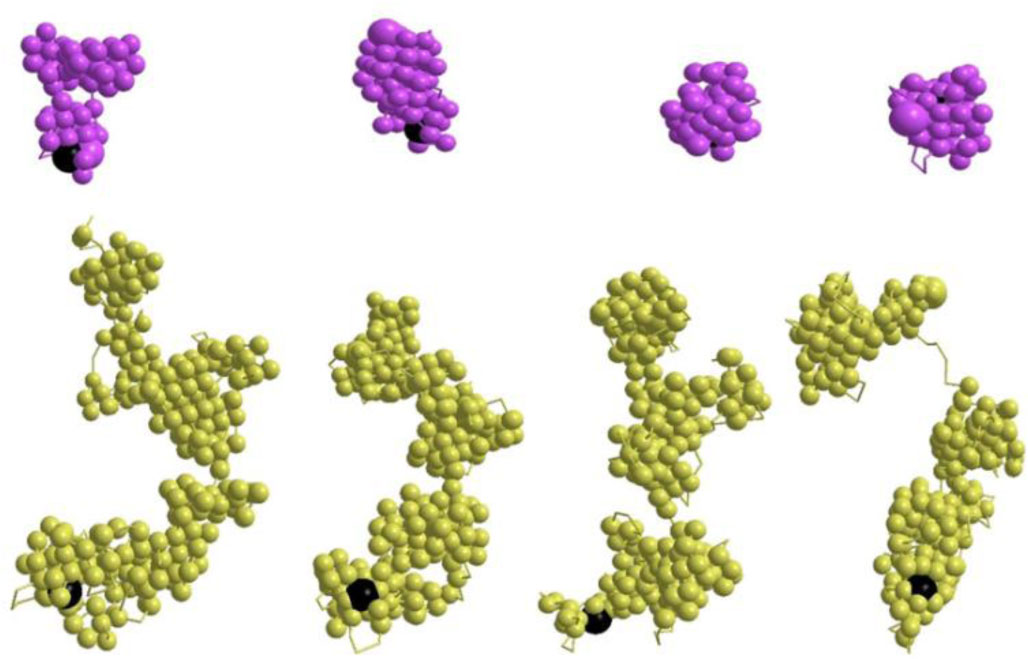
Citation: Li Bin, Muhammad Shahzad, Qi Bing, Muhammad Ahsan, Muhammad U Shoukat, Hafiz MA Khan, Nabeel AM Fahal. The probabilistic load flow analysis by considering uncertainty with correlated loads and photovoltaic generation using Copula theory[J]. AIMS Energy, 2018, 6(3): 414-435. doi: 10.3934/energy.2018.3.414
[1] | Panisak Boonamnaj, Pornthep Sompornpisut, Piyarat Nimmanpipug, R.B. Pandey . Thermal denaturation of a coronavirus envelope (CoVE) protein by a coarse-grained Monte Carlo simulation. AIMS Biophysics, 2022, 9(4): 330-340. doi: 10.3934/biophy.2022027 |
[2] | Warin Rangubpit, Pornthep Sompornpisut, R.B. Pandey . Thermal-induced unfolding-refolding of a nucleocapsid COVN protein. AIMS Biophysics, 2021, 8(1): 103-110. doi: 10.3934/biophy.2021007 |
[3] | Ken Takahashi, Takayuki Oda, Keiji Naruse . Coarse-grained molecular dynamics simulations of biomolecules. AIMS Biophysics, 2014, 1(1): 1-15. doi: 10.3934/biophy.2014.1.1 |
[4] | James C. L. Chow . Recent progress in Monte Carlo simulation on gold nanoparticle radiosensitization. AIMS Biophysics, 2018, 5(4): 231-244. doi: 10.3934/biophy.2018.4.231 |
[5] | Tomohiro Yanao, Sosuke Sano, Kenichi Yoshikawa . Chiral selection in wrapping, crossover, and braiding of DNA mediated by asymmetric bend-writhe elasticity. AIMS Biophysics, 2015, 2(4): 666-694. doi: 10.3934/biophy.2015.4.666 |
[6] | Masoud Jabbary, Hossein Rajabi . High-resolution phantom of a nephron for radiation nephrotoxicity evaluation in biophysical simulations. AIMS Biophysics, 2022, 9(2): 147-160. doi: 10.3934/biophy.2022013 |
[7] | Oleksii V. Khorolskyi, Nikolay P. Malomuzh . Macromolecular sizes of serum albumins in its aqueous solutions. AIMS Biophysics, 2020, 7(4): 219-235. doi: 10.3934/biophy.2020017 |
[8] | Davide Sala, Andrea Giachetti, Antonio Rosato . Molecular dynamics simulations of metalloproteins: A folding study of rubredoxin from Pyrococcus furiosus. AIMS Biophysics, 2018, 5(1): 77-96. doi: 10.3934/biophy.2018.1.77 |
[9] | Wei Zhang, Sheng Cao, Jessica L. Martin, Joachim D. Mueller, Louis M. Mansky . Morphology and ultrastructure of retrovirus particles. AIMS Biophysics, 2015, 2(3): 343-369. doi: 10.3934/biophy.2015.3.343 |
[10] | Stephanie H. DeLuca, Samuel L. DeLuca, Andrew Leaver-Fay, Jens Meiler . RosettaTMH: a method for membrane protein structure elucidation combining EPR distance restraints with assembly of transmembrane helices. AIMS Biophysics, 2016, 3(1): 1-26. doi: 10.3934/biophy.2016.1.1 |
Self-assembly of proteins [1]–[8] provides mechanical strength and stable dynamical response to the underlying environment such as membrane via its hierarchical morphology. It can provide a reliable and responsive pathways in ion channels [9]–[23] for selective transport of such specific elements as potassium ions. There are many examples of protein self-assembly with conflicting (adverse and cooperative) effects [3],[4]. For example, the self-assembly of proteins into a viral capsids is critical in preserving the genome from non-conducing external factors such as