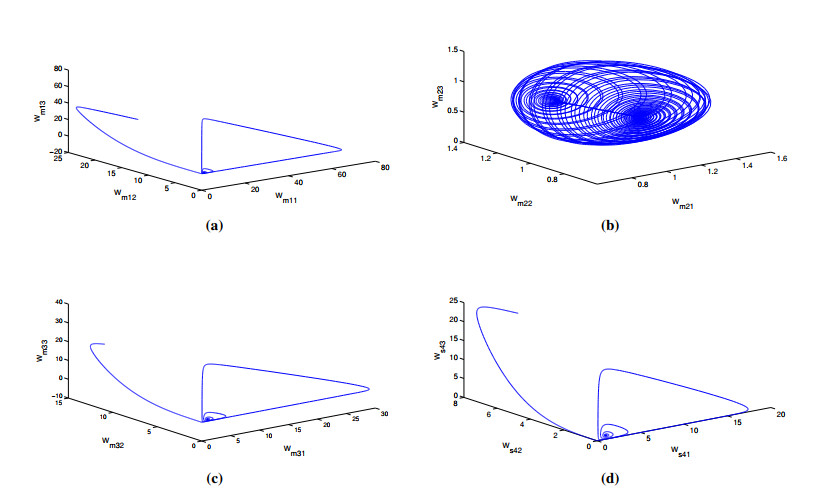
Citation: Siming Liu, Honglei Gao, Peng Hou, Yong Tan. Risk spillover effects of international crude oil market on China’s major markets[J]. AIMS Energy, 2019, 7(6): 819-840. doi: 10.3934/energy.2019.6.819
[1] | Xixia Ma, Rongsong Liu, Liming Cai . Stability of traveling wave solutions for a nonlocal Lotka-Volterra model. Mathematical Biosciences and Engineering, 2024, 21(1): 444-473. doi: 10.3934/mbe.2024020 |
[2] | S. Nakaoka, Y. Saito, Y. Takeuchi . Stability, delay, and chaotic behavior in a Lotka-Volterra predator-prey system. Mathematical Biosciences and Engineering, 2006, 3(1): 173-187. doi: 10.3934/mbe.2006.3.173 |
[3] | Pan Yang, Jianwen Feng, Xinchu Fu . Cluster collective behaviors via feedback pinning control induced by epidemic spread in a patchy population with dispersal. Mathematical Biosciences and Engineering, 2020, 17(5): 4718-4746. doi: 10.3934/mbe.2020259 |
[4] | Zigen Song, Jian Xu, Bin Zhen . Mixed-coexistence of periodic orbits and chaotic attractors in an inertial neural system with a nonmonotonic activation function. Mathematical Biosciences and Engineering, 2019, 16(6): 6406-6425. doi: 10.3934/mbe.2019320 |
[5] | Paul Georgescu, Hong Zhang, Daniel Maxin . The global stability of coexisting equilibria for three models of mutualism. Mathematical Biosciences and Engineering, 2016, 13(1): 101-118. doi: 10.3934/mbe.2016.13.101 |
[6] | Gayathri Vivekanandhan, Hamid Reza Abdolmohammadi, Hayder Natiq, Karthikeyan Rajagopal, Sajad Jafari, Hamidreza Namazi . Dynamic analysis of the discrete fractional-order Rulkov neuron map. Mathematical Biosciences and Engineering, 2023, 20(3): 4760-4781. doi: 10.3934/mbe.2023220 |
[7] | Biwen Li, Xuan Cheng . Synchronization analysis of coupled fractional-order neural networks with time-varying delays. Mathematical Biosciences and Engineering, 2023, 20(8): 14846-14865. doi: 10.3934/mbe.2023665 |
[8] | Yuanshi Wang, Donald L. DeAngelis . A mutualism-parasitism system modeling host and parasite with mutualism at low density. Mathematical Biosciences and Engineering, 2012, 9(2): 431-444. doi: 10.3934/mbe.2012.9.431 |
[9] | Xiaomeng Ma, Zhanbing Bai, Sujing Sun . Stability and bifurcation control for a fractional-order chemostat model with time delays and incommensurate orders. Mathematical Biosciences and Engineering, 2023, 20(1): 437-455. doi: 10.3934/mbe.2023020 |
[10] | Sai Zhang, Li Tang, Yan-Jun Liu . Formation deployment control of multi-agent systems modeled with PDE. Mathematical Biosciences and Engineering, 2022, 19(12): 13541-13559. doi: 10.3934/mbe.2022632 |
The constituent members in a system mainly found in nature can be interacting with each other through cooperation and competition. Demonstrations for such systems involve biological species, countries, businesses, and many more. It's very much intriguing to investigate in a comprehensive manner numerous social as well as biological interactions existent in dissimilar species/entities utilizing mathematical modeling. The predation and the competition species are the most famous interactions among all such types of interactions. Importantly, Lotka [1] and Volterra [2] in the 1920s have announced individually the classic equations portraying population dynamics. Such illustrious equations are notably described as predator-prey (PP) equations or Lotka-Volterra (LV) equations. In this structure, PP/LV model represents the most influential model for interacting populations. The interplay between prey and predator together with additional factors has been a prominent topic in mathematical ecology for a long period. Arneodo et al. [3] have established in 1980 that a generalized Lotka-Volterra biological system (GLVBS) would depict chaos phenomena in an ecosystem for some explicitly selected system parameters and initial conditions. Additionally, Samardzija and Greller [4] demonstrated in 1988 that GLVBS would procure chaotic reign from the stabled state via rising fractal torus. LV model was initially developed as a biological concept, yet it is utilized in enormous diversified branches for research [5,6,7,8]. Synchronization essentially is a methodology of having different chaotic systems (non-identical or identical) following exactly a similar trajectory, i.e., the dynamical attributes of the slave system are locked finally into the master system. Specifically, synchronization and control have a wide spectrum for applications in engineering and science, namely, secure communication [9], encryption [10,11], ecological model [12], robotics [13], neural network [14], etc. Recently, numerous types of secure communication approaches have been explored [15,16,17,18] such as chaos modulation [18,19,20,21], chaos shift keying [22,23] and chaos masking [9,17,20,24]. In chaos communication schemes, the typical key idea for transmitting a message through chaotic/hyperchaotic models is that a message signal is nested in the transmitter system/model which originates a chaotic/ disturbed signal. Afterwards, this disturbed signal has been emitted to the receiver through a universal channel. The message signal would finally be recovered by the receiver. A chaotic model has been intrinsically employed both as receiver and transmitter. Consequently, this area of chaotic synchronization & control has sought remarkable considerations among differential research fields.
