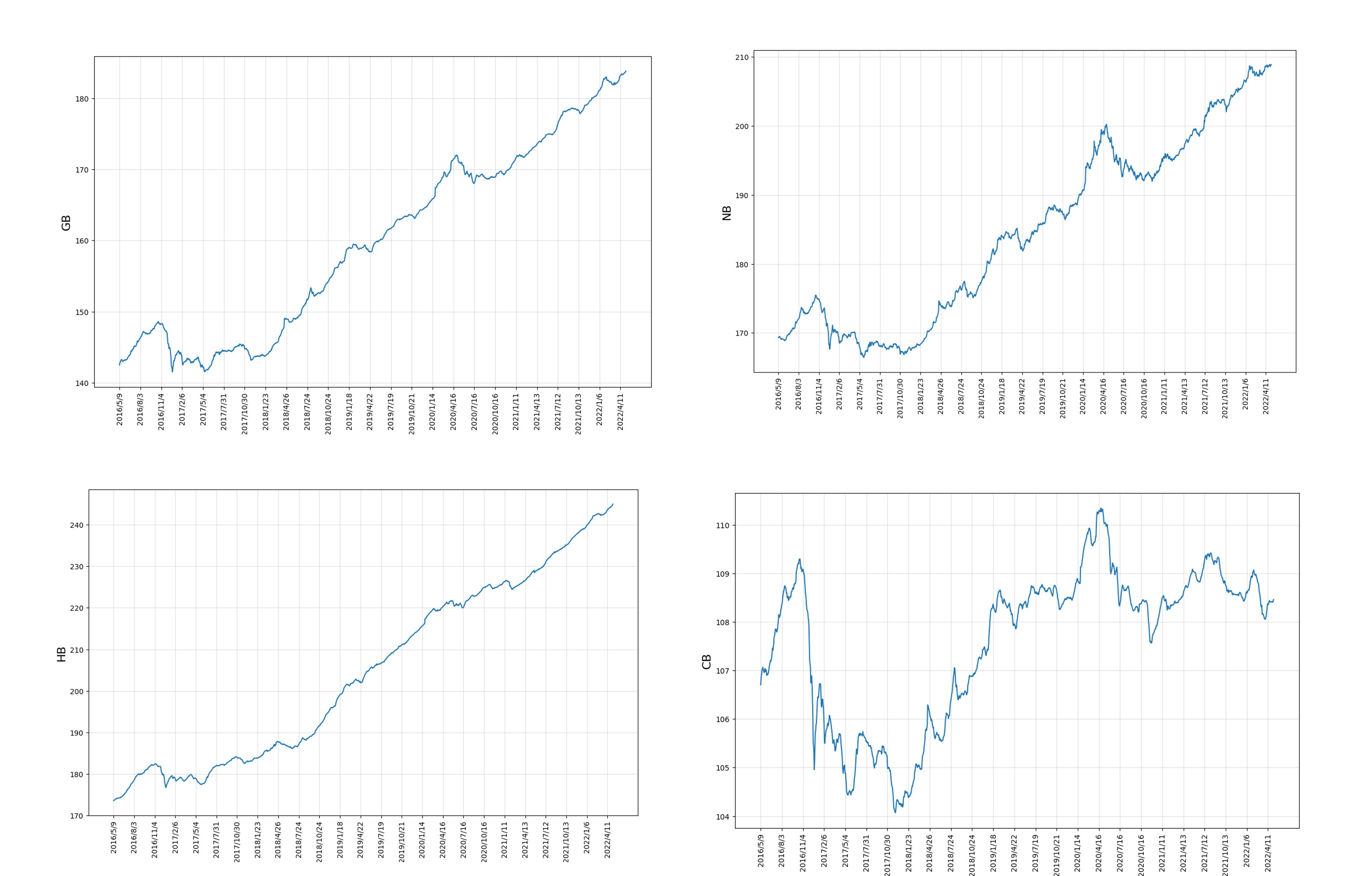
Citation: Dahye Kim, Kyung Dong Lee, Ki Choon Choi. Role of LAB in silage fermentation: Effect on nutritional quality and organic acid production—An overview[J]. AIMS Agriculture and Food, 2021, 6(1): 216-234. doi: 10.3934/agrfood.2021014
[1] | Samuel Asante Gyamerah, Bright Emmanuel Owusu, Ellis Kofi Akwaa-Sekyi . Modelling the mean and volatility spillover between green bond market and renewable energy stock market. Green Finance, 2022, 4(3): 310-328. doi: 10.3934/GF.2022015 |
[2] | Aikaterini (Katerina) Tsoukala, Georgios Tsiotas . Assessing green bond risk: an empirical investigation. Green Finance, 2021, 3(2): 222-252. doi: 10.3934/GF.2021012 |
[3] | Vilija Aleknevičienė, Raimonda Vilutytė . Short-term stock market reaction to the announcement of green bond issue: evidence from Nordic countries. Green Finance, 2024, 6(4): 728-744. doi: 10.3934/GF.2024028 |
[4] | Samuel Asante Gyamerah, Clement Asare . A critical review of the impact of uncertainties on green bonds. Green Finance, 2024, 6(1): 78-91. doi: 10.3934/GF.2024004 |
[5] | Tao Lin, Mingyue Du, Siyu Ren . How do green bonds affect green technology innovation? Firm evidence from China. Green Finance, 2022, 4(4): 492-511. doi: 10.3934/GF.2022024 |
[6] | Toyo Kawabata . Private governance schemes for green bond standard: influence on public authorities’ policy making. Green Finance, 2020, 2(1): 35-54. doi: 10.3934/GF.2020003 |
[7] | Vitor Miguel Ribeiro . Green bond market boom: did environmental, social and governance criteria play a role in reducing health-related uncertainty?. Green Finance, 2023, 5(1): 18-67. doi: 10.3934/GF.2023002 |
[8] | Kentaka Aruga, Md. Monirul Islam . Assessing investor preferences for environmental and sustainability bonds in Japan: A discrete choice experiment approach. Green Finance, 2025, 7(2): 200-222. doi: 10.3934/GF.2025008 |
[9] | Shahinur Rahman, Iqbal Hossain Moral, Mehedi Hassan, Gazi Shakhawat Hossain, Rumana Perveen . A systematic review of green finance in the banking industry: perspectives from a developing country. Green Finance, 2022, 4(3): 347-363. doi: 10.3934/GF.2022017 |
[10] | Fernando Palao, Ángel Pardo . Carbon and safe-haven flows. Green Finance, 2022, 4(4): 474-491. doi: 10.3934/GF.2022023 |
Environmental governance is challenged by the growing problem of global pollution. Policymakers and researchers have proposed various solutions in response. Among them, the concept of green finance is innovative and focuses on environmental interests to achieve sustainable development, and evaluates its activities' effectiveness through environmental benefits and resource utilization. Green bond is a financing tool that was vigorously developed and agrees with the concept of "carbon peak" advocated by the contemporary international community. Thus, they have received extensive attention from investors and institutions.
In 2007, the European Investment Bank (EIB) issued climate-conscious bonds, which is the world's first green bond. The United Nations Framework Convention on Climate Change established the concept of climate financing. The G20 and the International Monetary Fund officially confirmed that it is significant to progress the green bond market. The International Energy Agency also recommended the green bonds as one of the solutions to the problem of climate change financing (Chen & Zhang, 2022). Since 2013, investors have been increasingly concerned with environmental and climate issues, and green bonds have begun to develop leaps and bounds. There are more and more types of bonds, and the issuers are gradually diversified and the scope is gradually expanding.
Injurious for us is that human beings pollute the environment to evolve economy. It is green finance that is the most significant approach to facilitate the harmonious progression of economy and ecology (Bhatnagar et al., 2022). High-quality development is the prominent assignment to construct a comprehensive modern socialist country. As a part of high-quality development, the degree of green economic advancement has become an important indicator. The evolution of green finance can promote green development and is a key measure to realize the common prosperity. The first time a green bond was issued was in July 2015 in China. Since then, the market scale has gradually expanded. By the end of 2022, the stock of green bonds in China was about 3 trillion yuan, which has become the world's second largest green bond market. The green bonds have grown into a major factor in helping China's stable economic development and promoting environmental protection (Chen & Zhang, 2023; Wang & Tian, 2023).
At present, the demand for green capital is strong, with a severely deficiency of supply; thus, there exists a substantial financial gap. Therefore, it is crucial for China to develop green financing tools. Green bond is one of the most innovative and influential debt financing instruments, and has the dual characteristics of "green" and "bond". At the micro level, issuing the green bonds can expand financing channels for green projects and enterprises, and fill investment gaps (Wang & Xu, 2016). At the macro level, the continuous improvement of China's green bond market will contribute to the developing countries' sustainable evolution (Wang & Zeng, 2016).
The deepening reform of China's financial market system and the increasingly relaxed supervision of financial industry have led to more frequent interactions between financial sub-markets. However, high liquidity between financial markets also enhances risk. Once a sub-market has financial risks, the risk will spread rapidly to trigger a financial crisis. As a new type of global financial instrument, green bonds are bound to experience more diversified development and give birth to a variety of innovative financial products. Although it is an emerging market, it is becoming more and more closely related to other financial markets, which means that green bond prices and other financial asset prices are closely related to each other and influence each other. In the process of integration with China's traditional financial market, it is likely to accumulate certain risks and spillover risks.
In the current economic environment, China's economic development should not only pursue speed but also quality. Therefore, it is of great profound significance to promote green and sustainable economic transformation to solve China's economic and environmental problems. As China's financial market matures, the risks China faces are gradually emerging, which also tests the entire financial system. Preventing and controlling systemic risks has become an important topic in our economic activities. Therefore, exploring the spillover effect between China's green bond market (CGB) and traditional bond market for effective prevention and control of systemic risks is significant. We will focus on the traditional bond market and explore the interaction between the CGB and the traditional bond market. Studying mean, volatility and extreme risk spillover effects among financial markets has important practical value for promoting the CGB's progression, and provides a certain theoretical basis for issuers, investors, policy makers and regulators to help them adopt more effective policy measures.
There are mostly two aspects to discuss green bond (GB) in the light of available literature. On the one hand, scholars have different views on the pricing differences between GB and ordinary bonds, which has discounts compared to regular bonds. On the other hand, for the economic impact of GB, most scholars are devoted to explore the stock market's reaction to the corporate green bond issuance (Lin et al., 2022).
Compared with traditional bonds, GB is used to finance specific investments which fail to pose additional risks to investors. Bonds tend to offer higher profitability, liquidity, and stability than bank to satisfy the most diversified investors (Ezuma et al., 2022). GB is relatively convenient in financing. For corporate investors, financing advantages are greater (Gianfrate, 2019), which help enhance corporate value. In addition, equity liquidity drives a significant enhancement in the yield of GB (Zhang et al., 2020), and investment performance advantage of GB diminishes over time (Kanamura, 2020).
Some scholars have taken the spread among the GB and traditional bonds, that is green bond premium, as the subject of study when discussing the pricing factors of GB. Partridge found that by 2018, the green premium in the U.S. secondary municipal bond market reached 50 basis points (Partridge & Medda, 2020). Nanayakkara deemed that GB in global capital markets trade at a premium of 63 basis points (Colombage & Nanayakkara, 2020).
