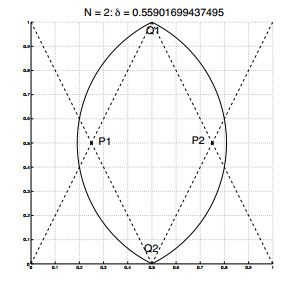
Neurosurgeons receive extensive and lengthy training to equip themselves with various technical skills, and neurosurgery require a great deal of pre-, intra- and postoperative clinical data collection, decision making, care and recovery. The last decade has seen a significant increase in the importance of artificial intelligence (AI) in neurosurgery. AI can provide a great promise in neurosurgery by complementing neurosurgeons' skills to provide the best possible interventional and noninterventional care for patients by enhancing diagnostic and prognostic outcomes in clinical treatment and help neurosurgeons with decision making during surgical interventions to improve patient outcomes. Furthermore, AI is playing a pivotal role in the production, processing and storage of clinical and experimental data. AI usage in neurosurgery can also reduce the costs associated with surgical care and provide high-quality healthcare to a broader population. Additionally, AI and neurosurgery can build a symbiotic relationship where AI helps to push the boundaries of neurosurgery, and neurosurgery can help AI to develop better and more robust algorithms. This review explores the role of AI in interventional and noninterventional aspects of neurosurgery during pre-, intra- and postoperative care, such as diagnosis, clinical decision making, surgical operation, prognosis, data acquisition, and research within the neurosurgical arena.
Citation: Mohammad Mofatteh. Neurosurgery and artificial intelligence[J]. AIMS Neuroscience, 2021, 8(4): 477-495. doi: 10.3934/Neuroscience.2021025
[1] | Tianji Wang, Qingdao Huang . A new Newton method for convex optimization problems with singular Hessian matrices. AIMS Mathematics, 2023, 8(9): 21161-21175. doi: 10.3934/math.20231078 |
[2] | Essam H. Houssein, Nagwan Abdel Samee, Maali Alabdulhafith, Mokhtar Said . Extraction of PEM fuel cell parameters using Walrus Optimizer. AIMS Mathematics, 2024, 9(5): 12726-12750. doi: 10.3934/math.2024622 |
[3] | Abdelwahed Motwake, Aisha Hassan Abdalla Hashim, Marwa Obayya, Majdy M. Eltahir . Enhancing land cover classification in remote sensing imagery using an optimal deep learning model. AIMS Mathematics, 2024, 9(1): 140-159. doi: 10.3934/math.2024009 |
[4] | Young Joon Ahn . G2/C1 Hermite interpolation of offset curves of parametric regular curves. AIMS Mathematics, 2023, 8(12): 31008-31021. doi: 10.3934/math.20231587 |
[5] | Yuna Zhao . Construction of blocked designs with multi block variables. AIMS Mathematics, 2021, 6(6): 6293-6308. doi: 10.3934/math.2021369 |
[6] | Yujun Zhu, Ju Ming . Lagrangian decomposition for stochastic TIMES energy system optimization model. AIMS Mathematics, 2022, 7(5): 7964-7996. doi: 10.3934/math.2022445 |
[7] | Li Dong, Jingyong Tang . New convergence analysis of a class of smoothing Newton-type methods for second-order cone complementarity problem. AIMS Mathematics, 2022, 7(9): 17612-17627. doi: 10.3934/math.2022970 |
[8] | Bothina El-Sobky, Yousria Abo-Elnaga, Gehan Ashry . A nonmonotone trust region technique with active-set and interior-point methods to solve nonlinearly constrained optimization problems. AIMS Mathematics, 2025, 10(2): 2509-2540. doi: 10.3934/math.2025117 |
[9] | Chenmin Ni, Muhammad Fadhil Marsani, Fam Pei Shan, Xiaopeng Zou . Flood prediction with optimized gated recurrent unit-temporal convolutional network and improved KDE error estimation. AIMS Mathematics, 2024, 9(6): 14681-14696. doi: 10.3934/math.2024714 |
[10] | Reem K. Alhefthi, Pakeeza Ashraf, Ayesha Abid, Shahram Rezapour, Abdul Ghaffar, Mustafa Inc . Exploring the flexibility of m-point quaternary approximating subdivision schemes with free parameter. AIMS Mathematics, 2024, 9(11): 33185-33214. doi: 10.3934/math.20241584 |
Neurosurgeons receive extensive and lengthy training to equip themselves with various technical skills, and neurosurgery require a great deal of pre-, intra- and postoperative clinical data collection, decision making, care and recovery. The last decade has seen a significant increase in the importance of artificial intelligence (AI) in neurosurgery. AI can provide a great promise in neurosurgery by complementing neurosurgeons' skills to provide the best possible interventional and noninterventional care for patients by enhancing diagnostic and prognostic outcomes in clinical treatment and help neurosurgeons with decision making during surgical interventions to improve patient outcomes. Furthermore, AI is playing a pivotal role in the production, processing and storage of clinical and experimental data. AI usage in neurosurgery can also reduce the costs associated with surgical care and provide high-quality healthcare to a broader population. Additionally, AI and neurosurgery can build a symbiotic relationship where AI helps to push the boundaries of neurosurgery, and neurosurgery can help AI to develop better and more robust algorithms. This review explores the role of AI in interventional and noninterventional aspects of neurosurgery during pre-, intra- and postoperative care, such as diagnosis, clinical decision making, surgical operation, prognosis, data acquisition, and research within the neurosurgical arena.
artificial intelligence;
brain-computer interface;
computer-assisted diagnosis;
computerised tomography;
deep learning;
machine learning;
magnetic resonance imaging;
traumatic brain injury;
temporal lobe epilepsy
We consider in this work the problem of optimally distributing N points inside a domain so that, when the Euclidean distance to the nearest design point is computed for all locations on the domain, the maximum of these distances is minimized. Equivalently, we seek to distribute the N points in such a way that equal-radius circles centred at these points required to cover the entire domain are as small as possible. In this work, we call this the optimal covering problem. We restrict our discussion to the unit square domain. We propose a numerical algorithm to identify optimal designs to high precision. The use of these optimal designs in approximations to two related problems involving the optimal placement of curves is also investigated.
