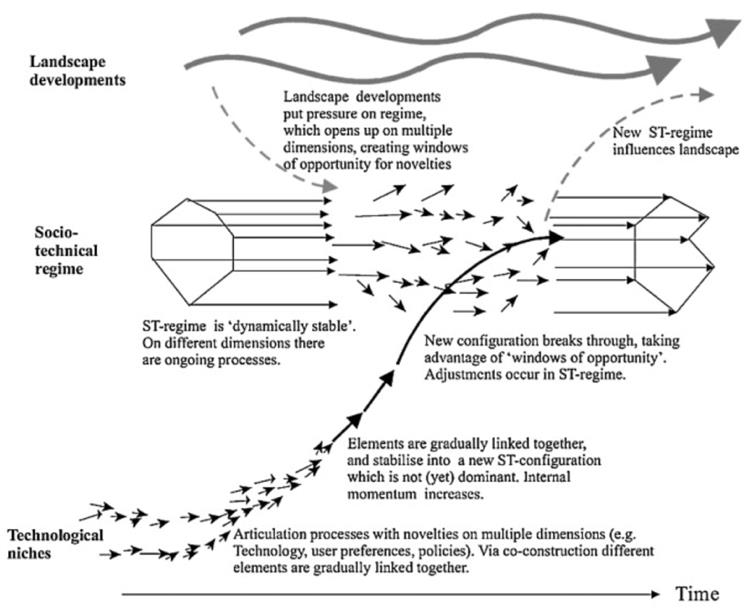
The paper investigates the socio-technical transition pathways related to innovation niches formation merging the theoretical framework of Strategic Niche Management (SNM) with the Social Network Analysis (SNA) technique. Specifically, focusing on the niche of the virtual and augmented reality, this work tries to shed light on the understanding of the niche development and the related transition towards sustainability. Combining SNM with SNA and utilizing a specific taxonomy, the study allows appreciating the actual cognitive interaction among niche members that determine the transition to succeed. Results show that the investigated niche is characterized by an embryonic stage of development which needs a better support to advance technological shift in order to reinforce the link between Industry 4.0 paradigm and Circular Economy (CE).
Citation: Patrizio Giganti, Pasquale Marcello Falcone. Socio-technical transitions and innovation niches: the case of the virtual and augmented reality in Europe[J]. AIMS Energy, 2021, 9(4): 755-774. doi: 10.3934/energy.2021035
[1] | Chukwuebuka Okafor, Christian Madu, Charles Ajaero, Juliet Ibekwe, Festus Otunomo . Situating coupled circular economy and energy transition in an emerging economy. AIMS Energy, 2021, 9(4): 651-675. doi: 10.3934/energy.2021031 |
[2] | Pasquale Marcello Falcone . Analysing stakeholders’ perspectives towards a socio-technical change: The energy transition journey in Gela Municipality. AIMS Energy, 2018, 6(4): 645-657. doi: 10.3934/energy.2018.4.645 |
[3] | Nadejda Komendantova, Masoud Yazdanpanah, Roshanak Shafiei . Studying young people’ views on deployment of renewable energy sources in Iran through the lenses of Social Cognitive Theory. AIMS Energy, 2018, 6(2): 216-228. doi: 10.3934/energy.2018.2.216 |
[4] | Chukwuebuka Okafor, Christian Madu, Charles Ajaero, Juliet Ibekwe, Happy Bebenimibo, Chinelo Nzekwe . Moving beyond fossil fuel in an oil-exporting and emerging economy: Paradigm shift. AIMS Energy, 2021, 9(2): 379-413. doi: 10.3934/energy.2021020 |
[5] | Rashmi Murali, Sangeeta Malhotra, Debajit Palit, Krishnapada Sasmal . Socio-technical assessment of solar photovoltaic systems implemented for rural electrification in selected villages of Sundarbans region of India. AIMS Energy, 2015, 3(4): 612-634. doi: 10.3934/energy.2015.4.612 |
[6] | Pasquale Marcello Falcone . Editorial to the 'Special Issue—Energy transition in a circular economy perspective' of AIMS Energy. AIMS Energy, 2022, 10(4): 582-584. doi: 10.3934/energy.2022029 |
[7] | Faris Elmanakhly, Andre DaCosta, Brittany Berry, Robert Stasko, Michael Fowler, Xiao-Yu Wu . Hydrogen economy transition plan: A case study on Ontario. AIMS Energy, 2021, 9(4): 775-811. doi: 10.3934/energy.2021036 |
[8] | Shahrouz Abolhosseini, Almas Heshmati, Masoomeh Rashidghalam . Energy security and competition over energy resources in Iran and Caucasus region. AIMS Energy, 2017, 5(2): 224-238. doi: 10.3934/energy.2017.2.224 |
[9] | R. H. Almeida, M. C. Brito . A review of technical options for solar charging stations in Asia and Africa. AIMS Energy, 2015, 3(3): 428-449. doi: 10.3934/energy.2015.3.428 |
[10] | Christopher R. Jones, Eckart Lange, Jian Kang, Aki Tsuchiya, Robert Howell, Aidan While, Richard J. Crisp, John Steel, Keelan Meade, Fei Qu, Danial Sturge, Agnes Bray . WindNet: Improving the impact assessment of wind power projects. AIMS Energy, 2014, 2(4): 461-484. doi: 10.3934/energy.2014.4.461 |
The paper investigates the socio-technical transition pathways related to innovation niches formation merging the theoretical framework of Strategic Niche Management (SNM) with the Social Network Analysis (SNA) technique. Specifically, focusing on the niche of the virtual and augmented reality, this work tries to shed light on the understanding of the niche development and the related transition towards sustainability. Combining SNM with SNA and utilizing a specific taxonomy, the study allows appreciating the actual cognitive interaction among niche members that determine the transition to succeed. Results show that the investigated niche is characterized by an embryonic stage of development which needs a better support to advance technological shift in order to reinforce the link between Industry 4.0 paradigm and Circular Economy (CE).
Socio-technical transitions are considerably complex, characterized by multi-scalar relations between actors in different domains of society [1]. Accordingly, the work of promoting them is challenging [2]. Literature on innovation studies dealing with socio-technical transitions provide relevant contributions analyzing the development of a technological niche by combining Strategic Niche Management (SNM) and Social Network Analysis (SNA).
The SNM was conceptualized to include innovation and experimentation-oriented strategies to allow the expansion of technological niches possibly able to supplant the existing regime [3]. Its perspective focuses on the key function of niches, defined as a protective space for path-breaking innovative technologies [4]. In seminal SNM contributions, based on insights from innovation studies and evolutionary economics on technological shifts, three (internal) important mechanisms were recognized for assessing the effective niche development: (ⅰ) convergence of expectations (i.e., consensus in early stages of implementation), (ⅱ) networking (i.e., collaborations among stakeholders), and (ⅲ) learning processes (i.e., diffusion of knowledge) [5].