Another scholar explores the reasons of "green premium". Through systematic literature review, MacAskill et al. (2021) found that the green premium variability for primary market is significant. Zerbib (2019) argued that GB is provided with a negative premium. The GB without third-party certification not only has no premium, but also has the risk of "greenwashing", and the credit spreads are significantly higher than ordinary bonds by 21 percentage points (Jiang & Fan, 2020; Qi & Liu, 2021). Social networks can submit useful information, and there are significant differences in the condition of investor sentiment on CB with different risk characteristics (Li et al., 2018). Investors' attention has different effects on the return and volatility of GB, and this relationship is time-varying (Pham & Huynh, 2020). The characteristics of GB will also affect the premium of GB. GB has certain advantages in issuing pricing, and the rating status of bond issuers and the government's invisible credit support will have different effects on GB spreads (Chen et al., 2021; Dou & Zhang, 2019; Gao & Ji, 2018).
In the study of the mean spillover effect, the most frequently used model by scholars is the VAR model, and on this basis, SVAR, MVAR and other models have been derived. Huynh (2021) studied the spillover risk effect of cryptocurrency market using VAR-SVAR and Granger causality. Gao & Li (2021) used the VAR model to predict error variance decomposition, calculated the DY spillover index to analyze the mean spillover effect between the GB and the traditional financial market. Liu & Wang (2018) used the MSVAR model to analyze the mean spillover effect of soybean futures between China and the United States. He (2017) verified that there is a mean spillover effect between the main board, the New Third Board and the GEM in the stock market through the VAR model. Naresh et al. (2017) used the VAR model to test the spillover between stock and commodity future markets.
The research on volatility spillover effects is becoming more and more mature. The research perspectives of scholars are diversified and there are two descriptions: One is to explore the volatility spillover effects within a financial market; and the other is to discuss the mutual spillover effect among different financial markets. Regarding the research methods, GARCH family models have been widely used because of their good modeling effect on volatility.
Zhou & Zhou (2006) used the multivariate GARCH model to analyze the volatility spillover effects of domestic and international crude oil markets, which proves that the direction of information transmission in the oil market is from the international market to the domestic market. Zhong et al. (2004) confirmed that there is an ability for the stock index future market to discover prices based on the modified EGARCH model. Yavas & Dedi (2016) studied the volatility spillover effects of German, British, Chinese, Russian and Turkish stock markets using the MARMA, GARCH, GARCH mean and EGARCH methods. Hu & Ma (2011) established the BEKK-MGARCH model to analyze the volatility spillover effect between the stock market and the bond market in different periods.
The CoVaR model is the main research method of extreme risk spillover effect, while with the widespread application of Copula, the combination of Copula and GARCH model, provides a new way of calculating CoVaR. Li & Fan (2011) measured the systemic risk premium of commercial banks in China using the CoVaR and quantile regression methods. The results showed that the systemic risk premium of state-owned banks is higher than that of joint-stock banks. When solving the problems related to systemic financial risk, Wairagu (2011) used the Copula function to quantitatively analyze the marginal contribution made by a particular financial institution to systemic financial risk, and also found that the correlation coefficient would affect the extreme risk spillover. With the wide application of Copula, its combination with the GARCH model provides a new idea for calculating CoVaR. Bai (2021) used the GARCH-DCC-Copula-CoVaR model to discuss the dynamic spillover effect of commercial banks on systemic risk of banks and their interdependence. Huang (2021) used the time-varying t-Copula model to study dynamic correlation and risk spillover heterogeneity between international oil prices and stock indexes of crude oil-related industries, with calculating %CoVaR to reflect the risk spillover intensity of international crude oil prices on Chinese oil-related industries. Some scholars have begun to calculate CoVaR by quantile regression. Zhu (2013) made a preliminary exploration of "Copula + Quantile". Kielmann & Manner (2022) combined the multivariate dynamic Copula model, quantile regression model based on D-vine and GAS Copula model to investigate the spillover effects between BRIC stock returns and different types of oil price shocks.
Currently, scholars' research mainly focuses on the spillover effect between GB and traditional financial market. Qin et al. (2019) constructed the MVMQ-CAViaR model to empirically analyze the tail risk spillover between low-carbon industry's stock market and the CGB, which found that the occurrence of labeled green bonds has changed dynamic process of one market shock affecting the tail risk of another market. There is a significant spillover effect between the assets of the GB and the traditional bond market, which leads to a significant hedging strategy, and the spillover effect is affected by the green labeling criteria (Tsoukala & Tsiotas, 2021). The volatility spillover effect between the two markets is bidirectional, showing a strong dynamic correlation, and the dynamic correlation coefficient fluctuates around the mean value (Reboredo et al., 2019; Wang, 2021).
Reboredo (2018) found that the GB is related to the corporate bond (CB) and the conventional bond (NB), and this relationship is time-varying. Moreover, as for the CB and NB, there is a significant influence of spillover effect for the GB. Through empirical analysis of the information transmission between GB and other assets, Su et al. (2022) found that there is a lack of preferences for green among the bond market's investors in China. It is the return and volatility of NB and CB that give impetus to GB; and there are different net spillover effects on different frequency scales for GB. Mensi et al. (2022) found that the connectivity between GB and the S&P 500 index is strengthened when a magnitude crash breaks out, and the spillover effect of government bonds were relatively less affected. The green energy and resource market has devoted significantly to the volatility spillover effect. Naeem et al. (2022) used the quantile connectivity method to conduct an empirical study and they found that the time-varying risk spillover is higher in the period of extreme high volatility, and there is a violent bidirectional risk spillover effect between GB and all assets except energy.
Overall, domestic and foreign scholars' research on GB mainly concentrate on theoretical research, and empirical analysis is relatively lacking, and the empirical research mostly focuses on the impact factors of GB pricing and GB spreads. From a macro perspective, there are fewer studies on the correlation between the GB and other financial markets, and they are in the process of continuous development and improvement. However, due to the late start of GB in China, there is little research on the law of development of China's bond market, especially on the degree of integration and risk linkage between it and traditional financial markets. Furthermore, in recent years, with the shortage of resources and the deepening environmental pollution, ecological problems have gradually become prominent. Developing green finance is an important way to build ecological civilization, indicating that China has elevated the concept of green financial development to a strategic level. At present, the majority of scholars have devoted to discuss mean spillover and volatility spillover, and the research on extreme risk spillover is shallow. In view of this, we establish VAR, DCC-GARCH and Copula-CoVaR models to study the China's spillover effect between the CGB and the traditional bond market from three perspectives: mean spillover, volatility spillover and extreme risk spillover, in order to provide new references and data support for issuers, investors, policy makers and regulators to take more effective policy measures.
At present, there are three principal indexes in CGB: China Bond China Climate-Aligned Bond Index, China Bond CIB Green Bond Index and China Bond Green Bond Index. The three indexes belong to the China Bond Wealth Index, and the green bonds listed on the interbank bond market, the Shanghai Stock Exchange and the Shenzhen Stock Exchange as samples. However, the sample size is diverse, and the volatility of the yield is also slightly different. China Bond Green Bond Index is the first batch of green bond index in China. It is compiled by China's official settlement agency. It is the green bond index with the longest history and the most influence in China, which can effectively represent the development of CGB. Moreover, it takes into account the mutual matching of different bond markets in the sample length. We select the GBI to represent the green bond market. For the traditional bond market, we regard the high-yield enterprise bond, corporate bond and conventional bond market as the proxy variables of the traditional bond market. Additionally, the China Bond High Yield Enterprise Bond Wealth Total Index (HYCB), the China Bond Treasury Bond Index (TBAI) and the China Bond Corporate Bond Index (EBAI) are selected as the proxy variables for the enterprise bond (HB), NB, and CB, respectively. The data in this article is obtained from the Wind database, which adopts index daily closing price, and the time interval is from 2016/5/9 to 2022/5/6. There are 1457 valid observation data for each market after excluding legal holidays and non-public trading days.
After obtaining the daily closing prices of the four market price indexes, we utilize the logarithmic difference operation method to calculate the four markets' logarithmic returns, while, r_gb、r_hb、r_nb and r_cb are used to represent the yields of green bond market, enterprise bond market, conventional bond market and corporate bond market, respectively. The results are shown in Table 1.
variable | Mean | Maximum | Minimum | Std.Dev. | Kurtosis | Skewness | J-Btest |
r_gb | 0.0174 | 0.8008 | −0.8183 | 0.0803 | 23.1679 | 0.2125 | 32378 |
r_hb | 0.0236 | 0.5562 | −0.5527 | 0.0621 | 13.2491 | 0.2847 | 10773 |
r_nb | 0.0144 | 0.3909 | −0.5585 | 0.0550 | 9.6374 | 0.6196 | 5609.5 |
r_cb | 0.0011 | 1.0538 | −0.9505 | 0.1256 | 15.8382 | −1.1160 | 15442 |
In the sample interval, the mean values of the four markets are positive, and the earnings performance of the HB is better than the other three markets. There is the smallest standard deviation for NB, which declares that the income fluctuation is the smallest with the strongest security. It can be found that the yields of GB, HB and NB are all right-skewed by examining the skewness index, while the CB has a left-skewed characteristic. Among them, the skewness of the CB is the highest and CGB is the lowest in deviation. Based on the kurtosis index, it can be found that there is a general phenomenon of leptokurtosis in the four markets. The J-B test of each market shows that the yield series of each market do not satisfy the normal distribution and have general characteristics of most financial time series.
The results in Table 2 indicates that the yield series of the four markets are all stationary on the basis of ADF statistics of each variable.
variable | ADF test | %1 threshold | %5 threshold | %10 threshold | Stationary |
r_gb | −8.6346 | −2.5659 | −1.9410 | −1.6166 | Stationary |
r_hb | −8.5242 | −2.5659 | −1.9410 | −1.6166 | Stationary |
r_nb | −9.8269 | −3.4328 | −2.8625 | −2.5673 | Stationary |
r_cb | −9, 1778 | −2.5659 | −1.9410 | −1.6166 | Stationary |
According to the literature review, it is clear that the VAR model, VAR derivative model and Granger causality test are commonly used by scholars in the study of mean spillover effects. The GARCH family models have been widely utilized in the analysis of volatility spillover effects because of their good modeling of volatility. Nowadays, they mainly focus on multivariate GARCH models. When measuring extreme risks, CoVaR not only measures the risks faced by individual markets itself, but also takes into account the market contagion effect. The Copula function makes the establishment of marginal distribution very flexible. Therefore, the VAR model is used to analyze the mean spillover effect, the DCC-GARCH model is used to analyze the volatility spillover effect and the Copula-CoVaR model is used to discuss the extreme risk spillover effect.
We study the mean spillover effect between the two markets by constructing a binary VAR(P) model. The formula is as follows:
{y1t=β10+β11y1,t−1+...+β1py1,t−p+γ11y2,t−1+...+γ1py2,t−p+ε1ty2t=β20+β21y1,t−1+...+β2py1,t−p+γ21y2,t−1+...+γ2py2,t−p+ε2t | (1) |
where p is the optimal lag order of the model, y1t, y2t are equation's two explained variables, the lag p values of y1t and y2t are the explanatory variables, y1, t-p and y2, t-p represent the lagged p-periods of y1t and y2t, while ε1t, ε2t are the perturbation terms, and β1i, γ2i (i = 1, 2, …, p) reflect the extent to which y1t and y2t are affected by their own prior period. γ1i (i = 1, 2, …, p) measures the influence of the lag term of y2t on y1t an, β2i (i = 1, 2, …, p) measures the influence of the lag term of y1t on y2t.