The covering problem arises in statistical applications, and is discussed in [6]. Here, the idea is to identify N experiments that give the best coverage of a two-dimensional parameter range. The problem also arises in the context of operations research in the optimal placement of service centres [1,4,17] or wireless transmitters [7]. The literature on the subject is disjoint and the current authors cannot claim to have identified all relevant material. The first time this problem was considered was in [15] in which conjectured optimal coverings of the square for N from one to ten were proposed based on numerical computation. These stand as optimal coverings except for N=6. In this case, a small improvement was found [8]. Proofs for the optimality of some small N cases can be found in [5] with little subsequent theoretical progress. Candidates for optimal coverings of the square up to N=30 based on numerical computation can be found in [11] and for N=31 to 100 in [16]. In this last article, references to other covering problems (sphere surface by circles, triangles by circles, etc.) can be found.
The related problem of circle packing has received much more attention. Early proofs for optimal packing in a square with small numbers of circles can be found in [12]. Numerically computed candidates for optimal solutions can be found in [10] for up to fifty circles in a square. Candidates of optimal packings of up to several thousand circles are kept up to date on a web site [14]. It is known that for the circle packing problem, free points ("rattlers") can occur that can lie anywhere within a certain region of the domain. This happens in the square for seven circles, for example [12]. For our covering problem in the square, we have not observed an optimal design that allows any point to be moved while still preserving the optimality of the design. This has also been observed by others [8,11,15,16] and we conjecture that for the covering problem in the square, rattlers do not occur. This influenced the numerical optimization strategy used in this article and by others for this covering problem. In the current work, a final numerical step involves a structure in which there are segments of the minimal length that lock the design points into the optimal configuration. For domains other than the square, the covering problem can have rattlers (consider the N=2 covering problem in a circular domain, for example). The methods in the literature and our own method can be extended to other domain shapes, but would have difficulty with problems with rattlers.
In another related problem, the optimal covering does not minimize the maximum distance of any location in the domain to the N points but rather the average distance over all locations. This problem can be considered easier than our covering problem since there is more regularity. It is known that as N→∞ optimal solutions lie on a hexagonal lattice [9] in the domain interior. This result relies on the fact that edge effects become negligible for large N when the average is taken.
In the current work, we present an algorithm for computing candidate optimal covering designs in the more difficult case where the maximum distance is minimized. The algorithm has two stages. In the first stage, the N points are placed randomly and moved heuristically towards an optimal candidate. Then, a Newton iteration is used to compute optimal locations to near machine precision. Our algorithm is described in more detail below. This iterative refinement idea is different from other approaches in the literature. In [15] the equivalent to our second stage of the algorithm used a mechanical analogy to shrinking, tensioned bars with frictionless pin joints to obtain high accuracy solutions. This was also the second stage strategy used in [11]. In that work, the first stage to identify approximate candidates was not done with a heuristic search but rather an optimization procedure to move the points to reduce the area not covered by the current design. In [16] a similar optimization idea is used without a second stage. For both these competitive algorithms, the iterative second stage we propose could be used to improve final convergence to high accuracy solutions.
The motivating problem for the authors is more difficult. It is to find a simple curve C in a given simply connected and bounded domain. The curve has given length and given starting and ending points and is such that the maximum distance of the curve to any point in the domain is minimized over the set of all such curves. It is not known whether this problem has well defined minimizers and there may be a high degree of degeneracy in locally optimal curves. It arises in the hydrogen fuel cell application, in the design of reactant gas flow channels [13]. Here, the starting and ending points are the channel inlet and outlet port locations. The channel should be laid out to supply reactant gas evenly to all parts of the fuel cell area, hence the desire to minimize distance to the channel path C. In the case where the guarantee of maximum distance to C is critical but the exact value of curve length is not, sub-optimal solutions of this problem can be found by connecting optimal point coverings to start and end points optimally. The resulting problem is the travelling salesman problem, known to be NP hard but for which many heuristic solution methods are known. A related problem is that of finding a connected network of given length that optimally covers a domain. This removes the requirement of given start and end locations and allows branch points in the network. This problem has been studied in the context of optimal transportation networks [3]. Some numerical results for such optimal networks in the square can be found in [2]. Here, the related problem of optimizing average rather than maximum distance is studied. For the maximum distance case, sub-optimal solutions that can guarantee a given maximal distance can be constructed by connecting optimal N point coverings with a network of shortest length. This is the Steiner tree problem, also known to be NP-hard but with many effective heuristic algorithms.
In section 2 below, our two-stage algorithm for the optimal covering problem is described. Some results of the method are shown in section 3. The use of the optimal covering points to find approximate solutions to the optimal curve problems is discussed in section 4.
The algorithm has two stages with iterations at each stage. We call the N points Pj to be placed design points. At each iteration of the first stage, the point Q in the domain that is farthest away from its nearest design points is found. Then, design points nearest to Q are moved towards it. In the second stage, an optimization problem is solved with Newton iterations that can find design points to machine precision. As with many optimization algorithms, the algorithm may find a local minimum rather than the true optimum. It is therefore intended that a set of solutions be computed from various random starting configurations with enough trials that the optimal configuration is included within this set. The number of such trials needed generally increases with N.
We define δ as the largest of the distances computed from every point in the domain to the nearest design point(s). We also let the theoretical minimum of δ be called δ∗ so that δ≥δ∗, with equality holding only when the configuration is optimal. To compute δ for a particular arrangement of design points, we admit the following locations in the domain as candidates for being one of the points Qi: the four corners, centres of circles situated on the domain boundary passing through two design points, and centres of circles situated strictly inside the domain passing through three design points. The centres of the M circles with radii equal to the largest of all the radii are taken as points Qi,i=1,...,M. Of course, to be an actual candidate, no design points can be strictly inside the circle. It is fairly easy to see that this finite set of possible Q does produce the largest distance δ in the square to the set of design points.
