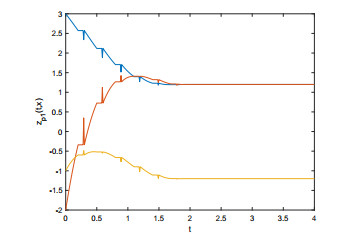
Citation: Lawrence O. Aghenta, M. Tariq Iqbal. Design and implementation of a low-cost, open source IoT-based SCADA system using ESP32 with OLED, ThingsBoard and MQTT protocol[J]. AIMS Electronics and Electrical Engineering, 2020, 4(1): 57-86. doi: 10.3934/ElectrEng.2020.1.57
[1] | Prasina Alexander, Fatemeh Parastesh, Ibrahim Ismael Hamarash, Anitha Karthikeyan, Sajad Jafari, Shaobo He . Effect of the electromagnetic induction on a modified memristive neural map model. Mathematical Biosciences and Engineering, 2023, 20(10): 17849-17865. doi: 10.3934/mbe.2023793 |
[2] | P. E. Greenwood, L. M. Ward . Rapidly forming, slowly evolving, spatial patterns from quasi-cycle Mexican Hat coupling. Mathematical Biosciences and Engineering, 2019, 16(6): 6769-6793. doi: 10.3934/mbe.2019338 |
[3] | Pan Wang, Xuechen Li, Qianqian Zheng . Synchronization of inertial complex-valued memristor-based neural networks with time-varying delays. Mathematical Biosciences and Engineering, 2024, 21(2): 3319-3334. doi: 10.3934/mbe.2024147 |
[4] | Mahtab Mehrabbeik, Fatemeh Parastesh, Janarthanan Ramadoss, Karthikeyan Rajagopal, Hamidreza Namazi, Sajad Jafari . Synchronization and chimera states in the network of electrochemically coupled memristive Rulkov neuron maps. Mathematical Biosciences and Engineering, 2021, 18(6): 9394-9409. doi: 10.3934/mbe.2021462 |
[5] | Shuai Zhang, Yongqing Yang, Xin Sui, Yanna Zhang . Synchronization of fractional-order memristive recurrent neural networks via aperiodically intermittent control. Mathematical Biosciences and Engineering, 2022, 19(11): 11717-11734. doi: 10.3934/mbe.2022545 |
[6] | Guowei Wang, Yan Fu . Spatiotemporal patterns and collective dynamics of bi-layer coupled Izhikevich neural networks with multi-area channels. Mathematical Biosciences and Engineering, 2023, 20(2): 3944-3969. doi: 10.3934/mbe.2023184 |
[7] | Karim El Laithy, Martin Bogdan . Synaptic energy drives the information processing mechanisms in spiking neural networks. Mathematical Biosciences and Engineering, 2014, 11(2): 233-256. doi: 10.3934/mbe.2014.11.233 |
[8] | Hai Lin, Jingcheng Wang . Pinning control of complex networks with time-varying inner and outer coupling. Mathematical Biosciences and Engineering, 2021, 18(4): 3435-3447. doi: 10.3934/mbe.2021172 |
[9] | Wenjing Wang, Jingjing Dong, Dong Xu, Zhilian Yan, Jianping Zhou . Synchronization control of time-delay neural networks via event-triggered non-fragile cost-guaranteed control. Mathematical Biosciences and Engineering, 2023, 20(1): 52-75. doi: 10.3934/mbe.2023004 |
[10] | Biwen Li, Xuan Cheng . Synchronization analysis of coupled fractional-order neural networks with time-varying delays. Mathematical Biosciences and Engineering, 2023, 20(8): 14846-14865. doi: 10.3934/mbe.2023665 |
The memristor is the fourth basic circuit element, and it was firstly proposed by Chua in 1971 [1]. The first memristor device was realized in 2008 [2], and since then it has received much attention from research area, such as neural networks, signal processing and artificial intelligence. As a particular type of neural networks, memristive neural networks (MNNs) have become an interesting topic for researchers [3,4,5,6]. MNNs can be regarded as a switch system since the memristive connection weights switch according to the system states. Compared with the traditional network model, MNNs have more equilibrium points and can better modify the dynamics of neurons in human brains. Lately, the dynamical analysis of MNNs has attracted great attention [7,8,9].
As we know, reaction-diffusion influences are important and common in chemistry, biology and neuroscience. Since the electrons in circuits move in a nonuniform electric field, the state of the electrons dose not only depend on time but also on the spatial information. Due to this reason, MNNs with reaction-diffusion terms can better modify the real-world systems than the traditional MNNs. Recently, the research on dynamics of reaction-diffusion MNNs has made great progress, such as stability [10], passivity [11,12], and asymptotical synchronization [13,14]. However, most of these results only focus on the cooperative interactions between neurons, while the competitive relationships have not been considered yet.
In the real world, competition and cooperation relationships coexist in many dynamic systems. For instance, in biological systems, interactions between cells may be cooperative or competitive; in social networks, relationships between people may be hostile or friendly. Hence, it is meaningful and necessary to consider the model of coopetition networks, in which the competition and cooperation relationships coexist [15,16,17,18,19,20,21]. Due to the existence of competition relationships, the traditional synchronization cannot be realized, and instead we will consider bipartite synchronization. In Reference [15], the idea of bipartite consensus in coopetition networks was first proposed, it requires that all nodes' states will reach the consensus in modulus but may not in sign. Recently, bipartite synchronization of coopetition networks has attracted the attention of many scholars [18,19,20,21]. In Reference [19], by designing an adaptive control strategy, the bipartite synchronization of coupled coopetition MNNs is investigated. In Reference [20], the model of inertial MNNs with antagonistic interactions is considered, and the criteria for bipartite synchronization are derived in both the leaderless and leader-following case. However, the above works only focus on the asymptotic or exponential synchronization, and how to improve the convergence speed in bipartite synchronization still remains an open topic.