Most prominently, synchronization theory has been in existence for over 30 years due to the phenomenal research of Pecora and Carroll [25] established in 1990 using drive-response/master-slave/leader-follower configuration. Consequently, many authors and researchers have started introducing and studying numerous control and synchronization methods [9,26,27,28,29,30,31,32,33,34,35,36] etc. to achieve stabilized chaotic systems for possessing stability. In [37], researchers discussed optimal synchronization issues in similar GLVBSs via optimal control methodology. In [38,39], the researchers studied the adaptive control method (ACM) to synchronize chaotic GLVBSs. Also, researchers [40] introduced a combination difference anti-synchronization scheme in similar chaotic GLVBSs via ACM. In addition, authors [41] investigated a combination synchronization scheme to control chaos existing in GLVBSs using active control strategy (ACS). Bai and Lonngren [42] first proposed ACS in 1997 for synchronizing and controlling chaos found in nonlinear dynamical systems. Furthermore, compound synchronization using ACS was first advocated by Sun et al. [43] in 2013. In [44], authors discussed compound difference anti-synchronization scheme in four chaotic systems out of which two chaotic systems are considered as GLVBSs using ACS and ACM along with applications in secure communications of chaos masking type in 2019. Some further research works [45,46] based on ACS have been reported in this direction. The considered chaotic GLVBS offers a generalization that allows higher-order biological terms. As a result, it may be of interest in cases where biological systems experience cataclysmic changes. Unfortunately, some species will be under competitive pressure in the coming years and decades. This work may be comprised as a step toward preserving as many currently living species as possible by using the proposed synchronization approach which is based on master-slave configuration and Lyapunov stability analysis.
In consideration of the aforementioned discussions and observations, our primary focus here is to develop a systematic approach for investigating compound difference anti-synchronization (CDAS) approach in 4 similar chaotic GLVBSs via ACS. The considered ACS is a very efficient yet theoretically rigorous approach for controlling chaos found in GLVBSs. Additionally, in view of widely known Lyapunov stability analysis (LSA) [47], we discuss actively designed biological control law & convergence for synchronization errors to attain CDAS synchronized states.
The major attributes for our proposed research in the present manuscript are:
● The proposed CDAS methodology considers four chaotic GLVBSs.
● It outlines a robust CDAS approach based active controller to achieve compound difference anti-synchronization in discussed GLVBSs & conducts oscillation in synchronization errors along with extremely fast convergence.
● The construction of the active control inputs has been executed in a much simplified fashion utilizing LSA & master-salve/ drive-response configuration.
● The proposed CDAS approach in four identical chaotic GLVBSs of integer order utilizing ACS has not yet been analyzed up to now. This depicts the novelty of our proposed research work.
This manuscript is outlined as follows: Section 2 presents the problem formulation of the CDAS scheme. Section 3 designs comprehensively the CDAS scheme using ACS. Section 4 consists of a few structural characteristics of considered GLVBS on which CDAS is investigated. Furthermore, the proper active controllers having nonlinear terms are designed to achieve the proposed CDAS strategy. Moreover, in view of Lyapunov's stability analysis (LSA), we have examined comprehensively the biological controlling laws for achieving global asymptotical stability of the error dynamics for the discussed model. In Section 5, numerical simulations through MATLAB are performed for the illustration of the efficacy and superiority of the given scheme. Lastly, we also have presented some conclusions and the future prospects of the discussed research work in Section 6.
