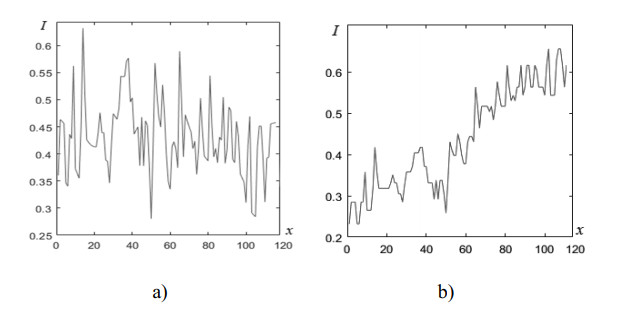
Citation: Gavin C Rider. A critique of the approach to controlling electrostatic risk in semiconductor production and identification of a potential risk from the use of equipotential bonding[J]. AIMS Electronics and Electrical Engineering, 2019, 3(4): 397-414. doi: 10.3934/ElectrEng.2019.4.397
[1] | Jin H. Jo, Zachary Rose, Jamie Cross, Evan Daebel, Andrew Verderber, John C. Kostelnick . Application of Airborne LiDAR Data and Geographic Information Systems (GIS) to Develop a Distributed Generation System for the Town of Normal, IL. AIMS Energy, 2015, 3(2): 173-183. doi: 10.3934/energy.2015.2.173 |
[2] | Chris Thankan, August Winters, Jin Ho Jo, Matt Aldeman . Feasibility of applying Illinois Solar for All (ILSFA) to the Bloomington Normal Water Reclamation District. AIMS Energy, 2021, 9(1): 117-137. doi: 10.3934/energy.2021007 |
[3] | Jin H. Jo, Kadi Ilves, Tyler Barth, Ellen Leszczynski . Implementation of a large-scale solar photovoltaic system at a higher education institution in Illinois, USA. AIMS Energy, 2017, 5(1): 54-62. doi: 10.3934/energy.2017.1.54 |
[4] | Peter Majewski, Rong Deng, Pablo R Dias, Megan Jones . Product stewardship considerations for solar photovoltaic panels. AIMS Energy, 2023, 11(1): 140-155. doi: 10.3934/energy.2023008 |
[5] | Rashmi Murali, Sangeeta Malhotra, Debajit Palit, Krishnapada Sasmal . Socio-technical assessment of solar photovoltaic systems implemented for rural electrification in selected villages of Sundarbans region of India. AIMS Energy, 2015, 3(4): 612-634. doi: 10.3934/energy.2015.4.612 |
[6] | Jin H. Jo, Kadi Ilves, Tyler Barth, Ellen Leszczynski . Correction: Implementation of a large-scale solar photovoltaic system at a higher education institution in Illinois, USA. AIMS Energy, 2017, 5(2): 313-315. doi: 10.3934/energy.2017.2.313 |
[7] | Arben Gjukaj, Rexhep Shaqiri, Qamil Kabashi, Vezir Rexhepi . Renewable energy integration and distributed generation in Kosovo: Challenges and solutions for enhanced energy quality. AIMS Energy, 2024, 12(3): 686-705. doi: 10.3934/energy.2024032 |
[8] | Virendra Sharma, Lata Gidwani . Recognition of disturbances in hybrid power system interfaced with battery energy storage system using combined features of Stockwell transform and Hilbert transform. AIMS Energy, 2019, 7(5): 671-687. doi: 10.3934/energy.2019.5.671 |
[9] | Abdulrahman Th. Mohammad, Amer Ibrahim Ismael . An equivalent photovoltaic solar system to solve the problems of electricity in Iraqi houses. AIMS Energy, 2019, 7(5): 660-670. doi: 10.3934/energy.2019.5.660 |
[10] | Fadhil Khadoum Alhousni, Firas Basim Ismail, Paul C. Okonkwo, Hassan Mohamed, Bright O. Okonkwo, Omar A. Al-Shahri . A review of PV solar energy system operations and applications in Dhofar Oman. AIMS Energy, 2022, 10(4): 858-884. doi: 10.3934/energy.2022039 |
In one of the oldest branches of medical science, i.e., dermatology exists a number of diseases in which early diagnosis is very important, and a significant part of humanity suffers from such diseases. For example, psoriasis affects about 3–4% of the population of our planet [1], and new cases of melanoma in the United States of America are registered to be more than 80 thousand per year [2]. A diagnosis at the early stage can either increase the likelihood of being cured (for melanoma) or reduce the likelihood of serious complications (for psoriasis). The severity of the disease allows us to assess quantitative measurements. For example, Psoriasis Area and Severity index (PASI) is often used by specialists in the clinical practice [3,4]. However, its calculation is a time-consuming process, with a high probability of subjective estimates. Therefore, the medical diagnostic systems in dermatology take an important role, increasing the efficiency and reliability of diagnosis.
The intelligent DRVI [5,6,7] is an important part of these systems above. The basic processes of medical diagnostic systems in dermatology are segmentation and identification. The purpose of segmentation is to reduce the amount of data processed for identification to determine the vector of features. These procedures should provide the quality of classification, the reliability of diagnostic solutions and the efficiency of image processing in DRVIs [8,9,10].