Therefore, SNM is an analytical framework that is proposed specifically to depict the introduction and diffusion of radically new sustainable technologies through an integral combination of technological progress and system-wide social-institutional transformation [6,7] where actors play a pivotal role. Actors might present a different "identity" at each relational interface; thus, the dynamic process through which actors interact is more important than the actors, themselves, or their organizational attributes [8]. Actors can represent different types of organizations (e.g., firms, public authorities, research institutions), as well as independent persons or members of an organization [9] and are crucial to explore the antecedents which prevailed in the past and still play a vital role [10]. Through interaction, their activities and properties can be shared, exploited and/or adapted with the aim of advancing the development of an innovation system [11,12]. The enrollment of new actors with field expertise into a niche can increase credibility and resources and thereby enhance the niche evolution [13]. It is widely acknowledged that network configuration plays a key role in explaining the potential diffusion of emerging technologies [5]. Accordingly, the SNA methodology is gaining increased attention among social scientists and practitioners [14], due to its ability to investigate complex relations among actors and actors' economic and socio-political behavior [15].
In 2008 Caniëls and Romijn [16] were among the first to systematically investigate the relational network of a niche using SNA. With this study they opened up the 'black box' in literature pertaining to the structure and functioning of the actor networks to explain the determinants of the successful functioning of a new niche technology. Later on, Lopolito et al. [17] presented a methodological conceptualization of innovation niches and suggest an empirical methodology, based on a SNA, aimed at capturing and measuring the internal development status of innovation niches. In 2013, Hermans et al. [5] uses the evolutionary perspective of SNM to investigate and explain the network dynamics of a collaborative innovation network of an agricultural niche. Falcone et al. [18] analyzed networking dynamics for fostering a sustainable transition looking at the evolutionary stage of the niche. Notwithstanding this interdisciplinary academic interest in the research topic, there is a lack of exploration with respect to circular economy (CE) concept and its relevance towards sustainability transition [19,20,21,22,23]. The analysis proposed in this paper tries to shed light on the relevant role of niche actors to understand the degree of development of a technological niche, namely, the European niche of the virtual and augmented reality. To the best of our knowledge, it is the first attempt to look at the virtual and augmented reality niche development using social network analysis. Specifically, by applying such methodology, the paper analyses the state of development of the European niche of the virtual reality (VR) and augmented reality (AR), extending its purpose to the recognition of the achievement of a sustainability transition by means the integration of CE and Industry 4.0 paradigm. The former refers to a system restorative and regenerative by design, which aims to maintain products, components and materials at their highest utility and value [24]. The latter describes an industrial paradigm that sees its building block in the adoption of the following technologies: big data and analytics, autonomous robots and vehicles, additive manufacturing, simulation, virtual and augmented reality, horizontal/vertical system integration, the Internet of Things (IoT), cloud, fog, edge technologies, blockchain and cyber-security [25]. Although there is an important distance between theory and practice [26], much literature discusses these two paradigms from different perspectives [27,28]. Regarding to CE, although scholars examine challenges and opportunities [29,30,31], only few contributions treat the technological approach for its implementation, in particular in Small and Medium Enterprises [32,33]. When moving to Industry 4.0, even though different contributions assess the support offered to companies by Key Enabling Technologies representing the paradigm [26,34], only few of them show that the environmental benefit is significantly achievable [35,36]. However, advanced technologies such as VR and AR can play an important role in the adoption of CE concepts and programs by governments, companies and society as a whole [37] providing a flywheel effect for our society to catalyze the shift towards sustainability. The concept underlying these considerations is that of Sustainability Transition (STR), that represents a radical transformation toward a sustainable society as a response to persistent problems challenging the contemporary modern society [38]. Sustainability transitions are long-standing, multi-dimensional, and essential transformation processes through which traditional socio-technical systems move towards new and more sustainable approaches of consumption and production [2]. The peculiarity of this new perspective is the central role played by the sustainability dimensions (i.e., environmental, social and economic) whose guidance and governance often play a fundamental role for the transition to succeed [39,40]. The multilevel perspective (MLP) is one of the main theoretical approaches to frame this change. Essentially, in the MLP, transitions occur as a result of the interface among three different levels: landscape (i.e., overall sociotechnical setting involving both tangible and intangible values), regime (i.e., dominant practices, rules and technologies that provide stability) and niches (i.e., protective spaces) [41]. Landscape factors could exert pressure on the incumbent regime and open windows of opportunities for niches to break through and conduce to radical shifts in socio-technical regimes (see Figure 1).
The MLP emphasizes that transition arises only when some pre-conditions are present: (1) alterations at the landscape level produce a suitable pressure upon the current regime, and (2) an innovation niche has adequately developed. Therefore, the ultimate success of a new technology crucially depends on the niche readiness (i.e., sufficiently mature) [43].
In this work, by apply the empirical methodology proposed by Lopolito et al. [17], our research objective was to capture and measure the internal development status of the European niche of the VR and AR looking at three niche mechanisms: ⅰ) willingness (i.e., convergence of expectations among relevant actors); ⅱ) power (i.e., networking processes among relevant actors); and ⅲ) knowledge (i.e., learning interaction among actors). Therefore, this paper presents in section 2 a description of the niche mechanisms. In section 3, materials and methods are reported. This allows to explore the results of this investigation in section 4. In section 5, some discussions are deduced. Finally, major conclusions are presented in section 6.
Once described the composition of MPL—to reach the purpose of the research—we need to focus our attention on the mechanisms belonging to micro level of the analysis, i.e., the technological niche (Figure 2).
The Figure 2 represents the interconnection between Multi Level Perspective and the niche formation.
In the upper part—that graphically shows the MLP approach—we can see that the socio-technical landscape and the innovation niches exert pressure on the incumbent technological regime. At the bottom are present three mechanisms (willingness, power and knowledge) that lead to a socio-technical transition once reached a sufficient degree of maturity at niche level [3]. Willingness refers to the convergence of actors' expectations towards a common view about the new technology which, in turn, determines a diffused willingness to actively participate in the innovation niche. Power considers the presence of powerful actors in the network as a crucial requirement for its development. Nevertheless, their presence only will not guarantee the prompt of the niche development, because it also depends on the distribution of power within the network of local actors [44]. Knowledge refers to the acquisition of an adequate amount of knowledge among the members of the network [45,46]. These three mechanisms are interrelated and mutually reinforcing, thus there is not always a clear causal relation among them [47]. The advancement along this logic allows us to categorize four stages of niche development, using a scientific taxonomy [17] as shown in Table 1.
Stage 1 | Stage 2 | Stage 3 | Stage 4 | |
Willingness | - | √ | √ | √ |
Power | - | - | √ | √ |
Knowledge | - | - | - | √ |
Niche Status | Absence | Embryonic | Proto-niche | Full |
In the first stage the three mechanisms are absent and the niche does not exist at all. In the second one the actors' expectations converge and we talk of "embryonic niche". In the third stage we add the presence of powerful subjects and the niche is called "proto-niche". In the fourth one the members of the network reach an adequate amount of knowledge too and the niche achieves the "full" status.
