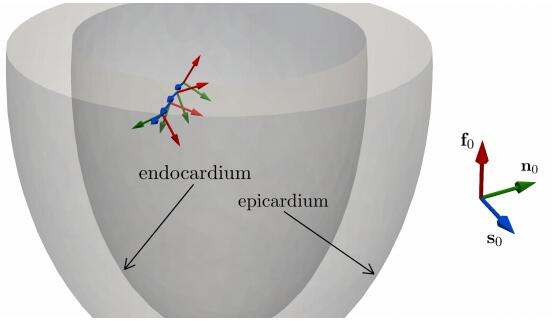
Citation: Antonello Gerbi, Luca Dedè, Alfio Quarteroni. A monolithic algorithm for the simulation of cardiac electromechanics in the human left ventricle[J]. Mathematics in Engineering, 2019, 1(1): 1-37. doi: 10.3934/Mine.2018.1.1
[1] | Luca Azzolin, Luca Dedè, Antonello Gerbi, Alfio Quarteroni . Effect of fibre orientation and bulk modulus on the electromechanical modelling of human ventricles. Mathematics in Engineering, 2020, 2(4): 614-638. doi: 10.3934/mine.2020028 |
[2] | Dmitrii Rachinskii . Bifurcation of relative periodic solutions in symmetric systems with hysteretic constitutive relations. Mathematics in Engineering, 2025, 7(2): 61-95. doi: 10.3934/mine.2025004 |
[3] | Luca Formaggia, Alessio Fumagalli, Anna Scotti . A multi-layer reactive transport model for fractured porous media. Mathematics in Engineering, 2022, 4(1): 1-32. doi: 10.3934/mine.2022008 |
[4] | Juan Pablo Borthagaray, Wenbo Li, Ricardo H. Nochetto . Finite element algorithms for nonlocal minimal graphs. Mathematics in Engineering, 2022, 4(2): 1-29. doi: 10.3934/mine.2022016 |
[5] | Ludovica Cicci, Stefania Fresca, Stefano Pagani, Andrea Manzoni, Alfio Quarteroni . Projection-based reduced order models for parameterized nonlinear time-dependent problems arising in cardiac mechanics. Mathematics in Engineering, 2023, 5(2): 1-38. doi: 10.3934/mine.2023026 |
[6] | Ivan Fumagalli . Discontinuous Galerkin method for a three-dimensional coupled fluid-poroelastic model with applications to brain fluid mechanics. Mathematics in Engineering, 2025, 7(2): 130-161. doi: 10.3934/mine.2025006 |
[7] | Daniele Cerroni, Florin Adrian Radu, Paolo Zunino . Numerical solvers for a poromechanic problem with a moving boundary. Mathematics in Engineering, 2019, 1(4): 824-848. doi: 10.3934/mine.2019.4.824 |
[8] | Gabriella Bretti, Emiliano Cristiani, Corrado Lattanzio, Amelio Maurizi, Benedetto Piccoli . Two algorithms for a fully coupled and consistently macroscopic PDE-ODE system modeling a moving bottleneck on a road. Mathematics in Engineering, 2019, 1(1): 55-83. doi: 10.3934/Mine.2018.1.55 |
[9] | Francesca Bonizzoni, Davide Pradovera, Michele Ruggeri . Rational-approximation-based model order reduction of Helmholtz frequency response problems with adaptive finite element snapshots. Mathematics in Engineering, 2023, 5(4): 1-38. doi: 10.3934/mine.2023074 |
[10] | Tommaso Tassi, Alberto Zingaro, Luca Dede' . A machine learning approach to enhance the SUPG stabilization method for advection-dominated differential problems. Mathematics in Engineering, 2023, 5(2): 1-26. doi: 10.3934/mine.2023032 |
The heart plays the crucial role of pumping blood into the circulatory system by providing all sort of vital substances to the cells. Moreover, cardiovascular related diseases represent the leading causes of death in the whole world [60]. While advancements in medical practice are continuously improving patients conditions and diseases outcomes, recent progresses in mathematical modeling and computational mechanics allow to perform realistic cardiovascular numerical simulations [38,65,76,77,84,97,105,107], thus potentially providing medical doctors and clinicians with valuable diagnostic and predictive tools. Moreover, clinical data and the application of image segmentation techniques to Magnetic Resonance Imaging (MRI) and Computed Tomography (CT) scans feed, as inputs, the mathematical models, thus allowing numerical simulations in subject- and patient-specific frameworks [23,55]; in addition, uncertainty quantification techniques allow to estimate the models parameters and data, and to cope with their variability [30,59].
The mathematical modeling of the heart involves several challenges intrinsically related to the complexity of its function [17,20,33]. A satisfactory model must be able to describe a wide range of different processes, such as the evolution of the transmembrane potential in the myocardium, the deformation caused by the muscles contraction, and the dynamics of the blood in the heart chambers and through the valves [31,71,76,77,96,98,99]. All these processes feature different temporal and spatial scales, yielding the so-called tyranny of scales [8]. A satisfactory knowledge of the models for isolated processes - the electrophysiology, the active and the passive mechanics - which we refer to as "single core models", is nowadays quite established; however, further theoretical and numerical studies are still necessary to better understand their mutual interactions [18,22,30,82]. In this work, we use state-of-the-art models in passive myocardial tissue modeling – the Holzapfel-Ogden model [46] – together with the active strain formulation [2,3] in combination with a recently proposed model for the transmurally heterogeneous thickening of the myocardium [7]; the latter is for the first time used in this paper for the integrated electromechanics modeling of the left ventricle (LV). The link between electrophysiology and mechanics is finally established through a model describing the shortening of the myocardial fibers [88], which is in turn triggered by a change in the ionic concentrations in the cardiac cells.
From the numerical viewpoint, we discretize in space the continuous single core models by means of the Finite Element Method (FEM) with both linear and quadratic elements, while the time discretization is carried on using Backward Differentiation Formulas (BDFs) of orders 1 and 2 [78]. BDFs are used both within implicit and semi-implicit schemes, the latter consisting in equal-order extrapolation of the unknowns in the nonlinear terms[16,37]; however, the semi-implicit scheme poses strong limitations on the choice of the timestep size with respect to the implicit scheme, even if the latter yields a nonlinear problem, which we approximate by means of the Newton method. Integrating the discrete single core models leads to the formulation of a monolithic algebraic coupled problem. Numerical coupling of cardiac electromechanics is however typically performed by means of segregated or staggered approaches, for which the electrophysiology and mechanics solvers are not applied simultaneously [4,5,18,38,54,84,107]. It is however known that partitioned schemes (segregated or staggered) do not generally guarantee unconditional stability [15], an issue not concerning instead the monolithic scheme.
Monolithic schemes for cardiac electromechanics of the heart were firstly proposed and analyzed in [24,25], where only the contraction phase was simulated and the blood pressure at the endocardium (the LV inner wall) was neglected. More recently, a monolithic scheme including a 0D model for the vascular system, heart valve, and atrial chamber model was proposed in [44]. However, other than being less explored, monolithic schemes have been used so far only for cardiac electromechanics within the framework of the active stress formulation and only for idealized or simplified LV geometries. Instead, in this paper we propose and successfully use a monolithic algorithm for the numerical simulation of cardiac electromechanics in the framework of the active strain formulation; in addition, we simulate full cardiac cycles (one heartbeat) for both idealized and patient-specific geometries. Essential components of our framework are: modeling FSI at the LV endocardium wall by means of
We solve the large, sparse block linear system stemming from the monolithic strategy by means of the GMRES method and, in order to speed-up the convergence of the linear solver, we employ a novel (right) preconditioner [89]. Our preconditioning strategy extends the one proposed in [27] for FSI problems and is based on the factorization of a block Gauss-Seidel preconditioner which exposes the multiphysics nature of the integrated problem, thus allowing to adapt its action on each single factor of the preconditioner. This strategy can be easily extended to a wide range of integrated multiphysics problems: the factors are indeed independently preconditioned by using algebraic multigrid [11] or domain decomposition [14,104] preconditioners tailored by exploiting physics-specific information (such as the number of equations) for each block; this local information would be instead lost by using a global monolithic preconditioner.
We use our monolithic electromechanics solver to perform numerical simulations in the High Performance Computing framework of the whole cardiac cycle, both for idealized and patient-specific LV geometries, and we analyze the numerical results in terms of clinically relevant indicators; specifically, we produce the so-called pressure-volume loops in order to assess the LV function and its properties.
The paper is organized as follows: in Sec. 2 we introduce the mathematical models for the electrophysiology, the mechanics and the activation of the myocardium; we then integrate them thus obtaining a continuous integrated model. In Sec. 3 we carry on the space and time numerical approximations of the single core models; in Sec. 4 we introduce the preconditioner used to solve the monolithic linear system arising after the time and space discretizations. In Sec. 5 we report and discuss the numerical results obtained with the proposed methods, and we finally draw our conclusions in Sec. 6.
The myocardium is a complex tissue composed of cardiomyocytes, organized in fibers and laminar collagen sheets [94], characterising the LV orthotropic internal structure. Therefore, the material properties of the LV are strongly dependent on the direction of fibers and sheets. First, the electrical conductivity of the cardiomyocytes is much larger in the longitudinal direction than transversally [52,75]; hence, at the macroscopic level, the transmembrane electric potential travels faster along the fibers. Secondly, it has been experimentally observed in [46] that the response of internal stresses to an external load significantly varies when measured among different directions since the myocardium is stiffer along the fibers. Finally, the contraction of the LV is made possible thanks to an active force acting on the cardiomyocytes lined up along the fibers direction [86]. In order to mathematically define fibers and sheets, we identify a local frame of reference by defining the mutually orthonormal vector fields
Electrophysiology models take into account the electrochemical reactions occuring in the myocardium [21] triggered by an electric impulse originated at the sino-atrial node and then conveyed through the Purkinje fibers [20]. Such signal causes a quick depolarization of the LV cardiomyocytes, meaning that the transmembrane potential (i.e. the difference in electric potential between the interior and the exterior of a cell) changes sign in few microseconds. The potential drives a change in the concentration of different ionic species flowing through the so-called ionic gates located on the cellular membrane; the concentration of these ionic species, in turn, influences the potential thus slowly repolarizing the cells. The continuous interaction between the ions concentration and the potential causes a cascade effect for which a fast traveling wave known as action potential propagates in the whole myocardium [57].
