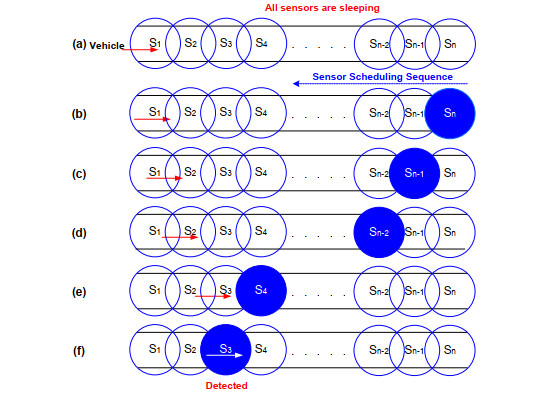
Citation: Joje Mar P. Sanchez, Marchee T. Picardal, Marnan T. Libres, Hedeliza A. Pineda, Ma. Lourdes B. Paloma, Judelynn M. Librinca, Reginald Raymund A. Caturza, Sherry P. Ramayla, Ruby L. Armada, Jay P. Picardal. Characterization of a river at risk: the case of Sapangdaku River in Toledo City, Cebu, Philippines[J]. AIMS Environmental Science, 2020, 7(6): 559-574. doi: 10.3934/environsci.2020035
[1] | Nicholas J. D. Wright . A review of the direct targets of the cannabinoids cannabidiol, Δ9-tetrahydrocannabinol, N-arachidonoylethanolamine and 2-arachidonoylglycerol. AIMS Neuroscience, 2024, 11(2): 144-165. doi: 10.3934/Neuroscience.2024009 |
[2] | Yinghua Zhang, Xinyue Wei, Wenhao Zhang, Feng Jin, Wenbo Cao, Mingjin Yue, Saijun Mo . The BDNF Val66Met polymorphism serves as a potential marker of body weight in patients with psychiatric disorders. AIMS Neuroscience, 2024, 11(2): 188-202. doi: 10.3934/Neuroscience.2024012 |
[3] | Abdulrahman Alhamyani, Prabhat R Napit, Haider Ali, Mostafa MH Ibrahim, Karen P Briski . Ventrolateral ventromedial hypothalamic nucleus GABA neuron adaptation to recurring Hypoglycemia correlates with up-regulated 5′-AMP-activated protein kinase activity. AIMS Neuroscience, 2021, 8(4): 510-525. doi: 10.3934/Neuroscience.2021027 |
[4] | Khue Vu Nguyen . β-Amyloid precursor protein (APP) and the human diseases. AIMS Neuroscience, 2019, 6(4): 273-281. doi: 10.3934/Neuroscience.2019.4.273 |
[5] | Ubaid Ansari, Jimmy Wen, Burhaan Syed, Dawnica Nadora, Romteen Sedighi, Denise Nadora, Vincent Chen, Forshing Lui . Analyzing the potential of neuronal pentraxin 2 as a biomarker in neurological disorders: A literature review. AIMS Neuroscience, 2024, 11(4): 505-519. doi: 10.3934/Neuroscience.2024031 |
[6] | Ubaid Ansari, Vincent Chen, Romteen Sedighi, Burhaan Syed, Zohaer Muttalib, Khadija Ansari, Fatima Ansari, Denise Nadora, Daniel Razick, Forshing Lui . Role of the UNC13 family in human diseases: A literature review. AIMS Neuroscience, 2023, 10(4): 388-400. doi: 10.3934/Neuroscience.2023029 |
[7] | Robin Quilliam, Samantha Quilliam, Morag Turnbull, Shelagh Parkinson, Godwin Oligbu . Catatonia as a presentation of autism in a child: a case report. AIMS Neuroscience, 2020, 7(3): 327-332. doi: 10.3934/Neuroscience.2020019 |
[8] | Khue Vu Nguyen . Potential molecular link between the β-amyloid precursor protein (APP) and hypoxanthine-guanine phosphoribosyltransferase (HGprt) enzyme in Lesch-Nyhan disease and cancer. AIMS Neuroscience, 2021, 8(4): 548-557. doi: 10.3934/Neuroscience.2021030 |
[9] | Graziella Orrù, Valentina Cesari, Eleonora Malloggi, Ciro Conversano, Danilo Menicucci, Alessandro Rotondo, Cristina Scarpazza, Laura Marchi, Angelo Gemignani . The effects of Transcranial Direct Current Stimulation on food craving and food intake in individuals affected by obesity and overweight: a mini review of the magnitude of the effects. AIMS Neuroscience, 2022, 9(3): 358-372. doi: 10.3934/Neuroscience.2022020 |
[10] | Mohammad Azizur Rahman, Shahdat Hossain, Noorlidah Abdullah, Norhaniza Aminudin . Brain proteomics links oxidative stress with metabolic and cellular stress response proteins in behavioural alteration of Alzheimer’s disease model rats. AIMS Neuroscience, 2019, 6(4): 299-315. doi: 10.3934/Neuroscience.2019.4.299 |
As discussed in [1], wireless sensor network based road segment surveillance is one of the key operations in military applications and with the rise of road traffic monitoring applications in civilian contexts, there is a renewed interest in this application area [2]. Traditional methods have mostly focused on full coverage, Always-Awake based techniques [3,4,5,6]. Always-awake techniques generally have very limited network lifetime because the sensors do not sleep during network operation, but they provide the smallest average detection time usually denoted by zero in literature. In order to increase network lifetime, the Duty Cycling based approaches allow all sensor nodes to start their sensing operation simultaneously for w seconds and after that the whole network goes to sleep for D seconds [7,8,9,10]. One of the earliest works which utilized scan waves for detection of intruding vehicles was proposed by Jeong et al. and is known as Virtual Scanning Algorithm (VISA) [16,17]. The work proposed in this paper is based on a modification of VISA. VISA uses the concepts of entrance points and protection points in a road network and sends waves of scan to detect vehicles as shown in Figure 1.