In segmentation, the data are combined to form segments according to one or more features, such as color, intensity and texture [11]. Texture segmentation is quite difficult in the diagnosis of such diseases as psoriasis, eczema and atopic dermatitis [4,8,9]. The quality of texture segmentation affects the reliability of diagnostic solutions in general.
For the implementation of the texture segmentation procedure in DRVI detection, classification methods are most used [12,13]. Detection methods include the following processes: the assessment of the sign of segmentation, the emphasis of the border between homogeneous areas in the image and the threshold and morphological processing of potential boundaries for homogeneous textural areas. Using detection methods, the segmentation is performed on the basis of one feature. This feature is converted into intensity and taken into account when determining the boundaries of homogeneous texture areas by using methods of contour segmentation. The advantage of detection methods is the simplicity of implementation and high efficiency of image processing. However, these methods are characterized by low noise immunity.
When using classification methods, the segmentation feature(s) is (are) assessed, the feature vectors are classified, the boundary points of homogeneous areas are selected and the boundaries are processed. Segmentation via classification methods is carried out according to several criteria. These methods allow segmentation to be applied to a wider class of images and textures [11]. The quality of the segmentation is determined by coincidence of borders for actually selected segmented homogeneous texture area and the pre-marked one. An advantage of classification methods is the high quality of segmentation. The disadvantages are the low efficiency of image processing and the need for a large training sample to ensure reliable classification.
The selection of the particular segmentation method depends on the type of texture model. The known types of textures are statistical and spectral. A distinctive feature of spectral texture models is that they can be described as a quasi-periodic signal. Statistical texture models assess the presence or absence of the spatial interaction between non-derivative textural elements. However, when diagnosing the characteristics of a number of dermatological diseases, such as the degree of psoriasis, a combined spectral-statistical type of texture can be used [14,15,16]. In this case, the psoriatic plaque looks like a red spot that is covered with large silvery-white scales and paraffin flakes. For these types of textures, employing detection and classification methods in texture segmentation does not provide the quality of segmentation necessary for practical use.
Selecting the type of texture model usually determines the approach for finding the features of the texture for subsequent identification. Statistical texture models make it possible to determine the moments and features of images for different ranges; for example, R, G, B, and/or features obtained on their basis (entropy, contrast, homogeneity, etc.) [17] often use the matrices of coincidences or energy characteristics [18]. Spectral texture models represent texture in terms of spatial frequency and allow spectral descriptors to be determined using Fourier spectra, the amplitude spectrum of which differs for textures according to the frequency characteristics and some statistical descriptors [19]; for example, the autocorrelation function can supplement the vector of features. The disadvantages of these approaches are that they require an additional investigation to select the calculation parameters and a sufficient set of features invariant to shifting and rotation, which is a characteristic feature for the application of noise levels.
Today, to determine the severity of psoriasis, doctors usually use one of the many known indices (PASI, BSA (Body Surface Area), etc.) [6,8]. The most popular among them is the PASI [3,6]. A significant number of these indices need the diseased areas to be determined. The calculation of the PASI takes into account the numerical ratio and area of the relevant parts of the human body, as well as the assessment of psoriasis signs, which are determined by a dermatologist and include erythema, peeling and infiltration. However, the PASI is characterized by a lack of nonlinearity. This disadvantage is especially significant with low PASI values, i.e., when determining whether a patient has the disease at all. A reliable answer to this question is especially important when diagnosing. This should be facilitated using the approach to determine the geometric moments and features [20], which are invariant to the parallel transfer and rotation angle of the object (i.e., scaling-resistant).
The analysis of the domain above shows that the existing medical diagnostic systems in dermatology do not provide the necessary reliability and/or efficiency. In addition, the efficiency of processing and the reliability of diagnostic solutions are largely determined by the quality of the segmentation of the medical and biological images to be processed. A significant number of such images are of combined spectral-statistical nature.
Among the most significant shortcomings of existing segmentation methods, we consider the following ones: the low quality of detection methods and the need for a large training sample to ensure the quality of classification methods for hybrid types of textures. Existing identification methods introduce errors into the calculation of the known indices when determining the degree of psoriasis.
Therefore, the authors aimed to increase the quality of segmentation by 1) improving the models of spectral-statistical textures, 2) developing an improved method for the segmentation of spectral-statistical textures and 3) designing the procedure for determining the vector of features to ensure sufficient quality classification, the reliability of the diagnostic solutions and the efficiency of image processing in DRVIs. In particular, it is expected to take into account the values of statistical and spectral textural features and determine the geometric moments and features on the basis of the results of calculating the characteristic points of the contours during the formation of the vector of features; at the stage of contour segmentation, apply the method using wavelets obtained by transforming the power graph.
As mentioned above, the texture of dermatological disease images can be of spectral, statistical or combined spectral-statistical nature [14,15,16]. For such types of textures, an improved vector-difference method of texture segmentation has been developed. It is based on idea of determining the modulus of the difference of vectors, the elements of which are the values of textural features, to convert the image of the texture into intensity. To assess the quality of segmentation, the Pratt's criterion [21,22] is used to compare the boundaries of perfectly and actually segmented textured images:
R=(1/I)∑IAi=11/(1+αd2i), | (1) |
where I = max(Ii, Ip); Ii, Ip—the number of points on the boundary of the segments of perfectly and actually segmented images, respectively; α—scaling constant, usually set to 1/9; di—the distance between the boundary point of the actually segmented image and the line that includes the points of ideal boundary of the segment.
