ω),vh)=[KτβijXiτ+K3βijXi3]Yjβ,b(wh,wh,vh)=blm,βik,jXilXkmYjβ,(ph,m(vh))=B∗βijPiYjβ | (4.5) |
where
{Kαβij=κν[(aαβ∂λφi,∂λφj)+α2τ(aαβar−1φi,r−1φj)]+(aαβφi,φj),Lτβij=κν(∂λaαβ∂λφiδατ,φj)−κνaαβ((Lασν∂σδντ+LασνΓνσλδλτ+Lα0νδντ)φi,φj),Cτβij=(κaαβCατφi,φj),C3βij=(κaαβCα3φi,φj),Kτβij=Kαβijδτα+Lτβij+Cτβij,K3βij=C3βij,blm,βik,j=κ(aαβ{δlλφi[∂λφkδαm+Γαλσφkδσm]+nαlmφiφk},φj),B∗βij=κ(ϕi,∂βφj). |
Proof. In this proof, we only prove
∗∇σwν=∂σwν+Γνσλwλ,∗∇σw3=∂σw3, |
we obtain
(L(wh),vh)=κ(−νaαβLασν∗∇σwνh−νaαβLα0νwνh,vβh)+κ(ν∂λaαβ∂λwαh,vβh)=κ(−νaαβLασν[∂σwνh+Γνσλwλh]−νaαβLα0νwνh,vβh)+κ(ν∂λaαβ∂λwαh,vβh)=κν(∂λaαβ∂λwαh−aαβLασν∂σwνh,vβh)−κν(aαβLασνΓνσλwλh+aαβLα0νwνh,vβh)=κν(∂λaαβ∂λφiδατ−aαβLασν∂σφiδντ,φj)XiτYjβ−κν(aαβLασνΓνσλφiδλτ+aαβLα0νφiδντ,,φj)XiτYjβ=LτβijXiτYjβ. |
The remainder of the argument is analogous and is left to the reader.
Next, we will give the discrete scheme on the right side of the formula (4.2). According to the definition of inner product in space
{(ˆFk,v)=(aαβˆFαk,vβ)=ˆFβjYjβ,<h,v>γout=∫−κνaαβ∂λφiXiαφjYjβnλdl+∫κaαβϕiPiφjYjαnβdl=ˆHβjYjβ. |
Thus, we obtain
KτβijXiτ+K3βijXi3+blm,βik,jXilXkm−B∗βijPi=Fβj, | (4.6) |
where
{a(w3h,v3h)=K33ijXi3Yj3,(L(w3h),v3h)=Lτ3ijXiτYj3+L33ijXi3Yj3,(C(w3h,ω),v3h)=C3αijXiαYj3+C33ijXi3Yj3,A0(w3h,v3h)=[Kα3ijXiα+K33ijXi3]Yj3,b(w3,w3,v3)=blm,3ik,jXilXkmYj3,(ph,m(vh))=B∗3ijPiYj3, | (4.7) |
where
{K33ij=κν(∂λφi,∂λφj)+κνα2τ(ar−1φi,r−1φj)+(φi,φj),Lτ3ij=−(κν[L3σλ(∂σφiδλτ+Γλστφi)+L30τφi],φj),L33ij=−(κνL3σ3∂σφi,φj),Cα3ij=(κC3αφi,φj),C33ij=(κC33φi,φj),K33ij=K33ij+L33ij+C33ij,Kα3ij=Lα3ij+Cα3ij,blm,3ik,j=κ(φiδβlδ3m∂βφk+n3lmφiφk,φj),B∗3ij=−κ(ϕi,εΘβ∂βφj+ε˜ΔΘφj). |
And its right terms are
{(ˆF3k,v3)=(ˆF3k,Yj3φj)=ˆF3jYj3,<h3,v3>γout=−∫κν∂λφiXi3φjYj3nλdl−∫κεΘβϕiPiφjYj3nλdl=ˆH3jYj3. |
It is easy to verify it, so its proof will not be given here. Then the bending operators becomes
Kα3ijXiα+K33ijXi3+bml,3ik,jXimXkl−B∗3ijPi=F3j, | (4.8) |
where
Finally, we obtain the finite element algebraic equations of equation (3.19), i.e.,
{KτβijXiτ+K3βijXi3+blm,βik,jXilXkm−B∗βijPi=Fβj,Kα3ijXiα+K33ijXi3+bml,3ik,jXimXkl−B∗3ijPi=F3j,MijXiα=−(φi[Xi3]−k,ϕj), | (4.9) |
where
Mα∗ij=(∂αφi+r−1φiδα2). |
In this section, for certain examples, the results of the new algorithm program and the traditional algorithm program will be compared to verify the accuracy of the new algorithm program. And the new algorithm program shows good performance. Then, based on the new algorithm, the flow state of the gas in the fan channel of an aeroengine is given.