We here formulate a methodology to examine compound difference anti-synchronization (CDAS) scheme viewing master-slave framework in four chaotic systems which would be utilized in the coming up sections.
Let the scaling master system be
˙wm1= f1(wm1), | (2.1) |
and the base second master systems be
˙wm2= f2(wm2), | (2.2) |
˙wm3= f3(wm3). | (2.3) |
Corresponding to the aforementioned master systems, let the slave system be
˙ws4= f4(ws4)+U(wm1,wm2,wm3,ws4), | (2.4) |
where wm1=(wm11,wm12,...,wm1n)T∈Rn, wm2=(wm21,wm22,...,wm2n)T∈Rn, wm3=(wm31,wm32,...,wm3n)T∈Rn, ws4=(ws41,ws42,...,ws4n)T∈Rn are the state variables of the respective chaotic systems (2.1)–(2.4), f1,f2,f3,f4:Rn→Rn are four continuous vector functions, U=(U1,U2,...,Un)T:Rn×Rn×Rn×Rn→Rn are appropriately constructed active controllers.
Compound difference anti-synchronization error (CDAS) is defined as
E=Sws4+Pwm1(Rwm3−Qwm2), |
where P=diag(p1,p2,.....,pn),Q=diag(q1,q2,.....,qn),R=diag(r1,r2,.....,rn),S=diag(s1,s2,.....,sn) and S≠0.
Definition: The master chaotic systems (2.1)–(2.3) are said to achieve CDAS with slave chaotic system (2.4) if
limt→∞‖E(t)‖=limt→∞‖Sws4(t)+Pwm1(t)(Rwm3(t)−Qwm2(t))‖=0. |
We now present our proposed CDAS approach in three master systems (2.1)–(2.3) and one slave system (2.4). We next construct the controllers based on CDAS approach by
Ui= ηisi−(f4)i−KiEisi, | (3.1) |
where ηi=pi(f1)i(riwm3i−qiwm2i)+piwm1i(ri(f3)i−qi(f2)i), for i=1,2,...,n.
Theorem: The systems (2.1)–(2.4) will attain the investigated CDAS approach globally and asymptotically if the active control functions are constructed in accordance with (3.1).
Proof. Considering the error as
Ei= siws4i+piwm1i(riwm3i−qiwm2i),fori=1,2,3,.....,n. |
Error dynamical system takes the form
˙Ei= si˙ws4i+pi˙wm1i(riwm3i−qiwm2i)+piwm1i(ri˙wm3i−qi˙wm2i)= si((f4)i+Ui)+pi(f1)i(riwm3i−qiwm2i)+piwm1i(ri(f3)i−qi(f2)i)= si((f4)i+Ui)+ηi, |
where ηi=pi(f1)i(riwm3i−qiwm2i)+piwm1i(ri(f3)i−qi(f2)i), i=1,2,3,....,n. This implies that
˙Ei= si((f4)i−ηisi−(f4)i−KiEisi)+ηi= −KiEi | (3.2) |
The classic Lyapunov function V(E(t)) is described by
V(E(t))= 12ETE= 12ΣE2i |
Differentiation of V(E(t)) gives
˙V(E(t))=ΣEi˙Ei |
Using Eq (3.2), one finds that
˙V(E(t))=ΣEi(−KiEi)= −ΣKiE2i). | (3.3) |
An appropriate selection of (K1,K1,.......,Kn) makes ˙V(E(t)) of eq (3.3), a negative definite. Consequently, by LSA [47], we obtain
limt→∞Ei(t)=0,(i=1,2,3). |
Hence, the master systems (2.1)–(2.3) and slave system (2.4) have attained desired CDAS strategy.
We now describe GLVBS as the scaling master system:
{˙wm11=wm11−wm11wm12+b3w2m11−b1w2m11wm13,˙wm12=−wm12+wm11wm12,˙wm13=b2wm13+b1w2m11wm13, | (4.1) |
where (wm11,wm12,wm13)T∈R3 is state vector of (4.1). Also, wm11 represents the prey population and wm12, wm13 denote the predator populations. For parameters b1=2.9851, b2=3, b3=2 and initial conditions (27.5,23.1,11.4), scaling master GLVBS displays chaotic/disturbed behaviour as depicted in Figure 1(a).
The base master systems are the identical chaotic GLVBSs prescribed respectively as:
{˙wm21=wm21−wm21wm22+b3w2m21−b1w2m21wm23,˙wm22=−wm22+wm21wm22,˙wm23=b2wm23+b1w2m21wm23, | (4.2) |
where (wm21,wm22,wm23)T∈R3 is state vector of (4.2). For parameter values b1=2.9851, b2=3, b3=2, this base master GLVBS shows chaotic/disturbed behaviour for initial conditions (1.2,1.2,1.2) as displayed in Figure 1(b).