The improved vector-difference method for texture segmentation includes the stages of image scanning using the processing window, identification, vector-difference transformation and contour segmentation. At the stage of identification, the primary identification vector is built.
The calculation of the values for the textural features is performed in the sliding window whose size is chosen according to the purpose of the processing task. This paper proposes the development of the method given in [21]. When performing the identification, the identification vector of features j for each i-th pixel of the image in the processing aperture →ci(c1,i,c2,i,…,cn,i) is received, where j=¯1,n and n is a number of features.
The construction of the identification vector involves two stages:
1) Calculating the difference of vectors in the (i+1)-th and i-th pixels of the image, i.e., determining the difference between →ci+1(c1,i+1,c2,i+1,…,cn,i+1) and →ci(c1,i,c2,i,…,cn,i).
2) Calculating the modulus of the difference between two vectors →ci+1(c1,i+1,c2,i+1,…,cn,i+1) and →ci(c1,i,c2,i,…,cn,i) using methods of vector algebra, as follows:
→c=|→ci+1(c1,i+1,c2,i+1,…,cn,i+1)−→ci(c1,i,c2,i,…,cn,i)|= | (2) |
=(→c1,i+1−c1,i,c2,i+1−c2,i,…,cn,i+1−cn,i). |
Then, the techniques of the contour segmentation are used [15,16].
Since the proposed method has features of both detection and classification approaches and the latter requires a representative training sample for its formation, a previously developed spectral-statistical texture model [21] was improved; it is described by the expression (5) below.
When modeling the spectral texture, the spatial-frequency properties of the variation of the intensity of the image pixels are taken into account [23]. Spectral texture models are characterized by the value of the background component and the change in spatial frequency. Then, the value of the intensity of the m-th row of the spectral textured image is represented as a combination of segments that do not intersect, as follows:
I(x,ym)=∪ki=1{ci(x,ym)+∑nj=1Aij(x,ym)sin(ωijmx)},x∈[qi−1,qi], | (3) |
where Aij(x,ym), ωijm—the amplitude and frequency of the j-th modulated oscillation on the i-th segment of the m-th image; ci(x,ym)—a representation of the background on the i-th segment for the m-th row of the image; q=(q0,...,qk+1)—a vector of boundaries of textured areas for the m-th row of the image when q0 = 1 and qk+1 = N + 1, where N is the number of pixels in the image row; x = 1, …, N; y = 1, …, M, where M represents the spatial coordinates.
The statistical texture models take into account the values of the background component and indicators of variation for the random field. The intensity of the m-th row for the image of the statistical texture is represented as a combination of segments that do not intersect, as follows:
I(x,ym)=∪ki=1{ci(x,ym)+Ni(x,ym)},x∈[qi−1,qi], | (4) |
where Ni(x,ym)—Gaussian noise with zero mean and variance σ2i on the i-th segment of the image.
The combined spectral-statistical texture model is characterized by the background component value, the random change in spatial frequency and random field variations. The intensity of the m-th row for the spectral-statistical model of texture is represented as follows:
I(x,ym)=∪ki=1{ci(x,ym)+Ni(x,ym)+∑nj=1Aij(x,ym)sin(ωijmx)},x∈[qi−1,qi], | (5) |
where Ni(x,ym)—Gaussian noise with zero mean and variance σ2i on the i-th segment of the image.
Taking into account the above given mathematical model of textures, to construct the image of the spectral-statistical texture, the method for identification of the spectral-statistical texture is proposed, whereby an identification vector is constructed; it is a combination of spectral and statistical textural features.
It is proposed to define each element of the vector for the statistical textural features as follows:
1) The processing aperture is formed for each pixel of an image of size M. The size of the processing aperture is selected depending on the purpose of the processing task.
2) In each row of the image matrix in the processing aperture for each pixel, the calculation of the average pixel intensity is performed as follows:
¯I=∑Mi=1 IiM. | (6) |
3) The statistical variance estimate is defined for each processing aperture per each pixel by applying the following quadratic-amplitude transformation:
¯σ=∑Mi=1 (Ii−¯I)2M, | (7) |
where Ii>¯I, i=¯1,M.
The following algorithm can define each element of the feature vector for the spectral texture:
1) A processing aperture of size M is formed for each pixel of the image. The size of the processing aperture is chosen depending on the purpose of the processing task.
2) A direct Fourier transform is performed in each row of the image matrix in the processing aperture of each image pixel, as follows:
ˆI(ω)=1M∑M−1xi=1I(xi,ym)e−j2πωxi/M, | (8) |
where ω=¯0,M−1.
3) A linear frequency conversion is carried out for each processing aperture of each pixel: z(ω)=k⋅ˆI(ω), and, as a result, the spectral composition of the texture changes to scattered.
4) The inverse Fourier transform is performed I(xi,ym)=∑M−1ω=1z(ω)ej2πωxi/M, xi=¯0,M−1.
5) The statistical estimate of the numerical characteristics of the scatter for each image pixel in the processing aperture is calculated according to (7).
The results of calculating the spectral texture of the psoriasis image are shown in Figure 1.