In this work, we consider the monodomain equation for the description of the evolution of the cellular transmembrane potential
{χ(Cm∂V∂t+Iion(V,w))=∇⋅(JF−1DmF−T∇V)+Iapp(t)in Ω0×(0,T),(JF−1DmF−T∇V)⋅N=0on ∂Ω0×(0,T),V=V0in Ω0×{0}. | (2.1) |
Here,
{dwidt=αi(V)(w∞i(V)−wi)+βi(V)wiin (0,T),wi(0)=wi,0,at t=0,for i=1,…,NI, | (2.2) |
where the unknowns
{∂V∂t+∑q∈{fi,so,si}Iq(V,w)=∇⋅(JF−1DmF−T∇V)+Iapp(t)in Ω0×(0,T),(JF−1DmF−T∇V)⋅N=0on ∂Ω0×(0,T),∂w∂t=α(V)(w∞(V)−w)+β(V)win Ω0×(0,T),V=V0,w=(1,1,0)Tin Ω0×{0}, | (2.3) |
since
α(V)=diag(1−HV1(V)τ−1(V),1−HV2(V)τ−2(V),1τ3(V)),β(V)=diag(−HV1(V)τ+1,−HV2(V)τ+2,0),w∞(V)=(1−HV−1(V),HVo(V)(w∞∗−1+Vτ∞2)+1−Vτ∞2,Hk3V3(V))T,Ifi(V,w1)=−HV1(V)(V−V1)(ˆV−V)τfiw1,Iso(V)=(1−HV2(V))(V−Vo)τo(V)+HV2(V)τso(V), |
and
An adequate mechanical model for the description of the myocardium's displacement must account for the tissue's complex behavior. Firstly, the internal stresses induced by a prescribed deformation are highly anisotropic [41] and in our formulation depend on the directions
We recall the momentum conservation equation in the reference configuration
{ρ∂2d∂t2−∇⋅P(d,γf)=0in Ω0×(0,T),q(d,∂d∂t)+P(d,γf)N=0on Γη0×(0,T),P(d,γf)N=pendo(t)Non Γendo0×(0,T),d=d0,∂d∂t=˙d0in Ω0×{0}. | (2.4) |
Here
P(d)=∂W(C)∂F. |
A very popular model for the myocardial tissue is the Holzapfel-Ogden [46] one. This is obtained by considering different contributions and by taking into account the anisotropic nature of the muscle:
˜W(C)=W1(I1)+W4f(I4f)+W4s(I4s)+W8fs(I8fs)=a2beb(I1−3)+∑i∈{f,s}ai2bi[eb⟨I4i−1⟩2−1]+afs2bfs[ebI28fs−1], |
where
The mechanical model should also account for the volumetric change to which the myocardium undergoes during the cardiac cycle. It has been observed in [19,113] that albeit this change is moderate, still it significantly ranges from 2% to 15%. For these reasons we use a nearly-incompressible formulation, which allows for small volume variations [28]. We multiplicatively decompose the deformation gradient
F=Fv¯F,Fv=J13I, | (2.5) |
where
Wvol(J)=B2(J−1)log(J), | (2.6) |
such that the larger is the bulk modulus
W=˜W+Wvol=W1(J−23I1)+W4f(I4f)+W4s(I4s)+W8fs(I8fs)+Wvol(J). |
We now proceed by modeling the active behavior of the myocardium driven by the stretching of the fibers by means of the active strain approach [2,3,63,86]. A virtual intermediate state
P=det(FA)PEF−TA,with PE=∂W(CE,J)∂FE. |
In Sec. 2.3, we will provide the explicit form of the tensor
P(d,FA)=aeb(J−23IE1−3)J−23(FEF−1A−IE13(FEFA)−T)+2afebf⟨IE4f−1⟩2⟨IE4f−1⟩(fE⊗fA)+2asebs⟨IE4s−1⟩2⟨IE4s−1⟩(sE⊗sA)+afsebfs(IE8fs)2IE8fs(fE⊗sA+sE⊗fA)+B2(J+Jlog(J)−1)(FEFA)−T, | (2.7) |
where
The activation model of the myocardium represents the link between the electrophysiology and the tissue (passive) mechanics. Instead of cellular models describing the complex dynamic taking place inside the sarcomeres [81], we exploit a phenomenological model for the local shortening of the fibers
{g(c)∂γf∂t−εΔγf=Φ(c,γf,d)in Ω0×(0,T),∇γf⋅N=0on ∂Ω0×(0,T),γf=0in Ω0×{0}. | (2.8) |
Here,
The contraction of the tissue is triggered by the calcium concentration exceeding a threshold value
Being
FA=I+γff0⊗f0+γss0⊗s0+γnn0⊗n0, |
which is symmetric and we set
γn=k′(λ)(1√1+γf−1). |
Indeed, the latter depends on an independent variable
k′(λ)=¯k′(¯kendoλ−λepiλendo−λepi+¯kepiλ−λendoλepi−λendo). | (2.9) |
Finally, in order to have
Writing together Eqs.(2.3), (2.4), and (2.8), the coupled electromechanics problem finally reads:
{∂V∂t+∑q∈{fi,so,si}Iq(V,w)=∇⋅(JF−1DmF−T∇V)−Iapp(t)in Ω0×(0,T),∂w∂t=α(V)(w∞(V)−w)+β(V)win Ω0×(0,T),ρ∂2d∂t2−∇0⋅P(d,γf)=0in Ω0×(0,T),g(w3)∂γf∂t−εΔγf=Φ(w3,γf,d)in Ω0×(0,T),(JF−1DmF−T∇V)⋅N=0on ∂Ω0×(0,T),q(d,∂d∂t)+P(d)N=0on Γη0×(0,T),P(d)N=pendo(t)Non Γendo0×(0,T),∇γf⋅N=0on ∂Ω0×(0,T),V=V0,w=(1,1,0)T,γf=0in Ω0×{0},d=d0,∂d∂t=˙d0in Ω0×{0}. | (2.10) |
We remark that at this stage the pressure
A common problem which has to be considered in biological modeling involving a fluid-structure interface is that the reference geometry
Pressure preload ([30,84,101,103]): the reference geometry
Pressure prestress ([47,100]): we compute internal stresses distribution such that the reference geometry is in equilibrium with the blood pressure
We adopt the pressure prestress approach since in the preload one the procedure returns a configuration which might be significantly different with respect to the initial geometry as shown in [47]. Indeed, we already know the loaded configuration from medical images. In order to compute
{∇0⋅P(d)=−∇0⋅P0in Ω0,(N⊗N)Kη⊥d+(I−N⊗N)Kη∥d+P(d)N=0on Γη0,P(d)N=pendo(0)Non Γendo0. | (2.11) |
Eq. (2.11) is the steady, passive version of problem (2.4) with the additive decomposition of the strain tensor. Moreover, we set:
pk=kS¯pendo,k=1,…,S, |
where
Geometry | Mesh | # Vertices | # Elements | |
Idealized(Fig. 6) | (a) | 4.6 mm | 1'827 | 3'010 |
(b) | 2.3 mm | 11'658 | 12'040 | |
(c) | 1.2 mm | 81'335 | 416'000 | |
Patient-specific(Fig. 7) | (d) | 0.8 mm | 126'031 | 637'379 |
In this section we describe the numerical approximation of the electromechanics problem (2.10). The FEM [78] is used for the space discretization of the PDEs, while BDF [78] are used for the time discretization.
We consider a mesh composed of tetrahedrons
*The MRI images are provided by Prof. J. Schwitter (Chief physician at the Centre Hospitalier Universitaire Vaudois CHUV, Lausanne) and Dr. P. Masci in the framework of the collaboration CMCS@EPFL-CHUV hospital.
We use the FEM for the spatial approximation of the monodomain equation (2.1). We first introduce the finite dimensional space
∫Ω0˙VhψidΩ0+∫Ω0(JF−1hDmF−Th∇Vh)⋅∇ψidΩ0+∑q∈{fi,so,si}∫Ω0Iq(Vh,wh)ψidΩ0=∫Ω0Iapp(t)ψidΩ0,∀i=1,…,NdofV, | (3.1) |
with
{M˙Vh(t)+K(dh(t))Vh(t)+Iion(Vh(t),wh(t))=Iapp(t)t∈(0,T],Vh(0)=V0,h, | (3.2) |
where
(Iion(Vh,wh))i=∑q∈{fi,so,si}∫Ω0Iq(Vh,wh)ψidΩ0,(Iapp(t))i=∫Ω0Iapp(t)ψidΩ0. | (3.3) |
We will discuss in Sec. 3.1.3 two different strategies for the approximation of the nonlinear term
The ionic model (2.2) is a system of ODEs that indirectly depends on the space variable through the transmembrane potential
wh(t)={wlh(t)}NIl=1andwlh(t)={wlj(t)}Ndofwj=1. | (3.4) |
The problem thus obtained reads: given
˙wlj+(αl(Vj)−βl(Vj))wlj=αl(Vj)w∞l(Vj), | (3.5) |
with
{˙wh(t)+U(Vh(t))wh(t)=Q(Vh(t)),t∈(0,T],wh(0)=w0,h, |
where the block matrix
As anticipated in Sec. 3.1.1, different choices can be made for the approximation of the term
State Variable Interpolation (SVI): the variables
∫Ω0Iq(Vh,wh)ψidΩ0=∑K∈Th(Nq∑s=1Iq(NdofV∑j=1Vj(t)ψj(xKs),NdofV∑j=1wj(t)ψj(xKs))ψi(xKs)ωKs). |
This approach corresponds to the standard FEM approximation.