digesting enzymes of the host cells, undesirable pH, temperature, etc. [3]. On the other hand, the self-assembly of proteins triggered by the conformational changes of the proteins may lead to undesirable agammaegation such as amyloid beta-proteins into fibrils. Of a diverse range of proteins with unique and universal response properties, we consider a transmembrane protein CorA [9]–[22] with a well-defined inner (iCorA) and outer (oCorA) segments [23]. Very recently, we have observed an unusual thermal response of CorA particularly of its inner segment (iCorA) in its native phase [24]. For example, we find that the size of iCorA reduces on heating while that of the oCorA shows less organized response in its response of its overall size in its native phase as illustrated in Figure 1.
In denatured phase, iCorA expands continuously and oCorA shows abrupt increase in its size in a narrow range of temperature [23],[24]. Because of a relatively large size of oCorA in comparison to iCorA, the snapshots of the entire protein (CorA) is not as clear to identify such trends which is dominated by the overall response of oCorA. The functional structure of CorA is, however, known to exist as a homo-pentamer [19] to provide coordinated open and close states for the selective transport of Mg2+ across the ion channels. Transport of ions depends on the permeation pathways and consequently on the conformation of individual proteins and their interacting network. How CorA proteins assemble collectively into a well-defined morphology is not well understood.
Despite numerous experimental investigations, understanding of the underlying pathways remain highly speculative. Computer simulations provide a viable tool to probe the structural response of proteins and its assembly that may help clarifying such speculative hypothesis. Extensive computer simulations have been recently performed to understand the structure of CorA protein embedded in a model membrane by all-atom MD simulations [19]. Such atomic-scale investigations are insightful in probing the small-scale structural response, however, it is limited to short time scale despite large-scale computer simulations; it is not feasible to examine large-scale structural responses by such approach without severe constraints. For example, the morphology of a set of five proteins with inner segment confined by a nanodisc seems to deform out if they start from a symmetric configuration (see Figure 2). Even with a large-scale all-atom MD simulation, it is rather difficult to see a significant dissociation besides fusion of the symmetry. Therefore, a large-scale coarse-grained analysis [23],[24] is needed to augment and clarify the distinctions (see below) if feasible. Perhaps protein-protein interaction may not be enough to direct five CorA proteins into a stable pentamer; other factors such as a nanodisc or membrane matrix may be needed for the stability. Before incorporating additional constitutive components to probe directed assembly, it would be important to understand the self-assembly of the proteins first.