A VAR model with yt and xt as variables can be constructed for the reason that this section intends to verify whether there is a causal relationship between yt and xt. The specific equations are as follows:
yt=p∑i=1αiyt−i+p∑i=1βixt−i+ε1t | (2) |
Then, the null hypothesis that xt is not causal for yt is: H0: β1 = β2 = … = βp = 0
The DCC-GARCH model considers that the correlation coefficient between series is a dynamic change rather than a fixed constant, which can better reflect the fluctuation influence among multiple series. Based on this advantage, we use the DCC-GARCH model to study the volatility spillover effect, which helps to better test the dynamic correlation between variables. Assuming that the time series yt presents a multivariate normal distribution structure with the mean of this multivariate normal distribution as μt, and the conditional covariance matrix as Ht, then the DCC-GARCH (1, 1) model is expressed as follows:
yt=μt+εtεt|Ft−1∼N(0,Ht)ε=H12tμtμt∼N(0,I)Ht=DtRtDt | (3) |
where, Ft-1 denotes the set of all information before t − 1. μt, εt and ut are N × 1 dimensional vectors, which represent the conditional mean, error term and normalized error term, respectively. In addition, Rt, Ht and Dt = diag (h11, t1/2, …, hNN, t1/2) are all N × N diagonal arrays, Rt represents the dynamic conditional correlation coefficient matrix, and Ht is the time-varying conditional variance-covariance matrix.
First, Dt is measured by calculating the time-varying conditional standard deviation of the univariate GARCH model. Then, the dynamic conditional correlation coefficient is calculated:
hii,t=ω+αε2i,t−1+βhii,t−1Rt=diag(q−1/122ii,t,...,q−1/122NN,t)Qtdiag(q−1/122ii,t,...,q−1/122NN,t)Qt=(qij,t)=(1−α−β)¯Q+αμt−1′+βQt−1 | (4) |
where Qt and Q are N × N dimensional positive definite matrices, which represent the variance-covariance matrices of conditional and unconditional standardized residuals, respectively. A and β represent non-negative shock and persistence parameters. Qt and Rt will change over time as long as α > 0, β > 0 and α + β < 1 are satisfied, otherwise, the model converges to the CCC-GARCH model. In the DCC-GARCH (1, 1) model, the dynamic correlation coefficient is:
ρ12,t=q12,t√q11,tq22,t | (5) |
What seems extraordinary difficult is to model the joint distribution when the random variables with different marginal distributions are not independent of each other. Under this circumstance, the Copula function is a remarkably outstanding instrument to model its correlation between multiple random variables with known marginal distributions. The Copula function is widely used in the study of complex dynamic dependence between markets, and it is also mainly used to describe the correlation between variables. There are two common Copula functions: The elliptic copula function and Archimedean copula function. We focus on the binary Copula function.
(1) The binary normal Copula function
For the normal Copula function, the marginal distribution in the Copula function C(u, v) is a normal distribution. The expression of C(u, v) is as follows :
C(u,υ,ρ)=∫ϕ−1(u)−∞∫ϕ−1(υ)−∞12π√1−ρ2exp(2ρrs−r2−s21(1−ρ2))drds | (6) |
where ϕ-1(·) is the inverse function of the standard normal distribution function, and the parameter ρ denotes the correlation between ϕ-1(u) and ϕ-1(v). The normal Copula function has tail symmetry.
(2) The binary t-Copula function
What is notable is that the parameters to be estimated in the t-Copula function have degrees of freedom κ in addition to the correlation coefficient ρ. The expression of the t-Copula function is as follows:
C(u,υ,ρ,κ)=∫T−1κ(u)−∞∫T−1κ(υ)−∞12π√1−ρ2exp(1+r2+s2−2ρrs2κ(1−ρ2))−(κ+2)2drds | (7) |
where T (·) represents the t distribution function.
The VaR method is based on the quartile principle to represent the size of the risk. The mathematical expression of VaR is as follows:
P(Δυ⩽VaR)=α | (8) |
where ∆v represents the value loss of a financial asset over a specific period, α is the confidence level, and VaR is the upper bound of possible loss.
Tsagkanos et al. (2022) used VaR based copulas to analyze the asymmetric risk spillover between GB and commodities. However, the deficiency of VaR method is that it only measures the risk level of a single market, and fails to take into account the common contagion effect between markets, which considerably reduces the risk assessment level of VaR method. Scholars proposed a new risk measurement method CoVaR based on VaR, trying to capture the risk spillover effects between financial institutions, while Girardi et al. (2013) adjusted the conditional events in CoVaR. They believe that more serious catastrophic events occur at the tail of the loss distribution compared to overly optimistic scenarios. The specific expression is as follows:
P(Xi⩽CoVaRi|jβ,t|Xj⩽VaRjα,t)=β | (9) |
CoVaRi|jβ,t is the conditional risk of market i with respect to market j at moment t under the significant level β, which can be decomposed into the unconditional risk of market i itself and the risk spillover △CoVaRi|jβ,t of market j to market i. Therefore, the downside risk spillover from market j to market i is expressed as follows:
ΔCoVaRi|jβ,t=ΔCoVaRi|jβ,t−VaRiα,t | (10) |
When the prices between markets are in different magnitudes, their VaR levels are also different, Therefore, further standardization is needed to obtain the risk spillover intensity from market j to market i:
%ΔCoVaRi|jβ,t=ΔCoVaRi|jβ,tVaRiα,t×100% | (11) |
Table 2 confirms the yields of the traditional bond market and CGB have passed the ADF test, which ensures the stability of the time series. Therefore, we can carry out Granger causality test on the three market combinations of NB & CGB, CB & CGB and HB & CGB.
From Table 3, it can be known that for HB and CB, there is a unidirectional causality between CGB and the two bond markets; there is a bidirectional causality between NB and CGB. The above conclusions are consistent with the results of VAR parameter estimation.
Null hypothesis | Lag order | χ2 | P value | conclusion |
r_hb does not Granger cause r_gb | 6 | 8.3197 | 0.3052 | Fail to reject |
r_gb does not Granger cause r_hb | 6 | 27.7403 | 0.0002 | Reject |
r_nb does not Granger cause r_gb | 5 | 10.5105 | 0.0327 | Reject |
r_gb does not Granger cause r_nb | 5 | 61.1701 | 0.0000 | Reject |
r_cb does not Granger cause r_gb | 6 | 2.6135 | 0.9562 | Fail to reject |
r_gb does not Granger cause r_cb | 6 | 11.0820 | 0.0305 | Reject |
In line with the AIC and SC information criteria, the six-order lag is selected to construct the VAR model between the HB and CGB. The estimated results of the model are shown in Table 4.
r_hb | r_gb | r_hb | r_gb | ||
r_hb(−1) | 1.0364** | −0.1179 | r_gb(−1) | −0.2639*** | 1.4445*** |
t | 29.3130 | −2.4860 | t | 9.9620 | 40.6270 |
r_hb(−2) | 0.0999 | 0.1796 | r_gb(−2) | −0.2544*** | −0.4434*** |
t | 1.8970 | 2.5420 | t | −6.0370 | −7.8390 |
r_hb(−3) | −0.0745 | −0.1345 | r_gb(−3) | 0.0101 | 0.1229 |
t | −1.4130 | −1.8990 | t | 0.2350 | 2.1310 |
r_hb(−4) | −0.0307 | 0.0109 | r_gb(−4) | −0.0477 | −0.0759 |
t | −0.5830 | 0.1550 | t | −1.1120 | −1.3190 |
r_hb(−5) | 0.1079 | 0.1048 | r_gb(−5) | 0.0073 | −0.0034 |
t | 2.0520 | 1.4850 | t | 0.1720 | −0.0600 |
r_hb(−6) | −0.1416*** | −0.0434 | r_gb(−6) | 0.0243 | −0.0439 |
t | −4.1210 | −0.9420 | t | 0.9010 | −1.2130 |
Note: ***, **, and * indicate statistically significant at the 1%, 5% and 10% level. |
The results of the VAR (6) model show that for autocorrelation, the yield of CGGB and HB have serial autocorrelation. In terms of mean spillover, CGB has an obvious negative explanatory effect on HB for two days. However, there is no obvious explanatory effect of HB on CGB. Therefore, there is a unidirectional negative mean spillover from CGB to the HB.
In line with the AIC and SC information criteria, the optimal lag order of the VAR model between NB and CGB is five-order.
The VAR (5) model's result illustrates that in terms of autocorrelation, there is autocorrelation between the yields of NB and CGB. For mean spillover, there is an asymmetric bidirectional mean spillover between NB and CGB. Conventional debt plays a negative influence on CGB, which is affected by a positive influence. Furthermore, CGB has a greater impact on NB numerically.
r_nb | r_gb | r_nb | r_gb | ||
r_nb(−1) | 1.2845** | 0.2228 | r_gb(−1) | −0.1611 | 1.1178*** |
t | 36.0980 | 11.0030 | t | −2.5790 | 31.4280 |
r_nb(−2) | −0.3195*** | −0.2212*** | r_gb(−2) | 0.2776** | −0.0423 |
t | −6.1680 | −7.5060 | t | 2.8760 | −0.7710 |
r_nb(−3) | 0.0897 | 0.0384 | r_gb(−3) | −0.1247 | −0.0143 |
t | 1.7000 | 1.2780 | t | −1.2840 | −0.2600 |
r_nb(−4) | −0.1010 | −0.0746 | r_gb(−4) | 0.1519 | 0.0272 |
t | −1.9360 | −2.5150 | t | 1.610 | 0.5070 |
r_nb(−5) | 0.0319 | 0.0318 | r_gb(−5) | −0.1312 | 0.0858** |
t | 0.8980 | 1.5720 | t | −2.3210 | −2.6670 |
Note: ***, **, and * indicate statistically significant at the 1%, 5% and 10% level. |
The six-order VAR model is established for the HB and CGB using AIC and SC criteria for comprehensive judgment. As far as autocorrelation, there is a significant autocorrelation and non-white noise process between the two markets. For mean spillover, CGGB has a unidirectional negative impact on CB.
r_cb | r_gb | r_cb | r_gb | ||
r_cb(−1) | 1.0364*** | −0.1179 | r_gb(−1) | 0 | 1.4445*** |
t | 29.3130 | −2.4860 | t | 9.9620 | 40.6270 |
r_cb(−2) | 0.0999 | 0.1796 | r_gb(−2) | −0.2544*** | −0.4434*** |
t | 1.8970 | 2.5420 | t | −6.0370 | −7.8390 |
r_cb(−3) | −0.0745 | −0.1345 | r_gb(−3) | 0.0101 | 0.1229 |
t | −1.4130 | −1.8990 | t | 0.2350 | 2.1310 |
r_cb(−4) | −0.0307 | 0.0109 | r_gb(−4) | −0.0477 | −0.0759 |
t | −0.5830 | 0.1550 | t | −1.1120 | −1.3190 |
r_cb(−5) | 0.1079 | 0.1048 | r_gb(−5) | 0.0073 | −0.0034 |
t | 2.0520 | 1.4850 | t | 0.1720 | −0.0600 |
r_cb(−6) | −0.1416*** | −0.0434 | r_gb(−6) | 0.0243 | −0.0439 |
t | −4.1210 | −0.9420 | t | 0.9010 | −1.2130 |
Note: ***, **, and * indicate statistically significant at the 1%, 5% and 10% level. |
After estimating the unknown parameters of the VAR model, we plot the results of residual cumulative sum curves to test the systematic stability of the model. The linear graphs are as follows:
The residual cumulative sum curves of the four market yields do not exceed the two red critical lines, indicating that there is a stability of the three sets of binary VAR models building r_gb with r_hb, r_nb and r_cb.