Synchronization is an important phenomenon in engineering and biological systems. As we know, the convergence speed is an important issue in synchronization. To overcome the constraints of long convergence time, the issue of finite-time synchronization (FTS) is proposed [24]. Different from asymptotic synchronization [22,23], FTS guarantees the system will reach synchronization in a finite time. However, the convergence time of FTS is related to the initial conditions [24,25,26,27], which may be unmeasurable in practice. To solve this problem, the idea of fixed-time synchronization (FxT) is introduced [28]. Although the FxTS control removes the limitation of initial dependence, its settling time is related to the control parameters [28,29,30,31]. Hence, the settling time of traditional FTS and FxTS control still depend on the initial conditions and system parameters, which is a great limitation for some practical applications. To solve this problem, prespecified-time synchronization (PTS) control is proposed [32,33,34,35], in which the settling time is independent of both initial value and control parameters. As we know, the investigation of prespecified-time control is still very limited, especially for the coupled networks with competitive interactions.
This paper is intended to explore the prespecified-time bipartite synchronization (PTBS) of CRDMNNs with both cooperative and competitive relationships. The main contributions of this work are as follows:
1) Different from most previous results that only focus on cooperative interactions, this paper considers the model of CRDMNNs with both cooperative and competitive relationships between neurons.
2) By designing a novel control protocol, criteria for leader-following PTBS and leaderless PTBS of CRDMNNs are derived. It is shown that under the proposed protocol, all nodes' states will reach synchronization in modulus (but might not in sign) within a prescribed time.
3) Different from the traditional FTS and FxTS methods, the prespecified-time control method in this work can lead to a predetermined settling time, which is independent of both initial values and system parameters.
Notations. In this paper, sgn(⋅) denotes the signum function. The symbol T denotes vector transposition. C1 denotes the set of continuous functions. λmax(A) is the maximal eigenvalue of matrix A. ⊗ denotes the Kronecker product. Ω={x=(x1,⋯,xϑ)T|hmk≤|xk|≤hMk,k=1,⋯,ϑ} is a bounded compact set with smooth boundary ∂Ω.
A signed graph is represented by G={P,J,G}, where P={p1,p2,⋯,pN} is the node set, J⊂P×P={(pi,pj)|pi,pj∈P} denotes the edge set, G=[Gij]∈RN×N is the adjacent matrix satisfying that Gij≠0 if (pi,pj)∈J, and Gij=0 otherwise. It is assumed that Gii=0. If the relationship between nodes pi and pj is competitive (cooperative), then Gij<0 (Gij>0). Due to the existence of negative edges, the elements in the Laplacian matrix L of a signed graph are given as
lik={∑j∈Ni|Gij|,k=i,Gik,k≠i. |
Definition 1. For a signed graph, it is called structurally balanced if the node set P can be divided into two subgroups P1 and P2, where P1∪P2=P and P1∩P2=∅, such that Gij≥0 for ∀pi,pj∈Pl(l∈{1,2}) and Gij≤0 for ∀pi∈Pl,pj∈Pk(k≠l,k,l∈{1,2}) [15].
Lemma 1. Suppose L is the Laplacian matrix of a signed graph G. If G is structurally balanced, then there is a gauge transformation matrix S=diag(s1,⋯,sN) with si∈{−1,1}, such that SLS has allnonnegative entries [15].
The model of CRDMNNs with competitive interactions is:
∂zp(t,x)∂t=DΔzp(t,x)−Czp(t,x)+A(zp(t,x))f(zp(t,x))+σ∑q∈Np|Gpq|Γ(sgn(Gpq)zq(t,x)−zp(t,x))+up(t,x),p=1,⋯,n | (2.1) |
where zp(t,x)=(zp1(t,x),⋯,zpn(t,x))T∈Rn denotes the state of the pth neural network at time t and space x∈Ω⊆Rz. f(zp(t,x))=(f1(zp1(t,x)),⋯,fn(zpn(t,x)))T∈Rn denotes the activation function. Δ=∑ϑk=1(∂2∂x2k) is the Laplace diffusion operator. D=diag(d1,⋯,dn)>0. C=diag(c1,c2,⋯,cn)>0. A(zp(t,x))=[akj(zpj(t,x))]n×n stands for the memristive connection weight matrix. σ is a positive number denoting the coupling strength, and Np is the neighbor set of node p. Γ=diag(γ1,γ2,⋯,γn)∈Rn×n is the inner coupling matrix. up(t,x) is the control input to be designed. G=(Gpq)N×N is the symmetric weighted adjacency matrix of the signed graph, representing the competitive-cooperation relationship between neurons. The memristive connection weights akj(zpj(t,x)) can be described as
akj(zpj(t,x))={ˊakj,|zpj(t,x)|≤Tj,ˊakj,|zpj(t,x)|>Tj, | (2.2) |
where Tj>0 is the switching jump, and ˊakj,ˊakj are real numbers. Define
a_kj=min{ˊakj,ˊakj},ˉakj=max{ˊakj,ˊakj},a+kj=max{|ˊakj|,|ˊakj|}. |
The initial condition and Dirichlet boundary condition of zp(t,x) are
{zp(t0,x)=ψp(x),x∈Ω,zp(t,x)=0,(t,x)∈[t0,+∞)×∂Ω. | (2.3) |
Remark 1. In this article, the reaction-diffusion term is contained in the MNNs model, and it is closer to the real networks because spatial location is considered. So, our results are more general than previous results in [19,20].
Next, we make the following assumptions.
Assumption 1. The cooperative-competitive network G is structurally balanced and connected.
Assumption 2. The activation function fp(⋅) is bounded, and there existconstants σp and Υ such that
|fp(x)−sqfp(y)|≤σp|x−sqy|,∀x,y∈R,|fp(x)|≤Υ,∀x∈R. |
In addition, define F=diag(σ1,⋯,σn)∈Rn×n.