Ionic Currents Interpolation (ICI): for each element,
∫Ω0Iq(Vh,wh)ψidΩ0≈∑K∈Th(Nq∑s=1NdofV∑j=1Iq(Vj(t),wj(t))ψj(xKs)ψi(xKs)ωKs). |
The two approaches are equivalent if
As previously done for the monodomain equation, we use the FEM to approximate the momentum equation (2.4). We denote by
\begin{gather*} \int_{\Omega_0} \rho_s \frac{\partial^2 {\bf d}_h}{\partial t^2} \cdot \mathit{\boldsymbol{\psi}}_i \, d\Omega_0 + \int_{\Omega_0} {\bf P}({\bf d}_h, \gamma_{f, h}) : \nabla_0 \mathit{\boldsymbol{\psi}}_i \, d\Omega_0 + \sum\limits_{\substack{\eta \in \{epi, base\}} } \int_{\Gamma_0^\eta} {\bf q}\left({\bf d}_h, \frac{\partial {\bf d_h}}{\partial t} \right) \cdot \mathit{\boldsymbol{\psi}}_i \, d\Gamma_0\\[0.1cm] \qquad\qquad\qquad\qquad = \int_{\Gamma_0^{endo}} p^{endo}(t){\bf N} \cdot \mathit{\boldsymbol{\psi}}_i \, d\Gamma_0, \qquad \forall i = 1, \dots, N^{dof}_{{\bf d}}, \end{gather*} |
with
We rewrite it in algebraic form as:
\begin{equation*} \left\{ \begin{aligned} &\rho_s \mathbb{M} \ddot{{\bf d}}_h(t) + \mathbb{F} \dot{{\bf d}}_h(t) + \mathbb{G} {\bf d}_h(t)+ {\bf S}({\bf d}_h(t), \mathit{\boldsymbol{\gamma}}_{f, h}(t)) = {\bf p}^{endo}(t) \quad t \in (0, T], \\ &{\bf d}_h(0) = {\bf d}_{0, h}, \quad \dot{{\bf d}}_h(0) = \dot{{\bf d}}_{0, h},&& \end{aligned} \right. \end{equation*} |
where
Finally, we once again use FEM to discretize in space the equation for the unknown
\begin{gather*} \int_{\Omega_0} \frac{\partial \gamma_{f, h}}{\partial t} \, \psi_i \, d\Omega_0 + \varepsilon \int_{\Omega_0} \frac{1}{g(c_h)} \nabla \gamma_{f, h} \cdot \nabla \psi_i \, d\Omega_0 \\ - \int_{\Omega_0} \frac{1}{g(c_h)} \Phi(c_h, \gamma_{f, h}, {\bf d}_h) \, \psi_i \, d\Omega_0 = 0, \qquad \forall i = 1, \dots, N^{dof}_{\gamma_f}, \end{gather*} |
with
\begin{equation*} \left\{ \begin{aligned} &\mathbb{M} \dot{\mathit{\boldsymbol{\gamma}}}_{f, h}(t) + \varepsilon \mathbb{K}( c(t) ) \mathit{\boldsymbol{\gamma}}_{f, h}(t) + \mathit{\boldsymbol{ \boldsymbol{\varPhi}}}(c(t), \mathit{\boldsymbol{\gamma}}_{f, h}(t), {\bf d}_h(t)) = {\bf 0} \quad t \in (0, T], \\[0.1cm] &\mathit{\boldsymbol{\gamma}}_{f, h}(0) = {\bf 0}.&& \end{aligned} \right. \end{equation*} |
After having applied the space discretization to the single core models, we obtain the semi-discretized formulation of problem (2.10) in the form of a nonlinear system of ODEs. We denote by
\begin{equation} \label{eq:semidisc} \left\{ \begin{aligned} &\mathcal{M} {\bf z}(t) + {\bf T}({\bf z}(t)) = {\bf h}(t) \qquad t \in (0, T], \\ &{\bf z}(0) = {\bf z}_0, \\ &\dot{{\bf z}}_{{\bf d}}(0) = \dot{{\bf d}}_{0, h}, \end{aligned} \right. \end{equation} | (3.6) |
where
\begin{gather*} \dot{z}_i(t^{n+1}) \approx \frac{1}{\Delta t} \left( \vartheta^{(Ⅰ)}_0 z_i^{n+1} - z_i^{RHS} \right), \qquad z_i^{RHS} = \sum\limits_{k = 1}^\sigma \vartheta^{(Ⅰ)}_k z_i^{n-k+1}, \\ \ddot{z}_i(t^{n+1}) \approx \frac{1}{(\Delta t)^2} \left( \vartheta^{(Ⅱ)}_0 z_i^{n+1} - z_i^{RHS} \right), \qquad z_i^{RHS} = \sum\limits_{k = 1}^{\sigma+1} \vartheta^{(Ⅱ)}_k z_i^{n-k+1}, \end{gather*} |
where
In the implicit case, we obtain the following nonlinear system:
\begin{equation} \label{eq:implicit_algebraic} {\bf A}({\bf z}^{n+1}) = {\bf b}^{n+1} \qquad n = \sigma, \dots, N_T-1, \end{equation} | (3.7) |
with
In the semi-implicit case, on the other hand, we extrapolate the variables in the nonlinear term
\begin{equation*} z_i(t^{n+1}) \approx z_i^* = \sum\limits_{k = 1}^{\sigma} \beta_k z_i^{n-k+1}. \end{equation*} |
We then avoid the nonlinear terms by partially evaluating
\begin{equation*} {\bf A}({\bf z}^{n+1}) \approx \mathbb{A}({\bf z}^*) {\bf z}^{n+1}+{\bf g}^{n+1} \qquad \text{for } n = \sigma, \dots, N_T-1. \end{equation*} |
By recalling Eq.(3.7), we hence obtain a system in the form:
\begin{equation} \label{eq:semi_implicit_algebraic} \mathbb{A}({\bf z}^*){\bf z}^{n+1} = \widetilde{{\bf b}}^{n+1} \qquad n = \sigma, \dots, N_T-1, \end{equation} | (3.8) |
with
By applying the Newton method [78] to (3.7), we iteratively solve for
\begin{equation} \label{eq:newton_iteration} \left\{ \begin{aligned} & \mathbb{J}^{n+1}_k \delta {\bf z}^{n+1}_{k+1} = - {\bf r}^{n+1}_k, \\ & {\bf z}^{n+1}_{k+1} = {\bf z}^{n+1}_k + \delta {\bf z}^{n+1}_{k+1}, \end{aligned} \right. \end{equation} | (3.9) |
for
\begin{equation*} \mathbb{J}_k^{n+1} = \left[ \begin{array}{c|c|c|c} \mathbb{J}_{{\bf w}}({\bf V}_k^{n+1})& \mathbb{J}_{{\bf w}{\bf V}}({\bf w}_k^{n+1}, {\bf V}_k^{n+1}) &0&0 \\ \hline \mathbb{J}_{{\bf V}{\bf w}}({\bf w}_k^{n+1}, {\bf V}_k^{n+1}) &\mathbb{J}_{{\bf V}}({\bf d}_k^{n+1})& 0&\mathbb{J}_{{\bf V}{\bf d}}({\bf V}_k^{n+1}, {\bf d}_k^{n+1}) \\ \hline \mathbb{J}_{\mathit{\boldsymbol{\gamma}}_f {\bf w}}({\bf w}_k^{n+1}, {\mathit{\boldsymbol{\gamma}}_f}_k^{n+1}, {\bf d}_k^{n+1})&0 & \mathbb{J}_{\mathit{\boldsymbol{\gamma}}_f}({\bf w}_k^{n+1}, {\mathit{\boldsymbol{\gamma}}_f}_k^{n+1}, {\bf d}_k^{n+1}) & \mathbb{J}_{\mathit{\boldsymbol{\gamma}}_f {\bf d}}({\bf w}_k^{n+1}, {\mathit{\boldsymbol{\gamma}}_f}_k^{n+1}, {\bf d}_k^{n+1})\\ \hline 0&0 &\mathbb{J}_{{\bf d}\mathit{\boldsymbol{\gamma}}_f}( {\mathit{\boldsymbol{\gamma}}_f}_k^{n+1}, {\bf d}_k^{n+1})& \mathbb{J}_{{\bf d}}({\mathit{\boldsymbol{\gamma}}_f}_k^{n+1}, {\bf d}_k^{n+1}) \end{array} \right], \end{equation*} |
while the residual is defined as
In this case, the block structure of the matrix associated to linear system (3.8) to be solved is sparser with respect to the implicit one, as
\begin{equation} \label{eq:semi_implicit_pattern} \mathbb{A}({\bf z}^*) = \left[ \begin{array}{c|c|c|c} \mathbb{A}_{{\bf w}}({\bf V}^*)& 0&0&0 \\ \hline \mathbb{A}_{{\bf V}{\bf w}}({\bf w}^*, {\bf V}^*) &\mathbb{A}_{{\bf V}}({\bf d}^*§)& 0&0 \\ \hline 0&0 & \mathbb{A}_{\mathit{\boldsymbol{\gamma}}_f}({\bf w}^*, {\mathit{\boldsymbol{\gamma}}_f}^*, {\bf d}^*)&0 \\ \hline 0&0&0& \mathbb{A}_{{\bf d}}({\mathit{\boldsymbol{\gamma}}_f}^*, {\bf d}^*) \end{array} \right]. \end{equation} | (3.10) |
The matrix (3.10) is block lower triangular thanks to the ordering of the variables in the electrophysiology model (3.6): indeed, by swapping the ionic and the electric blocks we would have obtained a block upper triangular matrix. The semi-implicit scheme has the clear advantage of avoiding, at each timestep, the solution of a nonlinear problem. Moreover, both the assembly of the system matrix
On the other hand, semi-implicit schemes may impose strong limitations on the timestep size
We will further investigate this issue in the future; however, for the next numerical simulations of this paper we will consider only the implicit scheme.