At each step of the algorithm, the value of δ is calculated systematically checking the cases listed above, with the centre(s) of the maximal circles denoted by Qi.
Once the locations of Qi have been identified, the next step might be to move at least one of the nearest design points toward them by a specified step size h, thereby possibly decreasing δ. However, this method performed poorly, especially for larger N. The reason for this may be that this method is more of a local search, converging only to the "nearest minimum", a suboptimal configuration for which no design point can be moved locally without increasing δ. In general, the more design points there are, the more local minima.
To promote a wider search and avoid local minima, we instead admit for points Qi the centres of all circles that have radius within a given tolerance (proportional to the step length h) of δ. We also set the step size relatively large at the start and decrease it gradually. The result is that more design points are moved farther from their original locations. Note that this combination of characteristics does not guarantee a decrease in δ from one step to the next, and so while the algorithm favours downhill moves, uphill transitions are allowed.
The following steps summarizes the first stage (the coarse search portion) of the algorithm, starting with a relatively large initial step length h:
(1) Compute δ.
(2) Make a list of all candidates for δ that are within a predetermined tolerance of δ (the tolerance proportional to the step length).
(3) Of this set of circles, move every design point on the boundary of a circle towards the centre. The magnitude of the move is h.
● if one design point at Pj lies on the boundary of M>1 circles of candidate radii δi within tolerance centred at Qi, i=1,2,…,M, the direction in which to move point j is computed as
→vj=M∑i=1δi(Qi−Pj), |
so that directions associated with larger distances are weighted more heavily. For a step size of h, the resulting position of point j would be P′j=Pj+h→vj|→vj|.
(4) Ensure all design points are still inside the square – if not, decrease the step size by a factor of two and move all points in the same direction. Repeat this step until all points are inside the square and reset the step size to its previous value.
(5) Repeat steps 1 through 4 until the value of δ begins to oscillate about a mean value for a predetermined number of times
(6) Decrease step size by a geometric factor, and return to step 1. Once the step size has been decreased to a predetermined value, end stage 1.
The above describes an algorithm that is capable of finding the vicinity of an optimal configuration, but is incapable of locating it to high precision. We proceed on the assumption that, when the configuration is optimal (or even just locally optimal), there are enough maximal locations in the domain for which the nearest design point is the same distance δ (δ∗ if optimal) away that the design point positions are fixed. As mentioned in the introduction, this idea relies on the conjecture that no rattlers appear in the optimal covering problem for the square.
This assumption allows for a more algebraic minimization. We seek to make all the links →c from circle centres Q to any design points P on its circle's edge to be of equal length (the optimal δ∗ if the coarse algorithm result is close enough to the optimal configuration). Variables in the optimization are the coordinates of the circle centres Q, the coordinates of the design points P, and δ. Since the number of links is usually different from the number of variables, a straight solve in the manner of equal number of equations and unknowns is not possible in general. We instead minimize, over the space of all variables, the following function for the case of K links:
Ha=K∑i=1(||P−Q||2i−δ2)2+aδ2. | (2.1) |
We make two notes here about Eq. 2.1. First, the subscript i indexes the ith link, between a design point P and circle centre (maximal distance point) Q. Second, the last term aδ2 is included to ensure that we are in fact, by minimizing Ha, converging to a configuration for which δ is a minimum. It also serves to prevent a singular Hessian matrix during the Newton iteration for the minimization of Ha (solving of ∇Ha=0) when there are fewer links than unknowns. We have found that a should start out roughly on the order of the final step size employed in the coarse search algorithm, and then be decreased during the Newton iteration.
A graphical depiction of the method can be seen from the converged N=2 optimal solution shown in Figure 1. Here, there are K=8 links, shown as dashed lines. The function Ha is minimized as a→0 over 6 unknowns: four for the coordinates of the two design points P and two more for the single coordinates of the two edge maximal distance points Q. The four corners are also maximal distance points Q but are fixed, not variables. During the course of the optimization, the structure of the problem can vary (a corner Q moving along an edge for example). The algorithm tracks these changes automatically.
For this procedure to converge to the optimal solution, it is imperative that the coarse algorithm identifies the "correct links" to set to equal lengths. The lengths of all the correct links must be longer than those of the extraneous links. To achieve this, the final step size hf used in the coarse search must be small enough. Once that is arranged, the task then is to separate the correct links from the extraneous ones. This is done as follows: we sort the L links (correct plus extraneous) according to their lengths so that δi<δj for i<j and compute Ri=δmax−δihf. If the optimal configuration has K correct links, RL−K−RL−K+1 is significantly larger than all other differences between adjacent values of R. As part of the algorithm, we have a threshholding technique to identify K. As mentioned above, the two stage method must be run starting from a sample of random initial conditions to be able to find optimal solutions. Past small N, there is no proof of optimality of the results.
The iterations of this second stage can fail to converge or lead to a local but not globally optimal solution. Convergence failure is normally due to an incorrect identification of the links used in the Newton step. This identification becomes increasingly difficult as N increases and the algorithm as a whole is suitable only for moderate N. However, if the links are correctly identified, the Newton procedure is robust and finds potentially optimal solutions quickly and to high accuracy. Thus, we believe our Newton approach is the strongest contribution of this work and can be combined with other stage 1 strategies.
We present some of our results for various N in Figure 2. Circles centred around each design point have a radius δ, and the dashed lines represent the links in the stage 2 process. Our results for N = 2–5 and 7 are proven optimal [5]. The N=6 has a close nearly optimum solution that is often found by the algorithm, first reported in [15]. The optimal case was first seen in [8]. It can be seen that as N increases, the optimal configurations near the boundaries become very subtle.
