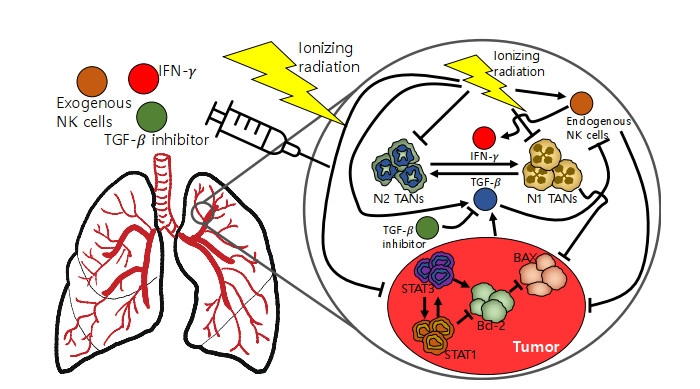
Neutrophils play a crucial role in the innate immune response as a first line of defense in many diseases, including cancer. Tumor-associated neutrophils (TANs) can either promote or inhibit tumor growth in various steps of cancer progression via mutual interactions with cancer cells in a complex tumor microenvironment (TME). In this study, we developed and analyzed mathematical models to investigate the role of natural killer cells (NK cells) and the dynamic transition between N1 and N2 TAN phenotypes in killing cancer cells through key signaling networks and how adjuvant therapy with radiation can be used in combination to increase anti-tumor efficacy. We examined the complex immune-tumor dynamics among N1/N2 TANs, NK cells, and tumor cells, communicating through key extracellular mediators (Transforming growth factor (TGF-β), Interferon gamma (IFN-γ)) and intracellular regulation in the apoptosis signaling network. We developed several tumor prevention strategies to eradicate tumors, including combination (IFN-γ, exogenous NK, TGF-β inhibitor) therapy and optimally-controlled ionizing radiation in a complex TME. Using this model, we investigated the fundamental mechanism of radiation-induced changes in the TME and the impact of internal and external immune composition on the tumor cell fate and their response to different treatment schedules.
Citation: Donggu Lee, Sunju Oh, Sean Lawler, Yangjin Kim. Bistable dynamics of TAN-NK cells in tumor growth and control of radiotherapy-induced neutropenia in lung cancer treatment[J]. Mathematical Biosciences and Engineering, 2025, 22(4): 744-809. doi: 10.3934/mbe.2025028
[1] | Hsiu-Chuan Wei . Mathematical modeling of tumor growth: the MCF-7 breast cancer cell line. Mathematical Biosciences and Engineering, 2019, 16(6): 6512-6535. doi: 10.3934/mbe.2019325 |
[2] | Erin N. Bodine, K. Lars Monia . A proton therapy model using discrete difference equations with an example of treating hepatocellular carcinoma. Mathematical Biosciences and Engineering, 2017, 14(4): 881-899. doi: 10.3934/mbe.2017047 |
[3] | H. J. Alsakaji, F. A. Rihan, K. Udhayakumar, F. El Ktaibi . Stochastic tumor-immune interaction model with external treatments and time delays: An optimal control problem. Mathematical Biosciences and Engineering, 2023, 20(11): 19270-19299. doi: 10.3934/mbe.2023852 |
[4] | Krzysztof Fujarewicz, Krzysztof Łakomiec . Adjoint sensitivity analysis of a tumor growth model and its application to spatiotemporal radiotherapy optimization. Mathematical Biosciences and Engineering, 2016, 13(6): 1131-1142. doi: 10.3934/mbe.2016034 |
[5] | Ghassen Haddad, Amira Kebir, Nadia Raissi, Amira Bouhali, Slimane Ben Miled . Optimal control model of tumor treatment in the context of cancer stem cell. Mathematical Biosciences and Engineering, 2022, 19(5): 4627-4642. doi: 10.3934/mbe.2022214 |
[6] | Craig Collins, K. Renee Fister, Bethany Key, Mary Williams . Blasting neuroblastoma using optimal control of chemotherapy. Mathematical Biosciences and Engineering, 2009, 6(3): 451-467. doi: 10.3934/mbe.2009.6.451 |
[7] | Samantha L Elliott, Emek Kose, Allison L Lewis, Anna E Steinfeld, Elizabeth A Zollinger . Modeling the stem cell hypothesis: Investigating the effects of cancer stem cells and TGF−β on tumor growth. Mathematical Biosciences and Engineering, 2019, 16(6): 7177-7194. doi: 10.3934/mbe.2019360 |
[8] | Mahya Mohammadi, M. Soltani, Cyrus Aghanajafi, Mohammad Kohandel . Investigation of the evolution of tumor-induced microvascular network under the inhibitory effect of anti-angiogenic factor, angiostatin: A mathematical study. Mathematical Biosciences and Engineering, 2023, 20(3): 5448-5480. doi: 10.3934/mbe.2023252 |
[9] | Andreas Wagner, Pirmin Schlicke, Marvin Fritz, Christina Kuttler, J. Tinsley Oden, Christian Schumann, Barbara Wohlmuth . A phase-field model for non-small cell lung cancer under the effects of immunotherapy. Mathematical Biosciences and Engineering, 2023, 20(10): 18670-18694. doi: 10.3934/mbe.2023828 |
[10] | Ge Song, Tianhai Tian, Xinan Zhang . A mathematical model of cell-mediated immune response to tumor. Mathematical Biosciences and Engineering, 2021, 18(1): 373-385. doi: 10.3934/mbe.2021020 |
Neutrophils play a crucial role in the innate immune response as a first line of defense in many diseases, including cancer. Tumor-associated neutrophils (TANs) can either promote or inhibit tumor growth in various steps of cancer progression via mutual interactions with cancer cells in a complex tumor microenvironment (TME). In this study, we developed and analyzed mathematical models to investigate the role of natural killer cells (NK cells) and the dynamic transition between N1 and N2 TAN phenotypes in killing cancer cells through key signaling networks and how adjuvant therapy with radiation can be used in combination to increase anti-tumor efficacy. We examined the complex immune-tumor dynamics among N1/N2 TANs, NK cells, and tumor cells, communicating through key extracellular mediators (Transforming growth factor (TGF-β), Interferon gamma (IFN-γ)) and intracellular regulation in the apoptosis signaling network. We developed several tumor prevention strategies to eradicate tumors, including combination (IFN-γ, exogenous NK, TGF-β inhibitor) therapy and optimally-controlled ionizing radiation in a complex TME. Using this model, we investigated the fundamental mechanism of radiation-induced changes in the TME and the impact of internal and external immune composition on the tumor cell fate and their response to different treatment schedules.
Lung cancer is the leading cause of cancer-related mortality worldwide (∼ 1.8 million (18%) deaths each year [1]). The most common type (∼85%) is non-small cell lung cancer (NSCLC) with common subtypes of lung squamous cell carcinoma (LUSC) and lung adenocarcinoma (LUAD) [2]. The tumor microenvironment (TME) includes various cell types such as immune cells (tumor-associated neutrophils (TANs), macrophages, T cells, natural killer cells) and stromal cells, extracellular matrix (ECM), and diffusible molecules such as cytokines, chemokines, and growth factors [3,4,5]. Certain types of immune cells in TME were reported to support tumor growth by suppressing immune activities [6] and exchanging tumor promoting factors such as angiogenic factors, ECM-degrading enzymes, and growth factors. It was shown that high degrees of tumor-infiltrating lymphocytes (TILs) in the large node-negative cancer tissue are positively associated with a decrease in disease recurrence and improved survival rates in NSCLC patients [4,7]. In particular, some of these cells show distinct functional characteristics in tumor-promoting or tumor-suppressing roles. For example, it is well established that tumor-associated macrophages (TAMs), termed M2 TAMs [6], promote tumor growth by secreting factors and cytokines [8,9]. Even though a classical form of neutrophils called N1 TANs present anti-tumor functions in the TME, increasing evidence now suggest that other neutrophils, called N2 TANs, can mediate aggressive tumor growth throughout the course of tumor progression toward malignancy [10,11,12,13,14,15]. For example, TANs infiltrate tumor tissue and enhance tumor growth and cancer cell invasion [16,17], leading to poor clinical outcomes [16,17] in many cancers, including renal carcinoma [18], metastatic melanoma [19], and bronchoalveolar carcinoma [17]. Clinically, the increased neutrophil to lymphocyte ratio (NLR) is positively correlated with poor prognosis in various cancers [20], including NSCLC [21], esophageal cancer [22], colon cancer [23,24], head and neck cancer [25], gastric cancer [26], hepatocellular carcinoma [27], bladder cancer [28], cervical cancer [29], or advanced pancreatic cancer [30]. The NLR is considered a potentially crucial biomarker for lung cancer patients [31]. Despite the significant potential for targeting these TANs [32], the detailed mechanism of this critical transition between those modes is poorly understood. Therefore, a better understanding of tumor-associated inflammation may help us identify novel therapeutic strategies [33].
The signal activator and transducer of transcription (STAT) protein family functions in a coordinated fashion to control key cellular processes such as cell division, cell growth, cell migration, and cell death [34,35,36]. The STAT family consists of seven members: STAT1, STAT2, STAT3, STAT4, STAT5 (STAT5A and STAT5B), and STAT6 [37]. Insufficient STAT1 signaling causes a defect in the response to external control modules in diseases such as cancers. For example, the lack of STAT1 promotes the migration and spread of lung cancer cells [37,38,39,40]. STAT3 plays an important role in a variety of biological functions, including inhibition of apoptosis, cell motility, and cell growth [37,38,41]. The relative expression of STAT1 and STAT3 may induce two cell fates: (ⅰ) An anti-apoptosis (down-regulation of STAT1 and up-regulation of STAT3) status and (ⅱ) an apoptosis (up-regulation of STAT1 and down-regulation of STAT3) status. Apoptosis is regulated by two key proteins downstream of STAT signaling, Bcl-2 and BAX, that inhibit or promote apoptosis, respectively [42,43,44]. The reduced DNA binding capability of STAT3 predates dynamic changes in the expression of anti-apoptotic Bcl-2 and pro-apoptotic BAX proteins (decreased Bcl-2 level and increased BAX levels) and induction of apoptosis [45]. How these two phenotypes are controlled in detail is largely unknown, and many experimental and clinical results suggest significant potential for therapeutic purposes.
Transforming Growth Factor-β (TGF-β) is a multifunctional cytokine that plays a critical role in the regulation of cell growth, differentiation, apoptosis, and immune function. TGF-β is involved in a wide range of biological processes, including embryonic development, wound healing, tissue repair, and immune responses [46,47,48]. TGF-β plays a complex role in various cancers, including lung cancer. Even though TGF-β acts as a tumor suppressor by inhibiting the growth and spread of cancer cells in an early stage of cancer progression, TGF-β can promote the growth and spread of cancer cells and facilitate tumor invasion and metastasis in later stages of the disease [49,50,51]. In lung cancer, TGF-β signaling pathways are often disrupted, which can result in the loss of the tumor suppressive effects of TGF-β and the acquisition of pro-tumorigenic functions [46,48]. TGF-β has also been implicated in promoting immune evasion in lung cancer. It can suppress the activity of immune cells, including T cells and natural killer (NK) cells, leading to reduced anti-tumor immune responses and metastasis of lung cancer [52,53]. TGF-β was also shown to skew neutrophil differentiation toward N2 TANs [15,54,55,56], and inhibition of TGF-β can reverse this effect [57,58]. Therefore, the development of TGF-β inhibitors as a potential therapeutic strategy in lung cancer is an active area of research.
Interferon gamma (IFN-γ) is a cytokine that plays an important role in the immune response to cancer. In lung cancer, IFN-γ has been shown to have both anti-tumor and pro-tumor effects, depending on the stage of the disease and the context of its action [59,60]. In lung cancer, IFN-γ can stimulate anti-tumor immune responses by activating immune cells, such as T cells and natural killer (NK) cells, which can directly kill cancer cells [61,62]. IFN-γ also enhances the expression of major histocompatibility complex (MHC) molecules on the surface of cancer cells, making them more visible to the immune system. Researchers [10,54,63] suggest that IFN-γ can stimulate the production and activation of N1 TANs in the TME, leading to enhanced anti-tumor immunity. In addition, IFN-γ has been shown to up-regulate the expression of chemokines that recruit N1 TANs to the tumor site [10,54,63]. Studies have also shown a positive correlation between IFN-γ and activation of STAT1 in lung cancer, leading to improved outcomes and increased sensitivity to chemotherapy and immunotherapy, and recruitment of N1 TANs [39,64,65].
NK cells are a type of lymphocyte that play an important role in the immune response to cancer. NK cells have been shown to have both anti-tumor and pro-tumor effects, depending on the stage of the disease and the context of their action in the tumor [52,66,67]. In lung cancer, NK cells are thought to play a protective role by recognizing and eliminating cancer cells. Studies have shown that higher levels of NK cell infiltration in lung tumors are associated with improved patient survival rates [68,69,70]. There is a positive correlation between NK cells and cytokines, particularly IFN-γ, which is a key activator of NK cell function [61,62]. IFN-γ can induce the expression of receptors on NK cells that recognize and kill cancer cells, as well as enhance their production of cytokines that stimulate other immune cells to target cancer [59,60]. TGF-β, on the other hand, has been shown to suppress the activity of NK cells, which can contribute to immune evasion and promote the aggressive growth and spread of cancer cells [71,72]. Thus, the relative balance between the effects of IFN-γ and TGF-β on NK cell activity can be an important factor in determining the outcome of the immune response to lung cancer. Exogenous NK cells administered to a patient as a form of immunotherapy are being studied as a potential treatment option for various cancers, including lung cancer [73,74,75,76]. In oncolytic virus therapy, injection of exogenous NK cells or depletion of NK cells in the TME were shown to improve tumor control compared to the control case where NK cells can decrease anti-tumor efficacy of OV-mediated cancer cell killing [74,76].
Radiation therapy (RT), one of the most common types of cancer treatment, uses high-energy X-rays to destroy cancer cells. While various types of RT such as 3D conformal radiation therapy, image guided radiation therapy (IGRT), intensity modulated radiation therapy (IMRT), and volumetric modulated arc therapy (VMAT) are used treat cancers, these may also affect normal healthy cells with different sensitivity [77,78]. In particular, ionizing radiation (IR) kills cancer cells by destroying double-stranded DNA of tumor cells [79,80]. Studies indicate a significant effect of IR on the dynamics of immune cells such as such as T cells, macrophages, dendritic cells, and NK cells [79]. In particular, IR induces the secretion of IFN-γ and tumor necrosis factor alpha (TNF-α) by NK cells through the ATM and NKκB pathway, resulting in anti-tumorigenic and apoptosis phenotypes [79]. IR-induced damage to tissues and cells is also shown to promote secretion of TGF-β by fibroblasts and N2 TANs [81,82,83], leading to up-regulation of N2 TANs [15].
The complex network of interactions between tumor and the immune system include NK cells, N2/N1 TANs, STAT signaling, and immune cytokines in response to radiation therapy (Figure 1). In this work, we employed the framework of the mathematical model to investigate the key role of NK cells and radiation in regulation of TAN transitions and the STAT signaling pathway in the TME. The mathematical model consists of a system of ordinary differential equations (ODEs) and partial differential equations (PDEs) in the extended model (Section 3.7) involving the following variables at time t:
C(t)=Density of cancer cellsN1(t)=Density of N1 TANsN2(t)=Density of N2 TANsKend(t)=Density of endogenous NK cellsKex(t)=Density of exogenous NK cellsG(t)=Concentration of TGF-βL(t)=Concentration of TGF-βinhibitorS(t)=Concentration of IFN-γS1(t)=Concentration of STAT1S3(t)=Concentration of STAT3B(t)=Concentration of Bcl-2X(t)=Concentration of BAX |
The mathematical model in this work is used (ⅰ) to investigate how NK cells regulate the critical balance between N1 and N2 TANs via TGF-β and immune molecules (IFN-γ and TGF-β inhibitor such as Galunisertib, which blocks TGF-β activity) in a complex interaction network, (ⅱ) to investigate how this perturbed N2-to-N1 ratio affect anti-tumor efficacy, (ⅲ) to investigate the role of intracellular signaling (STAT signaling) in regulation of the NK-controlling immune-tumor dynamics, (ⅳ) to identify the optimal schedule in a combination therapy (NK cells + IFN-γ + TGF-β inhibitor), and (ⅴ) to investigate how radiation therapy affect the overall complex nonlinear dynamics and to find the optimal dose schedule for the best outcomes in a clinic.
We developed a mathematical model based on a schematic diagram of key regulation of interaction among tumor cells, N1 TANs, N2 TANs, and NK cells in TME and intracellular STAT signaling within a tumor cell (Figure 1). The NK-controlled phenotypic balance between STAT signaling and N1/N2 TANs in the presence and absence of ionizing radiation regulates enhancement or suppression of tumor growth in response to TGF-β, IFN-γ, and TGF-β inhibitor.
To describe the time evolution of densities of N1 and N2 TANs, we took into account the transition from N1 TANs to N2 TANs via IL-6 and TGF-β [10,84], induction of N1 TANs in the presence of IFN-γ [10], natural decay processes of TANs, and the mutual inhibition between N1 and N2 TANs. The governing equations for densities of N1 and N2 TANs were modified from the model by Kim et al. [85]:
dN1dt=λS1S⏟source+k3k24k24+βN22⏟inhibition by N2−μN1N1⏟decay, | (2.1) |
dN2dt=λ6+λGG⏟source+k1k22k22+αN21⏟inhibition by N1−μN2N2⏟decay, | (2.2) |
where λ6 and λG are the source of N2 TANs from IL-6 and TGF-β, respectively, from the TME, λS1 is the source of N1 TANs from IFN-γ from the TME, α is the inhibition strength of N2 TANs by N1 TANs with scaling parameters (k1,k2), β is the inhibition strength of N1 TANs by N2 TANs with scaling parameters (k3,k4), and μN2 and μN1 are the decay rates of N2 TANs and N1 TANs, respectively. In this framework, the relative balance between IFN-γ and TGF-β determines either N1- or N2-dominant TME, which in turn either suppresses or promotes the tumor growth.
Different kinds of mathematical models of tumor growth were introduced in literature to reproduce empirical time series data of tumor size [86]: Exponential growth [87], logistic growth [88], Gompertz growth [89], and nonlinear models [90]. In particular, logistic growth patterns in the presence and absence of supportive and/or inhibitive factors were observed in the experimental setting [39,74,91,92,93,94] and used in the various mathematical models (ordinary or partial differential equations, stochastic, or multi-scale models) for successful fitting to experimental data [8,74,85,91,92,93,94,95,96,97,98,99,100,101,102,103,104].
The dynamics of the tumor cell density (C(t)) is governed by a production term (PC) and death processes (DC) as follows:
dCdt=PC⏟Production−DC⏟decay/death. | (2.3) |
We take the logistic growth model for proliferation of tumor cells,
PC=rC(1−CC0), | (2.4) |
where r is the proliferation rate of tumor cells and C0 is the carrying capacity of the tumor in a given TME (r,C0∈R+).
We assume that tumor cells are killed by immune cells and IFN-β-induced apoptosis. In the model, N1 TANs and NK cells (both endogenous and exogenous NK cells, when injected) eliminate the tumor cells from the TME. Bcl-2 and BAX are key players in regulating the apoptotic death of cancer cells in the presence of various biochemical stimuli such as IFN-β in the complex TME. As discussed below, the down-regulation of the gate keeper, Bcl-2 (B<thB) [42], and up-regulation of BAX (X>thX) [43,44] in response to high IFN-β levels lead to cell death of tumor cells [43,45,105,106]. These lead to the following cell death terms
DC=δ1N1C+δ2CI{B<thB,X>thX}+δ3C(Kend+Kex). | (2.5) |
Here, δ1 and δ3 are the killing rate of tumor cells by N1 TANs and NK cells, respectively, δ2 is the elimination rate of tumor cells by the IFN-β-mediated apoptosis (STAT-Bcl-2-BAX signaling pathway), and I[⋅] is an indicator function (giving 1 or 0 based on the intracellular conditions on the tumor cell {(B,X)∈R2:B<thB,X>thX}).