Lemma 2. If η(x)=η(x1,⋯,xϑ):Ω→R satisfies that η(x)∈C1(Ω) and η(x)|∂Ω=0, then [12]
∫Ωϑ∑k=1(η(x)∂2η(x)∂x2k)dx≤−ϑ∑k=1π2(hMk−hmk)2∫Ωη2(x)dx. |
Based on the feature of Laplacian matrix L of the signed graph, we have:
∑q∈Np|Gpq|Γ(sgn(Gpq)zq(t,x)−zp(t,x))=∑q∈NpGpqΓzq(t,x)−∑q∈Np|Gpq|Γzq(t,x)=N∑q=1lpqΓzq(t,x). | (2.4) |
Hence, CRDMNN (2.1) can be written as
∂zp(t,x)∂t=DΔzp(t,x)−Czp(t,x)+A(zp(t,x))f(zp(t,x))+σN∑q=1lpqΓzq(t,x)+up(t,x). | (2.5) |
Definition 2. The CRDMNNs System (2.5) is said to reach leaderless PTBS, if there exists a time T, which is a prespecified constant for any initial values, such that
limt→T−‖spzp(t,x)−sqzq(t,x)‖=0,p,q=1,⋯,N,‖spzp(t,x)−sqzq(t,x)‖≡0,∀t>T. |
Remark 2. In this work, we use zq(t,x)−zp(t,x) to represent the cooperation relationship between nodes p and q, and we use zq(t,x)+zp(t,x) to depict the competitive relationship between nodes p and q.
The System (2.5) can be rewritten as the following matrix form:
∂z(t,x)∂t=ˇDΔz(t,x)−ˇCz(t,x)+ˇA(z(t,x))f(z(t,x))+σ(L⊗Γ)z(t,x)+u(t,x). | (2.6) |
where
z(t,x)=(zT1(t,x),⋯,zTN(t,x))T,ˇD=IN⊗D,u(t,x)=(uT1(t,x),⋯,uTN(t,x))T,ˇC=IN⊗C,ˇA(z(t,x))=diag(A(z1(t,x)),⋯,A(zN(t,x))),f(z(t,x))=(fT(z1(t,x)),⋯,fT(zN(t,x)))T |
To achieve the main results, two classes of matrices are given. Define
M=[s1−s200⋯000s2−s30⋯0000s3−s4⋯00⋮⋮⋮⋮⋮⋮⋮0000…sN−1−sN](N−1)×N |
J=[s1s1s1⋯s1s10s2s2⋯s2s200s3s3⋯s3⋮⋮⋮⋮⋮⋮000…0sN−1000…00]N×(N−1) |
Definition 3. For the CRDMNN System (2.6), define:
d(z)=‖Mz(t,x)‖2=∫ΩzT(t,x)MTMz(t,x)dx, |
where M=M⊗In. Then, the networks' states can reach leaderless PTBS, if there exists a time T, which is a prescribed constant for any initial values, such that
limt→T−d(z)=0,d(z)≡0,∀t>T. |
Lemma 3. For the Laplacian matrix L∈RN×N, there is a matrix H∈R(N−1)×(N−1) given by H=MLJ, so that the following condition is satisfied:
HM=ML,Hij=j∑k=1sisklik−si+1skli+1,k |
For convenience, define
ˉA=IN⊗ˉA,ˉA1=IN−1⊗ˉA,H=H⊗Γ,ˆC1=IN−1⊗C,ˆD1=IN−1⊗D,F=IN−1⊗F,D∗=ϑ∑k=1π2(hMk−hmk)2ˆD1 |
To achieve leaderless PTBS, the following controller for System (2.5) is introduced:
up(t,x)=−KspN−1∑j=psgn(sjzj(t,x)−sj+1zj+1(t,x))−ξpzp(t,x)−ψrTμ(t)zp(t,x) | (3.1) |
where K=diag(k1,k2,⋯,kn)>0, ξp>0 and ψ>0 are control gains to be designed. r is a positive constant, and T>0 is a prespecified time chosen by users. Let tb=t0+T, and μ(t) is a function defined as:
μ(t)={Ttb−t,t∈[t0,tb),1,t∈[tb,+∞). | (3.2) |
The derivative of μ(t) can be calculated:
˙μ(t)={1Tμ2(t),t∈[t0,tb),0,t∈[tb,+∞). | (3.3) |
With the control protocol (3.1), the theorem can be achieved.
Theorem 1. Under Assumptions 1 and 2, if the control gains constants kj,ξp satisfy the following conditions
kj≥2n∑s=1(ˉajs−a_js)Υ,j=1,2,⋯,n,−˜ξ+λmax(−D∗−ˆC1+ˉA1ˉAT1+F2+σH)≤−ϕ2 |
where ˜ξ=minp{ξp}, and ϕ>0 is a positive constant, then the leaderless bipartite prespecified-time synchronization for CRDMNN (2.6) can be achieved with controller (3.1).