{˙wm31=wm31−wm31wm32+b3w2m31−b1w2m31wm33,˙wm32=−wm32+wm31wm32,˙wm33=b2wm33+b1w2m31wm33, | (4.3) |
where (wm31,wm32,wm33)T∈R3 is state vector of (4.3). For parameters b1=2.9851, b2=3, b3=2, this second base master GLVBS displays chaotic/disturbed behaviour for initial conditions (2.9,12.8,20.3) as shown in Figure 1(c).
The slave system, represented by similar GLVBS, is presented by
{˙ws41=ws41−ws41ws42+b3w2s41−b1w2s41ws43+U1,˙ws42=−ws42+ws41ws42+U2,˙ws43=b2ws43+b1w2s41ws43+U3, | (4.4) |
where (ws41,ws42,ws43)T∈R3 is state vector of (4.4). For parameter values, b1=2.9851, b2=3, b3=2 and initial conditions (5.1,7.4,20.8), the slave GLVBS exhibits chaotic/disturbed behaviour as mentioned in Figure 1(d).
Moreover, the detailed theoretical study for (4.1)–(4.4) can be found in [4]. Further, U1, U2 and U3 are controllers to be determined.
Next, the CDAS technique has been discussed for synchronizing the states of chaotic GLVBS. Also, LSA-based ACS is explored & the necessary stability criterion is established.
Here, we assume P=diag(p1,p2,p3), Q=diag(q1,q2,q3), R=diag(r1,r2,r3), S=diag(s1,s2,s3). The scaling factors pi,qi,ri,si for i=1,2,3 are selected as required and can assume the same or different values.
The error functions (E1,E2,E3) are defined as:
{E1=s1ws41+p1wm11(r1wm31−q1wm21),E2=s2ws42+p2wm12(r2wm32−q2wm22),E3=s3ws43+p3wm13(r3wm33−q3wm23). | (4.5) |
The major objective of the given work is the designing of active control functions Ui,(i=1,2,3) ensuring that the error functions represented in (4.5) must satisfy
limt→∞Ei(t)=0for(i=1,2,3). |
Therefore, subsequent error dynamics become
{˙E1=s1˙ws41+p1˙wm11(r1wm31−q1wm21)+p1wm11(r1˙wm31−q1˙wm21),˙E2=s2˙ws42+p2˙wm12(r2wm32−q2wm22)+p2wm12(r2˙wm32−q2˙wm22),˙E3=s3˙ws43+p3˙wm13(r3wm33−q3wm23)+p3wm13(r3˙wm33−q3˙wm23). | (4.6) |
Using (4.1), (4.2), (4.3), and (4.5) in (4.6), the error dynamics simplifies to
{˙E1=s1(ws41−ws41ws42+b3w2s41−b1w2s41ws43+U1)+p1(wm11−wm11wm12+b3w2m11−b1w2m11wm13)(r1wm31−q1wm21)+p1wm11(r1(wm31−wm31wm32+b3w2m31−b1w2m31wm33)−q1(wm21−wm21wm22+b3w2m21−b1w2m21wm23),˙E2=s2(−ws42+ws41ws42+U2)+p2(−wm12+wm11wm12)(r2wm32−q2wm22)+p2wm12(r2(−wm32+wm31wm32)−q2(−wm22+wm21wm22)),˙E3=s3(b2ws43+b1w2s41ws43+U3)+p3(b2wm13+b1w2m11wm13)(r3wm33−q3wm23)+p3wm13(r3(b2wm33+b1w2m31wm33)−q3(b2wm23+b1w2m21wm23)). | (4.7) |
Let us now choose the active controllers:
U1= η1s1−(f4)1−K1E1s1, | (4.8) |
where η1=p1(f1)1(r1wm31−q1wm21)+p1wm11(r1(f3)1−q1(f2)1), as described in (3.1).
U2= η2s2−(f4)2−K2E2s2, | (4.9) |
where η2=p2(f1)2(r2wm32−q2wm22)+p2wm12(r2(f3)2−q2(f2)2).
U3= η3s3−(f4)3−K3E3s3, | (4.10) |
where η3=p3(f1)3(r3wm33−q3wm23)+p3wm13(r3(f3)3−q3(f2)3) and K1>0,K2>0,K3>0 are gaining constants.
By substituting the controllers (4.8), (4.9) and (4.10) in (4.7), we obtain
{˙E1=−K1E1,˙E2=−K2E2,˙E3=−K3E3. | (4.11) |
Lyapunov function V(E(t)) is now described by
V(E(t))= 12[E21+E22+E23]. | (4.12) |
Obviously, the Lyapunov function V(E(t)) is +ve definite in R3. Therefore, the derivative of V(E(t)) as given in (4.12) can be formulated as:
˙V(E(t))= E1˙E1+E2˙E2+E3˙E3. | (4.13) |
Using (4.11) in (4.13), one finds that
˙V(E(t))= −K1E21−K2E22−K3E23<0, |
which displays that ˙V(E(t)) is -ve definite.
