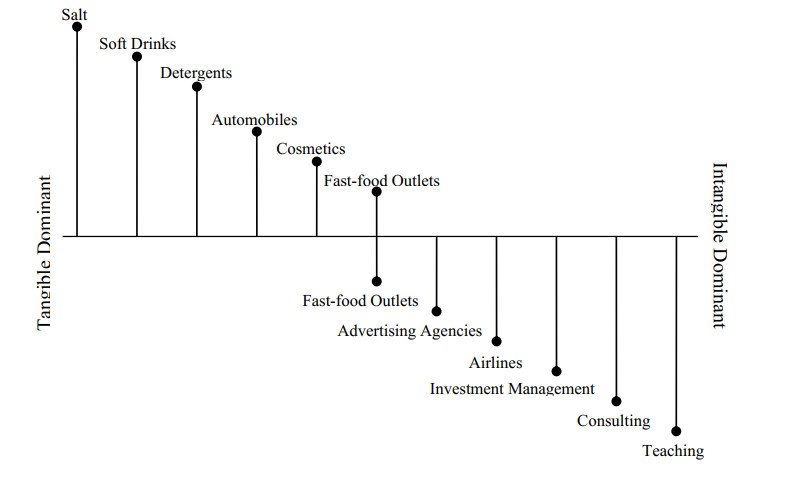
We present an empirical test of a new measure to classify organizations according to the tangibility of product (output) flows delivered to customers. Our measure exhibits the empirical consequences of using standard industrial classifications to assume that firms within the same industry either share identical properties or sell homogeneous products. To illustrate the misleading findings that can result from these assumptions, we investigate whether prior literature on capital structure provides a sensible interpretation of organizational behavior, based as it often is on an assumption that all firms within a given industrial classification sell durable goods. In contrast to the product-market literature based upon the trade-off theory of capital structure, that would predict that firms selling physical goods will have proportionately less debt, in fact, when firms within industries are classified using our measure, we find to the contrary. Our intention is not to displace existing systems of industry classification but is, rather, to highlight the dangers of drawing conclusions from assuming homogeneity amongst firms which are formally registered within the same industry.
Citation: Tiago Cardão-Pito, Julia A Smith, João da Silva Ferreira. Using accounting measures of (in)tangibility for organizational classifications[J]. Quantitative Finance and Economics, 2021, 5(2): 325-351. doi: 10.3934/QFE.2021015
[1] | Miquel Carreras-Simó, Germà Coenders . The relationship between asset and capital structure: a compositional approach with panel vector autoregressive models. Quantitative Finance and Economics, 2021, 5(4): 571-590. doi: 10.3934/QFE.2021025 |
[2] | Fisnik Morina, Simon Grima . The impact of pension fund assets on economic growth in transition countries, emerging economies, and developed countries. Quantitative Finance and Economics, 2022, 6(3): 459-504. doi: 10.3934/QFE.2022020 |
[3] | Conrado Diego García-Gómez, Mehmet Huseyin Bilgin, Ender Demir, José María Díez-Esteban . Leverage and performance: the case of the U.S. hospitality industry. Quantitative Finance and Economics, 2021, 5(2): 228-246. doi: 10.3934/QFE.2021010 |
[4] | Filipe Sardo, Zélia Serrasqueiro . Intellectual capital and high-tech firms' financing choices in the European context: a panel data analysis. Quantitative Finance and Economics, 2021, 5(1): 1-18. doi: 10.3934/QFE.2021001 |
[5] | Patrick Mumbi Chileshe . Banking structure and the bank lending channel of monetary policy transmission: Evidence from panel data methods. Quantitative Finance and Economics, 2018, 2(2): 497-524. doi: 10.3934/QFE.2018.2.497 |
[6] | Emmanuel Nkansah . Does climate change exposure affect the intensive and extensive margins of corporate R&D investment?. Quantitative Finance and Economics, 2025, 9(2): 317-354. doi: 10.3934/QFE.2025011 |
[7] | Ramzi Drissi, Jamel Boukhatem . A nonlinear adjustment in real exchange rates under transaction costs hypothesis in developed and emerging countries. Quantitative Finance and Economics, 2020, 4(2): 220-235. doi: 10.3934/QFE.2020010 |
[8] | Michael Jacobs Jr., Frank J. Sensenbrenner . A comparison of methodologies in the stress testing of credit risk – alternative scenario and dependency constructs. Quantitative Finance and Economics, 2018, 2(2): 294-324. doi: 10.3934/QFE.2018.2.294 |
[9] | Tinghui Li, Jiehua Ma . Does digital finance benefit the income of rural residents? A case study on China. Quantitative Finance and Economics, 2021, 5(4): 664-688. doi: 10.3934/QFE.2021030 |
[10] | Md Qamruzzaman, Jianguo Wei . Do financial inclusion, stock market development attract foreign capital flows in developing economy: a panel data investigation. Quantitative Finance and Economics, 2019, 3(1): 88-108. doi: 10.3934/QFE.2019.1.88 |
We present an empirical test of a new measure to classify organizations according to the tangibility of product (output) flows delivered to customers. Our measure exhibits the empirical consequences of using standard industrial classifications to assume that firms within the same industry either share identical properties or sell homogeneous products. To illustrate the misleading findings that can result from these assumptions, we investigate whether prior literature on capital structure provides a sensible interpretation of organizational behavior, based as it often is on an assumption that all firms within a given industrial classification sell durable goods. In contrast to the product-market literature based upon the trade-off theory of capital structure, that would predict that firms selling physical goods will have proportionately less debt, in fact, when firms within industries are classified using our measure, we find to the contrary. Our intention is not to displace existing systems of industry classification but is, rather, to highlight the dangers of drawing conclusions from assuming homogeneity amongst firms which are formally registered within the same industry.
Researchers tend to categorize firms as being related by the process of producing and selling a specific goods and/or service. Any given firm is quantitatively described as belonging to a group of firms registered within a given industry (McNally & Eng, 1980; Shi et al., 2017). However, industries can have highly complex systems (e.g. Tether, 2003), and industry classification is not binary (Peneder, 2002); there are hundreds of 4-digit and dozens of 2-digit SIC [Standard Industrial Classification] codes.1 If we use a simple binary variable to identify a firm's characteristics we assume that firms registered in that industry either share similar properties or sell homogeneous products (Draper, 1975; Evangelista et al., 2015; Scellato, 2007). Hence, we fail to incorporate the richness and diversity of many organizational forms that can exist in the same industry, leading to potentially misleading conclusions.
1The full list of SIC codes can be found at https://www.osha.gov/pls/imis/sic_manual.html.
An alternative stream of research identifies the intangible elements necessary for economic production as intangible assets or knowledge (Hunter et al., 2012; Penman, 2009; Marrocu et al., 2012; Mathews, 2003; Wines & Ferguson, 1993). Furthermore, the production of services and other intangible products might be observed in almost every industry (Cardao-Pito, 2010, 2012a, 2012b, 2016, 2017; Penman, 2009). The production of intangible and semi-intangible products may require properties that are distinguishable from the production of physical goods in an industry, classified according to the tangibility of their product (output) flows (e.g. Penman, 2009; Shostack, 1977; Zeithmal et al., 1985; Tether et al., 2001; Miles and Tether, 2001; Tollington and Spinelli, 2012; Santamarıa et al., 2012).
We propose a new measure of firms, classified according to the tangibility of their product (output) flows to customers (Cardao-Pito, 2010, 2012a, 2012b, 2016, 2017). This allows us to: (i) measure the tangibility of product flows at the firm level, by their observable tangibility (e.g. cost of goods sold, or amortizations of physical investments); and (ii) deduce immateriality through the absence of observable tangibility.2 It is clear that a firm selling services is selling intangible products, from an accounting standpoint (Hunter et al., 2012; Lev et al., 2009; Penman, 2009; Wines & Ferguson, 1993), and that a firm selling physical goods, such as cars or clothes, is selling tangible products. The merit of this paper is in its ability to classify those firms which fall into the middle ground; that is, those selling a combination of both services and physical goods, or products that are mixed in themselves (Coombs et al., 2003).
2On a related theme, see Lev et al.'s (2009) interesting discussion of "organizational capital".
To illustrate the potential of our new measure, we revisit well-established research in capital structure theory, which has suggested that firms selling durable goods should be lower leveraged than others. This claim derives from the trade-off theory of capital structure, which suggests that capital structure and organizations would be irrelevant in a supposedly "perfect market". However, because markets are "imperfect", there would be a trade-off between the benefits and costs of debt. According to this theory, firms consider this trade-off in reaching an optimal capital structure. Because they require greater physical infrastructures, required by their tangible products, durable goods firms have higher bankruptcy, liquidation and financial distress costs. Therefore, if trade-off theory is correct, these firms would be expected to have proportionately less debt in their capital structure (Modigliani & Miller, 1963; Baxter, 1967; Frank & Goyal, 2009; Miller, 1977; Schleifer & Vishny, 1997; Titman, 1984; Titman & Wessels, 1988; Banerjee et al., 2008; Shi et al., 2017).
Several researchers have claimed evidence for this claim, using SIC codes to describe firms, but our new measure allows for clearer observation of the heterogeneity among firms within industries, which was previously impossible. Hence, we can investigate whether prior literature provides a sensible interpretation of organizational behavior, in assuming that all firms within a given SIC classification sell homogenous durable goods. Making reference to well-cited studies, we demonstrate that researchers may reach misguided inferences because of their assumption that firms formally registered within one industry should automatically share homogeneous features (Arce et al., 2015). We use specific papers to illustrate analyses undertaken using SICs, but appreciate that other work could equally be tested in this manner.