Proof. Before proceeding, let y(t,x)=Mz(t,x), and then we get yi(t,x)=sizi(t,x)−si+1zi+1(t,x),i=1,⋯,N−1. Consider the Lyapunov functional
V(t)=12∫ΩzT(t,x)MTMz(t,x)dx. | (3.4) |
Computing the derivative along (2.6), it follows that
dV(t)dt=∫ΩzT(t,x)MTM˙z(t,x)dx=∫ΩzT(t,x)MTM(ˇDΔz(t,x)−ˇCz(t,x)+ˇA(z(t,x))f(z(t,x))+σ(L⊗Γ)z(t,x)+u(t,x))dx. | (3.5) |
From Lemma 2, we get
∫ΩzT(t,x)MTMˇDΔz(t,x)dx=∫ΩzT(t,x)MTˆD1MΔz(t,x)dx=∫ΩN−1∑i=1yTi(t,x)DΔyi(t,x)dx≤−ϑ∑k=1π2(hMk−hmk)2∫ΩN−1∑i=1yTi(t,x)Dyi(t,x)dx=−ϑ∑k=1π2(hMk−hmk)2∫ΩzT(t,x)MTˆD1Mz(t,x)dx=∫ΩzT(t,x)MTD∗Mz(t,x)dx. | (3.6) |
Note that
MˆA(z(t,x))f(z(t,x))=MˉAf(z(t,x))+M(ˇA(z(t,x))−ˉA)f(z(t,x)). | (3.7) |
Based on Assumption 2, we have
zT(t,x)MTM(ˇA(z(t,x))−ˉA)f(z(t,x))=N−1∑i=1yTi(t,x)(si(A(zi(t,x))−ˉA)f(zi(t,x))−si+1(A(zi+1(t,x))−ˉA)f(zi+1(t,x)))=N−1∑i=1n∑j=1yij(t,x)n∑s=1[(ajs(zis)−ˉajs)sifs(zis(t,x))−(ajs(zi+1,s)−ˉajs)si+1fs(zi+1,s(t,x))]≤N−1∑i=1n∑j=12n∑s=1(ˉajs−a_js)Υ|yij(t,x)|. | (3.8) |
Similarly, we get
zT(t,x)MTMˉAf(z(t,x))=zT(t,x)MTˉA1Mf(z(t,x))=N−1∑i=1(sizi(t,x)−si+1zi+1(t,x))TˉA(sif(zi(t,x))−si+1f(zi+1(t,x)))≤N−1∑i=1yTi(t,x)ˉAˉATyi(t,x)+N−1∑i=1yTi(t,x)F2yi(t,x)=zT(t,x)MTˉA1ˉAT1Mz(t,x)+zT(t,x)MTF2Mz(t,x). | (3.9) |
By Lemma 3, we get
zT(t,x)MTM(L⊗Γ)z(t,x)=zT(t,x)MT(M⊗In)(L⊗Γ)z(t,x)=zT(t,x)MT(ML⊗Γ)z(t,x)=zT(t,x)MT(HM⊗Γ)z(t,x)=zT(t,x)MT(H⊗Γ)(M⊗In)z(t,x)=zT(t,x)MTHMz(t,x), | (3.10) |
and
zT(t,x)MTMu(t,x)=−N−1∑i=1n∑j=1kjyij(t,x)sgn(yij(t,x))−˜ξzT(t,x)MTMz(t,x)−ψrTμ(t)zT(t,x)MTMz(t,x)=−N−1∑i=1n∑j=1kj|yij(t,x)|−(˜ξ+ψrTμ(t))zT(t,x)MTMz(t,x). | (3.11) |
From the condition in Theorem 1, we derive that
˙V(t)≤∫ΩzT(t,x)MT(−˜ξ−D∗−ˆC1+ˉA1ˉAT1+F2+σH)Mz(t,x)dx−2ψrTμ(t)V(t)≤−ϕV(t)−2ψrTμ(t)V(t). | (3.12) |
According to the definition of μ(t), defining Ω(t)=μr(t) yields
rTμ(t)=˙Ω(t)Ω(t),t∈[t0,tb). | (3.13) |
Multiplying Ω2ψ(t) on both sides of (3.12) yields
d[Ω2ψ(t)V(t)]dt≤−ϕ[Ω2ψ(t)V(t)]. | (3.14) |
Solving the above inequality yields
Ω2ψ(t)V(t)≤e−ϕ(t−t0)Ω2ψ(t0)V(t0). | (3.15) |
Then, we have
V(t)≤e−ϕ(t−t0)μ(t)−2rψμ(t0)2rψV(t0). | (3.16) |
Note that
μ(t0)2rψ=1,limt→t−bμ(t)−2rψ=0. | (3.17) |
which yields
limt→t−bV(t)=0. | (3.18) |
Hence, limt→t−b‖y(t,x)‖=0, and the systems can reach synchronization in prespecified time T.
Next, we prove that the synchronization can be maintained on [tb,+∞).
˙V(t)≤−(ϕ+2ψrT)V(t)=−ˆθV(t),t∈[tb,+∞). | (3.19) |
where ˆθ=ϕ+2ψrT>0.
Then, we can get
0≤V(t)≤V(tb)=0,t∈[tb,+∞). | (3.20) |
So, we obtain that V(t)≡0 over [tb,+∞). Hence, ‖y(t,x)‖≡0 on [tb,+∞). Based on Definition 3, leaderless PTBS for Systems (2.6) can be realized with controller (3.1). The proof is complete.
Remark 3. Different from [33,34,35], the competitive relationship between network nodes is considered in this paper, which is described by negative edges in the signed graph. It can be seen that the prescribed-time synchronization in the above papers is a special case of the bipartite prescribed-time synchronization in this work. On the other hand, compared with the asymptotic bipartite synchronization criteria in [19,20], our approach ensures that the CRDMNNs can reach bipartite synchronization within prespecified time tb, which is independent of initial values and control parameters.
Remark 4. Since there are switching parameters in the memristor system, the first term of controller (3.1) is designed to remove the influence of the memristor parameters. Then, the terms −ξpzp(t,x) and −ψrTμ(t)zp(t,x) are devoted to realizing the convergence in a prespecified time. The function μ(t) plays an important role to ensure the prespecified-time synchronization. It can be seen from (3.17) that μ(t0)2rψ=1,limt→t−bμ(t)−2rψ=0. In practical applications, there may exist a leader in the community. In this case, leader-following synchronization is a meaningful issue to be considered.
Next, we aim to investigate leader-following PTBS of CRDMNNs. Considering the following system with coupling delays:
∂zp(t,x)∂t=DΔzp(t,x)−Czp(t,x)+A(zp(t,x))f(zp(t,x))+σ∑q∈Np|Gpq|Γ(sgn(Gpq)zq(t−τ,x)−zp(t−τ,x))+up(t,x). | (3.21) |
The reference target system is:
∂z0(t,x)∂t=DΔz0(t,x)−Cz0(t,x)+A(z0(t,x))f(z0(t,x)). | (3.22) |
where z0(t,x)=(z01(t,x),z02(t,x),⋯,z0n(t,x))T∈Rn is the leader node.