Combining these proliferation/death terms lead to the governing equation for the cancer cell density:
dCdt=rC(1−CC0)⏟source−δ1N1C⏟killing by N1−δ3C(Kend+Kex)⏟killing by NKs−δ2CI{B<thB,X>thX}⏟apoptosis. | (2.6) |
Among several STAT family members [37], the relative balance between STAT1 and STAT3 plays a key role in regulation of various biological functions such as apoptotic status of given cell [37,38,41]. For instance, down-regulation of STAT1 and up-regulation of STAT3 lead to a stronger anti-apoptotic mode by maintaining the on-switch of the gate keeper, Bcl-2 [37,38,41], and can increase the invasive potential and aggressive spreading of lung cancer cells [37,38,39,40]. The mutual antagonism between STAT1 and STAT3 can reverse the gate keeping of the cell-death program. IFN-γ, the key inducer of the N2→N1 TAN transition [10,11,15], induces the apoptosis in malignant cancer cells [105] by upregulating STAT1 and down-regulating the STAT3. For instance, the reduced DNA binding capability of STAT3 predates dynamic changes in the expression of anti-apoptotic Bcl-2 and pro-apoptotic BAX proteins, inducing decreased Bcl-2 levels and increased BAX levels followed by apoptosis [45]. Thus, we assume that (ⅰ) IFN-γ induce STAT1 at a rate λS2 but inhibit STAT3 at a rate λS3 with scaling parameters (λ1,K1), (ⅱ) all intracellular molecules, STAT1, STAT3, Bcl-2, and BAX, undergo natural decay at rates μS1,μS3,μB and μX, respectively, and (ⅲ) mutual antagonism between STAT1 and STAT3 with inhibition parameters γ,δ and kinetic parameters (a1,a2,a3,a4). These biological observations (Figure 2A) and assumptions lead to the following dynamic systems of concentrations of STAT1 and STAT3 molecules within cancer cells (cf [107]):
dS1dt=λS2S⏟source+a1a22a22+γS23⏟inhibition by STAT3−μS1S1⏟decay, | (2.7) |
dS3dt=λ1K1+λS3S⏟source+a3a24a24+δS21⏟inhibition by STAT1−μS3S3⏟decay. | (2.8) |
Bcl-2 and BAX are downstream molecules whose molecular balance either inhibits or induce apoptosis [42,43,44]. While STAT1 induces apoptosis by inhibiting Bcl-2 (thus upregulation of BAX), STAT3 blocks the programmed cell death protocols by keeping Bcl-2 level intact. Thus, the STAT1-STAT3 signaling in Eqs (2.7) and (2.8) is connected to the following final steps of the apoptosis network [107]:
dBdt=λ2⏟source+a5a26a26+ωS21⏟inhibition by STAT1+λ3S3⏟S3→B−μBB⏟decay, | (2.9) |
dXdt=λ4⏟source+a7a28a28+ζB2⏟inhibition by Bcl-2−μXX⏟decay. | (2.10) |
TGF-β is secreted by cancer cells and induces the phenotypic transition from N1 to N2 TANs [10,84]. Thus, TGF-β inhibitors such as Galunisertib (LY2157299) can block this critical TGF-β-induced switch [108,109,110], thus indirectly inducing N1-favorable TME conditions. Thus, the governing equations of concentrations of TGF-β and its inhibitor are given by
dGdt=λCC⏟Production−γLLG⏟degradation by L −μGG⏟decay, | (2.11) |
dLdt=uL(t)⏟injection−μLL⏟decay, | (2.12) |
where λC is the secretion rate of TGF-β by tumor cells, γL is the degradation rate of TGF-β by TGF-β inhibitor, μG and μL are the decay rate of TGF-β and TGF-β inhibitor, respectively, and uL(t) is the external injection rate of the TGF-β inhibitor.
IFN-γ is secreted by stromal cells and immune cells, such as NK cells [111,112,113,114,115,116], and can induce the phenotypic transition from the N2-mode to N1-mode [10,84] in the TME. IFN-γ therapy alone or in a combination with other therapeutic drugs [60] has been reported to be effective in reducing tumor size [117,118,119,120] and in promoting the anti-tumor immune response [121]. In the model, we take into account the source, secretion by NK cells (both endogenous and exogenous NK cells), and natural decay. Thus, we get the governing equation:
dSdt=uS(t)⏟source+λKKend⏟production by NKs+λK′Kex⏟production by exo-NKs−μSS⏟decay, | (2.13) |
where uS(t) is the time-dependent injection rate of IFN-γ, λK and λK′ are the secretion rate of IFN-γ by endogenous and exogenous NK cells, respectively, and μS is the decay rate of IFN-γ.
NK cells are a type of immune cell that plays a critical role in the first line of defense against pathogenes and cancerous cells [112,113,114]. These immune cells are able to recognize and destroy infected or abnormal cells without the need for prior activation or antigen-specific recognition. NK cells have several mechanisms of killing, including the release of cytotoxic granules and the engagement of death receptors on target cells. In addition to their direct cytotoxic effects, NK cells also play a key role in regulating the immune response by producing cytokines such as IFN-γ and TNF-α. These cytokines not only stimulate other immune cells to mount an effective immune response but also have direct anti-tumor effects by inhibiting tumor growth and promoting the activation of other immune cells. However, these activities of NK cells may be effectively suppressed by other factors. For instance, TGF-β can inhibit NK cell activation [122,123,124] by inhibiting T-bet and IFN-γ, and suppresses NK metabolism by downregulating mTORC1, SREBP, and c-MYC [125]. Therefore, injection of exogenous NK cells, cells that are generated outside of the body and then administrated to a patient as a form of immunotherapy, may be an alternative way of overcoming suppressed immunity with safety and efficacy in a typical TME [126]. For instance, Kim et al. and others showed that the injection of exo-NK cells significantly increased anti-tumor efficacy, survival time in experiments, and in silico models [74,76]. We assume that (ⅰ) NK cells are supplied from the TME at a rate λNK, (ⅱ) NK cell activities are suppressed by TGF-β [125] with an inhibition strength θ and scaling parameters in a Hill-type function (k5,k6), (ⅲ) endogenous and exogenous NK cells go through the death processes at rates μK,μK′, respectively, and (ⅳ) exogenous NK cells are injected at a time-dependent rate uK(t). Based on these assumptions, the governing equations of densities of endogenous and exogenous NK cells, respectively, are given by
dKenddt=λNK⏟source+k5k26k26+θG2⏟inhibition−μKKend⏟decay, | (2.14) |
dKexdt=uK(t)⏟injection−μK′Kex⏟decay. | (2.15) |
Non-dimensionalization of the dimensional version of governing equations (2.1)–(2.15) is shown in Appendix A. Matlab (Mathworks) was used for computation of the mathematical model and corresponding optimal control problems. See Tables 1 and 2 for parameter values of the mathematical model.
Par | Description | Dimensionless Value | Refs |
(Dimensional Value) | |||
Cancer module | |||
r | Tumor growth rate | 0.015 (0.015h−1) | [60], Est |
C0 | Carrying capacity of a tumor | 1 (10−3g/cm3) | [60], Est |
δ1 | Killing rate of tumor cells by N1 TANs | 0.002 (20cm3/g⋅h) | [85], Est |
δ2 | Killing rate of tumor cells by apoptosis | 0.002 (0.002h−1) | Est |
δ3 | Killing rate of tumor cells by NK cells | 0.002 (0.002cm3/g⋅h) | Est |
N2/N1 modules | |||
λ6 | IL-6-induced signaling source of the N2 TANs | 3.5×10−4 (3.5×10−8g/cm2⋅h) | [85,192], Est |
λG | TGF-β signaling rate | 3.5×10−2 (3.5×103h−1) | [85], Est |
k1 | Autocatalytic production rate of N2 TANs | 0.14 (1.4×10−5g/cm3⋅h) | [85], Est |
k2 | Hill-type coefficient | 1 (1.0) | [85], Est |
α | Inhibition strength of N2 TANs by N1 TANs | 1.5 (1.5×108cm6/g2) | [85], Est |
μN2 | Decay rate of N2 TANs | 3.5×10−2 (0.035h−1) | [193,194] |
λN | neupogen signaling rate | 3.5×10−2 (70h−1) | Est |
λS1 | IFN-γ signaling rate | 3.5×10−2 (3.5×102h−1) | [85], Est |
k3 | Autocatalytic production rate of N1 TANs | 0.14 (1.4×10−5g/cm3⋅h) | [85], Est |
k4 | Hill-type coefficient | 1 (1.0) | [85], Est |
β | Inhibition strength of N1 TANs by N2 TANs | 1 (1×108cm6/g2) | [85], Est |
μN1 | Decay rate of N1 TANs | 3.5×10−2 (0.035h−1) | [193,194] |
Therapeutics | |||
λC | Secretion rate of TGF-β by cancer cells | 2.89×10−2 (2.89×10−8h−1) | [85], Est |
γL | Degradation rate of TGF-β by TGF-β inhibitor | 2.0 (1.4247×104cm3/g⋅h) | [85], Est |
μG | Decay rate of TGF-β | 2.89×10−2 (0.0289h−1) | [8,91,100,195] |
uL | TGF-β inhibitor injection rate | 0–0.5 (7.0192×10−8g/cm3⋅h) | Est |
μL | Decay rate of TGF-β inhibitor | 0.231 (0.231h−1) | [196] |
λK | Secretion rate of IFN-γ by NK cells | 4.62×10−2 (2.77×10−10h−1) | Est |
uS | IFN-γ injection rate | 0–0.2 (2×10−9g/cm3⋅h) | Est |
μS | Decay rate of IFN-β | 0.1386 (0.1386h−1) | [197] |
λNK | Source of endogenous NK cells | 4.1×10−3 (2×10−3g/cm3⋅h) | Est |
uK | NK cells injection rate | 0–0.01 (10−3g/cm3⋅h) | Est |
k5 | Reduced rate of NK cells by TGF-β | 0.02 (2×10−3g/cm3⋅h) | Est |
k6 | Hill-type coefficient | 1 (1.0) | Est |
θ | Inhibition strength of NK cells by TGF-β | 4 (4×1018cm6/g2) | Est |
μK | Decay rate of NK cells | 4.1×10−3 (0.0041h−1) | [198,199] |
μN | Decay rate of neupogen | 0.1733 (0.1733h−1) | [200,201] |
Par | Description | Dimensionless Value/(Dimensional Value) | Refs |
Intracellular modules | |||
λS2 | IFN-γ signaling rate | 2.89×10−2 (7.0227h−1) | [107,202], Est |
a1 | autocatalytic production rate (STAT1 module) | 0.1156 (2.8091×10−7g/cm3⋅h) | [107], Est |
a2 | Hill-type coefficient (STAT1 module) | 1.0 (1.0) | [107], Est |
γ | Inhibition strength of STAT1 by STAT3 | 1.5 (7.8765×1011cm6/g2) | [107], Est |
μS1 | Decay rate of STAT1 | 2.89×10−2 (0.0289h−1) | [203,204] |
λ1 | Signaling source of STAT3 | 2.89×10−2 (3.9882×10−8g/cm3⋅h) | [107], Est |
K1 | Inhibition parameter | 5.0 (5.0) | [107], Est |
λS3 | IFN-γ signaling rate | 1.0 (108cm3/g) | [107], Est |
a3 | autocatalytic production rate (STAT3 module) | 0.1156 (1.5953×10−7g/cm3⋅h) | [107], Est |
a4 | Hill-type coefficient (STAT3 module) | 1.0 (1.0) | [107], Est |
δ | Inhibition strength of STAT3 by STAT1 | 1.0 (4.1152×105cm6/g2) | [107], Est |
μS3 | Decay rate of STAT3 | 2.89×10−2 (0.0289h−1) | [203,204] |
λ2 | Signaling source of Bcl-2 | 5.8×10−3 (1.508×10−9g/cm3⋅h) | [107], Est |
a5 | autocatalytic production rate (Bcl-2 module) | 2.89×10−2 (7.514×10−9g/cm3⋅h) | [107], Est |
a6 | Hill-type coefficient (Bcl-2 module) | 1.0 (1.0) | [107], Est |
ω | Inhibition strength of Bcl-2 by STAT1 | 1.0 (1.6935×1011cm6/g2) | [107], Est |
λ3 | Signaling from STAT3 | 3.47×10−2 (0.0065h−1) | [107], Est |
μB | Relative decay rate of Bcl-2 | 3.47×10−2 (0.0347h−1) | [205,206] |
λ4 | Signaling source of BAX | 5.8×10−3 (4.3×10−5g/cm3⋅h) | [107], Est |
a7 | autocatalytic production rate (BAX module) | 0.1156 (8.52×10−4g/cm3⋅h) | [107], Est |
a8 | Hill-type coefficient (BAX module) | 1.0 (1.0) | [107], Est |
ζ | Inhibition strength of BAX by Bcl-2 | 1.0 (1.4793×1013cm6/g2) | [107], Est |
μX | Relative decay rate of BAX | 0.1445 (0.1445h−1) | [207,208] |
Threshold | |||
thN1 | Threshold of N1 TANs | 1.3 | [85], Est |
thN2 | Threshold of N2 TANs | 1.8 | [85], Est |
thS1 | Threshold of STAT1 | 1.8 | [107], Est |
thS3 | Threshold of STAT3 | 1.3 | [107], Est |
thB | Threshold of Bcl-2 | 1.44 | [107], Est |
thX | Threshold of BAX | 0.3 | [107], Est |
We first investigate the fundamental dynamics of N2-N1 systems in Eqs (2.1) and (2.2) in response to two key constant signals, NK cells (Kend) and TGF-β (G). In order to analyze the system structure at steady states, we can solve N2,N1 as a function of the NK cells (Kend) and TGF-β(G), respectively.
We observe qualitatively different structures of the bifurcation curves of N2,N1 in response to the fixed NK signal (Kend) in the presence of low (G=0.1; Figure 3A), intermediate (G=0.5, Figure 3B), and high (G=0.9; Figure 3C) TGF-β levels. The equilibrium point at the lower and upper branches in Figure 3A are stable, while the steady state in the middle branch is unstable. See the details in Supporting Information S3 File. This naturally creates a bi-stability window where either N1 TANs or N2 TANs can dominate the tumor microenvironment depending on the initial configuration of the N1/N2 populations. For instance, we have a bi-stability window WK=[0.74,1.35] in the NK cell spectrum when G=0.5 in Figure 3B. This bi-stability window is shifted to the left (lower sector of NK cell signal) in response to low TGF-β signal (Figure 3A) while the bistable region completely disappears in response to the high TGF-β level (Figure 3C). Figure 3D–F illustrate the bifurcation curves of the densities of N2,N1 TANs in response to the fixed TGF-β signal (G) in the presence of the low (Kend=0.1; Figure 3D), intermediate (Kend=1.0; Figure 3E), and high (Kend=2.0; Figure 3F) NK cell activities. We observe the opposite effect on the up- or down-regulation of N1 (and N2) TANs for various TGF-β levels. A bi-stability window emerges when NK cell activities are low (Figure 3D) but moves to the middle range of the TGF-β level (WG=[0.32,0.53], Figure 3E) as Kend increases (Kend=0.1→1) and is eliminated in response to the high level of NK cell activities (Figure 3F). In both cases, the onset and size of the bi-stability windows (WK,WG) depend on the input signal (Kend,G) and parameter values. By taking the critical thresholds, thN1(=1.3) and thN2(=1.8), in the structure of the bifurcation curves in response to both NK cell signal and TGF-β in Figure 3A–F, we can define the anti-tumorigenic (Pa) and tumorigenic (Pt) phases as follows:
Pa={(N1,N2)∈R2:N1>thN1,0≤N2<thN2}, | (3.1) |
Pt={(N1,N2)∈R2:0≤N1<thN1,N2>thN2}. | (3.2) |
When the TGF-β signal is low (G=0.1), the low NK cell activities lead to the co-existence of anti-tumorigenic (Pa) and tumorigenic (Pt) states, but the increased NK cell signals can induce Pa state (Figure 3A). For the intermediate level of TGF-β (G=0.5, Figure 3B), various stimuli from NK cells induce the nonlinear immune responses. For instance, a low level of NK cells (red asterisk in Figure 3B) induces Pt-mode in given TME. On the other hand, intermediate levels of NK signals (blue asterisk in Figure 3B) can generate the bi-stable state (Pa or Pa), resulting in either N1- or N2-dominant TME based on trajectories of solutions. As Kend is increased further, passing the right knee (Kend=1.35 of the N1 bifurcation curve (blue curve), the high level of NK cell activities enhance the immune balance toward the Pa-state where tumor growth can be suppressed. Conversely, as the NK cell signal is slowly decreased from a high level (blue asterisk in Figure 3B), the system tends to maintain the Pa-mode along the upper branch until it jumps down to the lower branch at the left knee point (Kend=0.74), returning to the opposite mode (Pt). Thus, the direction (increase or decrease) of the immune activities of NK cells can play a role in forming the tumorigenic or anti-tumorigenic mode of TANs in a given TME. When the TGF-β signal is high (G=0.9; Figure 3C), the immune system adapts to the N2-favorable mode Pt with a great potential of fast growing tumor regardless of the strength of the NK activities. We observe a different effect of TGF-β on determining the TAN-induced characteristics of TME in Figure 3D–F. For the intermediate level of NK cell activities (Kend=1; Figure 3E), the TAN system converges toward the Pt (or Pa) in response to high (or low) TGF-β stimuli while the intermediate TGF-β level can lead to a mixed state (Pt or Pa) with the bi-stability window WG. However, when NK activities are suppressed (Kend=0.1; Figure 3D), the system can adapt only to the bistable mode or Pt. In this case, the TAN system does not return to the suppression mode (Pa) even when the TGF-β signal is decreased to zero from a high level with TGF-β inhibitor treatment. On the other hand, when NK cell treatment is enhanced (Kend=1→2; Figure 3E → Figure 3F), the bi-stability window WG disappears and the system gets the higher transition point from Pa to Pt as G increases, resulting in the decreased N2-to-N1 ratio [85] and increased anti-tumorigenic potential. These are consistent with experimental observations [10,11,12,13,14]. Figure 3G shows the geometric illustration of the single mode (Pa (blue panel), Pt (pink panel)), and bi-stable mode (Pa or Pt) corresponding to (Kend= 0.1, 1.0, 2.0 in the N1−N2−Kend cube. The curves represent stable steady states (Ns1,Ns2,Ksend) in the N1−N2−Kend cube with the color coding (blue ∈Pa; red ∈Pt; green ∈Pa∪Pt). This implies that fluctuating NK cell activities can lead to active phenotypic switches of TANs between Pa and Pt with a nonlinear perturbation due to bi-stability in the middle. In a similar fashion, Figure 3H illustrates the structure of the tumorigenic or anti-tumorigenic regions and stable branches of equilibrium in the N1−N2−G cube. Thus, changes in the concentration of TGF-β can lead to different routes among different mono- or bistable-states of TANs. The phenotypic representation in response to various NK cell (Kend) and TGF-β (G) stimuli is shown in Figure 3I. For a low, intermediate, and high level of NK cell signals, the system can be exposed to various environments (Pa, Pt, or mixed) as G is increased. For instance, the system transits from Pa to a mixed state, and to Pt for the intermediate Kend level. In a similar fashion, the system goes through Pt→WG→Pa transition for intermediate G as Kend increases. Overall, the system adapts to the bi-stable system for lower Kend,G values and mono-stable system as either Kend or G is high. One can also observe that the relative balance between TGF-β and NK cells can determine whether the TME is pro- or anti-tumorigenic. This also suggests that the combined therapy of TGF-β inhibitor and exo-NK cells can effectively suppress the tumor growth.