In this part, a simple model is provided to give the comparison between results obtained by the dimension splitting method (DS method) and traditional 3D method (T3D method). In this example, we adopt stationary model and assume
51 2D-manifolds and 4987 elements per surface are used to partition the channel and for DS method while 254867 elements are used for T3D method. In this model, we assume
As mentioned above, the flow state determines the maximum thrust that aeroengine turbine fan can provide. Our main purpose is using the DS method to simulate the flow state of the aeroengine turbine fan. In addition to the alleviation of boundary layer effects and make parallelism easier, DS method can give one clear design objective
In this article, we adopt the blades provided by the partner airlines which shape can be shown in Figure 15. Its meshes in the R-coordinate system are shown in Figure 1, and 51 2D-manifolds are used in this case. The velocity at inlet is 80 m/s and the rotating angular velocity is 80 rad/s. Figure 11 – Figure 12 show the pressure distribution on the blade surface. Because of the rotation, positive pressure surface and negative pressure surface have different pressure distribution. The pressure distribution of the positive pressure surface is higher than that of the negative pressure surface. Because the blade is cocked up at the bottom (see Figure 15), the pressure distribution near the bottom of the blade is obviously different from other places. Through formula transformation, we present the 3D model of blade pressure and velocity in Figure 13 – Figure 14.
Meanwhile, we show the velocity distribution at outlet in Figure 16. The velocity on the outside is significantly higher than that on the inside, and the velocity in the middle of the channel is also higher than that near the blade, which is consistent with common sense. From Figure 16, we can find that the maximum velocity is located at the edge of the blade, which can reach 130 m/s.
In this work, we conduct numerical simulations for 3D flow in the flow channel of the aeroengine turbine fan based on the dimension splitting method. However, the simulation of the flow states between the blades is not the ultimate goal. Our ultimate goal is to use the simulation results to design or optimize the blades. Meanwhile, the parameterized blade surface provides convenience for blade design and optimization. Thus, it will be the focus of follow-up research to optimize the blade by combining the inverse problem. In addition, compressible flow and boundary layer phenomenons are also the focus of the follow-up research.
The work of G. Ju was partially supported by the NSF of China (No. 11731006), the Shenzhen Sci-Tech Fund (No. JCYJ20170818153840322), and Guangdong Provincial Key Laboratory of Computational Science and Material Design No. 2019B030301001. The work of R. Chen was supported by the NSF of China (No. 61531166003) and Shenzhen Sci-Tech fund (No. JSGG20170824154458183 and ZDSYS201703031711426). The work of J. Li was partially supported by the NSF of China (No. 11971221) and Shenzhen Sci-Tech fund (No. JCYJ20190809150413261). The work of K. Li was supported by the NSF of China under the grant No. 10971165 and 10771167.
[1] |
MoDL: Model based deep learning architecture for inverse problems. IEEE Transactions on Medical Imaging (2019) 38: 394-405. ![]() |
[2] |
M. N. Akinci, Detection of the cavities inside a target with near field orthogonality sampling method, 2018 18th Mediterranean Microwave Symposium (MMS), IEEE, (2018), 391–393. doi: 10.1109/MMS.2018.8612107
![]() |
[3] |
Determining a sound-soft polyhedral scatterer by a single far-field measurement. Proc. Amer. Math. Soc. (2005) 133: 1685-1691. ![]() |
[4] | E. Blåsten and H. Liu, On corners scattering stably, nearly non-scattering interrogating waves, and stable shape determination by a single far-field pattern, preprint, arXiv: 1611.03647. |
[5] | E. Blåsten and H. Liu, Recovering piecewise constant refractive indices by a single far-field pattern, preprint, arXiv: 1705.00815. |
[6] |
The inverse scattering problem for a penetrable cavity with internal measurements. AMS Contemporary Mathematics (2014) 615: 71-88. ![]() |
[7] |
Uniqueness in an inverse scattering problem within non-trapping polygonal obstacles with at most two incoming waves. Inverse Problems (2003) 19: 1361-1384. ![]() |
[8] |