The GARCH model parameter estimation is based on the AIC and SC criteria to clarify the optimal lag order of each yield series, and then the GARCH (1, 1) model is established. From Table 7, the ARCH coefficients and GARCH coefficients of the four market yield series are statistically significant at 1% level and greater than 0. Moreover, α+β<1, which indicates that it satisfies the significance and stability conditions of the GARCH model, and proves that the modeling is robust and feasible. Furthermore, the sum of α and β is close to 1, which proves that the volatility of these four market yield series has strong aggregation and persistence.
Constant | coefficient of ARCH(α) | coefficient of GARCH(β) | |
r_gb | 0.0008*** (4.649) | 0.4123*** (7.324) | 0.5593*** (10.884) |
r_hb | 0.0011*** (4.316) | 0.1768*** (4.228) | 0.5542*** (6.123) |
r_nb | 0.0011*** (3.343) | 0.1862*** (4.987) | 0.7608*** (16.230) |
r_cb | 0.0004*** (7.071) | 0.4760*** (7.508) | 0.4095*** (7.390) |
Note: ***, **, and * indicate statistically significant at the 1%, 5% and 10% level. |
We construct a DCC model to obtain the dynamic correlation coefficients between the traditional bond market and CGB after the GARCH model. From Table 8, it can be found that DCC1 and DCC2 of the three market portfolios are statistically significant at a 1% level, and both greater than 0, and the sum is less than 1, which expresses that the significance and stability conditions of the DCC model are satisfied and proves that the modeling is robust and feasible. DCC1 between the three traditional bond markets and CGB are all in the range of 0.0008 to 0.0011 with little difference, which indicates that the volatility of the dynamic correlation coefficients between the three traditional bond markets and CGB is roughly equal. The DCC2 of the combination of NB yield and CGB yield is the largest, which declares the dynamic correlation coefficient between the two deviates from the normal correlation to a greater extent, showing a stronger trend in the graph.
DCC1 | DCC2 | DCC1+DCC2 | |
r_gb&r_hb | 0.0008*** (4.649) | 0.4123*** (7.324) | 0.5593*** (10.884) |
r_gb&r_nb | 0.0011*** (4.316) | 0.8768*** (4.228) | 0.8779*** (6.123) |
r_gb&r_cb | 0.0011*** (3.343) | 0.1862*** (4.987) | 0.1873*** (16.230) |
Note: ***, **, and * indicate statistically significant at the 1%, 5% and 10% level. |
We show the statistical characteristics of the dynamic correlation coefficients and plot the dynamic correlation coefficient diagrams between HB, NB, CB and CGB, respectively.
Minimum | Maximum | Mean | |
r_gb&r_hb | −0.0904 | 0.9861 | 0.6471 |
r_gb&r_nb | 0.4353 | 0.9112 | 0.6457 |
r_gb&r_cb | 0.1402 | 0.9924 | 0.7517 |
The range of dynamic correlation coefficient between the HB yield and CGB yield is between 0.4 and 0.8 from the Figure 3. Among the three sets of dynamic correlation, the value is small and most of the time positive. Therefore, the relationship between the two markets possesses a positive and weak volatility spillover effect.
The yield correlation coefficient between the two markets is between 0.5 and 0.8 from Figure 4, which indicates that the relationship between them possesses a strong positive correlation and volatility spillover effect. In addition, in the first half of 2018 and 2020, the correlation between them reached a peak. At this time, there is a high degree of synergy, a large degree of volatility spillover and a large risk of allocation.
The coefficient between them is between 0.6 and 0.9, indicating that their relationship possesses a stronger positive correlation and volatility spillover effect from Figure 5. In addition, the coefficient fluctuates greatly over time, which illustrates that the volatility spillover effect is relatively unstable.
In a nutshell, the average value of the dynamic correlation coefficient among NB and CGB is the largest, indicating that this correlation between the two markets is the strongest, while the value among HB, CB and CGB is smaller, indicating that the correlation is weaker.
In order to select the optimal distribution, this paper fits the four bond markets' yields from Gaussian, Student-t, Skew Student-t, Ged and Skew-Ged distributions according to the log-likelihood value and information criterion. The maximum likelihood value and information criterion of GARCH (1, 1) of each yield series under each distribution assumption are shown as follows:
From Table 10, the fitting effects of Student-t and Skew-t are roughly equal, but both are better than the other distributions, with Skew-t distribution slightly better than Student-t distribution. Therefore, according to the criteria of this paper, the Skew-t distribution can be considered as the optimal distribution. We assume that all yield series obey Skew-t distribution, and establishes GARCH-Skew-t model as the marginal distribution of Copula joint distribution to make sure to fit Copula model and to compute CoVaR risk spillover accurately.
Distribution | Goodness of fit | r_gb | r_hb | r_nb | r_cb |
Student-t | AIC | −2241.656 | −2427.686 | −1223.579 | −2845.977 |
Log-likelihood | 3.0696 | 3.3251 | 1.6711 | 3.8997 | |
norm | AIC | −1939.099 | −2214.332 | −1112.48 | −2667.289 |
Log-likelihood | 2.6554 | 3.0334 | 1.5199 | 3.6556 | |
Ged | AIC | −2224.151 | −2411.007 | −1205.672 | −2825.152 |
Log-likelihood | 3.0455 | 3.3022 | 1.6465 | 3.8711 | |
Skew-t | AIC | −2241.749 | −2435.992 | −1223.802 | −2845.993 |
Log-likelihood | 3.0783 | 3.3352 | 1.6801 | 3.9083 | |
Skew-ged | AIC | −2224.401 | −2415.642 | −1206.369 | −2826.307 |
Log-likelihood | 3.0445 | 3.3072 | 1.6461 | 3.8713 |
In the GARCH-Skew-t model parameter estimation of the four bond markets, the coefficients of ARCH (α) and the coefficients of GARCH (β) are both statistically significant at the 1% level and greater than 0 accompanied by a summation less than 1 as shown in Table 11, which indicates that the significance and stability conditions of the GARCH model are satisfied, proving that the model is robust and feasible. Moreover, the skewness parameter (η) and the degree of freedom parameter (ν) of the Skew-t distribution are also statistically significant at the 1% level, which proves that the Skew-t distribution is suitable for the GARCH model's residual distribution again.
r_gb | r_hb | r_nb | r_cb | |
μ | 0.0200 | 0.0314 | 0.0198 | 0.0031 |
(8.8098) | (9.6331) | (5.8430) | (1.5098) | |
ω | 0.0000** | 0.0009** | 0.0004** | 0.0001** |
(3.4276) | (3.1156) | (2.4246) | (2.4053) | |
α | 0.0254*** | 0.2901*** | 0.0918*** | 0.2365*** |
(23.9653) | (3.1270) | (3.9852) | (3.4900) | |
β | 0.9718*** | 0.5191*** | 0.8897*** | 0.7389*** |
(159.1312) | (4.5860) | (34.0055) | (10.6590) | |
η | 0.9861*** | 1.1112*** | 1.0261*** | 1.0064*** |
(31.1209) | (27.6315) | (25.8812) | (28.1273) | |
ν | 3.0413*** | 3.0334*** | 3.8586*** | 3.7020*** |
(14.2022) | (10.9898) | (9.2521) | (9.9001) | |
Note: ***, **, and * indicate statistically significant at the 1%, 5% and 10% level. |
We utilize the binary Copula function to fit CGB and the traditional bond market. Based on AIC criterion, Copula function with the best fit is chosen to calculate CoVaR. Table 11 shows the specific AIC values of Copula.
From Table 12, the t-Copula function has the best fitting effect for the combination of CGB and HB. Regarding NB and CB, the SJC-Copula model has the best fitting effect among CGB and them. In Table 13, ρ and κ are parameters of t-Copula function, η and γ are parameters of SJC-Copula function.
Nomal | t-Copula | Clayton | Gumble | Frank | SJC-Copula | |
r_gb-r_hb | −570.86 | −603.12 | −434.19 | −563.31 | −499.18 | −595.51 |
r_gb-r_nb | −550.23 | −569.46 | −434.61 | −535.76 | −435.57 | −594.40 |
r_gb-r_cb | −924.04 | −964.64 | −737.38 | −910.20 | −797.14 | −976.19 |
Optimal Copula model | ρ | κ | η | γ | |
r_gb-r_hb | t-Copula | 0.58*** | 6.92*** | —— | —— |
r_gb-r_nb | SJC-Copula | —— | —— | 5.76** | 0.78*** |
r_gb-r_cb | SJC-Copula | —— | —— | 6.53*** | 1.67*** |
Note: ***, **, and * indicate statistically significant at the 1%, 5% and 10% level. |
We adopt the combination of static and dynamic methods to measure the risk spillover characteristics of CGB and the traditional bond market using CoVaR, △CoVaR and %△CoVaR. The summation of risk spillovers is delegated by CoVaR; △CoVaR stands for the relative amount of risk spillover, which measures the net risk spillover between markets; and %△CoVaR is a standardized variable of risk spillover, which measures the contribution of risk spillover. Table 14 is the result of a bidirectional static risk spillover effect between CGB and the traditional bond market at 95% level.
Direction | Downside risk spillover | ||
CoVaR | △CoVaR | %△CoVaR | |
r_gb→r_hb | −0.1906 | −0.1222 | 198.32% |
r_gb←r_hb | −0.2325 | −0.1414 | 65.10% |
r_gb→r_nb | −0.4101 | −0.2302 | 32.30% |
r_gb←r_nb | −0.2292 | −0.1381 | 61.30% |
r_gb→r_cb | −0.1591 | −0.0921 | 60.20% |
r_gb←r_cb | −0.2504 | −0.1594 | 86.12% |
First, as shown in Table 14, CoVaR and △CoVaR among the four markets are negative, and %△CoVaR is positive from the perspective of spillover direction, indicating that the relationship between the four markets possess a positive risk spillover effect. Second, with the point of spillover intensity, for the risk spillover effect between CGB and HB, the former contributes more to the latter's risk spillover; but for the risk spillover effect between CGB and NB and CB, the former contributes less to the latter's risk spillover. Finally, the risk spillover contributions of CGB to these three traditional bond markets are slightly different from the horizontal comparison of the market. CGB has the most violent risk impact on HB and the smallest contribution to the risk spillover of NB.
For the sake of portraying dynamic changes of the risk spillover among the four markets more clearly, this section plots time-varying graph of risk spillover, as follows: The black line indicates the traditional bond markets' unconditional risk value VaR, which means that the outside world does not involve them, and the red line indicates the CoVaR value of the HB, NB and CB under the green bond market's risky influence.
It can be seen from figure 6 to figure 8 that the values of VaR and CoVaR among the traditional bond markets are time-varying. During the sample period, the absolute value of CoVaR is always smaller than VaR, which shows that a positive risk spillover effect of CGB on the traditional bond market will continue. What is worthy of remark is that the △CoVaR of CGB with the NB is larger than that with the HB, which manifests that the substitution effect between NB and CGB is higher.