In Figure 4A,B, we show the sensitivity of Kend-dependent Bcl-2 (B) and BAX (X) to changes in the inhibition strength parameter γ of STAT1 by STAT3 (γ=0.5,1,1.5,5), respectively. As γ is increased, both the bifurcation curve B(Kend) and bi-stability window (DK) shift to the right, leading to a decrease in the size of the window (|DK|). Here, we define the apoptotic (Ta) and anti-apoptotic (Tt) phases based on the threshold values (thB,thX):
Ta={(B,X)∈R2:0≤B<thB;X>thX} | (3.3) |
Tt={(B,X)∈R2:B>thB;0≤X<thX}. | (3.4) |
As γ is decreased, the probability of the phenotypic switching to the apoptosis phase (Ta) is increased. For example, the Bcl-2 level is already in the Ta-phase (B<thB,X>thX) when Kend=1 in the case of the lower γ (γ=0.5; green solid curves in Figure 4A,B) while it is still in the anti-apoptosis phase (Tt; B>thB,X<thX) in the base case (γ=1.5; solid red curves in Figure 4A,B). On the other hand, the system adapts to a tumor-favoring situation even in the presence of the high level of NK cell activities as γ is increased. For example, the system will be in the Tt-phase for a high NK signal (Kend=3) when γ is high (γ=5; black dotted curves in Figure 4A,B) while it should be in the Ta-phase in the base case (γ=1.5). Figure 4C shows the phenotypic transition from a Ta-phase to a mixed phase (Ta+Tt), and to a Tt-phase under various NK cells conditions as γ increases. While the system adapts to the Ta-dominant TME regardless of strength of NK cell activities for small γ (0≤γ<γMIN), it forms the Tt-dominant TME regardless of NK cell conditions for larger γ (γ>γMAX). For the intermediate levels of γ (γMIN<γ<γMAX), the increased NK cell activity leads to the mode switches toward the apoptotic death of cancer cells: Tt→(Ta∪Tt)→Ta. In Figure 4D,E, we show the sensitivity of Kend-dependent Bcl-2 (B) and BAX (X) to changes in the inhibition strength parameter of STAT3 by STAT1 (δ=0.5,1,1.5,6), respectively. As δ is decreased, both the bifurcation curves (B(Kend),X(Kend)) and the bi-stability window (DK) shifts to the right with a decrease in the size of the window (|DK|).
An increase in δ leads to higher probability of the phenotypic switching to the apoptosis phase (Ta). For example, the level of Bcl-2 is already in the Ta-phase (B<thB,X>thX) when Kend=1 in the case of the higher δ (δ=6; green solid curves in Figure 4D,E) while it is still in the anti-apoptosis phase (Tt; B>thB,X<thX) in the base case (δ=1; solid red curves in Figure 4D,E). On the other hand, the system favors a tumor-promoting TME even in the presence of high NK cell activities when δ is small. For example, the system would be in the Tt-phase for a high NK cells signal (Kend=3) when δ is small (δ=0.5; black solid curves in Figure 4D,E) while it should be in the Ta-phase in the base case (δ=1). Figure 4F shows the cell fate distribution of cancer cells for various NK cell levels and δ's. For a fixed NK cell activity, an increase in δ leads to a phenotypic transition from a Tt-phase to a mixed phase (Ta+Tt), and to a Ta-phase. While the system favors the Tt-dominant TME regardless of strength of NK cell activities for small δ (0≤δ<δMIN), it forms the Ta-dominant TME regardless of NK cell conditions for larger δ (δ>δMAX). For the intermediate levels of δ (δMIN<δ<δMAX), the increased NK cell activity leads to two types of mode switches toward the apoptotic death of cancer cells: (ⅰ) Tt→(Ta∪Tt)→Ta (δMIN<δ≤δ†) (ⅱ) (Ta∪Tt)→Ta (δ†<δ<δMAX).
In the model developed in this paper, there are several parameters for which no experimental data are available, and these parameters may affect the simulation results. We selected all parameters in the model (λ6,λG,k1,k2,α,μN2,λS1,k3,k4,β,μN1,λS2,a1,a2,γ,μS1,λ1,K1,λS3,a3,a4,δ,μS3,λ2,a5, a6,ω,λ3,μB,λ4,a7,a8,ζ,μX,λK,μS,λC,μG,λNK,k5,k6,θ,μK,D,αRT,βRT,ϵN2,ϵN1,λRK,λRG,r,δ1, δ2,δ3) for sensitivity analysis. We investigated the sensitivity of the major variables (N1 TANs, N2 TANs, STAT1, STAT3, Bcl-2, BAX, IFN-γ, NK cells, TGF-β, and Cancer cells) to these parameters at various time points. Specifically, we computed partial rank correlation coefficients (PRCCs) for N1,N2,S1,S3,B,X,S,Kend,G, and C at times t=30 days in the model. We established a range for each of these parameters and divided each range into 10,000 intervals of uniform length. For each of these 54 parameters of interest, a PRCC value was calculated. A PRCC value is a real number between -1 and 1, with the sign indicating whether an increase in the parameter value either decreases (-) or increases (+) each variable at a given time. The sensitivity analysis described below was carried out using the method (General Latin Hypercube Sampling (LHS) scheme and Partial Rank Correlation Coefficient (PRCC)) [127].
Figure 5 shows the PRCC values of all variables at t=30day. Most of eleven parameters (λ6,λG,k1,k2,α,μN2,λS1,k3,k4,β,μN1) in the N1/N2 TANs system demonstrated sensitivity exclusively to either N1 or N2 TANs. Specifically, the N2 TANs activities are positively correlated with λG,k1,k2, β,μN1, indicating that increases in these parameters promote the N2 phenotype. Conversely, the N2 TANs activities are negatively correlated to α,μN2,λS1,k3,k4, highlighting their suppressive effect on the N2 phenotype. This analysis underscores the differential impact of each parameter on the balance between N2 and N1 TANs. On the other hand, 23 parameters involved in STAT signaling pathway show specific correlations with the players (STAT1 (S1), STAT3 (S3), BAX (B), and BAX (X)) in the signaling network. For instance, the parameters a1,a2,μS3,μB,a7, and a8 exhibited positive correlations with the BAX level, indicating that an increase in these parameters enhances the apoptotic phenotype. Conversely, parameters μS1,a3,a4,λ3,ζ, and μX showed negative correlations, suggesting that the larger value of these parameters suppresses the apoptosis of cancer cells. This analysis highlights the key factors influencing apoptotic regulation within the STAT signaling pathway. The rest of parameters are involved in the interactions between cancer cells, radiation therapy, and the immune system. This analysis evaluates how variations in these parameters influence key system dynamics, highlighting the relative impact of each parameter on tumor progression, immune response, and the efficacy of radiation therapy. The N1 TAN activities are positively correlated with λK and λRK but negatively correlated with μK and ϵNK. The N2 TAN activities, located on the opposite side in the TAN spectrum, show the opposite effect from changes in these parameters. On the other hand, the IFN-γ level is positively correlated with λK,λRK,D, and αRT but negatively correlated with μK. In a similar fashion, the STAT1 level and BAX expression are positively correlated with λK,λRK,D, and αRT but negatively correlated with μK due to the feed-forward structure of the intracellular signaling network. However, the antagonist STAT3 and Bcl2 in the direct downstream shows the opposite effect: a negative correlation with λK,λRK,D, and αRT and a positive correlation with μK due to mutual antagonism between STAT1 and STAT3 upstream of the signaling. NK cell activities are negatively correlated with μK but positively correlated with radiation-induction parameters D,αRT,βRT, and λRK. TGF-β is positively correlated with radiation dose packages D,αRT,βRT, and λRG, indicating strong impact from radiation, but do not show particular negative correlations with other parameters.
To ensure a thorough evaluation of model robustness, we have conducted sensitivity analysis across a biologically relevant range of parameters. The specific parameter ranges used in sensitivity analysis, including minimum, maximum, and baseline values, are summarized in Table 3.
Parameter | λ6 | λG | k1 | k2 | α | μN2 |
Minimum | 3.5×10−5 | 0.0035 | 0.014 | 0.1 | 0.15 | 0.0035 |
Baseline | 3.5×10−4 | 0.035 | 0.14 | 1 | 1.5 | 0.035 |
Maximum | 3.5×10−3 | 0.35 | 1.4 | 5 | 5 | 0.35 |
Parameter | λS1 | k3 | k4 | β | μN1 | λS2 |
Minimum | 0.0035 | 0.014 | 0.1 | 0.1 | 0.0035 | 2.89×10−3 |
Baseline | 0.035 | 0.14 | 1 | 1 | 0.035 | 0.0289 |
Maximum | 0.35 | 1.4 | 5 | 5 | 0.35 | 0.289 |
Parameter | a1 | a2 | γ | μS1 | λ1 | K1 |
Minimum | 1.156×10−2 | 0.1 | 0.15 | 2.89×10−3 | 2.89×10−3 | 0.5 |
Baseline | 0.1156 | 1 | 1 | 0.0289 | 0.0289 | 5 |
Maximum | 1.156 | 5 | 5 | 0.289 | 0.289 | 10 |
Parameter | λS3 | a3 | a4 | δ | μS3 | λ2 |
Minimum | 0.1 | 1.156×10−2 | 0.1 | 0.1 | 2.89×10−3 | 5.8×10−4 |
Baseline | 1 | 0.1156 | 1 | 1 | 0.0289 | 5.8×10−3 |
Maximum | 5 | 1.156 | 5 | 5 | 0.289 | 0.058 |
Parameter | a5 | a6 | ω | λ3 | μB | λ4 |
Minimum | 2.89×10−3 | 0.1 | 0.1 | 3.47×10−3 | 3.47×10−3 | 5.8×10−4 |
Baseline | 0.0289 | 1 | 1 | 0.0347 | 0.0347 | 5.8×10−3 |
Maximum | 0.289 | 5 | 5 | 0.347 | 0.347 | 0.058 |
Parameter | a7 | a8 | ζ | μX | λK | μS |
Minimum | 1.156×10−2 | 0.1 | 0.1 | 1.445×10−2 | 2.77×10−3 | 1.386×10−2 |
Baseline | 0.1156 | 1 | 1 | 0.1445 | 0.0277 | 0.1386 |
Maximum | 1.156 | 5 | 5 | 1.445 | 0.277 | 1.386 |
Parameter | λC | μG | λNK | k5 | k6 | θ |
Minimum | 2.89×10−3 | 2.89×10−3 | 2×10−4 | 2×10−4 | 0.1 | 0.4 |
Baseline | 0.0289 | 0.0289 | 2×10−3 | 2×10−3 | 1 | 4 |
Maximum | 0.289 | 0.289 | 2×10−2 | 2×10−2 | 5 | 8 |
Parameter | μK | D | αRT | βRT | ϵN2 | ϵN1 |
Minimum | 4.1×10−4 | 0 | 0.003 | 0.0003 | 0.05 | 0.05 |
Baseline | 4.1×10−3 | 0−5 | 0.03 | 0.003 | 0.5 | 0.5 |
Maximum | 0.041 | 5 | 0.3 | 0.03 | 1 | 1 |
Parameter | λRK | λRG | r | δ1 | δ2 | δ3 |
Minimum | 0.075 | 0.015 | 0.0015 | 0.0002 | 0.0002 | 0.0002 |
Baseline | 0.75 | 0.15 | 0.015 | 0.002 | 0.002 | 0.002 |
Maximum | 1.5 | 1.5 | 0.15 | 0.02 | 0.02 | 0.02 |
We now investigate the therapeutic effect of TGF-β inhibitor, IFN-γ, and exo-NK cells on tumor growth in this section.
These anti-tumor agents were injected once every 5 days by prescribing periodic functions uL(t),uS(t), and uK(t) in Eqs (2.12), (2.13), and (2.15), respectively, as follows (Figure 6):
uL(t)=6∑j=1Lsχ[tj,tj+hL],uS(t)=6∑j=1Ssχ[tj,tj+hS],uK(t)=6∑j=1Ksχ[tj,tj+hK]. | (3.5) |
Here, we set hL=1,Ls=0.5,τL(=tj+1−tj)=5 (pink boxes in Figure 6A1), hS=1,Ss=0.4,τS(=tj+1−tj)=5 (blue boxes in Figure 6B1), hK=1,Ks=0.08, and τK(=tj+1−tj)=5 (green boxes in Figure 6C1). The periodic injection of these therapeutic agents lead to fluctuation in these concentrations within TME (Figure 6A1–C1). While the TGF-β level (blue curve in Figure 6A1) is dramatically reduced by its inhibitor, it is not strong enough to transit the N2 TANs to N1 TANs due to the short duration of injection (Figure 6A3), leading to inefficient suppression of tumor growth (Figure 6A4). However, the elevated IFN-γ level (red curve in Figure 6B1) forms a N1-dominant TME (high N1 TAN and low N2 TAN in Figure 6B3), resulting in efficient cancer cell killing (6B4). Note that while this up-regulated IFN-γ slowly decreases the Bcl2 level, instant elevation of IFN-γ is not strong enough to upregulate the BAX level during this process (Figure 6B2). When NK cells are injected into the system, direct cancer cell killing from increased numbers of NK cells (Figure 6C1), indirect cell killing via intracellular mechanisms (Figure 6C2), and N1-mediated killing from increased IFN-γ (Figure 6C3) can effectively reduce the tumor size (Figure 6C4).
From now on, we investigate anti-tumor efficacy by monitoring relative TME states than periodic injections of anti-cancer drugs. We first investigate how controlled IFN-γ injection can affect tumor growth by maintaining either an N1-dominant TME or apoptotic status in cancer cells. Figure 7A–C show time courses of the density of N1 and N2 TANs, key molecules in apoptosis pathway (Bcl2 and BAX), and tumor population, respectively, in response to IFN-γ injection (blue boxes) at t=0,2,20.8, and 27 days.
While the injection duration was fixed, the injection time was determined so that N1-dominant TME is maintained (N1>thN1; blue curve in Figure 7A), leading to inhibition of tumor growth (Figure 7C; reduction of tumor size by 22%). In this case, the system does not adapt to apoptosis status (i.e., still in the state of down-regulation of BAX and up-regulation of Bcl-2; Figure 7B). Figure 7D–F show the time courses of TAN density (N2 and N1 TANs), concentrations of Bcl-2 and BAX, and tumor population, respectively, in the presence of apoptosis-controlled IFN-γ therapy. To prevent a rapid drop in BAX levels and maintain the apoptosis status (up-regulation of BAX and down-regulation of Bcl-2; Figure 7E) via STAT signaling, frequent IFN-γ injections are needed in the beginning. The next injection time was determined so that the Bcl-2 level stays below the threshold, maintaining the Ta-mode. In this strategy, frequent injection of IFN-γ at the early stage also induces the N1-dominant TME (Figure 7D). Thus, the combined effect (Ta+Pa) leads to a significant reduction in the tumor population (63% reduction compared to the control; Figure 7F).
In Figure 8, we consider the combination treatment of IFN-γ and TGF-β inhibitors. Galunisertib (LY2157299), a type of TGF-β inhibitor, is typically administered for 14 days, followed by a 14-day rest period in clinics [109,110]. Following the protocol, TGF-β inhibitor was injected for 14 days, beginning at day Tκ, in addition to injection of IFN-γ, four times at t=0,2,20.8,27 days as in Figure 7A–C. Figure 8A,B illustrate the injection rate of IFN-γ (blue box; uS=0.25) and TGF-β inhibitor (red box; uL=0.05) when Tκ=0 and Tκ=12, respectively.
Injection of the TGF-β inhibitor in the early stage (Tκ=0; red solid curve in Figure 8C) outperforms the case of injection in later time (Tκ=12; diamond solid curve in Figure 8C) in suppression of tumor growth due to extensive N1-mediated cancer cell killing at later times and extended duration of the anti-tumorigenic mode (Figure 8D). However, the system maintains the anti-apoptosis phase in both cases (Figure 8E). Figure 8F shows the nonlinear anti-tumor efficacy as a function of Tκ (0≤Tκ≤16). The maximum anti-tumor efficacy is obtained at an intermediate injection time of TGF-β inhibitor (Tκ=12; 20% reduction in the tumor population relative to the worst case (Tκ=0)).
The third strategy depicted in Figure 9 involves the use of NK cells. Figure 9A–C show the time courses of TAN density (N1 and N2 TANs), concentrations of Bcl-2 and BAX, and tumor population, respectively, when exo-NK cells were injected to maintain the N1-dominant TME. Two injections at t=0,2 days (green boxes in Figure 9A) were enough to upregulate N1 TANs (blue curve in Figure 9A) and down-regulate N2 TANs (red curve in Figure 9A) while the system is under Tt-mode (B>thB,X<thX) for most of the time (Figure 9B). On the other hand, in the case of apoptosis-controlled NK therapy, additional injections of exo-NK cells (green boxes in Figure 9D) were needed in order to maintain the apoptosis status (B<thB,X>thX; Figure 9E) via STAT signaling while maintaining the Pa-mode (N1>thN1,N2<thN2; Figure 9D). The tumor size in the apoptosis-controlled strategy (Figure 9F; 98% reduction compared to control) is better controlled than the case of TAN-controlled strategy (Figure 9C; 66% reduction compared to control) despite higher administration costs.
NK cells were shown to play a significant role in modulating the tumor microenvironment, i.e., either enhancing or decreasing anti-tumor efficacy in a combination therapy depending on the nature of interference of anti-tumor and immunosuppressive effects [74]. We consider immune-based combination therapy (exo-NK + TGF-β inhibitor) in Figure 10 to test the role of NK cells in regulating immune-tumor dynamics and cancer cell killing in the presence of TGF-β inhibitor treatment. Here, a fixed amount of TGF-β inhibitor was continuously injected over the time interval [Tκ,Tκ+τ] with various infusion times Tκ (0≤Tκ≤16, τ>0; pink boxes in Figure 10A,B) while exogenous NK cells were injected twice in the early stage as in Figure 9A (green boxes in Figure 10A,B). Figure 10C–E show time courses of cancer cell densities and corresponding trajectories of solutions (N2(t),N1(t)) and (B(t),X(t)) in the N2−N1 and B−X plane, respectively, in control and NK+ cases (Tκ=0 (solid, worst case), and 12 (diamond, best) in Figure 10C). While two injections of exogenous NK cells in these two cases were enough to switch N2 TANs to N1 TANs in the TME (Figure 10D), these kept cancer cells in the anti-apoptosis of the cancer cells (Figure 10E). Figure 10F summarizes the normalized tumor population at final time in response to the combination therapy with various infusion times (Tκ; 0≤Tκ≤16). The maximum anti-cancer efficacy is obtained when Tκ=12 while the efficacy is decreased as Tκ is further decreased.
We finally consider a combination of two drugs (IFN-γ and TGF-β inhibitor) and exo-NK cells in Figure 11. While IFN-γ (blue box) and NK cells (green box) are injected 4 times and 2 times as before, various infusion times Tκ of TGF-β inhibitor (pick box; 0≤Tκ≤16) were selected for testing (Figure 11A,B). Figure 11C shows the time courses of tumor populations when Tκ=16, 2 in response to triple combination treatment with the late (Figure 11A) and early (Figure 11B) injection of TGF-β inhibitor. In these cases, the combination treatment leads to the phenotypic transition from N2 TANs to N1 TANs (Figure 11D). Unlike sustained anti-apoptotic status in the NK+TGF-β inhibitor combination in Figure 10, these triple treatments also activate apoptosis signaling pathways within the cancer cells (Figure 11E). Figure 11F shows the tumor population at final time in control (red solid) and combination therapy with various Tκ values (0≤Tκ≤16). In Figure 11G, we summarize the normalized tumor population at the final time points in response to three combination treatments (IFN-γ+TGF-β inhibitor; NK+TGF-β inhibitor; IFN-γ+NK+TGF-β inhibitor).