In Figure 1, the left end of the road segment is the entrance point where the vehicle can get into the road network and the right end of the road segment is protection point which needs to be protected from intruding vehicles. In VISA design, all sensors are waked up one by one for a certain working time w from the direction of protection point towards entrance point after a network-wide silent time. This wave of sensing activities guarantees the detection of target and allows additional sleeping time for individual sensors. Jeong et al. also argue that the virtual scan of the opposite direction i.e., from the entrance point to the protection point cannot guarantee target detection if a very fast target enters just after the start of the network-wide silent time. VISA is very suitable and appropriate for military applications where there is a concept of designated entrance points and designated protection points, but the design of VISA does not consider detection in the case of two-way roads which is the most common type of roads in civilian applications. This paper proposes a dual sensing scheduling algorithm. The basic idea of dual sensing scheduling algorithm is to ensure detection of vehicles on two-way roads by using two scan-waves in opposite directions initiating from the midpoint sensor of a road segment. In simplest terms, dual sensing scheduling can be thought of as two parallel VISA scan waves but in opposite directions with midpoint sensor as the starting point of both of the scans or in terms of VISA terminology, midpoint sensor can be thought of as a protection point for both of the scans. This is illustrated in Figures 2 and 3 for the same road segment of length d which is shown in two sub-segments of length d/2 each. This type of parallel dual sensing puts quite a stringent demand on network lifetime but greatly reduces the average detection time.
The main contributions of the work presented in this paper are as follows:
● This work proposes DSSA scheme which extends VISA scheme so that sensing can be performed for two-way roads.
● This work provides analytical and simulation based results to compare network lifetime and average detection time of DSSA with VISA, duty cycling, and always awake schemes.
● The proposed hole handling scheme uses labeling to detect sensing hole locations and updates the schedule to guarantee sufficient sensing with remaining sensors.
● The hole handling scheme allows identification of simple as well as compound holes.
The rest of this paper is organized as follows: Section 2 briefly discusses related work followed by problem formulation in Section 3. In Section 4, comparison of analytical network lifetime and average detection time is described, followed by discussion of a simple hole handling algorithm in Section 5, which is followed by performance comparison in Section 6 and a discussion in Section 7. The paper is concluded in Section 8.
WSN based surveillance algorithms for infrastructure monitoring have mostly paid attention to full-coverage in two-dimensional open spaces [3,4,5,6]. In [4], Cardei et al. proposed a method to extend the sensor network life time by organizing the sensors into a maximal number of set covers that are activated successively. Only the sensors from the current active set are responsible for monitoring all targets and for transmitting the collected data, while all other nodes are in a low-energy sleep mode. They modeled the solution as the maximum set covers problem and designed two heuristics that efficiently compute the sets, using linear programming and greedy approach to monitor a set of static targets at known locations. In [11], Rabbat and Nowak presented an approach to source localization and tracking using received signal strength measurements. Based on incremental gradient descent-like optimization methods, their algorithm required small amounts of data to be communicated over short distances. In [12], Chen et al. proposed a fully decentralized, light-weight, dynamic clustering algorithm for target tracking. Instead of assuming the same role for all the sensors, they envisioned a hierarchical sensor network that is composed of two main items: a static backbone of sparsely placed high-capability sensors which will assume the role of a cluster head upon triggered by certain signal events; and low-end sensors whose function is to provide sensor information to cluster heads upon request. A cluster is formed, and a cluster head becomes active, when the acoustic signal strength detected by the cluster head exceeds a pre-determined threshold. The active cluster head then broadcasts an information solicitation packet, asking sensors in its vicinity to join the cluster and provide their sensing information. In [13], Yao et al. presented a localization algorithm based on the observation that signals from different nodes arrive at the target at different times. In [14], Gui et al. proposed a patrolling surveillance algorithm that allows a virtual patrol to move along a predefined path waking up sensors adjacent to the patrol's path according to a schedule in order to track the target. In [15], He et al. proposed a target detection system that allows a group of cooperating sensor devices to detect and track the positions of moving vehicles in an energy-efficient and stealthy manner. They traded off energy-awareness and surveillance performance by adaptively adjusting the sensitivity of the system. In [16,17], Jeong et al. noted that as compared to two-dimensional open space surveillance, the road network surveillance is different due to two reasons:
● the first reason is that the movement of target vehicles is confined within road segments,
● the second reason is that the location maps for the road network are normally known in advance which facilitates the location identification. In case if such a location map is not present, then a prior localization step is needed to find the locations of all road side sensors. The localization methods presented in [20] can be readily employed for this purpose.