Definition 4. For the CRDMNN System (3.21), it is said that the network can reach leader-following PTBS, if there exists a prespecified constant T, which is independent of any initial values or control parameters, such that
limt→T−‖zp(t,x)−spz0(t,x)‖=0,‖zp(t,x)−spz0(t,x)‖≡0,∀t>T, |
where sp=1 if p∈P1, and sp=−1 if p∈P2.
Define synchronization error δp(t,x)=zp(t,x)−spz0(t,x), and since ∑Nq=1lpqsq=0, we have
σ∑q∈Np|Gpq|Γ(sgn(Gpq)zq(t−τ,x)−zp(t−τ,x))=σN∑q=1lpqΓ(zq(t−τ,x)−sqz0(t−τ,x)+sqz0(t−τ,x))=σ∑q∈Np|Gpq|Γ(sgn(Gpq)δq(t−τ,x)−δp(t−τ,x)) | (3.23) |
Then, the error system can be obtained:
∂δp(t,x)∂t=DΔδp(t,x)−Cδp(t,x)+A(zp(t,x))f(zp(t,x))−spA(z0(t,x))f(z0(t,x))+σN∑q=1lpqΓδq(t−τ,x)+up(t,x). | (3.24) |
Define the norm
‖δp(t,x)‖2=(∫ΩδTp(t,x)δp(t,x)dx)12 |
The prespecified-time controller up(t,x) is designed as
up={−Ksgn(δp(t,x))−ξpδp(t,x)−2ψrTμ(t)δp(t,x)−ϕδp(t,x)‖δp(t,x)‖22∫tt−τδTp(θ,x)δp(θ,x)dθ−2ψrTμ(t)δp(t,x)‖δp(t,x)‖22∫tt−τδTp(θ,x)δp(θ,x)dθ,ifδp(t,x)≠0,0,otherwise, | (3.25) |
where K=diag(k1,k2,⋯,kn) with ki>0, ξp,ϕ,ψ>0 are the control gains. sgn(δp(t,x))=(sgn(δp1(t,x)),⋯,sgn(δpn(t,x)))T∈Rn. T, r and μ(t) are defined the same as in controller (3.1).
Theorem 2. Under Assumptions 1 and 2, suppose the following conditions hold:
ki>2n∑s=1a+isΥ,i=1,2,⋯,n,−˜ξ−cmin−ϑ∑k=1π2dmin(hMk−hmk)2+σ2λmax[(L⊗Γ)2]≤−ϕ2 |
where ϕ>0 is given in controller (3.25), ˜ξ=minp{ξp}, cmin=min{c1,c2,⋯,cn}, and dmin=min{d1,d2,⋯,dn}. Then, leader-following PTBS for Systems (3.21) and (3.22) can be achieved under controller (3.25).
Proof. Design the Lyapunov functional
V(t)=12N∑p=1∫ΩδTp(t,x)δp(t,x)dx+∫Ω∫tt−τδT(θ,x)δ(θ,x)dθdx. |
Computing the derivative along (3.24), we get
dV(t)dt=N∑p=1∫ΩδTp(t,x)˙δp(t,x)dx+∫ΩδT(t,x)δ(t,x)dx−∫ΩδT(t−τ,x)δ(t−τ,x)dx=N∑p=1∫ΩδTp(t,x)(−Cδp(t,x)+DΔδp(t,x)+A(zp(t,x))f(zp(t,x))−spA(z0(t,x))f(z0(t,x))+σN∑q=1lpqΓδq(t−τ,x)+up(t,x))dx+∫ΩδT(t,x)δ(t,x)dx−∫ΩδT(t−τ,x)δ(t−τ,x)dx. | (3.26) |
Based on Lemma 2, we get
∫ΩδTp(t,x)DΔδp(t,x)dx≤−ϑ∑k=1π2(hMk−hmk)2∫ΩδTp(t,x)Dδp(t,x)dx≤−ϑ∑k=1π2dmin(hMk−hmk)2∫ΩδTp(t,x)δp(t,x)dx. | (3.27) |
According to Assumption 2, we have
N∑p=1δTp(t,x)(A(zp)f(zp(t,x))−spA(z0)f(z0(t,x)))=N∑p=1n∑i=1δpi(t,x)n∑s=1(ais(zps)fs(zps(t,x))−spais(z0s)fs(z0s(t,x)))≤N∑p=1n∑i=12n∑s=1a+isΥ|δpi(t,x)|. | (3.28) |
For the coupling terms, it can be yielded that
σN∑p=1δTp(t,x)N∑q=1lpqΓδq(t−τ,x)=σδT(t,x)(L⊗Γ)δ(t−τ,x)≤δT(t−τ,x)δ(t−τ,x)+σ2δT(t,x)(L⊗Γ)2δ(t,x)≤δT(t−τ,x)δ(t−τ,x)+σ2λmax[(L⊗Γ)2]δT(t,x)δ(t,x) | (3.29) |
For the control term (3.25), we get
N∑p=1δTp(t,x)up(t,x)=N∑p=1δTp(t,x)(−Ksgn(δp(t,x))−ξpδp(t,x)−ψrTμ(t)δp(t,x)−ϕδp(t,x)‖δp(t,x)‖22|∫tt−τδTp(θ,x)δp(θ,x)dθ|−2ψrTμ(t)δp(t,x)‖δp(t,x)‖22|∫tt−τδTp(θ,x))δp(θ,x)dθ|)=−N∑p=1n∑i=1ki|δpi(t,x)|−N∑p=1ξpδTp(t,x)δp(t,x)−N∑p=1ϕ∫tt−τδTp(θ,x)δp(θ,x)dθ−2ψrTμ(t)N∑p=112δTp(t,x)δp(t,x)−2ψrTμ(t)N∑p=1∫tt−τδTp(θ,x)δp(θ,x)dθ. | (3.30) |
By combining the above inequalities, one has
dV(t)dt=∫ΩδT(t,x)(−˜ξ−cmin−ϑ∑k=1π2dmin(hMk−hmk)2+λmax[(L⊗Γ)2])δ(t,x)dx−ϕN∑p=1∫Ω∫tt−τδTp(θ,x))δp(θ,x)dθdx−2ψrTμ(t)⋅12N∑p=1∫ΩδTp(t,x)δp(t,x)dx−2ψrTμ(t)N∑p=1∫Ω∫tt−τδTp(θ,x)δp(θ,x)dθdx≤−ϕV(t)−2ψrTμ(t)V(t). | (3.31) |
Following the discussion in Theorem 1, we derive limt→t−b‖zp(t,x)−spz0(t,x)‖2=0 and ‖zp(t,x)−spz0(t,x)‖2≡0 on [tb,+∞). Thus, leader-following PTBS for Systems (3.21) and (3.22) can be realized with controller (3.25).
