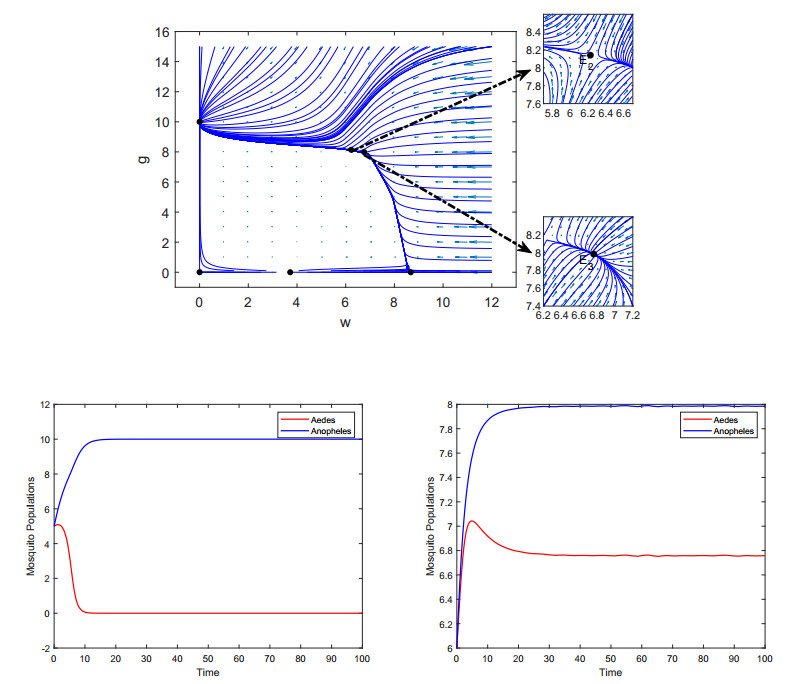
We investigated the shear thinning of normal and diabetic blood experimentally. The shear thinning of the blood has been analyzed using the Power-law model. Over the viscosity time course, the coagulation process of blood samples from diabetic and healthy subjects was observed. The shear thinning behavior of blood samples was examined in the shear rate ranging from 5 s−1 to 222 s−1, and viscosity time-course was studied at a shear rate of 50 s−1. The consistency coefficients were 8.638 ± 0.4860 mPa·s, and 6.658 ± 0.3219 mPa·s for diabetic blood and control, respectively. This difference was statistically significant (p < 0.0001). The parameters extracted from the viscosity–time curve were the time-to-gel point (TGP), maximum clot viscosity (MCV), and final clot viscosity (FCV). The diabetic blood exhibited a significantly high (p < 0.0001) shorter TGP (148.8 ± 6.024 s) than control (199.1 ± 4.865 s). A considerably higher MCV for diabetic blood (26.39 ± 1.451 cP) than the control (17.54 ± 2.324 cP) was reported. FCV for diabetic blood (10.89 ± 1.12 cP) was significantly higher than control (7.6 ± 0.8 cP). The viscosity time course as well as features obtained via the power-law model reflected the flow state of diabetic blood.
Citation: Mohamed A. Elblbesy, Bothaina A. Kandil. Hemorheological measurements over the shear-thinning regime. In vitro comparative study for hyperglycemia[J]. AIMS Biophysics, 2024, 11(2): 121-129. doi: 10.3934/biophy.2024008
[1] | Yang Li, Jia Li . Stage-structured discrete-time models for interacting wild and sterile mosquitoes with beverton-holt survivability. Mathematical Biosciences and Engineering, 2019, 16(2): 572-602. doi: 10.3934/mbe.2019028 |
[2] | Jia Li . Modeling of mosquitoes with dominant or recessive Transgenes and Allee effects. Mathematical Biosciences and Engineering, 2010, 7(1): 99-121. doi: 10.3934/mbe.2010.7.99 |
[3] | Liming Cai, Shangbing Ai, Guihong Fan . Dynamics of delayed mosquitoes populations models with two different strategies of releasing sterile mosquitoes. Mathematical Biosciences and Engineering, 2018, 15(5): 1181-1202. doi: 10.3934/mbe.2018054 |
[4] | Yuanxian Hui, Genghong Lin, Qiwen Sun . Oscillation threshold for a mosquito population suppression model with time delay. Mathematical Biosciences and Engineering, 2019, 16(6): 7362-7374. doi: 10.3934/mbe.2019367 |
[5] | Zhongcai Zhu, Xiaomei Feng, Xue He, Hongpeng Guo . Mirrored dynamics of a wild mosquito population suppression model with Ricker-type survival probability and time delay. Mathematical Biosciences and Engineering, 2024, 21(2): 1884-1898. doi: 10.3934/mbe.2024083 |
[6] | Rajivganthi Chinnathambi, Fathalla A. Rihan . Analysis and control of Aedes Aegypti mosquitoes using sterile-insect techniques with Wolbachia. Mathematical Biosciences and Engineering, 2022, 19(11): 11154-11171. doi: 10.3934/mbe.2022520 |
[7] | Yunfeng Liu, Guowei Sun, Lin Wang, Zhiming Guo . Establishing Wolbachia in the wild mosquito population: The effects of wind and critical patch size. Mathematical Biosciences and Engineering, 2019, 16(5): 4399-4414. doi: 10.3934/mbe.2019219 |
[8] | Yun Li, Hongyong Zhao, Kai Wang . Dynamics of an impulsive reaction-diffusion mosquitoes model with multiple control measures. Mathematical Biosciences and Engineering, 2023, 20(1): 775-806. doi: 10.3934/mbe.2023036 |
[9] | Mugen Huang, Moxun Tang, Jianshe Yu, Bo Zheng . The impact of mating competitiveness and incomplete cytoplasmic incompatibility on Wolbachia-driven mosquito population suppressio. Mathematical Biosciences and Engineering, 2019, 16(5): 4741-4757. doi: 10.3934/mbe.2019238 |
[10] | Bo Zheng, Lihong Chen, Qiwen Sun . Analyzing the control of dengue by releasing Wolbachia-infected male mosquitoes through a delay differential equation model. Mathematical Biosciences and Engineering, 2019, 16(5): 5531-5550. doi: 10.3934/mbe.2019275 |
We investigated the shear thinning of normal and diabetic blood experimentally. The shear thinning of the blood has been analyzed using the Power-law model. Over the viscosity time course, the coagulation process of blood samples from diabetic and healthy subjects was observed. The shear thinning behavior of blood samples was examined in the shear rate ranging from 5 s−1 to 222 s−1, and viscosity time-course was studied at a shear rate of 50 s−1. The consistency coefficients were 8.638 ± 0.4860 mPa·s, and 6.658 ± 0.3219 mPa·s for diabetic blood and control, respectively. This difference was statistically significant (p < 0.0001). The parameters extracted from the viscosity–time curve were the time-to-gel point (TGP), maximum clot viscosity (MCV), and final clot viscosity (FCV). The diabetic blood exhibited a significantly high (p < 0.0001) shorter TGP (148.8 ± 6.024 s) than control (199.1 ± 4.865 s). A considerably higher MCV for diabetic blood (26.39 ± 1.451 cP) than the control (17.54 ± 2.324 cP) was reported. FCV for diabetic blood (10.89 ± 1.12 cP) was significantly higher than control (7.6 ± 0.8 cP). The viscosity time course as well as features obtained via the power-law model reflected the flow state of diabetic blood.