The normalized tumor populations corresponding to different injection combinations of IFN-γ, TGF-β inhibitor, and NK cells are shown in the circular form in Figure 12A–C where the center represents the 0 population and the far edge along the radial direction indicates the 1 value. Thus, the length of the radial line is the normalized tumor population in [0,1] corresponding to each injection scheme. The different schemes were categorized by three groups based on the initial agent of IFN-γ (S in (A)), NK cells (K in (B)), and TGF-β inhibitor (L in (C)). Figure 12D–F show the temporal profiles of the Pa- and Ta-modes for various combinations of the combination therapies in Figure 12A–C. Simulation results suggest that combination 'LLSSKK' is the least effective in killing tumor cells while the 'KSSKLL' scheme is the most effective in increasing anti-tumor efficacy (Figure 12B,C,J). In the best scenario 'KSSKLL', we observe a wide range of the apoptotic status (blue region in Figure 12I) in response to the initial injection of NK cells and IFN-γ at earlier time points (Figure 12G) and the sustained anti-tumorigenic mode (blue region in Figure 12H) in response to TGF-β inhibitor treatment at later times (Figure 12G). On the contrary, in the worst scheme ('LLSSKK'), earlier two infusions of TGF-β inhibitor (red bars, Figure 12K) are not as effective in switching TANs to the Pa-mode (blue region in Figure 12L) and the system successfully transits to the Pa-mode only after IFN-γ injection after t=10 days. Two injections of IFN-γ in the middle temporal spot and additional injection of exogenous NK cells at later times (t>20days, Figure 12K) is not strong enough to induce the apoptotic status (Ta) later times (Figure 12M). These two factors, delay in the systemic transition to Pa-mode and lack of Ta-mode, result in relatively weak anti-tumor efficacy (blue curve, Figure 12J). In general, early injections of IFN-γ tend to form the wide range of Pa status but the narrow or none of Ta-mode (Figure 12D). These opposite effects lead to the intermediate level of cancer cell killing (left panel in Figure 12N). On the other hand, early injections of TGF-β inhibitor induce the narrower ranges of Pa in middle and small range of Ta-modes at later times (Figure 12F), leading to lower anti-tumor efficacy (right panel in Figure 12N). Finally, initial infusion of NK cells induce well-shaped ranges of Pa- and mixed (Pa∪Ta)-status, leading to effective, combined cancer cell killing (middle panel in Figure 12N).
In this section, we investigate how the complex interactions between IR and immune cells in the TME (Figure 1) regulate tumor growth and develop a new anti-cancer strategy by combining the TGF-β inhibitor to RT therapy. Here, we propose a mathematical model of tumor growth via tumor-NK-TAN interactions and cancer cell survival dynamics in the presence of radiotherapy (Figure 1). The linear-quadratic (LQ) model, one of the standard tools in radiation biology, has been used to calculate cell survival in response to delivered radiation dose [128,129]. In this framework, the survival probability [RT] of cells is calculated as follow [130]:
[RT]=exp(−BED)=exp(−αRTD−βRTD2), | (3.6) |
where the biologically effective dose (BED), BED=αRTD+βRTD2, is obtained from cell-specific radiosensitivity parameters (αRT and βRT) and the physical dose delivered per fraction (D). With the known BED for healthy cells and cancer cells, the killing rate of the cells by radiation is given by
μRT=μ0(1−[RT]), | (3.7) |
where μ0 is a basic killing rate due to radiation. We use the well-known relation αRT/βRT=10Gy [81,93,131] in this study. Based on experimental observation, we also assume that the effect of radiation on N1 and N2 TANs is lower than one for cancer cells. Thus, we set αRT=0.03Gy−1,βRT=0.003Gy−2.
In order to take into account the effect of the radiotherapy, we now use the modified version of Eqs (2.1), (2.2), (2.6), (2.14), and (2.11), for the densities of N1/N2 TANs, cancer cells, and NK cells, and concentration of TGF-β as follows:
dN1dt=λS1S+k3k24k24+βN22−μN1N1−εN1μRTN1, | (3.8) |
dN2dt=λ6+λGG+k1k22k22+αN21−μN2N2−εN2μRTN2, | (3.9) |
dCdt=rC(1−CC0)−δ1N1C−δ2CI{B<thB,X>thX}−δ3C(Kend+Kex)−μRTC, | (3.10) |
dKenddt=λNK+k5k26k26+θG2−μKKend+λRKμRT, | (3.11) |
dGdt=λCC−γLLG−μGG+λRGμRT, | (3.12) |
where εN2,εN1 are a scaling factor due to the low sensitivity of IR damage (εN2,εN1≪1), λRK,λRG are production rate of NK cells and TGF-β by IR, respectively [81]. We consider a treatment strategy using IR and TGF-β inhibitor through the Eqs (3.10)–(3.12). We set εN2=εN1=0.5. We also set λRK=0.05g/cm2 and λRG=0.01g/cm2.
In Figure 13, we investigate the effect of radiation on regulation of changes in the N1/N2 TAN composition in TME and overall anti-tumor efficacy. In this model, we set αRT=0.03 and βRT=0.003 following [81,93,131]. Radiation therapy can cause neutropenia (low neutrophil count) as it damages the rapidly dividing cells in the bone marrow, where white blood cells are produced. The severity and duration of radiation-induced neutropenia depend on the dose and location of the radiation therapy, as well as other individual factors such as age, overall health, and the presence of other medical conditions [132,133]. In our model, the radiation-mediated cell killing from different doses of radiation (Figure 13A, D=0,1,3,5) lowers the overall population and the landscapes of the levels of N1 and N2 TANs (Figure 13B,C) relative to control (D=0), illustrating consistent decrease in neutrophils in TME as shown in experiments. A decrease in TAN populations may interfere with strong anti-cancer activities by N1 TANs in the presence of NK cells or decrease the tumor-promoting effect by N2 TANs. As D increases, the bi-stable region disappears (Figure 13B,C). Radiotherapy is typically administered to cancer patients five times per week, with treatment schedules lasting from 3 to 9 weeks [134,135,136]. In our model, we simulate a total of 4 weeks of radiation therapy, with treatment administered five times per week. Figure 13D–E show the normalized tumor population and corresponding time courses for various radiation dose levels (D=1 (orange), 2 (yellow), and 3 (purple) relative to control (D=0; blue curves). Radiation with strong dose is quite effective in killing cancer cells (D≥2) but it can induce neutropenia [132,133]. During this radiation period, the system also stays in the tumorigenic mode even with low levels of radiation (Figure 13F).
The optimal control theory [137] was utilized to find optimal infusion strategies of anti-cancer drugs that minimize the tumor population and minimize administrative costs in mathematical oncology. Optimal control theory has been adapted to develop effective administration schedules of anti-cancer drugs in various types of cancer progression [138,139,140,141,142], including glioblastoma [76,143,144,145,146,147] and lung cancers [107,148]. In particular, an optimal control theory was used to block aggressive glioma cell infiltration by maintaining miR-451 levels [147] and regulating the cell cycle [146], or to optimize the cancer cell killing via controlling a nonlinear immune-tumor interaction such as NK cells [76] and traditional drugs such as bortezomib with OVs via the necroptosis pathways [143]. Here, we use an optimal control theory to reduce the total cancer population ∫C(t)dt and minimize the side effects and administrative costs uRT associated with radiation therapy by obtaining radiation schedule of uD. The control uD represents dosage of ionizing radiation. This control is also assumed to be bounded. That is, the control set is defined as
uD(t)∈[0,umaxD] for all t∈[ts,tf] | (3.13) |
where umaxD is the maximum dosage of ionizing radiation, which is administered in a given treatment period, and ts and tf are the start and end time of treatment with optimal control applied, respectively. The cost (side effects + administrative costs) associated with radiation therapy is calculated by
uRT=u0(1−e−αRTuD−βRTu2D) |
where u0 is a proportional constant. An objective function is to find the optimal dose strategy (temporal profiles and dose) of radiation over time for the minimal tumor population and minimal costs, leading to an objective function as follows:
J(uL(t),μRT(t))=∫tftsC(t)+C1uRT(t)+C2u2RT(t)dt, | (3.14) |
uRT=u0(1−e−αRTuD−βRTu2D), | (3.15) |
where C1 and C2 are the weight constant for each control. Both linear and quadratic terms in the integrand are used in costs associated with radiation in order to regularize the radiation dose in the system. Linear controls are more difficult to analyze compared to quadratic controls despite their higher degree of biological relevance. Numerical solutions of the control problems (3.14) and (3.15) was obtained using the forward-backward sweep method with shooting methods [137].
Since radiation therapy is performed five times a week [134,135,136] in a clinical setting, we applied the optimal control to adjust the radiation dose to achieve the goal of minimizing the tumor size within a time cycle (1 week). Figure 14A shows the time courses of radiation doses without (dashed blue) and with (solid pink) the application of optimal control theory, respectively. We can reduce the tumor size at the final time by optimal control (Figure 14C) with lower radiation dose (Figure 14B). Thus, optimally controlled schedule can lead to better clinical outcomes compared to regular radiation schedule with fixed dose and duration. The radiation therapy can kill tumor cells (>50%) relative to control (w/o treatment) as shown in experiments with the non small cell lung cancer cells implanted in C57Bl6 mice [149].
Radiotherapy (RT) is a common treatment option for cancer patients, and it is well known that it can stimulate a robust immune response, providing a strong basis for the combination of RT and immunotherapy (iRT) [150]. While radiation kills cancer cells and stimulates NK cell activities, it also stimulates TGF-β secretion. Furthermore, the radiation dose is usually set low in order to minimize the biophysical and biochemical strain on the patient's body. Here, we consider the iRT combination therapy (RT + TGF-β inhibitor) with low dose radiation and optimal control theory. TGF-β is a protein that plays a role in cell growth and division and is also involved in cancer development [48,80]. Preclinical studies have shown that combining radiotherapy with TGF-β inhibitors can enhance the anti-tumor efficacy of radiotherapy by increasing tumor cell killing and reducing the growth, spread of tumors, and angiogenesis [151,152]. TGF-β inhibitors have also been shown to decrease the adverse effects of radiotherapy on normal tissues, such as reducing inflammation and fibrosis [153,154]. While iRT is not yet a standard treatment option for cancer, it shows a great potential of eradicating tumor cells. iRT was also suggested to promote abscopal effect, i.e., radiation-mediated anti-tumor immune response with the regression of non-irradiated tumors at distant sites [150]. To investigate the anti-tumor efficacy of the combination therapy, two injection schedules of the TGF-β inhibitor were selected in addition to regular RT (Figure 14): Tκ=16 (Figure 15A), 6 (Figure 15B). Suppression of the TGF-β level (red curves in Figure 15C) in response to the TGF-β inhibitor induces the transition from the tumorigenic to anti-tumorigenic mode (Figure 15D) in both cases. Ionizing radiation in the combination therapy further upregulates NK cell activities (blue curves in Figure 15C), which increases anti-tumor efficacy. Thus, this combination therapy leads to effective cancer cell killing compared to control (RT−TGF-β inhibitor−) (Figure 15D). Figure 15F shows normalized tumor populations for various Tκ's (Tκ=1,…,16), implying the importance of scheduling of the TGF-β inhibitor. For instance, the tumor size when Tκ=6 is reduced by about 40% compared to the worst case (Tκ=16) and NK cell activities are also higher when Tκ=6.
In order to treat stage 3 or 4 cancer patients, a large amount of radiation is necessary [155,156,157] but this can cause a serious side effect, such as neutropenia. Neutropenia, characterized by a deficiency of neutrophils (comprising N1 TANs + N2 TANs) in the bloodstream, is one of frequent complications of extended-field radiotherapy. This condition often leads to treatment disruptions and elevates the susceptibility to infections or bleeding [158,159]. For patients undergoing simultaneous chemotherapy and radiotherapy targeting the mediastinum, the administration of CSF support is not a favorable option due to heightened risks of complications and mortality [160,161]. However, in scenarios where chemotherapy is not part of the treatment regimen, CSF support could be contemplated for patients undergoing RT alone if there is substantial and prolonged neutropenia, particularly when RT involves a considerable volume of active bone marrow. The spontaneous resolution of neutropenia following RT alone can be protracted, often taking around 3–4 weeks after exposure to an intermediate dose of total body radiotherapy [162]. Hence, the utilization of a medication known as neupogen (G-CSF) is warranted to address neutropenia. Here, we develop a new combined RT treatment strategy for a high-grade tumor where the patient is treated with a high radiation dose and neutropenia is minimized. We set C(0)=0.5 to reflect the later stage tumor. In this framework, neutropenia is defined by
neutropenia={(N1,N2)∈R2|Ni<1,Ni(t)=N1(t)+N2(t)thN} |
where thN=3. Figure 16A shows the temporal profiles of Ni for various radiation dose (D=0,1,2,3,4,5). When the radiation dose is low (D=0 or 1 in Figure 16A), the system does not induce neutropenia during the treatment (Ni(t)>1,∀t∈[0,30]) though the anti-tumor efficacy is also low (D=1, red dashed curve in Figure 16B). A higher radiation dose (D≥2) is needed to eradicate tumor cells for stage 3 or 4 cancer patients, as shown in Figure 16B. However, these higher radiation dose strategies lead to neutropenia (D≥2; yellow boxes in Figure 16A). To reduce the duration of radiation-induced neutropenia, specific agents such as neupogen [163] have been used. For the following simulations, we set D=2 for high dose RT. In Figure 16C–H, we investigate the effect of neupogen on controlling neutropenia during RT. We assume that (ⅰ) neupogen is injected during time interval [Tκ,Tκ+τ] at a fixed injection rate uN=0.1733, and (ⅱ) neupogen is administered for 14 days (i.e., τ=14days), similar to the TGF-β inhibitor treatment (Figure 15), to control neutropenia following [164]. By modifying Eq (3.8) for N1 TAN and introducing a new variable, neupogen concentration (N), we have the following dynamics:
dN1dt=λNN+λS1S+k3k24k24+βN22−μN1N1−ϵN1μRTN1, | (3.16) |
dNdt=uNI[Tκ,Tκ+τ]−μNN. | (3.17) |
Here, λN is the source of N1 TANs from bone marrow stem cell from neupogen, μN is the decay rate of neupogen, the indicator function I[a,b] gives 1 when t∈[a,b], or zero otherwise.
Figure 16C–E show the temporal changes in the TAN (N2 (red), N1 (blue)) densities in control (RT), and combination (RT+neupogen) therapy with Tκ=0 (D), 10 (E). The neutropenia index (Ni) stays above the threshold value for most of the time when neupogen is injected after 10 days (Tκ=10, red curve in Figure 16F), leading to weak neutropenia. On the other hand, the index drops down below 1 with a significant duration in response to the early injection of neupogen (Tκ=0, blue curve in Figure 16F), leading to cyclic neutropenia (yellow boxes in Figure 16F). Temporal discrete patterns of neutropenia in response to neupogen treatment of sequential injection time (Tκ=0,1,…,16) are shown in Figure 16G. Earlier injection of neupogen tends to generate more frequent, extensive neutropenia, including wider ranges of neutropenia at later times. Administration of neupogen at later times induces narrow strips of neutropenia but do not form these later times. Figure 16H summarizes the cumulative duration of RT-induced neutropenia (CDRN) for various Tκ's (Tκ=0,1,…,16). Neupogen infusion at 0 day (blue) and 10 days (red) gives rise to the least and most favorable outcomes, respectively. In the best scenario (Tκ=10, red box in Figure 16H), the cumulative duration of neutropenia was reduced by 90% compared to the worst case scenario (Tκ=0, blue box in Figure 16H). Therefore, our results suggest that even with the use of high-dose radiation in stage 3–4 cancer patients, the proper administration of neupogen can mitigate neutropenia and effectively eliminate cancer cells.
In this section, we extend the ODE model to the PDE model in order to capture the spatio-temporal evolution of cancer cells in the presence of NK cells and TANs in a heterogenous tumor microenvironment. The mass balance equation for the main variable w(x,t) at space x and time (t) is given by
∂w∂t=−∇⋅Jw+Pw | (3.18) |
where Jw is the flux, and Pw is the net production rate of the variable w. The flux Jw is due to the cell motility (C,N1,N2,Kend, and Kex) or diffusion process of diffusible molecules (G,S), such as
Jw=−Dw(x)∇w | (3.19) |
where Dw(x) is the space-dependent diffusion coefficient of w. By taking the reaction part of the original governing equations (2.6)–(2.13) for Pw for each variable, we get the following governing equations in the heterogenous TME:
∂C∂t=∇⋅(DC(x))∇C)+rC(1−CC0)−δ1N1C−δ2CI{B<thB,X>thX}−δ3C(Kend+Kex), | (3.20) |
∂N1∂t=∇⋅(DN1(x))∇N1)+λS1S+k3k24k24+βN22−μN1N1, | (3.21) |
∂N2∂t=∇⋅(DN2∇N2(x))+λ6+λGG+k1k22k22+αN21−μN2N2, | (3.22) |
∂Kend∂t=∇⋅(DK(x))∇Kend)+λNK+k5k26k26+θG2−μKKend, | (3.23) |
∂Kex∂t=∇⋅(DK(x))∇Kex)+uK(t)−μK′Kex, | (3.24) |
∂G∂t=∇⋅(DG(x))∇G)+λCC−γLLG−μGG, | (3.25) |
∂S∂t=∇⋅(DS(x))∇S)+uS(t)+λKKend+λK′Kex−μSS. | (3.26) |
dS1dt=λS2S+a1a22a22+γS23−μS1S1, | (3.27) |
dS3dt=λ1K1+λS3S+a3a24a24+δS21−μS3S3, | (3.28) |
dBdt=λ2+a5a26a26+ωS21+λ3S3−μBB, | (3.29) |
dXdt=λ4+a7a28a28+ζB2−μXX. | (3.30) |
The list of parameters of diffusion coefficients are given in Table 4. Our computation domain is Ω=[0,1] in a dimensionless version, and we impose Neumann (no-flux) boundary conditions for all relevant variables (w=C,N1,N2,Kend,Kex,G,S):
Dw∂w∂x|x=0=0,Dw∂w∂x|x=1=0. | (3.31) |
Par | Description | Value | Refs |
Random motility/Diffusion coefficient | |||
DC | Cancer cells | 3.6×10−6cm2/h | [8,91,100,209,210] |
DN2 | N2 TANs | 3.6×10−6cm2/h | [91,211] |
DN1 | N1 TANs | 3.6×10−6cm2/h | [91,211] |
DK | NK cells | 3.6×10−6cm2/h | [74] |
DG | TGF-β | 3.5×10−3cm2/h | [212,213,214] |
DS | IFN-γ | 3.5×10−3cm2/h | Est |
We first investigate the role of spatial distribution of NK cells and N1/N2 TANs in the regulation of tumor growth on a spatial domain Ω with two subdomains, Ω1 and Ω2: Ω1=[0,0.5], Ω2=[0.5,1], Ω=Ω1∪Ω2. We set the initial conditions as follows: (ⅰ) The cancer cells are positioned at the center (Figure 17A-1), C(x,0)=e−100∗(x−0.5)2; (ⅱ) N1 TANs are immersed within the tumor on the left (Ω1) whereas N2 TANs already reside in the tumor region on the right (Ω2 (Figure 17B-1), N1(x,0)=5.0×e−200∗(x−2/6)2+5.0×e−200∗(x−5/6)2, N2(x,0)=5.0×e−200∗(x−1/6)2)+5.0×e−200∗(x−4/6)2, and (ⅲ) NK cells are positioned near the boundary around BV (Figure 17C-1). Initial conditions of cytokines (TGF-β and IFN-γ; Figure 17D-1) and intracellular variables in the apoptotic signaling network (Bcl-2 and BAX) are set to be zero in the domain. Figure 17A–E shows spatial profiles of the densities of key players (cancer cells, N1/N2 TANs, NK cells, TGF-β, IFN-γ, Bcl-2 and BAX) at various time points (t=0,7,14,60days). The relatively high proportion of N2 TANs in the tumor region in Ω2 (Figure 17I) promotes tumor growth in the early stage compared to the case on the left domain where tumor growth is suppressed from N1 TANs.
This accelerated cancer growth leads to an elevated level of TGF-β (Figure 17D,G), which in turn suppresses NK cells activity (Figure 17C) and level of IFN-γ (Figure 17D,F), which leads to up-regulation of Bcl-2 and down-regulation of BAX within the tumor (Figure 17E). Figure 17J shows time courses of tumor populations in Ω1 (red) and Ω2 (blue), respectively. Here, the population of tumor cells was calculated by integrating the tumor cell density over the spatial domains: ˆC1(t)=∫Ω1C(x,t)dx (blue), ˆC2(t)=∫Ω2C(x,t)dx (red). Other populations of other cells and levels of cytokines were calculated in a similar fashion: IFN-γ (ˆS1(t)=∫Ω1S(x,t)dx, ˆS2(t)=∫Ω2S(x,t)dx in Figure 17F)); TGF-β (ˆG1(t)=∫Ω1G(x,t)dx, ˆG2(t)=∫Ω2G(x,t)dx in Figure 17G); N1 TANs (ˆN1,1(t)=∫Ω1N1(x,t)dx, (ˆN1,2(t)=∫Ω2N1(x,t)dx in Figure 17H); N2 TANs (ˆN2,1(t)=∫Ω1N2(x,t)dx, ˆN2,2(t)=∫Ω2N2(x,t)dx in Figure 17H). Thus immune conditions such as the N1-N2 ratio create either the immunosuppressive or immune-active tumor microenvironment (Figure 17B,G). leading to different tumor growth rates (Figure 17J).