We rewrite, for the sake of simplicity, the linear system associated to a single Newton iteration for the implicit scheme (3.9) in the following form:
\begin{equation} \label{linear_problem} \mathbb{J} \, \delta {\bf z} = -{\bf r}, \qquad \text{with } \mathbb{J} = \left[ \begin{array}{c|c|c|c} J_{11}& J_{12}&0&0 \\ \hline J_{21} & J_{22}&0 & J_{24} \\ \hline J_{31}&0 & J_{33} & J_{34} \\ \hline 0&0 & J_{43}& J_{44} \end{array} \right]. \end{equation} | (4.1) |
We use the GMRES method [89] for the solution of the linear problem (4.1) as
The choice of the preconditioner is critical in order to ensure the convergence of the linear solver; while this is true in general, it is even more relevant in the case of monolithic multiphysics problems [51]. Indeed, using a black-box algebraic preconditioner for problem (4.1) the information related to each differential problem associated to a single core model would be neglected; we instead consider a strategy exploiting such information at the block level, that is we create a preconditioner that exploits the "physics" of the coupled problem at the level of the block structure. Following [26,27,35] for FSI problems, we propose a block Gauss-Seidel preconditioner
\begin{equation*} \mathcal{P} = \left[ \begin{array}{c|c|c|c} J_{11}&0&0&0 \\ \hline J_{21} & J_{22}&0&0 \\ \hline J_{31}&0 & J_{33}&0 \\ \hline J&0 & J_{43}&J_{44} \end{array} \right]. \end{equation*} |
\begin{equation*} \mathcal{P} = \underbrace{ \left[ \begin{array}{c|c|c|c} J_{11}&0&0&0 \\ \hline 0&I&0&0 \\ \hline 0&0&I&0 \\ \hline 0&0&0&I \end{array} \right]}_{\mathcal{P}_1 = \mathcal{P}_{ion}} \underbrace{ \left[ \begin{array}{c|c|c|c} I&0&0&0 \\ \hline J_{21} & J_{22}&0&0 \\ \hline 0&0&I&0 \\ \hline 0&0&0&I \end{array} \right]}_{\mathcal{P}_2 = \mathcal{P}_{pot}} \underbrace{ \left[ \begin{array}{c|c|c|c} I&0&0&0 \\ \hline 0&I&0&0 \\ \hline J_{31}&0 & J_{33}&0 \\ \hline 0&0&0&I \end{array} \right]}_{\mathcal{P}_3 = \mathcal{P}_{act}} \underbrace{ \left[ \begin{array}{c|c|c|c} I&0&0&0 \\ \hline 0&I&0&0 \\ \hline 0&0&I&0 \\ \hline 0&0&J_{43}& J_{44} \end{array} \right].}_{\mathcal{P}_4 = \mathcal{P}_{mec}} \end{equation*} |
This decomposition can also be interpreted as an inexact factorization of matrix
\begin{equation} \label{eq:precond_linear_problem} \left\{ \begin{aligned} &\mathbb{J} \mathcal{P}^{-1}{\bf y} = -{\bf r} \\ &\mathcal{P} \delta {\bf z} = {\bf y}. \end{aligned} \right. \end{equation} | (4.2) |
Since each diagonal block
\begin{equation} \label{precond_application} \left\{ \begin{aligned} &\delta {\bf z}_{{\bf w}} && = J_{11}^{-1} {\bf y}_{{\bf w}}, \\ &\delta {\bf z}_V && = J_{22}^{-1} ({\bf y}_V - J_{21} \delta {\bf z}_{{\bf w}} ), \\ &\delta {\bf z}_{{\bf d}} && = J_{33}^{-1} ({\bf y}_{{\bf d}} - J_{31} \delta {\bf z}_{{\bf w}} ), \\ &\delta {\bf z}_{\mathit{\boldsymbol{\gamma}}_f} && = J_{44}^{-1} ({\bf y}_{\mathit{\boldsymbol{\gamma}}_f} - J_{43} \delta {\bf z}_{{\bf d}} ); \end{aligned} \right. \end{equation} | (4.3) |
the solution of each linear system in (4.3) is carried out by using again the GMRES method and by exploiting Algebraic Multigrid (AMG) preconditioners [11]. This new preconditioner
For our simulations we will consider a full heartbeat, by taking the conventional duration of
(1) an isovolumic contraction phase in which the endocardial pressure
(2) an ejection phase characterized by a decrement in the ventricular volume
(3) an isovolumic relaxation phase during which
(4) a filling phase starting with the opening of the mitral valve causing an increment of
When necessary, we compute the volume
We then model the endocardial pressure
1) Isovolumic phase I: At each timestep, we iteratively solve problem (2.10) by updating at each subiteration the pressure in the following manner:
\begin{equation} \label{eq:isochoric} p^{n+1}_{k+1} = p^{n+1}_k+\frac{V^{n+1}_k-V^n}{C_p} \qquad k = 0, \dots \end{equation} | (4.4) |
with
2) Ejection phase: A two-element Windkessel model [110] of the form:
\begin{equation} \label{eq:windkessel} \left\{ \begin{aligned} &C\frac{dp}{dt} = -\frac{p}{R}-\frac{dV}{dt} \quad t \in (T', T"] \\[0.1cm] &p(T') = \overline{p} \end{aligned} \right. \end{equation} | (4.5) |
is solved in the pressure variable with a BDF scheme of order
3) Isovolumic phase II: Modeled as the first isovolumic phase. This phase ends when the pressure reaches the minimal value of
4) Filling phase: The pressure is linearly increased at each timestep so that it reaches the EDP value at the final time
Even if (4.5) is strongly coupled with (2.10) during the ejection phase, for simplicity we solve the two problems in a segregated fashion.
We now describe the geometries used for the numerical simulation of the integrated electromechanics problem. As anticipated in Sec. 3, we use both an idealized prolate geometry and a patient-specific geometry segmented from MRI images as detailed in Sec. 5.1. Moreover, in Sec. 5.2, we outline the procedure that is exploited to define the fibers and the sheets fields on the different meshes. We conclude this section by showing and analyzing the results of the simulations.
Image segmentation is the process of locating regions of interest (ROI) in the form of a subset of pixels [42]. In biomedical applications, this accounts to assign different flags to regions containing different types of tissues and/or fluids. This result, depending on the properties of the biological material which is to be segmented, can often be achieved through semi-automatic or automatic procedures (see e.g. [32,49,106] for arteries and blood vessels and [10,109] for the Purkinje network), exploiting a large set of different techniques. However, since the development of algorithms for the segmentation of the chambers of the heart [72,114,115] is beyond the scope of this work, we used a manual procedure based on the brightness of the pixels of the aforementioned 3D MRI images. We first apply a threshold filter to select the smallest set of pixels containing the whole myocardium; then, we manually remove artifacts and features that we decide to neglect such as the papillary muscles and the upper part of the LV. Finally, a Gaussian smoothing procedure [111] was performed to improve the quality of the mesh. See Figure 5.
The image was taken at the end of the diastolic phase, that is when the LV reaches its maximum enlargement. The internal volume of the patient-specific myocardium at this stage of the heartbeat measures approximately 95 ml.
Unlike the geometry, the fibers and sheets field distribution in the myocardium may not be extracted from medical images such as MRIs unless special procedures are applied [80]. For this reason, several mathematically rule-based definition of the fields have been used in literature [38,56,61,86], which try to approximate their orientation. At the epicardium and at the endocardium, the fiber direction is tangential to the boundary, while the sheet direction belongs to the plane identified by the normal and the ventricle centerline. Then, in the most general case, angles
We setup a total of five numerical simulations, each for a single heartbeat, using the four meshes shown in Figures 6-7. The monodomain, the activation, and the mechanics equations are approximated with
Geometry | Mesh | # Vertices | # Elements | |
Idealized(Fig. 6) | (a) | 4.6 mm | 1'827 | 3'010 |
(b) | 2.3 mm | 11'658 | 12'040 | |
(c) | 1.2 mm | 81'335 | 416'000 | |
Patient-specific(Fig. 7) | (d) | 0.8 mm | 126'031 | 637'379 |
Geometry | Mesh | # CPUs | ||
Idealized | (a) | 1 | 14'616 | 1 |
(b) | 1 | 93'264 | 6 | |
(c) | 1 | 650'680 | 45 | |
(a) | 2 | 93'264 | 6 | |
Patient-specific | (d) | 1 | 1'008'248 | 72 |
We use LifeV†, an open-source finite element library for the solution of problems described by PDEs in a High Performance Computing framework, to implement the numerical methods. All the computations were carried out using Piz Daint, a Cray XC50/XC40 supercomputer installed at the Swiss National Supercomputing Center (CSCS)‡.
As detailed in Sec. 4 we exploit the Newton method for the solution of the nonlinear system (3.7) by setting a maximum number of 5 iterations per timestep. Moreover we use the relative residual as stopping criterion, with a tolerance equal to
For simplicity, we initiate the electric signal propagation by applying at the same time 2-milliseconds long stimuli at three distinct points on the endocardium; we remark that, even if this pacing strategy is not fully realistic since the electrical signal delivered by the Purkinje network activates asynchronously hundreds of cells [83,108], it provides physically meaningful results. The other parameters used for the simulations are reported in Table 3.
| | | | | | | | | | | | | |
| | | | | | | | | | | 12 | 500 | |
| | | | | | | | | | | | | |
50 | 10 | 1500 | 0 | | 5 | 1 | 0 | 0 | | | | |
We compare in Figure 8 the transmembrane potential obtained using
We observe from Figure 9 a difference of several milliseconds in the complete activation of the myocardium, which is significant in the electrophysiology characteristic time scales. However, because of the multiscale nature of the integrated problem, the mechanics is not affected by such delay as can be observed in Figure 11. Here, the only remarkable difference between the three results is the underestimation of the displacement magnitude in the simulation with the coarsest mesh (a). In this case, the number of degrees of freedom is not sufficient to either fullfill the nearly-incompressible constraint nor to represent the large deformations observable in the other two cases. The deformation of the patient-specific mesh (d) at the same times is shown in Figure 12.
In both the idealized and the patient-specific cases, a significant thickening of the myocardium walls takes place, which is in accordance with experimental observations [79]. The model, with the set of parameters in Table 3, produces however a significant rotation of the LV: a recent work [74] suggests that this behavior is related to the choice of the incompressibility constraint, the bulk modulus
In order to better evaluate the ability to describe the LV activity of the integrated numerical model for a full cardiac cycle, pressure-volume (pV) loops are also represented. A comparison with in-vivo measurements would be pointless in the case of the ideal geometry, however we stress the fact that the pV-loops reported in Figure 14 are in both qualitative and quantitative agreement with those observed experimentally as e.g. in [87]; moreover, the maximal endocardial pressure, which is reached during the ejection phase, is approximately 110 mmHg and hence lays well within the physiological range of average individuals [91]. The difference between the four cases in Figure 14 accounts to a maximum variation of about 2 % in the minimal ventricle volume during the second isochoric phase.
The pV-loop for the patient-specific case is reported in Figure 15. Experimental measurements of the pressure and of the volume for the patient under study were not available, therefore we did not carry on a quantitative comparison of our results against patient-specific data. However, we once again observe that the values of the pV-loop are in line with real data [87].
We then report in Table 4, the timestep used for each simulation, the average number of Newton iterations, the average number of fixed point iterations for each of the two isochoric phases, and the average time spent for different operations during a single timestep. The overall time required for the complete simulations is also reported. Table 4 clearly shows that finer meshes require larger computational resources for a full heartbeat simulation (
Geometry | Mesh | |||||||||
Idealized | (a) | 1 | 3.02 | 2.31 | 2.55 | 2.15 | 0.023 | 0.006 | 5h 41m | |
(b) | 1 | 3.37 | 2.14 | 3.44 | 6.67 | 0.163 | 0.019 | 18h 21m | ||
(c) | 1 | 4.23 | 2.49 | 3.26 | 9.19 | 0.632 | 0.040 | 46h 42m | ||
(a) | 2 | 3.29 | 1.68 | 2.11 | 6.47 | 0.178 | 0.025 | 30h 18m | ||
Patient-specific | (d) | 1 | 4.44 | 1.96 | 4.51 | 22.33 | 1.692 | 0.076 | 68h 37m |
Finally, we test the strong scalability of the proposed monolithic solver for cardiac electromechanics. With this aim, we further refine the ideal mesh of Figure 6, thus obtaining a finer one featuring 4'629'817 vertices and 26'624'000 tetrahedra, which is more adequate for the strong scalability test. We then set
§Performing the strong scalability test with a number of CPUs smaller than 800 was not feasible with this mesh; indeed, memory limitations occurred due the excessive number of unknowns per single CPU.
In this work we proposed a novel monolithic solver for the simulation of the integrated electromechanics problem for the LV of the human heart. We coupled the monodomain equation, the ionic minimal model, the activation model for the fibers contraction, and the myocardial mechanics in the active strain framework with a transmurally variable activation. The interaction of the myocardium with the blood inside the ventricle was taken into account by prestressing and by solving additional 0D problems for the fluid.