According to (2), the spectral-statistical textural feature →σr was calculated as the modulus of the difference of two vectors, with the preliminary normalization of the values of the statistical →σi and spectral →σi+1 textures being performed according to their maximum values:
→σi=→σi→σi,max,→σi+1=→σi+1→σi+1, max, | (9) |
where →σi,max and →σi+1,max—the maximum values of the variation features of the statistical and spectral textures, respectively.
During the study, a sample of 30 model images of spectral-statistical textures was constructed according to (5). The size of the images was 512 × 512, and the pixel intensity of the images varied from 0 to 255. Each model image contained two areas with different frequencies ω1 and ω2 (period/degree), and the amplitude A = 1; the random change of amplitude and frequency occurred due to the superimposition of the Gaussian noise with a zero mean and the varying Gaussian interference for different image segments. The reliability of the classification method for homogeneous areas of test images with different levels of interference under the conditions of identifying spectral and statistical textures (concurrently and separately) based on the confusion matrix was estimated (Table 1). It was performed using the TPR metrics, which measure the probability for the correct detection of texture pixels, and the FPR metrics that measure the reliability of the erroneous assignment of pixels to the spectral-statistical texture area if they do not really belong to this area (i.e., the probability of the false alarm) (Table 2).
Test image markup | q | Image markup on the basis of spectral and statistical textures, % | Image markup on the basis of spectral texture, % | Image markup on the basis of statistical texture, % | |||
Area 1 | Area 2 | Area 1 | Area 2 | Area 1 | Area 2 | ||
Area 1 | 1 | 54.14 | 45.86 | 89.42 | 10.58 | 69.37 | 30.63 |
Area 2 | 36.54 | 63.46 | 72.29 | 27.70 | 34.05 | 65.95 | |
Area 1 | 2 | 52.76 | 47.24 | 96.21 | 3.79 | 72.36 | 27.64 |
Area 2 | 36.91 | 63.09 | 77.12 | 22.88 | 35.09 | 64.90 | |
Area 1 | 5 | 71.89 | 28.10 | 93.77 | 6.23 | 67.29 | 32.71 |
Area 2 | 39.62 | 60.39 | 60.78 | 39.22 | 34.87 | 65.13 | |
Area 1 | 10 | 95.39 | 4.60 | 80.74 | 19.26 | 80.74 | 42.92 |
Area 2 | 35.74 | 64.26 | 38.14 | 61.86 | 39.82 | 60.18 | |
Area 1 | 20 | 95.05 | 4.95 | 81.44 | 18.56 | 40.18 | 59.82 |
Area 2 | 19.48 | 80.52 | 28.27 | 71.73 | 47.07 | 52.93 | |
Area 1 | 50 | 93.82 | 6.18 | 83.69 | 16.30 | 18.07 | 81.93 |
Area 2 | 12.38 | 87.61 | 15.42 | 84.58 | 51.09 | 48.91 | |
Area 1 | 100 | 94.54 | 5.46 | 87.35 | 12.65 | 15.31 | 84.69 |
Area 2 | 1.95 | 98.05 | 11.46 | 88.54 | 53.36 | 46.64 |
Signal-to-noise ratio | On the basis of spectral and statistical textures | On the basis of spectral texture | On the basis of statistical texture |
1 | 0.59 | 0.56 | 0.68 |
2 | 0.58 | 0.55 | 0.67 |
5 | 0.64 | 0.60 | 0.65 |
10 | 0.73 | 0.67 | 0.66 |
20 | 0.83 | 0.74 | 0.46 |
50 | 0.88 | 0.84 | 0.26 |
100 | 0.97 | 0.88 | 0.22 |
The results of comparative analysis are shown in Table 2 for the identification on the basis of the spectral and statistical texture; the authors confirmed the following outcomes. The probability for the correct detection of the spectral-statistical texture (TPR) was not less than 1.6 times more than one based on the spectral texture, and it was not less than six times more than one based on the statistical texture at a signal-to-noise ratio equal to 5 or more. The probability of the false alarm (FPR) was less than 0.4 when the signal-to-noise ratio was less than 5, and it was not more than 0.06 when the signal-to-noise ratio was more than 5.
Based on [21] and the proposed method for the identification of spectral-statistical textures, the improved vector-difference method of spectral-statistical texture was developed. To implement this method, contour segmentation was applied using wavelets obtained by transforming the graph of the power function, as they are characterized by a low error associated with determining the coordinates of intensity difference points for the underlying transformation [24].
The improved segmentation method for spectral-statistical textures includes the following stages:
1) Calculating the feature values of the statistical texture c1 (numerical characteristics of statistical texture scatter) and spectral textural features c2 (numerical characteristic of spectral texture scatter) for the i-th pixel of the image in the processing aperture.
2) Generating the identification vector of features for each i-th pixel of the image in processing aperture →ci(c1,i,c2,i) in the feature space.
3) Calculating the difference between the vectors in the (i+1)-th and i-th pixels of the image, i.e., calculating the difference between the following vectors (2): →ci+1(c1,i+1,c2,i+1) and →ci(c1,i,c2,i).
4) Calculating the modulus of the difference between two vectors →ci+1(c1,i+1,c2,i+1) and →ci(c1,i,c2,i).
5) Contour segmentation using wavelets obtained by transforming the graph of the power function [24].