We now investigate the role of physical microenvironment in the spatial dynamics by considering two subdomains, Ω− and Ω+: Ω−=[0,0.5], Ω+=[0.5,1], Ω=Ω−∪Ω+. In order to reflect the different tissue compositions in Ω− and Ω+, the diffusion coefficienst of all diffusible variables in Ω− were set to be 100-fold smaller than those in Ω+, i.e., Dw|Ω−=Dw|Ω+100, w=C,N1,N2,Kend,Kex,G,S. The Initial conditions are prescribed as in Figure 17 except TANs. In this analysis, we assume that N2 TANs are immersed within the tumor as in a typical tumor microenvironment. Populations of cells and levels of cytokines on each subdomain were calculated as before, for instance, ˆC−(t)=∫Ω−C(x,t)dx, ˆC+(t)=∫Ω+C(x,t)dx for IFN-γ for tumor population and ˆS−(t)=∫Ω−S(x,t)dx, ˆS+(t)=∫Ω+S(x,t)dx for IFN-γ in Ω− and Ω+, respectively. Figure 18A illustrates the different spatial profiles of cancer cells in Ω− and Ω+ under different landscapes of tissue composition. The initial growth rate of the tumor in Ω− is lower due to the smaller diffusion rate (Figure 18A-2). This essentially leads to a sequential chain of events in Ω−: Lower secretion rate of TGF-β (Figure 18D; Figure 18F), lower transition to N2 TANs (Figure 18B; Figure 18G), lower intratumoral infiltration rate of NK cells (Figure 18C; Figure 18H), and lower apoptosis rate of cancer cells (Figure 18E). These feedback cycles between the tumor and immune cells via cytokines results in the lower level of tumor growth in Ω− and faster proliferation of cancer cells in Ω+ in the later stage of cancer progression (Figure 18I).
In Figure 19, we investigate the effect of the combination therapy (NK cells + IFN-γ) on tumor growth by varying the injection locations within the computational domain [0,1]. To assess the spatial influence of therapeutic administration on anti-tumor efficacy, the injection sites are divided into two distinct regions: The boundary region (Ωb=[0,0.1]⋃[0.9,1]) for drug infusion through blood vessels and a region at the center (Ωc=[0.45,0.55]) for direct intratumoral injection. To systematically compare the effects of drug injections at different sites, we modify Eqs (3.24) and (3.26) by adding injection terms as follow:
∂Kex∂t=∇⋅(DK∇Kex)+uK(v1IΩc(x)+v2IΩb(x))−μK′Kex, | (3.32) |
∂S∂t=∇⋅(DS∇S)+uS(v1IΩc(x)+v2IΩb(x))+λKKend+λK′Kex−μSS. | (3.33) |
Here, IΩi(=IΩi(x),i=c,b) is an indicator function (giving 1 or 0 based on the subdomain x∈Ωi) and the injection rates are now expressed as a weighted distribution between the two regions: uKv1IΩc+uKv2IΩb,uSv1IΩc+uSv2IΩb, where the total injection amount remains constant, i.e., ∫Ωv1IΩc+v2IΩbdx=v0, constant. Figure 19A shows the cancer cell population at final time in response to injection of the therapeutic drugs with various injection rates v1=0,0.1,…,0.9,1 on Ωc. The best anti-tumor efficacy is obtained when 70% of these agents is injected in the center and the remaining 30% is injected on the periphery of the tumor (v1=0.7; Figure 19A; blue in Figure 19B)) while the worst case comes from the injection of all resources only at the periphery (v1=0) without intratumoral injection. Figure 19B shows the time courses of cancer cell population for three representative cases (v1=0.0,0.7,1.0). While the anti-tumor agents injected on Ωb (v1=0) (Figure 19C-1) can effectively kill tumor cells only on the periphery of the tumor mass (Figure 19C-2), the intratumoral injection (Figure 19E-1) is very effective in killing cancer cells in the central, local part of the tumor mass with limited anti-tumor efficacy on the periphery (Figure 19E-2). The systemic injection on both the boundary and center (Figure 19D-1) leads to balanced suppression of tumor growth on both sites, leading to better anti-tumor efficacy (Figure 19B).
It is well known that many immune cells such as macrophages, TANs, and NK cells in a TME play a significant role in controlling tumor growth by interacting with a tumor via various cytokines and chemokines. In this paper, we investigated the role of NK cells in regulating the well-known opposite effect of N1 and N2 TANs on tumor growth by mathematical models in the presence and absence of various therapeutic approaches, including ionizing radiation. While the role of NK cells in regulation of the TME can be either tumor-promoting or tumor-suppressive [74], tumor-secreted TGF-β was shown to suppress NK cell activity, thus blocking N2→N1 transition by down-regulation of the IFN-γ level [59,60,71,72]. For example, TGF-β may suppress the function of NK cells in lung cancer by the following mechanism [165]: (ⅰ) Changes the receptor spectrum of NK cells in lung cancer patients [166,167,168] and (ⅱ) induction of NK cell polarization toward angiogenesis [169]. Immune cells may also suppress NK cell function by secreting immunosuppressive agents [170,171,172]. For instance, studies found that the relative population of Tregs [173] in the lung TME is higher than that in normal tissues and peripheral blood, both of which can secrete TGF-β [53,168,174,175]. On the other hand, radiation therapy can enhance critical infiltration of the NK cells in TME, leading to better clinical outcomes [73,74,75,76]. The release of cytokines such as IFN-γ was shown to activate NK cells and induce the chemotactic movement toward the TME [79,80,81,82,83]. NK cells play a significant role in regulation of tumor growth and there is an urgent need to develop NK cell-based cancer immunotherapies through recent technologies [176].
While the mutual interaction between TANs and tumor cells is important in TME, the dynamical system introduced in [85] is valid only when TANs are the dominant major players in the tumor-immune communication such as in the in vitro system and this ignores the significant roles of NK cells in the immune microenvironment. In this work, we developed a new model by extending the framework developed in [85] to take into account the key biological mechanisms of NK cells in regulation of the TAN-tumor interactions as well as neutrophenia, leading to finding the new dynamical regimes that were not observed in the reduced model. This extension also enabled us to perform the mathematical analysis of the more comprehensive tumor-immune interactions and development of new combination treatment strategies involving radiotherapy. In this work, we developed a mathematical model by a system of ordinary differential equations (ODEs) to investigate the effect of NK cells on controlling the TME including N1 and N2 TANs and RT-induced neutropenia. Mathematical analysis showed the complex behaviors of phenotypic switches between N2 TANs and N1 TANs, thus nonlinear tumor growth, in response to fluctuating TGF-β and NK cells (Figure 3). We developed several therapeutic strategies of slowing down tumor growth by using the network structure of TANs and STAT signaling. In particular, we developed various injection schedules of two drugs (IFN-γ and TGF-β inhibitor) and NK cells with a goal of determining the most effective treatment regimen with the lowest possible dose (Figures 6–11). Due to the competitive nature of three cell death programs (apoptosis via Bcl2-BAX, N1-mediated killing, NK-mediated killing) in tumor cell killing, synergistic effects were observed within a particular schedule bands and injection orders. For instance, injection of IFN-γ and NK cells in the minimum amount required to induce the anti-tumorigenic phenotype (Figures 7 and 9), followed by induction of the apoptosis phenotype (Figure 11) can induce better strategic outcomes in reducing the tumor size.
Radiation therapy can induce upregulation of TGF-β, a tumor-promoting factor, despite its efficient killing of tumor cells [15,81,82,83]. The combination of radiation and the TGF-β inhibitor is effective in mitigating the pro-cancer effect of TGF-β (Figure 15) due to enhancement of NK cell activities by radiation. Under the same schedule of radiotherapy and TGF-beta inhibitor [109,110,134,135,136], the phenotypic changes of tumor-associated neutrophils, thus anti-tumor efficacy, varied depending on the timing of TGF-beta inhibitor injection, leading to a better treatment strategy (Figure 15).
Our findings in the PDE-based model highlight the complex interplay between tumor diffusion dynamics and immune regulation in a tumor microenvironment where the different spatial distribution of N2-N2 ratio or different tumor dispersal speed and NK infiltration in a harsh environment affect the immunosuppressive influence of N2 TANs and NK-mediated cancer killing, and the overall tumor-immune dynamics (Figures 17 and 18). We also found that the strategic approach of injecting therapeutic agents at different locations (infusion through blood vessels or direct intratumoral injection) may lead to better clinical outcomes (Figure 19), as shown in oncolytic virus therapy [102,177].
This work should be a starting point of finding the fundamental mechanism of biochemical interactions of N1/N2 TANs-tumor-NK cells. In the TME, there are many other critical factors that we did not consider in this work, such as M1/M2 TAMs [9], signaling networks [178], angiogenesis [179,180,181], tumor-associated fibroblasts [182], tumor ECM remodeling and restructuring [3,182,183], CSF-1 [8,184], and NET [185,186,187] which can also play a pivotal role in regulation of cancer progression. In addition, mutations in STAT1 and STAT3 molecules (loss of function (LOF) or gain of function (GOF)) were not considered [188,189]. While we focused on TANs due to their rapid phenotypic plasticity and influence on tumor-immune interactions [15], M1/M2 TAMs are another critical component of the tumor microenvironment [9,190]. Both TAMs and TANs contribute to immunosuppressive and pro-tumorigenic pathways, often governed by overlapping cytokines such as TGF-β and IL-6 [6,191]. In future work, we will extend this model to incorporate TAM dynamics and their interactions with TANs, providing a more comprehensive framework for understanding tumor progression and immune modulation. These factors could impact tumor invasion and growth. We plan to investigate the role of these important factors in near future work.
The authors declare they have not used artificial intelligence (AI) tools in the creation of this article.
This work was supported by the National Research Foundation of Korea (NRF) grant funded by the Korea government (MSIT) (No. RS-2024-00347728). This paper was written as part of Konkuk University's research support program for its faculty on sabbatical leave in 2024.
Yangjin Kim is an editorial board member for Mathematical Biosciences and Engineering and was not involved in the editorial review or the decision to publish this article. The authors declare no conflict of interest.
We utilized dimensionless expression when deriving the results presented in the main text. Consequently, in our mathematical model, we proceed with a process of non-dimensionalization to remove units in all variables. The reference value for each variable is specified in Table A1.
Par | Description | Value | Refs |
C∗ | Concentration of cancer cells | 1.0×10−3g/cm3 | [91,187] |
N∗1 | Concentration of N1 TANs | 1.0×10−4g/cm3 | [187,211] |
N∗2 | Concentration of N2 TANs | =N∗1 | [187,211] |
G∗ | Concentration of TGF-β | 1ng/mL | [215] |
L∗ | Concentration of TGF-β inhibitor | 380nM | [216,217] |
S∗ | Concentration of IFN-γ | 10ng/mL | [202] |
K∗ | Concentration of NK cells | 1.0×10−3g/mm3 | [74,218] |
S∗1 | Concentration of STAT1 | 2.43μg/mL | [219] |
S∗3 | Concentration of STAT3 | 1.38μg/mL | [219] |
B∗ | Concentration of Bcl-2 | 1.0×10−2μM | [220] |
X∗ | Concentration of BAX | 351μM | [221] |
N∗ | Concentration of Neupogen | 50ng/mL | [222,223] |
Let variables C(t),N1(t),N2(t),G(t),L(t),S(t), Kend(t), Kex(t), S1(t),S3(t),B(t), and X(t) be densities of cancer cells, N1 and N2 TANs, TGF-β, TGF-β inhibitor, IFN-γ, endogenous and exogenous NK cells, and activities of STAT1, STAT3, Bcl-2, and BAX, respectively, at time t. The governing equations for the rate change of all variables are
dCdt=rC(1−CC0)−δ1N1C−δ2CI{B<thB,X>thX}−δ3C(Kend+Kex), | (A1) |
dN1dt=λS1S+k3k24k24+βN22−μN1N1, | (A2) |
dN2dt=λ6+λGG+k1k22k22+αN21−μN2N2, | (A3) |
dGdt=λCC−γLLG−μGG, | (A4) |
dLdt=uL(t)−μLL, | (A5) |
dSdt=uS(t)+λKKend+λK′Kex−μSS, | (A6) |
dKenddt=λNK+k5k26k26+θG2−μKKend, | (A7) |
dKexdt=uK(t)−μK′Kex. | (A8) |
dS1dt=λS2S+a1a22a22+γS23−μS1S1, | (A9) |
dS3dt=λ1K1+λS3S+a3a24a24+δS21−μS3S3, | (A10) |
dBdt=λ2+a5a26a26+ωS21+λ3S3−μBB, | (A11) |
dXdt=λ4+a7a28a28+ζB2−μXX. | (A12) |
By defining following and using reference values of each variable in Table A1
ˉt=τt,ˉC=CC∗,¯N2=N2N∗2,¯N1=N1N∗1,ˉG=GG∗,ˉL=LL∗,ˉS=SS∗,ˉKend=KendK∗,¯Kex=KexK∗,ˉμN2=μN2τ,ˉμN1=μN1τˉμG=μGτ,ˉμL=μLτ,ˉμS=μSτ,ˉμK=μKτ,ˉμK′=μK′τ,ˉr=rτ,¯C0=C0C∗,¯δ1=δ1N∗1τ,¯δ2=δ2τ,¯δ3=δ3K∗τ,¯λ6=λ6τN∗2,¯λG=λGG∗τN∗2,¯k1=k1τN∗2,¯k2=k2,ˉα=α(N∗1)2,ˉλS1=λS1S∗τN∗1,¯k3=k3τN∗1,¯k4=k4,ˉβ=β(N∗2)2,¯λC=λCC∗τG∗,¯γL=γLL∗τ,ˉuL(t)=uL(t)τL∗,ˉuS(t)=uS(t)τS∗,¯λK=λKK∗τS∗,¯λK′=λK′K∗τS∗,¯λNK=λNKτK∗,¯k5=k5τK∗,¯k6=k6,ˉθ=θ(G∗)2,ˉuK(t)=uK(t)τK∗,¯S1=S1S∗1,¯S3=S3S∗3,ˉB=BB∗,ˉX=XX∗,ˉS=SS∗,ˉμS1=μS1τ,ˉμS3=μS3τˉμB=μBτ,ˉμX=μXτ,ˉλS2=λS2S∗τS∗1,¯a1=a1τS∗1,¯a2=a2,ˉγ=γ(S∗3)2,¯λ1=λ1τS∗3,¯K1=K1,ˉλS3=λS3S∗,¯a3=a3τS∗3,¯a4=a4,ˉδ=δ(S∗1)2,¯λ2=λ2τB∗,¯a5=a5τB∗,¯a6=a6,ˉω=ω(S∗1)2,¯λ3=λ3S∗3τB∗,¯λ4=λ4τX∗,¯a7=a7τX∗,¯a8=a8,ˉζ=ζ(B∗)2. | (A13) |
we get the dimensionless equations as follows:
dˉCdˉt=ˉrˉC(1−ˉC¯C0)−¯δ1¯N1ˉC−¯δ2ˉCI{B<thB,X>thX}−¯δ3ˉC(ˉKend+ˉKex), | (A14) |
d¯N1dˉt=ˉλS1ˉS+¯k3¯k42¯k42+ˉβ¯N22−ˉμN1¯N1, | (A15) |
d¯N2dˉt=¯λ6+¯λGˉG+¯k1¯k22¯k22+ˉα¯N12−ˉμN2¯N2, | (A16) |
dˉGdˉt=¯λCˉC−¯γLˉLˉG−ˉμGˉG, | (A17) |
dˉLdˉt=ˉuL(t)−ˉμLˉL, | (A18) |
dˉSdˉt=ˉuS(t)+¯λKˉKend+¯λK′ˉKex−ˉμSˉS, | (A19) |
dˉKenddˉt=¯λNK+¯k5¯k62¯k62+ˉθˉG2−ˉμKˉKend, | (A20) |
dˉKexdˉt=ˉuK(t)−ˉμK′ˉKex. | (A21) |
d¯S1dˉt=ˉλS2ˉS+¯a1¯a22¯a22+ˉγ¯S32−ˉμS1¯S1, | (A22) |
d¯S3dˉt=¯λ1¯K1+ˉλS3ˉS+¯a3¯a42¯a42+ˉδ¯S12−ˉμS3¯S3, | (A23) |
dˉBdˉt=¯λ2+¯a5¯a62¯a62+ˉω¯S12+¯λ3¯S3−ˉμBˉB, | (A24) |
dˉXdˉt=¯λ4+¯a7¯a82¯a82+ˉζˉB2−ˉμXˉX. | (A25) |
The parameter estimation for the N2/N1 TAN modules and STAT signaling module is conducted based on biological observations, literature data, and mathematical assumptions to ensure an accurate representation of tumor-immune interactions. Key parameters were chosen to reflect the autocatalytic effects, inhibition mechanisms, and transition dynamics. The estimation process aimed to capture the essential behaviors of the system, including positive feedback loops, mutual inhibition, and phenotypic transitions, while maintaining biological realism. Below, we outline the key parameter values and their derivations.
μN2, μN1: The half-life of TANs within the circulation (T1/2) was reported to be 20 hours [193,194]. By taking T1/2=20h, we get μN2=μN1=ln(2)T1/2∼0.035h−1.
λ6: The reference value for N2 TANs is taken as N∗2=1.0×10−4g/cm3, with a signal source of N2 TANs by IL6 (3.5×10−8g/cm3⋅h), we estimate the dimensionless value of signaling strength to the N2 TANs to be λ6=3.5×10−8g/cm3⋅h1h⋅1.0×10−4g/cm3=3.5×10−4.
k1, k3: We take the dimensionless parameter value k1=0.14 by assuming that the autocatalytic rate of N2 TANs is at least 4-fold larger than its negative contribution from natural decay (μN2N2∗) in the absence of the inhibitory by N1 TANs. This assumption is based on the ratio k1μN2=0.140.035=4, ensuring that the self-enhancement of N2 TANs significantly outweighs their decay dynamics. In a similar fashion, we get the autocatalytic rate of N1 TANs, k3=0.14.
k2, k4: The Hill-type coefficients (k2,k4) are fixed to be unity.
μS1, μS3: The half-life of STAT family within the circulation (T1/2) was reported to be 24 hours [203,204]. By taking T1/2=24h, we get μS1=μS3=ln(2)T1/2∼0.0289h−1.
μB: The half-life of Bcl-2 within the circulation (T1/2) was reported to be 20 hours [205,206]. By taking T1/2=20h, we get μB=ln(2)T1/2∼0.0347h−1.
μX: The half-life of BAX within the circulation (T1/2) was reported to be 4.8 hours [207,208]. By taking T1/2=4.8h, we get μX=ln(2)T1/2∼0.1445h−1.
λ2, λ4: The reference value for Bcl-2 is taken as B∗=0.01μM=2.6×10−7g/cm3 (molecular weight of Bcl-2 is 26kDa), with a signal source of Bcl-2 (1.508×10−9g/cm3⋅h), we estimate the dimensionless value of signaling strength to the Bcl-2 to be λ2=1.508×10−9g/cm3⋅h1h⋅2.6×10−7g/cm3=0.0058. Similarly, by taking the reference value of BAX (X∗=351μM=7.371×10−3g/cm3), and taking the signal source of BAX (4.3×10−5g/cm3⋅h), we estimate the dimensionless value of signaling source to be λ4=4.3×10−5g/cm3⋅h1h⋅7.371×10−3g/cm3=0.0058.
a5, λ3: We take the dimensionless value a5=0.0289 by assuming that the autocatalytic rate of Bcl-2 is same as its negative contribution (μS1B∗) in the absence of the inhibitory by STAT1. This assumption is based on the ratio a5μS1=0.02890.0289=1, ensuring that the self-enhancement of Bcl-2 significantly same their decay dynamics. Additionally, we assume that the STAT3-induced signaling rate is at least 1.2-fold larger than its negative contribution (μS1B∗). We estimate the dimensionless parameter value of STAT3-induced signaling rate λ3=1.2×μS1B∗=0.0347.
a1, a3, a7: The dimensionless parameter a1 is estimated as a1=4.0×μS1S∗1=0.1156 by assuming that the autocatalytic rate a1 of STAT1 is at least 4-fold greater than its negative contribution, in the absence of inhibition by STAT3. Using the same assumption, we obtain: a3=4.0×μS1S∗1=0.1156 and a7=4.0×μS1S∗1=0.1156.
a2, a4, a6, a8: The Hill-type coefficients are fixed to be unity.