Jeong et al. proposed Virtual Scanning Algorithm (VISA) which has already been discussed in the previous section. Jeong et al. also proposed a hole-handling mechanism within the same work. In [2], Chen et al. proposed a twice deployment node balance (TDNB) algorithm which provides better performance than VISA in terms of network lifetime by dividing the deployment of the sensor nodes into two phases instead of deploying all the sensor nodes at one time.
As mentioned in [1], wireless sensor networks are comprised of thousands of nodes with limited energy budget. As time passes, these sensor nodes start draining their energy and become dead. In a highly dense network deployment, a large number of such dead nodes in a polygonal shape area are considered as a communication void or a sensing hole. These communication voids are extremely dangerous for greedy geographical routing algorithms as the next hop forwarding process can get stuck at the boundaries of sensing holes. Identification of these holes is crucial for many services in a sensor network. In [18], Fang et al. discuss two hole identification algorithms based on the concept of Delaunay Triangulation. Another very interesting work related to hole detection/identification is reported in [19] where Funke et al. have discussed the use of connectivity and hop-distance information extracted from communication/connectivity graphs of the sensor nodes. This information is exploited in order to reveal the boundaries of holes. In contrast, [17] discusses hole handling approach called MST-based labeling which handles the case of sensing holes after the holes have already been identified either using hole detection algorithms or by analyzing the reported status information collected at a central scheduler node. MST-based labeling algorithm uses concepts of hole labeling, clustering, and minimum spanning trees (MST) to label and assign each endpoint set of a sensing hole to either a protection point or entrance point cluster based on its proximity.
The hole handling algorithm proposed in this paper uses simple concept of labeling the nodes using a special numbering scheme and based on their reported status, carry out a modification in the sleeping and working schedule of the remaining active sensor nodes in every road segment. The simplicity of the proposed algorithm makes it straightforward for every road segment to modify the sensing/sleeping schedule.
Following are separate statements for problem formulation of both problems although in simulation both the techniques are being studied together.
The problem is to ensure that all intruding targets from both sides of the road on a two-way road segment are detected before they reach midpoint of the road segment keeping in mind to achieve a suitable lifetime for the sensor network.
The goal of hole handling problem is to mark the boundaries of each communication void (also referred to as a sensing hole) created in the road segment by either absence of placement at startup or draining of energy budget of sensor nodes and to update the sleeping/working schedule accordingly while keeping the optimization of network lifetime in consideration.
The next section describes the dual sensing scheduling algorithm along with analytical performance comparison with other techniques.
The assumption is that n sensors are placed on a road segment of length d. Each sensor has a sensing radius of r which is sufficient to scan the width of the road. Let w be the minimum working time needed by a sensor in order that the sensor can reliably detect a target. Let v be the maximum target speed. The targets can enter from both sides of the road segment. The traditional full coverage algorithms where sensors remain turned on all the time are called Always-Awake. A better design can be built based on the observation that it takes at least d/v seconds for a target to pass a road segment of length d at a maximum speed v. Therefore, all sensors in the road segment can sleep together for d/v seconds, which is defined as the silent time of the road network. After this silent time, all nodes wake up simultaneously for detection. This technique is called Duty Cycling. The VISA technique is shown in Figure 1. After all sensors sleep for d/v seconds, sensors are waked up one by one for working time w from the rightmost sensor Sn toward the leftmost sensor S1. This scan-wave of sensing activities guarantees the detection [16,17] as shown in Figure 1. The dual sensing scheduling algorithm as shown in Figure 2 and Figure 3 initiates two parallel scan-waves from the midpoint of the road segment d approximately designated by sensor position Sn/2 after all the sensors on the road segment have slept for a time d/2v. The objective of dual sensing algorithm is to ensure detection of vehicles entering from both sides of the road segment. This feature of dual sensing algorithm provides detection of target vehicle in half of the time as compared to VISA. This is discussed in the next two subsections.
In order to compare the sensor network lifetime, the parameters used by Jeong et al. in [17] are being used as it is. These parameters are shown in Table 1. In Table 2, overall analytical results for all the four techniques are presented. Figure 4 shows the comparison of lifetime among the four techniques. The road segment considered in this figure is 390m in length with 195 sensors deployed on the road side for detecting vehicles coming from both directions. The assumed vehicle speed is 64km/hr(40miles/hr). For example, for w=1s, VISA has a lifetime of 30.3713hr, Dual Sensing 15.1856hr, Duty Cycling 3.2113hr and Always-Awake 0.14hr which is equal to total lifetime of one roadside sensor i.e., Dlife.