We proceed to the two curve optimization problems discussed in the Introduction. Recall that the motivating problem is: given a closed and bounded domain Ω (the unit square in our computations) find a curve C of given length L in Ω such that
δ=maxx∈Ωdist(x,C) |
is minimized with one of two additional conditions:
A: C is a simple curve beginning at given point x0 (inlet) and ending at given point x1 (outlet).
B: C is connected.
One could also consider the "inverse problem" of finding a curve C with a given value of δ of smallest length. We provide sub-optimal solutions to these problems based on the N=16 covering solution shown in Figure 2. By sub-optimal, we mean curves that have a value δ below a given tolerance (from application criteria) but may not have the smallest length that can achieve that tolerance. Taking the inlet to be the lower left hand corner and the outlet to be the upper right for condition A above, we compute the travelling salesman problem to find the curve of minimum length between the points. This curve is guaranteed to be no more than a distance δ=0.169427051598 from every point in the domain by the property of the N=16 interior points. However, it is not the simple, continuous curve of the smallest length that has this property. The result is shown in Figure 3. Also shown is the heuristic solution to the connected curve (allowing branches) with this property (condition B above). This is found by applying a heuristic Steiner algorithm to the configuration for N=16 design points. This curve network is also guaranteed to be no more than a distance δ=0.169427051598 from every point in the domain. We do not claim that this is the curve with this property that has the shortest length. However, it is known that such optimal curves in the average distance problem can have branch points that must occur at Steiner angles [2,3].
We present a new algorithm for finding optimal covering points. It has an optimization second stage with a carefully constructed objective function that can find optimal designs to machine precision. Our second stage could be used in other methods that have heuristics that tend to approximate optimal configurations. The optimal designs are used to find approximate solutions to two related optimal curve problems, one of which is of interest to an aspect of hydrogen fuel cell design.
The first author would like to thank the NSERC USRA programme for the funding that allowed him to work on this problem. The second author acknowledges NSERC Canada research funding and the contributions to the literature search by another USRA student, Cheng Zhang.
The authors declare no conflict of interest.
[1] |
Wise J (2020) Life as a neurosurgeon. BMJ 368: m395. doi: 10.1136/bmj.m395
![]() |
[2] |
Kaptigau WM, Rosenfeld JV, Kevau I, et al. (2016) The establishment and development of neurosurgery services in Papua New Guinea. World J Surg 40: 251-257. doi: 10.1007/s00268-015-3268-1
![]() |
[3] |
Rolston JD, Zygourakis CC, Han SJ, et al. (2014) Medical errors in neurosurgery. Surg Neurol Int 5: S435-S440. doi: 10.4103/2152-7806.142777
![]() |
[4] |
Kwoh YS, Hou J, Jonckheere EA, et al. (1988) A robot with improved absolute positioning accuracy for CT guided stereotactic brain surgery. IEEE Trans Biomed Eng 35: 153-160. doi: 10.1109/10.1354
![]() |
[5] | Aziz T, Roy H (2021) Deep Brain Stimulation. Oxford Research Encyclopedia of Psychology Oxford University Press. |
[6] |
Panesar SS, Kliot M, Parrish R, et al. (2020) Promises and perils of artificial intelligence in neurosurgery. Neurosurgery 87: 33-44. doi: 10.1093/neuros/nyz471
![]() |
[7] | Bohl MA, Oppenlander ME, Spetzler R (2016) A prospective cohort evaluation of a robotic, auto-navigating operating microscope. Cureus 8: e662-e662. |
[8] |
Lanfranco AR, Castellanos AE, Desai JP, et al. (2004) Robotic surgery: a current perspective. Ann Surg 239: 14-21. doi: 10.1097/01.sla.0000103020.19595.7d
![]() |
[9] |
Shimizu S, Kuroda H, Mochizuki T, et al. (2020) Ergonomics-based positioning of the operating handle of surgical microscopes. Neurol Med-Chir 60: 313-316. doi: 10.2176/nmc.rc.2020-0018
![]() |
[10] | Van Bavel J (2013) The world population explosion: causes, backgrounds and pro-jections for the future. Facts Views Vision Obgyn 5: 281-291. |
[11] |
Vaupel JW (2010) Biodemography of human ageing. Nature 464: 536-542. doi: 10.1038/nature08984
![]() |
[12] |
You D, Hug L, Ejdemyr S, et al. (2015) Global, regional, and national levels and trends in under-5 mortality between 1990 and 2015, with scenario-based projections to 2030: a systematic analysis by the UN Inter-agency Group for Child Mortality Estimation. Lancet 386: 2275-2286. doi: 10.1016/S0140-6736(15)00120-8
![]() |
[13] |
Aluttis C, Bishaw T, Frank MW (2014) The workforce for health in a globalized context-global shortages and international migration. Global Health Action 7: 23611-23611. doi: 10.3402/gha.v7.23611
![]() |
[14] |
Senders JT, Arnaout O, Karhade AV, et al. (2018) Natural and artificial intelligence in neurosurgery: a systematic review. Neurosurgery 83: 181-192. doi: 10.1093/neuros/nyx384