These three mechanisms represent also the meeting point between the Strategic Niche Management and the Social Network Analysis. SNM captures and measures the internal development status of the innovation niche. It represents a theoretical framework designed to facilitate the introduction and diffusion of radical new technologies, according to which intensive networking among social actors constitutes a crucial process for their incubation. SNA studies the network structure helping to explain the spread of emerging technologies [48]. It allows the generation of in-depth insights into the composition of the network and its effects on the innovation performance, being the success of the latter related to the structure and functioning of such network [16].
The application of the Social Network Analysis to the Strategic Niche Management allows us to draw attention to the cognitive interaction among niche members that determine the willingness and the knowledge, as well as the resource interdependence that is at the basis of the power. Among the indicators functional to measure the willingness can be considered the mean of the values attached by each actor to the niche option and the proportion of actors assigning a value equal to or greater than a recognized threshold. The analysis of the distribution of the power within the niche can be instead realized by means of the in-degree network centralization index [49], accrediting to a single actor a central and powerful position in the network when the index shows an high value.
It is calculated with the following formula:
Cin−D=∑ni=1[C∗in−D−Cin−Di](n−1)2 | (1) |
where Cin-Di is the sum of received ties by actor i; Cin-D* is the largest sum of received ties observed, and n is the number of niche members.
Finally, the presence of adequate knowledge flows in the network is detected by measuring the density of knowledge flows Dk—an effective and well-known measure of knowledge flows within a system [50]—that represents the communication relations with a knowledge content existing among any pair of actors.
Denoting these with Lk, the density of knowledge relations can be calculated as follows:
Dk=Lkn(n−1)/2 | (2) |
where the denominator represents the maximum possible number of ties, depending on the number of actors n. The maximum attainable value by this measure is 1, and this happens when the network—fully saturated—shows as already existent all the possible knowledge relations. Instead, the minimum value that indicates the absence of an interactive learning process is 0.
Yet, the internal knowledge dynamics can also be affected by structural features like the presence of cliques and small world properties [51].
VR and AR are technologies that could be conceptualized on a spectrum spanning reality to virtuality.
There is no established and widely accepted definition of them due to the rapid developments occurring in the VR/AR technology landscape. VR is a computer-generated scenario that simulates a real-world experience [52], it is a technology that allows people to experience a virtual environment [53]. Instead, AR combines real-world experience with computer-generated content [54], it allows virtual imagery information to be overlaid onto a live direct or indirect real-world environment. Both involve the interaction with virtual objects in real time, but while the first permits a full immersion—in which the user is submerged into a full 3D experience where the physical objects are linked to the virtual world [55]—the second presents the user with virtual overlays on a real environment. However, rather than be considered as two distinct technology outcomes, VR and AR should be evaluated as part of a spectrum of outcomes that span from reality to virtuality.
The data utilized in this research for the application of SNM and SNA are extrapolated from the report "Virtual reality and its potential for Europe", a study that provides a snapshot of this industry in Europe during the period 2016/2017 [56]. The total production value of the VR/AR European industry was expected to increase between €15 billion and €34 billion by 2020, showing as frontrunner countries France, the UK, Germany, the Netherlands, Sweden, Spain and Switzerland, followed by Finland, Denmark, Italy, Greece, Poland, Estonia and the Czech Republic [56].
The analyzed actors were identified considering the following map (Figure 3) and deemed as exhaustive for the representativeness of the VR and AR European ecosystem.
The network is represented by 121 actors divided in 8 categories, most of these containing subcategories too. The category of VR ecosystem support is divided in 5 Associations and 11 Support institutions. The one of Awareness and community building in 9 Events and 3 Blogs. Then we have 14 VR research centers, 39 VR companies representing the supply and 20 representing the demand. Policy makers contain 1 European and 3 Local actors. Funding actors are 5 Private and 5 Public. Finally, the Services are split in 3 Consulting & training and 3 Rental companies.
Gathering for countries of origin and starting from the most representative ones, data present: France, with 35 actors; the United Kingdom, 28; the Netherlands, 15; Germany, 12; Sweden, 8; Italy, 3; the European Union itself, 3; Greece, Scotland, Switzerland and Estonia, 2; Belgium, Finland, Hungary, Ireland, Poland and Spain, 1. In the network are present two foreigner countries too: California and Taiwan, respectively with 2 and 1 actors.
Once the network has been defined, for the application of SNA was necessary to investigate the three mechanisms through a questionnaire-based exercise. First a pilot questionnaire was administrated to a subnetwork of actors to retrieve some feedbacks. The selection of such subnetwork was based upon a hypothetical consideration of a representative actor for each category. Thus, eight actors were primarily interviewed by telephone. Building on this exercise a more robust set of questions have been prepared and successively circulated by email to the all sample over the time period January to April 2021. After two reminders we were able to obtain the full list of actors taking part to the questionnaire. The goal was the exploration of the local expectations, the existence of networking activities with powerful actors, and the presence of exchange of knowledge. The questions were the following:
Q. Willingness
A project for the development and use of supports of virtual reality and augmented reality is going to be planned in the European Union. The plan envisages experiments in the realization of applications, the establishment of facilities for their production and tax exemptions for some categories of users. Would you like to join the project?
(1 = very low, 2 = low, 3 = medium, 4 = high, 5 = very high)
Q. Power
In your opinion, in order to make this project successful, the participation of which actors is important?
(1 = because of its capability to support the VR and AR ecosystem empowerment, 2 = because of its awareness and community building, 3 = because of its research applied experience, 4 = because of its capability to commercially apply the VR and AR technology, 5 = because of its capability to politically coordinate the development, 6 = because of its capability to finance projects, 7 = because of its capacity to offer traversal services)
Q. Knowledge
Could you mark, among the following actors, those with whom you have/had knowledge exchange on virtual and augmented reality?
(1 = received, 2 = exchanged, 3 = transmitted)
In Table 2 are summarized research procedures and measurements adopted, taking into consideration their potentials and limits.
Willingness | Power | Knowledge | Potentials | Limits | |
Procedure 1 | Analysis of categories and subcategories of the entire network | Analysis of categories and subcategories of the entire network | Analysis of categories and subcategories of the entire network | Procedure useful in the next steps without considering the structure of the countries. | Structural characteristics not considered could present relevant information. |
Procedure 2 | - | Analysis of the composition of each cluster representing a single country considering the possible internal and external interactions. | Analysis of the composition of each cluster representing a single country considering the possible internal and external interactions. | Possibility to discover potential new connections among actors. | No rule able to describe the method. |
Procedure 3 | - | - | Comparison with power results. | Change to justify previous results. | No rule able to describe the method. |
Measurements | Individuation of the mean of the values attached by single actors and consideration of the proportion of actors assigning a value equal to or greater than a recognized threshold | Cin−D=∑ni=1[C∗in−D−Cin−Di](n−1)2 | Dk=Lkn(n−1)/2 Detection of cliques and small world properties |
Making tangible data collected, understanding the status of the niche and elaboration of solutions to reach the full status. | Exclusive focus on sought parameters. |
Willingness represents an important step in moving towards the initial uncertainty and distance from the adoption of the new technology that can affect the way goals are defined and arranged. The challenge related to this process of convergence is to analyze how contrasting visions and expectations enroll actors into coalitions of support, come to define their interests, and shape the way that they seek to respond to selection pressures [39]. The system used in this paper for the measurement of willingness was the simple individuation of the mean of the values attached by each actor to the niche option and the consideration of the proportion of actors attaching a value equal to or greater than a specific threshold [17].