The use of Standard Industrial Classifications (SICs), persists today in quantitative research (Abor, 2007; Arce et al., 2015; Bhabra et al., 2008; Byard et al., 2017; Chan et al., 2007; Consoli & Elche, 2013; Dedman et al., 2009; Evangelista et al., 2015; Frank & Goyal, 2009; Glosser and Golden, 2005; Kaplinsky & Santos-Paulino, 2006; Mackay and Phillips, 2005; Orhangazi, 2008; Rauh and Sufi, 2012; Stadler and Nobes, 2014; Wang, 2009). However, this is to neglect the complexity that exists in actual industries, which might lead to inaccurate conclusions. We present below an example of capital structure research, which remains topical in academic literature (e.g. Al-Najjar and Hussainev, 2011; Cambini and Rondi, 2012; Jacob et al., 2011; Khalid, 2011; Korteweg, 2010; Mateev and Ivanov, 2011; Seelantha, 2010; His et al., 2017; Titman, 2002; Titman and Tsyplakov, 2007; Ughetto, 2008).
The claim regarding SIC codes that we test here is made by a number of researchers: viz. that firms selling durable goods will be lower leveraged than others (Titman, 1984; Titman and Wessels, 1988; Banerjee et al., 2008). While some now acknowledge that additional factors impact a firm's capital structure (e.g. Fan et al., 2012; Korteweg, 2010), we argue that others are still making inappropriate use of methods of industry classification. Researchers such as Acha et al. (2004) have attempted to incorporate more detailed industry classifications into their analysis, but they remain reliant upon SIC codes, because "they are the most widely available industry classifications covering our sample period" (Chava and Jarrow, 2004).3
3Note that these authors then merge 10 classifications into 4 (miscellaneous; manufacturing & mineral; transportation, communication & utilities; and finance, insurance & real estate), for ease of analysis, but in detriment to a more refined piece of work.
Our example comes from a series of studies used to defend the trade-off theory of capital structure, originating in Modigliani and Miller's (1958) seminal work (Titman, 2002), assuming the irrelevance of operating decisions; i.e., the left-hand side of the balance sheet is irrelevant to its right-hand side, and vice versa. However, we know that capital structure is not empirically irrelevant and is strongly correlated with other economic characteristics of firms (e.g. assets, profitability, Rajan and Zingales, 1995). To explain the empirical significance of capital structure, Modigliani and Miller (1963) introduced their trade-off theory, arguing that, in a "perfect market", capital structures and organizations would be irrelevant. However, because "markets are imperfect", there is a trade-off between the benefits and costs of debt. Firms assess this trade-off to reach an optimal capital structure. Accordingly, there are three leading market imperfections: taxes and regulations; transaction costs of financial distress and bankruptcy; and agency costs (see also Modigliani and Miller, 1963; Baxter, 1967; Miller, 1977; Shleifer and Vishny, 1997).
The claim that firms selling durable goods should have less debt than others is used in previous research to support the trade-off theory of capital structure, as the importance of products (as captured by SIC codes) is said to be indirectly reflected in a firm's liquidation and/or bankruptcy costs (Titman, 1984). In this way, Titman's (1984) objective was to explore "one source of contracting costs … [and therefore 'market imperfections'] … which is indirectly related to bankruptcy (and liquidation costs)".
Several aspects of trade-off theory have already been empirically refuted. For instance, it has been found that firms with higher profitability tend to have less debt (e.g. Rajan and Zingales, 1995; Dempsey, 2013, 2014; Fama and French, 2002). This is contrary to the trade-off prediction that more profitable firms will borrow more in order to obtain tax benefits. Furthermore, surveys of financial managers show that practitioners do not always adhere to capital structure theories of financial economics (Beattie et al., 2006; Cohen and Yagil, 2007; Correira and Cramer, 2008; Graham and Harvey, 2001; Volpe and Woodlock, 2008).
Titman's (1984) paper is a theoretical contribution, which does not empirically test its predictions. That falls to subsequent papers (e.g. Banerjee et al., 2008; Kale and Shahrur, 2007; Titman and Wessels, 1988), which follow the same reasoning to interpret their empirical findings. However, these authors studied firms listed on stock exchanges, and we know that the liquidation or bankruptcy of a firm listed on a stock market is not a frequent event.4 For instance, Chava and Jarrow (2004) studied listed American companies over the period 1962-1999, finding that the mean yearly bankruptcy, as a percentage of total active firms, was merely 0.65% (median: 0.56%). As the risk of bankruptcy for listed firms seems to be low, provision for financial distress might not be a priority. Further, Andrade and Kaplan (1998) argue that financial distress is negligible for firms that do not face adverse economic shocks, even if they are highly leveraged.
4The same might not apply to firms that are not listed in capital markets.
In exploring Titman's (1984) predictions that firms selling durable goods are lower leveraged, Titman and Wessels (1998) identify durable-good-selling firms by including "a dummy variable equal to one for firms with SIC codes between 3400 and 4000 (firms producing machines and equipment) and zero otherwise as a separate attribute affecting the debt ratios". By definition, all firms registered within these industries therefore produce machines and equipment and, according to Titman and Wessels (1988) "firms manufacturing machines and equipment should be financed with relatively less debt" (in comparison to firms that do not produce machines and equipment). Banerjee et al. (2008) accept and replicate this use of a binary measure of industry classification in their own subsequent work.
While the use of SIC codes is recognized and common-practice, we argue that it ignores the possibility of firms operating across sectors, for example, by providing a combination of both manufactured products and associated services, or other intangible products (Coombs et al., 2003; Cardão-Pito, 2010, 2012a, 2012b, 2016; Hunter et al., 2012; Penman, 2009; Tollington and Spinelli, 2012). It is possible that, within the same industry, firms can exhibit extreme heterogeneity in the products they sell, or the intangible assets, such as labor, they possess (Glosser & Golden, 2005). This point is ignored by those who also fail to consider the substantial heterogeneity among industries. Further investigation is justified into why physical-good intensive firms that require substantial investments in machinery, plant, equipment, stock of goods, and raw materials need less debt than semi-intangible or intangible-product-intensive firms which, having fewer material requirements, could be more easily self-financed.
The relationship between product flows and a firm's capital structure is reinforced by the operational needs inherent in the materiality of the flows of products carried through commercial transactions (Cardao-Pito, 2010, 2012a, 2012b, 2016, 2017). For instance, we cannot simply dismiss the fact that, in order to produce an automobile, a firm not only requires heavyweight machinery, storage facilities and quite tangible raw materials, but also intangible flows of work associated with the production of such machines; whereas in order to provide an intangible service, another firm might need only convenient space and a few employees.
This need for additional operational inputs is not considered explicitly by earlier work in financial economics. On the other hand, interdisciplinary literature on the subject of products and their definitions does consider whether or not physical products, such as food or cars, can be distinguished from intangible products, such as services or software (Cardao-Pito, 2010, 2012a, 2012b, 2016, 2017; Hunter et al., 2012; Levinthal, 2006; Lovelock and Gummeson, 2004; Lovelock and Wirtz, 2011; Miles and Tether, 2001; Nelson and Winter, 1982; Parasuraman et al., 1985; Penman, 2009; Rathmell, 1966; Santamarıa et al., 2012; Tether et al., 2001; Winter, 2006; Zeithmal et al., 1985). Further, it is acknowledged that products can be classified according to their degree of tangibility (Shostack, 1977), ranging from those observed to be "tangible dominant" (e.g. salt or soft drinks) to those which are "intangible dominant" (e.g. services, such as consulting or teaching). Shostack's classification of products according to their intangibility is reproduced in Figure 1, with products appearing on a continuum from "tangible dominant" to "intangible dominant". In the middle of the scale are products that include both tangible and intangible elements. For example, the provision of meals in fast-food outlets requires a combination of tangible products (viz. food and drink) and intangible services, such as waitressing and marketing. Figure 1 does not have a Y-axis, because Shostack advanced a theoretical system for classifying products according to their tangibility without addressing the question of its measurement. He classified soft-drink companies, such as Coca-Cola and Pepsi-Cola, on the tangible-intensive side of products. However, our new measurement system shows that the business models of such firms are highly dependent on intangible components; for instance, on marketing or brand awareness. Likewise, although Shostack classified airline companies on the intangible-dominant side of the product continuum, airlines are highly dependent upon physical elements such as planes, airports, and expensive jet fuel.
Intangible flow theory (Cardao-Pito, 2010, 2012a, 2012b, 2016, 2017) was developed as a means of appreciating that flows of material elements (such as physical goods or cash) are consummated by human related intangibles (Peneder, 2002). Compare, for example, Becker (1962), who discusses the importance of investing in human capital (Peneder, 2002). Marrocu et al. (2012) show how intangible, or knowledge-based, capital (viz. "information" or "communication flows") affects a firm's productivity. And Mathews (2003) discusses a resource-based view of firms and the economy, which captures the dynamics and change of intangible, as well as tangible, assets and goods (e.g. "service flows"). These intangible flows cannot be precisely appraised at an actual, or even an approximate value, and have properties precluding their classification as either assets or capital. This theory uses the "precision" approach to define tangibility, as something which can be identified with precision, and not simply because it can be touched, as in an alternative definition.5 Cash flows which occur at a precise moment in time are deemed to be highly tangible, whether or not a digital means of payment is involved (e.g. by credit card, or through an on-line transaction). On the other hand, predictions about eventual future cash flows are considered to be highly intangible, where they cannot be established with precision. Furthermore, the theory accepts that we can never measure intangibility. Instead, the best we can do is to transform previously intangible dimensions into tangible dimensions, by measuring them with precision; hence the paradox of measuring intangibility (Bloom, 2009).
5tangible adj. capable of being touched; affecting the sense of touch; touchable (Oxford English Dictionary, 2012; OED.com).
To summarize the mechanics of intangible flow theory (Cardao-Pito, 2010, 2012a, 2012b, 2016), consider the example of a customer who buys a product from an organization. The customer, through his actions enters into a chain of intangible flows. For example, the organization places an advertisement about the product (information flow), and the customer reads the advertisement (communicational flow). He then goes to the store to buy the product from a sales assistant (service flow), who enters the storage room to retrieve the particular item (work flow). At the same time, the customer will enter a tangible flow process, in exchanging tangible cash for the physical good and the associated service provided. Alternatively, we might consider the case where a customer pays for a tangible flow in the form of a physical good, or for an intangible product in the form of a service, or a mixed product in the form of a mixture of physical good and service, or a hybrid product.