The daily closing price indexes of CGB, NB, CB and HB are selected in this paper to analyze the spillover effect among the four markets from May 9, 2016 to May 6, 2022. The specific conclusions are as follows:
First, there is a mean spillover effect among the four markets. Regarding mean spillover's direction, mean spillover from the CGB to HB and CB is unidirectional, while mean spillover between NB and CGB possess the bidirectional characteristics. When paying attention to the degree of mean spillover, CGB has the strongest spillover to NB, while HB and CB are less affected by the mean spillover of CGB.
Second, the relationship between the four markets is a certain linkage and positive volatility spillover. Thus, the largest volatility spillover with CGB is the conventional bond market. In contrast, the synergy between CGB and HB is low. The dynamic correlation coefficient between CGB and CB fluctuates greatly at times, indicating that the volatility spillover effect is relatively unstable.
Third, the relationship from the traditional bond market to CGB about risk spillover direction is positive. Obviously, CGB has a greater contribution to risk spillover of HB, while it contributes less to risk spillover of NB and CB. As far as dynamic action, the risk spillover from CGB to the traditional bond market fluctuates over time.
The empirical results of this paper are similar to Gao et al. (2021) previous research conclusions. At present, more theoretical studies are currently exploring the problems encountered in the development of CGB and the formulation of policies, and since green bonds started late in China and there are few existing literatures that use China as the research subject, we quantitatively analyze the spillover effects of CGB with the NB, HB and CB to provide a comprehensive familiarization of the risk correlation characteristics between CGB and the traditional bond market. In addition, the existing studies on spillover effects are from the perspective of mean and volatility. On this basis, we add research on extreme risk spillover, and reasonably use the Copula-CoVaR model to further explore and analyze the extreme risk spillover between CGB and traditional bond market as well as the correlation between them, which is conducive to reasonable risk prevention.
Through research and analysis, we found that the dynamic correlation and extreme risk spillover among the four markets are time-varying. During economic turbulence, such as the trade disputes between China and the United States in 2018 and the outbreak of the COVID-19 in 2020, the linkage and risk spillover among them have significantly enhanced. Therefore, investors need to show solicitude for the influence of major crisis events. When the market fluctuates violently, they should overcome panic, not blindly follow the crowd, and invest cautiously. Moreover, investors should increase their capacity to understand policy changes, learn to correctly view the fluctuations of the GCB and avoid irrational behavior. Investors should be aware of the correlation between GCB and related assets when formulating portfolio investment strategies, rationally allocate resources to enhance the accuracy and precision of investment decisions and maximize returns. Green bonds are a great diversification tool, so investors can consider adding green bonds to their portfolios.
In addition, the empirical results show that the information transmission between CGB and other markets is not smooth. The government's policy guidance can help the green bond market to build a favorable institutional framework, so it is necessary for the government to increase policy support for green bonds.
All authors declare that we have not used Artificial Intelligence (AI) tools in the creation of this article.
This paper is sponsored by the following project funds: National Social Science Fund Major Project Research on Statistical Measurement and Evaluation of Green Finance Development (22&ZD161).
All authors declare no conflicts of interest in this paper.
[1] | Doonan BM, Kaiser AG, Stanley DF, et al. (2004) Silage in the farming system. In: Kaiser AG, Piltz JW, Burns HM, et al. (Eds), Successful silage 2nd ed. Dairy Australia and South Wales Department of Primary Industries, New South Wales, 1–25. |
[2] | Wee YJ, Kim JN, Ryu HW (2006) Biotechnological Production of Lactic Acid and Its Recent Applications. Food Technol Biotechnol 44: 163–172. |
[3] | Asmahan AA (2010) Beneficial role of lactic acid bacteria in food preservation and human health: A review. Res J Microbiol 5: 1213–1221. |
[4] | Kowalska JD, Nowak A, Śliżewska K, et al. (2020) Anti-Salmonella Potential of New Lactobacillus Strains with the Application in the Poultry Industry. Pol J Microbiol 69: 5–18. |
[5] | Buxton DR, Muck RE, Harrison JH (2003) Microbiology of ensiling, American Society of Agronomy. Silage Science and Technology Madison, Wisconsin USA, 31–93. |
[6] | Guo L, Yao D, Li D, et al. (2019) Effects of lactic acid bacteria isolated from rumen fluid and feces of dairy cows on fermentation quality, microbial community, and in vitro digestibility of alfalfa silage. Front Microbiol 10: 2998. |
[7] | Contreras-Govea FE, Muck RE, Broderick GA, et al. (2012) Lactobacillus plantarum effects on silage fermentation and in vitro microbial yield. Anim Feed Sci Technol 179: 61–68. |
[8] | Pot B, Salvetti E, Mattarelli P, et al. (2019) The potential impact of the Lactobacillus name change: The results of an expert meeting organised by the Lactic Acid Bacteria Industrial Platform (LABIP). Trends Food Sci Technol 94: 105–113. |
[9] | Holzer M, Mayrhuber E, Danner H, et al. (2003) The role of Lactobacillus buchneri in forage preservation. Trends Biotechnol 21: 282–287. |
[10] | Tarraran L, Mazzoli R (2018) Alternative strategies for lignocellulose fermentation through lactic acid bacteria: the state of the art and perspectives. FEMS Microbiol Lett 365. |
[11] | Hafner SD, Howard C, Muck RE, et al. (2013) Emission of volatile organic compounds from silage: Compounds, sources, and implications. Atmos Environ 77: 827–839. |
[12] | Elferink SJWHO, Driehuis F, Gottschal JC, et al. (2000) Silage fermentation processes and their manipulation. FAO Plant Prod Prot Papers 17–30. |
[13] | Schnürer J, Magnusson J (2005) Antifungal lactic acid bacteria as biopreservatives. Trends Food Sci Technol 16: 70–78. |
[14] | Guan H, Shuai Y, Yan Y, et al. (2020) Microbial community and fermentation dynamics of corn silage prepared with heat-resistant lactic acid bacteria in a hot environment. Microorganisms 8: 179. |
[15] | Muck RE, Nadeau EMG, McAllister TA, et al. (2018) Silage review: Recent advances and future uses of silage additives. J Dairy Sci 101: 3980–4000. |
[16] | Blajman JE, Paez RB, Vinderola CG, et al. (2018) A meta-analysis on the effectiveness of homofermentative and heterofermentative lactic acid bacteria for corn silage. J Appl Microbiol 125: 1655–1669. |
[17] | Oliveira AS, Weinberg ZG, Ogunade IM, et al (2017) Meta-analysis of effects of inoculation with homofermentative and facultative heterofermentative lactic acid bacteria on silage fermentation, aerobic stability, and the performance of dairy cows. J Dairy Sci 100: 4587–4603. |
[18] | Bernardes TF, Chizzotti FHM (2012) Technological innovations in silage production and utilization. Rev Bras Saúde Prod Anim 13: 629–641. |
[19] | Queiroz OCM, Ogunade IM, Weinberg Z, et al. (2018) Silage review: Foodborne pathogens in silage and their mitigation by silage additives. J Dairy Sci 101: 4132–4142. |
[20] | McDonald P, Henderson AR, Heron SJE (1991) The biochemistry of silage. Chalcombe publications, 1–45. |
[21] | Xu D, Ding W, Ke W, et al. (2018) Modulation of metabolome and bacterial community in whole crop corn silage by inoculating homofermentative Lactobacillus plantarum and heterofermentative Lactobacillus buchneri. Front Microbiol 9: 3299. |
[22] | Gobbetti M, De Angelis M, Corsetti A, et al. (2005) Biochemistry and physiology of sourdough lactic acid bacteria. Trends Food Sci Technol 16: 57–69. |
[23] | Morales-Estrada AI, López-Merino A, Gutierrez-Mendez N, et al. (2016) Partial characterization of bacteriocin produced by halotolerant Pediococcus acidilactici Strain QC38 isolated from traditional Cotija Cheese. Pol J Microbiol 65: 279–285. |
[24] | Olofsson TC, Vásquez A (2008) Detection and identification of a novel lactic acid bacterial flora within the honey stomach of the honeybee Apis mellifera. Curr Microbiol 57: 356–363. |
[25] | Papadelli M, Zoumpopoulou G, Georgalaki M, et al. (2015) Evaluation of two lactic acid bacteria starter cultures for the fermentation of natural black table olives (Olea europaea L cv Kalamon). Pol J Microbiol 64: 265–271. |
[26] | Sornplang P, Piyadeatsoontorn S (2016) Probiotic isolates from unconventional sources: a review. J Anim Sci Technol 58: 26. |
[27] | Holzapfel WH (1995) Culture media for non-sporulating Gram-positive food spoilage bacteria. In Corry JEL, Curtis GDW, Baird RM. (Eds), Progress in Industrial Microbiology. Elsevier, 89–109. |