Parameter | Definition |
Dlife | Lifetime that a sensor can work continuously corresponding to its energy budget |
Dnet | Sensor network lifetime |
Dwork | Working time that a sensor needs to work for reliable detection. Normally Dwork=w |
Dsleep | Sleeping time of each sensor |
Dscan | Scan time that a virtual scan wave moves along the road segment. |
Dscan=nw for VISA and Dscan=n2w for DSSA | |
Dsilent | Silent time that the whole sensor network remains silent; that is, time that a |
target passes through the road segment of length d. | |
Dsilent=dv for VISA and Dsilent=d2v for DSSA | |
Dperiod | Schedule period of the sensor network. Dperiod=Dscan+Dsilent |
Technique | Sleeping | Working | Network | Average |
Time (Dsleep) | Time (Dwork) | Lifetime (Dnet) | Detection Time | |
Always-Awake | 0 | Dlife | Dlife | 0 |
Duty Cycling | dv | w | (Dlifew)(w+dv) | d22v(wv+d) |
VISA | (n−1)w+dv | w | (Dlifew)(nw+dv) | d2v |
Dual Sensing | (n2−1)w+d2v | w | (Dlifew)(n2w+d2v) | d4v |
Figure 5 compares the average detection time after a target enters a road segment among the four techniques. VISA detects with a constant delay d/2v and Dual Sensing with a constant delay of d/4v regardless of the working time w as proved in Appendix A. For example, for w=1s, VISA detects target within 10.9688s, Dual Sensing does within 5.4844s, Duty Cycling does within 10.2668s and Always-Awake does without any delay. Therefore, Dual Sensing outperforms Duty Cycling as well as VISA in terms of average detection time.
Holes might be created in the wireless sensor network due to several reasons. Two of these reasons are:
● Holes due to imperfections in the sensor placement process
● Holes due to draining of energy budget of sensor nodes
The hole handling method proposed in this work is based on the concept of labeling of all the entrance points, protection points, and other road side sensors with a numbering scheme. The proposed method assumes the presence of a DSSA Scheduling Access Point (AP) with the responsibility of periodically receiving status messages from all the sensor nodes, creating an ordered status list, and then examining the ordered status list in order to detect any missing status entry/entries which pinpoint the presence of hole(s). This examination process for sensing holes will result in identification of all holes belonging to the following two types:
● Simple hole: It is created due to one malfunctioning sensor.
● Compound hole: It is created due to malfunctioning of contiguous sensors.
In order to carry out the desired hole type identification, the hole handling mechanism uses a node labeling scheme to assign a unique label to every sensor node formed of three numbers i.j.k where i and j represent the left entrance point and right entrance point of the road segment in consideration, k represents the individual sensor node, and k has the range from 1 to (n−2). The left entrance point is assigned the label i.j.0 and the right entrance point is assigned the label i.j.(n−1) where n is the number of sensor nodes in one road segment. For the first road segment shown in Figure 6, the labeling scheme is shown in Figure 7.
Using the proposed labeling scheme, the left and right boundary nodes of a simple hole are identified as shown in Figure 8. The left and right boundary nodes mark the region where the sensing coverage has been weakened and these markings act as a barrier around that hole and these markings can also be used for any further action to either rectify the problem or just to simply avoid any forwarding along those regions which have developed communication voids. This information can be extremely useful for any data forwarding algorithm also.
The left and right boundary nodes of a compound hole are identified as shown in Figure 9. The size of the compound hole can be determined by using the formula kright−boundary−node−kleft−boundary−node−1.
After identifying the size of the hole, the DSSA scheduler sends updated sleeping schedule (in accordance with the formulas shown in Table 2 for Dsleep) to the remaining functioning nodes in that particular segment so that the surveillance quality of that particular road segment is not compromised. This has the effect of reducing the sleep times of the remaining nodes and as will be observed in the simulation results, it will reduce the network lifetime also. A future extension of this work might be to remove the DSSA Scheduler altogether and compute all the sensing schedules in a distributed way through entrance and protection points in the road network.
In this section, performance results of DSSA approach are being reported after comparing with three other schemes for road network surveillance. The performance metrics chosen for study are exactly like the ones chosen for the analytical case i.e., network lifetime and average detection time. Since the most popular work in the road network surveillance for military scenarios is the VISA approach, therefore, DSSA is compared with VISA and additionally with Duty Cycling and Always-Awake approaches. In the performance comparison, the effect of the working time (Dwork) on network lifetime and average detection time has been studied. In addition, the effect of simple sensing holes on the network performance has also been studied. The simulation has been performed in C++ language. 18 different values of working times have been used to study the effects. Hole handling has only been compared with MST-based labeling method which is reported in [16] as part of VISA approach.