The protein channel involves cooperative response of many proteins along with numerous constitutive elements of a cellular environment such as lipid molecules, ions, osmolytes, different types of proteins, etc. Although it is not possible to incorporate all constitutive elements in such a computer simulation at once but it may be feasible to investigate effect of each component first before incorporating many. Effect of crowded environment on protein folding and its structural stability has been a subject of intense studies both experimentally [25]–[27] as well as by computer simulations [28]–[32]; these citations are some as examples as there are too many articles published on this subject to cite all. In this article, we focus on the effect of crowded environment created by presence of proteins itself in order to investigate their collective structures. Our goal is to understand the stability of the multi-scale morphologies of interacting proteins in its native and denatured phases. The model is described in the next section, followed by results and discussion with a concluding remark at the end.
We consider a coarse-grained model [24] of the protein chain on a cubic lattice for simplicity, efficiency, and practical utility: it incorporates specificity of each residue, ample degrees of freedom (unlike minimalist lattice models) for residues to perform their stochastic movements and their covalent bonds to fluctuate. The internal structures of residue (i.e., fine-grained details at atomistic scale) are ignored, however, the specificity of residues is considered via their unique residue-residue interactions (see below) which is critical in capturing the unique characteristics of the protein. In our coarse-grained representation, CorA is a chain of 351 nodes (residues) tethered together on a cubic lattice by flexible peptide bonds. A node represents a residue and occupies a unit cell (of size (2a)3, with lattice constant (a); the bond length between consecutive residues varies between 2 and √(10) in unit of lattice constant (a) [33]. Typically, a protein chain is placed in the simulation box in a random configuration, initially with minimum bond length (2a) between the consecutive nodes; this initial configuration is further randomized by allowing each node to perform its stochastic movement (to its 26 neighboring cells with varying distance) with the strictly implemented excluded volume constraint. The bond-fluctuating mechanism has been used extensively in addressing a range of complex problems in polymers [33]; we have extended its utility to model protein chains [23],[24]. Coarse-graining of the simulation box (cubic lattice) and the residues representation by a lattice cell make it one of the efficient computational method for modeling such systems while retaining the potential for fine-graining [34] to further enhance the degrees of freedom.
Each residue interacts with the neighboring residues within a range (rc) of interaction with a generalized Lennard-Jones potential,
Structural evolution of protein chains (CorA) are analyzed in detail as they interact, associate, and dissociate in their native (relatively low temperature) to denatured (high temperature) phases. The sample is prepared by inserting protein chains randomly first in the simulation box; protein chains are moved around with excluded volume constraints to randomize their conformation and distribution further. The protein chain CorA consists of 351 residue, inserting many chains, each of length (Lc = 351) in a simulation box of size L3 (= 3503) become difficult beyond a certain number (Nc = 5 − 100) of chains due to onset of jamming. The computer processing unit (CPU) also increases with the number of chains. Therefore, the number of protein chains is restricted to perform computer simulations with reasonable CPUs (order of days to week) while capturing the cooperative and competing effects of residue-residue, segmental, and overall protein-protein interactions on the local and global association and dissociation of protein chains. Further, relaxation time to reach steady-state (by examining the temporal evolution of various physical quantities) also increases which makes it difficult to assess the thermal response of physical quantities in equilibrium. The protein concentration is defined by the fraction p of the lattice sites occupied by the residues of the protein chains, i.e., p = 8 × Nc × Lc/L3. The number of protein chains Nc = 5 − 100 each with length Lc = 351 (protein chain of CorA) and Lc = 61 (inner segment of protein) are considered with simulation box of size L = 350, and 150 respectively. Note that the simulation box becomes crowded at a lower protein concentrations with longer chain lengths.
Each residue performs its stochastic movements with the Metropolis algorithm which involves selecting a residue randomly at a site say i of a randomly selected protein chain and attempting to move it to one of its neighboring site say j. If the excluded volume constraints and limits on the bond length for the proposed move are satisfied then we calculate the corresponding change in energy ΔEij = Ej − Ei between its old (Ei) and new (Ej) configurations, and move the node with the Boltzmann probability exp(− ΔEij/T) where T is the temperature in reduced unit of the Boltzmann constant (kB). Attempts to move each node once defines the unit Monte Carlo step (MCS), i.e., Nc × Lc attempts to move randomly selected nodes in a simulation box with Nc protein chains each with Lc residues defines the unit MCS. Simulations were performed for sufficiently long time steps (typically 10 million time steps) to make sure that system has reached its steady state equilibrium with a number of independent samples (10–100) to estimate the average values of the physical quantities. A number of local and global physical quantities were determined such as the energy of each residue and protein chain, contact map, their mobility, mean square displacement of the center of mass of the protein, radius of gyration, and its structure factor. These physical quantities are in arbitrary units, i.e., the length is in units of the lattice constant which is different from many all-atom simulations where realistic units for size and time scales are used via calibration of σ and εij from experimental data for simplified systems. It is difficult to quantify physical quantities in absolute units due to the phenomenological nature of the interactions (Eq 1). The trend in response of the physical quantities to such parameters as the temperature and network density should however be qualitatively comparable with appropriate observations.