For a large sample of organizations, observed over a long period of time, Cardao-Pito (2010) discovered that the tangibility of product flows is systematically (and positively) associated with capital structure. His findings are not compatible with the prediction that firms selling physical goods should be lower leveraged, if we accept that durable goods must be highly tangible. We therefore adopt the measure of operating intangibility to investigate whether the firms analyzed by the likes of Titman and Wessels (1988) and Banerjee et al. (2008) are actually physical good intensive firms. We propose that, if our analysis allows us to identify the subsample of firms that are physical-good intensive, we would then wish also to examine whether those firms have more or less debt in their capital structure than others, in order to compare our results with prior research on capital structure in organizations (Findlay & Williams, 1987).
Considering the possible complexities of goods and services that can occur, the interesting question arises as to how we might classify corporations according to the tangibility of the flows of products they provide to their customers. The concept of operating tangibility aims to address this question by considering the weight that intangible activities carry in the productive process of an organization. Operating intangibility is inferred through the absence of tangibility in the total operating costs, in the form of cost of goods sold and amortizations of property, equipment and facilities. Specifically, it may be measured as follows:
(1) |
(2) |
(3) |
In Equation (3) above we capture the weight that intangible related expenses (e.g. selling, R & D, administration, marketing, personnel expenses) carry of total operating costs. Thus we can infer a firm's level of operating intangibility, from the financial data readily available in its balance sheet and/or income statement. As we explain above, intangibility cannot be measured using a "precision" approach. Instead, here we use relevant tangible elements as a proxy for the intangibility of operating product flows. Xl4me.
Another key variable in this study is DEBTLEVERAGE, which describes the proportion that debt carries in the capital structure of firms [leverage]. We divide total debt by total capital (debt plus equity) and study the relationship between LOI and DEBTLEVERAGE vis-à-vis previous findings of the product market literature described above. We use control variables to report mediating effects, which make reference to standard research in capital structure studies (e.g. Khalid, 2011; Mateev and Ivanov, 2011; Baker and Wurgler, 2002; Dempsey, 2013, 2014; Fama and French, 2002; Rajan and Zingales, 1995). These are as follows:
1. LOI = general proxy for the Level of Operating Intangibility [1 - (cost of goods sold + amortization and depreciation of fixed assets)/(total operating expenses)].
2. DEBTLEVERAGE = ratio of total debt to equity plus total debt.
3. INTANGIBLE_ASSETS = ratio of intangible assets to total assets on the balance sheet.
4. SIZE = the log of firm's sales, after sales values have been deflated by the consumer price index (CPI). The CPI data were obtained from the Reuters' Datastream database (Datastream International).
5. CAPEX_PPE = capital expenditure on property, plant & equipment, expressed as a fraction of total assets.
6. PROFITABILITY = net income/total assets.
7. MARKET_TO_BOOK = (market value of equity)/(book value of equity).
To enable replicability testing, Appendix A explicitly identifies the data-mnemonics to create these variables from the merged CRSP-COMPUSTAT industrial database.
To confirm our findings we used a number of procedures, which produced similar results, including: Fama & Macbeth (1973) regressions with Newy & West (1987) t-values (creating yearly regressions for each of the 41 years, and a final averaged regression model), with several control variables; and random effects models, using general least squares, and maximum likelihood estimators. To examine whether our findings are being driven by extreme observations, we repeated the tests on a sample with outliers omitted. We eliminated extreme observations for size, profitability, leverage, market-to-book, investments in tangible fixed assets and intangible assets which fell into the top or bottom 1% of observations. When reporting regressions, we describe the results both with and without outlier observations. Moreover, we describe residual behavior for each regression.
Our findings are based upon all usable industrial data observations in the merged CRSP-COMPUSTAT database6 for firms listed on the three major US stock exchanges, namely NYSE, AMEX and NASDAQ, during the years 1966-2006. To compute our key variable, LOI, according to the definition above, we have excluded observations with missing values in the variables necessary to compute this variable LOI. We also exclude observations with either missing or negative values for total assets, debt or equity.
6 Source: CRSP, Center for Research in Security Prices. Graduate School of Business, The University of Chicago [2006]. Used with permission. All rights reserved. www.crsp.uchicago.edu.
A total of 97,660 observations fulfil the conditions for the variables employed in this study. Of these observations only 5 observations ( = 0.005%) were deleted because LOI could not be computed, or was located outside the interval [0, 1]. The number of LOI observations outside the expected interval is tiny, hence not materially relevant. The main sample contains annualized data for 9607 firms, or 97,655 observations in total. This paper reports the findings obtained from this sample. Table 1 presents descriptive statistics for these variables. The LOI variable has a mean value of 0.263 and standard deviation of 0.166 for the full sample, which is to say that the sample's firms devote on average 73.7% of their operating costs to costs with goods sold and amortizations and depreciations.
Variable | Mean | Std. Dev. | 25 th Pctl. | Median | 75th Pctl. |
LOI | 0.263 | 0.166 | 0.140 | 0.230 | 0.350 |
DEBTLEVERAGE | 0.320 | 0.236 | 0.116 | 0.308 | 0.483 |
INTANGIBLE_ASSETS | 0.064 | 0.124 | 0.000 | 0.006 | 0.067 |
SIZE | 5.713 | 1.867 | 4.361 | 5.564 | 6.909 |
CAPEX_PPE | 0.070 | 0.076 | 0.023 | 0.050 | 0.092 |
PROFITABILITY | 0.064 | 0.190 | 0.020 | 0.054 | 0.090 |
MARKET_TO_BOOK | 3.528 | 73.553 | 1.055 | 1.693 | 2.792 |
The division of a sample into quintiles is well established in prior research (e.g. Lazonick and Mazzucato, 2013; Bellone et al., 2008; Dempsey, 2013, 2014; Fama and French, 2002; Baldwin and Sabourin, 2002; Dobrev, 1999). We have also implemented a quintile analysis to explore the nature of the relationship between LOI and DEBTLEVERAGE. The approach we adopt below is to examine, in a novel way, the intangibility of organizations and the ways in which our measure of intangibility identifies the tangibility of product flows. Our observations are grouped into quintiles, according to the mean measure of the level of operating intangibility (LOI), computed using all sample observations. The entire sample is therefore subdivided into five sets, and firms with analogous mean levels of operating intangibility are grouped together. The total 9,607 firms in our sample have been divided by quintile. Each quintile contains approximately the same number of firms viz. 1,921 or 1,922. When a firm is classified into one quintile, all of its observations are classified in that same quintile.
Table 2 describes the allocation of firms and the respective number of observations, mean-LOI and LOI-variability, by quintile. Column 1 numbers the quintiles 1 to 5, with quintile 1 containing those with the lowest mean-LOI, and 5 containing those firms with the highest mean-LOI. In column 2, we have the number of firms appearing in each quintile. The number of observations is given in column 3. Discrepancies between the numbers of observations appearing in each quintile are explained by the different number of annualized observations available for each firm. The mean LOI, which must, by definition, fall between zero and 1, is given in column 4, and ranges from 0.09 (highly tangible in quintile 1) to 0.555 (highly intangible in quintile 5). This is followed by the standard deviations. The 25th percentile, median and 75th percentile measures are given in the final three columns.
Quintile | Firms | Observations | Mean | Std. Dev. | 25th Pctl. | Median | 75th Pctl. |
1 | 1,921 | 21,629 | 0.090 | 0.042 | 0.061 | 0.089 | 0.116 |
2 | 1,921 | 23,414 | 0.178 | 0.049 | 0.149 | 0.176 | 0.204 |
3 | 1,922 | 21,185 | 0.261 | 0.058 | 0.227 | 0.257 | 0.291 |
4 | 1,921 | 17,779 | 0.363 | 0.073 | 0.319 | 0.359 | 0.403 |
5 | 1,922 | 13,648 | 0.555 | 0.141 | 0.461 | 0.527 | 0.623 |
Total | 9,607 | 97,655 |
Using the above measure of LOI, Cardao-Pito (2010) classifies several specific well-known firms, according to their intangibility, on a scale inspired by Shostack's (1977) generic earlier classification [see Figure 2]. To the left, at one end of the scale, are the physical good intensive firms, which include Ford (auto manufacturer) and Chevron (Oil Production). On the right, at the other extreme, the measure of LOI classifies intangible product intensive firms, such as Microsoft (computer software, licensing and support) and Yahoo (web browser and online services). In between, we have, for example, General Electric (whose products include a wide range of tangible and intangible goods, such as appliances, aviation, consumer electronics, electrical distribution, electric motors, energy, entertainment, finance, gas, healthcare, lighting, locomotives, oil, software, water, weapons, wind turbines), and AT & T (a multinational telecommunications corporation, providing both mobile telephony and fixed telephony, and a broadband subscription television service).
Figure 2 allows us to identify what can be a misconception about products from some financial researchers, who take the stance that firms selling durable goods should have less debt in their capital structure than others. Following Titman's (1984) theoretical reasoning, firms such as computer and automobile companies would be considered to be durable goods firms. On the other hand, firms like hotels and retail establishments would be classified as non-durable goods firms. The scale shown in Figure 2 would indeed place firms producing automobiles and computers on the physical good (tangible) intensive side of the operating intangibility scale. However, hotels and retail establishments can also be reliant on durable goods. For example, the Hilton Hotels Corporation would traditionally be considered to be a pure service firm. But consider that networks of hotels rely intensively on physical and durable commodities to generate their cash flows; hotels need the physical buildings, the land, and the furniture, in order to provide their service. Hence, as shown in Figure 2, the Hilton Hotel Corporation's mean Level of Operating Intangibility is slightly higher than that of Chevron. Likewise, Wal-Mart, a supermarket chain, is placed on the physical-good intensive side of the scale, because the physical goods component of its sales is substantial.