Dengue fever, malaria, Zika, and epidemic B encephalitis are all diseases transmitted by mosquitoes, which we call mosquito-borne diseases. Nowadays, mosquito-borne diseases are becoming more and more widespread and dangerous to human health. The prevention and control of these diseases has attracted the attention of the World Health Organization and others. It is the fifth leading cause of death from infectious diseases worldwide (after respiratory infections, HIV/AIDS, diarrhoeal diseases, and tuberculosis) and the second leading cause of death from infectious diseases in Africa after HIV/AIDS. Today, malaria is a public health problem in 109 countries, covering territories inhabited by some 3.3 billion people, and about half of the world's population is at risk of malaria, especially in low-income countries [1]. There is also dengue fever, which was first recorded in Southeast Asia in the middle of the last century. Since then, dengue fever has become a widespread mosquito-borne disease in humans [2]. As there is no safe and available vaccine, the control of mosquito densities is an effective way to prevent and control mosquito-borne diseases. However, traditional mosquito control methods (e.g., removing mosquito breeding sites, spraying insecticides, etc.) are effective in removing mosquitoes from areas in the short term, but they have the disadvantages of being "environmentally polluting", "poorly specific", and "unsustainable" [3]. Indoor and outdoor insecticide spraying has been applied for many years for controlling mosquito populations. Despite some mosquito-borne diseases having been successfully controlled in many regions through vector-targeted intervention such as insecticide-treated bed nets (ITNs) and indoor residual sprays (IRS), massive and long time spraying of adulticide is not recommended [4]. The new approaches to mosquito-borne disease prevention and control are urgently needed.
The sterile insect technology (SIT) is used to reduce wild mosquito populations [5,6]. SIT is an environmentally friendly alternative strategy that is gaining renewed interest for the control of mosquito populations. It uses chemical, physical, radiation, or genetic modification techniques to enable male mosquitoes to mate but not reproduce [7]. Utilizing radical or other chemical or physical methods, male mosquitoes are genetically modified to be sterile so that they are incapable of producing offspring despite being sexually active. These sterile male mosquitoes are released into the natural environment and mate with wild females, which either do not produce fertilized eggs or the eggs they do produce do not hatch. Repeatedly releasing large numbers of sterile male mosquitoes into a given environment will result in a significant reduction or even extinction of mosquitoes in that area [8]. Mathematical models have been developed to control mosquito populations using SIT, particularly for the study of interaction dynamics in mosquito populations or mosquito control [9,10]. Esteva et al. [11] proposed a mathematical model to assess the effects of irradiated (or transgenic) male insects introduction into previously infested areas. Flores [12] considered a mathematical model of eradication by competition between wild and sterile species in the presence of immigrants. Li [13] presented two discrete-time models for interacting populations of wild and genetically altered mosquitoes, and reference [14] studied continuous-time models for interacting wild and transgenic mosquito populations. Yu [15] studied the existence and stability of two periodic orbits of the interaction of wild and the released sterile mosquitoes model. Li et al. [16] formulated stage-structured continuous-time mathematical models for the interactive dynamics of the wild and sterile mosquitoes. Additionally, Li [5,17,18,19] and Yu [20,21,22] have done a lot of outstanding work. Many dynamical models had also been developed for the control of infectious diseases [23,24,25,26,27]. In addition, there are many national and international scholars [28,29] who have conducted related studies on sterile mosquitoes, which have greatly assisted in the completion of this paper.
Building on previous studies, we consider the Allee effect [30] on mosquito populations. Low population densities are most likely to occur when mosquitoes move into new territories, which may produce a mate-finding Allee effect. Although the study of the Allee effect has a long history in mathematical biology, most people consider the strong Allee effect to be the Allee effect, thus ignoring the weak Allee effect [31]. In fact, there are strong and weak Allee effects. The wild mosquito populations display strong or weak Allee effect [32,33]. If the average growth rate of low population densities is lower than the growth rate of high densities, but still positive, there is a weak Allee effect. On the contrary, it can become very low, becoming negative below a certain value, which is called the Allee threshold, when it is a strong Allee effect. If the number of populations affected by the strong Allee effect drops below this threshold, then the population growth rate will become negative and the population will decrease until it approaches zero or becomes extinct. In this paper, the strong Allee effect and weak Allee effect of Aedes aegypti are considered successively.
However, previous models have focused on one type of mosquito in one area and less on multiple types of mosquitoes in one area [19,21,28]. Previous researches have focused on changes in the number of single mosquito populations after releasing sterile mosquitoes, with less attention paid to changes in the number of mosquito competitors. In this paper, we mainly improve on the model of [5] by introducing a competitive relationship, and we focus on the changes in the number of Aedes and their competitors after the release of sterile mosquitoes as the release value changes. Different species of mosquitoes transmit different diseases, for example, Aedes aegypti transmits dengue fever and Anopheles transmits malaria. It is assumed that there are two types of mosquitoes (Anopheles and Aedes) in an area where dengue fever is a serious problem. We also assume that mosquito populations are homogeneous and do not distinguish between their sexes. To control the spread of dengue fever, sterile Aedes are released into the area. To simplify the model, only the Allee effect of Aedes is considered. The existence and stability of the equilibrium under different conditions are analyzed, and numerical simulations are carried out to observe the changes in the numbers of Anopheles and Aedes.
The paper is organized as follows. In Section 2, we consider the strong Allee effect on Aedes populations. In Section 3, we consider the weak Allee effect on Aedes populations. In Section 4, we give the two models for the impulsive releases of sterile Aedes and perform numerical simulations. In Section 5, we finally give a brief discussion on our findings.
Based on [5], we assume that mosquitoes obey logistic growth while considering interspecific competition. The population of Aedes is denoted as w and the population of Anopheles mosquitoes is denoted as z. Since the two species of mosquitoes compete with each other, a decrease in one leads to a decrease in competition for food in the water by the other, which leads to an increase in the population of the other species. We consider Aedes populations with the strong Allee effect. The competition model for the two types of mosquitoes is as follows,
{dwdt=aC(w)(w−m)(1−wK)w−μw−ξ1wz,dzdt=rz(1−zK)−ξ2wz, |
where C(w) is the number of matings per individual, per unit of time; a is the number of wild offspring produced per mate; ξ1 indicates the effect of Anopheles on Aedes; ξ2 indicates the effect of Aedes on Anopheles; μ is the natural mortality rate of Aedes; r is the intrinsic growth rate by Anopheles; m is the parameter that responds to the strength of the Allee effect, where it is assumed that 0<m≪K; and K is the maximum capacity of the external environment.