D. Colton and R. Kress, Inverse Acoustic and Electromagnetic Scattering Theory, 4nd edition, Applied Mathematical Sciences, 93. Springer, Cham, New York, 2019. doi: 10.1007/978-3-030-30351-8
![]() |
[9] | (2017) Deep Learning. London: MIT Press. |
[10] |
Reconstruction of a crack with the incident waves and measurements inside a penetrable cavity. J. Inverse Ill-Posed Probl. (2019) 27: 643-656. ![]() |
[11] |
The inverse scattering problem for a partially coated cavity with interior measurements. Appl. Anal. (2014) 93: 936-956. ![]() |
[12] |
Testing the integrity of some cavity-the Cauchy problem and the range test. Appl. Numer. Math. (2008) 58: 899-914. ![]() |
[13] |
The method of fundamental solutions for the identification of a scatterer with impedance boundary condition in interior inverse acoustic scattering. Eng. Anal. Bound. Elem. (2018) 92: 218-224. ![]() |
[14] |
An inverse scattering approach for geometric body generation: A machine learning perspective. Mathematics in Engineering (2019) 1: 800-823. ![]() |
[15] |
Near-field imaging of interior cavities. Commun. Comput. Phys. (2015) 17: 542-563. ![]() |
[16] |
X. Liu, The factorization method for cavities, Inverse Problems, 30 (2014), 015006, 18 pp. doi: 10.1088/0266-5611/30/1/015006
![]() |
[17] |
H. Liu, A global uniqueness for formally determined inverse electromagnetic obstacle scattering, Inverse Problems, 24 (2008), 035018, 13 pp. doi: 10.1088/0266-5611/24/3/035018
![]() |
[18] |
Stable determination of sound-hard polyhedral scatterers by a minimal number of scattering measurements. J. Differential Equations (2017) 262: 1631-1670. ![]() |
[19] |
Mosco convergence for H(curl) spaces, higher integrability for Maxwell's equations, and stability in direct and inverse EM scattering problems. J. Eur. Math. Soc. (JEMS) (2019) 21: 2945-2993. ![]() |
[20] |
Uniqueness in an inverse acoustic obstacle scattering problem for both sound-hard and sound-soft polyhedral scatterers. Inverse Problems (2006) 22: 515-524. ![]() |
[21] |
On unique determination of partially coated polyhedral scatterers with far field measurements. Inverse Problems (2007) 23: 297-308. ![]() |
[22] |
H. Qin and F. Cakoni, Nonlinear integral equations for shape reconstruction in the inverse interior scattering problem, Inverse Problems, 27 (2011), 035005, 17 pp. doi: 10.1088/0266-5611/27/3/035005
![]() |
[23] |
The inverse scattering problem for cavities. Appl. Numer. Math. (2012) 62: 699-708. ![]() |
[24] |
The inverse scattering problem for cavities with impedance boundary condition. Adv. Comput. Math. (2012) 36: 157-174. ![]() |
[25] |
Shape reconstruction in inverse scattering by an inhomogeneous cavity with internal measurements. SIAM J. Imaging Sci. (2019) 12: 788-808. ![]() |
[26] |
Stable determination of sound-soft polyhedral scatterers by a single measurement. Indiana Univ. Math. J. (2008) 57: 1377-1408. ![]() |
[27] |
Embedding deep learning in inverse scattering problems. IEEE Transactions on Computational Imaging (2020) 6: 46-56. ![]() |
[28] |
The reciprocity gap functional method for the inverse scattering problem for cavities. Appl. Anal. (2016) 95: 1327-1346. ![]() |
[29] |
Convergence of gradient method for a fully recurrent neural network. Soft Computing (2010) 14: 245-250. ![]() |
[30] |
D. Xu, Z. Li, W. Wu, X. Ding and D. Qu, Convergence of gradient descent algorithm for a recurrent neuron, International Symposium on Neural Networks, Springer, Berlin, Heidelberg, (2007), 117–122. doi: 10.1007/978-3-540-72395-0_16
![]() |
[31] |
W. Yin, W. Yang and H. Liu, A neural network scheme for recovering scattering obstacles with limited phaseless far-field data, Journal of Computational Physics, 417 (2020), 109594. doi: 10.1016/j.jcp.2020.109594
![]() |
[32] |
A decomposition method for an interior inverse scattering problem. Inverse Problems and Imaging (2013) 7: 291-303. ![]() |
[33] |
D. Zhang, Y. Wang, Y. Guo and J. Li, Uniqueness in inverse cavity scattering problems with phaseless near-field data, Inverse Problems, 36 (2020), 025004. doi: 10.1088/1361-6420/ab53ee
![]() |
1. | Roland Fürbacher, Gerhard Liedl, Gabriel Grünsteidl, Andreas Otto, Icing Wind Tunnel and Erosion Field Tests of Superhydrophobic Surfaces Caused by Femtosecond Laser Processing, 2024, 4, 2674-032X, 155, 10.3390/wind4020008 | |
2. | Xiaojing Tian, Weiqi Ye, Liang Xu, Anjian Yang, Langming Huang, Shenglong Jin, Optimization research on laminated cooling structure for gas turbines: A review, 2025, 13, 2333-8334, 354, 10.3934/energy.2025014 |