Previous research might not have captured this essential point. While a consultancy firm may sell highly intangible services, networks of hotels sell services that are associated with a high degree of tangibility of physical goods, which are essential to the service provision. Similarly, the majority of sales of cars and computers to the final customer come most often from retail outlets that traditionally would be considered to be intangible service providers. However, their business model is essentially based upon trading physical goods. Hence this paper uses an alternative conception of product flows and, therefore, an alternative definition of "durable good firms", to those used in earlier work to examine whether physical good firms have more or less debt in their capital structure.
Note that Figure 1 represents a theoretical system for classifying products according to their tangibility. However, it does not contain a y-Axis because, as we have noted earlier, Shostack did not suggest an actual measurement system for that theoretical proposal. On the other hand, Figure 2, developed from our own work does indeed contain a y-Axis, because we are suggesting a concrete system for measuring the tangibility of a firm's product (output) outflows delivered to customers. Furthermore, our new measurement system allows us to correct some misinterpretations in Shostack (1977), such as classifying marketing and brand-intensive companies like Coca-Cola on the tangible-dominant side of products; or airline companies, that are highly reliant on physical planes, airports and jet fuel, on the intangible-dominant side.
Titman and Wessels (1988) and Banerjee et al. (2008) used SIC codes ranging from 3400 to 4000 to identify their sample of firms selling durable goods. This ignores firms in, for example, heavy construction industries (SIC = 1600), household furniture (SIC = 2510), leather products (SIC = 3100), and wholesale-durable goods (SIC = 5000).
Our sample contains 2,298 firms ( = 27,454 observations) registered with codes between 3400 and 4000 i.e. identified by previous authors as durable goods selling firms. By choosing this set of industry codes we could be misled to believe that firms selling durable goods have less debt than others because the mean DEBTLEVERAGE in this subsample ( = 0.26) is lower than the mean DEBTLEVERAGE of the entire sample ( = 0.32). Figure 3 plots the distribution of the firms, according to the level of operating tangibility we describe above. We find that those firms with SIC codes between 3400 and 4000, that appear in the lower LOI quintiles, corresponding to the most physical-good intensive firms, are in the minority. Only 290 ( = 13%) firms are classified according to the most material goods LOI quintile, and only 799 ( = 35%) are classified in quintiles 1 or 2. The remaining 1,499 ( = 65%) firms are classified into the higher LOI quintiles (3-5), which correspond to the semi-intangible and intangible product intensive firms. Figure 3 displays how firms registered with SIC codes between 3400 and 4000 are distributed, compared to the full sample of all firms, and to firms without codes between 3400 and 4000.
For the same sub-sample of firms with SIC codes 3400 to 4000, Figure 4 plots the mean DEBTLEVERAGE, by LOI quintile. We find empirical support for the hypothesis that firms selling flows of material goods to their customers will tend to have more debt leverage in their capital structure than firms that are not focused on selling physical goods. This is to contradict the prediction of the product market literature, but it also consistent with recent findings, such as those of Fan et al. (2012) who find a positive relationship between leverage and asset tangibility (also Khalid, 2011; Zhang, 2010); that it, those with greater physical assets have higher levels of debt relative to equity. As Figure 4 shows clearly, the mean debt leverage for the firms in this sub-sample tends to remain relatively stable for the LOI quintiles 1 and 2, at mean values of 31% and 31%, respectively, then starts to decrease significantly after LOI quintile 3, as the level of intangibility increases. At quintile 5 the mean debt leverage is only 15%, a similar pattern to the full sample containing all firms. It is clear therefore that many firms selling semi-intangible and intangible-intensive products (outputs) are registered with an industry code (SIC) lying between 3400 and 4000. Furthermore, although mean DEBTLEVERAGE is lower for the subsample of firms registered in those industries, the decrease in mean DEBTLEVERAGE from quintile 1 to quintile 5 is similar to that for the full sample and for firms that do not have an industry code (SIC) between 3,400 and 4,000 In these three cases, firms that are tangible intensive (quintile 1 and 2) have mean DEBTLEVERAGE higher than firms captured in the most intangible intensive quintile (quintile 5). In quintile 1, the mean DEBTLEVERAGE is 36% for the full sample, 31% for firms with SIC codes between 3,400 and 4000, and 37% for the other firms. By contrast, in the most intangible-intensive quintile 5 the mean DEBTLEVERAGE is 21% for the full sample, 15% for firms with SIC between 3,400 and 4000, and 23% for the rest. Hence, the assumption that firms in the industries chosen by earlier authors have a homogeneous debt profile is not supported by LOI quintile analysis. Furthermore, the claim that firms selling durable goods have less debt in their capital structure than firms that do not sell durable goods is empirically refuted.
Table 3 presents the Spearman correlations for the variables studied. As expected, the LOI variable has a significant negative correlation with debt leverage (ρ = −19.7%, p < 0.001). One matter worthy of further investigation is the connection between LOI and debt over time, given the range of our sample (1966-2006). Thus we created a sub-sample for each year. Figure 5 displays the mean values for LOI and DEBTLEVERAGE in the yearly sub-samples. As we observe, there is limited variation in the mean DEBTLEVERAGE. During the 41 years of the sample, the highest annual mean value for debt as a proportion of the capital structure is 36%; whereas the minimum mean value is 27%. On the other hand, we identify a clear pattern of increasing annual mean values for the variable LOI. In 1966, for the sample firms, the mean value for LOI was 19%; whereas between 2000 and 2006 the mean value for this variable ranged from 28% to 32%. This change may arise from different types of firms entering the stock market and, therefore, the sample, given that the LOI value by firm tends to be very stable over time (Cardao-Pito, 2010).
LOI | DEBTLEVERAGE | INTANGIBLE_ASSETS | SIZE | CAPEX_PPE | PROFITABILITY | MARKET_TO_BOOK | |
LOI | 1.00 | ||||||
DEBTLEVERAGE | −0.197 | 1.00 | |||||
INTANGIBLE_ASSETS | 0.058 | 0.106 | 1.00 | ||||
SIZE | −0.267 | 0.178 | 0.212 | 1.00 | |||
CAPEX_PPE | −0.247 | −0.0611 | −0.112 | 0.170 | 1.00 | ||
PROFITABILITY | 0.040 | −0.501 | −0.071 | 0.031 | 0.276 | 1.00 | |
MARKET_TO_BOOK | 0.252 | −0.150 | 0.097 | 0.061 | 0.090 | 0.377 | 1.00 |
We further investigate the Spearman correlations between LOI and DEBTLEVERAGE for the different subsamples, by year [see Figure 6]. In each year, the Spearman correlation between these two variables is negative. The correlation is always above 10% in absolute terms for each of the 41 years. There are only 11 years [ = 27%], where this correlation is lower than 15%. In the other years, this correlation is systematically higher than 15%. Perhaps the population of intangible intensive firms listed on US stock markets before the 1980s is limited. Possibly therefore, firms listed before the 1980s were mostly physical good intensive firms. As Figure 6 shows us, at the beginning of the 1980s, the mean LOI starts increasing as well as the Spearman correlation between LOI and DEBTLEVERAGE. There is a slight decline in correlations after 2000, possibly following the Dotcom crash, when several high-technology firms left the market. However, from 2003 on the correlations again increased, in absolute values, on an annual basis.
Our analyses employed regressions, controlling for fixed effects (FE) of years and individual effects of firms (Balestra and Krishnakumar, 2008; Baltagi, 2008; Matyas and Sevestre, 2008), in order to ensure that our results are not driven by specific years or specific firm effects (Cardao-Pito, 2017; Petersen, 2009). Wooldridge (2002) suggests that a FE model is well suited to unbalanced panel data samples, such as ours (Orhangazi, 2008). Fixed effect estimators could be consistent and asymptotically normal estimators for unbalanced panel data, given that certain conditions discussed in Wooldridge (2002) are verified. However, FE requires that we have at least three observations for each cross section [firm] (T > 2), as a minimal condition to be computed. To compute panel data we use the Procedure Panel in SAS, which for consistency of findings only accepts cross-sections [firms] of at least 4 observations (T > 3). Hence, in producing FE models, we only use firms having 4 or more yearly observations. Furthermore, in order to test whether our findings might be driven by extreme observations, we test our FE model both including and excluding outlier observations from the sample. Besides describing the size and number of firms in each sample tested, Table 4 describes the average and standard deviation of the number of observations by firm, in each case tested. For the four samples tested, the average number of observations by firms is between 12.7 (s.d.:8.7) and 14.5 (s.d.:9.5). Some observations have been deleted for missing the required information, or a firm may have opted to be out of the stock market for a period; and in two sub-samples outliers have been removed.