[28] | Campana R, van Hemert S, Baffone W (2017) Strain-specific probiotic properties of lactic acid bacteria and their interference with human intestinal pathogens invasion. Gut Pathog 9: 12. |
[29] | Wu Z, Xu S, Yun Y, et al. (2019) Effect of 3-phenyllactic acid and 3-phenyllactic acid-producing lactic acid bacteria on the characteristics of alfalfa silage. Agriculture 10: 10. |
[30] | Ni K, Wang Y, Li D, et al. (2015) Characterization, identification and application of lactic Acid bacteria isolated from forage paddy rice silage. PLoS One 10: e0121967. |
[31] | Borreani G, Tabacco E, Schmidt RJ, et al. (2018) Silage review: Factors affecting dry matter and quality losses in silages. J Dairy Sci 101: 3952–3979. |
[32] | Zhang Y, Zhao X, Chen W, et al. (2019) Effects of adding various silage additives to whole corn crops at ensiling on performance, rumen fermentation, and serum physiological characteristics of growing-finishing cattle. Animals 9: 695. |
[33] | Soundharrajan I, Kim DH, Srisesharam S, et al. (2017) Application of customised bacterial inoculants for grass haylage production and its effectiveness on nutrient composition and fermentation quality of haylage. 3 Biotech 7: 321. |
[34] | Driehuis F, Elferink SJ, Spoelstra SF (1999) Anaerobic lactic acid degradation during ensilage of whole crop maize inoculated with Lactobacillus buchneri inhibits yeast growth and improves aerobic stability. J Appl Microbiol 87: 583–594. |
[35] | Muck R (2013) Recent advances in silage microbiology. Agr Food Sci 22: 3–15. |
[36] | Cai Y, Benno Y, Ogawa M, et al. (1998) Influence of Lactobacillus spp. from an inoculant and of Weissella and Leuconostoc spp. from forage crops on silage fermentation. Appl Environ Microbiol 64: 2982–2987. |
[37] | Ellis JL, Hindrichsen IK, Klop G, et al. (2016) Effects of lactic acid bacteria silage inoculation on methane emission and productivity of Holstein Friesian dairy cattle. J Dairy Sci 99: 7159–7174. |
[38] | Ni K, Wang F, Zhu B, et al. (2017) Effects of lactic acid bacteria and molasses additives on the microbial community and fermentation quality of soybean silage. Bioresour Technol 238: 706–715. |
[39] | Zhao J, Dong Z, Li J, et al. (2018) Ensiling as pretreatment of rice straw: The effect of hemicellulase and Lactobacillus plantarum on hemicellulose degradation and cellulose conversion. Bioresour Technol 266: 158–165. |
[40] | Haag NL, Nagele HJ, Fritz T, et al. (2015) Effects of ensiling treatments on lactic acid production and supplementary methane formation of maize and amaranth--An advanced green biorefining approach. Bioresour Technol 178: 217–225. |
[41] | Yang L, Yuan X, Li J, et al. (2019) Dynamics of microbial community and fermentation quality during ensiling of sterile and nonsterile alfalfa with or without Lactobacillus plantarum inoculant. Bioresour Technol 275: 280–287. |
[42] | Bao W, Mi Z, Xu H, et al. (2016) Assessing quality of Medicago sativa silage by monitoring bacterial composition with single molecule, real-time sequencing technology and various physiological parameters. Sci Rep 6: 28358. |
[43] | Adetoye A, Pinloche E, Adeniyi BA, et al. (2018) Characterization and anti-Salmonella activities of lactic acid bacteria isolated from cattle faeces. BMC Microbiol 18: 96. |
[44] | Xu Z, He H, Zhang S, et al. (2017) Characterization of feruloyl esterases produced by the four Lactobacillus Species: L. amylovorus, L. acidophilus, L. farciminis and L. fermentum, isolated from ensiled corn stover. Front Microbiol 8: 941. |
[45] | Duniere L, Gleizal A, Chaucheyras-Durand F, et al. (2011) Fate of Escherichia coli O26 in corn silage experimentally contaminated at ensiling, at silo opening, or after aerobic exposure, and protective effect of various bacterial inoculants. Appl Environ Microbiol 77: 8696–8704. |
[46] | Li M, Shan G, Zhou H, et al. (2017) CO2 production, dissolution and pressure dynamics during silage production: multi-sensor-based insight into parameter interactions. Sci Rep 7: 14721. |
[47] | Mora-Villalobos JA, Montero-Zamora J, Barboza N, et al (2020) Multi-Product lactic acid bacteria fermentations: A review. Fermentation 6: 23. |
[48] | Su R, Ni K, Wang T, et al. (2019) Effects of ferulic acid esterase-producing Lactobacillus fermentum and cellulase additives on the fermentation quality and microbial community of alfalfa silage. Peer J 7: e7712. |
[49] | Buxton DR, Muck RE, Harrison JH (2003) Preharvest plant factors affecting ensiling. Silage Sci Technol 42: 199–250. |
[50] | Axelsson L (1998) Lactic acid bacteria: Classification and physiology. In: Salminen S, von Wright A, Ouwehand A. (Eds), Lactic acid bacteria. Marcel Dekker Inc, New York, 1–67. |
[51] | Rooke JA, Hatfield RD (2003) Biochemistry of ensiling. USDA-ARS/UNL Faculty, 1399. |
[52] | Tanizawa Y, Tohno M, Kaminuma E, et al. (2015) Complete genome sequence and analysis of Lactobacillus hokkaidonensis LOOC260 (T), a psychrotrophic lactic acid bacterium isolated from silage. BMC Genomics 16: 240. |
[53] | Liu J, Wang Q, Zou H, et al. (2013) Glucose metabolic flux distribution of Lactobacillus amylophilus during lactic acid production using kitchen waste saccharified solution. Microb Biotechnol 6: 685–693, |
[54] | Wolfe AJ (2015) Glycolysis for Microbiome Generation. Microbiol Spectr 3: 10.1128. |
[55] | Melchiorsen CR, Jensen NBS, Christensen B, et al. (2001) Dynamics of pyruvate metabolism in Lactococcus Lactis. Biotechnol Bioeng 74: 271–279. |
[56] | Fushinobu S (2010) Unique sugar metabolic pathways of bifidobacteria. Biosci Biotechnol Biochem 74: 2374–2384, |
[57] | Macfarlane GT, Macfarlane S (2012) Bacteria, colonic fermentation, and gastrointestinal health. J AOAC Int 95: 50–60. |
[58] | Zaunmuller T, Eichert M, Richter H, et al. (2006) Variations in the energy metabolism of biotechnologically relevant heterofermentative lactic acid bacteria during growth on sugars and organic acids. Appl Microbiol Biotechnol 72: 421–429. |
[59] | Iskandar CF, Cailliez-Grimal C, Borges F, et al. (2019) Review of lactose and galactose metabolism in Lactic Acid Bacteria dedicated to expert genomic annotation. Trends Food Sci Technol 88: 121–132. |
[60] | Jones EC, Barnes RJ (1967) Non-volatile organic acids of grasses. J Sci. Food Agric 18: 321–324. |
[61] | Kung Jr L, Shaver RD, Grant RJ, et al. (2018) Silage review: Interpretation of chemical, microbial, and organoleptic components of silages. J Dairy Sci 101: 4020–4033. |
[62] | Milanovic V, Osimani A, Garofalo C, et al. (2020) Selection of cereal-sourced lactic acid bacteria as candidate starters for the baking industry. PLoS One 15: e0236190. |
[63] | Vieco-Saiz N, Belguesmia Y, Raspoet R, et al. (2019) Benefits and inputs from lactic acid bacteria and their bacteriocins as alternatives to antibiotic growth promoters during food-animal production. Front Microbiol 10: 57. |
[64] | Adebayo-Tayo B, Fashogbon R (2020) In vitro antioxidant, antibacterial, in vivo immunomodulatory, antitumor and hematological potential of exopolysaccharide produced by wild type and mutant Lactobacillus delbureckii subsp. bulgaricus. Heliyon 6: e03268. |
[65] | Hellal A, Amrouche L, Ferhat Z, et al. (2011) Characterization of bacteriocin from Lactococcus isolated from traditional Algerian dairy products. Ann Microbiol 62: 177–185. |
[66] | Li D, Ni K, Pang H, et al. (2015) Identification and antimicrobial activity detection of lactic acid bacteria isolated from corn stover silage. Asian-Australas J Anim Sci 28: 620–631. |
[67] | Byrne CM, Kiely PO, Bolton DJ, et al. (2002) Fate of Escherichia coli O157: H7 during Silage Fermentation. J Food Prot 65: 1854–1860. |
[68] | Pedroso AF, Adesogan AT, Queiroz OCM, et al. (2010) Control of Escherichia coli O157: H7 in corn silage with or without various inoculants: efficacy and mode of action. J Dairy Sci 93: 1098–1104. |
[69] | Bjornsdottir K, Breidt Jr F, McFeeters RF (2006) Protective effects of organic acids on survival of Escherichia coli O157: H7 in acidic environments. Appl Environ Microbiol 72: 660–664. |
[70] | Ogunade IM, Jiang Y, Kim DH, et al. (2017) Fate of Escherichia coli O157: H7 and bacterial diversity in corn silage contaminated with the pathogen and treated with chemical or microbial additives. J Dairy Sci 100: 1780–1794. |
[71] | Amado IR, Fuciños C, Fajardo P, et al. (2012) Evaluation of two bacteriocin-producing probiotic lactic acid bacteria as inoculants for controlling Listeria monocytogenes in grass and maize silages. Anim Feed Sci Technol 175: 137–149. |
[72] | Amado IR, Fucinos C, Fajardo P, et al. (2016) Pediocin SA-1: A selective bacteriocin for controlling Listeria monocytogenes in maize silages. J Dairy Sci 99: 8070–8080. |
[73] | Gavrilova E, Anisimova E, Gabdelkhadieva A, et al. (2019) Newly isolated lactic acid bacteria from silage targeting biofilms of foodborne pathogens during milk fermentation. BMC Microbiol 19: 248. |