We considered both implicit and semi-implicit BDF schemes for our monolithic solver together with a preconditioning strategy based on block Gauss-Seidel preconditioner which exploits the multiphysics structure of the coupled problem. The strong limitations on the timestep size in the semi-implicit case are such that the implicit scheme is by far the most efficient for numerical simulations lasting for one or multiple heartbeats. We solve the coupled problem in the High Performance Computing framework and we performed a scalability analysis for a benchmark test involving more than 37'000'000 unknowns.
The results obtained by simulating a complete heartbeat with both ideal and patient-specific geometries are qualitatively in agreement with physiological values measured in healthy patients. Moreover, the comparison of the simulations on the idealized geometry with different mesh sizes shows that the proposed numerical model is able to capture the physical solution with satisfactory accuracy even in the case of relatively coarse meshes. From our simulations we observe that choosing a mesh with maximum size
This research was partially supported by the Swiss Platform for Advanced Scientific Computing (PASC, project "Integrative HPC Framework for Coupled Cardiac Simulations"). We also gratefully acknowledge the Swiss National Supercomputing Center (CSCS) for providing the CPU resources for the numerical simulations under project ID s635. Finally, we acknowledge the support from the ERC Advanced Grant iHEART, “An Integrated Heart Model for the simulation of the cardiac function”, 2017-2022, P.I.A. Quarteroni (ERC-2016-ADG, project ID: 740132).
We acknowledge Prof. J. Schwitter and Dr. P. Masci (CHUV) for providing the MRI images used in this work and for the constructive discussions on the topic from a physician's perspective. We also thank Prof. P. Tozzi (CHUV) for the invaluable insights in the functioning of the human heart. Finally, we acknowledge both Dr. D. Forti and MER S. Deparis (EPFL) for the useful discussions and their precious help with the LifeV library.
The authors declare no conflict of interest.
[1] |
Aliev RR, Panfilov AV (1996) A simple two-variable model of cardiac excitation. Chaos Soliton Fract 7: 293–301. doi: 10.1016/0960-0779(95)00089-5
![]() |
[2] |
Ambrosi D, Arioli G, Nobile F, et al. (2011) Electromechanical coupling in cardiac dynamics: the active strain approach. SIAM J Appl Math 71: 605–621. doi: 10.1137/100788379
![]() |
[3] | Ambrosi D, Pezzuto S (2012) Active stress vs. active strain in mechanobiology: constitutive issues. J Elasticity 107: 199–212. |
[4] |
Augustin CM, Neic A, Liebmann M, et al. (2016) Anatomically accurate high resolution modeling of human whole heart electromechanics: a strongly scalable algebraic multigrid solver method for nonlinear deformation. J Comput Phys 305: 622–646. doi: 10.1016/j.jcp.2015.10.045
![]() |
[5] |
Baillargeon B, Rebelo N, Fox DD, et al. (2014) The living heart project: a robust and integrative simulator for human heart function. Eur J Mech-A/Solids 48: 38–47. doi: 10.1016/j.euromechsol.2014.04.001
![]() |
[6] | Barbarotta L (2014) A mathematical and numerical study of the left ventricular contraction based on the reconstruction of a patient specific geometry. Master thesis, Politecnico di Milano, Italy. |
[7] | Barbarotta L, Rossi S, Dedè L, et al. (2017) Numerical validation of a transmurally heterogeneous orthotropic activation model for ventricular contraction. MOX report, Politecnico di Milano 62/2017. |
[8] | Batterman R (2013) The tyranny of scales. Oxford Handbook of The Philosophy of Physics, 256–286. |
[9] |
Bendahmane M, Bürger R, Ruiz-Baier R (2010) A finite volume scheme for cardiac propagation in media with isotropic conductivities. Math Comput Simulat 80: 1821–1840. doi: 10.1016/j.matcom.2009.12.010
![]() |
[10] | Bordas R, Grau V, Burton RAB, et al. (2010) Integrated approach for the study of anatomical variability in the cardiac purkinje system: from high resolution MRI to electrophysiology simulation. Annual International Conference of the IEEE Engineering in Medicine and Biology Society 2010: 6793–6796. |
[11] | Briggs WL, Henson VE, McCormick SF (2000) A Multigrid Tutorial. SIAM. |
[12] |
Bueno-Orovio A, Cherry EM, Fenton FH (2008) Minimal model for human ventricular action potentials in tissue. J Theor Biol 253: 544–560. doi: 10.1016/j.jtbi.2008.03.029
![]() |
[13] | Burman E, Ern A (2003) The discrete maximum principle for stabilized finite element methods. In Numerical Mathematics and Advanced Applications, Springer, 557–566. |
[14] |
Cai XC, Sarkis M (1999) A restricted additive Schwarz preconditioner for general sparse linear systems. SIAM J Sci Comput 21: 792–797. doi: 10.1137/S106482759732678X
![]() |
[15] |
Causin P, Gerbeau JF, Nobile F (2005) Added-mass effect in the design of partitioned algorithms for fluid-structure problems. Comput Method Appl M 194: 4506–4527. doi: 10.1016/j.cma.2004.12.005
![]() |
[16] | Cellier FE, Kofman E (2006) Continuous System Simulation. Springer Science & Business Media. |
[17] | Chabiniok R, Wang VY, Hadjicharalambous M, et al. (2016) Multiphysics and multiscale modelling, data-model fusion and integration of organ physiology in the clinic: ventricular cardiac mechanics. Interface Focus 6: 15–83. |
[18] |
Chapelle D, Fernández M, Gerbeau JF, et al. (2009) Numerical simulation of the electromechanical activity of the heart. International Conference on Functional Imaging and Modeling of Heart 5528: 357–365. doi: 10.1007/978-3-642-01932-6_39
![]() |
[19] | Cheng A, Langer F, Rodriguez F, et al. (2005) Transmural cardiac strains in the lateral wall of the ovine left ventricle. Am J Physiol-Heart C 288(4): H1546–H1556. |
[20] | Colli Franzone P, Pavarino LF, Savaré G (2006) Computational electrocardiology: mathematical and numerical modeling. In Complex Systems in Biomedicine, Springer, 187–241. |
[21] | Colli Franzone P, Pavarino LF, Scacchi S (2014) Mathematical Cardiac Electrophysiology, Springer. |
[22] |
Costabal FS, Concha FA, Hurtado DE, et al. (2017) The importance of mechano-electrical feedback and inertia in cardiac electromechanics. Comput Method Appl M 320: 352–368. doi: 10.1016/j.cma.2017.03.015
![]() |
[23] |
Coupé P, Manjón JV, Fonov V, et al. (2011) Patch-based segmentation using expert priors: Application to hippocampus and ventricle segmentation. NeuroImage 54: 940–954. doi: 10.1016/j.neuroimage.2010.09.018
![]() |
[24] |
Dal H, Göktepe S, Kaliske M, et al. (2011) A three-field, bi-domain based approach to the strongly coupled electromechanics of the heart. Proceedings in Applied Mathematics and Mechanics 11: 931–934. doi: 10.1002/pamm.201110442
![]() |
[25] |
Dal H, Göktepe S, Kaliske M, et al. (2013) A fully implicit finite element method for bidomain models of cardiac electromechanics. Comput Method Appl M 253: 323–336. doi: 10.1016/j.cma.2012.07.004
![]() |
[26] |
Deparis S, Forti D, Gervasio P, et al. (2016) INTERNODES: an accurate interpolation-based method for coupling the Galerkin solutions of pdes on subdomains featuring non-conforming interfaces. Comput Fluids 141: 22–41. doi: 10.1016/j.compfluid.2016.03.033
![]() |
[27] |
Deparis S, Forti D, Grandperrin G, et al. (2016) FaCSI: A block parallel preconditioner for fluid-structure interaction in hemodynamics. J Comput Phys 327: 700–718. doi: 10.1016/j.jcp.2016.10.005
![]() |
[28] |
Doll S, Schweizerhof K (2000) On the development of volumetric strain energy functions. J Appl Mech 67: 17–21. doi: 10.1115/1.321146
![]() |
[29] |
Dupraz M, Filippi S, Gizzi A, et al. (2015) Finite element and finite volume-element simulation of pseudo-ECGs and cardiac alternans. Math Meth Appl Sci 38: 1046–1058. doi: 10.1002/mma.3127
![]() |
[30] |
Eriksson TSE, Prassl AJ, Plank G, et al. (2013) Influence of myocardial fiber/sheet orientations on left ventricular mechanical contraction. Math Mech Solids 18: 592–606. doi: 10.1177/1081286513485779
![]() |
[31] | Fedele M (2014) A patient specific aortic valve model based on moving resistive immersed surfaces. Master thesis, Politecnico di Milano, Italy. |
[32] | Fedele M, Faggiano E, Barbarotta L, et al. (2015) Semi-automatic three-dimensional vessel segmentation using a connected component localization of the region-scalable fitting energy. In 2015 9th International Symposium on Image and Signal Processing and Analysis (ISPA), 72–77. |
[33] | Formaggia L, Quarteroni A, Veneziani A (2010) Cardiovascular Mathematics: Modeling and Simulation of the Circulatory System, volume 1. Springer Science & Business Media. |
[34] | Forti D (2016) Parallel algorithms for the solution of large-scale fluid-structure interaction problems in hemodynamics. PhD thesis, EPFL, Switzerland. |
[35] |
Forti D, Bukac M, Quaini A, et al. (2017) A monolithic approach to fluid-composite structure interaction. J Sci Comput 72: 396–421. doi: 10.1007/s10915-017-0363-5
![]() |