As we can see in Table 3, 56 (46%) out of 121 expressed a medium value of willingness, 45 (37%) a high one and 20 (17%) a very high value. No one showed a low or very low interest. The mean value expressed is the medium, that represents the threshold too. Having all the other answers (in total 54%) with a higher value, we can say that the measurements reveal that the network has developed a certain convergence of expectation towards these technologies.
Willingness | ||||||
Value | 1 | 2 | 3 | 4 | 5 | Tot |
Nodes/Arcs | 0 | 0 | 56 | 45 | 20 | 121 |
In order to achieve a sufficient level of power, the second mechanism of niche formation, it is necessary the development of a real networking process among actors. The presence of powerful players within the niche—considered as a small network of dedicated actors—becomes a fundamental requirement for its growth. However, the existence of powerful actors only does not allow a niche to spur. Power is both an individual and a network feature.
Using the in-degree network centrality we were able to measure it, obtaining a value of 0.057.
Cin−D=∑ni=1[C∗in−D−Cin−Di](n−1)2; | (3) |
Cin−D=830(121−1)2=0.057 | (4) |
Just for recalling what we said above, here we sum ∑ the differences between the largest value of received ties observed Cin-D* (in our case 8) and all the single actors' ties received Cin-Di (from 1 to 121). The result is then divided for the difference between the number of the members of the network n and 1, all squared.
The larger is the indicator, the more likely is that a single actor would be quite central, and thus powerful, with the remaining actors less central. In our case the value is low, also if the network shows three nodes receiving in proportion more connections than the other.
Let's see now the graphical representation of the network realized through the software SocNetV (Figure 4).
The network in figure shows in red the connections outside the countries and in black the arcs among the members of the same nation. As we can see, there is convergence in the choice of the three actors of the EU as point of reference, that are the same that own the largest value of received ties observed Cin-D*.
The Q. Power index allowed us to understand the importance of the actors in the network and their motivation.
Looking at Figure 4 and Table 4 is possible to realize that the motivation is related to the category to which each actor belongs. In order of preference, 33 actors are chosen for the capability to commercially apply the VR and AR technology, 28 for the capacity to finance projects, 23 for the ability of awareness and community building, 22 actors for the competence to support the VR ecosystem empowerment, 11 for the potential to politically coordinate the development, 8 for the matured research applied experience and 5 for the possibility to offer traversal services.
Power | ||||||||
Value | 1 | 2 | 3 | 4 | 5 | 6 | 7 | Tot |
Arcs/Connections | 22 | 23 | 8 | 33 | 11 | 28 | 5 | 130 |
It is quite interesting the fact that the three actors mentioned above—those that own the largest value of received ties observed—don't belong to the category of those actors that are chosen for the capability to commercially apply the VR and AR technology.
The next key mechanism for innovation niche development is the acquisition of an adequate amount of knowledge that depends on the complex character of socio-technical systems transitions, where the knowledge of a large group of experts needs to be integrated in order to consider all relevant expertise and interests. As tacit and un-codified knowledge, the learning process within the niche, can only be acquired by means of in-depth and direct interactions that are influenced by the architecture of the network in which actors operate. The last mechanism was measured by means of the density of knowledge flows that assumes the value of 0.018. It shows that only around 1% of all possible learning patters are actually active, showing a rather poor performance related to the third mechanism of niche creation.
Dk=Lkn(n−1)/2; | (5) |
Dk=133121(121−1)/2=0.018 | (6) |
At the numerator we found the communication relations with a knowledge content existing among any pair of actors Lk, and at the denominator the number of actors n, multiplied by the number of actors minus 1, all divided by 2.
As for the network of the power, it follows the graphical representation (Figure 5) of the network of the knowledge through the software SocNetV.
The composition of the knowledge's network is quite similar to the Power one (all the same actors participate to the sharing of knowledge). Also, the figure shows in red the connections that come from the outside of the countries and in black arcs among the members of the same nation. The actors that share more knowledge among the network are again the EU ones.
Following, the overall results of the questionnaires.
Looking at Table 5 it is possible to see that 84 actors receive, 2 share and 47 transmit knowledge to the other. As mentioned above, despite the measurement of the density of knowledge flows is an effective method for the appraising of the knowledge inside the network, it is fair to acknowledge that the internal knowledge dynamics can also be affected by structural features like the presence of cliques and small world properties [51].
Knowledge | ||||
Value | 1 | 2 | 3 | Tot |
Arcs/Connections | 84 | 2 | 47 | 133 |
A network is commonly considered a small world when the average distance among two any nodes grows as the logarithm of the number of nodes log(N), [57]. "Grows as" meaning the values of distances close to log(N), and at the same time the clustering coefficient remains high.
The average shortest path length—represented by the sum of pair-wise distances divided by the number of existing shortest paths—in the network is equal to 1.579. Instead, the logarithm of 121 is 4.79.
Let's see now the Clustering Coefficient, that quantifies how close each node and its neighbors are to be a complete clique, a sub-graph. Its value ranges from 0 and 1, which indicates that the node is involved in many transitive relations. In the examined network the local Clustering Coefficient is equal to 0 and the Group Clustering Coefficient too. This means that there are no cliques. Therefore, also through this structural analysis, we can assume that the network does not show adequate knowledge flows, not presenting features like cliques and small world properties.
The results obtained show that the 46% of the actors show a medium willingness (the threshold) to join the project for the development of the VR and AR technologies, as well as the remaining 54% express the values "high" and "very high". The level of willingness needed for the formation of the niche has been therefore reached.
Regarding to power, the analysis shows an in-degree network centrality of 0.057, a very low result due to the absence of very powerful actors in the network (also if those belonging to EU received more connections than the others). Thus, the needed level for the formation of the niche has not been reached.
Finally, the measured density of knowledge flows of 0.018 and the absence of structural features (cliques and small world properties) indicate that the learning process, needed for the sharing of common experience and so for reaching the full niche level, is still low.
Looking at the niche development status, as showed in Table 6, we can conclude that the VR and AR niche in the European contest is still in an embryonic stage. It exists a certain interest towards the new technology, but a more extensive networking with powerful actors and an intensive process of knowledge sharing is needed in order for a full niche to occur.