An experimental study of the improved vector-difference segmentation method was carried out; the stage of contour segmentation wavelets obtained by transforming the graph of the power function [21] were used. For the segmentation of the test images of the spectral-statistical texture (Figure 2) at different signal-to-noise ratios, the improved method was applied at the stage of the contour segmentation using wavelets obtained by transforming the graph of power function (s<1) and employing the Canny method. Figure 2 shows the results of applying the improved method and the original method [21] to the test image at a signal-to-noise ratio of 20.
To determine the quality of segmentation with different values of the signal-to-noise ratio, the Pratt's criterion (1) was used and the graphs for the dependence of the Pratt's criterion indicator on the signal-to-noise ratio for the improved method and original method [21] were constructed (Figure 3). It was found that, at the signal-to-noise ratio q > 5, the improved method for texture segmentation using wavelets obtained by transforming the graph of the power function outperformed the segmentation quality of the original method [21] by 1.1–1.2 times.
The segmentation modulus of the spectral-statistical textures based on the improved vector-difference segmentation method for DRVIs in medical diagnostic systems was developed (Figure 4).
According to the block diagram (Figure 4), the input image of the recognition object (Figure 5) enters the pre-processing block. In this block, a homomorphic filter is used to remove the multiplicative noise that occurs due to the uneven illumination of the object when the image is received. The processed image enters the texture-type identification block, where the type of texture is identified using the method of texture identification based on multifractal indicators and spectral textural features [26]. The resulting texture image enters the classification block for the texture type, where homogeneous image fragments are assigned to the corresponding texture class.
The developed segmentation modulus of spectral-statistical textures was applied for the segmentation of psoriatic disease images in DRVIs of automated medical diagnostic systems. In Figure 5, images of psoriasis on the skin are shown, and they can be attributed to different types of textures: spectral-statistical a), statistical b) and spectral c); a selected rectangular fragment of the image that was used to calculate the features is shown.
The results of classifying the psoriatic skin disease texture images in the feature space of the two main components a1 and a2 are presented in Figure 6 for different types of textures: spectral-statistical a), statistical b) and spectral c).
Table 3 shows example images of psoriatic skin disease with expert markup, and the image obtained by applying the improved vector-difference method of texture segmentation.
Input image | Input image with expert markup | Result of segmentation with expert markup | Result of segmentation after contour selection |
![]() |
![]() |
![]() |
![]() |
The testing results show that the probability of correctly detecting the disease area in the images of psoriatic skin disease was, on average, 0.96, with a probability of a false alarm of 0.05, which is better than the original method [21].
Further, at the stage of identification, the vector of features can be supplemented. The initial data of this stage are the result of tracing the border of the textured area [24] (in this paper-psoriatic spots). It is necessary to determine the features of the shape of textured areas, which are highlighted at the segmentation stage. Currently, the healthcare professional has to manually estimate the perimeter and the area of the psoriatic spot to calculate the indices for further diagnosis. Since there can be many such spots (depending on the degree of disease), an automated system requires both high efficiency and precise calculations.
When diagnosing psoriasis, the vector of features is subject to the requirements of noise immunity, invariance to scaling, shifting in the field of view and rotation, and sufficient computational efficiency, quality and efficiency.
Such possibilities are provided owing to the identification method, which is based on the geometric moments of features (GMFs) [27]:
mαβ=∫Lx0∫Ly0I(x,y)xαyβdxdy, | (10) |
where I(x,y)—the image being processed; Lx, Ly—geometric dimensions of the image; α, β—orders of the moment.
The GMF method has a number of advantages. It is characterized by high noise immunity when smoothing the features of moments as integral characteristics. The ensemble of features can be increased to achieve stable recognition.
For recognition, the features, which are based on the GMFs and invariance to scale and rotation, are defined. To do this, the GMFs are calculated in the polar coordinate system:
μp=∫Rmax0∫2π0ρpI(ρ,ϕ)dρdϕ, | (11) |
where I(ρ,ϕ)—image brightness function in the polar coordinate system; ρ,ϕ—polar coordinates of the pixels relative to the center of the object weight; p—order of the moment; Rmax—distance from the center of the figure weight (psoriatic spots) to the most distant point.
In addition, the information on characteristic points [28], was obtained by performing a Lebesgue integration [29]:
μp=∬AρpQ(ρ,ϕ)ψ(x∩A), | (12) |
where А is the set of real numbers; Х is the subset of the coordinates of the characteristic points in the polar coordinate system; ψ—measure of a set of real numbers; Q—set of characteristic points for the contour.
Because the Lebesgue measure in the polar coordinate system is the area of a triangle [28] when the number of characteristic points is large, it is considered that sin(Δϕi)≈Δϕi, and the expression for characteristic points becomes as follows:
Cp=|∑Ji=0ρ(p+2)i0Δϕi|. | (13) |
In this paper, the characteristic points are the points of the contour inflection. Since spots with a complex shape can have many such points, an areal method of finding the extrema using the Haar wavelet transform was applied [30]. This method aims to find the extremes of multi-modal objective functions with high noise. Unlike common methods for finding the extrema of such functions (zero, first, second order), the method not only increases the probability of determining the global extremum, but it also improves the performance of such a search. Moreover, the method improves the performance of the procedure for determining the characteristic points of the contour [31], as well as the performance of the identification procedure and diagnosis in dermatological DRVIs. This method was used for the first time to solve the problem of identification when determining the signs of psoriatic spots. Compared with existing methods based on the first derivative [24], this enhances the noise immunity of the identification procedure and reduces the sensitivity to minor local irregularities in the contour of psoriatic spots (i.e., it reduces the number of characteristic points) due to the smoothing properties of the wavelet transform [32,33]. At the identification stage, this also reduced the variance in the cluster of traits.