We study the effects of protein-protein interaction on a number of local and global physical quantities as proteins organize, associate and disassociate as a function of temperature and their concentration (i.e., the number of proteins). Our system has reached the steady-state equilibrium during the course of simulations (i.e., in 107 time steps) for almost entire temperature regime and concentrations except in extreme limits (i.e., high temperature and higher concentrations) where the relaxation time is too large due to entanglement and jamming of extended structures of proteins. Nevertheless, it is illustrative to understand the organizing morphologies as the protein chains assemble, entangle, and disassociate.
Representative snapshots of CorA and that of its inner segments (iCorA) with 100 protein chains each in the simulation box is presented in Figure 3 at a low (T = 0.020) and a high (T = 0.040) temperature. Both, the protein chains and its inner segments appear to disperse almost uniformly. A closer inspection shows that, in native (low temperature) phase, CorA proteins dissociate more on the scale comparable to the size of a protein chain in general while the clusters of inner segments appear to form isolated globular bundles. The residue-residue interaction is more conducive to agglomeration of chain with the inner segments than that of the full protein (CorA). Corresponding snapshots at a higher temperature (T = 0.040) show different morphologies where some degree of phase-separation seem to occur with CorA as the proteins continue to dissociate, expand, and entangle. The spreading of extended inner segments at T = 0.040 reduces the segregation of protein clusters seen at the low temperature. Attempts are made to quantify our qualitative observations of complex morphologies by analyzing the structure factor (see below).
Contact maps (Figures S1–S4) of the assembly of CorA chains and inner segments at a low temperature (T = 0.020) seem to suggest a more compact morphology of iCorA in crowded protein environment than that of CorA. At high temperature (T = 0.040), frequency of segmental contacts is much lower in their crowded protein matrices than that at the low temperature; segmental contacts in chains with inner segment is still higher than that with CorA. The average number (Nn) of residues around each is a measure of the contact profile which is presented in Figures 4 and 5 for a range of temperatures (T = 0.020−0.040). Obviously, the residue contact density decreases on increasing the temperature with more contacts at low temperatures (native phase) and less in denatured phase. Variation in pattern of the contact profiles with the temperature however, reveals how the protein segments organize during the self-assembly that leads to a global morphology. At the low temperature (T = 0.020), most part of the protein chains seem to be surrounded by proteins leading to a compact (globular) morphology. Onset of preferential contacts emerges on raising the temperature (T = 0.025). For example, second half of residues in chains with only inner segment (iCorA) of the protein (residues 316F − 351L) have higher contact density than the first half (residues 291M − 315N) (Figure 5). Preferential contact becomes more localized (316F − 325W) on increasing the temperature further (T = 0.030). Even though the contact density of many residues is relatively high in self-assembled morphology in CorA, its random distribution along the backbone makes less defined at higher temperatures (Figure 4). Further, the fraction of segments with relatively low contact density remains appreciably high. Thus, the protein-protein interaction in inner segments is likely to provide a more stable support for a collective morphology of CorA protein.
How does the size of protein chains depend on the protein concentration in native and denatured phases? How are residues distributed as the protein chains associate and dissociate? We address it by analyzing the radius of gyration of each protein and structure factor of the self-organized assembly. Variations of the average radius of gyration of the protein CorA with the concentration is presented in figure 6 at representative low and high temperatures. The radius of gyration of the protein does not show much variation with the protein concentration at low temperatures (inset Figure 6). At the high temperature (T = 0.040) the radius of gyration grows continuously with the concentration reaching a steady-state value in the crowded regime. It should be pointed out that the entanglement is enhanced at higher protein concentrations. We think that a large increase of the radius of gyration is caused by the competition between entanglement and the thermal expansion of chains.
Figure 7 shows that the radius of gyration of chains with only inner segments increases with the protein concentration p systematically at low temperatures (T = 0.015, 0.020) in its native phase and exhibits little variation with p at higher temperatures (T ≥ 0.025) in its denatured phase. We therefore think that the protein-protein interaction among the inner-segments of the protein in its native phase may be more responsive to self-organized collective morphology of proteins.