In view of LSA [47], we, therefore, understand that CDAS error dynamics is globally as well as asymptotically stable, i.e., CDAS error E(t)→0 asymptotically for t→∞ to each initial value E(0)∈R3.
This section conducts a few simulation results for illustrating the efficacy of the investigated CDAS scheme in identical chaotic GLVBSs using ACS. We use 4th order Runge-Kutta algorithm for solving the considered ordinary differential equations. Initial conditions for three master systems (4.1)–(4.3) and slave system (4.4) are (27.5,23.1,11.4), (1.2,1.2,1.2), (2.9,12.8,20.3) and (14.5,3.4,10.1) respectively. We attain the CDAS technique among three masters (4.1)–(4.3) and corresponding one slave system (4.4) by taking pi=qi=ri=si=1, which implies that the slave system would be entirely anti-synchronized with the compound of three master models for i=1,2,3. In addition, the control gains (K1,K2,K3) are taken as 2. Also, Figure 2(a)–(c) indicates the CDAS synchronized trajectories of three master (4.1)–(4.3) & one slave system (4.4) respectively. Moreover, synchronization error functions (E1,E2,E3)=(51.85,275.36,238.54) approach 0 as t tends to infinity which is exhibited via Figure 2(d). Hence, the proposed CDAS strategy in three masters and one slave models/systems has been demonstrated computationally.
In this work, the investigated CDAS approach in similar four chaotic GLVBSs using ACS has been analyzed. Lyapunov's stability analysis has been used to construct proper active nonlinear controllers. The considered error system, on the evolution of time, converges to zero globally & asymptotically via our appropriately designed simple active controllers. Additionally, numerical simulations via MATLAB suggest that the newly described nonlinear control functions are immensely efficient in synchronizing the chaotic regime found in GLVBSs to fitting set points which exhibit the efficacy and supremacy of our proposed CDAS strategy. Exceptionally, both analytic theory and computational results are in complete agreement. Our proposed approach is simple yet analytically precise. The control and synchronization among the complex GLVBSs with the complex dynamical network would be an open research problem. Also, in this direction, we may extend the considered CDAS technique on chaotic systems that interfered with model uncertainties as well as external disturbances.
The authors gratefully acknowledge Qassim University, represented by the Deanship of Scientific Research, on the financial support for this research under the number 10163-qec-2020-1-3-I during the academic year 1441 AH/2020 AD.
The authors declare there is no conflict of interest.
[1] |
Balcilar M, Hammoudeh S, Toparli E (2018) On the risk spillover across the oil market, stock market, and the oil related CDS sectors: A volatility impulse response approach. Energy Econ 74: 813-827. doi: 10.1016/j.eneco.2018.07.027
![]() |
[2] |
Bouri E (2015) A broadened causality in variance approach to assess the risk dynamics between crude oil prices and the jordanian stock market. Energy Policy 85: 271-279. doi: 10.1016/j.enpol.2015.06.001
![]() |
[3] |
Ji Q, Liu B, Fan Y (2019) Risk dependence of CoVaR and structural change between oil prices and exchange rates: A time-varying copula model. Energy Econ 77: 80-92. doi: 10.1016/j.eneco.2018.07.012
![]() |
[4] |
Li X, Wei Y (2018) The dependence and risk spillover between crude oil market and China stock market: New evidence from a variational mode decomposition-based copula method. Energy Econ 74: 565-581. doi: 10.1016/j.eneco.2018.07.011
![]() |
[5] |
Mensi W, Hammoudeh S, Shahzad S, et al. (2017) Oil and foreign exchange market tail dependence and risk spillovers for MENA, emerging and developed countries: VMD decomposition based copulas. Energy Econ 67: 476-495. doi: 10.1016/j.eneco.2017.08.036
![]() |
[6] |
Mensi W, Hammoudeh S, Shahzad S, et al. (2017) Modeling systemic risk and dependence structure between oil and stock markets using a variational mode decomposition-based copula method. J Bank Financ 75: 258-279. doi: 10.1016/j.jbankfin.2016.11.017
![]() |
[7] |