The population of sterile Aedes is denoted as g. Sterile mosquitoes are released in the adult stage, so competition with Anopheles mosquitoes is not considered. We also assume that C(w)=c is constant and merge c and a to write it as a. The competition model after constant release of sterile mosquitoes is
{dwdt=aww+g(w−m)(1−wK)w−μw−ξ1wz,dgdt=b−μg,dzdt=rz(1−zK)−ξ2wz, |
where b is the releasing amount of the sterile Aedes mosquitoes and is constant.
Assuming that the loss of released males is compensated by new releasing, that is, g=bμ, the following limit system can be obtained,
{dwdt=aww+bμ(w−m)(1−wK)w−μw−ξ1wz,dzdt=rz(1−zK)−ξ2wz. | (2.1) |
To begin, let ρ1=am+aK, ρ2=amK+μK, and the system (2.1) has two equilibria: O(0,0), B1(0,K). Next, we will prove the existence of the boundary equilibria on the w-axis.
In fact, define
P(w):=aw3−ρ1w2+ρ2w+bK=0. |
We have
P′(w)=3aw2−2ρ1w+ρ2. |
Let
α=3μKK2+m2−mK. |
If a≤α, then P′(w)≥0; thus, it is known that P(w) is a monotonically increasing function:
P(0)>0andlimw→∞P(w)=∞. |
Therefore, the boundary equilibrium does not exist in this condition. When a>α, there exists 0<w1<w2 such that P′(w1)=P′(w2)=0, where
w1,2=ρ1∓√ρ21−3aρ23a, |
w1 and w2 are the local minimum and local maximum value points of P(w), respectively. Define
b1:=(−aw22+ρ1w2−ρ2)w2K. | (2.2) |
When P(w2)>0 (i.e., b>b1), the system (2.1) does not have the boundary equilibria on the w-axis; when P(w2)=0 (i.e., b=b1), the system (2.1) only has a boundary equilibrium B2(w2,0); when P(w2)<0 (i.e., b<b1), the system (2.1) has two boundary equilibria B3(¯w1,0) and B4(¯w2,0)(¯w1<w2<¯w2).
Finally, we show the existence of positive equilibria E(w∗,(1−ξ2w∗/r)K). It is obvious that 1−ξ2w∗/r>0 is a necessary condition for the existence of positive equilibria.
Let
Q(w):=−aw3+(ρ1+ξ1ξ2K2r)w2−(ρ2+ξ1K2−ξ1ξ2bμrK2)w−bK−ξ1bμK2, |
then we obtain
Q′(w)=−3aw2+2(ρ1+ξ1ξ2K2r)w−ρ2−ξ1K2+ξ1ξ2bμrK2. |
Its discriminant is
Δ=4(K2−mK+m2)a2+(−12ξ1K2−12μK+12bμrξ1ξ2K2+8mξ1ξ2K2r+8ξ1ξ2K3r)a+4ξ21ξ22K4r2≐Aa2+Ba+C. |
If Δ≤0 (i.e., a≤−B+√B2−4AC2A), by definition, we have
Q(0)=−bK−ξ1bμK2<0andlimw→∞Q(w)=−∞. |
Therefore, the system (2.1) doesn't have positive equilibrium in this condition.
If Δ>0 (i.e., a>−B+√B2−4AC2A), there exists 0<w∗1<w∗2, such that Q′(w∗1)=Q′(w∗2)=0, where
w∗1=ρ1+ξ1ξ2K2r−√(ρ1+ξ1ξ2K2r)2−3a(ρ2+ξ1K2−bξ1ξ2K2μr)3a, |
and
w∗2=ρ1+ξ1ξ2K2r+√(ρ1+ξ1ξ2K2r)2−3a(ρ2+ξ1K2−bξ1ξ2K2μr)3a. |
w∗1 and w∗2 are the local minimum and local maximum value points of Q(w), respectively. Define
b2:=aμw∗32−(μρ1+μξ1ξ2K2r)w∗22+(μρ2+μξ1K2)w∗2ξ1ξ2K2rw∗2−μK−ξ1K2. | (2.3) |
When Q(w∗2)<0 (i.e., b>b2), the system (2.1) does not have the positive equilibria; when Q(w∗2)=0 (i.e., b=b2), the system (2.1) only has a positive equilibrium E1 (w∗2,(1−ξ2w∗2/r)K); when Q(w∗2)>0 (i.e., b<b2), the system (2.1) has two positive equilibria E2,3(¯w∗1,2,(1−ξ2¯w∗1,2/r)K), where ¯w∗1<w∗2<¯w∗2.
Therefore, we can summarize our findings with the following theorems.
Theorem 2.1. There exist equilibria O(0,0) and B1(0,K) for system (2.1).
(i) If b1<b2, then when 0<b<b1, the system (2.1) has two positive equilibria E2, E3 and two boundary equilibria B3, B4; when b=b1, the system (2.1) has two positive equilibria E2, E3 and a boundary equilibrium B2; when b1<b<b2, the system (2.1) has two positive equilibria E2, E3 and does not have the boundary equilibria on the w-axis; when b=b2, the system (2.1) has a positive equilibrium E1 and does not have the boundary equilibria on the w-axis; when b>b2, the system (2.1) does not have the positive equilibria and the boundary equilibria on the w-axis.
(ii) If b1=b2, then when b>b1, the system (2.1) does not have the boundary equilibria on the w-axis and the positive equilibria; when b=b1, the system (2.1) only has a positive equilibrium E1 and a boundary equilibrium B2; when b<b1, the system (2.1) has two positive equilibria E2, E3 and two boundary equilibria B3, B4.
(iii) If b1>b2, when 0<b<b2, the system (2.1) has two positive equilibria E2, E3 and two boundary equilibria B3, B4; when b=b2, the system (2.1) has a positive equilibrium E1 and has two boundary equilibria B3, B4; when b2<b<b1, the system (2.1) does not have the positive equilibria and has two boundary equilibria B3, B4; when b=b1, the system (2.1) does not have the positive equilibria and has a boundary equilibrium B2; when b>b1, the system (2.1) does not have the positive equilibria and the boundary equilibria on the w-axis.
From the linearization of system (2.1), it is trivial to show that the equilibrium O(0,0) is a saddle point and the equilibrium B1(0,K) is a locally asymptotically stable node.