Experimental research confirmed that the computational performance of the identification procedure is improved by 1.2 times. The quality of the identification procedure, based on variance, was improved by 1.3 times for the studied set of images.
This paper presents a significant extension of the method in [21]; this includes the following:
1) A method for the identification of spectral-statistical textures has been proposed; the importance of statistical and spectral texture features is taken into account to increase the probability of the correct detection of the spectral-statistical texture relative to the identification based on the spectral or statistical texture.
2) The model of spectral-statistical textures, as characterized by the value of the background component, random change of spatial frequency and indicators of random field variation, has been improved. In the proposed model, the random change of spatial frequency and field variation are carried out by adding Gaussian noise with a zero mean and variance to the corresponding segment of the image.
3) The improved vector-difference method for spectral-statistical textures was developed for application based on the wavelets at the stage of contour segmentation, which are characterized by low error during the determination of the coordinates of intensity differences; this increases the reliability of homogeneous textured areas.
4) A segmentation modulus of spectral-statistical textures for medical psoriasis images in DRVIs has been developed. The method of identification is implemented in the blocks for identification and classification of the texture type [26].
5) It is proposed to use the geometric moments and features of psoriatic spots at the identification stage after calculating them on the basis of determining the characteristic points of their contour, and by applying Lebesgue integration with a search for the characteristic points using the areal method [30].
The main advantages of the proposed approach as compared to the method in [21] are as follows: (i) the features are invariant to parallel transfers because the zero of the polar coordinate system is combined with the center of gravity of the figure; (ii) features are resistant to scaling because the radius vectors of characteristic points are normalized; (iii) the features are invariant to the angle of rotation of the object; (iv) invariance is provided as a result of summing the results obtained by traversing the contour; high noise immunity of the method is provided by taking into account the smoothing properties of the moments and employing an areal method to search the characteristic points described above; (v) the ensemble of features is easily built up for stable recognition; and (vi) the high computational efficiency of the method due to the small number of processed characteristic points.
We should note that the problem of identifying psoriatic spots is related to the significant number of features. They are as follows: (i) a large number of variants of the shapes of these spots (perimeter, area) that depend on the damage degree; (ii) the characteristics of methods based on the wavelet transform, which is required to increase the efficiency for the significant number of studies regarding the length of the wavelet function carrier (For example, in the issue of identification, it is influenced by the variability of spot shapes.); (iii) it is interesting in this regard to be able to determine the specified parameters of the wavelet function for different coefficients, which is a common technique for determining the severity of the disease for medical professionals.
Taking into account the above, we plan to explore those features in the further research.
The analysis of the subject area showed that existing textural segmentation methods do not provide the quality necessary for the practice of segmentation for combined spectral-statistical textures. Therefore, the model of spectral-statistical texture has been improved, the method for the identification of spectral-statistical textures has been developed and the method of vector-difference segmentation for computer-based diagnostic systems in dermatology has been improved. It has been proven that the developed methods provide high-quality texture segmentation and high processing performance compared to the classification methods. The analysis of the classification reliability for homogeneous areas of spectral-statistical textures confirmed the following outcomes. When identifying based on spectral and statistical textures, the probability of correctly detecting the spectral-statistical texture is 1.6 times higher than that based on the spectral texture, and six times higher than that based on the statistical texture at a signal-to-noise ratio of 5 or more. The probability of a false alarm is less than 0.4 when the signal-to-noise ratio is less than 5, and not more than 0.06 when the signal-to-noise ratio is more than 5. The test results confirmed that the probability of the correct detection of the disease area in images of psoriatic skin disease is, on average, 0.96, and the probability of a false alarm is 0.05, which are sufficient for the needs of practice. The proposed procedure for determining the vector of features for the purpose of image recognition in dermatologic diagnostic systems, which is based on processing the characteristic points, reduces the amount of processed data required for sufficient performance and quality.
The conducted research makes it possible to recommend the developed method to be used for investigating psoriatic disease and in adjacent areas of medical diagnostics.
In future research, the authors are going to 1) identify the most informative features based on the characteristic points for different degrees and types of psoriasis, 2) investigate the properties and 3) determine the parameters of the areal method with wavelet transform to solve the problem of segmentation for different degrees of disease.
The work on this paper is funded by the National Natural Science Foundation of China under Grant No. 61772180, and the Key R & D plan of Hubei Province (2020BHB004, 2020BAB012).
The authors declare that the research was conducted in the absence of any commercial or financial relationships that could be construed as a potential conflict of interest.