Stage 1 | Stage 2 | Stage 3 | Stage 4 | |
Willingness | - | 46% medium, 54% higher | √ | √ |
Power | - | 0.057 | √ | √ |
Knowledge | - | 0.018 no cliques and small world properties | - | √ |
Niche Status | Absence | Embryonic | Proto-niche | Full |
However, after years of development and research, is possible to state that VR and AR technology has achieved a turning point for large scale adoption going beyond its early exploratory stage. As illustrated in the Gartner hype cycle below—having passed the peak of expectations—virtual and augmented reality are entering in a period of acceleration and growth, where a significant part of the population is expected to adopt the VR in about 5 years and in 10 the AR [56].
VR is currently ahead of AR when it comes to realistic expectations and real potential of use in business area, as well as in the consumer one. Its industry is expected to growth in the years to come, considering that over 50% of the companies observed in the research indicated to have expanded their VR teams, that the number of new related start-ups has increased, and that an increasing number of consumers are actively requesting VR solutions. Considering these results with a view to CE—as already said—advanced technologies such as VR and AR can play an important role, despite has not been deeply analyzed yet the way in which such technologies favor the transition [58,59]. However, in the last years, several contributions tried to support the thesis. In 2014 Baines and Lightfoot [60] discussed about how Industry 4.0 technologies—radically reshaping the way companies deliver existing services [61]—allow the introduction of sterilized business models facilitating the transition towards CE [62,63]. De Sousa Jabbour et al. [64] emphasized how Industry 4.0 and CE have motivated the business organizations to move beyond the linear supply chain and offered a new outlook of production and consumption. Ávila-Gutiérrez et al., [65] considering a standard framework for sustainability from CE 4.0, underlined the synergistic effects between the implementation of sustainability through the CE paradigm and the approach of Industry 4.0, showing a greater scope and efficiency of the frameworks, techniques and tools, together with multilevel and multiscale Triple Bottom Line integration.
In relation of such considerations concerning the importance role of VR and AR in the adoption of CE concepts and programs, let's see how the methodology can help the niche in reaching the full status of development.
The key actor for encouraging the growth mechanism of the niche is the European Commission (node 102), an actor that in the last years: supported European researchers and entrepreneurs in the scaling up of the ICT innovation system through the EU Research and innovation program (Horizon 2020) with a found of €80 billion available from 2014 to 2020; assisted small and medium enterprises with another found of €2.8 billion; introduced—in cooperation with the European Investment Found (EIF)—the Pan-European Venture Capital Founds-of-Founds to boost the levels of investment in new generations of highly innovative European firms; aided the progress in internet access and connectivity through the Digital Single Market Strategy; and urged Europe's cultural and creative sectors to catch the opportunities of digital age with the Creative Europe program [56].
Focusing our attention on power, if the European Commission reaches 62 connections (1 unit and half more than the half of the total number of actors), Cin-D* will be equal to 62 and we will obtain an in-degree network centrality equal to 0.507; the first considerable value for the needed level of power. (Here is suggested the reading of the Spread sheet N.2).
Cin−D=∑ni=1[C∗in−D−Cin−Di](n−1)2; | (7) |
Cin−D=7309(121−1)2=0.507 | (8) |
Let's see now the graphical representation in Figure 7.
The graph shows the physical structure of the network that reached a sufficient level of power. For achieving this result, 62 actors should indicate node 102 as important actor to participate to the project.
Once again, we remember that the choice of an actor in Q. Power is related to the category to which it belongs, and in this case does not exist a better actor than a political one to encourage the growth mechanism of this niche. Let's see now if with the same addition of connections, we will be able to reach the needed level of knowledge too.
To replicate the simulation, 53 actors (62-9, already pointed by 102) should answer to Q. Knowledge declaring to receive information from the European Commission. (Be aware, if they claimed to "share" information the added arcs would be double, undirected).
Coming back to the first calculation, we add this value to the previous Lk and divide for the denominator. The density of knowledge flows assumes value of 0.025, still far from a valid level for a full niche to occur.
Dk=Lkn(n−1)/2; | (9) |
Dk=186121(121−1)/2=0.025 | (10) |
As said above, the internal knowledge dynamics can also be affected by structural features like the presence of cliques and small world properties [51].
Aware that the logarithm of the number of nodes remains equal to 4.79, we need to compare it with the new average shortest path length and calculate the Clustering Coefficient considering the presence of cliques.
The average shortest path length of our network is 1.460 (very far from the value of the logarithm of the number of nodes), the local Clustering Coefficient is equal to 0 and there are no cliques in the network. Thus, it does not achieve the needed level of knowledge for an innovative niche to occur.
Nonetheless—coming back to the formula—we can use it to know the value of Lk that would allow Dk to reach the level needed. We do that replacing Lk with X and multiplying the denominator for 0.51. Solving the calculation, we will obtain an X equal to 3703, that represent the communication relations with a knowledge content existing among any pair of actors needed.
Dk=Lkn(n−1)/2; | (11) |
Dk=X7260=0.51 | (12) |
X=7260⋅0.51=3703 |
The achievement of 3703 transmissions of information inside the network—together with the level of power already implemented—will allow the niche of virtual and augmented reality to reach the status of full niche.
The proposed study tries to shed light on an empirical methodology relevant for the understanding of the development of a technological niche. Such methodology—that sees the application of SNM together with SNA—is capable of interacting with the structural characteristics of the network to achieve a complete socio-technical transition. Its employment in the study of the European ecosystem of the virtual and augmented reality shows as it is still in an embryonic stage, presenting a poor presence of powerful actors and a weak amount of knowledge among the members of the network. Although its application showed the potential for the understanding of the development of such technological niche, the research procedures and measurements used revealed limits due to the utilization of data not directly obtained from the actors considered, but extrapolated from available information and from the structure of the niche itself. However, the study of such ecosystem does not end in itself but tries to extend its contribution to the recognition of the achievement of sustainability transition by means the integration of Industry 4.0 paradigm and CE. This, in view of the literature examined that underlines the need for more researches aimed at highlighting the way in which technologies belonging to Industry 4.0 can actually play an important role in the transition to CE.
Moving away from the methodological and transitional perspective, assuming a pragmatic point of view, what emerges from the analysis is that the European VR and AR industry still needs to face the lack of: risk funding, a pro-risk and experimentation mentality, cooperation among European countries, links between market and research, and an adequate infrastructure and resources. To stimulate the growth of the niche, policy makers should: re-address funding schemes for start-ups [66], cut the application processes to obtain funding, focus on supporting incubators and accelerators, fund more applied research and demonstrators, support educational and training programs, train entrepreneurial skills, and support networking and help showcase success stories across Europe.
The authors declare no conflict of interest.