μS: The half-life of IFN-γ within the circulation (T1/2) was reported to be 5 hours [197]. By taking T1/2=5h, we get μS=ln(2)T1/2∼0.1386h−1.
μG: The half-life of TGF-β within the circulation (T1/2) was reported to be 24 hours [8,91,100,195]. By taking T1/2=24h, we get μG=ln(2)T1/2∼0.0289h−1.
μL: The half-life of TGF-β inhibitor within the circulation (T1/2) was reported to be 3 hours [196]. By taking T1/2=3h, we get μL=ln(2)T1/2∼0.231h−1.
μK: The half-life of NK cells within the circulation (T1/2) was reported to be 170 hours [198,199]. By taking T1/2=170h, we get μK=ln(2)T1/2∼0.0041h−1.
μN: The half-life of neupogen within the circulation (T1/2) was reported to be 4 hours [200,201]. By taking T1/2=4h, we get μN=ln(2)T1/2∼0.1773h−1.
In this section, we focus on two key interacting subsystems that primarily govern tumor phenotype regulation in the tumor microenvironment (TME): (ⅰ) N1/N2 TANs dynamics in the presence NK cells in response to varying TGF-β, and (ⅱ) intracellular, apoptosis signaling pathway in the presence of NK cells. These two subsystems are linked through shared cytokines and NK cell interactions, but their stability properties with respect to cytokine and NK cell levels in the subsystem are a crucial part of comprehensive understanding of the whole system due to their distinct functional roles. This approach aligns with a quasi-steady-state assumption, where we evaluate stability by fixing certain variables at physiologically relevant levels to determine the possible stable phenotypic outcomes.
The dynamics of the tumor-associated neutrophils (TANs) is essentially governed by ordinary differential equations:
dN1dt=λS1S+k3k24k24+βN22−μN1N1=F1(N1,N2,S,Kend), | (A26) |
dN2dt=λ6+λGG+k1k22k22+αN21−μN2N2=F2(N1,N2,S,Kend), | (A27) |
dSdt=λKKend−μSS=F3(N1,N2,S,Kend), | (A28) |
dKenddt=λNK+k5k26k26+θG2−μKKend=F4(N1,N2,S,Kend). | (A29) |
Then, the equilibrium state of Eqs (A27)–(A29) satisfies
NE1=(λS1SE+k3k24k24+β(NE2)2)1μN1, | (A30) |
NE2=(λ6+λGG+k1k22k22+α(NE1)2)1μN2, | (A31) |
SE=(λKKEend)1μS, | (A32) |
KEend=(λNK+k5k26k26+θG2)1μK. | (A33) |
for low (G=0), intermediate (G=0.4), and high (G=1) values of G. The Jacobian matrix, J, is given by:
J=[∂F1∂N1∂F1∂N2∂F1∂S∂F1∂Kend∂F2∂N1∂F2∂N2∂F2∂S∂F2∂Kend∂F3∂N1∂F3∂N2∂F3∂S∂F3∂Kend∂F4∂N1∂F4∂N2∂F4∂S∂F4∂Kend]=[−μN1−2k3k24βNE2(k24+β(NE2)2)2λS10−2k1k22αNE1(k22+α(NE1)2)2−μN20000−μSλK000−μK] | (A34) |
Then, the characteristic polynomial is given by
det(J−ΛI)=(Λ+μS)(Λ+μK)(Λ2+(μN2+μN1)Λ+μN2μN1−AB)=0 | (A35) |
where I is the identity matrix and defines A=−2k1k22αNE1(k22+α(NE1)2)2 and B=−2k3k24βNE2(k24+β(NE2)2)2. We get two real eigenvalues (Λ1=−μS and Λ2=−μK) and remaining two real eigenvalue (Λ3,4=12(−(μN2+μN1)±√(μN2−μN1)2+4AB)). Here, since μN2=μN1, we have Λ3,4=−μN2±√AB (or −μN1±√AB). Since all of our parameters are positive, AB>0. Therefore, Λ4(=−μN2−√AB)<0, and we can determine the stability of the equilibrium point by examining the sign of Λ3(=−μN2+√AB). If AB<μ2N2, the Λ3<0 and the equilibrium is stable. On contrary, if AB>μ2N2, the Λ3>0 and the equilibrium is unstable. By substituting parameters in the model, we get The Jacobian matrix, J, is given by:
J=[−0.035B0.0350A−0.0350000−0.13860.0277000−0.0041] | (A36) |
where A=−21NE150((1+1.5(NE1)2)2) and B=−14NE250((1+(NE2)2)2). When G=0, we get Λ3=−0.0245, leading to a stable steady state. Also, the high level of TGFβ (G=1) leads to a stable state (Λ3=−0.0185<0). For an intermediate level of TGFβ (G=0.4 in the the bi-stability window), we get Λ3=−0.0095 when (NE1,NE2,SE,KEend)≈(2.7635,0.7311,0.1569,0.7852), Λ3=0.0053 when (NE1,NE2,SE,KEend)≈(1.1780,1.7081,0.1569,0.7852), and Λ3=−0.0090 when (NE1,NE2,SE,KEend)≈(0.4764,3.3939,0.1569,0.7852), leading two stable steady state and one unstable steady state.
We first examine Λ3, whose sign is determined by A and B while Λ1<0,Λ2<0,Λ4 for various TGF-β values. However, the sign of Λ3(=−μN2+√AB) can be either positive or negative, depending on the equilibrium point that is determined by G. Figure A1A shows the third eigenvalue (Λ3) for various TGF-β values. Figure A1B,C shows the steady state of the densities of N1 TANs and N2 TANs for various Gs, respectively. Here, the red (or blue) circles represent unstable (or stable) steady states in Figure A1. For example, the steady states on the middle branch of the bifurcation curves for N2 TANs (Figure A1B) and N1 TANs (Figure A1C) are unstable due to the positive eigenvalues (Figure A1A).
We also investigated the bifurcation curves for NK cells in the main text (Figure 4 in main text). To investigate the bifurcation curves for NK cells, it is necessary to fix the level of TGF-β (G) and obtain the eigenvalue using equation of tumor-associated neutrophils (N1 TANs and N2 TANs) and IFN-γ:
dN1dt=λS1S+k3k24k24+βN22−μN1N1=G1(N1,N2,S), | (A37) |
dN2dt=λ6+λGG+k1k22k22+αN21−μN2N2=G2(N1,N2,S), | (A38) |
dSdt=λKKend−μSS=G3(N1,N2,S). | (A39) |
The Jacobian matrix, J1, is given by:
J1=[∂G1∂N1∂G1∂N2∂G1∂S∂G2∂N1∂G2∂N2∂G2∂S∂G3∂N1∂G3∂N2∂G3∂S]=[−μN1−2k3k24βNE2(k24+β(NE2)2)2λS1−2k1k22αNE1(k22+α(NE1)2)2−μN2000−μS] | (A40) |
Then, the characteristic polynomial is given by
det(J1−ΛI)=(Λ+μS)(Λ2+(μN2+μN1)Λ+μN2μN1−AB)=0 | (A41) |
where A=−2k1k22αNE1(k22+α(NE1)2)2 and B=−2k3k24βNE2(k24+β(NE2)2)2. We get one real eigenvalue (Λ1=−μS) and remaining two eigenvalue (Λ2,3=−μN2±√AB since μN2=μN1). Since all of our parameters are positive, AB is also always positive as well. Therefore, Λ3(=−μN2−√AB) is always negative, and we can determine the stability of the equilibrium point by examining the sign of Λ2(=−μN2+√AB). In this case, Λ1,3 are always negative real number for various NK cells at the intermediate level of TGF-β (G=0.5). Figure A2A shows the second eigenvalue (Λ2) for various NK cells at G=0.5. Figure A2B,C shows the steady state of the densities of N1 TANs and N2 TANs for various NK cells at G=0.5. Also, the second eigenvalue is positive at middle branch steady state of the bifurcation curves and the equilibrium is unstable.
Finally, we investigate stability analysis of intracellular signaling (STAT signaling) for various NK cells.
dS1dt=λS2S+a1a22a22+γS23−μS1S1=H1(S1,S3,B,X,S), | (A42) |
dS3dt=λ1K1+λS3S+a3a24a24+δS21−μS3S3=H2(S1,S3,B,X,S), | (A43) |
dBdt=λ2+a5a26a26+ωS21+λ3S3−μBB=H3(S1,S3,B,X,S), | (A44) |
dXdt=λ4+a7a28a28+ζB2−μXX=H4(S1,S3,B,X,S), | (A45) |
dSdt=λKKend−μSS=H5(S1,S3,B,X,S). | (A46) |
At the equilibrium (SE1,SE3,BE,XE,SE) of Eqs (A42)–(A46), we have
SE1=(λS2S+a1a22a22+γ(SE3)2)1μS1, | (A47) |
SE3=(λ1K1+λS3S+a3a24a24+δ(SE1)2)1μS3, | (A48) |
BE=(λ2+a5a26a26+ω(SE1)2+λ3SE3)1μB, | (A49) |
XE=(λ4+a7a28a28+ζ(BE)2)1μX, | (A50) |
SE=(λKKend)1μS, | (A51) |
J=[∂H1∂S1∂H1∂S3∂H1∂B∂H1∂X∂H1∂S∂H2∂S1∂H2∂S3∂H2∂B∂H2∂X∂H2∂S∂H3∂S1∂H3∂S3∂H3∂B∂H3∂X∂H3∂S∂H4∂S1∂H4∂S3∂H4∂B∂H4∂X∂H4∂S∂H5∂S1∂H5∂S3∂H5∂B∂H5∂X∂H5∂S]=[−μS1−2a1a22γSE3(a22+γ(SE3)2)200λS2−2a3a24δSE1(a24+δ(SE1)2)2−μS300−λ1λS3(K1+λS3S)2−2a5a26ωSE1(a26+ω(SE1)2)2λ3−μB0000−2a7a28ζBE(a28+ζ(BE)2)2−μX00000−μS] | (A52) |
Then, the characteristic polynomial is given by
det(J2−ΛI)=(Λ+μS)(Λ+μB)(Λ+μX)(Λ2+(μS1+μS3)Λ+μS1μS3−AB)=0 | (A53) |
where A=−2a1a22γSE3(a22+γ(SE3)2)2 and B=−2a3a24δSE1(a24+δ(SE1)2)2. We get three negative real eigenvalues (Λ1=−μS<0, Λ2=−μB<0, Λ3=−μX<0) and two remaining eigenvalues Λ4,5 (Λ4,5=−μS1±√AB since μS1=μS3). Since all of our parameters and steady states are positive, AB>0. Therefore, Λ1,2,3 and Λ5(=−μS1−√AB) are always negative, and we can determine the stability of the equilibrium point by examining the sign of Λ4(=−μS1+√AB). Figure A3A shows the fourth eigenvalue (Λ4) for various Kend. The red (or blue) circles represent the unstable (or stable) steady states. Figure A3B,C shows steady states of apoptotic cells (Bcl-2 and BAX, respectively) for various Kend. The positive fourth eigenvalue along the middle branch of the bifurcation curves indicates the unstable status of the intracellular signaling network.
Angiogenesis: the development of new blood vessels, especially in tissues where circulation has been impaired by trauma or disease, e.g., cancer.
Apoptosis: programmed cell death characterized by nuclear breakdown and removal of remains by phagocytes.
BAX: Bcl2 Associated X.
Bcl-2: B-cell lymphoma 2.
BED: Biologically effective dose.
c-MYC: c-Myelocytomatosis oncogene.
CSF-1: Colony stimulating factor 1.
Cytokine: extracellular signaling protein that acts as a local mediator in cellecell communication. Those involved in taxis are sometimes called chemokines.
ECM: Extracellular matrix.
GOF: gain of function.
Growth factor: an extracellular signaling molecule that stimu- lates a cell to grow or proliferate, e.g., vascular endothelial growth factor (VEGF) and fibroblast growth factor (FGF).
IFN-γ: Interferon gamma.
IGRT: Image guided radiation therapy.
IL-6: Interleukin 6.
IMRT: Intensity modulated radiation therapy.
IR: Ionizing radiation.
LOF: loss of function.
LUAD: Lung adenocarcinoma.
LUSC: Lung squamous cell carcinoma.
Metastasis: the process by which cancer spreads from the site of initiation of the primary tumor to distant locations in the body. This occurs via either the circulatory system or the lymphatic system.
MHC: Major histocompatibility complex.
mTORC1: mammalian target of rapamycin complex 1.
NETs: Neutrophil extracellular traps.
Neutropenia: Low neutrophil count.
NFκB: Nuclear factor kappa-light-chain-enhancer of activated B cells.
NK cells: Natural killer cells.
NLR: Neutrophil to lymphocyte ratio.
NSCLC: Non-small cell lung cancer.
ODE: ordinary differential equation.
PDE: partial differential equation.
RT: Radiation therapy.
SREBP: Sterol Regulatory Element-Binding Protein.
STAT: Signal Transducer and Activator of Transcriptions.
TAMs: Tumor-associated macrophages.
TANs: Tumor-associated neutrophils.
TGF-β: Transforming growth factor-beta.
TILs: Tumor-infiltrating lymphocytes TME: Tumor microenvironment.
TNF-α: Tumor necrosis factor-α.
VMAT: Volumetric modulated arc therapy.
[1] |
H. Sung, J. Ferlay, R. Siegel, M. Laversanne, I. Soerjomataram, A. Jemal, et al., Global cancer statistics 2020: GLOBOCAN estimates of incidence and mortality worldwide for 36 cancers in 185 countries, CA Cancer J. Clin., 71 (2021), 209–249. https://doi.org/10.3322/caac.21660 doi: 10.3322/caac.21660
![]() |
[2] |
J. Molina, P. Yang, S. Cassivi, S. Schild, A. Adjei, Non-small cell lung cancer: epidemiology, risk factors, treatment, and survivorship, Mayo Clin. Proc., 83 (2008), 584–594. https://doi.org/10.4065/83.5.584 doi: 10.4065/83.5.584
![]() |
[3] |
N. Altorki, G. Markowitz, D. Gao, J. Port, A. Saxena, B. Stiles, et al., The lung microenvironment: an important regulator of tumour growth and metastasis, Nat. Rev. Cancer, 19 (2019), 9–31. https://doi.org/10.1038/s41568-018-0081-9 doi: 10.1038/s41568-018-0081-9
![]() |
[4] |
X. Yan, S. Jiao, G. Zhang, Y. Guan, J. Wang, Tumor-associated immune factors are associated with recurrence and metastasis in non-small cell lung cancer, Cancer Gene Ther., 24 (2017), 57–63. https://doi.org/10.1038/cgt.2016.40 doi: 10.1038/cgt.2016.40
![]() |
[5] |
D. Lambrechts, E. Wauters, B. Boeckx, S. Aibar, D. Nittner, O. Burton, et al., Phenotype molding of stromal cells in the lung tumor microenvironment, Nat. Med., 24 (2018), 1277–1289. https://doi.org/10.1038/s41591-018-0096-5 doi: 10.1038/s41591-018-0096-5
![]() |
[6] |
A. Mantovani, S. Sozzani, M. Locati, P. Allavena, A. Sica, Macrophage polarization: tumor-associated macrophages as a paradigm for polarized M2 mononuclear phagocytes, Trends Immunol., 23 (2002), 549–555. https://doi.org/10.1016/S1471-4906(02)02302-5 doi: 10.1016/S1471-4906(02)02302-5
![]() |
[7] |
A. Kilic, R. Landreneau, J. Luketich, A. Pennathur, M. Schuchert, Density of tumor-infiltrating lymphocytes correlates with disease recurrence and survival in patients with large non-small-cell lung cancer tumors, J. Surg. Res., 167 (2011), 207–210. https://doi.org/10.1016/j.jss.2009.08.029 doi: 10.1016/j.jss.2009.08.029
![]() |
[8] |
Y. Kim, H. Jeon, H. Othmer, The role of the tumor microenvironment in glioblastoma: A mathematical model, IEEE Trans. Biomed. Eng., 64 (2017), 519–527. https://doi.org/10.1109/TBME.2016.2637828 doi: 10.1109/TBME.2016.2637828
![]() |
[9] |
A. Yuan, Y. Hsiao, H. Chen, H. Chen, C. Ho, Y. Chen, et al., Opposite effects of M1 and M2 macrophage subtypes on lung cancer progression, Sci. Rep., 5 (2015), 14273. https://doi.org/10.1038/srep14273 doi: 10.1038/srep14273
![]() |
[10] |
M. Shaul, L. Levy, J. Sun, I. Mishalian, S. Singhal, V. Kapoor, et al., Tumor-associated neutrophils display a distinct N1 profile following TGFbeta modulation: A transcriptomics analysis of pro- vs. antitumor TANs, Oncoimmunology, 5 (2016), e1232221. https://doi.org/10.1080/2162402X.2016.1232221 doi: 10.1080/2162402X.2016.1232221
![]() |
[11] |
C. Hagerling, Z. Werb, Neutrophils: Critical components in experimental animal models of cancer, Semin. Immunol., 28 (2016), 197–204. https://doi.org/10.1016/j.smim.2016.02.003 doi: 10.1016/j.smim.2016.02.003
![]() |
[12] |
R. Sionov, Z. Fridlender, Z. Granot, The multifaceted roles neutrophils play in the tumor microenvironment, Cancer Microenviron., 8 (2015), 125–158. https://doi.org/10.1007/s12307-014-0147-5 doi: 10.1007/s12307-014-0147-5
![]() |
[13] |
A. Swierczak, K. Mouchemore, J. Hamilton, R. Anderson, Neutrophils: important contributors to tumor progression and metastasis, Cancer Metast. Rev., 34 (2015), 735–751. https://doi.org/10.1007/s10555-015-9594-9 doi: 10.1007/s10555-015-9594-9
![]() |
[14] |
W. Liang, N. Ferrara, The complex role of neutrophils in tumor angiogenesis and metastasis, Cancer Immunol. Res., 4 (2016), 83–91. https://doi.org/10.1158/2326-6066.CIR-15-0313 doi: 10.1158/2326-6066.CIR-15-0313
![]() |
[15] |
Z. G. Fridlender, J. Sun, S. Kim, V. Kapoor, G. Cheng, L. Ling, et al., Polarization of tumor-associated neutrophil phenotype by TGF-beta: N1 versus N2 TAN, Cancer Cell, 16 (2009), 183–194, https://doi.org/10.1016/j.ccr.2009.06.017. doi: 10.1016/j.ccr.2009.06.017
![]() |
[16] | J. Foekens, C. Ries, M. Look, C. Gippner-Steppert, J. Klijn, M. Jochum, The prognostic value of polymorphonuclear leukocyte elastase in patients with primary breast cancer, Cancer Res., 63 (2003), 337–441. |
[17] | A. Bellocq, M. Antoine, A. Flahault, C. Philippe, B. Crestani, J. Bernaudin, et al., Neutrophil alveolitis in bronchioloalveolar carcinoma: induction by tumor-derived interleukin-8 and relation to clinical outcome, Am. J. Pathol., 152 (1998), 83–92. |
[18] |
J. Atzpodien, M. Reitz, Peripheral blood neutrophils as independent immunologic predictor of response and long-term survival upon immunotherapy in metastatic renal-cell carcinom, Cancer Biother. Radiopharm., 23 (2008), 129–134. https://doi.org/10.1089/cbr.2007.0429 doi: 10.1089/cbr.2007.0429
![]() |
[19] |
H. Schmidt, L. Bastholt, P. Geertsen, I. Christensen, S. Larsen, J. Gehl, et al., Elevated neutrophil and monocyte counts in peripheral blood are associated with poor survival in patients with metastatic melanoma: a prognostic model, Br. J. Cancer, 93 (2005), 273–278. https://doi.org/10.1038/sj.bjc.6602702 doi: 10.1038/sj.bjc.6602702
![]() |
[20] | A. J. Templeton, M. G. McNamara, B. Šeruga, F. Vera-Badillo, P. Aneja, A. Ocaña, et al., Prognostic role of neutrophil-to-lymphocyte ratio in solid tumors: a systematic review and meta-analysis, J. Natl. Cancer Inst., 106 (2014), dju124. |
[21] |