Pavlova I, De Boyrie ME, Parhizgari AM (2018) A dynamic spillover analysis of crude oil effects on the sovereign credit risk of exporting countries. Q Rev Econ Financ 68: 10-22. doi: 10.1016/j.qref.2018.03.003
![]() |
[8] |
Shahzad S, Mensi W, Hammoudeh S, et al. (2018) Extreme dependence and risk spillovers between oil and Islamic stock markets. Emerg Mark Rev 34: 42-63. doi: 10.1016/j.ememar.2017.10.003
![]() |
[9] |
Wen D, Wang GJ, Ma C, et al. (2019) Risk spillovers between oil and stock markets: A VAR for VaR analysis. Energy Econ 80: 524-535. doi: 10.1016/j.eneco.2019.02.005
![]() |
[10] |
Du L, He Y (2015) Extreme risk spillovers between crude oil and stock markets. Energy Econ 51: 455-465. doi: 10.1016/j.eneco.2015.08.007
![]() |
[11] |
Hong Y, Liu Y, Wang S (2009) Granger causality in risk and detection of extreme risk spillover between financial markets. J Econometrics 150: 271-287. doi: 10.1016/j.jeconom.2008.12.013
![]() |
[12] |
Elyasiani E, Gambarelli L, Muzzioli S (2017) The information content of corridor volatility measures during calm and turmoil periods. Quant Financ Econ 1: 454-473. doi: 10.3934/QFE.2017.4.454
![]() |
[13] |
Hamao Y, Masulis RW, Ng V (1990) Correlations in price changes and volatility across international stock markets. Rev Financ Stud 3: 281-307. doi: 10.1093/rfs/3.2.281
![]() |
[14] |
Hong Y (2001) A test for volatility spillover with applications to exchange rates. J Econometrics 103: 183-224. doi: 10.1016/S0304-4076(01)00043-4
![]() |
[15] |
Madaleno M, Vieira E (2018) Volatility analysis of returns and risk: family versus nonfamily firms. Quant Financ Econ 2: 348-372. doi: 10.3934/QFE.2018.2.348
![]() |
[16] |
Kang W, Ratti RA, Yoon KH (2015) The impact of oil price shocks on the stock market return and volatility relationship. J Int Financ Mark Inst Money 34: 41-54. doi: 10.1016/j.intfin.2014.11.002
![]() |
[17] |
Xu W, Ma F, Chen W, et al. (2019) Asymmetric volatility spillovers between oil and stock markets: Evidence from China and the United States. Energy Econ 80: 310-320. doi: 10.1016/j.eneco.2019.01.014
![]() |
[18] |
Peng C, Zhu H, Guo Y, et al. (2018) Risk spillover of international crude oil to China's firms: Evidence from granger causality across quantile. Energy Econ 72: 188-199. doi: 10.1016/j.eneco.2018.04.007
![]() |
[19] |
Zhang J (2019) Oil and gas trade between China and countries and regions along the 'Belt and Road': A panoramic perspective. Energy Policy 129: 1111-1120. doi: 10.1016/j.enpol.2019.03.020
![]() |
[20] |
Ren YH, Gu WW (2017) The impact on RMB internationalization process: Exchange rate expectation and foreign trade. Quant Financ Econ 1:114-124. doi: 10.3934/QFE.2017.1.114
![]() |
[21] | Babalos V, Stavroyiannis S, Gupta R (2015) Do commodity investors herd? Evidence from a time-varying stochastic volatility model. Resour Policy 46: 281-287. |
[22] |
Irwin SH, Sanders DR (2011) Index funds, financialization, and commodity futures markets. Appl Econ Perspect Policy 33: 1-31. doi: 10.1093/aepp/ppq032
![]() |
[23] |
Tang K, Xiong W (2012) Index investment and the financialization of commodities. Financ Anal J 68: 54-74. doi: 10.2469/faj.v68.n6.5
![]() |
[24] |
Wen S, An H, Huang S, et al. (2019) Dynamic impact of China's stock market on the international commodity market. Resour Policy 61: 564-571. doi: 10.1016/j.resourpol.2018.06.009
![]() |
[25] | Zhang YJ, Chevallier J, Guesmi K (2017) 'De‐financialization' of commodities? Evidence from stock, crude oil and natural gas markets. Energy Econ 68: 228-239. |
[26] |
Adams Z, Glück T (2015) Financialization in commodity markets: A passing trend or the new normal? J Bank Financ 60: 93-111. doi: 10.1016/j.jbankfin.2015.07.008
![]() |
[27] | Cheng IH, Xiong W (2013) The financialization of commodity markets. NBER Working Paper No.19642. |
[28] |
Diebold FX, Yilmaz K (2012) Better to give than to receive: Predictive directional measurement of volatility spillovers. Int J Forecasting 28: 57-66. doi: 10.1016/j.ijforecast.2011.02.006
![]() |
[29] |
Hamilton JD (1983) Oil and the macroeconomy since World War II. J Polit Econ 91: 228-248. doi: 10.1086/261140
![]() |
[30] |
An Y, Sun M, Gao C, et al. (2018) Analysis of the impact of crude oil price fluctuations on China's stock market in different periods-Based on time series network model. Physica A 492: 1016-1031. doi: 10.1016/j.physa.2017.11.032