We then investigate the stability of the boundary equilibria. The Jacobian matrix of system (2.1) at the boundary equilibria has the following form,
JB=(D−ξ1w0r−ξ2w), |
where
D=bμ(−4aw3+3aKw2+3amw2−2amKw)−3aw4+2aKw3+2amw3−amKw2K(w+bμ)2−μ. |
After calculations, we have
det(JB)=bμ(ξ2w−r)(4aw3−3a(m+K)w2+2amKw)+(ξ2w−r)(3aw4−2a(m+K)w3+amKw2)K(w+bμ)2+μ(ξ2w−r)=1K(w+bμ)2[2μKw(ξ2w−r)bμ+μK(ξ2w−r)(bμ)2+bμ(ξ2w−r)(4aw3−3a(m+K)w2+2amKw)+(ξ2w−r)w2(3aw2−2a(m+K)w+am+μK)]=1K(w+bμ)2[μK(ξ2w−r)(bμ)2+bμ(ξ2w−r)(4aw3−3a(m+K)w2+2amKw+2μKw)+(ξ2w−r)w2P′(w)]=1K(w+bμ)2[bμ(ξ2w−r)(aw3−a(m+K)w2+(amK+μK)w+bK)+((ξ2w−r)w2+bμ(ξ2w−r)w)P′(w)]=1K(w+bμ)2[bμ(ξ2w−r)P(w)+((ξ2w−r)w2+bμ(ξ2w−r)w)P′(w)]. |
If r−ξ2w<0, since P(¯w1)=0, P′(¯w1)<0, then det(JB3)<0; thus, the equilibrium B3(¯w1,0) is a saddle point. Since P(¯w2)=0, P′(¯w2)>0, then det(JB4)>0; thus, D<0, tr(JB4)<0, and the equilibrium B4(¯w2,0) is a locally asymptotically stable node.
If r−ξ2w>0, since P(¯w1)=0,P′(¯w1)<0, then det(JB3)>0; thus, D>0, tr(JB3)>0, and the equilibrium B3(¯w1,0) is a saddle point. Since P(¯w2)=0,P′(¯w2)>0, then det(JB4)<0; thus, the equilibrium B4(¯w2,0) is a saddle point.
We finally investigate the stability of the positive equilibria. The Jacobian matrix of system (2.1) at the positive equilibria has the following form,
JE=(F−ξ1w−ξ2z−rzK), |
where
F=bμ(−4aw3+3aKw2+3amw2−2amKw)−3aw4+2aKw3+2amw3−amKw2K(w+bμ)2−μ−ξ1z. |
After calculations, we obtain
det(JE)=bμ(r−ξ2w)(4aw3−3a(m+K)w2+2amKw)+(r−ξ2w)(3aw4−2a(m+K)w3+amKw2)K(w+bμ)2+μr(1−ξ2w)+ξ1rK(1−ξ2w)2−rξ1ξ2wK(1−ξ2w)=bμr(1−ξ2w)(4aw3−3a(m+K)w2+2amKw)+r(1−ξ2w)(3aw4−2a(m+K)w3+amKw2)K(w+bμ)2+(1−ξ2w)(μr+rξ1K)=(rbμP(w)+r(w2+bμw)P′(w)K(w+bμ)2+rξ1K−2ξ1ξ2Kw)(1−ξ2wr). |
Suppose
rbμP(¯w∗)+r(¯w∗2+bμ¯w∗)P′(¯w)K(¯w∗+bμ)2+rξ1K−2ξ1ξ2K¯w∗>0, | (2.4) |
then det(JE)>0. Thus, F<0, tr(JE)<0, and the positive equilibrium is a locally asymptotically stable node.
On the other hand, suppose
rbμP(¯w∗)+r(¯w∗2+bμ¯w∗)P′(¯w∗)K(¯w∗+bμ)2+rξ1K−2ξ1ξ2Kw∗<0, | (2.5) |
then det(JE)<0, and the positive equilibrium is a saddle point.
Thus, we can summarize our proven results with the following theorem.
Theorem 2.2. The equilibrium O(0,0) is a saddle point and B1(0,K) is a locally asymptotically stable node.
(i) If 1−ξ2w<0, then the equilibrium B2(¯w∗1,0) is a saddle point and the equilibrium B3(¯w∗2,0) is a locally asymptotically stable node. The system (2.1) does not have the positive equilibria.
(ii) If 1−ξ2w>0, then the equilibria B2(¯w∗1,0) and B3(¯w∗2,0) are saddle points. When (2.4) holds, the positive equilibrium is a saddle point, and when (2.5) holds, the positive equilibrium is a locally asymptotically stable node.
For clarity, in this chapter we fix the system parameters in our simulations as follows,
a=1,r=0.4737,μ=0.5,m=1,ξ1=0.03764,ξ2=0.01414,K=10. | (2.6) |
For simplicity of calculation, we omit the order of magnitude of K.
We note that K is mainly determined by the environmental conditions in the study area and is proportional to the area size. Our simulations show that a different value of K yields different mosquito abundance but hopefully does not change the dynamics considerably, which allows us to take K=10 as a representative example. Substituting these values into (2.2) and (2.1), we obtain the threshold release values,
b1=9.2671,b2=4.5394. |
When the release parameter is b=4.5<b2, B1(0,10) is a locally asymptotically stable node. 1−ξ2w∗/r=0.7963>0, therefore, there exist two positive equilibria, E2(6.225,8.142), which is a locally asymptotically stable node, and E3(6.762,7.982), which is a saddle point. Trajectories may approach either B1 or E2, depending on their initial conditions. Solutions with different initial values approach either B1 or E2, as shown in the lower left and right figures in Figure 1, respectively. That is, Aedes may be wiped out, or the two types of mosquitoes may coexist, depending on the initial sizes of the Aedes and Anopheles.We give the following numerical examples to illustrate the dynamics of system (2.1).
We show in the following examples the effect of different thresholds on the positive and boundary equilibria, in order to confirm the results in (iii) of Theorem 2.1.
When the release parameter is b=4.6>b2, B1(0,10) is also a locally asymptotically stable node. The system doesn't have the positive equilibria, and solutions approach B1 as shown in the left and right figures in Figure 2. This means that the Aedes mosquito will become extinct in the wild, so threshold b2 has guiding significance for releasing sterile Aedes mosquitoes. As long as release parameter is greater than b2, wild Aedes mosquitoes will become extinct regardless of the initial value.
The cases when b is greater than b2 and b is slightly less than b1 and have been simulated numerically in the case above. Next, the cases for when b=9.2<b1 and b=9.3>b1 will be simulated in the left and right figures of Figure 3, respectively. Numerical simulations show the conditions for the existence of boundary equilibria.