H. Maymani, K. Hess, R. Groisberg, D. Hong, A. Naing, S. Piha-Paul, et al., Predicting outcomes in patients with advanced non-small cell lung cancer enrolled in early phaseimmunotherapy trials, Lung Cancer, 120 (2018), 137–141. https://doi.org/10.1016/j.lungcan.2018.03.020 doi: 10.1016/j.lungcan.2018.03.020
![]() |
[22] |
J. Wang, Y. Jia, N. Wang, X. Zhang, B. Tan, G. Zhang, et al., The clinical significance of tumor-infiltrating neutrophils and neutrophil-to-CD8+ lymphocyte ratio in patients with resectable esophageal squamous cell carcinoma, J. Transl. Med., 12 (2014), 7. https://doi.org/10.1186/1479-5876-12-7 doi: 10.1186/1479-5876-12-7
![]() |
[23] |
R. Dolan, S. McSorley, J. Park, D. Watt, C. Roxburgh, P. Horgan, et al., The prognostic value of systemic inflammation in patients undergoing surgery for colon cancer: comparison of composite ratios and cumulative scores, Br. J. Cancer, 119 (2018), 40–51. https://doi.org/10.1038/s41416-018-0095-9 doi: 10.1038/s41416-018-0095-9
![]() |
[24] |
Y. Tao, L. Ding, G. Yang, J. Qiu, D. Wang, H. Wang, et al., Predictive impact of the inflammation-based indices in colorectal cancer patients with adjuvantchemotherapy, Cancer Med., 7 (2018), 2876–2886. https://doi.org/10.1002/cam4.1542 doi: 10.1002/cam4.1542
![]() |
[25] |
T. Tham, Y. Bardash, S. Herman, P. Costantino, Neutrophil-to-lymphocyte ratio as a prognostic indicator in head and neck cancer: A systematicreview and meta-analysis, Head Neck, 40 (2018), 2546–2557. https://doi.org/10.1002/hed.25324 doi: 10.1002/hed.25324
![]() |
[26] |
Z. Huang, Y. Liu, C. Yang, X. Li, C. Pan, J. Rao, et al., Combined neutrophil/platelet/lymphocyte/differentiation score predicts chemosensitivity in advanced gastric cancer, BMC Cancer, 18 (2018), 515. https://doi.org/10.1186/s12885-018-4414-6 doi: 10.1186/s12885-018-4414-6
![]() |
[27] | D. Galun, A. Bogdanovic, J. D. Kovac, P. Bulajic, Z. Loncar, M. Zuvela, Preoperative neutrophil-to-lymphocyte ratio as a prognostic predictor after curative-intent surgeryfor hepatocellular carcinoma: experience from a developing country, Cancer Manage. Res., 10 (2018), 977–988. |
[28] | J. Kaiser, H. Li, S. North, R. Leibowitz-Amit, J. Seah, N. Morshed, et al., The prognostic role of the change in neutrophil-to-lymphocyte ratio during neoadjuvantchemotherapy in patients with muscle-invasive bladder cancer: A retrospective, multi-institutional study, Bladder Cancer, 4 (2018), 185–194. |
[29] |
M. Zhu, M. Feng, F. He, B. Han, K. Ma, X. Zeng, et al., Pretreatment neutrophil-lymphocyte and platelet-lymphocyte ratio predict clinical outcome and prognosis for cervical cancer, Clin. Chim. Acta, 483 (2018), 296–302. https://doi.org/10.1016/j.cca.2018.05.025 doi: 10.1016/j.cca.2018.05.025
![]() |
[30] | P. Xue, M. Kanai, Y. Mori, T. Nishimura, N. Uza, Y. Kodama, et al., Neutrophil-to-lymphocyte ratio for predicting palliative chemotherapy outcomes in advancedpancreatic cancer patients, Cancer Med., 3 (2014), 406–415. |
[31] |
W. Park, G. Lopes, Perspectives: Neutrophil-to-lymphocyte ratio as a potential biomarker in immune checkpoint inhibitor for non-small-cell lung cancer, Clin. Lung Cancer, 20 (2019), 143–147. https://doi.org/10.1016/j.cllc.2018.12.003 doi: 10.1016/j.cllc.2018.12.003
![]() |
[32] |
T. Tuting, K. de Visser, How neutrophils promote metastasis, Science, 352 (2016), 145–146. https://doi.org/10.1126/science.aaf7300 doi: 10.1126/science.aaf7300
![]() |
[33] |
E. Jalilian, F. Abolhasani-Zadeh, A. Afgar, A. Samoudi, H. Zeinalynezhad, L. Langroudi, Neutralizing tumor-related inflammation and reprogramming of cancer-associated fibroblasts by curcumin in breast cancer therapy, Sci. Rep., 13 (2023), 20770. https://doi.org/10.1038/s41598-023-48073-w doi: 10.1038/s41598-023-48073-w
![]() |
[34] |
G. Evan, E. Harrington, A. Fanidi, H. Land, B. Amati, M. Bennett, Integrated control of cell proliferation and cell death by the c-myc oncogene, Philos. Trans. R. Soc. Lond. B Biol. Sci., 345 (1994), 269–275. https://doi.org/10.1098/rstb.1994.0105 doi: 10.1098/rstb.1994.0105
![]() |
[35] |
T. Libermann, L. Zerbini, Targeting transcription factors for cancer gene therapy, Curr. Gene Ther., 6 (2006), 17–33. https://doi.org/10.2174/156652306775515501 doi: 10.2174/156652306775515501
![]() |
[36] |
M. Marin, A. Karis, P. Visser, F. Grosveld, S. Philipsen, Transcription factor Sp1 is essential for early embryonic development but dispensable for cell growth and differentiation, Cell, 89 (1997), 619–628. https://doi.org/10.1016/S0092-8674(00)80243-3 doi: 10.1016/S0092-8674(00)80243-3
![]() |
[37] |
S. Akira, Functional roles of stat family proteins: lessons from knockout mice, Stem Cells, 17 (1999), 138–146. https://doi.org/10.1002/stem.170138 doi: 10.1002/stem.170138
![]() |
[38] |
H. Yu, D. Pardoll, R. Jove, STATs in cancer inflammation and immunity: a leading role for STAT3, Nat. Rev. Cancer, 9 (2009), 798–809. https://doi.org/10.1038/nrc2734 doi: 10.1038/nrc2734
![]() |
[39] |
J. Chen, J. Zhao, L. Chen, N. Dong, Z. Ying, Z. Cai, et al., STAT1 modification improves therapeutic effects of interferons on lung cancer cells, J. Transl. Med., 13 (2015), 293. https://doi.org/10.1186/s12967-015-0656-0 doi: 10.1186/s12967-015-0656-0
![]() |
[40] |
J. Yang, Y. Liu, X. Mai, S. Lu, L. Jin, X. Tai, STAT1-induced upregulation of LINC00467 promotes the proliferation migration of lung adenocarcinoma cells by epigenetically silencing DKK1 to activate Wnt/β-catenin signaling pathway, Biochem. Biophys. Res. Commun., 514 (2019), 118–126. https://doi.org/10.1016/j.bbrc.2019.04.107 doi: 10.1016/j.bbrc.2019.04.107
![]() |
[41] | C. L. Yang, Y. Y. Liu, Y. G. Ma, Y. X. Xue, D. G. Liu, Y. Ren, et al., Curcumin blocks small cell lung cancer cells migration, invasion, angiogenesis, cell cycle and neoplasia through janus kinase-STAT3 signalling pathway, PLoS One, 7 (2012), e37960. |
[42] |
F. Pezzella, H. Turley, I. Kuzu, M. Tungekar, M. Dunnill, C. Pierce, et al., bcl-2 protein in non-small-cell lung carcinoma, N. Engl. J. Med., 329 (1993), 690–694. https://doi.org/10.1056/NEJM199309023291003 doi: 10.1056/NEJM199309023291003
![]() |
[43] |
J. Pawlowski, A. Kraft, Bax-induced apoptotic cell death, Proc. Natl. Acad. Sci. U.S.A., 97 (2000), 529–531. https://doi.org/10.1073/pnas.97.2.529 doi: 10.1073/pnas.97.2.529
![]() |
[44] | I. Porebska, E. Wyrodek, M. Kosacka, J. Adamiak, R. Jankowska, A. Harlozinska-Szmyrka, Apoptotic markers p53, Bcl-2 and Bax in primary lung cancer, In Vivo, 20 (2006), 599–604. |
[45] |
M. Nielsen, C. Kaestel, K. Eriksen, A. Woetmann, T. Stokkedal, K. Kaltoft, et al., Inhibition of constitutively activated Stat3 correlates with altered Bcl-2/Bax expression and induction of apoptosis in mycosis fungoides tumor cells, Leukemia, 13 (1999), 735–738. https://doi.org/10.1038/sj.leu.2401415 doi: 10.1038/sj.leu.2401415
![]() |
[46] | J. Massague, TGFbeta in cancer, Cell, 134 (2008), 215–230. |
[47] |
C. David, J. Massague, Contextual determinants of TGFβ action in development, immunity and cancer, Nat. Rev. Mol. Cell Biol., 19 (2018), 419–435. https://doi.org/10.1038/s41580-018-0007-0 doi: 10.1038/s41580-018-0007-0
![]() |
[48] |
R. Akhurst, A. Hata, Targeting the TGFβ signalling pathway in disease, Nat. Rev. Drug Discov., 11 (2012), 790–811. https://doi.org/10.1038/nrd3810 doi: 10.1038/nrd3810
![]() |
[49] |
R. Akhurst, R. Derynck, TGF-β signaling in cancer–a double-edged sword, Trends Cell Biol., 11 (2001), S44–S51. https://doi.org/10.1016/S0962-8924(01)82259-5 doi: 10.1016/S0962-8924(01)82259-5
![]() |
[50] |
M. Li, R. Flavell, TGF-β: a master of all T cell trades, Cell, 134 (2008), 392–404. https://doi.org/10.1016/j.cell.2008.07.025 doi: 10.1016/j.cell.2008.07.025
![]() |
[51] |
C. Bellomo, L. Caja, A. Moustakas, Transforming growth factor β as regulator of cancer stemness and metastasis, Br. J. Cancer, 115 (2016), 761–769. https://doi.org/10.1038/bjc.2016.255 doi: 10.1038/bjc.2016.255
![]() |
[52] |
O. Aktas, A. Ozturk, B. Erman, S. Erus, S. Tanju, S. Dilege, Role of natural killer cells in lung cancer, J. Cancer Res. Clin. Oncol., 144 (2018), 997–1003. https://doi.org/10.1007/s00432-018-2635-3 doi: 10.1007/s00432-018-2635-3
![]() |
[53] |
R. Castriconi, C. Cantoni, M. Chiesa, M. Vitale, E. Marcenaro, R. Conte, et al., Transforming growth factor β1 inhibits expression of NKp30 and NKG2D receptors: consequences for the NK-mediated killing of dendritic cells, Proc. Natl. Acad. Sci. U.S.A., 100 (2003), 4120–4125. https://doi.org/10.1073/pnas.0730640100 doi: 10.1073/pnas.0730640100
![]() |
[54] |
M. Shaul, Z. Fridlender, Cancer related circulating and tumor-associated neutrophils - subtypes, sources and function, FEBS J., 285 (2018), 4316–4342. https://doi.org/10.1111/febs.14524 doi: 10.1111/febs.14524
![]() |
[55] |
S. Saha, S. Biswas, Tumor-associated neutrophils show phenotypic and functional divergence in human lung cancer, Cancer Cell, 30 (2016), 11–13. https://doi.org/10.1016/j.ccell.2016.06.016 doi: 10.1016/j.ccell.2016.06.016
![]() |
[56] |
L. Shen, J. Smith, Z. Shen, M. Eriksson, C. Sentman, C. Wira, Inhibition of human neutrophil degranulation by transforming growth factor-β1, Clin. Exp. Immunol., 149 (2007), 155–161. https://doi.org/10.1111/j.1365-2249.2007.03376.x doi: 10.1111/j.1365-2249.2007.03376.x
![]() |
[57] |
L. Andzinski, N. Kasnitz, S. Stahnke, C. Wu, M. Gereke, M. von Kockritz-Blickwede, et al., Type Ⅰ IFNs induce anti-tumor polarization of tumor associated neutrophils in mice and human, Int. J. Cancer, 138 (2016), 1982–1993. https://doi.org/10.1002/ijc.29945 doi: 10.1002/ijc.29945
![]() |
[58] |
J. Jablonska, S. Leschner, K. Westphal, S. Lienenklaus, S. Weiss, Neutrophils responsive to endogenous IFN-β regulate tumor angiogenesis and growth in a mouse tumor model, J. Clin. Invest., 120 (2010), 1151–1164. https://doi.org/10.1172/JCI37223 doi: 10.1172/JCI37223
![]() |
[59] |
F. Wang, S. Zhang, R. Jeon, I. Vuckovic, X. Jiang, A. Lerman, et al., Interferon gamma induces reversible metabolic reprogramming of M1 macrophages to sustain cell viability and pro-inflammatory activity, EBioMedicine, 30 (2018), 303–316. https://doi.org/10.1016/j.ebiom.2018.02.009 doi: 10.1016/j.ebiom.2018.02.009
![]() |
[60] |
J. Catani, R. Medrano, A. Hunger, P. D. Valle, S. Adjemian, D. Zanatta, et al., Intratumoral immunization by p19Arf and interferon-β gene transfer in a heterotopic mouse model of lung carcinoma, Transl. Oncol., 9 (2016), 565–574. https://doi.org/10.1016/j.tranon.2016.09.011 doi: 10.1016/j.tranon.2016.09.011
![]() |
[61] |
J. Swann, Y. Hayakawa, N. Zerafa, K. Sheehan, B. Scott, R. Schreiber, et al., Type Ⅰ IFN contributes to NK cell homeostasis, activation, and antitumor function, J. Immunol., 178 (2007), 7540–7549. https://doi.org/10.4049/jimmunol.178.12.7540 doi: 10.4049/jimmunol.178.12.7540
![]() |
[62] |
Q. Lin, L. Rong, X. Jia, R. Li, B. Yu, J. Hu, et al., IFN-γ-dependent NK cell activation is essential to metastasis suppression by engineered salmonella, Nat. Commun., 12 (2021), 2537. https://doi.org/10.1038/s41467-021-22755-3 doi: 10.1038/s41467-021-22755-3
![]() |
[63] |
M. Masucci, M. Minopoli, M. Carriero, Tumor associated neutrophils. their role in tumorigenesis, metastasis, prognosis and therapy, Front. Oncol., 9 (2019), 1146. https://doi.org/10.3389/fonc.2019.01146 doi: 10.3389/fonc.2019.01146
![]() |
[64] |
Y. Gao, J. Yang, Y. Cai, S. Fu, N. Zhang, X. Fu, et al., IFN-γ-mediated inhibition of lung cancer correlates with PD‐L1 expression and is regulated by PI3K-AKT signaling, Int. J. Cancer, 143 (2018), 931–943. https://doi.org/10.1002/ijc.31357 doi: 10.1002/ijc.31357
![]() |
[65] |
D. Jorgovanovic, M. Song, L. Wang, Y. Zhang, Roles of IFN-γ in tumor progression and regression: a review, Biomark Res., 8 (2020), 49. https://doi.org/10.1186/s40364-020-00228-x doi: 10.1186/s40364-020-00228-x
![]() |
[66] |
J. Cong, H. Wei, Natural killer cells in the lungs, Front. Immunol., 10 (2019), 1416. https://doi.org/10.3389/fimmu.2019.01416 doi: 10.3389/fimmu.2019.01416
![]() |
[67] |
M. Vitale, C. Cantoni, G. Pietra, M. Mingari, L. Moretta, Effect of tumor cells and tumor microenvironment on NK-cell function, Eur. J. Immunol., 44 (2014), 1582–1592. https://doi.org/10.1002/eji.201344272 doi: 10.1002/eji.201344272
![]() |
[68] |
D. Pardoll, The blockade of immune checkpoints in cancer immunotherapy, Nat. Rev. Cancer, 12 (2012), 252–264. https://doi.org/10.1038/nrc3239 doi: 10.1038/nrc3239
![]() |
[69] |
J. Gao, J. Ward, C. Pettaway, L. Shi, S. Subudhi, L. Vence, et al., Vista is an inhibitory immune checkpoint that is increased after ipilimumab therapy in patients with prostate cancer, Nat. Med., 23 (2017), 551–555. https://doi.org/10.1038/nm.4308 doi: 10.1038/nm.4308
![]() |
[70] |
R. Tallerico, C. Garofalo, E. Carbone, A new biological feature of natural killer cells: The recognition of solid tumor-derived cancer stem cells, Front. Immunol., 7 (2016), 179. https://doi.org/10.3389/fimmu.2016.00179 doi: 10.3389/fimmu.2016.00179
![]() |
[71] |
S. Viel, A. Marçais, F. Guimaraes, R. Loftus, J. Rabilloud, M. Grau, et al., TGF-β inhibits the activation and functions of NK cells by repressing the mTOR pathway, Sci. Signal, 9 (2016), ra19. https://doi.org/10.1126/scisignal.aad1884 doi: 10.1126/scisignal.aad1884
![]() |
[72] |
F. Otegbeye, E. Ojo, S. Moreton, N. Mackowski, D. Lee, M. de Lima, et al., Inhibiting TGF-beta signaling preserves the function of highly activated, in vitro expanded natural killer cells in aml and colon cancer models, PLoS One, 13 (2018), e0191358. https://doi.org/10.1371/journal.pone.0191358 doi: 10.1371/journal.pone.0191358
![]() |
[73] |
R. Sun, J. Luo, D. Li, Y. Shu, C. Luo, S. Wang, et al., Neutrophils with protumor potential could efficiently suppress tumor growth after cytokine priming and in presence of normal NK cells, Oncotarget, 5 (2014), 12621–12634. https://doi.org/10.18632/oncotarget.2181 doi: 10.18632/oncotarget.2181
![]() |
[74] |
Y. Kim, J. Yoo, T. Lee, J. Liu, J. Yu, M. Caligiuri, et al., Complex role of NK cells in regulation of oncolytic virus-bortezomib therapy, Proc. Natl. Acad. Sci. U.S.A., 115 (2018), 4927–4932. https://doi.org/10.1073/pnas.1715295115 doi: 10.1073/pnas.1715295115
![]() |
[75] |
S. Lim, T. Kim, J. Lee, C. Sonn, K. Kim, J. Kim, et al., Ex vivo expansion of highly cytotoxic human NK cells by cocultivation with irradiated tumor cells for adoptive immunotherapy, Cancer Res., 73 (2013), 2598–2607. https://doi.org/10.1158/0008-5472.CAN-12-2893 doi: 10.1158/0008-5472.CAN-12-2893
![]() |
[76] |
A. Aspirin, A. de Los Reyes V, Y. Kim, Polytherapeutic strategies with oncolytic virus-bortezomib and adjuvant NK cells in cancer treatment, J. R. Soc. Interface, 18 (2021), 20200669. https://doi.org/10.1098/rsif.2020.0669 doi: 10.1098/rsif.2020.0669
![]() |
[77] |
J. Sia, R. Szmyd, E. Hau, H. Gee, Molecular mechanisms of radiation-induced cancer cell death: A primer, Front. Cell Dev. Biol., 8 (2020), 41. https://doi.org/10.3389/fcell.2020.00041 doi: 10.3389/fcell.2020.00041
![]() |
[78] |
A. Koushik, K. Harish, H. Avinash, Principles of radiation oncology: a beams eye view for a surgeon, Indian J. Surg. Oncol., 4 (2013), 255–262. https://doi.org/10.1007/s13193-013-0231-1 doi: 10.1007/s13193-013-0231-1
![]() |
[79] |
J. Chen, X. Liu, Z. Zeng, J. Li, Y. Luo, W. Sun, et al., Immunomodulation of NK cells by ionizing radiation, Front. Oncol., 10 (2020), 874. https://doi.org/10.3389/fonc.2020.00874 doi: 10.3389/fonc.2020.00874
![]() |
[80] |