[36] | Gee MW, Siefert CM, Hu JJ, et al. (2006) ML 5.0 smoothed aggregation users guide. Technical report, Technical Report SAND2006-2649, Sandia National Laboratories. |
[37] |
Gervasio P, Saleri F, Veneziani A (2006) Algebraic fractional-step schemes with spectral methods for the incompressible Navier-Stokes equations. J Comput Phys 214: 347–365. doi: 10.1016/j.jcp.2005.09.018
![]() |
[38] |
Göktepe S, Kuhl E (2010) Electromechanics of the heart: a unified approach to the strongly coupled excitation-contraction problem. Comput Mech 45: 227–243. doi: 10.1007/s00466-009-0434-z
![]() |
[39] | Gordon AM, Huxley AF, Julian FJ (1966) The variation in isometric tension with sarcomere length in vertebrate muscle fibres. J Phys 184: 170–192. |
[40] | Guccione JM, McCulloch AD (1991) Finite element modeling of ventricular mechanics. In Theory of Heart, Springer, 121–144. |
[41] |
Guccione JM, McCulloch AD, Waldman LK (1991) Passive material properties of intact ventricular myocardium determined from a cylindrical model. J Biomech Eng-Tasme 113: 42–55. doi: 10.1115/1.2894084
![]() |
[42] |
Haralick RM, Shapiro LG (1985) Image segmentation techniques. Comput Vis Graph Imag Proc 29: 100–132. doi: 10.1016/S0734-189X(85)90153-7
![]() |
[43] |
Heroux MA, Bartlett RA, Howle VE, et al. (2005) An overview of the Trilinos project. ACM T Math Software 31: 397–423. doi: 10.1145/1089014.1089021
![]() |
[44] | Hirschvogel M, Bassilious M, Jagschies L, et al. (2017) A monolithic 3D-0D coupled closed-loop model of the heart and the vascular system: experiment-based parameter estimation for patient-specific cardiac mechanics. Int J Numer Meth Bio 33. |
[45] | Hodgkin AL, Huxley AF (1952) A quantitative description of membrane current and its application to conduction and excitation in nerve. J Phys 117: 500–544. |
[46] |
Holzapfel GA, Ogden RW (2009) Constitutive modelling of passive myocardium: a structurally based framework for material characterization. Philos T R Soc A 367: 3445–3475. doi: 10.1098/rsta.2009.0091
![]() |
[47] |
Hsu MC, Bazilevs Y (2011) Blood vessel tissue prestress modeling for vascular fluid-structure interaction simulation. Finite Elem Anal Des 47: 593–599. doi: 10.1016/j.finel.2010.12.015
![]() |
[48] | Hunter PJ, Nash MP, Sands GB (1997) Computational electromechanics of the heart. Comput Bio Heart 12: 347–407. |
[49] |
Isgum I, Staring M, Rutten A, et al. (2009) Multi-atlas-based segmentation with local decision fusion – application to cardiac and aortic segmentation in CT scans. IEEE T Med Imaging 28: 1000–1010. doi: 10.1109/TMI.2008.2011480
![]() |
[50] | Katz AM (2010) Physiology of the Heart. Lippincott Williams & Wilkins. |
[51] |
Keyes DE, McInnes LC, Woodward C, et al. (2013) Multiphysics simulations: Challenges and opportunities. Internat J High Perform Comput App 27: 4–83. doi: 10.1177/1094342012468181
![]() |
[52] |
Knisley SB, Trayanova N, Aguel F (1999) Roles of electric field and fiber structure in cardiac electric stimulation. Biophys J 77: 1404–1417. doi: 10.1016/S0006-3495(99)76989-4
![]() |
[53] |
Krishnamoorthi S, Sarkar M, Klug WS (2013) Numerical quadrature and operator splitting in finite element methods for cardiac electrophysiology. Int J Numer Meth Bio 29: 1243–1266. doi: 10.1002/cnm.2573
![]() |
[54] |
Land S, Niederer SA, Smith NP (2012) Efficient computational methods for strongly coupled cardiac electromechanics. IEEE T Bio-Med Eng 59: 1219–1228. doi: 10.1109/TBME.2011.2112359
![]() |
[55] |
Lee HY, Codella NCF, Cham MD, et al. (2010) Automatic left ventricle segmentation using iterative thresholding and an active contour model with adaptation on short-axis cardiac MRI. IEEE T Bio-Med Eng 57: 905–913. doi: 10.1109/TBME.2009.2014545
![]() |
[56] |
LeGrice I, Hunter P, Young A, et al. (2001) The architecture of the heart: a data-based model. Philos T R Soc A: Math Phys Engng Sci 359: 1217–1232. doi: 10.1098/rsta.2001.0827
![]() |
[57] | Luo C, Rudy Y (1991) A model of the ventricular cardiac action potential. Depolarization, repolarization, and their interaction. Circ Res 68: 1501–1526. |
[58] | Luo C, Rudy Y (1994) A dynamic model of the cardiac ventricular action potential. I. Simulations of ionic currents and concentration changes. Circ Res 74: 1071–1096. |
[59] | Manzoni A, Pagani S, Lassila T (2016) Accurate solution of Bayesian inverse uncertainty quantification problems combining reduced basis methods and reduction error models. SIAM/ASA J UQ 4: 380–412. |
[60] |
Murray CJL, Ortblad KF, Guinovart C, et al. (2014) Global, regional, and national incidence and mortality for hiv, tuberculosis, and malaria during 1990–2013: a systematic analysis for the global burden of disease study 2013. The Lancet 384: 1005–1070. doi: 10.1016/S0140-6736(14)60844-8
![]() |
[61] |
Nickerson D, Smith N, Hunter P (2005) New developments in a strongly coupled cardiac electromechanical model. Europace 7: 118–127. doi: 10.1016/eupace/7.Supplement_1.118-b
![]() |
[62] |
Niederer SA, Kerfoot E, Benson AP, et al. (2011) Verification of cardiac tissue electrophysiology simulators using an n-version benchmark. Philos T R Soc A 369: 4331–4351. doi: 10.1098/rsta.2011.0139
![]() |
[63] |
Nobile F, Quarteroni A, Ruiz-Baier R (2012) An active strain electromechanical model for cardiac tissue. Int J Numer Meth Bio 28: 52–71. doi: 10.1002/cnm.1468
![]() |
[64] | Noble D (1962) A modification of the Hodgkin-Huxley equations applicable to purkinje fibre action and pacemaker potentials. J Phys 160: 317–352. |
[65] |
Nordsletten DA, Niederer SA, Nash MP, et al. (2011) Coupling multi-physics models to cardiac mechanics. Prog Biophys Mol Bio 104: 77–88. doi: 10.1016/j.pbiomolbio.2009.11.001
![]() |
[66] | Ogden RW (1997) Non-linear elastic deformations. Courier Corporation. |
[67] |
Omens JH, May KD, McCulloch AD (1991) Transmural distribution of three-dimensional strain in the isolated arrested canine left ventricle. Am J Physiol-Heart C 261: 918–928. doi: 10.1152/ajpheart.1991.261.3.H918
![]() |
[68] |
Patelli AS, Dedè L, Lassila T, et al. (2017) Isogeometric approximation of cardiac electrophysiology models on surfaces: An accuracy study with application to the human left atrium. Comput Method Appl M 317: 248–273. doi: 10.1016/j.cma.2016.12.022
![]() |
[69] |
Pathmanathan P, Bernabeu MO, Niederer SA, et al. (2012) Computational modelling of cardiac electrophysiology: explanation of the variability of results from different numerical solvers. Int J Numer Meth Bio 28: 890–903. doi: 10.1002/cnm.2467
![]() |
[70] |
Pathmanathan P, Mirams GR, Southern J, et al. (2011) The significant effect of the choice of ionic current integration method in cardiac electro-physiological simulations. Int J Numer Meth Bio Engng 27: 1751–1770. doi: 10.1002/cnm.1438
![]() |
[71] |
Pennacchio M, Savaré G, Colli Franzone P (2005) Multiscale modeling for the bioelectric activity of the heart. SIAM J Math Anal 37: 1333–1370. doi: 10.1137/040615249
![]() |
[72] | Peters J, Ecabert O, Meyer C, et al. (2007) Automatic whole heart segmentation in static magnetic resonance image volumes. MICCAI 1: 402–410. |
[73] | Pezzuto S (2013) Mechanics of the heart: constitutive issues and numerical experiments. PhD thesis, Politecnico di Milano, Italy. |
[74] |
Pezzuto S, Ambrosi D (2014) Active contraction of the cardiac ventricle and distortion of the microstructural architecture. Int J Numer Meth Bio Engng 30: 1578–1596. doi: 10.1002/cnm.2690
![]() |
[75] |
Potse M, Dubé B, Richer J, et al. (2006) A comparison of monodomain and bidomain reaction-diffusion models for action potential propagation in the human heart. IEEE T Bio-Med Eng 53: 2425–2435. doi: 10.1109/TBME.2006.880875
![]() |
[76] |
Quarteroni A, Lassila T, Rossi S, et al. (2017) Integrated heart - coupling multiscale and multiphysics models for the simulation of the cardiac function. Comput Method Appl M 314: 345–407. doi: 10.1016/j.cma.2016.05.031
![]() |
[77] |
Quarteroni A, Manzoni A, Vergara C (2017) The cardiovascular system: Mathematical modelling, numerical algorithms and clinical applications. Acta Num 26: 365–590. doi: 10.1017/S0962492917000046
![]() |
[78] | Quarteroni A, Sacco R, Saleri F (2010) Numerical Mathematics, volume 37, Springer Science & Business Media. |
[79] |
Quinn TA, Kohl P (2013) Combining wet and dry research: experience with model development for cardiac mechano-electric structure-function studies. Cardiovasc Res 97: 601–611. doi: 10.1093/cvr/cvt003
![]() |
[80] |
Reese TG, Weisskoff RM, Smith RN, et al. (1995) Imaging myocardial fiber architecture in vivo with magnetic resonance. Magn Reson Med 34: 786–791. doi: 10.1002/mrm.1910340603
![]() |
[81] | Regazzoni F, Dedè L, Quarteroni A (2017) Active contraction of cardiac cells: a model for sarcomere dynamics with cooperative interactions. MOX report, Politecnico di Milano, 48/2017. |
[82] | Rocha BM, Lino B, dos Santos RW, et al. (2009) A two dimensional model of coupled electromechanics in cardiac tissue. In World Congress on Medical Physics and Biomedical Engineering, September 7-12, 2009, Munich, Germany, Springer, 2081–2084. |