[74] | Wang X, Wang W, Lv H, et al. (2020) Probiotic potential and wide-spectrum antimicrobial activity of lactic acid bacteria isolated from infant feces. Probiotics & Antimicro Prot DOI: 10.1007/s12602-020-09658-3. |
[75] | Ogunade IM, Kim DH, Jiang Y, et al. (2016) Control of Escherichia coli O157: H7 in contaminated alfalfa silage: Effects of silage additives. J Dairy Sci 99: 4427–4436. |
[76] | Davidson PM, Taylor TM, Schmidt SE. Chemical Preservatives and natural antimicrobial compounds. Food Microbiology: Fundamentals and Frontiers 4th Ed, 765–801. |
[77] | Santos AO, Avila CLS, Schwan RF (2013) Selection of tropical lactic acid bacteria for enhancing the quality of maize silage. J Dairy Sci 96: 7777–7789. |
[78] | Arques JL, Rodriguez E, Langa S, et al. (2015) Antimicrobial activity of lactic acid bacteria in dairy products and gut: effect on pathogens. Biomed Res Int 2015: 584183. |
[79] | Broberg A, Jacobsson K, Strom K, et al. (2007) Metabolite profiles of lactic acid bacteria in grass silage. Appl Environ Microbiol 73: 5547–5552. |
[80] | Wilkinson JM, Rinne M (2018) Highlights of progress in silage conservation and future perspectives. Grass Forage Sci 73: 40–52. |
[81] | Miron J, Zuckerman E, Adin G, et al. (2007) Comparison of two forage sorghum varieties with corn and the effect of feeding their silages on eating behavior and lactation performance of dairy cows. Anim Feed Sci Technol 139: 23–39. |
[82] | Da Silva TC, Da Silva LD, Santos EM, et al. (2017) Importance of the fermentation to produce high-quality silage. Fermentation Processes 1–20. |
[83] | DoiK, Nishizaki Y, Kimura H, et al. (2013) Identification of thermo tolerant lactic acid bacteria isolated from silage prepared in the hot and humid climate of Southwestern Japan. SpringerPlus 2: 485. |
[84] | Pinho RMA, Santos EM, Pinto de Carvalho GG, et al. (2013) Microbial and fermentation profiles, losses and chemical composition of silages of buffel grass harvested at different cutting heights. Rev Bras Zootec 42: 850–856. |
[85] | Khota W, Pholsen S, Higgs D, et al. (2016) Natural lactic acid bacteria population of tropical grasses and their fermentation factor analysis of silage prepared with cellulase and inoculant. J Dairy Sci 99: 9768–9781. |
[86] | Ennahar S, Cai Y, Fujita Y (2003) Phylogenetic diversity of lactic acid bacteria associated with paddy rice silage as determined by 16S ribosomal DNA analysis. Appl Environ Microbiol 69: 444–451. |
[87] | Tamang JP, Cotter PD, Endo A, et al. (2020) Fermented foods in a global age: East meets West. Compr Rev Food Sci Food saf 19: 184–217. |
[88] | Rezac S, Kok CR, Heermann M, Hutkins R (2018) Fermented foods as a dietary source of live organisms. Front Microbiol 9: 1785. |
[89] | Andlauer W, Furst P (2003) Absorption and metabolism of phenolic phytochemicals in the isolated rat small intestine with special reference to isoflavones and anthocyanins–A review. Polishy J Food Nutri Sci 12: 117–127. |
[90] | Babaeinasab Y, Rouzbehan Y, Fazaeli H, et al. (2015) Chemical composition, silage fermentation characteristics, and in vitro ruminal fermentation parameters of potato-wheat straw silage treated with molasses and lactic acid bacteria and corn silage. J Anim Sci 93: 4377–4386. |
[91] | Zhang LY, Liu S, Zhao XJ, et al. (2019) Lactobacillus rhamnosus GG modulates gastrointestinal absorption, excretion patterns, and toxicity in Holstein calves fed a single dose of aflatoxin B1. J Dairy Sci 102: 1330–1340. |
[92] | Goel G, Raghav M, Beniwal V, et al. (2015) Anaerobic degradation of tannins in Acacia nilotica pods by Enterococcus faecalis in co-culture with ruminal microbiota. J Gen Appl Microbiol 61: 31–33. |
[93] | Aggoun M, Boussada A, Arhab R, et al. (2017) Effect of natural bioflavonoid on in vitro ruminal microbiota activity in sheep rumen liquor. J BioSci Biotechnol 6: 31–35. |
[94] | Zhang F, Wang X, Lu W, et al. (2019) Improved quality of corn silage when combining cellulose-decomposing bacteria and Lactobacillus buchneri during silage fermentation. Biomed Res Int 2019: 4361358. |
[95] | Sun L, Wang Z, Gentu G, et al. (2019) Changes in microbial population and chemical composition of corn stover during field exposure and effects on silage fermentation and in vitro digestibility. Asian-Australas J Anim Sci 32: 815–825. |
1. | Zhongzhe Ouyang, Min Lu, Systemic Financial Risk Forecasting with Decomposition–Clustering-Ensemble Learning Approach: Evidence from China, 2024, 16, 2073-8994, 480, 10.3390/sym16040480 | |
2. | Gaoke Liao, Yanling Li, Mengxin Wang, Contagion network of idiosyncratic volatility: Does corporate environmental responsibility matter?, 2024, 129, 01409883, 107168, 10.1016/j.eneco.2023.107168 | |
3. | Yi-Chiuan Wang, Yi-hao Lai, Jyh-Lin Wu, Asymmetries in risk spillovers between currency and stock markets: Evidence from the CoVaR-copula approach, 2024, 63, 0924-865X, 1083, 10.1007/s11156-024-01285-1 | |
4. | Zisheng Ouyang, Zhen Chen, Xuewei Zhou, Zhongzhe Ouyang, Imported risk in global financial markets: Evidence from cross-market connectedness, 2025, 10629408, 102374, 10.1016/j.najef.2025.102374 |
variable | Mean | Maximum | Minimum | Std.Dev. | Kurtosis | Skewness | J-Btest |
r_gb | 0.0174 | 0.8008 | −0.8183 | 0.0803 | 23.1679 | 0.2125 | 32378 |
r_hb | 0.0236 | 0.5562 | −0.5527 | 0.0621 | 13.2491 | 0.2847 | 10773 |
r_nb | 0.0144 | 0.3909 | −0.5585 | 0.0550 | 9.6374 | 0.6196 | 5609.5 |
r_cb | 0.0011 | 1.0538 | −0.9505 | 0.1256 | 15.8382 | −1.1160 | 15442 |
variable | ADF test | %1 threshold | %5 threshold | %10 threshold | Stationary |
r_gb | −8.6346 | −2.5659 | −1.9410 | −1.6166 | Stationary |
r_hb | −8.5242 | −2.5659 | −1.9410 | −1.6166 | Stationary |
r_nb | −9.8269 | −3.4328 | −2.8625 | −2.5673 | Stationary |
r_cb | −9, 1778 | −2.5659 | −1.9410 | −1.6166 | Stationary |
Null hypothesis | Lag order | χ2 | P value | conclusion |
r_hb does not Granger cause r_gb | 6 | 8.3197 | 0.3052 | Fail to reject |
r_gb does not Granger cause r_hb | 6 | 27.7403 | 0.0002 | Reject |
r_nb does not Granger cause r_gb | 5 | 10.5105 | 0.0327 | Reject |
r_gb does not Granger cause r_nb | 5 | 61.1701 | 0.0000 | Reject |
r_cb does not Granger cause r_gb | 6 | 2.6135 | 0.9562 | Fail to reject |
r_gb does not Granger cause r_cb | 6 | 11.0820 | 0.0305 | Reject |
r_hb | r_gb | r_hb | r_gb | ||
r_hb(−1) | 1.0364** | −0.1179 | r_gb(−1) | −0.2639*** | 1.4445*** |
t | 29.3130 | −2.4860 | t | 9.9620 | 40.6270 |
r_hb(−2) | 0.0999 | 0.1796 | r_gb(−2) | −0.2544*** | −0.4434*** |
t | 1.8970 | 2.5420 | t | −6.0370 | −7.8390 |
r_hb(−3) | −0.0745 | −0.1345 | r_gb(−3) | 0.0101 | 0.1229 |
t | −1.4130 | −1.8990 | t | 0.2350 | 2.1310 |
r_hb(−4) | −0.0307 | 0.0109 | r_gb(−4) | −0.0477 | −0.0759 |
t | −0.5830 | 0.1550 | t | −1.1120 | −1.3190 |
r_hb(−5) | 0.1079 | 0.1048 | r_gb(−5) | 0.0073 | −0.0034 |
t | 2.0520 | 1.4850 | t | 0.1720 | −0.0600 |
r_hb(−6) | −0.1416*** | −0.0434 | r_gb(−6) | 0.0243 | −0.0439 |
t | −4.1210 | −0.9420 | t | 0.9010 | −1.2130 |
Note: ***, **, and * indicate statistically significant at the 1%, 5% and 10% level. |
r_nb | r_gb | r_nb | r_gb | ||
r_nb(−1) | 1.2845** | 0.2228 | r_gb(−1) | −0.1611 | 1.1178*** |
t | 36.0980 | 11.0030 | t | −2.5790 | 31.4280 |
r_nb(−2) | −0.3195*** | −0.2212*** | r_gb(−2) | 0.2776** | −0.0423 |
t | −6.1680 | −7.5060 | t | 2.8760 | −0.7710 |
r_nb(−3) | 0.0897 | 0.0384 | r_gb(−3) | −0.1247 | −0.0143 |
t | 1.7000 | 1.2780 | t | −1.2840 | −0.2600 |
r_nb(−4) | −0.1010 | −0.0746 | r_gb(−4) | 0.1519 | 0.0272 |
t | −1.9360 | −2.5150 | t | 1.610 | 0.5070 |
r_nb(−5) | 0.0319 | 0.0318 | r_gb(−5) | −0.1312 | 0.0858** |
t | 0.8980 | 1.5720 | t | −2.3210 | −2.6670 |
Note: ***, **, and * indicate statistically significant at the 1%, 5% and 10% level. |
r_cb | r_gb | r_cb | r_gb | ||
r_cb(−1) | 1.0364*** | −0.1179 | r_gb(−1) | 0 | 1.4445*** |
t | 29.3130 | −2.4860 | t | 9.9620 | 40.6270 |
r_cb(−2) | 0.0999 | 0.1796 | r_gb(−2) | −0.2544*** | −0.4434*** |
t | 1.8970 | 2.5420 | t | −6.0370 | −7.8390 |
r_cb(−3) | −0.0745 | −0.1345 | r_gb(−3) | 0.0101 | 0.1229 |
t | −1.4130 | −1.8990 | t | 0.2350 | 2.1310 |
r_cb(−4) | −0.0307 | 0.0109 | r_gb(−4) | −0.0477 | −0.0759 |
t | −0.5830 | 0.1550 | t | −1.1120 | −1.3190 |
r_cb(−5) | 0.1079 | 0.1048 | r_gb(−5) | 0.0073 | −0.0034 |
t | 2.0520 | 1.4850 | t | 0.1720 | −0.0600 |
r_cb(−6) | −0.1416*** | −0.0434 | r_gb(−6) | 0.0243 | −0.0439 |
t | −4.1210 | −0.9420 | t | 0.9010 | −1.2130 |
Note: ***, **, and * indicate statistically significant at the 1%, 5% and 10% level. |
Constant | coefficient of ARCH(α) | coefficient of GARCH(β) | |
r_gb | 0.0008*** (4.649) | 0.4123*** (7.324) | 0.5593*** (10.884) |
r_hb | 0.0011*** (4.316) | 0.1768*** (4.228) | 0.5542*** (6.123) |
r_nb | 0.0011*** (3.343) | 0.1862*** (4.987) | 0.7608*** (16.230) |
r_cb | 0.0004*** (7.071) | 0.4760*** (7.508) | 0.4095*** (7.390) |
Note: ***, **, and * indicate statistically significant at the 1%, 5% and 10% level. |
DCC1 | DCC2 | DCC1+DCC2 | |
r_gb&r_hb | 0.0008*** (4.649) | 0.4123*** (7.324) | 0.5593*** (10.884) |
r_gb&r_nb | 0.0011*** (4.316) | 0.8768*** (4.228) | 0.8779*** (6.123) |