In cases where a small mosquito population size may make finding a mate difficult, we assume the existence of the weak Allee effect such that the mating rate is C(w+g)=c0(w+g)/(1+w+g), where c0 is the maximum mating rate. Under these assumptions, which are still written as a after we have merged c0 into the birth rate, the dynamics of mosquito interactions are governed by the following system,
{dwdt=aw1+w+g(1−wK)w−μw−ξ1wz,dgdt=b−μg,dzdt=rz(1−zK)−ξ2wz. |
Similarly, let g=bμ, and the following limit system can be obtained,
{dwdt=aw1+w+bμ(1−wK)w−μw−ξ1wz,dzdt=rz(1−zK)−ξ2wz. | (3.1) |
To begin, the system (3.1) has the origin O′(0,0) as a trivial equilibrium. There exist boundary equilibria B′1(0,K), B′2(w3,0), B′3(w4,0), where
w3=aK−μK+√(aK−μK)2−4aK(μ+b)2a,w4=aK−μK−√(aK−μK)2−4aK(μ+b)2a. |
We then consider the positive equilibria of system (3.1), whose components satisfy the following equations,
{aw1+w+bμ(1−wK)−μ−ξ1z=0,r(1−zK)−ξ2w=0. |
This system of equations has two solutions,
E′1=(w∗3,(1−ξ2w∗3r)K), |
E′2=(w∗4,(1−ξ2w∗4r)K), |
where
w∗3=bξ1ξ2K2−μrξ1K2+μξ1ξ2K2+aμrK−μ2rK−√s12μ(ar−ξ1ξ2K2),w∗4=bξ1ξ2K2−μrξ1K2+μξ1ξ2K2+aμrK−μ2rK+√s12μ(ar−ξ1ξ2K2), |
s1=(bξ1ξ2K2−μrξ1K2+μξ1ξ2K2+aμrK−μ2rK)2−4μrK(ξ1K+μ)(b+μ)(ar−ξ1ξ2K2). |
We discuss positive equilibria, so let 1−ξ2w∗/r>0 and s1>0. It is apparent from s1>0 that b<b3 or b>b4, where
b3:=μ(−rξ21ξ2K3−ξ21ξ22K3−arξ1ξ2K2−μrξ1ξ2K2+2ar2ξ1K+2aμr2−2√s2)ξ21ξ22K3, |
b4:=μ(−rξ21ξ2K3−ξ21ξ22K3−arξ1ξ2K2−μrξ1ξ2K2+2ar2ξ1K+2aμr2+2√s2)ξ21ξ22K3, |
s2=ar2ξ31ξ22K5+aμr2ξ21ξ22K4−ar3ξ31ξ2K4−a2r3ξ21ξ2K3−2aμr3ξ21ξ2K3−a2μr3ξ1ξ2K2+a2r4ξ21K2−aμ2r3ξ1ξ2K2+2a2μr4ξ1K+a2μ2r4. |
It is apparent from 1−ξ2w∗3/r>0 that b>b5, where
b5:=arξ2K−μrξ2K−μξ22K−ar2ξ22K. |
If ar−ξ1ξ2K2<0, bξ1ξ2K2−μrξ1K2+μξ1ξ2K2+aμrK−μ2rK<0, then the system (3.1) has a positive equilibrium w∗3; when ar−ξ1ξ2K2<0, bξ1ξ2K2−μrξ1K2+μξ1ξ2K2+aμrK−μ2rK>0, then the system (3.1) has a positive equilibrium w∗3; when ar−ξ1ξ2K2>0, bξ1ξ2K2−μrξ1K2+μξ1ξ2K2+aμrK−μ2rK<0, then the system (3.1) doesn't have positive equilibria; when ar−ξ1ξ2K2>0, bξ1ξ2K2−μrξ1K2+μξ1ξ2K2+aμrK−μ2rK>0, then the system (3.1) has two positive equilibria w∗3 and w∗4.
Define
b6:=μrξ1K−μξ1ξ2K−aμr+μ2rξ1ξ2K. |
Thus, we can summarize what we have proved as the following theorem.
Theorem 3.1. When b>b5, b<b3, and b>b4 satisfy one of them.
(i) If ar−ξ1ξ2K2<0, then the system (3.1) only has a positive equilibrium.
(ii) If ar−ξ1ξ2K2>0, when b>b6, the system (3.1) has two positive equilibria; when b<b6, the system (3.1) doesn't have the positive equilibria.
The origin O′(0,0) is a trivial equilibrium and the Jacobian matrices at O′ and B′1 are
JO′=(−μ00r), |
and
JB′1=(−ξ1K−μ0−ξ2K−r). |
It is easy to see O′ is a saddle point and B′1 is a stable node.
Thus, the Jacobian matrices of system (3.1) at the boundary equilibrium B′2, B′3 are
JB′2=(F1−ξ1w30G1), |
JB′3=(F2−ξ1w40G2), |
where
F1=aμw3(−μw23−2(μ+b)w3+μK+bK)((1+w3)μ+b)2K,G1=r−ξ2w3, | (3.2a) |
and
F2=aμw4(−μw24−2(μ+b)w4+μK+bK)((1+w4)μ+b)2K,G2=r−ξ2w4. | (3.2b) |
If we plug
aw(1−wK)1+w+bμ−μ=0 |
back into (3.2a) and (3.2b), we obtain
F1=−aμKw23+μ2+μbμw3+μ+b,F2=−aμKw24+μ2+μbμw4+μ+b. |
A routine computation gives rise to
G1=2ar−aξ2K+μξ2K+ξ2√(aK−μK)2−4aK(μ+b)2a, |
G2=2ar−aξ2K+μξ2K−ξ2√(aK−μK)2−4aK(μ+b)2a, |
and we obtain G1>G2>0. Define
b7:=(a−μ)2K−4aμ4a. |
If b<b7, then F1<0, F2<0, and it is easy to show that B′2, B′3 are both saddle points. If b>b7, then F1>0, F2>0, and it is easy to show that B′2, B′3 are both unstable nodes.
To discuss the stability of the positive equilibria, the system (3.1) can be expressed as
{dwdt=ξ1w(f1(w)−z),dzdt=rzK(f2(w)−z), | (3.3) |
where
f1(w)=aw(1−wK)ξ1(1+w+bμ)−μξ1,f2(w)=K−ξ2Krw. |
It follows from
f′1(w)=aμ((−w2−2w+K)μ+(K−2w)b)((w+1)μ+b)2ξ1K,f″1(w)=−2aμ(b+μ)(μK+b+μ)ξ1K(μw+b+μ)3<0, |
that f1(w) is an upper convex function.