First of all, the simulation-based overall system trend for network lifetime was studied with a fixed energy budget of 60 kilojoules. It was found that the VISA scheduler can sustain for 27.77hr, DSSA for 13.88hr, Duty Cycling for 1.94hr, and Always-Awake for 0.1041hr. For the network lifetime comparison in all of the following subsections, the same fixed energy budget was used but for average detection time comparison, a full day energy budget was used for accurate results. A small section of an actual road network was used for two-way road endpoints, entrance, and protection points.
Figure 10 shows the comparison of network lifetime among the four techniques reported in seconds. For the analytical comparison, it was shown in hours, therefore the values on Y-axis are different from the analytical case. In general, for a fixed energy budget of 60 kilojoules, and for w=1s, DSSA provides a lifetime which is 6.25 times greater than Duty Cycling and 133.5 times greater than the Always Awake approaches.
Figure 11 shows the comparison of average detection time in seconds for the four techniques. The general observation is that almost for all the considered working times, DSSA provides an average detection time which is on average two times better (smaller) than the VISA approach.
In order to observe the effect of increasing the number of sensors within a sensor range of 2r where r is the transmission radius, number of sensors in 2r range were varied from 2 to 20 with increments of 2. The increase in the number of sensors lengthens the network lifetime for both DSSA and VISA approaches as shown in Figure 12. This is due to the fact that an increase in the number of sensor nodes in a particular region leads to shortening of scan time Dscan, permitting all the sensor nodes to sleep longer. Similarly, if the number of sensor nodes in a given sensor range 2r are decreased, it has a drastic effect on network lifetime as fewer nodes leads to smaller sleeping time which drains the sensor nodes much faster. This trend can also be observed in the same Figure 12 for case of 2 nodes. However, the high sensor density does not contribute much to the network lifetime to Duty Cycling and Always-Awake, since their sleeping time is independent of the number of sensors. It should be noted that DSSA is using two parallel scans at the same time, hence its network lifetime will always be smaller than VISA approach.
Figure 13 shows the effect of increasing number of sensors on the average detection time. Initially, when the number of sensors is less than 10, the lower number of sensors near endpoints makes the possibility of detection higher resulting in shorter detection time. But, as more and more sensors are added along the same sensing segments, the average detection time smoothes out after 10 sensors per sensing range. This is due to the failure probabilities of sensors as more sensors are deployed, they fail often, leading to depriving the average detection time of getting any considerable advantage of the presence of increased number of sensors.
Figure 14 shows the comparison of network lifetime between MST-based labeling and LHHA reported in seconds and hours. As shown in Figure 14, MST-based labeling gives longer lifetime than LHHA becuase it is using VISA scan. Although the network lifetime will be shorter in the presence of holes, the general trend remains the same with MST outperforming LHHA in terms of network lifetime.
Figure 15 shows the comparison of average detection time between MST-based labeling and LHHA reported in seconds. As shown in Figure 15, LHHA provides faster detection than MST-based labeling because it is using DSSA scan. The general trend remains the same with LHHA outperforming MST in terms of average detection time.
All the obtained simulation results are consistent with the analytical results confirming that the DSSA approach can easily be adopted for two-way roads in civilian scenarios. VISA approach of [16] continues to be the best approach for military scenarios.
A possible extension of this road network surveillance algorithm may be to look for ways in which energy harvesting techniques as well as fault-tolerance techniques can be combined with the dual sensing scheduling schemes with the outcome that instead of simply marking/labeling faulty sensors or those who have drained their energy budget, there can be either recharging or reconfiguration with spare sensors. A combination of energy harvesting/fault tolerance and scheduling will have the effect of greatly increasing total network lifetime.
Two possibilities for energy harvesting are as follows:
● Solar energy harvesting may be used which involves use of photovoltaic cell array to power sensor nodes on roadside.
● RF energy harvesting may be used by flying an unmanned aerial vehicle (UAV) to enable the wireless charging at short-range. In addition, RF wake-up is also possible at long-range.
Two possibilities for fault-tolerance are as follows:
● A fault-tolerant triple modular redundancy system with a majority voter circuit can be implemented to afford spatial redundancy to the endpoint sensors.
● A fault-avoidance reconfiguration system can be implemented which allows a non-functioning roadside sensor to be substituted by a functioning spare sensor. More than one spare can be provided for critical entry points in the road network.
This paper has presented a Dual Sensing Scheduling algorithm which is a modified version of the VISA technique. Using the concepts mainly derived from the VISA technique, the distinct feature of the proposed algorithm is that it provides target detection for two-way road segments with constant delay. Application of Dual Sensing on a larger size road network has also been studied. A hole handling method has also been proposed to label the hole boundaries and update the sleeping schedule.
The authors wish to acknowledge the support provided by the Systems & Control Group at the Department of Electrical, Computer, & Software Engineering of the University of Auckland.
The authors declare that they have no conflict of interest.
Average detection time for Dual Sensing Scheduling has been derived with the following assumptions:
● Speed of car remains same.
● Speed of approaching car is denoted by v.