r_gb&r_cb | 0.0011*** (3.343) | 0.1862*** (4.987) | 0.1873*** (16.230) |
Note: ***, **, and * indicate statistically significant at the 1%, 5% and 10% level. |
Minimum | Maximum | Mean | |
r_gb&r_hb | −0.0904 | 0.9861 | 0.6471 |
r_gb&r_nb | 0.4353 | 0.9112 | 0.6457 |
r_gb&r_cb | 0.1402 | 0.9924 | 0.7517 |
Distribution | Goodness of fit | r_gb | r_hb | r_nb | r_cb |
Student-t | AIC | −2241.656 | −2427.686 | −1223.579 | −2845.977 |
Log-likelihood | 3.0696 | 3.3251 | 1.6711 | 3.8997 | |
norm | AIC | −1939.099 | −2214.332 | −1112.48 | −2667.289 |
Log-likelihood | 2.6554 | 3.0334 | 1.5199 | 3.6556 | |
Ged | AIC | −2224.151 | −2411.007 | −1205.672 | −2825.152 |
Log-likelihood | 3.0455 | 3.3022 | 1.6465 | 3.8711 | |
Skew-t | AIC | −2241.749 | −2435.992 | −1223.802 | −2845.993 |
Log-likelihood | 3.0783 | 3.3352 | 1.6801 | 3.9083 | |
Skew-ged | AIC | −2224.401 | −2415.642 | −1206.369 | −2826.307 |
Log-likelihood | 3.0445 | 3.3072 | 1.6461 | 3.8713 |
r_gb | r_hb | r_nb | r_cb | |
μ | 0.0200 | 0.0314 | 0.0198 | 0.0031 |
(8.8098) | (9.6331) | (5.8430) | (1.5098) | |
ω | 0.0000** | 0.0009** | 0.0004** | 0.0001** |
(3.4276) | (3.1156) | (2.4246) | (2.4053) | |
α | 0.0254*** | 0.2901*** | 0.0918*** | 0.2365*** |
(23.9653) | (3.1270) | (3.9852) | (3.4900) | |
β | 0.9718*** | 0.5191*** | 0.8897*** | 0.7389*** |
(159.1312) | (4.5860) | (34.0055) | (10.6590) | |
η | 0.9861*** | 1.1112*** | 1.0261*** | 1.0064*** |
(31.1209) | (27.6315) | (25.8812) | (28.1273) | |
ν | 3.0413*** | 3.0334*** | 3.8586*** | 3.7020*** |
(14.2022) | (10.9898) | (9.2521) | (9.9001) | |
Note: ***, **, and * indicate statistically significant at the 1%, 5% and 10% level. |
Nomal | t-Copula | Clayton | Gumble | Frank | SJC-Copula | |
r_gb-r_hb | −570.86 | −603.12 | −434.19 | −563.31 | −499.18 | −595.51 |
r_gb-r_nb | −550.23 | −569.46 | −434.61 | −535.76 | −435.57 | −594.40 |
r_gb-r_cb | −924.04 | −964.64 | −737.38 | −910.20 | −797.14 | −976.19 |
Optimal Copula model | ρ | κ | η | γ | |
r_gb-r_hb | t-Copula | 0.58*** | 6.92*** | —— | —— |
r_gb-r_nb | SJC-Copula | —— | —— | 5.76** | 0.78*** |
r_gb-r_cb | SJC-Copula | —— | —— | 6.53*** | 1.67*** |
Note: ***, **, and * indicate statistically significant at the 1%, 5% and 10% level. |
Direction | Downside risk spillover | ||
CoVaR | △CoVaR | %△CoVaR | |
r_gb→r_hb | −0.1906 | −0.1222 | 198.32% |
r_gb←r_hb | −0.2325 | −0.1414 | 65.10% |
r_gb→r_nb | −0.4101 | −0.2302 | 32.30% |
r_gb←r_nb | −0.2292 | −0.1381 | 61.30% |
r_gb→r_cb | −0.1591 | −0.0921 | 60.20% |
r_gb←r_cb | −0.2504 | −0.1594 | 86.12% |
variable | Mean | Maximum | Minimum | Std.Dev. | Kurtosis | Skewness | J-Btest |
r_gb | 0.0174 | 0.8008 | −0.8183 | 0.0803 | 23.1679 | 0.2125 | 32378 |
r_hb | 0.0236 | 0.5562 | −0.5527 | 0.0621 | 13.2491 | 0.2847 | 10773 |
r_nb | 0.0144 | 0.3909 | −0.5585 | 0.0550 | 9.6374 | 0.6196 | 5609.5 |
r_cb | 0.0011 | 1.0538 | −0.9505 | 0.1256 | 15.8382 | −1.1160 | 15442 |
variable | ADF test | %1 threshold | %5 threshold | %10 threshold | Stationary |
r_gb | −8.6346 | −2.5659 | −1.9410 | −1.6166 | Stationary |
r_hb | −8.5242 | −2.5659 | −1.9410 | −1.6166 | Stationary |
r_nb | −9.8269 | −3.4328 | −2.8625 | −2.5673 | Stationary |
r_cb | −9, 1778 | −2.5659 | −1.9410 | −1.6166 | Stationary |
Null hypothesis | Lag order | χ2 | P value | conclusion |
r_hb does not Granger cause r_gb | 6 | 8.3197 | 0.3052 | Fail to reject |
r_gb does not Granger cause r_hb | 6 | 27.7403 | 0.0002 | Reject |
r_nb does not Granger cause r_gb | 5 | 10.5105 | 0.0327 | Reject |
r_gb does not Granger cause r_nb | 5 | 61.1701 | 0.0000 | Reject |
r_cb does not Granger cause r_gb | 6 | 2.6135 | 0.9562 | Fail to reject |
r_gb does not Granger cause r_cb | 6 | 11.0820 | 0.0305 | Reject |
r_hb | r_gb | r_hb | r_gb | ||
r_hb(−1) | 1.0364** | −0.1179 | r_gb(−1) | −0.2639*** | 1.4445*** |
t | 29.3130 | −2.4860 | t | 9.9620 | 40.6270 |
r_hb(−2) | 0.0999 | 0.1796 | r_gb(−2) | −0.2544*** | −0.4434*** |
t | 1.8970 | 2.5420 | t | −6.0370 | −7.8390 |
r_hb(−3) | −0.0745 | −0.1345 | r_gb(−3) | 0.0101 | 0.1229 |
t | −1.4130 | −1.8990 | t | 0.2350 | 2.1310 |
r_hb(−4) | −0.0307 | 0.0109 | r_gb(−4) | −0.0477 | −0.0759 |
t | −0.5830 | 0.1550 | t | −1.1120 | −1.3190 |
r_hb(−5) | 0.1079 | 0.1048 | r_gb(−5) | 0.0073 | −0.0034 |
t | 2.0520 | 1.4850 | t | 0.1720 | −0.0600 |
r_hb(−6) | −0.1416*** | −0.0434 | r_gb(−6) | 0.0243 | −0.0439 |
t | −4.1210 | −0.9420 | t | 0.9010 | −1.2130 |
Note: ***, **, and * indicate statistically significant at the 1%, 5% and 10% level. |
r_nb | r_gb | r_nb | r_gb | ||
r_nb(−1) | 1.2845** | 0.2228 | r_gb(−1) | −0.1611 | 1.1178*** |
t | 36.0980 | 11.0030 | t | −2.5790 | 31.4280 |
r_nb(−2) | −0.3195*** | −0.2212*** | r_gb(−2) | 0.2776** | −0.0423 |
t | −6.1680 | −7.5060 | t | 2.8760 | −0.7710 |
r_nb(−3) | 0.0897 | 0.0384 | r_gb(−3) | −0.1247 | −0.0143 |
t | 1.7000 | 1.2780 | t | −1.2840 | −0.2600 |
r_nb(−4) | −0.1010 | −0.0746 | r_gb(−4) | 0.1519 | 0.0272 |
t | −1.9360 | −2.5150 | t | 1.610 | 0.5070 |
r_nb(−5) | 0.0319 | 0.0318 | r_gb(−5) | −0.1312 | 0.0858** |
t | 0.8980 | 1.5720 | t | −2.3210 | −2.6670 |
Note: ***, **, and * indicate statistically significant at the 1%, 5% and 10% level. |
r_cb | r_gb | r_cb | r_gb | ||
r_cb(−1) | 1.0364*** | −0.1179 | r_gb(−1) | 0 | 1.4445*** |
t | 29.3130 | −2.4860 | t | 9.9620 | 40.6270 |
r_cb(−2) | 0.0999 | 0.1796 | r_gb(−2) | −0.2544*** | −0.4434*** |
t | 1.8970 | 2.5420 | t | −6.0370 | −7.8390 |
r_cb(−3) | −0.0745 | −0.1345 | r_gb(−3) | 0.0101 | 0.1229 |
t | −1.4130 | −1.8990 | t | 0.2350 | 2.1310 |
r_cb(−4) | −0.0307 | 0.0109 | r_gb(−4) | −0.0477 | −0.0759 |
t | −0.5830 | 0.1550 | t | −1.1120 | −1.3190 |
r_cb(−5) | 0.1079 | 0.1048 | r_gb(−5) | 0.0073 | −0.0034 |
t | 2.0520 | 1.4850 | t | 0.1720 | −0.0600 |
r_cb(−6) | −0.1416*** | −0.0434 | r_gb(−6) | 0.0243 | −0.0439 |
t | −4.1210 | −0.9420 | t | 0.9010 | −1.2130 |
Note: ***, **, and * indicate statistically significant at the 1%, 5% and 10% level. |
Constant | coefficient of ARCH(α) | coefficient of GARCH(β) | |
r_gb | 0.0008*** (4.649) | 0.4123*** (7.324) | 0.5593*** (10.884) |
r_hb | 0.0011*** (4.316) | 0.1768*** (4.228) | 0.5542*** (6.123) |
r_nb | 0.0011*** (3.343) | 0.1862*** (4.987) | 0.7608*** (16.230) |
r_cb | 0.0004*** (7.071) | 0.4760*** (7.508) | 0.4095*** (7.390) |
Note: ***, **, and * indicate statistically significant at the 1%, 5% and 10% level. |
DCC1 | DCC2 | DCC1+DCC2 | |
r_gb&r_hb | 0.0008*** (4.649) | 0.4123*** (7.324) | 0.5593*** (10.884) |
r_gb&r_nb | 0.0011*** (4.316) | 0.8768*** (4.228) | 0.8779*** (6.123) |
r_gb&r_cb | 0.0011*** (3.343) | 0.1862*** (4.987) | 0.1873*** (16.230) |
Note: ***, **, and * indicate statistically significant at the 1%, 5% and 10% level. |
Minimum | Maximum | Mean | |
r_gb&r_hb | −0.0904 | 0.9861 | 0.6471 |
r_gb&r_nb | 0.4353 | 0.9112 | 0.6457 |
r_gb&r_cb | 0.1402 | 0.9924 | 0.7517 |
Distribution | Goodness of fit | r_gb | r_hb | r_nb | r_cb |
Student-t | AIC | −2241.656 | −2427.686 | −1223.579 | −2845.977 |
Log-likelihood | 3.0696 | 3.3251 | 1.6711 | 3.8997 | |
norm | AIC | −1939.099 | −2214.332 | −1112.48 | −2667.289 |
Log-likelihood | 2.6554 | 3.0334 | 1.5199 | 3.6556 | |
Ged | AIC | −2224.151 | −2411.007 | −1205.672 | −2825.152 |
Log-likelihood | 3.0455 | 3.3022 | 1.6465 | 3.8711 | |
Skew-t | AIC | −2241.749 | −2435.992 | −1223.802 | −2845.993 |
Log-likelihood | 3.0783 | 3.3352 | 1.6801 | 3.9083 | |
Skew-ged | AIC | −2224.401 | −2415.642 | −1206.369 | −2826.307 |
Log-likelihood | 3.0445 | 3.3072 | 1.6461 | 3.8713 |
r_gb | r_hb | r_nb | r_cb | |
μ | 0.0200 | 0.0314 | 0.0198 | 0.0031 |
(8.8098) | (9.6331) | (5.8430) | (1.5098) | |
ω | 0.0000** | 0.0009** | 0.0004** | 0.0001** |
(3.4276) | (3.1156) | (2.4246) | (2.4053) | |
α | 0.0254*** | 0.2901*** | 0.0918*** | 0.2365*** |
(23.9653) | (3.1270) | (3.9852) | (3.4900) | |
β | 0.9718*** | 0.5191*** | 0.8897*** | 0.7389*** |
(159.1312) | (4.5860) | (34.0055) | (10.6590) | |
η | 0.9861*** | 1.1112*** | 1.0261*** | 1.0064*** |
(31.1209) | (27.6315) | (25.8812) | (28.1273) | |
ν | 3.0413*** | 3.0334*** | 3.8586*** | 3.7020*** |
(14.2022) | (10.9898) | (9.2521) | (9.9001) | |
Note: ***, **, and * indicate statistically significant at the 1%, 5% and 10% level. |
Nomal | t-Copula | Clayton | Gumble | Frank | SJC-Copula | |
r_gb-r_hb | −570.86 | −603.12 | −434.19 | −563.31 | −499.18 | −595.51 |
r_gb-r_nb | −550.23 | −569.46 | −434.61 | −535.76 | −435.57 | −594.40 |
r_gb-r_cb | −924.04 | −964.64 | −737.38 | −910.20 | −797.14 | −976.19 |
Optimal Copula model | ρ | κ | η | γ | |
r_gb-r_hb | t-Copula | 0.58*** | 6.92*** | —— | —— |
r_gb-r_nb | SJC-Copula | —— | —— | 5.76** | 0.78*** |
r_gb-r_cb | SJC-Copula | —— | —— | 6.53*** | 1.67*** |
Note: ***, **, and * indicate statistically significant at the 1%, 5% and 10% level. |
Direction | Downside risk spillover | ||
CoVaR | △CoVaR | %△CoVaR | |
r_gb→r_hb | −0.1906 | −0.1222 | 198.32% |
r_gb←r_hb | −0.2325 | −0.1414 | 65.10% |
r_gb→r_nb | −0.4101 | −0.2302 | 32.30% |
r_gb←r_nb | −0.2292 | −0.1381 | 61.30% |
r_gb→r_cb | −0.1591 | −0.0921 | 60.20% |
r_gb←r_cb | −0.2504 | −0.1594 | 86.12% |