[1] |
Essletzbichler J (2012) Renewable energy technology and path creation: A multi-scalar approach to energy transition in the UK. Eur Plan Stud 20: 791-816. doi: 10.1080/09654313.2012.667926
![]() |
[2] |
Markard J, Raven R, Truffer B (2012) Sustainability transitions: An emerging field of research and its prospects. Res Policy 41: 955-967. doi: 10.1016/j.respol.2012.02.013
![]() |
[3] |
Kemp R, Schot J, Hoogma R (1998) Regime shifts to sustainability through processes of niche formation: The approach of strategic niche management. Technol Anal Strategic Manage 10: 175-198. doi: 10.1080/09537329808524310
![]() |
[4] |
Schot J, Hoogma R, Elzen B (1994) Strategies for shifting technological systems: The case of the automobile system. Futures 26: 1060-1076. doi: 10.1016/0016-3287(94)90073-6
![]() |
[5] |
Hermans F, Van Apeldoorn D, Stuiver M, et al. (2013) Niches and networks: Explaining network evolution through niche formation processes. Res Policy 42: 613-623. doi: 10.1016/j.respol.2012.10.004
![]() |
[6] |
Kemp R, Loorbach D, Rotmans J (2007) Transition management as a model for managing processes of co-evolution towards sustainable development. Int J Sustainable Dev World Ecol 14: 78-91. doi: 10.1080/13504500709469709
![]() |
[7] | Elzen B, Geels FW, Green K (2004) System innovation and the transition to sustainability: theory, evidence and policy. Edward Elgar Publishing, ISBN 1845423429. |
[8] |
Arranz N, Arroyabe MF, Schumann M (2020) The role of NPOs and international actors in the national innovation system: A network-based approach. Technol Forecast Soc Change 159: 120183. doi: 10.1016/j.techfore.2020.120183
![]() |
[9] | Falcone PM, D'Alisa G, Germani AR, et al. (2020) When all seemed lost. A social network analysis of the waste-related environmental movement in Campania, Italy. Polit Geogr, 77. |
[10] | Upadhyay A (2020) Antecedents of green supply chain practices in developing economies. Manage Environ Qual An Int J. |
[11] |
Musiolik J, Markard J, Hekkert M (2012) Networks and network resources in technological innovation systems: Towards a conceptual framework for system building. Technol Forecast Soc Change 79: 1032-1048. doi: 10.1016/j.techfore.2012.01.003
![]() |
[12] |
Morone P, Falcone PM, Tartiu VE (2019) Food waste valorisation: Assessing the effectiveness of collaborative research networks through the lenses of a COST action. J Clean Prod 238: 117868. doi: 10.1016/j.jclepro.2019.117868
![]() |
[13] |
Magnusson T, Berggren C (2018) Competing innovation systems and the need for redeployment in sustainability transitions. Technol Forecast Soc Change 126: 217-230. doi: 10.1016/j.techfore.2017.08.014
![]() |
[14] |
Leon RD, Rodríguez-Rodríguez R, Gómez-Gasquet P, et al. (2017) Social network analysis: A tool for evaluating and predicting future knowledge flows from an insurance organization. Technol Forecast Soc Change 114: 103-118. doi: 10.1016/j.techfore.2016.07.032
![]() |
[15] |
Nugroho Y, Saritas O (2009) Incorporating network perspectives in foresight: A methodological proposal. Foresight 11: 21-41. doi: 10.1108/14636680911004948
![]() |
[16] |
Caniëls MCJ, Romijn HA (2008) Actor networks in strategic niche management: insights from social network theory. Futures 40: 613-629. doi: 10.1016/j.futures.2007.12.005
![]() |
[17] |
Lopolito A, Morone P, Sisto R (2011) Innovation niches and socio-technical transition: A case study of bio-refinery production. Futures 43: 27-38. doi: 10.1016/j.futures.2010.03.002
![]() |
[18] |
Falcone PM, Lopolito A, Sica E (2018) The networking dynamics of the Italian biofuel industry in time of crisis: Finding an effective instrument mix for fostering a sustainable energy transition. Energy Policy 112: 334-348. doi: 10.1016/j.enpol.2017.10.036
![]() |
[19] |
Upadhyay A, Laing T, Kumar V, et al. (2021) Exploring barriers and drivers to the implementation of circular economy practices in the mining industry. Resour Policy 72: 102037. doi: 10.1016/j.resourpol.2021.102037
![]() |
[20] | Upadhyay A, Akter S, Adams L, et al. (2019) Investigating "circular business models" in the manufacturing and service sectors. J Manuf Technol Manage, 30. |
[21] |
Upadhyay A, Mukhuty S, Kumar V, et al. (2021) Blockchain technology and the circular economy: Implications for sustainability and social responsibility. J Clean Prod 293: 126130. doi: 10.1016/j.jclepro.2021.126130
![]() |
[22] | Upadhyay A, Kumar A, Akter S (2021) An analysis of UK retailers' initiatives towards circular economy transition and policy-driven directions. Clean Technol Environ Policy, 1-9. |
[23] |
Falcone PM (2019) Tourism-based circular economy in Salento (South Italy): A SWOT-ANP analysis. Soc Sci 8: 216. doi: 10.3390/socsci8070216
![]() |
[24] | MacArthur E (2013) Towards the circular economy. J Ind Ecol 2: 23-44. |
[25] | Rüßmann M, Lorenz M, Gerbert P, et al. (2015) Industry 4.0: The future of productivity and growth in manufacturing industries. Bost Consult Gr 9: 54-89. |
[26] |
Gorissen L, Vrancken K, Manshoven S (2016) Transition thinking and business model innovation—towards a transformative business model and new role for the reuse centers of Limburg, Belgium. Sustainability 8: 112. doi: 10.3390/su8020112
![]() |
[27] | Liao Y, Deschamps F, Loures ER, et al. (2017) Past, present and future of Industry 4.0-a systematic literature review and research agenda proposal. Int J Prod Res 55: 3609-3629. |
[28] | Smart P, Hemel S, Lettice F, et al. (2017) Pre-paradigmatic status of industrial sustainability: a systematic review. Int J Oper Prod Manage, 37. |
[29] |
Ge X, Jackson J (2014) The big data application strategy for cost reduction in automotive industry. SAE Int J Commer Veh 7: 588-598. doi: 10.4271/2014-01-2410
![]() |
[30] |
Antikainen M, Uusitalo T, Kivikytö-Reponen P (2018) Digitalisation as an enabler of circular economy. Procedia CIRP 73: 45-49. doi: 10.1016/j.procir.2018.04.027
![]() |
[31] | Askoxylakis I (2018) A framework for pairing circular economy and the Internet of Things. In Proceedings of the 2018 IEEE International Conference on Communications (ICC); IEEE, 1-6. |
[32] | Wang Y, Zhang M, Zuo Y (2016) Potential applications of IoT-based product lifecycle energy management. In Proceedings of the 2016 IEEE 11th Conference on Industrial Electronics and Applications (ICIEA), 1999-2002. |