● Each roadside sensor covers a segment of length dn.
● There are n sensors on the whole segment. But the segment is divided into two equal fragments each of length d2.
● Dual sensing performs scanning of road segment with a speed of dnw where w is working time.
● Relative speed between scan and car is dnw+v.
Keeping the entrance of cars while the scanning is in progress or when all the sensors are sleeping for a fixed sleeping time of d2v, two scenarios are possible:
Scenario 1: Car enters a road segment from an entrance point while a scan is in progress:
If a car enters at time tA during the scanning phase, the scan operation has already traversed a distance of dtAnw and hence it will require only the remaining time (d2−dtAnw)/(dnw+v) time to detect the car. This time after simplification will become (nwd−2dta)2(nwv+d). Integrating this expression for tA over an interval of [0,n2w] gives us the average detection time for this scenario:
E[Dscanning]=∫n2w0(nwd−2dtA)2(nwv+d)2nwdtA | (A-1) |
E[Dscanning]=nwd4(nwv+d) | (A-2) |
Scenario 2: Car enters a road segment from an entrance point while the whole segment is silent due to segment-wide sleeping time:
If a car enters at time tA after the whole network is in silence phase, the car has already traversed a time tBv because it entered at a certain time tB before even the detection was started at time (d2v). Therefore it takes (d2−tBv)/(dnw+v) amount of time before it gets detected where time tB=d2v−tA. There is also a need to add the time tB along with the aforementioned time.
Hence, average detection time for this scenario will be tB+(d2−tBv)/(dnw+v). After substituting the value of tB and simplifying, it will become (nwd2−dta+d22v)(nwv+d). Integrating this expression over an interval of [0,d2v] gives us the average detection time for this scenario:
E[Dsleeping]=∫d2v0(nwd2−dtA+d22v)(nwv+d)2vddtA | (A-3) |
E[Dsleeping]=2nwd+d2v4(nwv+d) | (A-4) |
Combining the two scenarios
The above two scenarios can be combined using the following equation to give the value of average detection time:
E[D]=n2wn2w+d2vE[Dscanning]+d2vn2w+d2vE[Dsleeping] | (A-5) |
E[D]=d4v | (A-6) |
[1] | Gorme JB, Maniquiz MC, Song P, et al. (2010) The water quality of the Pasig River in the City of Manila, Philippines: Current status, management and future recovery. Environ Eng Res 15: 173–179. |
[2] | Catchilar GC (2008) Fundamentals of Environmental Science. Mandaluyong City: National Book Store. |
[3] | Mora C, Tittensor DP, Adl S, et al. (2011) How many species are there on Earth and in the ocean? PLoS Biol 9: e1001127. |
[4] | Shiklomanov L (1993) World freshwater resources. Water in crisis: A guide to the fresh water resources. New York: Oxford University Press, 13–24. |
[5] | Gleick PH (1996) Water resources. Encyclopedia of climate and weather. New York: Oxford University Press, 2: 817–823. |
[6] | Lansing JS, Lansing P, Erazo J (1998) The value of a river. J Pol Ecol 5: 1–22. |
[7] | Jordaan JM (2009) The uses of river water and impacts. Fresh Surface Water 3: 1–10. |
[8] | Environmental Management Bureau (2014) National water quality report 2006–2013. Quezon City: Department of Environment and Natural Resources-EMB. |
[9] | World Bank (2003) Philippines – environment monitor 2003. Available from: http://documents1.worldbank.org/curated/en/144581468776089600/pdf/282970PH0Environment0monitor.pdf |
[10] | Dyer SD, Peng C, McAvoy DC, et al. (2003) The influence of untreated wastewater to aquatic communities in the Balatuin River, the Philippines. Chemosphere 52: 43–53. |
[11] | Environmental Management Bureau (2016) Annual Report for CY 2016. Quezon City: Department of Environment and Natural Resources-EMB. |
[12] | Picardal JP, Bendoy A, Calumba JR, et al. (2012) Impacts of waste disposal practices and water utilization of riverside dwellers on physico-chemical and microbiological properties of Butuanon River, Central Visayas, Philippines. CNU J High Educ Sp.: 78–100. |
[13] | Oquiñena-Paler, MKM, Ancog, R (2014) Copper, lead and zinc concentration in water, sediments and catfish (Clarias macrocephalus gunther) from Butuanon River, Metro Cebu, Philippines. IOSR J Environ Sci Toxicol Food Tech 8: 49–56. |
[14] | Lo JM, Sakamoto H. Heavy metals distribution in the surface sediments from central west coast of Cebu, Philippines. J Sediment Sco Jap 62: 31–41. |
[15] | Paringit, EC, Otadoy, RS (2017) LiDAR surveys and Flood mapping of Sapangdaku River. Quezon City: University of the Philippines Training Center for Applied Geodesy and Photogrammetry. |