Let f′1(w)=0. We can get the maximum value point,
w0=−b−μ+√μbK+μ2K+b2+2μb+μ2μ. |
If z1=f1(w) and z2=f2(w) intersect in the first quadrant, the maximum value of f1(w) must be positive. Assuming that z1=f1(w) and z2=f2(w) have two intersections E′1 and E′2 in the first quadrant, the first quadrant is divided into five regions, as shown in Figure 4. In regions III, IV, and V, dwdt<0, and in regions I and II, dwdt>0. In regions II and III, dzdt<0, and in regions I, IV, and V, dzdt>0. It follows that E′1 is a saddle point and E′2 is a stable node. Thus, we can summarize what we have proved as the following theorem.
Theorem 3.2. O′ is a saddle point and B′1 is a stable node.
(i) If b<b7, then B′2, B′3 are both saddle points. If b>b7, then F1>0, F2>0. Thus, B′2, B′3 are unstable nodes.
(ii) If the system (3.1) has only one positive equilibrium, then it is a saddle point.
(iii) If the system (3.1) has two positive equilibria, then E′1 is a saddle point and E′2 is a stable node.
We use the following parameters in this example,
a=2,r=0.7,μ=0.7,ξ1=0.3764,ξ2=0.28,K=10. | (3.4) |
ar−ξ1ξ2K2=−9.1392<0 is satisfied. The threshold release values are b3=−5.3240, b4=−0.9838, b5=1.3000, b6=0.4456. For b=0.5<b5, E′1 is the only positive equilibrium of system (3.1), which is a saddle point. For b=1.4>b5, system (3.1) doesn't have the positive equilibria. The dynamics are shown in Figure 5.
In the left figure of this example, parameters are given as
a=1.5,r=2,μ=0.5,ξ1=0.04,ξ2=0.34,K=10. | (3.5) |
ar−ξ1ξ2K2=1.6400>0 is satisfied. The threshold release values are b3=0.3759, b4=21.7210, b5=0.1920, b6=−4.9118. For b=0.3>b5>b6, there exist two positive equilibria, E′1, which is a saddle point, and E′2, which is a stable node.
In the right figure of this example, with the parameters
a=2,r=2,μ=0.01,ξ1=0.3,ξ2=0.1,K=10. | (3.6) |
ar−ξ1ξ2K2=1>0 is satisfied. The threshold release values are b3=0.0016, b4=0.3806, b5=−40.2100, b6=0.00573. For b=0.0015<b3<b6, the system (3.1) doesn't have the positive equilibria.
A constant policy was applied in recent field trials of mosquito suppression. During the mosquito active months, a constant amount of sterile males were released in each control site at regular intervals, about two or three times per week. Suppose that the release starts at time t=0, and infected males of a constant amount c are released after a constant waiting period T. Let Ti=iT, i=0,1,2,⋯, then g(t) is increased by c when t=Ti. Since infected mosquitoes are released at the adult stage where intraspecific competition is much less than for larvae, we assume that g(t) decays exponentially and that the mortality rate μ is the same as in wild mosquitoes. We improve model (2.1) or (3.1) to get the model of mosquito population suppression under a constant release policy as follows,
{dwdt=aww+g(w−m)(1−wK)w−μw−ξ1wz,dgdt=−μg,dzdt=rz(1−zK)−ξ2wz,g(t)=0,t≤0;g(0+)=c;g(T+i)=c+g(Ti),i=1,2,⋯, | (4.1) |
or
{dwdt=aw1+w+g(1−wK)w−μw−ξ1wz,dgdt=−μg,dzdt=rz(1−zK)−ξ2wz,g(t)=0,t≤0;g(0+)=c;g(T+i)=c+g(Ti),i=1,2,⋯. | (4.2) |
In the last equality, g(T+i) is understood as limt→T+ig(t), and g(Ti), not written as g(T−i) for simplicity of notation, is interpreted as limt→T−ig(t).
We can find the upper and lower bounds of g(t) in this system of impulse differential equations.
Theorem 4.1. Let (w(t),g(t),z(t)) be a solution of (4.1) or (4.2), then
limt→∞infg(t)=ceμT−1andlimt→∞supg(t)=c1−e−μT. | (4.3) |
Proof. g(t) is continuously differentiable away from the jumping points Ti, and
g(t)=g(T+i)e−μ(t−Ti) in (Ti,Ti+1]. |
This leads to the iteration formulas for the two sequences {g(Ti)} and {g(T+i)}
g(Ti+1)=g(T+i)e−μT=[c+g(Ti)]e−μT,g(T+i+1)=c+g(T+i)e−μT. | (4.4) |
Evidently, g(T1)>g(T0)=0, and g(T+1)=c+g(T1)>c=g(T+0). In general, if g(Tk+1)>g(Tk) for an integer k≥0, then
g(Tk+2)=[c+g(Tk+1)]e−μT>[c+g(Tk)]e−μT=g(Tk+1). |
By induction, it is easy to see that the sequence {g(Ti)} is strictly increasing. The same can be proven by similar induction for the sequence {g(T+i)}. Next, we will prove
g(Ti)<ceμT−1 and g(T+i)<c1−e−μT. | (4.5) |
Both inequalities in (4.5) are apparently correct when i=0. Assuming that the first one holds for i=k≥0, from the first equality in (4.4), it can be seen that
g(Tk+1)=(c+g(Tk))e−μT<(c+ceμT−1)e−μT=ceμT−1. |
The first inequality in Eq (4.5) was verified through induction. The second inequality can be proved in a similar way. Thus, the two sequences {g(Ti)} and {g(T+i)} converge, and by (4.4) we find
limi→∞g(Ti)=ceμT−1 and limi→∞g(T+i)=c1−e−μT. | (4.6) |
Because g(t) decreases strictly in (Ti,Ti+1], we see that g(t) approaches its upper limit along the sequence {g(T+i)} and its lower limit along {g(Ti)}. Thus, (4.6) implies (4.3).
Further, we can obtain the following theorem.
Theorem 4.2. System (4.1) or system (4.2) has a unique periodic solution ˜g(t) with period T, where
˜g(t)=ce−μ(t−Ti)1−e−μT,in(Ti,Ti+1], |
and ˜g(t) is globally asymptotically stable.
Proof. According to Theorem 4.1 and Lemma 3.3 in [34], it follows that
˜g(t)=ce−μ(t−Ti)1−e−μT, in (Ti,Ti+1]. |
Integrating the second equation of (4.1) or (4.2), we obtain
g(t)=g(T+i)e−μ(t−Ti). | (4.7) |
In fact, we can deduce the following stroboscopic map of (4.7):
g(T+i+1)=g(T+i)e−μT+c. | (4.8) |
(4.8) has a unique positive equilibrium
g∗=c1−e−μT. |
Using iterative technique on (4.8), we obtain
g(T+i)=c(1+e−μT+⋯+e−μTi−1)=c(1−e−μTi)1−e−μT. |
Thus, limi→∞g(T+i)=g∗, so ˜g(t) is globally asymptotically stable.