[33] |
Soroka A, Liu Y, Han L, et al. (2017) Big data driven customer insights for SMEs in redistributed manufacturing. Procedia CIRP 63: 692-697. doi: 10.1016/j.procir.2017.03.319
![]() |
[34] | Isaksson O, Hallstedt SI, Rönnbäck AÖ (2018) Digitalisation, sustainability and servitisation: Consequences on product development capabilities in manufacturing firms. DS 91 Proc Nord 2018, Linköping, Sweden, 14th—17th August 2018. |
[35] | van Schaik A, Reuter MA (2016) Recycling indices visualizing the performance of the circular economy. World Met Erzmetall 69: 5-20. |
[36] |
Lahrour Y, Brissaud DA (2018) technical assessment of product/component re-manufacturability for additive remanufacturing. Procedia Cirp 69: 142-147. doi: 10.1016/j.procir.2017.11.105
![]() |
[37] |
Demestichas K, Daskalakis E (2020) Information and Communication Technology Solutions for the Circular Economy. Sustainability 12: 7272. doi: 10.3390/su12187272
![]() |
[38] | Grin J, Rotmans J, Schot J (2010) Transitions to sustainable development: new directions in the study of long term transformative change; Routledge; ISBN 1135151180. |
[39] |
Smith A, Stirling A, Berkhout F (2005) The governance of sustainable socio-technical transitions. Res Policy 34: 1491-1510. doi: 10.1016/j.respol.2005.07.005
![]() |
[40] | Jæger B, Menebo MM, Upadhyay A (2021) Identification of environmental supply chain bottlenecks: a case study of the Ethiopian healthcare supply chain. Manage Environ Qual An Int J. |
[41] |
Geels FW (2002) Technological transitions as evolutionary reconfiguration processes: A multi-level perspective and a case-study. Res Policy 31: 1257-1274. doi: 10.1016/S0048-7333(02)00062-8
![]() |
[42] |
Geels FW (2011) The multi-level perspective on sustainability transitions: Responses to seven criticisms. Environ Innov Soc Transitions 1: 24-40. doi: 10.1016/j.eist.2011.02.002
![]() |
[43] |
Falcone PM (2018) Analysing stakeholders' perspectives towards a socio-technical change: The energy transition journey in Gela Municipality. AIMS Energy 6: 645-657. doi: 10.3934/energy.2018.4.645
![]() |
[44] |
Geels FW, Schot J (2007) Typology of sociotechnical transition pathways. Res Policy 36: 399-417. doi: 10.1016/j.respol.2007.01.003
![]() |
[45] |
White GRT, Cicmil S, Upadhyay A, et al. (2019) The soft side of knowledge transfer partnerships between universities and small to medium enterprises: An exploratory study to understand process improvement. Prod Plan Control 30: 907-918. doi: 10.1080/09537287.2019.1582098
![]() |
[46] |
Scholz RW, Spoerri A, Lang DJ (2009) Problem structuring for transitions: The case of Swiss waste management. Futures 41: 171-181. doi: 10.1016/j.futures.2008.09.013
![]() |
[47] | Raven R (2005) Strategic niche management for biomass. Eindhoven Univ Netherlands. |
[48] |
Spielman DJ, Davis K, Negash M, et al. (2011) Rural innovation systems and networks: findings from a study of Ethiopian smallholders. Agric Human Values 28: 195-212. doi: 10.1007/s10460-010-9273-y
![]() |
[49] |
Freeman LC (1978) Centrality in social networks conceptual clarification. Soc Networks 1: 215-239. doi: 10.1016/0378-8733(78)90021-7
![]() |
[50] |
Meagher K, Rogers M (2004) Network density and R & D spillovers. J Econ Behav Organ 53: 237-260. doi: 10.1016/j.jebo.2002.10.004
![]() |
[51] |
Cowan R, Jonard N (2004) Network structure and the diffusion of knowledge. J Econ Dyn Control 28: 1557-1575. doi: 10.1016/j.jedc.2003.04.002
![]() |
[52] | Steuer J (1992) Defining virtual reality: Dimensions determining telepresence. J Commun 42: 73-93. |
[53] | Lee K (2012) Augmented reality in education and training. TechTrends 56: 13-21. |
[54] |
Azuma RT (1997) A survey of augmented reality. Presence Teleoperators Virtual Environ 6: 355-385. doi: 10.1162/pres.1997.6.4.355
![]() |
[55] | Hirve SA, Kunjir A, Shaikh B, et al. (2017) An approach towards data visualization based on AR principles. In Proceedings of the 2017 International Conference on Big Data Analytics and Computational Intelligence (ICBDAC), 128-133. |
[56] | Bezegová E, Ledgard M, Molemaker RJ, et al. (2017) Virtual reality and its potential for Europe. Ecorys, Bruxells. |
[57] | Watts DJ (2004) Small worlds: the dynamics of networks between order and randomness; Princeton university press; ISBN 0691117047. |
[58] |
Nobre GC, Tavares E (2017) Scientific literature analysis on big data and internet of things applications on circular economy: a bibliometric study. Scientometrics 111: 463-492. doi: 10.1007/s11192-017-2281-6
![]() |
[59] |
Bressanelli G, Adrodegari F, Perona M, et al. (2018) Exploring how usage-focused business models enable circular economy through digital technologies. Sustainability 10: 639. doi: 10.3390/su10030639
![]() |
[60] |
Baines T, Lightfoot HW (2014) Servitization of the manufacturing firm. Int J Oper Prod Manage 34: 2-35. doi: 10.1108/IJOPM-02-2012-0086
![]() |
[61] | Kamp B, Parry G (2017) Servitization and advanced business services as levers for competitiveness. Ind Mark Manage, 60. |
[62] |
Lenka S, Parida V, Wincent J (2017) Digitalization capabilities as enablers of value co‐creation in servitizing firms. Psychol Mark 34: 92-100. doi: 10.1002/mar.20975
![]() |
[63] |
Despeisse M, Baumers M, Brown P, et al. (2017) Unlocking value for a circular economy through 3D printing: A research agenda. Technol Forecast Soc Change 115: 75-84. doi: 10.1016/j.techfore.2016.09.021
![]() |
[64] | de Sousa Jabbour ABL, Jabbour CJC, Godinho Filho M, et al. (2018) Industry 4.0 and the circular economy: a proposed research agenda and original roadmap for sustainable operations. Ann Oper Res 270: 273-286. |
[65] | Ávila-Gutiérrez MJ, Martín-Gómez A, Aguayo-González F, et al. (2019) Standardization framework for sustainability from circular economy 4.0. Sustainability 11: 6490. |
[66] | Falcone PM (2020) Environmental regulation and green investments: The role of green finance. Int J Green Econ, 14. |
1. | Patrizio Giganti, Pasquale Marcello Falcone, Strategic Niche Management for Sustainability: A Systematic Literature Review, 2022, 14, 2071-1050, 1680, 10.3390/su14031680 | |