[16] | American Public Health Association, American Water Works Association, & Water Environment Association (2005) Standard methods for the examination of water and wastewater (21st Edition). Washington, DC: APHA-AWWA-WEF. |
[17] | Environmental Protection Agency (2012) 5.1 Stream flow. Available from: https://archive.epa.gov/water/archive/web/html/vms51.html |
[18] | Environmental Protection Agency (2012) 5.9 Conductivity. Available from: https://archive.epa.gov/water/archive/web/html/vms59.html |
[19] | Minnesota Pollution Control Agency (2008) Turbidity: description, impact on water quality, sources, measures- A general overview. Available from: https://www.pca.state.mn.us/sites/default/files/wq-iw3-21.pdf |
[20] | National Institute of Water and Atmospheric Research (2016) Streamflow. Available from: https://niwa.co.nz/ |
[21] | Ling TY, Soo CL, Liew JJ, et al. (2017) Application of multivariate statistical analysis in evaluation of surface river water quality of a tropical river. J Chem 2017. |
[22] | Byrne P, Wood PJ, Reid I (2012) The impairment of river systems by metal mine contamination: A review including remediation options. Crit Rev Environ Sci Technol 42: 2017–2077. |
[23] | Haddaway NR, Cooke SJ, Lesser P, et al. (2019) Evidence of the impacts of metal mining and the effectiveness of mining mitigation measures on social–ecological systems in Arctic and boreal regions: a systematic map protocol. Environ Evid 8. |
[24] | Atlas Mining (2013) Safeguarding the natural balance of the environment[Internet] |
[25] | Maglangit FF, Galapate RP, Bensig, EO (2014) Physicochemical-assessment of the water quality of Buhisan River, Cebu, Philippines. Int J Res Environ Sci Tech 4: 83–87 |
[26] | Maglangit FF, Galapate RP, Bensig, EO (2014) Physicochemical-assessment of the water quality of Bulacao River, Cebu, Philippines. J Biodiv Environ Sci 5: 518–525. |
[27] | Kheira R, Boualem R (2015) Impact of quarry sand exploitation on surface water flow quality—case of El Harrach stream channel, Algeria. Desalin Water Treat 57: 21189–21200. |
[28] | Li H, Shi A, Li M, et al. (2013) Effect of pH, temperature, dissolved oxygen, and flow rate of overlying water on heavy metals release from storm sewer sediments. J Chem Article ID 434012. |
[29] | Nyanti L, Soo C, Danial-Nakhaie M, et al. (2018) Effects of water temperature and pH on total suspended solids tolerance of Malaysian native and exotic fish species. AACL Bioflux 11: 565–573. |
[30] | Regional Aquatics Monitoring Program (2018) Water quality indicators: Temperature and dissolved oxygen. Available from: http://www.ramp-alberta.org/river/water+sediment+quality/chemical/temperature+and+dissolved+oxygen.aspx#:~:text=Water%20temperature%20is%20one%20of,decreases%20as%20water%20temperature%20increases. |
[31] | State Water Resources Control Board (2004) The clean water team guidance compendium for watershed monitoring and assessment. Available from: https://www.waterboards.ca.gov/water_issues/programs/swamp/cwt_guidance.html |
[32] | Halliday, SJ, Skeffington, RA, Bowes, MJ, et al. (2008) The water quality of the River Enbourne, UK: Observation from high-frequency in a rural, lowland river system. Water 6: 150–180. |
[33] | Green JA, Pavlish JA, Merritt RG, et al. (2005) Hydraulic impacts of quarries and gravel pits. Legislative Commission on Minnesota Resources. Available from: https://files.dnr.state.mn.us/publications/waters/hdraulic-impacts-of-quarries.pdf |
[34] | Wood MS (2014) Estimating suspended sediment in rivers using acoustic Doppler meters. US Geological Survey Fact Sheet. US Geological Survey |
[35] | Simon TP (1999) Assessing the sustainability and biological integrity of water resources using fish communities. New York: CRC Press |
[36] | Armstrong DS, Parker GW, Richards TA (2004) Evaluation of streamflow requirements for habitat protection by comparison to streamflow characteristics at index streamflow-gaging stations in Southern New England. US Geological Survey |
[37] | Maine Department of Environmental Protection (2016) Dragonfly & damselfly larvae (Odonata). Available from: https://www.maine.gov/dep/water/monitoring/biomonitoring/sampling/bugs/dragonsanddamsels.html |
[38] | Mesner N, Geiger J (2005) Dissolved Oxygen. Utah State University Extension. Available from: https://extension.usu.edu/waterquality/files-ou/whats-in-your-water/do/NR_WQ_2005-16dissolvedoxygen.pdf |
[39] | Vancouver Water Resources Education Center (2020) Water quality: Temperature, pH and dissolved oxygen. Available from: https://www.cityofvancouver.us/sites/default/files/fileattachments/public_works/page/18517/water_quality_tempph_do.pdf |