Full Sample | Sub sample of firms with Industry Code (SIC) between 3,400 and 4,000 | ||||
Computation A: All observations | Computation B: Removing outliers | Computation C: All observations | Computation D: Removing outliers | ||
Intercept | 0.254*** | 0.239*** | 0.245*** | 0.259*** | |
(4.18) | (4.40) | (3.53) | (4.19) | ||
LOI | −0.065*** | −0.077*** | −0.196*** | −0.226*** | |
(−7.36) | (−8.87) | (−9.92) | (−12.23) | ||
INTANGIBLE_ASSETS | 0.285*** | 0.253*** | 0.355*** | 0.329*** | |
(41.25) | (35.92) | (26.80) | (26.04) | ||
SIZE | 0.013*** | 0.020*** | 0.006*** | 0.012*** | |
(13.45) | (22.00) | (3.46) | (7.46) | ||
CAPEX_PPE | 0.080*** | 0.099*** | 0.046** | 0.082*** | |
(8.76) | (9.53) | (2.16) | (3.91) | ||
PROFITABILITY | −0.119*** | −1.324*** | −0.136*** | −1.268*** | |
(−36.84) | (−117.19) | (−23.68) | (−68.00) | ||
MARKET_TO_BOOK | 0.000*** | 0.021*** | 0.000*** | 0.020*** | |
(7.10) | (57.59) | (7.14) | (30.39) | ||
R-Square | 0.694 | 0.7481 | 0.6071 | 0.6744 | |
F-Value | 23.92 | 21.19 | 15.62 | 15.49 | |
Observations | 92,477 | 84,960 | 26,444 | 24944 | |
Firms | 7,070 | 6,686 | 1,812 | 1,759 | |
Average number of observations by firm | 13.1 | 12.7 | 14.6 | 14.1 | |
St. deviat. of observations by firm | 9.0 | 8.7 | 9.5 | 9.3 | |
Fixed effects for firms | yes | yes | yes | yes | |
Fixed effects for years | yes | yes | yes | yes | |
Beginning Year | 1966 | 1966 | 1966 | 1966 | |
End Year | 2006 | 2006 | 2006 | 2006 | |
Notes:
1. The models were computed using a fixed effects/panel data specification controlling the fixed effects of firms and years. 2. T-test results are given in brackets: *Significant at 10% level; **Significant at 5% level; *** Significant at 1% level. |
Employing the variables described in Section 3.1 and Appendix A, we tested the following regression model:
(4) |
Accordingly, we have produced four different computations: A: full sample with all observations; B: full sample removing outliers; C: Subsample of industries SIC [3400, 4000] with all observations; and D: Subsample of industries SIC [3400, 4000] removing outliers. The results are presented in Table 4, which shows the relationship between the tangibility of the flows of products sold by firms to their customers and the proportion of debt in the firms' capital structures. This is similar for both the full sample and the sub-sample of firms with a SIC code between 3400 and 4000, and it therefore questions the prediction derived from the trade-off theory of capital structure discussed earlier. In each of the four computations, the goodness of fit (R2) is significant, ranging from 0.61 to 0.75.
The level of operating intangibility is consistently strongly negatively correlated with having lower leverage, despite including control variables that are common in capital structure literature. For the full sample, the coefficient is −0.065, with a strong t-value of −7.36. This association increases in computation B where outliers are removed from the full sample, where we find a coefficient of −0.077 and t-value of −8.87. Moreover, the association between LOI and DEBTLEVERAGE is even stronger in the subsample containing only firms registered in industries with SIC codes between 3400 and 4000. In computation C, the coefficient is −0.196 and the t-value −9.92. Excluding outliers, we find a coefficient of −0.226 with a t-value of −12.23. Therefore, these findings give us further empirical support for the hypothesis that higher product flow intangibility is associated with higher debt leverage; and thereby empirically refute the prediction of the existing product market literature that firms selling durable goods have less debt.
Although we do not wish to establish a predictive model of the association between the tangibility of a firm's flows of products and its capital structure, there are at least two logical channels through which the materiality of a firm's flows of products might directly or indirectly affect its capital structure. First, the collateral value associated with physical-good tangibility and related investments offers protection against default to lenders when negotiating debt contracts (see Jimenez et al. 2006). However, the inherent characteristics of services preclude their consideration as assets. Therefore, creditors cannot take possession in the same manner in which they would hold material economic elements such as physical goods or cash. Second, self-financing is generally considered to be the preferred source (e.g. Graham and Harvey, 2001; Fama and French, 2005), and product sales are the principal mechanisms for generating the operating cash in-flows. If higher intangible-product-intensive firms could more often finance their investments without obtaining external capital (e.g. through debt, equity or hybrid securities), then this ability could have an impact upon their capital structures.
Computed through the Procedure Panel in SAS, Figure 7 displays the behavior of the residuals in the four computations in Table 4. In all cases, and as predicted by Wooldridge (2002), the residual behavior is consistent and asymptotically normally distributed and the mean square error is near zero. However, when including outliers in the sample, a few extreme error terms are far from the mean of zero. The exclusion of outliers in computations B and D eliminates those extreme error term observations, and significantly restricts the dispersion of the error term distribution. Moreover, as we have seen above, the elimination of outliers increases the strength and significance of the association between LOI and DEBTLEVERAGE, which is already quite robust when outlier observations are included.
A limitation of this study is identified at the core of intangible flow theory: material elements are consummated by human related intangible flows that cannot be precisely appraised at an actual or approximate value, and have properties precluding their classification as assets or capitals. Thus, although mathematical and quantitative research methodologies are highly relevant for science, they are inadequate when it comes to the study of economy and society (Cardao-Pito, 2010, 2012a, 2012b, 2016, 2017). To investigate industries, we cannot simply use quantitative methods. The most well-crafted empirical analysis could not substitute for direct observations, surveys, ethnographic analysis, or case studies of actual industries. By definition, intangibility cannot be measured with precision. Thus, it cannot be perfectly captured by quantitative methods that rely upon precise measurements.
We have made use of a method to demonstrate an empirical correlation not compatible with published prescriptive predictions of product market research. However, we are aware that we use an approximation to infer the intangibility of operating product flows, which is not complete. We can only measure tangible flows e.g. of material elements, such as cash flows or physical goods. Hence, we accept that there is endogeneity in the relationship between our key variables of LOI and DEBTLEVERAGE. Endogeneity in a variable may arise from three major sources, or combinations thereof; errors-in-variables; omitted variables; or simultaneous causality between variables (Bascle, 2008; Wooldridge, 2006; Hamilton and Nickerson 2003; Shaver, 1998). In our view, all these issues arise when one deals with intangibility. Errors-in-variables and omitted variables naturally arise from the fact that intangibility cannot be measured with precision (cf. Wines & Ferguson, 1993). Further, the quantification may rely upon insufficient accounting data, as is generally the case with the other variables. Moreover, it is quite difficult to disentangle isolated causality when considering non-measurable intangibility.
Hence, we can display a useful correlation to a certain degree. As a second contribution, we have provided empirical evidence against a component of the trade-off theory of capital structure. We have conclusively refuted the claim that firms selling durable goods will have less debt in their capital structures. However, we cannot arrive at a conclusion about the direction of causality; that is, in identifying whether operating intangibility is a cause of debt in the capital structure, or vice versa. Nevertheless, in this study we are not attempting to identify a predictive model to calculate a firm's capital structure. The LOI variable is itself working as a proxy and instrumental variable for the level of operating intangibility, which can be improved in future research. Although our analysis has included linear regression models traditionally used in accounting, finance and management research (Dyckman, 2016), the relation between LOI and capital structure might be better captured through non-linear models. Indeed, Figure 8 presents a scatter-plot graph with the sample observations for the variables DEBTLEVERAGE and LOI, alongside a simple OLS regression for these two variables. As the graph exhibits, the linear regression might not be a perfect method for capturing the systematic curve observed in the graph; whereas for an LOI sensibly higher than 30% the behavior of the relationship between LOI and DEBTLEVERAGE tends to follows a curve through which DEBTLEVERAGE starts to decrease as LOI increases.
Our analysis was not restricted to linear regression models; instead, we intend to demonstrate that there is a relevant empirical correlation between the tangibility of product flows and the capital structure, which is not compatible with the prediction that firms selling durable goods must have less debt in their capital structure. Therefore, the relevant correlation found is an appreciable addition to the product market and capital structure theory literature.
Academics and practitioners alike can benefit from a deeper understanding of the complex dynamics by which firms in the same industry both compete and cooperate, as well as the intricate environment that might exist within each specific industry. The research of industries can greatly benefit from both quantitative and qualitative research methods. Furthermore, industries can be studied from different perspectives (e.g. Mazzoleni and Nelson, 2013). We would by no means propose that industry classification systems ought to be abandoned.
Nonetheless, we have shown that the common practice of assuming that SIC industrial classifications imply that same-industry firms automatically share identical properties or sell homogenous products is not merely a matter of empirical convenience. In fact, that assumption may have consequences for the inferences obtained by researchers. The example presented in this article was obtained from capital structure research and it adds another argument to empirically contradict Modigliani and Miller's (1963) trade-off theory developing from their work in 1958.
The findings of our empirical investigations do not support the prediction that firms selling durable goods will have a lower proportion of debt than others, due to trade-offs involving bankruptcy costs. In previous work (e.g. Titman, 1984; Titman and Wessels, 1998; Banerjee et al., 2008), researchers used certain industries to proxy for durable goods firms. However, our analysis finds that physical good-intensive firms are actually much fewer in number than intangible product-intensive firms and, indeed, the former have a greater proportion of debt in their capital structure, in contrast to the findings of earlier studies. As such, we suggest that the industrial classification of firms by SIC, as used by many researchers, is an insufficiently precise or accurate measure of what actually happens in organizations and industries. It simply cannot be used to assume that firms registered in the same industry will automatically have similar properties. Hence, we provide empirical evidence to refute this component of the trade-off theory of capital structure. Besides introducing a specific example, perhaps we have inaugurated a new line of research that could inquire into whether the industry homogeneity assumption might have misguided researchers in other studies.
We have employed a novel method for capturing both the physical and intangible attributes of firms and industries that a single SIC code cannot possible encompass on its own. Our measure enables the researcher to take a variety of evidence, in a relatively simple and straightforward manner, from published financial statements, and to use this evidence to determine the level of tangibility (or intangibility) inherent in a particular organization. Whether we have firms that provide purely services, firms which are in manufacturing, or a hybrid of the two, we can use this measure to analyze organizations and/or the industries in which they operate. This method is to be used not as an alternative but as a complement to other research and management tools. As explained by intangible flow theory, by definition, intangibility cannot be measured with precision. We can only precisely quantify the tangible elements associated to intangibility in order to infer it. Furthermore, we do not advocate that operating intangibility immediately causes less debt in capital structures, or vice versa. Nor do we take the stance that a certain level of operating intangibility should be accompanied by automatic and non-reflected organizational decisions. Instead, we are content to have introduced a descriptive account of the facts that is not compatible with the aforementioned prescriptive type of research.