[1] | Rider G (2003) Reticle Electrostatic Protection Seminar, International Sematech. Available from: https://www.researchgate.net/publication/327228305_Sematech_ESD_Symposium_Field_Simulation_Presentation |
[2] | Bellmore D (2002) Controlling ESD In Automated Handling Equipment. Electrical Overstress/Electrostatic Discharge Symposium 226-235. |
[3] | Bruner J (2002) Design of Semiconductor Manufacturing Equipment for Electrostatic Compatibility. Sematech ESD Symposium. |
[4] | Mechanical specification for a 150 mm reticle SMIF pod (RSP150) used to transport and store a 6 inch reticle. SEMI Standard E111. Available from: https://www.semi.org/en/collaborate/standards |
[5] |
Montoya J, Levit L, Englisch A (2001) A Study of the Mechanisms for ESD Damage to Reticles. IEEE T Electron Pack 24: 78-85. doi: 10.1109/6104.930957
![]() |
[6] | Levit L, Weil G (2001) Reticle boxes, ESD Control and Electrostatic Shielding. Sematech ESD Impact and Control Workshop. |
[7] | Chubb J (2000) Measuring The Shielding Performance Of Materials. IEEE Industry Applications Conference. |
[8] | Rider G (2003) EFM: A Pernicious New Electric Field Induced Damage Mechanism in Reticles. Sematech ESD Symposium. Available from: https://www.researchgate.net/profile/Gavin_Rider/contributions |
[9] | Rider G (2003) Quantification of the risk of field induced damage to reticles. Sematech ESD Symposium. Available from: https://www.researchgate.net/profile/Gavin_Rider/contributions |
[10] |
Rudack AC, Pendley M, Gagnon P, et al. (2003) Induced ESD Damage on Photomasks: A Reticle Evaluation. 23rd Annual BACUS Symposium on Photomask Technology 5256: 1136-1142. doi: 10.1117/12.518126
![]() |
[11] | Helmholz D, Lering M (2006) Reticle Carrier Material as ESD Protection. Photomask Technology 6349: 634952. |
[12] | Rider G, Kalkur T (2008) Experimental quantification of reticle electrostatic damage below the threshold for ESD. Metrology, Inspection, and Process Control for Microlithography XXII 6922: 69221Y. |
[13] | Rider G (2008) Electric field-induced progressive CD degradation in reticles. Photomask Technology 7122: 71220G. |
[14] |
Ben-Zvi G, Dmitriev V, Graitzer E, et al. (2008) Very High Sensitivity Mask Transmittance Mapping and Measurements Based on Non Imaging Optics with Galileo. Photomask and Next-Generation Lithography Mask Technology XV 7028:702828. doi: 10.1117/12.798462
![]() |
[15] | Ben-Zvi G, private communication of data produced by Brian Grenon Consultants. |
[16] | Rider G (2008) How to protect reticles from electrostatic damage. SPIE Digital Library, November 2018. |
[17] | Montoya J, Levit L, Englisch A (2000) A Study of the Mechanisms for ESD Damage to Reticles. Sematech ESD Symposium. |
[18] | Sebald T, Rider G (2009) High sensitivity electric field monitoring system for control of field-induced CD degradation in reticles (EFM). Photomask and Next-Generation Lithography Mask Technology XVI 7379: 73791P. |
[19] |
Labovitz S, Ben-Zvi G, Dmitriev V, et al. (2008) Detection of Progressive Transmission Loss Due to Haze with Galileo Mask DUV Transmittance Mapping Based on Non Imaging Optics. Photomask Technology 7122: 712216. doi: 10.1117/12.801558
![]() |
[20] | Sengupta D, Pavlidis D (2003) Control of semiconductor epitaxy by application of an external field. CFD Research Corp Huntsville AL. |
[21] |
Derenyi I, Lee C, Barabasi AL (1998) Ratchet Effect in Surface Electromigration: Smoothing Surfaces by an AC Field. Phys Rev Lett 80: 1473. doi: 10.1103/PhysRevLett.80.1473
![]() |
[22] |
Ratsch C, Scheffler M (1998) Density Functional Theory Calculations of Hopping Rates of Surface Diffusion. Phys Rev B 58: 13163. doi: 10.1103/PhysRevB.58.13163
![]() |
[23] |
Rudack A, Levit L, Williams A (2002) Mask Damage by Electrostatic Discharge: A Reticle Printability Evaluation. Optical Microlithography XV 4691: 1340-1347. doi: 10.1117/12.474516
![]() |
[24] |
Wallash A, Levit L (2003) Electrical breakdown and ESD phenomena for devices with nanometer-to-micron gaps. Reliability, Testing, and Characterization of MEMS/MOEMS li 4980: 87-96. doi: 10.1117/12.478191
![]() |
[25] | Diaz CH (1994) Automation of Electrical Overstress Characterization for Semiconductor Devices. Hewlett Packard Journal 45: 106. |