![]() |
[15] |
Sullivan R, Alatise OI, Anderson BO, et al. (2015) Global cancer surgery: delivering safe, affordable, and timely cancer surgery. Lancet Oncol 16: 1193-1224. doi: 10.1016/S1470-2045(15)00223-5
![]() |
[16] |
Michael CD, Abbas R, Graham F, et al. (2019) Global neurosurgery: the current capacity and deficit in the provision of essential neurosurgical care. Executive summary of the global neurosurgery initiative at the program in global surgery and social change. J Neurosurg 130: 1055-1064. doi: 10.3171/2017.11.JNS171500
![]() |
[17] |
Swagoto M, Maria P, Abbas R, et al. (2019) The global neurosurgical workforce: a mixed-methods assessment of density and growth. J Neurosurg 130: 1142-1148. doi: 10.3171/2018.10.JNS171723
![]() |
[18] |
Kato Y, Liew BS, Sufianov AA, et al. (2020) Review of global neurosurgery education: horizon of neurosurgery in the developing countries. Chin Neurosurg J 6: 19. doi: 10.1186/s41016-020-00194-1
![]() |
[19] |
Solomou G, Murphy S, Bandyopadhyay S, et al. (2020) Neurosurgery specialty training in the UK: What you need to know to be shortlisted for an interview. Ann Med Surg 57: 287-290. doi: 10.1016/j.amsu.2020.07.047
![]() |
[20] |
Mooney MA, Yoon S, Cole T, et al. (2019) Cost transparency in neurosurgery: a single-institution analysis of patient out-of-pocket spending in 13673 consecutive neurosurgery cases. Neurosurgery 84: 1280-1289. doi: 10.1093/neuros/nyy185
![]() |
[21] |
Yoon JS, Tang OY, Lawton MT (2019) Volume–cost relationship in neurosurgery: analysis of 12,129,029 admissions from the national inpatient sample. World Neurosurg 129: e791-e802. doi: 10.1016/j.wneu.2019.06.034
![]() |
[22] |
Obermeyer Z, Emanuel EJ (2016) Predicting the future–Big data, Machine learning, and Clinical medicine. N Engl J Med 375: 1216-1219. doi: 10.1056/NEJMp1606181
![]() |
[23] | Cruz JA, Wishart DS (2007) Applications of machine learning in cancer prediction and prognosis. Cancer Inform 2: 59-77. |
[24] |
Marcus HJ, Williams S, Hughes-Hallett A, et al. (2017) Predicting surgical outcome in patients with glioblastoma multiforme using pre-operative magnetic resonance imaging: development and preliminary validation of a grading system. Neurosurg Rev 40: 621-631. doi: 10.1007/s10143-017-0817-0
![]() |
[25] |
Rudie JD, Rauschecker AM, Bryan RN, et al. (2019) Emerging applications of artificial intelligence in neuro-oncology. Radiology 290: 607-618. doi: 10.1148/radiol.2018181928
![]() |
[26] |
Deo RC (2015) Machine learning in medicine. Circulation 132: 1920-1930. doi: 10.1161/CIRCULATIONAHA.115.001593
![]() |
[27] |
Lane T (2018) A short history of robotic surgery. Ann R Coll Surge Engl 100: 5-7. doi: 10.1308/rcsann.supp1.5
![]() |
[28] |
Sheetz KH, Claflin J, Dimick JB (2020) Trends in the adoption of robotic surgery for common surgical procedures. JAMA Network Open 3: e1918911-e1918911. doi: 10.1001/jamanetworkopen.2019.18911
![]() |
[29] |
Topol EJ (2019) High-performance medicine: the convergence of human and artificial intelligence. Nat Med 25: 44-56. doi: 10.1038/s41591-018-0300-7
![]() |
[30] |
Jordan MI, Mitchell TM (2015) Machine learning: Trends, perspectives, and prospects. Science 349: 255. doi: 10.1126/science.aaa8415
![]() |
[31] |
Senders JT, Staples PC, Karhade AV, et al. (2018) Machine learning and neurosurgical outcome prediction: a systematic review. World Neurosurg 109: 476-486. doi: 10.1016/j.wneu.2017.09.149
![]() |
[32] |
LeCun Y, Bengio Y, Hinton G (2015) Deep learning. Nature 521: 436-444. doi: 10.1038/nature14539
![]() |
[33] |
Alvin MD, Lubelski D, Alam R, et al. (2018) Spine surgeon treatment variability: the impact on costs. Global Spine J 8: 498-506. doi: 10.1177/2192568217739610
![]() |
[34] |
Daniels AH, Ames CP, Smith JS, et al. (2014) Variability in spine surgery procedures performed during orthopaedic and neurological surgery residency training: an analysis of ACGME case log data. J Bone Joint Surg Am 96: e196. doi: 10.2106/JBJS.M.01562
![]() |
[35] |
Deyo RA, Mirza SK (2006) Trends and variations in the use of spine surgery. Clin Orthop Relat Res 443: 139-146. doi: 10.1097/01.blo.0000198726.62514.75
![]() |
[36] |
Mroz TE, Lubelski D, Williams SK, et al. (2014) Differences in the surgical treatment of recurrent lumbar disc herniation among spine surgeons in the United States. Spine J 14: 2334-2343. doi: 10.1016/j.spinee.2014.01.037
![]() |
[37] |
Rasouli JJ, Shao J, Neifert S, et al. (2020) Artificial intelligence and robotics in spine surgery. Global Spine J 11: 556-564. doi: 10.1177/2192568220915718
![]() |
[38] |
Arvind V, Kim JS, Oermann EK, et al. (2018) Predicting surgical complications in adult patients undergoing anterior cervical discectomy and fusion using nachine learning. Neurospine 15: 329-337. doi: 10.14245/ns.1836248.124
![]() |
[39] |
Galbusera F, Casaroli G, Bassani T (2019) Artificial intelligence and machine learning in spine research. JOR Spine 2: e1044. doi: 10.1002/jsp2.1044
![]() |
[40] |
Kim JS, Merrill RK, Arvind V, et al. (2018) Examining the ability of artificial neural networks machine learning models to accurately predict complications following posterior lumbar spine fusion. Spine 43: 853-860. doi: 10.1097/BRS.0000000000002442