This research did not receive any specific grant from funding agencies in the public, commercial, or not-for-profit sectors.
All authors declare no conflicts of interest in this paper.
[1] |
Abor J (2007) Industry classification and the capital structure of Ghanaian SMEs. Stud Econ Financ 24: 207-219. doi: 10.1108/10867370710817392
![]() |
[2] |
Acha V, Davies A, Hobday M, et al. (2004) Exploring the capital goods economy: complex product systems in the UK. Ind Corporate Change 13: 505-529. doi: 10.1093/icc/dth020
![]() |
[3] |
Al-Najjar B, Hussainev K (2011) Revisiting the capital-structure puzzle: UK evidence. J Risk Financ12: 329-338. doi: 10.1108/15265941111158505
![]() |
[4] |
Andrade G, Kaplan S (1998) How Costly Is Financial (Not Economic) Distress? Evidence from Highly Leveraged Transactions That Became Distressed. J Financ 53: 1443-1493. doi: 10.1111/0022-1082.00062
![]() |
[5] |
Arce DG, Cook DO, Kieschnick RL (2015) On the evolution of corporate capital structures. J Evol Econ 25: 561-583. doi: 10.1007/s00191-015-0394-8
![]() |
[6] |
Baker M, Wurgler J (2002) Market Timing and Capital Structure. J Financ 57: 1-32. doi: 10.1111/1540-6261.00414
![]() |
[7] |
Baldwin J, Sabourin D (2002) Advanced technology use and firm performance in Canadian manufacturing in the 1990s. Ind Corporate Change 11: 761-789. doi: 10.1093/icc/11.4.761
![]() |
[8] | Balestra P, Krishnakumar J (2008) Fixed Effects Models and Fixed Coefficients Models, In: Matyas, L. and P. Sevestre, Editors, The Econometrics of Panel Data, 3rd Edition, Springer. |
[9] | Baltagi BH (2008) Econometric Analysis of Panel Data (fourth ed) John Wiley & Sons, Ltd., Chichester, West Sussex, UK. |
[10] |
Banerjee S, Dasgupta S, Kim Y (2008) Buyer-Supplier Relationships and the Stakeholder Theory of Capital Structure. J Financ 63: 2507-2551. doi: 10.1111/j.1540-6261.2008.01403.x
![]() |
[11] |
Bascle G (2008) Controlling for endogeneity with instrumental variables in strategic management research. Strat Organ 6: 285-327. doi: 10.1177/1476127008094339
![]() |
[12] | Baxter N (1967) Leverage, risk of ruin and the cost of capital. J Financ 22: 395-403. |
[13] |
Becker G (1962) Investment in human capital: a theoretical analysis. J Polit Econ 70: 9-49. doi: 10.1086/258724
![]() |
[14] |
Bellone F, Musso P, Nesta L, et al. (2008) Market selection along the firm life cycle. Ind Corporate Change 17: 753-777. doi: 10.1093/icc/dtn025
![]() |
[15] |
Beattie V, Goodacre A, Thomson S (2006) Corporate Financing Decisions: UK Survey Evidence. J Bus Financ Account 33: 1402-1434. doi: 10.1111/j.1468-5957.2006.00640.x
![]() |
[16] |
Bhabra HS, Liu T, Tirtiroglu D (2008) Capital structure choice in a nascent market: evidence from listed firms in China. Financ Manage 37: 341-364. doi: 10.1111/j.1755-053X.2008.00015.x
![]() |
[17] |
Bloom M (2009) Accounting for goodwill. Abacus 45: 379-389. doi: 10.1111/j.1467-6281.2009.00295.x
![]() |
[18] | Byard D, Darrough M, Suh J, et al. (2017) Finding diamonds in the rough: Analysts' selective following of loss-reporting firms. J Bus Fin Acc 00: 1-26. |
[19] |
Cambini C, Rondi L (2012) Capital structure and investment in regulated network utilities: evidence from EU telecoms. Ind Corporate Change 21: 31-71. doi: 10.1093/icc/dtr035
![]() |
[20] | Cardao-Pito T (2010) The Level of Operating Intangibility and the Economic Characteristics of Firms. paper presented to the American Accounting Association Annual Conference, San Francisco, July 31-August 4, mimeo. |
[21] | Cardao-Pito T (2012a) Intangible flow theory, operating intangibility, and other economic characteristics of firms. Ph.d thesis, University of Strathclyde, Glasgow, Scotland. Available online at British Library: Ethos electronic theses online services. |
[22] |
Cardao-Pito T (2012b) Intangible Flow Theory. Am J Econ Sociol 71: 328-353. doi: 10.1111/j.1536-7150.2012.00833.x
![]() |
[23] |
Cardao-Pito T (2016) A law for the social sciences regarding us human beings. J Interdiscip Econ 28: 202-229. doi: 10.1177/0260107916643471
![]() |
[24] | Cardao-Pito T (2017) Organizations as producers of operating product flows to members of society. SAGE Open 7. |
[25] |
Chan LKC, Lakonishok J, Swaminathan B (2007) Industry classifications and return comovement. Financ Anal J 63: 56-70. doi: 10.2469/faj.v63.n6.4927
![]() |
[26] |
Chava S, Jarrow RA (2004) Bankruptcy prediction with industry effects. Rev Financ 8: 537-567. doi: 10.1093/rof/8.4.537
![]() |
[27] | Cohen G, Yagil J (2007) A multinational survey of corporate financial policies. J Appl Financ 17: 57-69. |
[28] |
Consoli D, Elche D (2013) The evolving knowledge base of professional service sectors. J Evol Econ 23: 477-501. doi: 10.1007/s00191-012-0277-1
![]() |
[29] |
Coombs R, Harvey M, Tether BS (2003) Analysing distributed processes of provision and innovation. Ind Corporate Change 12: 1125-1155. doi: 10.1093/icc/12.6.1125
![]() |
[30] |
Correira C, Cramer P (2008) An analysis of cost of capital, capital structure and capital budgeting practices: a survey of South African listed companies. Meditari Accountancy Res 16: 31-52. doi: 10.1108/10222529200800011
![]() |
[31] | Dedman E, Mouselli S, Shen Y, et al. (2009) Accounting, intangible assets, stock market activity, and measurement and disclosure policy—views from the U.K. Abacus 45: 312-341. |
[32] | Dempsey M (2013) The capital asset pricing model (CAPM): The history of a failed revolutionary idea in finance? Abacus 49: 7-23. |
[33] | Dempsey M (2014) The Modigliani and Miller propositions: The history of a failed foundation for corporate finance? Abacus 50: 279-295. |
[34] |
Dobrev S (1999) The dynamics of the Bulgarian newspaper industry in a period of transition: organizational adaptation, structural inertia and political change. Ind Corporate Change 8: 573-605. doi: 10.1093/icc/8.3.573
![]() |
[35] |
Draper PR (1975) Industry Influences on Share Price Variability. J Bus Financ Account 2: 169-185. doi: 10.1111/j.1468-5957.1975.tb00931.x
![]() |
[36] |
Dyckman T (2016) Significance Testing: We Can Do Better. Abacus 52: 319-342. doi: 10.1111/abac.12078
![]() |
[37] |
Evangelista R, Lucchese M, Meliciani V (2015) Business services and the export performances of manufacturing industries. J Evol Econ 25: 959-981. doi: 10.1007/s00191-015-0400-1
![]() |
[38] |
Fama E, MacBeth J (1973) Risk, Return, and Equilibrium: Empirical Tests. J Polit Econ 81: 607-636. doi: 10.1086/260061
![]() |
[39] |
Fama E, French K (2002) Testing Trade-Off and Pecking Order Predictions about Dividends and Debt. Rev Financ Stud 15: 1-33. doi: 10.1093/rfs/15.1.1
![]() |
[40] |
Fan JPH, Titman S, Twite G (2012) An international comparison of capital structure and debt maturity choices. J Financ Quant Anal 47: 23-56. doi: 10.1017/S0022109011000597
![]() |
[41] |
Findlay MC, Williams EE (1987) Toward a positive theory of corporate financial policy. Abacus 23: 107-121. doi: 10.1111/j.1467-6281.1987.tb00144.x
![]() |
[42] | Frank MZ, Goyal VK (2009) Capital structure decisions: which factors are reliably important? Financ Manage 38: 1-37. |
[43] |
Glosser S, Golden L (2005) Is labour becoming more or less flexible? Changing dynamic behaviour and asymmetries of labour input in US manufacturing. Cambridge J Econ 29: 535-557. doi: 10.1093/cje/bei006
![]() |
[44] |
Graham J, Harvey C (2001) The theory and practice of corporate finance: evidence from the field. J Financ Econ 61: 187-243. doi: 10.1016/S0304-405X(01)00044-7
![]() |
[45] |
Hamilton BH, Nickerson J (2003) Correcting for Endogeneity in Strategic Management Research. Strat Organ 1: 51-78. doi: 10.1177/1476127003001001218
![]() |
[46] |
Hunter L, Webster E, Wyatt A (2012) Accounting for expenditure on intangibles. Abacus 48: 104-145. doi: 10.1111/j.1467-6281.2012.00359.x