In mosquito control, it is more desirable to suppress wild populations to low levels for a short period of time to interrupt the spread of mosquito-borne diseases. As shown in the left figure of Figure 7, we assume (2.6) again and that the males are released twice in 5 days (T=2.5), each releasing the amount c=12.3. Aedes with strong Allee effect is controlled within 5 weeks. As shown in the right figure of Figure 7, parameters are given as (3.5) and the males are released twice in 5 days (T=2.5), each releasing the amount c=1.35. Aedes with weak Allee effect is controlled within 5 weeks. Because of the extinction of Aedes, the population of Anopheles will approach the environmental capacity at a rapid rate.
We hold the constant waiting period T=2.5, and the releasing amount c becomes 11, 11.6, and 11.9, respectively. The wild Aedes populations with strong Allee effect are shown in the left figure of Figure 8. Numerical simulations show that the amount of release affects how quickly mosquito populations are suppressed. The wild Aedes population curves almost overlap in the first ten days. When the releasing amount is c=12.9, it takes 25 days to suppress Aedes populations. When the releasing amount is c=12.6, it takes 28 days. When the releasing amount is c=12, it is hard to suppress wild Aedes populations. Similarly, we also hold the constant waiting period T=2.5, and the releasing amount c becomes 1.05, 1.65, and 1.95, respectively. The wild Aedes population with weak Allee effect is shown in the right figure of Figure 8. When the releasing amount is c=1.95, it takes 21 days to suppress Aedes populations. When the releasing amount is c=1.65, it takes 25 days. When the releasing amount is c=1.05, it takes 80 days.
In this example, we hold the releasing amount c=12.3, and the constant waiting period T becomes 1.5, 2, 2.5, 3, respectively. The wild Aedes populations with strong Allee effect are shown in the left figure of Figure 9. Numerical simulations show that the length of the constant waiting period affects how quickly mosquito populations are suppressed. When the constant waiting period is T=1.5, it takes 12 days to suppress Aedes populations. When the constant waiting period is T=2, it takes 15 days. When the constant waiting period is T=3, it is hard to suppress wild Aedes populations. The wild Aedes population with weak Allee is shown in the right figure of Figure 9. When the constant waiting period is T=1.5, it takes 18 days to suppress Aedes populations. When the constant waiting period is T=2, it takes 24 days. When the constant waiting period is T=3, it takes 60 days.
The SIT is used to reduce or eradicate wild mosquitoes that transmit mosquito-borne diseases. Nevertheless, understanding and evaluating the impact of releasing sterile mosquitoes into the field is highly challenging due to the intricate dynamics between wild and sterile mosquitoes. In order to gain a deeper understanding of these complex interaction dynamics and to offer valuable guidance for the development of effective release strategies for sterile mosquitoes, we have taken into account the Allee effect and competition between Aedes aegypti and Anopheles mosquitoes.
We investigated the existence and stability of all potential equilibria within the system (2.1). Additionally, we established two specific threshold release values for the system (2.1), which led to variations in the number of equilibria depending on whether the release parameter was above, equal to, or below one of these thresholds. We further showed that for system (2.1), if the smaller threshold was exceeded, all solutions approach globally boundary equilibrium where the wild Aedes mosquito component was equal to zero. On the other hand, if the smaller threshold was not reached and the boundary equilibrium was still locally asymptotically stable, then there were two positive equilibria, one of which was a saddle point and the other was an asymptotically stable node. We also studied the existence and stability of all potential equilibria in system (3.1). In addition, we defined seven specific thresholds for the system (3.1) that led to changes in the number of equilibria and stability of equilibria.
For systems (4.1) and (4.2), we considered a model with impulsive releases of sterile mosquitoes, i.e., c sterile mosquitoes released at intervals T, and gave upper and lower bounds on the number of sterile mosquito populations. The relationship between different releases and the number of days required to suppress the population was also shown through numerical simulations. When the wild Aedes mosquito population approaches zero, the number of sterile mosquitoes is positive, meaning that even if wild mosquitoes become extinct, there are still sterile mosquitoes in system. This is not surprising, as sterile mosquitoes are constantly being released regardless of whether the wild mosquito population is high or low. From an economic point of view, this is clearly not the best strategy. It is hoped that better release methods will be available in the future to minimize economic losses.
While these findings seem exciting and promising, there is still more to be done with our research. We provide for systems (2.1) and (3.1) analyses of the interaction dynamics of wild and sterile mosquitoes in the specific case where wild and released Aedes aegypti have the same mortality rate. However, if their mortality rates differ, the dynamics could be very different and this needs to be explored. All these tasks are being carried out or planned in our other projects.
The authors declare they have not used Artificial Intelligence (AI) tools in the creation of this article.
The authors thank the two anonymous reviewers for their careful reading and valuable comments and suggestions. Meanwhile, this work is supported by the National Natural Science Foundation of China (12361101).
The authors declare there is no conflict of interest.