![]() |
[41] |
Emblem KE, Nedregaard B, Hald JK, et al. (2009) Automatic glioma characterization from dynamic susceptibility contrast imaging: brain tumor segmentation using knowledge-based fuzzy clustering. J Magne Reson Imaging 30: 1-10. doi: 10.1002/jmri.21815
![]() |
[42] |
Emblem KE, Pinho MC, Zöllner FG, et al. (2014) A generic support vector machine model for preoperative glioma survival associations. Radiology 275: 228-234. doi: 10.1148/radiol.14140770
![]() |
[43] |
Brady AP (2017) Error and discrepancy in radiology: inevitable or avoidable? Insights Imaging 8: 171-182. doi: 10.1007/s13244-016-0534-1
![]() |
[44] |
Siarkowski M, Lin K, Li SS, et al. (2020) Meta-analysis of interventions to reduce door to needle times in acute ischaemic stroke patients. BMJ Open Qual 9: e000915. doi: 10.1136/bmjoq-2020-000915
![]() |
[45] |
Mun SK, Wong KH, Lo S-CB, et al. (2021) Artificial Intelligence for the Future Radiology Diagnostic Service. Front Mol Biosci 7: 614258. doi: 10.3389/fmolb.2020.614258
![]() |
[46] |
Furlan Anthony J (2006) Time is brain. Stroke 37: 2863-2864. doi: 10.1161/01.STR.0000251852.07152.63
![]() |
[47] |
Fonarow Gregg C, Smith Eric E, Saver Jeffrey L, et al. (2011) Improving door-to-needle times in acute ischemic stroke. Stroke 42: 2983-2989. doi: 10.1161/STROKEAHA.111.621342
![]() |
[48] |
Man S, Xian Y, Holmes DN, et al. (2020) Association between thrombolytic door-to-needle time and 1-year mortality and readmission in patients with acute ischemic stroke. JAMA 323: 2170-2184. doi: 10.1001/jama.2020.5697
![]() |
[49] |
Nagaratnam K, Harston G, Flossmann E, et al. (2020) Innovative use of artificial intelligence and digital communication in acute stroke pathway in response to COVID-19. Future Healthcare J 7: 169-173. doi: 10.7861/fhj.2020-0034
![]() |
[50] |
Yamashita K, Yoshiura T, Arimura H, et al. (2008) Performance evaluation of radiologists with artificial neural network for differential diagnosis of intra-axial cerebral tumors on MR images. Am J Neuroradiol 29: 1153-1158. doi: 10.3174/ajnr.A1037
![]() |
[51] |
Kassahun Y, Perrone R, De Momi E, et al. (2014) Automatic classification of epilepsy types using ontology-based and genetics-based machine learning. Artif Intell Med 61: 79-88. doi: 10.1016/j.artmed.2014.03.001
![]() |
[52] |
Bidiwala S, Pittman T (2004) Neural network classification of pediatric posterior fossa tumors using clinical and imaging data. Pediatr Neurosurg 40: 8-15. doi: 10.1159/000076571
![]() |
[53] |
Zhang B, Chang K, Ramkissoon S, et al. (2017) Multimodal MRI features predict isocitrate dehydrogenase genotype in high-grade gliomas. Neuro Oncol 19: 109-117. doi: 10.1093/neuonc/now121
![]() |
[54] |
Titano JJ, Badgeley M, Schefflein J, et al. (2018) Automated deep-neural-network surveillance of cranial images for acute neurologic events. Nat Med 24: 1337-1341. doi: 10.1038/s41591-018-0147-y
![]() |
[55] |
Ueda D, Yamamoto A, Nishimori M, et al. (2018) Deep Learning for MR Angiography: Automated Detection of Cerebral Aneurysms. Radiology 290: 187-194. doi: 10.1148/radiol.2018180901
![]() |
[56] |
Dolz J, Betrouni N, Quidet M, et al. (2016) Stacking denoising auto-encoders in a deep network to segment the brainstem on MRI in brain cancer patients: A clinical study. Comput Med Imag Grap 52: 8-18. doi: 10.1016/j.compmedimag.2016.03.003
![]() |
[57] |
Cohen KB, Glass B, Greiner HM, et al. (2016) Methodological issues in predicting pediatric epilepsy surgery candidates through natural language processing and machine learning. Biomed Inform Insights 8: 11-18. doi: 10.4137/BII.S38308
![]() |
[58] |
Dumont TM, Rughani AI, Tranmer BI (2011) Prediction of symptomatic cerebral vasospasm after aneurysmal subarachnoid hemorrhage with an artificial neural network: feasibility and comparison with logistic regression models. World Neurosurgery 75: 57-63. doi: 10.1016/j.wneu.2010.07.007
![]() |
[59] |
Nielsen A, Hansen Mikkel B, Tietze A, et al. (2018) Prediction of tissue outcome and assessment of treatment effect in acute ischemic stroke using deep learning. Stroke 49: 1394-1401. doi: 10.1161/STROKEAHA.117.019740
![]() |
[60] |
Lüders H, Acharya J, Baumgartner C, et al. (1998) Semiological seizure classification. Epilepsia 39: 1006-1013. doi: 10.1111/j.1528-1157.1998.tb01452.x
![]() |
[61] |
Emblem KE, Nedregaard B, Nome T, et al. (2008) Glioma grading by using histogram analysis of blood volume heterogeneity from MR-derived cerebral blood volume maps. Radiology 247: 808-817. doi: 10.1148/radiol.2473070571
![]() |
[62] | Lev MH, Ozsunar Y, Henson JW, et al. (2004) Glial tumor grading and outcome prediction using dynamic spin-echo MR susceptibility mapping compared with conventional contrast-enhanced MR: confounding effect of elevated rCBV of oligodendroglimoas. Am J Neuroradiol 25: 214-221. |
[63] |
Marcus AP, Marcus HJ, Camp SJ, et al. (2020) Improved prediction of surgical resectability in patients with glioblastoma using an artificial neural network. Sci Rep 10: 5143. doi: 10.1038/s41598-020-62160-2