[40] | Samudro G, Mangkoedihardjo S (2010) Review on BOD, COD and BOD/COD ratio: A triangle zone for toxic, biodegradable and stable levels. Int J Acad Res 2: 235–239 |
[41] | Jordão, CP, Pereira, MG, Matos, AT, et al. (2005) Influence of domestic and industrial waste discharges on water quality at Minas Gerais State, Brazil. J Braz Chem Soc 16: 241–250 |
[42] | Ogunfowokon AO, Okoh EK, Adenuga AA, et al. (2005) An assessment of the impact of point source pollution from a university sewage treatment oxidation pond on a receiving stream- A preliminary study. J App Sci 5: 36–43 |
[43] | Raisbeck MF, Riker SL, Tate CM, et al. (2008) Water Quality for Wyoming Livestock & Wildlife: A Review of the Literature pertaining to Health Effects of Inorganic Contaminants. University of Wyoming Department of Veterinary Sciences |
[44] | Rodrigues ACM, Jesus FT, Fernandes MAF, et al. (2013) Mercury toxicity to freshwater organisms: Extrapolation using species sensitivity distribution. Bull Environ Contam Toxicol, 91: 191–196 |
[45] | Paul S, Mandal A, Bhattacharjee P, et al. (2019) Evaluation of water quality and toxicity after exposure of lead nitrate in fresh water fish, major source of water pollution. Egypt J Aquat Res, 45: 345–351 |
[46] | Woody CA, O'Neal SL (2012) Effects of copper on fish and aquatic resources. The Nature Conservancy. Available from: https://www.conservationgateway.org/ConservationByGeography/NorthAmerica/UnitedStates/alaska/sw/cpa/Documents/W2013ECopperF062012.pdf |
[47] | Oram B (2020) Drinking water and other waters bacterial testing and screening. Water Research Center. Available from: https://water-research.net/index.php/water-testing/bacteria-testing/coliform-bacteria |
[48] | World Health Organization (2003) Guidelines for drinking-water quality. Geneva: WHO |
[49] | Seo M, Lee H, Kim Y (2019) Relationship between coliform bacteria and water quality factors at Weir Stations in the Nakdong River, South Korea. Water 11: 1171 |
[50] | Simeonov V, Stratis JA, Samaraetal C (2003) Assessment of the surface water quality in Northern Greece. Water Res 37: 4119–4124 |
[51] | Bilotta GS, Brazier RE (2008) Understanding the influenceof suspended solids on water quality and aquatic biota. Water Res 42: 2849–2861 |
[52] | Phung D, Huang C, Rutherford S (2015) Temporal and spatial assessment of river surface water quality using multivariate statistical techniques: A study in Can Tho City, a Mekong Deltaarea, Vietnam. Environ Monito Assess 187: 5. |
Parameter | Definition |
Dlife | Lifetime that a sensor can work continuously corresponding to its energy budget |
Dnet | Sensor network lifetime |
Dwork | Working time that a sensor needs to work for reliable detection. Normally Dwork=w |
Dsleep | Sleeping time of each sensor |
Dscan | Scan time that a virtual scan wave moves along the road segment. |
Dscan=nw for VISA and Dscan=n2w for DSSA | |
Dsilent | Silent time that the whole sensor network remains silent; that is, time that a |
target passes through the road segment of length d. | |
Dsilent=dv for VISA and Dsilent=d2v for DSSA | |
Dperiod | Schedule period of the sensor network. Dperiod=Dscan+Dsilent |
Technique | Sleeping | Working | Network | Average |
Time (Dsleep) | Time (Dwork) | Lifetime (Dnet) | Detection Time | |
Always-Awake | 0 | Dlife | Dlife | 0 |
Duty Cycling | dv | w | (Dlifew)(w+dv) | d22v(wv+d) |
VISA | (n−1)w+dv | w | (Dlifew)(nw+dv) | d2v |
Dual Sensing | (n2−1)w+d2v | w | (Dlifew)(n2w+d2v) | d4v |
Parameter | Definition |
Dlife | Lifetime that a sensor can work continuously corresponding to its energy budget |
Dnet | Sensor network lifetime |
Dwork | Working time that a sensor needs to work for reliable detection. Normally Dwork=w |
Dsleep | Sleeping time of each sensor |
Dscan | Scan time that a virtual scan wave moves along the road segment. |
Dscan=nw for VISA and Dscan=n2w for DSSA | |
Dsilent | Silent time that the whole sensor network remains silent; that is, time that a |
target passes through the road segment of length d. | |
Dsilent=dv for VISA and Dsilent=d2v for DSSA | |
Dperiod | Schedule period of the sensor network. Dperiod=Dscan+Dsilent |
Technique | Sleeping | Working | Network | Average |
Time (Dsleep) | Time (Dwork) | Lifetime (Dnet) | Detection Time | |
Always-Awake | 0 | Dlife | Dlife | 0 |
Duty Cycling | dv | w | (Dlifew)(w+dv) | d22v(wv+d) |
VISA | (n−1)w+dv | w | (Dlifew)(nw+dv) | d2v |
Dual Sensing | (n2−1)w+d2v | w | (Dlifew)(n2w+d2v) | d4v |