2. | Na Jin, Naiding Yang, Sayed Muhammad Fawad Sharif, Ruimeng Li, Juan Du, Influence of knowledge flow and knowledge stock on the technological niche through absorptive capacity in the R&D network, 2022, 0953-7325, 1, 10.1080/09537325.2022.2098101 | |
3. | Svetlana Zemlyak, Olga Gusarova, Svetlana Sivakova, Assessing the Influence of Collaborative Technology Adoption—Mediating Role of Sociotechnical, Organizational, and Economic Factors, 2022, 14, 2071-1050, 14271, 10.3390/su142114271 | |
4. | Julio Cabero-Almenara, Julio Barroso-Osuna, Rosabel Martinez-Roig, Mixed, Augmented and Virtual, Reality Applied to the Teaching of Mathematics for Architects, 2021, 11, 2076-3417, 7125, 10.3390/app11157125 | |
5. | Ghazala Rasheed, Muzafar Khan, Noman Malik, Adnan Akhunzada, Measuring Learnability through Virtual Reality Laboratory Application: A User Study, 2021, 13, 2071-1050, 10812, 10.3390/su131910812 | |
6. | Somaiieh Rokhsaritalemi, Abolghasem Sadeghi-Niaraki, Ho-San Kang, Jong-Won Lee, Soo-Mi Choi, Ubiquitous Tourist System Based on Multicriteria Decision Making and Augmented Reality, 2022, 12, 2076-3417, 5241, 10.3390/app12105241 | |
7. | Pasquale Marcello Falcone, Editorial to the 'Special Issue—Energy transition in a circular economy perspective' of AIMS Energy, 2022, 10, 2333-8334, 582, 10.3934/energy.2022029 | |
8. | Tina Katika, Ioannis Karaseitanidis, Dimitra Tsiakou, Christos Makropoulos, Angelos Amditis, Augmented Reality (AR) Supporting Citizen Engagement in Circular Economy, 2022, 2, 2730-597X, 1077, 10.1007/s43615-021-00137-7 | |
9. | Cristian Barra, Pasquale Marcello Falcone, Unraveling the impact of economic policy uncertainty on environmental efficiency: How do institutional quality and political orientation matter?, 2024, 36, 0954-1985, 1450, 10.1111/ecpo.12297 | |
10. | Caetano C R Penna, Oscar Yandy Romero Goyeneche, Cristian Matti, Exploring indicators for monitoring sociotechnical system transitions through portfolio networks, 2023, 50, 0302-3427, 719, 10.1093/scipol/scad015 |
Stage 1 | Stage 2 | Stage 3 | Stage 4 | |
Willingness | - | √ | √ | √ |
Power | - | - | √ | √ |
Knowledge | - | - | - | √ |
Niche Status | Absence | Embryonic | Proto-niche | Full |
Willingness | Power | Knowledge | Potentials | Limits | |
Procedure 1 | Analysis of categories and subcategories of the entire network | Analysis of categories and subcategories of the entire network | Analysis of categories and subcategories of the entire network | Procedure useful in the next steps without considering the structure of the countries. | Structural characteristics not considered could present relevant information. |
Procedure 2 | - | Analysis of the composition of each cluster representing a single country considering the possible internal and external interactions. | Analysis of the composition of each cluster representing a single country considering the possible internal and external interactions. | Possibility to discover potential new connections among actors. | No rule able to describe the method. |
Procedure 3 | - | - | Comparison with power results. | Change to justify previous results. | No rule able to describe the method. |
Measurements | Individuation of the mean of the values attached by single actors and consideration of the proportion of actors assigning a value equal to or greater than a recognized threshold | Cin−D=∑ni=1[C∗in−D−Cin−Di](n−1)2 | Dk=Lkn(n−1)/2 Detection of cliques and small world properties |
Making tangible data collected, understanding the status of the niche and elaboration of solutions to reach the full status. | Exclusive focus on sought parameters. |
Willingness | ||||||
Value | 1 | 2 | 3 | 4 | 5 | Tot |
Nodes/Arcs | 0 | 0 | 56 | 45 | 20 | 121 |
Power | ||||||||
Value | 1 | 2 | 3 | 4 | 5 | 6 | 7 | Tot |
Arcs/Connections | 22 | 23 | 8 | 33 | 11 | 28 | 5 | 130 |
Knowledge | ||||
Value | 1 | 2 | 3 | Tot |
Arcs/Connections | 84 | 2 | 47 | 133 |
Stage 1 | Stage 2 | Stage 3 | Stage 4 | |
Willingness | - | 46% medium, 54% higher | √ | √ |
Power | - | 0.057 | √ | √ |
Knowledge | - | 0.018 no cliques and small world properties | - | √ |
Niche Status | Absence | Embryonic | Proto-niche | Full |
Stage 1 | Stage 2 | Stage 3 | Stage 4 | |
Willingness | - | √ | √ | √ |
Power | - | - | √ | √ |
Knowledge | - | - | - | √ |
Niche Status | Absence | Embryonic | Proto-niche | Full |
Willingness | Power | Knowledge | Potentials | Limits | |
Procedure 1 | Analysis of categories and subcategories of the entire network | Analysis of categories and subcategories of the entire network | Analysis of categories and subcategories of the entire network | Procedure useful in the next steps without considering the structure of the countries. | Structural characteristics not considered could present relevant information. |
Procedure 2 | - | Analysis of the composition of each cluster representing a single country considering the possible internal and external interactions. | Analysis of the composition of each cluster representing a single country considering the possible internal and external interactions. | Possibility to discover potential new connections among actors. | No rule able to describe the method. |
Procedure 3 | - | - | Comparison with power results. | Change to justify previous results. | No rule able to describe the method. |
Measurements | Individuation of the mean of the values attached by single actors and consideration of the proportion of actors assigning a value equal to or greater than a recognized threshold | Cin−D=∑ni=1[C∗in−D−Cin−Di](n−1)2 | Dk=Lkn(n−1)/2 Detection of cliques and small world properties |
Making tangible data collected, understanding the status of the niche and elaboration of solutions to reach the full status. | Exclusive focus on sought parameters. |
Willingness | ||||||
Value | 1 | 2 | 3 | 4 | 5 | Tot |
Nodes/Arcs | 0 | 0 | 56 | 45 | 20 | 121 |
Power | ||||||||
Value | 1 | 2 | 3 | 4 | 5 | 6 | 7 | Tot |
Arcs/Connections | 22 | 23 | 8 | 33 | 11 | 28 | 5 | 130 |
Knowledge | ||||
Value | 1 | 2 | 3 | Tot |
Arcs/Connections | 84 | 2 | 47 | 133 |
Stage 1 | Stage 2 | Stage 3 | Stage 4 | |
Willingness | - | 46% medium, 54% higher | √ | √ |
Power | - | 0.057 | √ | √ |
Knowledge | - | 0.018 no cliques and small world properties | - | √ |
Niche Status | Absence | Embryonic | Proto-niche | Full |