![]() |
[64] |
Young R, Babb J, Law M, et al. (2007) Comparison of region-of-interest analysis with three different histogram analysis methods in the determination of perfusion metrics in patients with brain gliomas. J Magn Reson Imaging 26: 1053-1063. doi: 10.1002/jmri.21064
![]() |
[65] |
Duffau H, Capelle L (2004) Preferential brain locations of low-grade gliomas. Cancer 100: 2622-2626. doi: 10.1002/cncr.20297
![]() |
[66] |
Clarke LP, Velthuizen RP, Clark M, et al. (1998) MRI measurement of brain tumor response: comparison of visual metric and automatic segmentation. Magn Reson Imaging 16: 271-279. doi: 10.1016/S0730-725X(97)00302-0
![]() |
[67] |
Pfirrmann CWA, Metzdorf A, Zanetti M, et al. (2001) Magnetic resonance classification of lumbar intervertebral disc degeneration. Spine 26: 1873-1878. doi: 10.1097/00007632-200109010-00011
![]() |
[68] |
Toyoda H, Takahashi S, Hoshino M, et al. (2017) Characterizing the course of back pain after osteoporotic vertebral fracture: a hierarchical cluster analysis of a prospective cohort study. Arch Osteoporos 12: 82. doi: 10.1007/s11657-017-0377-5
![]() |
[69] |
Jeffrey EA, Craig M, Zhiyue JW, et al. (1997) Prediction of posterior fossa tumor type in children by means of magnetic resonance image properties, spectroscopy, and neural networks. J Neurosurg 86: 755-761. doi: 10.3171/jns.1997.86.5.0755
![]() |
[70] |
Kitajima M, Hirai T, Katsuragawa S, et al. (2009) Differentiation of common large sellar-suprasellar masses: effect of artificial neural network on radiologists' diagnosis performance. Acad Radiol 16: 313-320. doi: 10.1016/j.acra.2008.09.015
![]() |
[71] |
Christy PS, Tervonen O, Scheithauer BW, et al. (1995) Use of a neural network and a multiple regression model to predict histologic grade of astrocytoma from MRI appearances. Neuroradiology 37: 89-93. doi: 10.1007/BF00588619
![]() |
[72] |
Juntu J, Sijbers J, De Backer S, et al. (2010) Machine learning study of several classifiers trained with texture analysis features to differentiate benign from malignant soft-tissue tumors in T1-MRI images. J Magn Reson Imaging 31: 680-689. doi: 10.1002/jmri.22095
![]() |
[73] |
Zhao Z-X, Lan K, Xiao JH, et al. (2010) A new method to classify pathologic grades of astrocytomas based on magnetic resonance imaging appearances. Neurology India 58: 685-690. doi: 10.4103/0028-3886.72161
![]() |
[74] |
Sinha M, Kennedy CS, Ramundo, et al. (2001) Artificial neural network predicts CT scan abnormalities in pediatric patients with closed head injury. J Trauma 50: 308-312. doi: 10.1097/00005373-200102000-00018
![]() |
[75] |
Chiang S, Levin HS, Haneef Z (2015) Computer-automated focus lateralization of temporal lobe epilepsy using fMRI. J Magn Reson Imaging 41: 1689-1694. doi: 10.1002/jmri.24696
![]() |
[76] |
Berg AT, Vickrey BG, Langfitt JT, et al. (2003) The multicenter study of epilepsy surgery: recruitment and selection for surgery. Epilepsia 44: 1425-1433. doi: 10.1046/j.1528-1157.2003.24203.x
![]() |
[77] |
Tankus A, Yeshurun Y, Fried I (2009) An automatic measure for classifying clusters of suspected spikes into single cells versus multiunits. J Neural Eng 6: 056001. doi: 10.1088/1741-2560/6/5/056001
![]() |
[78] |
Anand IR, Travis MD, Zhenyu L, et al. (2010) Use of an artificial neural network to predict head injury outcome. J Neurosurg 113: 585-590. doi: 10.3171/2009.11.JNS09857
![]() |
[79] |
Chang K, Bai HX, Zhou H, et al. (2018) Residual convolutional neural network for the determination of IDH status in low- and high-grade gliomas from MR Imaging. Clin Cancer Res 24: 1073-1081. doi: 10.1158/1078-0432.CCR-17-2236
![]() |
[80] |
Yu J, Shi Z, Lian Y, et al. (2017) Noninvasive IDH1 mutation estimation based on a quantitative radiomics approach for grade II glioma. Eur Radiol 27: 3509-3522. doi: 10.1007/s00330-016-4653-3
![]() |
[81] |
Hollon TC, Pandian B, Adapa AR, et al. (2020) Near real-time intraoperative brain tumor diagnosis using stimulated raman histology and deep neural networks. Nat Med 26: 52-58. doi: 10.1038/s41591-019-0715-9
![]() |
[82] |
Gal AA, Cagle PT (2005) The 100-year anniversary of the description of the frozen section procedure. JAMA 294: 3135-3137. doi: 10.1001/jama.294.24.3135
![]() |
[83] | Novis D, Zarbo R (1997) Interinstitutional comparison of frozen section turnaround time. A college of American Pathologists Q-Probes study of 32868 frozen sections in 700 hospitals. Arch Pathol Lab Med 121: 559-567. |
[84] |
Mosa ASM, Yoo I, Sheets L (2012) A systematic review of healthcare applications for smartphones. BMC Med Inform Decis Mak 12: 67. doi: 10.1186/1472-6947-12-67
![]() |
[85] | Bardram JE, Bossen C (2005) Mobility Work: The spatial dimension of collaboration at a hospital. CSCW 14: 131-160. |
[86] |
Hector EJ (2016) Pediatric neurosurgery telemedicine clinics: a model to provide care to geographically underserved areas of the United States and its territories. J Neurosurg Pediatr 18: 753-757. doi: 10.3171/2016.6.PEDS16202
![]() |
[87] |
Reider-Demer M, Raja P, Martin N, et al. (2018) Prospective and retrospective study of videoconference telemedicine follow-up after elective neurosurgery: results of a pilot program. Neurosurg Rev 41: 497-501. doi: 10.1007/s10143-017-0878-0