Overall distribution of residues over multiple length scales can be assessed by analyzing the structure factor S(q) which is defined as,
Variation of the structure factor (S(q)) with the wave length (λ) of the self-assembly of the protein (CorA) is presented in Figure 8 at temperature T = 0.030 for a wide range of protein concentrations, dilute (Nc = 5) to crowded (Nc = 100) regime. Note that the radius of gyration has reached a steady-state value for all concentrations of the protein at T = 0.030. We see that D∼2.6 for each protein (λ∼Rg), D∼2.2 at Rg ≤ λ ≤ 50 (in unit of lattice constant). For all residues distributed over the entire simulation box, λ∼L, the effective dimension decreases systematically from D∼2 with Nc = 100 to D∼1 with Nc = 5 where each protein chain appears isolated. At high temperature (T = 0.040), it is difficult to reach steady state during the course of simulations for the radius of gyration of CorA proteins at higher protein concentrations due to entanglements (Figure S5). Variation of the structure factor with the wave vector (Figure S5) reveals a rather tenuous morphology (λ from size of a residue to about third of the simulation box, L/3 much larger than Rg of protein chains) of the entangled fiberous protein chains at the high temperature with an effective dimension D∼1.7.
The structure factor of residues' distribution in a dilute-to-crowded protein environment (Nc = 5 − 100) of chains with inner segments is presented in Figure 9 at a high temperature T = 0.040. Each protein chain appear to maintain globular conformation (D ≥ 3), at λ ≤ Rg; on larger scale λ > Rg, the density varies with length scale, i.e., D∼2.6 and 3.4 with increasing length scale up to about half the size of the simulation box (λ = L/2). The overall morphology (λ∼L), seem to become more tenuous on reducing the number of protein chains; D∼1.6 with Nc = 100. Note that the radius of gyration of the protein increases on increasing the number Nc of protein chains (inset Figure 9).
The variation of the structure factor with the wavelength in the most crowded environment (Nc = 100) is presented in Figure 10 for a range of temperatures. The globular morphology (D ≥ 3) of individual protein chain seems to persist at lower temperatures (T = 0.015, 0.025) at length scale comparable to its size (λ∼Rg). On a larger-scale (Rg < λ < 3 Rg), the assembled structure is not as compact (D∼2.5), but the overall assembly remains compact (solid with D∼3) for its spread over the simulation box at the low temperature (T = 0.015). The multi-scale morphology of self-organizing proteins is heterogenious regardless of its globular conformations in its native phase. The morphology remains relatively dense D∼2.5 even at a much higher temperature, i.e., T = 0.030. On large length scale, (λ∼L), the self-assembled morphology appears tenuous. The morpholgy adopts to a random coil D∼1.8 at high temperature T = 0.040 where onset of a regular long range structure appear to sets in (with an oscillation in S(q)).
Self-organized structures of interacting proteins (CorA) and its inner segments are investigated by a coarse-grained Monte Carlo simulation as a function of protein concentration at a range of low to high temperatures. Visual inspection show clear distinctions in morphology of the assembly in dilute solution and that in the crowded (dense) matrix at both low and high temperatures. CorA proteins seem to dissociate more on the scale comparable to the size of the proteins while the clusters of chains with inner segments phase-separate in its native phase (low temperatures). It appears that the protein-protein segmental interaction is more conducive to agglomeration of inner segments than that of the outer segments of the protein.
Variation in pattern of the contact profiles with the temperature reveals how the protein segments organize during the self-assembly that leads to a global morphology. We find that a relatively lower fraction of residues in outer segments (residues 1M-290V) of the protein participate in segmental globularization (with a random distribution) in comparison to that of the inner core segment (291M… 351L) with well-defined globular region. The radius of gyration of the protein does not vary much with the protein concentration at low temperatures. However, it shows enormous growth as the entanglement increases with the concentration at high temperature. In contrast, the radius of gyration of chains with inner segments increases with the protein concentration p systematically at low temperatures (T = 0.015, 0.020) in its native phase. We therefore think that the protein-protein interaction among the inner-segments of the protein in its native phase is conducive to responsiveness of the self-organizing pathways.