![]() |
[47] | Jacob O, Allen M, Feinstein SP (2011) Distortion in corporate valuation: implications of capital structure changes. Managerial Financ 38: 681-696. |
[48] |
Jimenez G, Salas V, Saurina J (2006) Determinants of Collateral. J Financ Econ 81: 255-281. doi: 10.1016/j.jfineco.2005.06.003
![]() |
[49] |
Kale J, Shahrur H (2007) Corporate Capital Structure and the Characteristics of Suppliers and Customers. J Financ Econ 83: 321-365. doi: 10.1016/j.jfineco.2005.12.007
![]() |
[50] |
Kaplinsky R, Santos-Paulino A (2006) A disaggregated analysis of EU imports: the implications for the study of patterns of trade and technology. Cambridge J Econ 30: 587-611. doi: 10.1093/cje/bei098
![]() |
[51] | Khalid S (2011) Financial reforms and dynamics of capital structure choice: a case of publically listed firms of Pakistan. J Manage Res 3: 1-16. |
[52] |
Korteweg A (2010) The net benefits to leverage. J Financ 65: 2137-2170. doi: 10.1111/j.1540-6261.2010.01612.x
![]() |
[53] | Lazonik W, Mazzucato M (2013) The risk-reward nexus in the innovation-inequality relationship: who takes the risks? Who gets the rewards? Ind Corporate Change 22: 1093-1128. |
[54] |
Lev B, Radhakrishnan S, Zhang W (2009) Organization capital. Abacus 45: 275-298. doi: 10.1111/j.1467-6281.2009.00289.x
![]() |
[55] |
Levinthal D (2006) The Neo-Schumpeterian theory of the firm and the strategy field. Ind Corporate Change 15: 391-394. doi: 10.1093/icc/dtl004
![]() |
[56] |
Lovelock C, Gummesson E (2004) Whither Services Marketing? In Search of a New Paradigm and Fresh Perspectives. J Serv Res 7: 20-41. doi: 10.1177/1094670504266131
![]() |
[57] | Lovelock C, Wirtz J (2011) Services Marketing: People, Technology and Strategy, Seventh edition, NY. Pearson. |
[58] | MacKay P, Phillips GM (2005) How does industry affect firm financial structure? Rev Financ Stud 18: 1433-1466. |
[59] |
Marrocu E, Paci R, Pontis M (2012) Intangible capital and firms' productivity. Ind Corporate Change 21: 377-402. doi: 10.1093/icc/dtr042
![]() |
[60] | Mateev M, Ivanov K (2011) How SME uniqueness affects capital structure: evidence from central and Eastern Europe panel data. Q J Financ Account 50: 115-143. |
[61] | Matyas L, Sevestre P (2008) The Econometrics of Panel Data, 3rd Edition, Springer. |
[62] |
Mathews JA (2003) Competitive dynamics and economic learning: An extended resource-based view. Ind Corporate Change 12: 115-145. doi: 10.1093/icc/12.1.115
![]() |
[63] |
Mazzoleni R, Nelson R (2013) An interpretive history of challenges to neoclassical microeconomics and how they have fared. Ind Corporate Change 22: 1409-1451. doi: 10.1093/icc/dtt031
![]() |
[64] |
McNally GM, Eng LH (1980) Management accounting practices and company characteristics. Abacus 16: 142-150. doi: 10.1111/j.1467-6281.1980.tb00094.x
![]() |
[65] | Miles ID, Tether B (2001) Surveying Innovation in Service: Measurement and Policy Interpretation Issues, Luxembourg: European Commission Publication. |
[66] | Miller MH (1977) Debt and taxes. J Financ 32: 261-275. |
[67] | Modigliani F, Miller M (1958) The Cost of Capital, Corporation Finance and the Theory of Investment. Am Econ Rev 68: 261-297. |
[68] | Modigliani F, Miller MH (1963) Corporate income taxes and the cost of capital: a correction. Am Econ Rev: 433-443. |
[69] | Nelson R, Winter S (1982) An Evolutionary Theory of Economic Change, Cambridge: Harvard University Press. |
[70] |
Newey WK, West KD (1987) Hypothesis testing with efficient method of moments estimation. Int Econ Rev 28: 777-787. doi: 10.2307/2526578
![]() |
[71] |
Orhangazi O (2008) Financialisation and capital accumulation in the non-financial corporate sector. Cambridge J Econ 32: 863-886. doi: 10.1093/cje/ben009
![]() |
[72] |
Parasuraman A, Zeithaml VA, Berry LL (1985) A Conceptual Model of Service Quality and Its Implications for Future Research. J Mark 49: 41-50. doi: 10.1177/002224298504900403
![]() |
[73] |
Peneder M (2002) Intangible investment and human resources. J Evol Econ 12: 107-134. doi: 10.1007/s00191-002-0103-2
![]() |
[74] |
Penman SH (2009) Accounting for intangible assets: There is also an income statement. Abacus 45: 358-371. doi: 10.1111/j.1467-6281.2009.00293.x
![]() |
[75] |
Petersen MA (2009) Estimating standard errors in finance panel data sets: Comparing approaches. Rev Financ Stud 22: 435-480. doi: 10.1093/rfs/hhn053
![]() |
[76] |
Rajan R, Zingales L (1995) What Do We Know about Capital Structure? Some Evidence from International Data. J Financ 50: 1421-1460. doi: 10.1111/j.1540-6261.1995.tb05184.x
![]() |
[77] |
Rathmell JM (1966) What is meant by services. J Mark 30: 32-36. doi: 10.1177/002224296603000407
![]() |
[78] |
Rauh JD, Sufi A (2012) Explaining corporate capital structure: product markets, leases, and asset similarity. Rev Financ 16: 115-155. doi: 10.1093/rof/rfr023
![]() |
[79] |
Santamaría L, Nieto MJ, Miles I (2012) Service innovation in manufacturing firms: Evidence from Spain. Technovation 32: 144-155. doi: 10.1016/j.technovation.2011.08.006
![]() |
[80] |
Scellato G (2007) Patents, firm size and financial constraints: an empirical analysis for a panel of Italian manufacturing firms. Cambridge J Econ 31: 55-76. doi: 10.1093/cje/bel006
![]() |
[81] | Seelantha SL (2010) Determinants of capital structure: further evidence from China. Manage Financ Mark 5: 106-126. |
[82] | Shaver MJ (1998) Accounting for Endogeneity When Assessing Strategy Performance: Does Entry Mode Choice Affect FDI Survival? Manage Sci 44: 571-586. |
[83] | Shi G, Sun J, Zhang L (2017) Product market competition and earnings management: A firm-level analysis. J Bus Fin Acc. |
[84] |
Shleifer A, Vishny RW (1997) A survey of corporate governance. J Financ 52: 737-783. doi: 10.1111/j.1540-6261.1997.tb04820.x
![]() |
[85] |
Shostack G (1977) Breaking Free From Product Marketing. J Mark 41: 73-80. doi: 10.1177/002224297704100219
![]() |
[86] |
Stadler C, Nobes CW (2014) The influence of country, industry, and topic factors on IFRS policy choice. Abacus 50: 386-421. doi: 10.1111/abac.12035
![]() |
[87] |
Tether BS (2003) The sources and aims of innovation in services: variety between and within sectors. Econ Innovation New Technol 12: 481-505. doi: 10.1080/1043859022000029221
![]() |
[88] |
Tether B, Hipp C, Miles I (2001) Standardisation and Particularisation in Services: evidence from Germany. Res Policy 30: 1115-1138. doi: 10.1016/S0048-7333(00)00133-5
![]() |
[89] |
Titman S (1984) The Determinants of Capital Structure on a Firm's Liquidation Decision. J Financ Econ 13: 137-151. doi: 10.1016/0304-405X(84)90035-7
![]() |
[90] |
Titman S (2002) The Modigliani and Miller Theorem and the Integration of Financial Markets. Financ Manage 31: 101-115. doi: 10.2307/3666323
![]() |
[91] |
Titman S, Tsyplakov S (2007) A dynamic model of optimal capital structure. Rev Financ 11: 401-451. doi: 10.1093/rof/rfm017
![]() |
[92] |
Titman S, Wessels R (1988) The Determinants of Capital Structure Choice. J Financ 43: 1-19. doi: 10.1111/j.1540-6261.1988.tb02585.x
![]() |
[93] |
Tollington T, Spinelli G (2012) Applying Wand and Weber's surface and deep structure approaches to financial reporting systems. Abacus 48: 502-517. doi: 10.1111/j.1467-6281.2012.00376.x
![]() |
[94] | Ughetto E (2008) Does internal finance matter for R & D? New evidence from a panel of Italian firms. Cambridge J Econ 32: 907-925. |
[95] | Volpe R, Woodlock P (2008) A survey of board financial literacy. Corporate Financ Rev 12: 16-21. |
[96] |
Wang L (2009) Ownership, size, and the formal structure of organizations: evidence from US public and private firms, 1992-2002. Ind Corporate Change 18: 595-636. doi: 10.1093/icc/dtp018
![]() |
[97] |