![]() |
[31] |
Atems B, Kapper D, Lam E (2015) Do exchange rates respond asymmetrically to shocks in the crude oil market? Energy Econ 49: 227-238. doi: 10.1016/j.eneco.2015.01.027
![]() |
[32] |
Bildirici ME, Badu MM (2018) The effects of oil prices on confidence and stock return in China, India and Russia. Quant Financ Econ 2: 884-903. doi: 10.3934/QFE.2018.4.884
![]() |
[33] | Chen H, Liu L, Wang Y, et al. (2016) Oil price shocks and U.S. dollar exchange rates. Energy 112: 1036-1048. |
[34] |
Ghosh S, Kanjilal K (2016) Co-movement of international crude oil price and Indian stock market: evidences from nonlinear cointegration tests. Energy Econ 53: 111-117. doi: 10.1016/j.eneco.2014.11.002
![]() |
[35] |
Kang SH, Mciver R, Yoon SM (2017) Dynamic spillover effects among crude oil, precious metal, and agricultural commodity futures markets. Energy Econ 62: 19-32. doi: 10.1016/j.eneco.2016.12.011
![]() |
[36] |
Khalfaoui R, Boutahar M, Boubaker H (2015) Analyzing volatility spillovers and hedging between oil and stock markets: Evidence from wavelet analysis. Energy Econ 49: 540-549. doi: 10.1016/j.eneco.2015.03.023
![]() |
[37] |
Luo J, Ji Q (2018) High-frequency volatility connectedness between the US crude oil market and China's agricultural commodity markets. Energy Econ 76: 424-438. doi: 10.1016/j.eneco.2018.10.031
![]() |
[38] |
Mensi W, Beljid M, Boubaker A, et al. (2013) Correlations and volatility spillovers across commodity and stock markets: Linking energies, food, and gold. Econ Model 32: 15-22. doi: 10.1016/j.econmod.2013.01.023
![]() |
[39] |
Turhan MI, Sensoy A, Hacihasanoglu E (2014) A comparative analysis of the dynamic relationship between oil prices and exchange rates. J Int Financ Mark Inst Money 32: 397-414. doi: 10.1016/j.intfin.2014.07.003
![]() |
[40] |
Ji Q, Bouri E, Roubaud D, et al. (2018) Risk spillover between energy and agricultural commodity markets: A dependence-switching CoVaR-copula model. Energy Econ 75: 14-27. doi: 10.1016/j.eneco.2018.08.015
![]() |
[41] |
Algieri B, Leccadito A (2017) Assessing contagion risk from energy and non-energy commodity markets. Energy Econ 62: 312-322. doi: 10.1016/j.eneco.2017.01.006
![]() |
[42] |
Wang GJ, Xie C, Jiang ZQ, et al. (2016) Who are the net senders and recipients of volatility spillovers in China's financial markets? Financ Res Lett 18: 255-262. doi: 10.1016/j.frl.2016.04.025
![]() |
[43] |
Demirer M, Diebold FX, Liu L, et al. (2018). Estimating global bank network connectedness. J App Econom 33: 1-15. doi: 10.1002/jae.2585
![]() |
[44] |
Wang GJ, Xie C, Zhao LF, et al. (2018) Volatility connectedness in the Chinese banking system: Do state-owned commercial banks contribute more? J In Financ Mark Inst Money 57: 205-230. doi: 10.1016/j.intfin.2018.07.008
![]() |
[45] |
Yi SY, Xu ZS, Wang GJ (2018) Volatility connectedness in the cryptocurrency market: Is Bitcoin a dominant cryptocurrency? Int Rev Financ Anal 60: 98-114. doi: 10.1016/j.irfa.2018.08.012
![]() |
[46] |
Koop G, Pesaran MH, Potter SM (1996) Impulse response analysis in nonlinear multivariate models. J Econometrics 74:119-147. doi: 10.1016/0304-4076(95)01753-4
![]() |
[47] |
Pesaran MH, Shin Y (1998) Generalised impulse response analysis in linear multivariate models. Econ Lett 58: 17-29. doi: 10.1016/S0165-1765(97)00214-0
![]() |
[48] |
Engle RF, Manganelli S (2004) CAViaR: Conditional autoregressive value at risk by regression quantiles. J Bus Econ Stat 22: 367-381. doi: 10.1198/073500104000000370
![]() |
[49] | Qureshi K (2016) Value-at-Risk: The effect of autoregression in a quantile process. arXiv preprint arXiv: 1605.04940. |
[50] |
Bernardi M, Catania L (2016) Comparison of Value-at-Risk models using the MCS approach. Comput Stat 31: 579-608. doi: 10.1007/s00180-016-0646-6
![]() |
[51] |
Ferraty F, Quintela-Del-Río A (2016) Conditional VAR and expected shortfall: A new functional approach. Econ Rev 35: 263-292. doi: 10.1080/07474938.2013.807107
![]() |
[52] |
Gerlach R, Lu ZD, Huang H (2013) Exponentially smoothing the skewed laplace distribution for Value-at-Risk forecasting. J Forecasting 32: 534-550. doi: 10.1002/for.2255