[1] |
Nader E, Skinner S, Romana M, et al. (2019) Blood rheology: key parameters, impact on blood flow, role in sickle cell disease and effects of exercise. Front Physiol 10: 1329. https://doi.org/10.3389/fphys.2019.01329 ![]() |
[2] | Savov Y, Antonova N, Zvetkova E, et al. (2006) Whole blood viscosity and erythrocyte hematometric indices in chronic heroin addicts. Clin Hemorheol Micro 35: 129-133. |
[3] | Pop GAM, Duncker DJ, Gardien M, et al. (2002) The clinical significance of whole blood viscosity in (cardio)vascular medicine. Neth Heart J 10: 512-516. |
[4] |
Antonova N (2012) On some mathematical models in hemorheology. Biotechnol Biotec Eq 26: 3286-3291. https://doi.org/10.5504/BBEQ.2012.0069 ![]() |
[5] |
Reinhart WH, Piety NZ, Goede JS, et al. (2015) Effect of osmolality on erythrocyte rheology and perfusion of an artificial microvascular network. Microvasc Res 98: 102-107. https://doi.org/10.1016/j.mvr.2015.01.010 ![]() |
[6] |
Cho YI, Kensey KR (1991) Effects of the non-Newtonian viscosity of blood on flows in a diseased arterial vessel. Part 1: steady flows. Biorheology 28: 241-262. https://doi.org/10.3233/bir-1991-283-415 ![]() |
[7] |
Connes P, Alexy T, Detterich J, et al. (2016) The role of blood rheology in sickle cell disease. Blood Rev 30: 111-118. https://doi.org/10.1016/j.blre.2015.08.005 ![]() |
[8] |
Hund SJ, Kameneva MV, Antaki JF (2017) A quasi-mechanistic mathematical representation for blood viscosity. Fluids 2: 10. https://doi.org/10.3390/fluids2010010 ![]() |
[9] |
Cho YI, Mooney MP, Cho DJ (2008) Hemorheological disorders in diabetes mellitus. J Diabetes Sci Technol 2: 1130-1138. https://doi.org/10.1177/193229680800200622 ![]() |
[10] |
Stegenga ME, van der Crabben SN, Levi M, et al. (2006) Hyperglycemia stimulates coagulation, whereas hyperinsulinemia impairs fibrinolysis in healthy humans. Diabetes 55: 1807-1812. https://doi.org/10.2337/db05-1543 ![]() |
[11] |
Sun JH, Han KQ, Xu M, et al. (2022) Blood viscosity in subjects with type 2 diabetes Mellitus: roles of hyperglycemia and elevated plasma fibrinogen. Front Physiol 13: 827428. https://doi.org/10.3389/fphys.2022.827428 ![]() |
[12] |
Li XL, Weber NC, Cohn DM, et al. (2021) Effects of hyperglycemia and diabetes Mellitus on coagulation and hemostasis. J Clin Med 10: 2419. https://doi.org/10.3390/jcm10112419 ![]() |
[13] | Arsana PM, Firani NK, Fatonah S, et al. (2022) Detection of hemostasis abnormalities in type 2 diabetes Mellitus using thromboelastography. J ASEAN Fed Endocr Soc 10: 2419. 37: 42-48. https://doi.org/10.15605/jafes.037.02.12 |
[14] | Le Dévéhat C, Vimeux M, Khodabandehlou T (2004) Blood rheology in patients with diabetes mellitus. Clin Hemorheol Microcirc 30: 297-300. |
[15] |
Riccio A, Cefalo CMA, Mazzanti C, et al. (2023) Whole blood viscosity is associated with reduced myocardial mechano-energetic efficiency in nondiabetic individuals. Eur J Clin Invest 54: e14127. https://doi.org/10.1111/eci.14127 ![]() |
[16] |
Wi MJ, Kim YM, Kim CH, et al. (2023) Effectiveness and safety of fufang danshen dripping pill (cardiotonic pill) on blood viscosity and hemorheological factors for cardiovascular event prevention in patients with type 2 diabetes Mellitus: systematic review and meta-analysis. Medicina 59: 1730. https://doi.org/10.3390/medicina59101730 ![]() |
[17] | Wang YX NCD statistics 2017-2020: risk and management of hyperglycemia, hyperlipidemia, and obesity-induced hypertension. in Third International Conference on Biological Engineering and Medical Science (2024). https://doi.org/10.1117/12.3013165 |
[18] |
Ranucci M, Ranucci M, Baryshnikova E (2018) An ex-vivo model of shear-rate-based activation of blood coagulation. Blood Coagul Fibrinolysis 29: 172-177. https://doi.org/10.1097/mbc.0000000000000688 ![]() |
[19] |
Ranucci M, Laddomada T, Ranucci M, et al. (2014) Blood viscosity during coagulation at different shear rates. Physiol Rep 2: e12065. https://doi.org/10.14814/phy2.12065 ![]() |
[20] |
Evans PA, Hawkins K, Lawrence M, et al. (2008) Rheometry and associated techniques for blood coagulation studies. Med Eng Phys 30: 671-679. https://doi.org/10.1016/j.medengphy.2007.08.005 ![]() |
[21] | Bodnár T, Sequeira A, Prosi M (2011) On the shear-thinning and viscoelastic effects of blood flow under various flow rates. Appl Math Comput 217: 5055-5067. https://doi.org/10.1016/j.amc.2010.07.054 |
[22] |
Antonova N, Tsiberkin K, Podtaev S, et al. (2016) Comparative study between microvascular tone regulation and rheological properties of blood in patients with type 2 diabetes mellitus. Clin Hemorheol Microcirc 64: 837-844. https://doi.org/10.3233/ch-168000 ![]() |
[23] |
Kannojiya V, Das AK, Das PK (2020) Simulation of blood as fluid: A review from rheological aspects. IEEE Rev Biomed Eng 14: 327-341. https://doi.org/10.1109/rbme.2020.3011182 ![]() |
[24] |
Wajihah SA, Sankar DS (2023) A review on non-Newtonian fluid models for multi-layered blood rheology in constricted arteries. Arch Appl Mech 93: 1771-1796. https://doi.org/10.1007/s00419-023-02368-6 ![]() |
[25] |
Rimmer T, Fleming J, Kohner EM (1990) Hypoxic viscosity and diabetic retinopathy. Br J Ophthalmol 74: 400-404. https://doi.org/10.1136/bjo.74.7.400 ![]() |
[26] |
Merimee TJ (1990) Diabetic retinopathy: a synthesis of perspectives. N Engl J Med 322: 978-983. https://doi.org/10.1056/NEJM199004053221406 ![]() |
[27] |
Baskurt OK, Meiselman HJ (2003) Blood rheology and hemodynamics. Semin Thromb Hemost 29: 435-450. https://doi.org/10.1055/s-2003-44551 ![]() |
[28] |
Irace C, Carallo C, Scavelli F, et al. (2014) Blood viscosity in subjects with normoglycemia and prediabetes. Diabetes Care 37: 488-492. https://doi.org/10.2337/dc13-1374 ![]() |
[29] |
Behbahani M, Behr M, Hormes M, et al. (2009) A review of computational fluid dynamics analysis of blood pumps. Eur J Appl Math 20: 363-397. https://doi.org/10.1017/S0956792509007839 ![]() |
[30] | Hussain A, Puniyani R, Kar S (1994) Quantification of blood viscosity using power law model in cerebrovascular accidents and high risk controls. Clin Hemorheol Micro 14: 685-696. https://doi.org/10.3233/CH-1994-14507 |
[31] | Komatsu R, Tsushima N, Matsuyama T (1997) Effects of glucagon administration on microcirculation and blood rheology. Clin Hemorheol Micro 17: 271-277. |
[32] | Ercan M, Konukoğlu D, Erdem T, et al. (2002) The effects of cholesterol levels on hemorheological parameters in diabetic patients. Clin Hemorheol Micro 26: 257-263. |
[33] |
Kim HK, Kim JE, Park SH, et al. (2014) High coagulation factor levels and low protein C levels contribute to enhanced thrombin generation in patients with diabetes who do not have macrovascular complications. J Diabetes Complications 28: 365-369. https://doi.org/10.1016/j.jdiacomp.2014.01.006 ![]() |
[34] |
Weingarz L, Schwonberg J, Schindewolf M, et al. (2013) Prevalence of thrombophilia according to age at the first manifestation of venous thromboembolism: results from the MAISTHRO registry. Br J Haematol 163: 655-665. https://doi.org/10.1111/bjh.12575 ![]() |
[35] | Marcinkowska-Gapińska A, Kowal P (2009) Comparative analysis of chosen hemorheological methods in a group of stroke patients. Clin Hemorheol Micro 41: 27-33. https://doi.org/10.3233/ch-2009-1151 |