[26] | Azizi N, Yiannacouras P (2003) Gate Oxide Breakdown. ECE1768 - Reliability of Integrated Circuits. |
[27] | Pey KL, Tung CH (2007) Physical characterization of breakdown in metal-oxide-semiconductor transistors. SPIE Newsroom 2-4. |
[28] |
Hsu DC, Wang MT, Lee J (2007) Electrical characteristics and reliability properties of metal-oxide-semiconductor field-effect transistors with ZrO2 gate dielectric. J Appl Phys 101: 094105. doi: 10.1063/1.2723861
![]() |
[29] | Shaw D (2017) Diffusion in Semiconductors. Springer Handbook of Electronic and Photonic Materials. |
[30] | Sonnenfeld G, Goebel K, Celaya J (2008) An Agile Accelerated Aging, Characterization and Scenario Simulation System for Gate Controlled Power Transistors. IEEE Autotestcon 208-215. |
[31] | Guide for the Handling of Reticles and Other Extremely Electrostatic Sensitive (EES) Items Within Specially Designated Areas. SEMI Standard E163-0212. Available from: http://ams.semi.org/ebusiness/standards/semistandard.asp |
Test image markup | q | Image markup on the basis of spectral and statistical textures, % | Image markup on the basis of spectral texture, % | Image markup on the basis of statistical texture, % | |||
Area 1 | Area 2 | Area 1 | Area 2 | Area 1 | Area 2 | ||
Area 1 | 1 | 54.14 | 45.86 | 89.42 | 10.58 | 69.37 | 30.63 |
Area 2 | 36.54 | 63.46 | 72.29 | 27.70 | 34.05 | 65.95 | |
Area 1 | 2 | 52.76 | 47.24 | 96.21 | 3.79 | 72.36 | 27.64 |
Area 2 | 36.91 | 63.09 | 77.12 | 22.88 | 35.09 | 64.90 | |
Area 1 | 5 | 71.89 | 28.10 | 93.77 | 6.23 | 67.29 | 32.71 |
Area 2 | 39.62 | 60.39 | 60.78 | 39.22 | 34.87 | 65.13 | |
Area 1 | 10 | 95.39 | 4.60 | 80.74 | 19.26 | 80.74 | 42.92 |
Area 2 | 35.74 | 64.26 | 38.14 | 61.86 | 39.82 | 60.18 | |
Area 1 | 20 | 95.05 | 4.95 | 81.44 | 18.56 | 40.18 | 59.82 |
Area 2 | 19.48 | 80.52 | 28.27 | 71.73 | 47.07 | 52.93 | |
Area 1 | 50 | 93.82 | 6.18 | 83.69 | 16.30 | 18.07 | 81.93 |
Area 2 | 12.38 | 87.61 | 15.42 | 84.58 | 51.09 | 48.91 | |
Area 1 | 100 | 94.54 | 5.46 | 87.35 | 12.65 | 15.31 | 84.69 |
Area 2 | 1.95 | 98.05 | 11.46 | 88.54 | 53.36 | 46.64 |
Signal-to-noise ratio | On the basis of spectral and statistical textures | On the basis of spectral texture | On the basis of statistical texture |
1 | 0.59 | 0.56 | 0.68 |
2 | 0.58 | 0.55 | 0.67 |
5 | 0.64 | 0.60 | 0.65 |
10 | 0.73 | 0.67 | 0.66 |
20 | 0.83 | 0.74 | 0.46 |
50 | 0.88 | 0.84 | 0.26 |
100 | 0.97 | 0.88 | 0.22 |
Input image | Input image with expert markup | Result of segmentation with expert markup | Result of segmentation after contour selection |
![]() |
![]() |
![]() |
![]() |
Test image markup | q | Image markup on the basis of spectral and statistical textures, % | Image markup on the basis of spectral texture, % | Image markup on the basis of statistical texture, % | |||
Area 1 | Area 2 | Area 1 | Area 2 | Area 1 | Area 2 | ||
Area 1 | 1 | 54.14 | 45.86 | 89.42 | 10.58 | 69.37 | 30.63 |
Area 2 | 36.54 | 63.46 | 72.29 | 27.70 | 34.05 | 65.95 | |
Area 1 | 2 | 52.76 | 47.24 | 96.21 | 3.79 | 72.36 | 27.64 |
Area 2 | 36.91 | 63.09 | 77.12 | 22.88 | 35.09 | 64.90 | |
Area 1 | 5 | 71.89 | 28.10 | 93.77 | 6.23 | 67.29 | 32.71 |
Area 2 | 39.62 | 60.39 | 60.78 | 39.22 | 34.87 | 65.13 | |
Area 1 | 10 | 95.39 | 4.60 | 80.74 | 19.26 | 80.74 | 42.92 |
Area 2 | 35.74 | 64.26 | 38.14 | 61.86 | 39.82 | 60.18 | |
Area 1 | 20 | 95.05 | 4.95 | 81.44 | 18.56 | 40.18 | 59.82 |
Area 2 | 19.48 | 80.52 | 28.27 | 71.73 | 47.07 | 52.93 | |
Area 1 | 50 | 93.82 | 6.18 | 83.69 | 16.30 | 18.07 | 81.93 |
Area 2 | 12.38 | 87.61 | 15.42 | 84.58 | 51.09 | 48.91 | |
Area 1 | 100 | 94.54 | 5.46 | 87.35 | 12.65 | 15.31 | 84.69 |
Area 2 | 1.95 | 98.05 | 11.46 | 88.54 | 53.36 | 46.64 |
Signal-to-noise ratio | On the basis of spectral and statistical textures | On the basis of spectral texture | On the basis of statistical texture |
1 | 0.59 | 0.56 | 0.68 |
2 | 0.58 | 0.55 | 0.67 |
5 | 0.64 | 0.60 | 0.65 |
10 | 0.73 | 0.67 | 0.66 |
20 | 0.83 | 0.74 | 0.46 |
50 | 0.88 | 0.84 | 0.26 |
100 | 0.97 | 0.88 | 0.22 |
Input image | Input image with expert markup | Result of segmentation with expert markup | Result of segmentation after contour selection |
![]() |
![]() |
![]() |
![]() |