![]() |
[88] | Susan RS (2018) Editorial. Telemedicine for elective neurosurgical routine follow-up care: a promising patient-centered and cost-effective alternative to in-person clinic visits. Neurosurg Focus 44: E18. |
[89] |
Semple JL, Armstrong KA (2017) Mobile applications for postoperative monitoring after discharge. CMAJ 189: E22-E24. doi: 10.1503/cmaj.160195
![]() |
[90] |
Layard Horsfall H, Palmisciano P, Khan DZ, et al. (2021) Attitudes of the surgical team toward artificial intelligence in neurosurgery: international 2-stage cross-sectional survey. World Neurosurg 146: e724-e730. doi: 10.1016/j.wneu.2020.10.171
![]() |
[91] |
Tsermoulas G, Zisakis A, Flint G, et al. (2020) Challenges to neurosurgery during the coronavirus disease 2019 (COVID-19) pandemic. World Neurosurg 139: 519-525. doi: 10.1016/j.wneu.2020.05.108
![]() |
[92] |
Zemmar A, Lozano AM, Nelson BJ (2020) The rise of robots in surgical environments during COVID-19. Nat Mach Intell 2: 566-572. doi: 10.1038/s42256-020-00238-2
![]() |
[93] | Michael CD, Ronnie EB, Abbas R, et al. (2018) Pediatric neurosurgical workforce, access to care, equipment and training needs worldwide. Neurosurg Focus 45: E13. |
[94] |
Mofatteh M (2021) Risk factors associated with stress, anxiety, and depression among university undergraduate students. AIMS Public Health 8: 36-65. doi: 10.3934/publichealth.2021004
![]() |
[95] |
Stein SC (2018) Cost-effectiveness research in neurosurgery: we can and we must. Neurosurgery 83: 871-878. doi: 10.1093/neuros/nyx583
![]() |
[96] |
Sejnowski TJ (2020) The unreasonable effectiveness of deep learning in artificial intelligence. Proc Natl Acad Sci 117: 30033-30038. doi: 10.1073/pnas.1907373117
![]() |
[97] |
Bell C, Shenoy P, Chalodhorn R, et al. (2008) Control of a humanoid robot by a noninvasive brain-computer interface in humans. J Neural Eng 5: 214-220. doi: 10.1088/1741-2560/5/2/012
![]() |
[98] |
Zhang X, Ma Z, Zheng H, et al. (2020) The combination of brain-computer interfaces and artificial intelligence: applications and challenges. Ann Transl Med 8: 712. doi: 10.21037/atm.2019.11.109
![]() |
[99] | National Spinal Cord Injury Statistical Center (2021) Facts and Figures at a Glance Birmingham, AL: University of Alabama at Birmingham. |
[100] |
Li M, Cui Y, Hao D, et al. (2015) An adaptive feature extraction method in BCI-based rehabilitation. J Intell Fuzzy Syst 28: 525-535. doi: 10.3233/IFS-141329
![]() |
[101] |
Bouton CE, Shaikhouni A, Annetta NV, et al. (2016) Restoring cortical control of functional movement in a human with quadriplegia. Nature 533: 247-250. doi: 10.1038/nature17435
![]() |
[102] |
Flesher SN, Downey JE, Weiss JM, et al. (2021) A brain-computer interface that evokes tactile sensations improves robotic arm control. Science 372: 831. doi: 10.1126/science.abd0380
![]() |
[103] |
Bauer R, Gharabaghi A (2015) Reinforcement learning for adaptive threshold control of restorative brain-computer interfaces: a Bayesian simulation. Front Neurosci 9: 36. doi: 10.3389/fnins.2015.00036
![]() |
[104] |
Palmisciano P, Jamjoom AAB, Taylor D, et al. (2020) Attitudes of patients and their relatives toward artificial intelligence in neurosurgery. World Neurosurg 138: e627-e633. doi: 10.1016/j.wneu.2020.03.029
![]() |
[105] |
Leite M, Leal A, Figueiredo P (2013) Transfer function between EEG and BOLD signals of epileptic activity. Front Neurology 4: 1. doi: 10.3389/fneur.2013.00001
![]() |
[106] |
Abdolmaleki P, Mihara F, Masuda K, et al. (1997) Neural networks analysis of astrocytic gliomas from MRI appearances. Cancer Lett 118: 69-78. doi: 10.1016/S0304-3835(97)00233-4
![]() |
[107] |
Chari A, Budhdeo S, Sparks R, et al. (2021) Brain-machine interfaces: The role of the neurosurgeon. World Neurosurg 146: 140-147. doi: 10.1016/j.wneu.2020.11.028
![]() |
[108] |
Bonaci T, Calo R, Chizeck HJ (2015) App stores for the brain: privacy and security in brain-computer interfaces. IEEE Technol Soc Mag 34: 32-39. doi: 10.1109/MTS.2015.2425551
![]() |
[109] | Collins JW, Marcus HJ, Ghazi A, et al. (2021) Ethical implications of AI in robotic surgical training: A Delphi consensus statement. Eur Urol Focus . |
[110] |
Groiss SJ, Wojtecki L, Südmeyer M, et al. (2009) Deep brain stimulation in Parkinson's disease. Ther Adv Neurol Disord 2: 20-28. doi: 10.1177/1756285609339382
![]() |
[111] |
Mofatteh M (2020) mRNA localization and local translation in neurons. AIMS Neurosci 7: 299-310. doi: 10.3934/Neuroscience.2020016
![]() |
[112] | Mofatteh M (2021) Neurodegeneration and axonal mRNA transportation. Am J Neurodegener Dis 10: 1-12. |
[113] |
Pinto dos Santos D, Giese D, Brodehl S, et al. (2019) Medical students' attitude towards artificial intelligence: a multicentre survey. Eur Radiol 29: 1640-1646. doi: 10.1007/s00330-018-5601-1
![]() |
1. | Kin Keung Lai, Mohd Hassan, Jitendra Kumar Maurya, Sanjeev Kumar Singh, Shashi Kant Mishra, Multiobjective Convex Optimization in Real Banach Space, 2021, 13, 2073-8994, 2148, 10.3390/sym13112148 |