Remark 5. Different from [12,13,14], the competitive relationship and coupling delay are considered in this paper, which can better modify the real network model. Due to the existence of antagonistic interactions and coupling delays, the traditional synchronization methods in [12,13,14] cannot be directly used to realize PTBS. Moreover, the convergence speed is required to be prescribed instead of asymptotic in this work, and thus the Theorem in our work greatly generalizes the previous results on bipartite synchronization.
Remark 6. In Reference [27], by applying nonsmooth analysis and some novel inequality techniques in the complex field, several nonseparation method-based fixed-time synchronization criteria are derived. In Reference [30], the FXTS of dynamic systems are reconsidered in this article based on special functions from the view of improving the estimate accuracy for settling time and reducing the chattering caused by the sign function. In this paper, the convergence time is prescribed in advance according to actual requirements, and thus our criteria are less conservative and more flexible.
To verify our theorem, two simulation examples are proposed in this section. We consider a network with 3 nodes, and since the structural balance condition is satisfied, the node set V can be separated into V1={1,2} and V2={3}. Then, one has s1=s2=1 and s3=−1. We first consider Theorem 1.
Example 1. Choose the CRDMNNs with 2 neurons as below:
∂zp(t,x)∂t=DΔzp(t,x)−Czp(t,x)+A(zp(t,x))f(zp(t,x))+σ∑q∈Np|Gpq|Γ(sgn(Gpq)zq(t,x)−zp(t,x))+up(t,x) | (4.1) |
where zp(t,x)=(zp1(t,x),zp2(t,x))T, D=diag(1,1),C=diag(2,2), and Ω={x|−1≤x≤1}. The memristive matrix A(zp(t,x)) switches between ˇA and ˆA, where
ˆA=[0.40.10.10.1],ˇA=[0.1−0.1−0.1−0.1] | (4.2) |
The activation functions are chosen as
fp(x)=0.5(|x+1|−|x−1|). | (4.3) |
It can be checked that F=0.5I2 and Υ=0.5. Hence, the conditions in Assumption 2 are satisfied. Choose Γ=I2 and σ=1. The Laplacian matrix is
L=[2−1−102−11−11]. |
The controller is
up(t,x)=−KspN−1∑j=psgn(sjzj(t,x)−sj+1zj+1(t,x))−ξpzp(t,x)−ψrTμ(t)zp(t,x). | (4.4) |
Choose feedback matrix K=0.6I2, ξp=0.5,ψ=1.5,r=2, ϕ=1. Let t0=0, and set the prespecified time tb=2. It can be checked that
kj≥22∑s=1(ˉajs−a_js)Υ,j=1,2−˜ξ+λmax(−D∗−ˆC1+ˉA1ˉAT1+F2+σH)≤−ϕ2 |
Thus, the conditions in Theorem 1 are satisfied. Choosing initial values in [−3,3], Figures 1 and 2 depict the evolutions of states zp1(t,x) and zp2(t,x). It can be seen that system (4.1) can reach leaderless PTBS in prespecified time tb under control law (4.4).
Next, we consider the leader-following PTBS in Theorem 2.
Example 2. Consider the following 2-node CRDMNN with coupling delays:
∂zp(t,x)∂t=DΔzp(t,x)−Czp(t,x)+A(zp(t,x))f(zp(t,x))+σ∑q∈Np|Gpq|Γ(sgn(Gpq)zq(t−τ,x)−zp(t−τ,x))+up(t,x) | (4.5) |
where τ=0.5. Other parameters are the same as in Example 1. The desired reference target is
∂z0(t,x)∂t=DΔz0(t,x)−Cz0(t,x)+A(z0(t,x))f(z0(t,x)). | (4.6) |
Let εp(t,x)=zp(t,x)−spz0(t,x), and the error system is
∂εp(t,x)∂t=DΔεp(t,x)−Cεp(t,x)+A(zp(t,x))f(zp(t,x))−spA(z0(t,x))f(z0(t,x))+σN∑q=1lpqΓεq(t−τ,x)+up(t,x). | (4.7) |
The prespecified-time controller up(t,x) is chosen as (3.25). Choose ki=2,ξp=2. Other parameters are the same as in Example 1. It can be checked that
ki>22∑s=1a+isΥ,−˜ξ−cmin−ϑ∑k=1π2dmin(hMk−hmk)2+σ2λmax[(L⊗Γ)2]≤−ϕ2 |
Thus, the condition in Theorem 2 holds, and the leader-following PTBS is achieved. Take initial random conditions in the interval [−3,3], and Figure 3 describes the synchronization error εp(t,x) between system (4.5) and (4.6) with controller (3.25). From the simulations, leader-following synchronization can be achieved in prescribed time tb=2.
This paper investigates the prespecified-time bipartite synchronization (PTBS) of CRDMNNs with both cooperative and competitive interactions. Two types of PTBS are considered in our work: leaderless PTBS and leader-following PTBS. In addition, the coupling delays are considered in the leader-following case. By designing suitable Lyapunov functionals and novel control protocols, two criteria are derived for leaderless PTBS and leader-following PTBS based on a structural balance condition. Compared with FTS and FxTS, the settling time in our theorem can be predetermined according to the task, which is independent of the initial values and control parameters.
Our future study will focus on 1) PTBS control of neural networks under time-scale and 2) PTBS control of quaternion-valued neural networks.