Wines G, Ferguson C (1993) An empirical investigation of accounting methods for goodwill and identifiable intangible assets: 1985 to 1989. Abacus 29: 90-105. doi: 10.1111/j.1467-6281.1993.tb00423.x
![]() |
[98] |
Winter SG (2006) Toward a Neo-Schumpeterian theory of the firm. Ind Corporate Change 15: 125-141. doi: 10.1093/icc/dtj006
![]() |
[99] | Wooldridge J (2002) Econometric Analysis of Cross Section and Panel Data, Boston, MIT Press. |
[100] | Wooldridge J (2006) Introductory Econometrics: A Modern Approach, 3rd edition. Mason, OH: Thomson-South Western. |
[101] |
Zeithaml VA, Parasuraman A, Berry LL (1985) Problems and Strategies in Services Marketing. J Mark 49: 33-46. doi: 10.1177/002224298504900203
![]() |
[102] | Zhang Y (2010) The product category effects on capital structure: evidence from the SMEs of British manufacturing industry. Int J Bus Manage 5: 86-112. |
![]() |
![]() |
1. | Tiago Cardao-Pito, Hypothesis that Tobin’s q captures organizations’ debt levels instead of their growth opportunities and intangible assets, 2022, 10, 2332-2039, 10.1080/23322039.2022.2132636 | |
2. | Chikazhe Lovemore, Desderio Chavunduka, Shakemore Chinofunga, Rumbidzai Patience Marere, Oniwel Chifamba, Martha Kaviya, Promoting perceived service quality and organisational performance through customer retention strategies: the moderating role of ICT, 2023, 28, 2183-4172, 193, 10.1108/EJMS-01-2023-0003 | |
3. | Shan Huang, Khor Teik Huat, Yue Liu, Study on the influence of Chinese traditional culture on corporate environmental responsibility, 2023, 20, 1551-0018, 14281, 10.3934/mbe.2023639 | |
4. | Tiago Cardao-Pito, Jyldyz Abdyrakhmanova, Metaphysical Status of Money and Sustainable Organizations and Ecosystems, 2024, 23, 1740-3812, 257, 10.1007/s40926-024-00309-z |
Variable | Mean | Std. Dev. | 25 th Pctl. | Median | 75th Pctl. |
LOI | 0.263 | 0.166 | 0.140 | 0.230 | 0.350 |
DEBTLEVERAGE | 0.320 | 0.236 | 0.116 | 0.308 | 0.483 |
INTANGIBLE_ASSETS | 0.064 | 0.124 | 0.000 | 0.006 | 0.067 |
SIZE | 5.713 | 1.867 | 4.361 | 5.564 | 6.909 |
CAPEX_PPE | 0.070 | 0.076 | 0.023 | 0.050 | 0.092 |
PROFITABILITY | 0.064 | 0.190 | 0.020 | 0.054 | 0.090 |
MARKET_TO_BOOK | 3.528 | 73.553 | 1.055 | 1.693 | 2.792 |
Quintile | Firms | Observations | Mean | Std. Dev. | 25th Pctl. | Median | 75th Pctl. |
1 | 1,921 | 21,629 | 0.090 | 0.042 | 0.061 | 0.089 | 0.116 |
2 | 1,921 | 23,414 | 0.178 | 0.049 | 0.149 | 0.176 | 0.204 |
3 | 1,922 | 21,185 | 0.261 | 0.058 | 0.227 | 0.257 | 0.291 |
4 | 1,921 | 17,779 | 0.363 | 0.073 | 0.319 | 0.359 | 0.403 |
5 | 1,922 | 13,648 | 0.555 | 0.141 | 0.461 | 0.527 | 0.623 |
Total | 9,607 | 97,655 |
LOI | DEBTLEVERAGE | INTANGIBLE_ASSETS | SIZE | CAPEX_PPE | PROFITABILITY | MARKET_TO_BOOK | |
LOI | 1.00 | ||||||
DEBTLEVERAGE | −0.197 | 1.00 | |||||
INTANGIBLE_ASSETS | 0.058 | 0.106 | 1.00 | ||||
SIZE | −0.267 | 0.178 | 0.212 | 1.00 | |||
CAPEX_PPE | −0.247 | −0.0611 | −0.112 | 0.170 | 1.00 | ||
PROFITABILITY | 0.040 | −0.501 | −0.071 | 0.031 | 0.276 | 1.00 | |
MARKET_TO_BOOK | 0.252 | −0.150 | 0.097 | 0.061 | 0.090 | 0.377 | 1.00 |
Full Sample | Sub sample of firms with Industry Code (SIC) between 3,400 and 4,000 | ||||
Computation A: All observations | Computation B: Removing outliers | Computation C: All observations | Computation D: Removing outliers | ||
Intercept | 0.254*** | 0.239*** | 0.245*** | 0.259*** | |
(4.18) | (4.40) | (3.53) | (4.19) | ||
LOI | −0.065*** | −0.077*** | −0.196*** | −0.226*** | |
(−7.36) | (−8.87) | (−9.92) | (−12.23) | ||
INTANGIBLE_ASSETS | 0.285*** | 0.253*** | 0.355*** | 0.329*** | |
(41.25) | (35.92) | (26.80) | (26.04) | ||
SIZE | 0.013*** | 0.020*** | 0.006*** | 0.012*** | |
(13.45) | (22.00) | (3.46) | (7.46) | ||
CAPEX_PPE | 0.080*** | 0.099*** | 0.046** | 0.082*** | |
(8.76) | (9.53) | (2.16) | (3.91) | ||
PROFITABILITY | −0.119*** | −1.324*** | −0.136*** | −1.268*** | |
(−36.84) | (−117.19) | (−23.68) | (−68.00) | ||
MARKET_TO_BOOK | 0.000*** | 0.021*** | 0.000*** | 0.020*** | |
(7.10) | (57.59) | (7.14) | (30.39) | ||
R-Square | 0.694 | 0.7481 | 0.6071 | 0.6744 | |
F-Value | 23.92 | 21.19 | 15.62 | 15.49 | |
Observations | 92,477 | 84,960 | 26,444 | 24944 | |
Firms | 7,070 | 6,686 | 1,812 | 1,759 | |
Average number of observations by firm | 13.1 | 12.7 | 14.6 | 14.1 | |
St. deviat. of observations by firm | 9.0 | 8.7 | 9.5 | 9.3 | |
Fixed effects for firms | yes | yes | yes | yes | |
Fixed effects for years | yes | yes | yes | yes | |
Beginning Year | 1966 | 1966 | 1966 | 1966 | |
End Year | 2006 | 2006 | 2006 | 2006 | |
Notes:
1. The models were computed using a fixed effects/panel data specification controlling the fixed effects of firms and years. 2. T-test results are given in brackets: *Significant at 10% level; **Significant at 5% level; *** Significant at 1% level. |
Variable | Mean | Std. Dev. | 25 th Pctl. | Median | 75th Pctl. |
LOI | 0.263 | 0.166 | 0.140 | 0.230 | 0.350 |
DEBTLEVERAGE | 0.320 | 0.236 | 0.116 | 0.308 | 0.483 |
INTANGIBLE_ASSETS | 0.064 | 0.124 | 0.000 | 0.006 | 0.067 |
SIZE | 5.713 | 1.867 | 4.361 | 5.564 | 6.909 |
CAPEX_PPE | 0.070 | 0.076 | 0.023 | 0.050 | 0.092 |
PROFITABILITY | 0.064 | 0.190 | 0.020 | 0.054 | 0.090 |
MARKET_TO_BOOK | 3.528 | 73.553 | 1.055 | 1.693 | 2.792 |
Quintile | Firms | Observations | Mean | Std. Dev. | 25th Pctl. | Median | 75th Pctl. |
1 | 1,921 | 21,629 | 0.090 | 0.042 | 0.061 | 0.089 | 0.116 |
2 | 1,921 | 23,414 | 0.178 | 0.049 | 0.149 | 0.176 | 0.204 |
3 | 1,922 | 21,185 | 0.261 | 0.058 | 0.227 | 0.257 | 0.291 |
4 | 1,921 | 17,779 | 0.363 | 0.073 | 0.319 | 0.359 | 0.403 |
5 | 1,922 | 13,648 | 0.555 | 0.141 | 0.461 | 0.527 | 0.623 |
Total | 9,607 | 97,655 |
LOI | DEBTLEVERAGE | INTANGIBLE_ASSETS | SIZE | CAPEX_PPE | PROFITABILITY | MARKET_TO_BOOK | |
LOI | 1.00 | ||||||
DEBTLEVERAGE | −0.197 | 1.00 | |||||
INTANGIBLE_ASSETS | 0.058 | 0.106 | 1.00 | ||||
SIZE | −0.267 | 0.178 | 0.212 | 1.00 | |||
CAPEX_PPE | −0.247 | −0.0611 | −0.112 | 0.170 | 1.00 | ||
PROFITABILITY | 0.040 | −0.501 | −0.071 | 0.031 | 0.276 | 1.00 | |
MARKET_TO_BOOK | 0.252 | −0.150 | 0.097 | 0.061 | 0.090 | 0.377 | 1.00 |
Full Sample | Sub sample of firms with Industry Code (SIC) between 3,400 and 4,000 | ||||
Computation A: All observations | Computation B: Removing outliers | Computation C: All observations | Computation D: Removing outliers | ||
Intercept | 0.254*** | 0.239*** | 0.245*** | 0.259*** | |
(4.18) | (4.40) | (3.53) | (4.19) | ||
LOI | −0.065*** | −0.077*** | −0.196*** | −0.226*** | |
(−7.36) | (−8.87) | (−9.92) | (−12.23) | ||
INTANGIBLE_ASSETS | 0.285*** | 0.253*** | 0.355*** | 0.329*** | |
(41.25) | (35.92) | (26.80) | (26.04) | ||
SIZE | 0.013*** | 0.020*** | 0.006*** | 0.012*** | |
(13.45) | (22.00) | (3.46) | (7.46) | ||
CAPEX_PPE | 0.080*** | 0.099*** | 0.046** | 0.082*** | |
(8.76) | (9.53) | (2.16) | (3.91) | ||
PROFITABILITY | −0.119*** | −1.324*** | −0.136*** | −1.268*** | |
(−36.84) | (−117.19) | (−23.68) | (−68.00) | ||
MARKET_TO_BOOK | 0.000*** | 0.021*** | 0.000*** | 0.020*** | |
(7.10) | (57.59) | (7.14) | (30.39) | ||
R-Square | 0.694 | 0.7481 | 0.6071 | 0.6744 | |
F-Value | 23.92 | 21.19 | 15.62 | 15.49 | |
Observations | 92,477 | 84,960 | 26,444 | 24944 | |
Firms | 7,070 | 6,686 | 1,812 | 1,759 | |
Average number of observations by firm | 13.1 | 12.7 | 14.6 | 14.1 | |
St. deviat. of observations by firm | 9.0 | 8.7 | 9.5 | 9.3 | |
Fixed effects for firms | yes | yes | yes | yes | |
Fixed effects for years | yes | yes | yes | yes | |
Beginning Year | 1966 | 1966 | 1966 | 1966 | |
End Year | 2006 | 2006 | 2006 | 2006 | |
Notes:
1. The models were computed using a fixed effects/panel data specification controlling the fixed effects of firms and years. 2. T-test results are given in brackets: *Significant at 10% level; **Significant at 5% level; *** Significant at 1% level. |