![]() |
[53] |
Gkillas K, Katsiampa P (2018) An application of extreme value theory to cryptocurrencies. Econ Lett 164: 109-111. doi: 10.1016/j.econlet.2018.01.020
![]() |
[54] |
Li ZH, Dong H, Huang ZH, et al. (2018) Asymmetric effects on risks of Virtual Financial Assets (VFAs) in different regimes: A case of bitcoin. Quant Financ Econ 2: 860-883. doi: 10.3934/QFE.2018.4.860
![]() |
[55] |
Righi MB, Ceretta PS (2015) Forecasting value at risk and expected shortfall based on serial pair-copula constructions. Expert Sys Appl 42: 6380-6390. doi: 10.1016/j.eswa.2015.04.023
![]() |
[56] |
Whitea H, Kimb TH, Manganelli S (2015) VAR for VaR: Measuring tail dependence using multivariate regression quantiles. J Econometrics 187: 169-188. doi: 10.1016/j.jeconom.2015.02.004
![]() |
[57] |
Gong X, Wen F, Xia XH, et al. (2017) Investigating the risk-return trade-off for crude oil futures using high-frequency data. Appl Energy 196: 152-161. doi: 10.1016/j.apenergy.2016.11.112
![]() |
[58] | Chen SL, Liu SM, Cai RJ, et al. (2019) The factors that influence exchange-rate risk: Evidence in China, Emerg Mark Financ Tr. |
[59] |
Dong H, Liu Y, Chang JQ (2019) The heterogeneous linkage of economic policy uncertainty and oil return risks. Green Financ 1: 46-66. doi: 10.3934/GF.2019.1.46
![]() |
[60] |
Liao GK, Li ZH, Du ZQ, et al. (2019) The heterogeneous interconnections between supply or demand side and oil risks. Energies 12: 2226. doi: 10.3390/en12112226
![]() |
[61] |
An Y, Sun M, Gao CX, et al. (2018) Analysis of the impact of crude oil price fluctuations on China's stock market in different periods-based on time series network model. Physica A 492: 1016-1031. doi: 10.1016/j.physa.2017.11.032
![]() |
[62] |
Arouri MEH, Jouini J, Nguyen DK (2012) On the impacts of oil price fluctuations on European equity markets: Volatility spillover and hedging effectiveness. Energy Econ 34: 611-617. doi: 10.1016/j.eneco.2011.08.009
![]() |
[63] |
Zhang YL, Wang J (2019) Linkage influence of energy market on financial market by multiscale complexity synchronization. Physica A 516: 254-266. doi: 10.1016/j.physa.2018.10.038
![]() |
[64] |
Guo J (2018) Co-movement of international copper prices, China's economic activity, and stock returns: Structural breaks and volatility dynamics. Glob Financ J 36: 62-77. doi: 10.1016/j.gfj.2018.01.001
![]() |
[65] |
Zheng X, Chen BM (2014) Identification of stock market forces in the system adaptation framework. Inform Sci 265: 105-122. doi: 10.1016/j.ins.2013.12.028
![]() |
[66] |
Zhang C, Chen X (2014) The impact of global oil price shocks on China's bulk commodity markets and fundamental industries. Energy Policy 66: 32-41. doi: 10.1016/j.enpol.2013.09.067
![]() |
[67] |
Burdekin RCK, Siklos PL (2018) Quantifying the impact of the November 2014 Shanghai-Hong Kong stock connect. Int Rev Econ Financ 57: 156-163. doi: 10.1016/j.iref.2018.01.001
![]() |
[68] |
Ruan Q, Bao J, Zhang M, et al. (2019) The effects of exchange rate regime reform on RMB markets: A new perspective based on MF-DCCA. Physica A 522: 122-134. doi: 10.1016/j.physa.2019.01.110
![]() |
[69] |
Ahmad W, Rais S, Shaik AR (2018) Modelling the directional spillovers from DJIM Index to conventional benchmarks: Different this time? Q Rev Econ Financ 67: 14-27. doi: 10.1016/j.qref.2017.04.012
![]() |
[70] |
Antonakakis N, Kizys R (2015) Dynamic spillovers between commodity and currency markets. Int Rev Financ Anal 41: 303-319. doi: 10.1016/j.irfa.2015.01.016
![]() |
[71] |
Gillaizeau M, Jayasekera R, Maaitah A, et al. (2019) Giver and the receiver: Understanding spillover effects and predictive power in cross-market Bitcoin prices. Int Rev Financ Anal 63: 86-104. doi: 10.1016/j.irfa.2019.03.005
![]() |
1. | Cheng-Jun 成俊 Xie 解, Xiang-Qing 向清 Lu 卢, Finite time hybrid synchronization of heterogeneous duplex complex networks via time-varying intermittent control, 2025, 34, 1674-1056, 040601, 10.1088/1674-1056/adacc6 | |
2. | Cheng-jun Xie, Ying Zhang, Qiang Wei, Finite time internal and external synchronisation control for multi-layer complex networks with multiple time-varying delays via rapid response intermittent control, 2025, 0020-7179, 1, 10.1080/00207179.2025.2503307 |