This work is supported by the Natural Science Foundation of Jiangsu Province (Grant No. BK20210635).
The authors have no conflict of interest.
[1] | Aghenta LO and Iqbal MT (2019) Design and Dynamic Modelling of a Hybrid Power System for a House in Nigeria. Int J Photoenergy 2019: 1-13. |
[2] | IEC White Paper (2019) Electrical Energy Storage. Available from: https://www.iec.ch/whitepaper/pdf/iecWP-energystorage-LR-en.pdf. |
[3] |
Lee J, Lee S, Cho H, et al. (2018) Supervisory Control and Data Acquisition for Standalone Hybrid Power Generation Systems. Sustainable Computing: Informatics and Systems 20: 141-154. doi: 10.1016/j.suscom.2017.11.003
![]() |
[4] | Stouffer K, Falco J and Kent K (2011) Guide to Supervisory Control and Data Acquisition (SCADA) and Industrial Control Systems Security-Recommendations of the National Institute of Standards and Technology. Special Publication 800-82. |
[5] |
Jiao D and Sun J (2018) Real-Time Visualization of Geo-Sensor Data Based on the ProtocolCoupling Symbol Construction Method. ISPRS Int J Geo-Inf 7: 460. doi: 10.3390/ijgi7120460
![]() |
[6] | Lu X (2014) Supervisory Control and Data Acquisition System Design for CO2 Enhanced Oil Recovery. Master of Engineering Thesis, Technical Report No. UCB/EECS-2014-123. EECS Department, University of California at Berkeley. |
[7] |
Sajid A, Abbas H and Saleem K (2016) Cloud-Assisted IoT-Based SCADA Systems Security: A Review of the State of the Art and Future Challenges. IEEE Access 4: 1375-1384. doi: 10.1109/ACCESS.2016.2549047
![]() |
[8] |
Al-Fuqaha A, Guizani M, Mohammadi M, et al. (2015) Internet of Things: A Survey on Enabling Technologies, Protocols, and Applications. IEEE Commun Surv Tut 17: 2347-2376. doi: 10.1109/COMST.2015.2444095
![]() |
[9] | Sethi P and Sarangi SR (2017) Internet of Things: Architectures, Protocols, and Applications. Journal of Electrical and Computer Engineering 2017: 1-25. |
[10] | Nicola M, Nicola C, Duta M, et al. (2018) SCADA Systems Architecture Based on OPC and Web Servers and Integration of Applications for Industrial Process Control. International Journal of Control Science and Engineering 8: 13-21. |
[11] | Alavi SA, Rahimian A, Mehran K, et al. (2018) An IoT-Based Data Collection Platform for Situational Awareness-Centric Microgrids. 2018 IEEE Canadian Conference on Electrical and Computer Engineering (CCECE) 1-4. |
[12] | Kao K, Chieng W and Jeng S (2018) Design and development of an IoT-based web application for an intelligent remote SCADA system. 2018 IOP Conference Series: Materials Science and Engineering 323. |
[13] | Li W, Wang J, Yen C, et al. (2018) Cloud supervisory control system based on JustIoT. 2018 IEEE International Conference on Smart Manufacturing, Industrial and Logistics Engineering (SMILE) 17-20. |
[14] | Sarierao BS and Prakasarao A (2018) Smart Healthcare Monitoring System Using MQTT Protocol. 2018 3rd International Conference for Convergence in Technology (I2CT) 1-5. |
[15] |
Wu F, Wu T and Yuce M (2018) An Internet-of-Things (IoT) Network System for Connected Safety and Health Monitoring Applications. Sensors 19: 21. doi: 10.3390/s19010021
![]() |
[16] | Yi D, Binwen F, Xiaoming K, et al. (2016) Design and implementation of mobile health monitoring system based on MQTT protocol. 2016 IEEE Advanced Information Management, Communicates, Electronic and Automation Control Conference (IMCEC) 1679-1682. |
[17] | Kodali RK and Soratkal S (2016) MQTT based home automation system using ESP8266. 2016 IEEE Region 10 Humanitarian Technology Conference (R10-HTC) 1-5. |
[18] |
Bassoli M, Bianchi V and Munari I (2018) A Plug and Play IoT Wi-Fi Smart Home System for Human Monitoring. Electronics 7: 200. doi: 10.3390/electronics7090200
![]() |
[19] |
Chang CY, Kuo CH, Chen JC, et al. (2015) Design and Implementation of an IoT Access Point for Smart Home. Applied Sciences 5: 1882-1903. doi: 10.3390/app5041882
![]() |
[20] |
Lee Y, Hsiao W, Huang C, et al. (2016) An integrated cloud-based smart home management system with community hierarchy. IEEE T Consum Electr 62: 1-9. doi: 10.1109/TCE.2016.7448556
![]() |
[21] | Pirbhulal S, Zhang H, Alahi ME, et al. (2017) Erratum: Sandeep P., et al. A Novel Secure IoTBased Smart Home Automation System Using a Wireless Sensor Network. Sensors 17: 69. |
[22] | Sahadevan A, Mathew D, Mookathana J, et al. (2017) An Offline Online Strategy for IoT Using MQTT. 2017 IEEE 4th International Conference on Cyber Security and Cloud Computing (CSCloud) 369-373. |
[23] | Mishra B (2018) TMCAS: An MQTT based Collision Avoidance System for Railway networks. 2018 18th International Conference on Computational Science and Applications (ICCSA) 1-6. |
[24] | Kodali RK (2016) An implementation of MQTT using CC3200. 2016 International Conference on Control, Instrumentation, Communication and Computational Technologies (ICCICCT) 582-587. |
[25] | Dow C, Cheng S and Hwang S (2016) A MQTT-based guide and notification service system. 2016 IEEE 7th Annual Information Technology, Electronics and Mobile Communication Conference (IEMCON) 1-4. |