[83] |
Romero D, Sebastian R, Bijnens BH, et al. (2010) Effects of the Purkinje system and cardiac geometry on biventricular pacing: a model study. Ann Biomed Eng 38: 1388–1398. doi: 10.1007/s10439-010-9926-4
![]() |
[84] | Rossi S (2014) Anisotropic modeling of cardiac mechanical activation. PhD thesis, EPFL, Switzerland. |
[85] |
Rossi S, Lassila T, Ruiz-Baier R, et al. (2014) Thermodynamically consistent orthotropic activation model capturing ventricular systolic wall thickening in cardiac electromechanics. Eur J Mech A-Solid 48: 129–142. doi: 10.1016/j.euromechsol.2013.10.009
![]() |
[86] |
Rossi S, Ruiz-Baier R, Pavarino LF, et al. (2012) Orthotropic active strain models for the numerical simulation of cardiac biomechanics. Int J Numer Meth Bio 28: 761–788. doi: 10.1002/cnm.2473
![]() |
[87] |
Royse CF, Royse AG (2005) The myocardial and vascular effects of bupivacaine, levobupivacaine, and ropivacaine using pressure volume loops. Anesth Analg 101: 679–687. doi: 10.1213/01.ANE.0000157123.69327.6A
![]() |
[88] |
Ruiz-Baier R, Gizzi A, Rossi S, et al. (2014) Mathematical modelling of active contraction in isolated cardiomyocytes. Math Med Biol 31: 259–283. doi: 10.1093/imammb/dqt009
![]() |
[89] | Saad Y (2003) Iterative Methods for Sparse Linear Systems. SIAM. |
[90] |
Satz JE, Kanter HL, Green KG, et al. (1994) Tissue-specific determinants of anisotropic conduction velocity in canine atrial and ventricular myocardium. Circ Res 74: 1065–1070. doi: 10.1161/01.RES.74.6.1065
![]() |
[91] |
Sagawa K (1978) The ventricular pressure-volume diagram revisited. Circ Res 43: 677–687. doi: 10.1161/01.RES.43.5.677
![]() |
[92] |
Sainte-Marie J, Chapelle D, Cimrman R, et al. (2006) Modeling and estimation of the cardiac electromechanical activity. Comput Struct 84: 1743–1759. doi: 10.1016/j.compstruc.2006.05.003
![]() |
[93] |
Sansour C (2008) On the physical assumptions underlying the volumetric-isochoric split and the case of anisotropy. Eur J Mech A-Solid 27: 28–39. doi: 10.1016/j.euromechsol.2007.04.001
![]() |
[94] |
Sengupta PP, Korinek J, Belohlavek M, et al. (2006) Left ventricular structure and function. J Am College Card 48: 1988–2001. doi: 10.1016/j.jacc.2006.08.030
![]() |
[95] | Simo JC, Taylor RL (1991) Quasi-incompressible finite elasticity in principal stretches. continuum basis and numerical algorithms. Comput Method Appl M 85: 273–310. |
[96] |
Smith NP, Nickerson DP, Crampin EJ, et al. (2004) Multiscale computational modelling of the heart. Acta Num 13: 371–431. doi: 10.1017/S0962492904000200
![]() |
[97] |
Sugiura S,Washio T, Hatano A, et al. (2012) Multi-scale simulations of cardiac electrophysiology and mechanics using the university of tokyo heart simulator. Prog Biophys Mol Bio 110: 380–389. doi: 10.1016/j.pbiomolbio.2012.07.001
![]() |
[98] |
Tagliabue A, Dedè L, Quarteroni A (2017) Complex blood flow patterns in an idealized left ventricle: a numerical study. Chaos 27: 93939–93964. doi: 10.1063/1.5002120
![]() |
[99] |
Tagliabue A, Dedè L, Quarteroni A (2017) Fluid dynamics of an idealized left ventricle: the extended Nitsche's method for the treatment of heart valves as mixed time varying boundary conditions. Int J Numer Meth Fl 85: 135–164. doi: 10.1002/fld.4375
![]() |
[100] |
Takizawa K, Bazilevs Y, Tezduyar TE (2012) Space-time and ale-vms techniques for patient-specific cardiovascular fluid-structure interaction modeling. Arch Comput Method E 19: 171–225. doi: 10.1007/s11831-012-9071-3
![]() |
[101] |
Takizawa K, Christopher J, Tezduyar TE, et al. (2010) Space-time finite element computation of arterial fluid-structure interactions with patient-specific data. Int J Numer Meth Bio 26: 101–116. doi: 10.1002/cnm.1241
![]() |
[102] |
Ten Tusscher KHWJ, Noble D, Noble PJ, et al. (2004) A model for human ventricular tissue. Am J Physiol-Heart C 286: 1573–1589. doi: 10.1152/ajpheart.00794.2003
![]() |
[103] |
Tezduyar TE, Sathe S, Schwaab M, et al. (2008) Arterial fluid mechanics modeling with the stabilized space-time fluid-structure interaction technique. Int J Numer Meth Fl 57: 601–629. doi: 10.1002/fld.1633
![]() |
[104] | Toselli A, Widlund OB (2005) Domain decomposition methods: algorithms and theory, volume 34, Springer. |
[105] |
Trayanova NA (2011) Whole-heart modeling applications to cardiac electrophysiology and electromechanics. Circ Res 108: 113–128. doi: 10.1161/CIRCRESAHA.110.223610
![]() |
[106] | Trentin C, Faggiano E, Conti M, et al. (2015) An automatic tool for thoracic aorta segmentation and 3D geometric analysis. International Symposium on Image and Signal Processing and Analysis (ISPA), 60–65. |
[107] |
Usyk TP, LeGrice IJ, McCulloch AD (2002) Computational model of three-dimensional cardiac electromechanics. Comput Vis Sci 4: 249–257. doi: 10.1007/s00791-002-0081-9
![]() |
[108] |
Vergara C, Lange M, Palamara S, et al. (2016) A coupled 3D-1D numerical monodomain solver for cardiac electrical activation in the myocardium with detailed purkinje network. J Computat Phys 308: 218–238. doi: 10.1016/j.jcp.2015.12.016
![]() |
[109] |
Vergara C, Palamara S, Catanzariti D, et al. (2014) Patient-specific generation of the Purkinje network driven by clinicalm easurements of a normal propagation. Med Biol Eng Comput 52: 813–826. doi: 10.1007/s11517-014-1183-5
![]() |
[110] |
Westerhof N, Lankhaar JW,Westerhof BE (2009) The arterial Windkessel. Med Biol Eng Comput 47: 131–141. doi: 10.1007/s11517-008-0359-2
![]() |
[111] |
Wink AM, Roerdink JBTM (2004) Denoising functional MR images: a comparison of wavelet denoising and Gaussian smoothing. IEEE T Med Imaging 23: 374–387. doi: 10.1109/TMI.2004.824234
![]() |
[112] |
Wong J, Kuhl E (2014) Generating fibre orientation maps in human heart models using poisson interpolation. Comput Method Biomec 17: 1217–1226. doi: 10.1080/10255842.2012.739167
![]() |
[113] |
Yin FC, Chan CC, Judd RM (1996) Compressibility of perfused passive myocardium. Am J Physiol-Heart C 271: H1864–H1870. doi: 10.1152/ajpheart.1996.271.5.H1864
![]() |
[114] | Zheng Y, Barbu A, Georgescu B, et al. (2007) Fast automatic heart chamber segmentation from 3D CT data using marginal space learning and steerable features. International Conference on Computer Vision, 1–8. |
[115] |
Zhuang X, Rhode KS, Razavi RS, et al. (2010) A registration-based propagation framework for automatic whole heart segmentation of cardiac MRI. IEEE T Med Imaging 29: 1612–1625. doi: 10.1109/TMI.2010.2047112
![]() |
1. | Francesco Regazzoni, Luca Dedè, Alfio Quarteroni, Active Force Generation in Cardiac Muscle Cells: Mathematical Modeling and Numerical Simulation of the Actin-Myosin Interaction, 2020, 2305-221X, 10.1007/s10013-020-00433-z | |
2. | Matteo Salvador, Luca Dede’, Alfio Quarteroni, An intergrid transfer operator using radial basis functions with application to cardiac electromechanics, 2020, 66, 0178-7675, 491, 10.1007/s00466-020-01861-x | |
3. | F. Regazzoni, A. Quarteroni, An oscillation-free fully staggered algorithm for velocity-dependent active models of cardiac mechanics, 2021, 373, 00457825, 113506, 10.1016/j.cma.2020.113506 | |
4. | F. Regazzoni, L. Dedè, A. Quarteroni, Machine learning of multiscale active force generation models for the efficient simulation of cardiac electromechanics, 2020, 370, 00457825, 113268, 10.1016/j.cma.2020.113268 | |
5. | Stefano Pagani, Luca Dede’, Andrea Manzoni, Alfio Quarteroni, Data integration for the numerical simulation of cardiac electrophysiology, 2021, 0147-8389, 10.1111/pace.14198 | |
6. | Francesco Regazzoni, Luca Dedè, Alfio Quarteroni, Daniel A Beard, Biophysically detailed mathematical models of multiscale cardiac active mechanics, 2020, 16, 1553-7358, e1008294, 10.1371/journal.pcbi.1008294 | |
7. | Francesco Regazzoni, Luca Dedè, Alfio Quarteroni, Active contraction of cardiac cells: a reduced model for sarcomere dynamics with cooperative interactions, 2018, 17, 1617-7959, 1663, 10.1007/s10237-018-1049-0 | |
8. | Luca Barbarotta, Simone Rossi, Luca Dedè, Alfio Quarteroni, A transmurally heterogeneous orthotropic activation model for ventricular contraction and its numerical validation, 2018, 34, 2040-7939, 10.1002/cnm.3137 | |
9. | Luca Pegolotti, Luca Dedè, Alfio Quarteroni, Isogeometric Analysis of the electrophysiology in the human heart: Numerical simulation of the bidomain equations on the atria, 2019, 343, 00457825, 52, 10.1016/j.cma.2018.08.032 | |
10. | L. Dede’, A. Gerbi, A. Quarteroni, 2020, Chapter 3, 978-3-030-45196-7, 81, 10.1007/978-3-030-45197-4_3 | |
11. | Mahmoud Moustafa, Mohd Hafiz Mohd, Ahmad Izani Ismail, Farah Aini Abdullah, Dynamical analysis of a fractional order eco-epidemiological model with nonlinear incidence rate and prey refuge, 2021, 65, 1598-5865, 623, 10.1007/s12190-020-01408-6 | |
12. | Hongyan Zhao, Fangxin Zhou, Huaping Liu, Deep learning for diplomatic video analysis, 2020, 79, 1380-7501, 4811, 10.1007/s11042-018-6650-9 | |