The multi-scale morphology (isolated globular bundles to entangled network) is quantified by evaluating an effective dimension D from the scaling analysis of the structure factor with the wave vector. In general, the effective dimension of the assembled morphology of protein chains is much lower than that of its inner segments. For example, the most compact morphology of the proteins with D∼2.6 (λ∼Rg) even at the highest protein concentrations (most crowded) appears at low temperature while it remains almost extended in a random configuration with D ≤ 2 at almost entire temperature and concentration range considered here. In contrast, the self-assembled morphology of chains with inner segments remains globular D∼3 at almost all length scales in its native phase. It is difficult to identify main cause of a stable pentamer configuration due to complexity of the crowded proteins resulting from inter- and intra-chain residue-residue interactions, competition between the self-organizing mechanism and steric constraints, and entanglement. Based on our analysis presented here, we believe that a stronger protein-protein interactions among the inner segments of the protein is conducing to its collective stable self-assembly.
[1] |
Bechrakis DA, Sparis PD (2004) Correlation of wind speed between neighboring measuring stations. IEEE T Energy Conver 19: 400–406. doi: 10.1109/TEC.2004.827040
![]() |
[2] | Villanueva D, Feijóo A, Pazos JL (2010) Correlation between power generated by wind turbines from different locations. Available from: http://proceedings.ewea.org/ewec2010/allfiles2/20_EWEC2010presentation.pdf. |
[3] | Jong MD, Papaefthymiou G, Palensky P (2017) A framework for incorporation of infeed uncertainty in power system risk-based security assessment. IEEE T Power Syst 33: 613–621. |
[4] |
Kirschen D, Jayaweera D (2007) Comparison of risk-based and deterministic security assessments. IET Gener Transm Dis 1: 527–533. doi: 10.1049/iet-gtd:20060368
![]() |
[5] | Zhang P, Lee ST (2004) Probabilistic load flow computation using the method of combined cumulants and Gram-Charlier expansion. IEEE T Power Syst 19: 676–682. |
[6] |
Nijhuis M, Gibescu M, Cobben S (2017) Gaussian mixture based probabilistic load flow for LV-network planning. IEEE T Power Syst 32: 2878–2886. doi: 10.1109/TPWRS.2016.2628959
![]() |
[7] |
Valverde G, Saric A, Terzija V (2012) Probabilistic load flow with non-Gaussian correlated random variables using Gaussian mixture models. IET Gener Transm Dis 6: 701–709. doi: 10.1049/iet-gtd.2011.0545
![]() |
[8] |
Prusty BR, Jena D (2016) Combined cumulant and Gaussian mixture approximation for correlated probabilistic load flow studies: A new approach. Csee J Power Energ Syst 2: 71–78. doi: 10.17775/CSEEJPES.2016.00024
![]() |
[9] |
Caramia P, Carpinelli G, Varilone P (2010) Point estimate schemes for probabilistic three-phase load flow. Electr Pow Syst Res 80: 168–175. doi: 10.1016/j.epsr.2009.08.020
![]() |
[10] |
Morales JM, Perez-Ruiz J (2007) Point estimate schemes to solve the probabilistic power flow. IEEE T Power Syst 22: 1594–1601. doi: 10.1109/TPWRS.2007.907515
![]() |
[11] | Kloubert ML, Rehtanz C (2017) Enhancement to the combination of point estimate method and Gram-Charlier Expansion method for probabilistic load flow computations. PowerTech, IEEE Manchester 2017: 1–6. |
[12] | Wang Y, Zhang N, Chen Q, et al. (2016) Dependent discrete convolution based probabilistic load flow for the active distribution system. IEEE T Sustain Energ 8: 1000–1009. |
[13] |
Zhang N, Kang C, Singh C, et al. (2016) Copula based dependent discrete convolution for power system uncertainty analysis. IEEE T Power Syst 31: 5204–5205. doi: 10.1109/TPWRS.2016.2521328
![]() |
[14] | Cai D, Chen J, Shi D, et al. (2012) Enhancements to the cumulant method for probabilistic load flow studies. Pow Energ Soc Gen Meeting 59: 1–8. |
[15] |