[26] | Bryce R, Shaw T and Srivastava G (2018) MQTT-G: A Publish/Subscribe Protocol with Geolocation. 2018 41st International Conference on Telecommunications and Signal Processing (TSP) 1-4. |
[27] | Dhar P and Gupta P (2016) Intelligent parking Cloud services based on IoT using MQTT protocol. 2016 International Conference on Automatic Control and Dynamic Optimization Techniques (ICACDOT) 30-34. |
[28] | Muladi M, Sendari S and Widiyaningtyas T (2018) Outdoor Air Quality Monitor Using MQTT Protocol on Smart Campus Network. 2018 International Conference on Sustainable Information Engineering and Technology (SIET) 216-219. |
[29] | Atmoko RA and Yang D (2018) Online Monitoring and Controlling Industrial Arm Robot Using MQTT Protocol. 2018 IEEE International Conference on Robotics, Biomimetics, and Intelligent Computational Systems (Robionetics) 12-16. |
[30] | De Paolis LT, De Luca V and Paiano R (2018) Sensor data collection and analytics with thingsboard and spark streaming. 2018 IEEE Workshop on Environmental, Energy, and Structural Monitoring Systems (EESMS) 1-6. |
[31] |
Pesch A and Scavelli P (2019) Condition Monitoring of Active Magnetic Bearings on the Internet of Things. Actuators 8: 17. doi: 10.3390/act8010017
![]() |
[32] |
Reaves B and Morris T (2012) An open virtual testbed for industrial control system security research. Int J Inf Secur 11: 215-229. doi: 10.1007/s10207-012-0164-7
![]() |
[33] |
Hadžiosmanović D, Bolzoni D and Hartel PH (2012) A log mining approach for process monitoring in SCADA. Int J Inf Secur 11: 231-251. doi: 10.1007/s10207-012-0163-8
![]() |
[34] | Unique Automation Portfolio. Available from: https://new.siemens.com/ca/en/products/automation. |
[35] |
Sultana T and Wahid KA (2019) Choice of Application Layer Protocols for Next Generation Video Surveillance Using Internet of Video Things. IEEE Access 7: 41607-41624. doi: 10.1109/ACCESS.2019.2907525
![]() |
[36] |
Moustafa N, Turnbull B and Choo KR (2019) An Ensemble Intrusion Detection Technique Based on Proposed Statistical Flow Features for Protecting Network Traffic of Internet of Things. IEEE Internet of Things Journal 6: 4815-4830. doi: 10.1109/JIOT.2018.2871719
![]() |
[37] |
Babovic ZB, Protic J and Milutinovic V (2016) Web Performance Evaluation for Internet of Things Applications. IEEE Access 4: 6974-6992. doi: 10.1109/ACCESS.2016.2615181
![]() |
[38] | Ismail AA, Hamza HS and Kotb AM (2018) Performance Evaluation of Open Source IoT Platforms. 2018 IEEE Global Conference on Internet of Things (GCIoT) 1-5. |
[39] | ThingsBoard API Reference. Available from: https://thingsboard.io/docs/reference/mqtt-api/. |
[40] | Nuratch S (2018) Applying the MQTT Protocol on Embedded System for Smart Sensors/Actuators and IoT Applications. 2018 15th International Conference on Electrical Engineering/Electronics, Computer, Telecommunications and Information Technology (ECTI-CON) 628-631. |
[41] | Aghenta LO and Iqbal MT (2019) Low-Cost, Open Source IoT-Based SCADA System Design Using Thinger.IO and ESP32 Thing. Electronics 8: 822. |
[42] | ESP32 TTGO. Available from: http://esp32-ttgo.blogspot.com. |
[43] |
Zhong X and Liang Y (2016) Raspberry Pi: An Effective Vehicle in Teaching the Internet of Things in Computer Science and Engineering. Electronics 5: 56. doi: 10.3390/electronics5030056
![]() |
[44] | ThingsBoard Documentation. Available from: https://thingsboard.io/docs/. |
[45] | Nasr PM and Yazdian-Varjani A (2018) Toward Operator Access Management in SCADA System: Deontological Threat Mitigation. IEEE T Ind Inform 14: 3314-3324. |
1. | Peng Liu, Ting Liu, Junwei Sun, Zhigang Zeng, Predefined-Time Synchronization of Multiple Fuzzy Recurrent Neural Networks via a New Scaling Function, 2024, 32, 1063-6706, 1527, 10.1109/TFUZZ.2023.3327682 | |
2. | Kai Wu, Ming Tang, Han Ren, Liang Zhao, Quantized pinning bipartite synchronization of fractional-order coupled reaction–diffusion neural networks with time-varying delays, 2023, 174, 09600779, 113907, 10.1016/j.chaos.2023.113907 | |
3. | Pan Wang, Xuechen Li, Qianqian Zheng, Synchronization of inertial complex-valued memristor-based neural networks with time-varying delays, 2024, 21, 1551-0018, 3319, 10.3934/mbe.2024147 | |
4. | Mengjiao Wang, Mingyu An, Shaobo He, Xinan Zhang, Herbert Ho-Ching Iu, Zhijun Li, Two-dimensional memristive hyperchaotic maps with different coupling frames and its hardware implementation, 2023, 33, 1054-1500, 10.1063/5.0154516 | |
5. | Ting Yuan, Huizhen Qu, Dong Pan, Random periodic oscillations and global mean-square exponential stability of discrete-space and discrete-time stochastic competitive neural networks with Dirichlet boundary condition, 2023, 45, 10641246, 3729, 10.3233/JIFS-230821 | |
6. | Lisha Yan, Xia Huang, Zhen Wang, Min Xiao, Bipartite synchronization of coopetitive MNNs subject to communication delays via dynamic switching event-triggered control, 2025, 0924-090X, 10.1007/s11071-025-10966-y | |
7. | Yanli Huang, Wenjing Duan, Tse Chiu Wong, 2024, Robust General Decay Synchronization of State and Spatial Diffusion Coupled Reaction-diffusion Memristive Neural Networks, 979-8-3315-0475-5, 616, 10.1109/CSIS-IAC63491.2024.10919282 |