13. | Zhaolun Wang, Numerical Analysis of Deformation Control of Deep Foundation Pit in Ulanqab City, 2021, 0960-3182, 10.1007/s10706-021-01836-6 | |
14. | Alessandro Nitti, Josef Kiendl, Alessio Gizzi, Alessandro Reali, Marco D. de Tullio, A curvilinear isogeometric framework for the electromechanical activation of thin muscular tissues, 2021, 382, 00457825, 113877, 10.1016/j.cma.2021.113877 | |
15. | Balaram Murthy Chintakindi, Mohammad Farukh Hashmi, SSAD: Single-Shot Multi-Scale Attentive Detector for Autonomous Driving, 2023, 0256-4602, 1, 10.1080/02564602.2023.2176932 | |
16. | Chengsheng Luo, Linjun Wang, Youxiang Xie, Baojia Chen, A New Conjugate Gradient Method for Moving Force Identification of Vehicle–Bridge System, 2022, 2523-3920, 10.1007/s42417-022-00824-1 | |
17. | Junhua Liu, Xiaoli Liu, Fu Wang, Xuejiao Ma, Xiaohang Yue, Equilibrium decisions and coordination of a logistics service supply chain with bilateral corporate social responsibility, 2022, 56, 0399-0559, 2595, 10.1051/ro/2022115 | |
18. | F. Regazzoni, A. Quarteroni, Accelerating the convergence to a limit cycle in 3D cardiac electromechanical simulations through a data-driven 0D emulator, 2021, 135, 00104825, 104641, 10.1016/j.compbiomed.2021.104641 | |
19. | F. Regazzoni, M. Salvador, P.C. Africa, M. Fedele, L. Dedè, A. Quarteroni, A cardiac electromechanical model coupled with a lumped-parameter model for closed-loop blood circulation, 2022, 457, 00219991, 111083, 10.1016/j.jcp.2022.111083 | |
20. | Roberto Piersanti, Francesco Regazzoni, Matteo Salvador, Antonio F. Corno, Luca Dede’, Christian Vergara, Alfio Quarteroni, 3D–0D closed-loop model for the simulation of cardiac biventricular electromechanics, 2022, 391, 00457825, 114607, 10.1016/j.cma.2022.114607 | |
21. | Michele Bucelli, Alberto Zingaro, Pasquale Claudio Africa, Ivan Fumagalli, Luca Dede', Alfio Quarteroni, A mathematical model that integrates cardiac electrophysiology, mechanics, and fluid dynamics: Application to the human left heart, 2023, 2040-7939, 10.1002/cnm.3678 | |
22. | Barış Cansız, Michael Kaliske, A comparative study of fully implicit staggered and monolithic solution methods. Part I: Coupled bidomain equations of cardiac electrophysiology, 2022, 407, 03770427, 114021, 10.1016/j.cam.2021.114021 | |
23. | Alfio Quarteroni, Luca Dedè, Francesco Regazzoni, Modeling the cardiac electromechanical function: A mathematical journey, 2022, 59, 0273-0979, 371, 10.1090/bull/1738 | |
24. | F. Regazzoni, M. Salvador, L. Dede’, A. Quarteroni, A machine learning method for real-time numerical simulations of cardiac electromechanics, 2022, 393, 00457825, 114825, 10.1016/j.cma.2022.114825 | |
25. | Feng Wang, Guanghui Song, Jingqi Xuan, Han Wu, 2021, Chapter 201, 978-3-030-70664-7, 1860, 10.1007/978-3-030-70665-4_201 | |
26. | Tobias Gerach, Steffen Schuler, Jonathan Fröhlich, Laura Lindner, Ekaterina Kovacheva, Robin Moss, Eike Moritz Wülfers, Gunnar Seemann, Christian Wieners, Axel Loewe, Electro-Mechanical Whole-Heart Digital Twins: A Fully Coupled Multi-Physics Approach, 2021, 9, 2227-7390, 1247, 10.3390/math9111247 | |
27. | Matteo Salvador, Marco Fedele, Pasquale Claudio Africa, Eric Sung, Luca Dede', Adityo Prakosa, Jonathan Chrispin, Natalia Trayanova, Alfio Quarteroni, Electromechanical modeling of human ventricles with ischemic cardiomyopathy: numerical simulations in sinus rhythm and under arrhythmia, 2021, 136, 00104825, 104674, 10.1016/j.compbiomed.2021.104674 | |
28. | Matteo Salvador, Francesco Regazzoni, Stefano Pagani, Luca Dede', Natalia Trayanova, Alfio Quarteroni, The role of mechano-electric feedbacks and hemodynamic coupling in scar-related ventricular tachycardia, 2022, 142, 00104825, 105203, 10.1016/j.compbiomed.2021.105203 | |
29. | Marco Fedele, Roberto Piersanti, Francesco Regazzoni, Matteo Salvador, Pasquale Claudio Africa, Michele Bucelli, Alberto Zingaro, Luca Dede’, Alfio Quarteroni, A comprehensive and biophysically detailed computational model of the whole human heart electromechanics, 2023, 410, 00457825, 115983, 10.1016/j.cma.2023.115983 | |
30. | Ludovica Cicci, Stefania Fresca, Elena Zappon, Stefano Pagani, Francesco Regazzoni, Luca Dede', Andrea Manzoni, Alfio Quarteroni, 2023, 9780323899673, 403, 10.1016/B978-0-32-389967-3.00028-7 | |
31. | Michele Bucelli, Martin Geraint Gabriel, Alfio Quarteroni, Giacomo Gigante, Christian Vergara, A stable loosely-coupled scheme for cardiac electro-fluid-structure interaction, 2023, 490, 00219991, 112326, 10.1016/j.jcp.2023.112326 | |
32. | Michele Bucelli, Francesco Regazzoni, Luca Dede’, Alfio Quarteroni, Preserving the positivity of the deformation gradient determinant in intergrid interpolation by combining RBFs and SVD: Application to cardiac electromechanics, 2023, 00457825, 116292, 10.1016/j.cma.2023.116292 | |
33. | Ludovica Cicci, Stefania Fresca, Andrea Manzoni, Alfio Quarteroni, Efficient approximation of cardiac mechanics through reduced‐order modeling with deep learning‐based operator approximation, 2023, 2040-7939, 10.1002/cnm.3783 | |
34. | Benjamin Maier, Dominik Göddeke, Felix Huber, Thomas Klotz, Oliver Röhrle, Miriam Schulte, OpenDiHu: An efficient and scalable framework for biophysical simulations of the neuromuscular system, 2024, 18777503, 102291, 10.1016/j.jocs.2024.102291 | |
35. | Barış Cansız, Michael Kaliske, A comparative study of fully implicit staggered and monolithic solution methods. Part II: Coupled excitation–contraction equations of cardiac electromechanics, 2024, 442, 03770427, 115704, 10.1016/j.cam.2023.115704 | |
36. | Elena Zappon, Matteo Salvador, Roberto Piersanti, Francesco Regazzoni, Luca Dede’, Alfio Quarteroni, An integrated heart–torso electromechanical model for the simulation of electrophysiological outputs accounting for myocardial deformation, 2024, 427, 00457825, 117077, 10.1016/j.cma.2024.117077 | |
37. | Giorgos Troulliotis, Alison Duncan, Xiao Yun Xu, Alessandro Gandaglia, Fillipo Naso, Hendrik Versteeg, Saeed Mirsadraee, Sotiris Korossis, Effect of Excitation Sequence of Myocardial Contraction on the Mechanical Response of the Left Ventricle, 2024, 13504533, 104255, 10.1016/j.medengphy.2024.104255 | |
38. | Francesco Regazzoni, Stabilization of loosely coupled schemes for 0D–3D fluid–structure interaction problems with application to cardiovascular modelling, 2025, 157, 0029-599X, 249, 10.1007/s00211-025-01452-z | |
39. | Jiří Vaverka, Jiří Burša, A modification of Holzapfel–Ogden hyperelastic model of myocardium better describing its passive mechanical behavior, 2025, 111, 09977538, 105586, 10.1016/j.euromechsol.2025.105586 |
Geometry | Mesh | # Vertices | # Elements | |
Idealized(Fig. 6) | (a) | 4.6 mm | 1'827 | 3'010 |
(b) | 2.3 mm | 11'658 | 12'040 | |
(c) | 1.2 mm | 81'335 | 416'000 | |
Patient-specific(Fig. 7) | (d) | 0.8 mm | 126'031 | 637'379 |
Geometry | Mesh | # Vertices | # Elements | |
Idealized(Fig. 6) | (a) | 4.6 mm | 1'827 | 3'010 |
(b) | 2.3 mm | 11'658 | 12'040 | |
(c) | 1.2 mm | 81'335 | 416'000 | |
Patient-specific(Fig. 7) | (d) | 0.8 mm | 126'031 | 637'379 |
Geometry | Mesh | # CPUs | ||
Idealized | (a) | 1 | 14'616 | 1 |
(b) | 1 | 93'264 | 6 | |
(c) | 1 | 650'680 | 45 | |
(a) | 2 | 93'264 | 6 | |
Patient-specific | (d) | 1 | 1'008'248 | 72 |
| | | | | | | | | | | | | |
| | | | | | | | | | | 12 | 500 | |
| | | | | | | | | | | | | |
50 | 10 | 1500 | 0 | | 5 | 1 | 0 | 0 | | | | |
Geometry | Mesh | |||||||||
Idealized | (a) | 1 | 3.02 | 2.31 | 2.55 | 2.15 | 0.023 | 0.006 | 5h 41m | |
(b) | 1 | 3.37 | 2.14 | 3.44 | 6.67 | 0.163 | 0.019 | 18h 21m | ||
(c) | 1 | 4.23 | 2.49 | 3.26 | 9.19 | 0.632 | 0.040 | 46h 42m | ||
(a) | 2 | 3.29 | 1.68 | 2.11 | 6.47 | 0.178 | 0.025 | 30h 18m | ||
Patient-specific | (d) | 1 | 4.44 | 1.96 | 4.51 | 22.33 | 1.692 | 0.076 | 68h 37m |
Geometry | Mesh | # Vertices | # Elements | |
Idealized(Fig. 6) | (a) | 4.6 mm | 1'827 | 3'010 |
(b) | 2.3 mm | 11'658 | 12'040 | |
(c) | 1.2 mm | 81'335 | 416'000 | |
Patient-specific(Fig. 7) | (d) | 0.8 mm | 126'031 | 637'379 |
Geometry | Mesh | # Vertices | # Elements | |
Idealized(Fig. 6) | (a) | 4.6 mm | 1'827 | 3'010 |
(b) | 2.3 mm | 11'658 | 12'040 | |
(c) | 1.2 mm | 81'335 | 416'000 | |
Patient-specific(Fig. 7) | (d) | 0.8 mm | 126'031 | 637'379 |
Geometry | Mesh | # CPUs | ||
Idealized | (a) | 1 | 14'616 | 1 |
(b) | 1 | 93'264 | 6 | |
(c) | 1 | 650'680 | 45 | |
(a) | 2 | 93'264 | 6 | |
Patient-specific | (d) | 1 | 1'008'248 | 72 |
| | | | | | | | | | | | | |
| | | | | | | | | | | 12 | 500 | |
| | | | | | | | | | | | | |
50 | 10 | 1500 | 0 | | 5 | 1 | 0 | 0 | | | | |
Geometry | Mesh | |||||||||
Idealized | (a) | 1 | 3.02 | 2.31 | 2.55 | 2.15 | 0.023 | 0.006 | 5h 41m | |
(b) | 1 | 3.37 | 2.14 | 3.44 | 6.67 | 0.163 | 0.019 | 18h 21m | ||
(c) | 1 | 4.23 | 2.49 | 3.26 | 9.19 | 0.632 | 0.040 | 46h 42m | ||
(a) | 2 | 3.29 | 1.68 | 2.11 | 6.47 | 0.178 | 0.025 | 30h 18m | ||
Patient-specific | (d) | 1 | 4.44 | 1.96 | 4.51 | 22.33 | 1.692 | 0.076 | 68h 37m |