Aien M, Fotuhi-Firuzabad M, Aminifar F (2012) Probabilistic load flow in correlated uncertain environment using unscented transformation. IEEE T Power Syst 27: 2233–2241. doi: 10.1109/TPWRS.2012.2191804
![]() |
[16] |
Fang S, Cheng H, Xu G (2016) A modified Nataf transformation-based extended quasi-monte carlo simulation method for solving probabilistic load flow. Electr Mach Pow Syst 44: 1735–1744. doi: 10.1080/15325008.2016.1173130
![]() |
[17] |
Ren Z, Koh CS (2013) A second-order design sensitivity-assisted Monte Carlo simulation method for reliability evaluation of the electromagnetic devices. J Electr Eng Technol 8: 780–786. doi: 10.5370/JEET.2013.8.4.780
![]() |
[18] | Tang L, Wen F, Salam MA, et al. (2015) Transmission system planning considering integration of renewable energy resources. Pow Energ Eng Conf 2015: 1–5. |
[19] | Ayodele TR (2016) Analysis of monte carlo simulation sampling techniques on small signal stability of wind generator-connected power system. J Eng Sci Technol 11: 563–583. |
[20] |
Prusty BR, Jena D (2017) A critical review on probabilistic load flow studies in uncertainty constrained power systems with photovoltaic generation and a new approach. Renew Sust Energ Rev 69: 1286–1302. doi: 10.1016/j.rser.2016.12.044
![]() |
[21] |
Morales JM, Baringo L, Conejo AJ, et al. (2010) Probabilistic power flow with correlated wind sources. Let Gener Transm Dis 4: 641–651. doi: 10.1049/iet-gtd.2009.0639
![]() |
[22] |
Usaola J (2010) Probabilistic load flow with correlated wind power injections. Electr Pow Syst Res 80: 528–536. doi: 10.1016/j.epsr.2009.10.023
![]() |
[23] |
Gupta N (2016) Probabilistic load flow with detailed wind generator models considering correlated wind generation and correlated loads. Renew Energ 94: 96–105. doi: 10.1016/j.renene.2016.03.030
![]() |
[24] |
Prusty BR, Jena D (2017) A sensitivity matrix-based temperature-augmented probabilistic load flow study. IEEE T Ind Appl 53: 2506–2516. doi: 10.1109/TIA.2017.2660462
![]() |
[25] | Kenari MT, Sepasian MS, Nazar MS, et al. (2017) The combined cumulants and Laplace transform method for probabilistic load flow analysis. Let Gener Transm Dis. |
[26] |
Wu W, Wang K, Li G, et al. (2016) Probabilistic load flow calculation using cumulants and multiple integrals. Let Gener Transm Dis 10: 1703–1709. doi: 10.1049/iet-gtd.2015.1129
![]() |
[27] | Nelsen RB (2007) An introduction to copulas. Springer Science & Business Media. |
[28] | Market Analysis and Information System (MAISY). Utility Customer Energy Use & Hourly Load Databases. Available from: http://www.maisy.com/. |
[29] | National Renewable Energy Laboratory (NREL). Obtain Solar Power data: Western State California. Available from: https://www.nrel.gov/grid/solar-power-data.html. |
[30] | Macdonald IA (2009) Comparison of sampling techniques on the performance of Monte-Carlo based sensitivity analysis. Eleventh International IBPSA Conference, 992–999. |
[31] |
Yu H, Chung CY, Wong KP, et al. (2009) Probabilistic load flow evaluation with hybrid latin hypercube sampling and cholesky decomposition. IEEE T Power Syst 24: 661–667. doi: 10.1109/TPWRS.2009.2016589
![]() |
[32] | University of Washington Electrical Engineering: Power System Test Case Archive (January 2018). Available from: http://www.ee.washington.edu/research/pstca. |
[33] |
Karaki S, Chedid R, Ramadan R (1999) Probabilistic performance assessment of autonomous solar-wind energy conversion systems. IEEE T Energy Conver 14: 766–772. doi: 10.1109/60.790949
![]() |
[34] | Reddy JB, Reddy DN (2004) Probabilistic performance assessment of a roof top wind, solar photo voltaic hybrid energy system. Rel Maint S-RAMS 2004: 654–658. |
1. | Warin Rangubpit, Sunan Kitjaruwankul, Pornthep Sompornpisut, R.B. Pandey, Pinning the conformation of a protein (CorA) in a solute matrix with selective binding, 2020, 556, 03784371, 124823, 10.1016/j.physa.2020.124823 | |
2. | Pornthep Sompornpisut, R. B. Pandey, Self-Organized Morphology and Multiscale Structures of CoVE Proteins, 2021, 73, 1047-4838, 2347, 10.1007/s11837-021-04711-0 |