S. Formenti, S. Demaria, Combining radiotherapy and cancer immunotherapy: a paradigm shift, J. Natl. Cancer Inst., 105 (2013), 256–265. https://doi.org/10.1093/jnci/djs629 doi: 10.1093/jnci/djs629
![]() |
[81] |
X. Lai, A. Friedman, Mathematical modeling of cancer treatment with radiation and PD-L1 inhibitor, Sci. China Math., 63 (2020), 465–484. https://doi.org/10.1007/s11425-019-1648-6 doi: 10.1007/s11425-019-1648-6
![]() |
[82] |
D. Lonergan, A. Mikulec, M. Hanasono, M. Kita, R. Koch, Growth factor profile of irradiated human dermal fibroblasts using a serum-free method, Plast Reconstr. Surg., 111 (2003), 1960–1968. https://doi.org/10.1097/01.PRS.0000055065.41599.75 doi: 10.1097/01.PRS.0000055065.41599.75
![]() |
[83] |
J. Santibanez, M. Quintanilla, C. Bernabeu, TGF-β/TGF-β receptor system and its role in physiological and pathological conditions, Clin. Sci., 121 (2011), 233–251. https://doi.org/10.1042/CS20110086 doi: 10.1042/CS20110086
![]() |
[84] |
D. Powell, A. Huttenlocher, Neutrophils in the tumor microenvironment, Trends Immunol., 37 (2016), 41–52. https://doi.org/10.1016/j.it.2015.11.008 doi: 10.1016/j.it.2015.11.008
![]() |
[85] |
Y. Kim, D. Lee, J. Lee, S. Lee, S. Lawler, Role of tumor-associated neutrophils in regulation of tumor growth in lung cancer development: A mathematical model, PLoS One, 14 (2019), e0211041. https://doi.org/10.1371/journal.pone.0211041 doi: 10.1371/journal.pone.0211041
![]() |
[86] | E. Demidenko, Mixed Models: Theory and Applications with R, 2nd edition, Wiley Series, 2013. |
[87] | V. Collins, R. Loeffler, H. Tivey, Observations on growth rates of human tumors, Am. J. Roentgenol. Radium. Ther. Nucl. Med., 76 (1956), 988–1000. |
[88] |
A. Tsoularis, J. Wallace, Analysis of logistic growth models, Math. Biosci., 179 (2002), 21–55. https://doi.org/10.1016/S0025-5564(02)00096-2 doi: 10.1016/S0025-5564(02)00096-2
![]() |
[89] |
P. Charles, The gompertz curve as a growth curve, Proc. Natl. Acad. Sci. U.S.A., 18 (1932), 1–8. https://doi.org/10.1073/pnas.18.1.1 doi: 10.1073/pnas.18.1.1
![]() |
[90] |
H. Murphy, H. Jaafari, H. Dobrovolny, Differences in predictions of ode models of tumor growth: a cautionary example, BMC Cancer, 16 (2016), 163. https://doi.org/10.1186/s12885-016-2164-x doi: 10.1186/s12885-016-2164-x
![]() |
[91] |
Y. Kim, J. Wallace, F. Li, M. Ostrowski, A. Friedman, Transformed epithelial cells and fibroblasts/myofibroblasts interaction in breast tumor: a mathematical model and experiments, J. Math. Biol., 61 (2010), 401–421. https://doi.org/10.1007/s00285-009-0307-2 doi: 10.1007/s00285-009-0307-2
![]() |
[92] |
A. Stein, T. Demuth, D. Mobley, M. Berens, L. Sander, A mathematical model of glioblastoma tumor spheroid invasion in a three-dimensional in vitro experiment, Biophys. J., 92 (2007), 356–365. https://doi.org/10.1529/biophysj.106.093468 doi: 10.1529/biophysj.106.093468
![]() |
[93] |
H. Enderling, A. Anderson, M. Chaplain, A. Munro, J. Vaidya, Mathematical modelling of radiotherapy strategies for early breast cancer, J. Theor. Biol., 241 (2006), 158–71. https://doi.org/10.1016/j.jtbi.2005.11.015 doi: 10.1016/j.jtbi.2005.11.015
![]() |
[94] |
J. Weis, M. Miga, T. Yankeelov, Three-dimensional image-based mechanical modeling for predicting the response of breast cancer to neoadjuvant therapy, Comput. Methods Appl. Mech. Eng., 314 (2017), 494–512. https://doi.org/10.1016/j.cma.2016.08.024 doi: 10.1016/j.cma.2016.08.024
![]() |
[95] |
Y. Kim, J. Lee, C. Lee, S. Lawler, Role of senescent tumor cells in building a cytokine shield in the tumor microenvironment: mathematical modeling, J. Math. Biol., 86 (2022), 14. https://doi.org/10.1007/s00285-022-01850-z doi: 10.1007/s00285-022-01850-z
![]() |
[96] |
Y. Kim, D. Lee, S. Lawler, Collective invasion of glioma cells through OCT1 signalling and interaction with reactive astrocytes after surgery, Phil. Trans. R. Soc. B, 375 (2020), 20190390. https://doi.org/10.1098/rstb.2019.0390 doi: 10.1098/rstb.2019.0390
![]() |
[97] |
Y. Kim, J. Lee, D. Lee, H. Othmer, Synergistic effects of bortezomib-OV therapy and anti-invasive strategies in glioblastoma: a mathematical model, Cancers, 11 (2019), 215. https://doi.org/10.3390/cancers11020215 doi: 10.3390/cancers11020215
![]() |
[98] |
H. Enderling, M. Chaplain, A. Anderson, J. Vaidya, A mathematical model of breast cancer development, local treatment and recurrence, J. Theor. Biol., 246 (2007), 245–259. https://doi.org/10.1016/j.jtbi.2006.12.010 doi: 10.1016/j.jtbi.2006.12.010
![]() |
[99] |
D. Corwin, C. Holdsworth, R. Rockne, A. Trister, M. Mrugala, J. Rockhill, et al., Toward patient-specific, biologically optimized radiation therapy plans for the treatment of glioblastoma, PLoS One, 8 (2013), e79115. https://doi.org/10.1371/journal.pone.0079115 doi: 10.1371/journal.pone.0079115
![]() |
[100] |
Y. Kim, A. Friedman, Interaction of tumor with its microenvironment: A mathematical model, Bull. Math. Biol., 72 (2010), 1029–1068. https://doi.org/10.1007/s11538-009-9481-z doi: 10.1007/s11538-009-9481-z
![]() |
[101] | P. Maini, Modelling aspects of tumour metabolism, in Proceedings of the International Congress of Mathematicians 2010 (ICM 2010), (2011), 3091–3104. https://doi.org/10.1142/9789814324359_0181 |
[102] |
Y. Kim, H. Lee, N. Dmitrieva, J. Kim, B. Kaur, A. Friedman, Choindroitinase ABC Ⅰ-mediated enhancement of oncolytic virus spread and anti-tumor efficacy: A mathematical model, PLoS One, 9 (2014), e102499. https://doi.org/10.1371/journal.pone.0102499 doi: 10.1371/journal.pone.0102499
![]() |
[103] |
K. Swanson, E. Alvord, J. Murray, Virtual resection of gliomas: Effect of extent of resection on recurrence, Math. Comput. Modell., 37 (2003), 1177–1190. https://doi.org/10.1016/S0895-7177(03)00129-8 doi: 10.1016/S0895-7177(03)00129-8
![]() |
[104] |
Y. Kim, S. Roh, S. Lawler, A. Friedman, miR451 and AMPK/MARK mutual antagonism in glioma cells migration and proliferation, PLoS One, 6 (2011), e28293. https://doi.org/10.1371/journal.pone.0028293 doi: 10.1371/journal.pone.0028293
![]() |
[105] |
K. Kotredes, A. Gamero, Interferons as inducers of apoptosis in malignant cells, J. Interferon Cytokine Res., 33 (2013), 162–170. https://doi.org/10.1089/jir.2012.0110 doi: 10.1089/jir.2012.0110
![]() |
[106] |
A. Groeger, V. Esposito, A. D. Luca, R. Cassandro, G. Tonini, V. Ambrogi, et al., Prognostic value of immunohistochemical expression of p53, bax, Bcl‐2 and Bcl‐xL in resected non‐small‐cell lung cancers, Histopathology, 44 (2004), 54–63. https://doi.org/10.1111/j.1365-2559.2004.01750.x doi: 10.1111/j.1365-2559.2004.01750.x
![]() |
[107] |
J. Lee, D. Lee, Y. Kim, Mathematical model of stat signalling pathways in cancer development and optimal control approaches, R. Soc. Open Sci., 9 (2021), 210594. https://doi.org/10.1098/rsos.210594 doi: 10.1098/rsos.210594
![]() |
[108] |
C. Huang, C. Chung, T. Hu, J. Chen, P. Liu, C. Chen, Recent progress in TGF-β inhibitors for cancer therapy, Biomed. Pharmacother., 134 (2021), 111046. https://doi.org/10.1016/j.biopha.2020.111046 doi: 10.1016/j.biopha.2020.111046
![]() |
[109] | S. Herbertz, J. S. Sawyer, A. J. Stauber, I. Gueorguieva, K. E. Driscoll, S. T. Estrem, et al., Clinical development of galunisertib (LY2157299 monohydrate), a small molecule inhibitor of transforming growth factor-beta signaling pathway, Drug Des. Dev. Ther., 9 (2015), 4479–4499. |
[110] |
R. Kelley, E. Gane, E. Assenat, J. Siebler, P. Galle, P. Merle, et al., A phase 2 study of galunisertib (TGF-β1 receptor type Ⅰ inhibitor) and sorafenib in patients with advanced hepatocellular carcinoma, Clin. Transl. Gastroenterol., 10 (2019), e00056. https://doi.org/10.14309/ctg.0000000000000056 doi: 10.14309/ctg.0000000000000056
![]() |
[111] |
N. Bjorkstrom, H. Ljunggren, J. Michaelsson, Emerging insights into natural killer cells in human peripheral tissues, Nat. Rev. Immunol., 16 (2016), 310–320. https://doi.org/10.1038/nri.2016.34 doi: 10.1038/nri.2016.34
![]() |
[112] |
J. Lee, K. Park, J. Ryu, H. Bae, A. Choi, H. Lee, et al., Natural killer cell activity for IFN-gamma production as a supportive diagnostic marker for gastric cancer, Oncotarget, 8 (2017), 70431–70440. https://doi.org/10.18632/oncotarget.19712 doi: 10.18632/oncotarget.19712
![]() |
[113] |
Y. Rocca, M. Roberti, E. Julia, M. Pampena, L. Bruno, S. Rivero, et al., Phenotypic and functional dysregulated blood NK cells in colorectal cancer patients can be activated by cetuximab plus IL-2 or IL-15, Front. Immunol., 7 (2016), 413. https://doi.org/10.3389/fimmu.2016.00413 doi: 10.3389/fimmu.2016.00413
![]() |
[114] |
M. Lodoen, L. Lanier, Natural killer cells as an initial defense against pathogens, Curr. Opin. Immunol., 18 (2006), 391–398. https://doi.org/10.1080/08913810608443667 doi: 10.1080/08913810608443667
![]() |
[115] |
J. Siren, T. Sareneva, J. Pirhonen, M. Strengell, V. Veckman, I. Julkunen, et al., Cytokine and contact-dependent activation of natural killer cells by influenza a or sendai virus-infected macrophages, J. Gen. Virol., 85 (2004), 2357–2364. https://doi.org/10.1099/vir.0.80105-0 doi: 10.1099/vir.0.80105-0
![]() |
[116] |
A. Iversen, P. Norris, C. Ware, C. Benedict, Human NK cells inhibit cytomegalovirus replication through a noncytolytic mechanism involving lymphotoxin-dependent induction of IFN-beta, J. Immunol., 175 (2005), 7568–7574. https://doi.org/10.4049/jimmunol.175.11.7568 doi: 10.4049/jimmunol.175.11.7568
![]() |
[117] |
M. Studeny, F. Marini, J. Dembinski, C. Zompetta, M. Cabreira-Hansen, B. Bekele, et al., Mesenchymal stem cells: potential precursors for tumor stroma and targeted-delivery vehicles for anticancer agents, J. Natl. Cancer Inst., 96 (2004), 1593–603. https://doi.org/10.1093/jnci/djh299 doi: 10.1093/jnci/djh299
![]() |
[118] |
M. Chiantore, S. Vannucchi, R. Accardi, M. Tommasino, Z. Percario, G. Vaccari, et al., Interferon-β induces cellular senescence in cutaneous human papilloma virus-transformed human keratinocytes by affecting p53 transactivating activity, PLoS One, 7 (2012), e36909. https://doi.org/10.1371/journal.pone.0036909 doi: 10.1371/journal.pone.0036909
![]() |
[119] |
A. Takaoka, S. Hayakawa, H. Yanai, D. Stoiber, H. Negishi, H. Kikuchi, et al., Integration of interferon-α/β signalling to p53 responses in tumour suppression and antiviral defence, Nature, 424 (2003), 516–523. https://doi.org/10.1038/nature01850 doi: 10.1038/nature01850
![]() |
[120] |
F. Zhang, S. Sriram, Identification and characterization of the interferon-beta-mediated p53 signal pathway in human peripheral blood mononuclear cells, Immunology, 128 (2009), e905–e918. https://doi.org/10.1111/j.1365-2567.2009.03104.x doi: 10.1111/j.1365-2567.2009.03104.x
![]() |
[121] |
D. Ghosh, P. Parida, Interferon therapy in lung cancer: Current perspectives, Curr. Cancer Ther. Rev., 12 (2016), 237–245. https://doi.org/10.1016/j.explore.2016.04.001 doi: 10.1016/j.explore.2016.04.001
![]() |
[122] |
B. Liu, X. Zhu, L. Kong, M. Wang, C. Spanoudis, P. Chaturvedi, et al., Bifunctional TGF-β trap/IL-15 protein complex elicits potent NK cell and CD8+ T cell immunity against solid tumors, Mol. Ther., 29 (2021), 2949–2962. https://doi.org/10.1109/TFUZZ.2020.3009755 doi: 10.1109/TFUZZ.2020.3009755
![]() |
[123] |
J. Marcoe, J. Lim, K. Schaubert, N. Fodil-Cornu, M. Matka, A. McCubbrey, et al., TGF-β is responsible for NK cell immaturity during ontogeny and increased susceptibility to infection during mouse infancy, Nat. Immunol., 13 (2012), 843–850. https://doi.org/10.1038/ni.2388 doi: 10.1038/ni.2388
![]() |
[124] |
R. Rouce, H. Shaim, T. Sekine, G. Weber, B. Ballard, S. Ku, et al., The TGF-β/SMAD pathway is an important mechanism for NK cell immune evasion in childhood B-acute lymphoblastic leukemia, Leukemia, 30 (2016), 800–811. https://doi.org/10.1038/leu.2015.327 doi: 10.1038/leu.2015.327
![]() |
[125] |
S. Regis, A. Dondero, F. Caliendo, C. Bottino, R. Castriconi, NK cell function regulation by TGF-β-induced epigenetic mechanisms, Front. Immunol., 11 (2020), 311. https://doi.org/10.3389/fimmu.2020.00311 doi: 10.3389/fimmu.2020.00311
![]() |
[126] |
T. Laskowski, A. Biederstadt, K. Rezvani, Natural killer cells in antitumour adoptive cell immunotherapy, Nat. Rev. Cancer, 22 (2022), 557–575. https://doi.org/10.1038/s41568-022-00491-0 doi: 10.1038/s41568-022-00491-0
![]() |
[127] |
S. Marino, I. Hogue, C. Ray, D. Kirschner, A methodology for performing global uncertainty and sensitivity analysis in systems biology, J. Theor. Biol., 254 (2008), 178–196. https://doi.org/10.1016/j.jtbi.2008.04.011 doi: 10.1016/j.jtbi.2008.04.011
![]() |
[128] |
J. Fowler, The linear-quadratic formula and progress in fractionated radiotherapy, Br. J. Radiol., 62 (1989), 679–694. https://doi.org/10.1259/0007-1285-62-740-679 doi: 10.1259/0007-1285-62-740-679
![]() |
[129] | G. Wiernik, Fractionation in radiotherapy, Anticancer Res., 3 (1983), 283–297. |
[130] |
S. McMahon, The linear quadratic model: usage, interpretation and challenges, Phys. Med. Biol., 64 (2019), 01TR01. https://doi.org/10.1088/1361-6560/aaf26a doi: 10.1088/1361-6560/aaf26a
![]() |
[131] |
H. Enderling, M. Chaplain, P. Hahnfeldt, Quantitative modeling of tumor dynamics and radiotherapy, Acta Biotheor., 58 (2010), 341–353. https://doi.org/10.1007/s10441-010-9111-z doi: 10.1007/s10441-010-9111-z
![]() |
[132] |
J. Harrold, P. Gisleskog, I. Delor, P. Jacqmin, J. Perez-Ruixo, A. Narayanan, et al., Quantification of radiation injury on neutropenia and the link between absolute neutrophil count time course and overall survival in nonhuman primates treated with G-CSF, Pharm. Res., 37 (2020), 102. https://doi.org/10.1007/s11095-020-02839-3 doi: 10.1007/s11095-020-02839-3
![]() |
[133] |
A. Wisdom, C. Hong, A. Lin, Y. Xiang, D. Cooper, J. Zhang, et al., Neutrophils promote tumor resistance to radiation therapy, Proc. Natl. Acad. Sci. U.S.A., 116 (2019), 18584–18589. https://doi.org/10.1073/pnas.1901562116 doi: 10.1073/pnas.1901562116
![]() |
[134] |
H. Thames, S. Bentzen, I. Turesson, M. Overgaard, W. V. den Bogaert, Time-dose factors in radiotherapy: a review of the human data, Radiother. Oncol., 19 (1990), 219–235. https://doi.org/10.1016/0167-8140(90)90149-Q doi: 10.1016/0167-8140(90)90149-Q
![]() |
[135] |
P. Blake, A. Swart, J. Orton, H. Kitchener, T. Whelan, H. Lukka, et al., Adjuvant external beam radiotherapy in the treatment of endometrial cancer (MRC ASTEC and NCIC CTG EN. 5 randomised trials): pooled trial results, systematic review, and meta-analysis, Lancet, 373 (2009), 137–146. https://doi.org/10.1016/S0140-6736(08)61767-5 doi: 10.1016/S0140-6736(08)61767-5
![]() |
[136] |
S. Faria, W. Schlupp, H. Chiminazzo Jr, Radiotherapy in the treatment of vertebral hemangiomas, Int. J. Radiat. Oncol. Biol. Phys., 11 (1985), 387–390. https://doi.org/10.1016/0360-3016(85)90162-2 doi: 10.1016/0360-3016(85)90162-2
![]() |
[137] | S. Lenhart, J. Workman, Optimal Control Applied to Biological Models, 1st edition, Chapman and Hall/CRC, 2007. |
[138] |
A. Jarrett, D. Faghihi, D. Ii, E. Lima, J. Virostko, G. Biros, et al., Optimal control theory for personalized therapeutic regimens in oncology: Background, history, challenges, and opportunities, J. Clin. Med., 9 (2020), 1314. https://doi.org/10.3390/jcm9051314 doi: 10.3390/jcm9051314
![]() |
[139] |
K. Bahrami, M. Kim, Optimal control of multiplicative control systems arising from cancer therapy, IEEE Trans. Autom. Control, 20 (1975), 537–542. https://doi.org/10.1109/TAC.1975.1101019 doi: 10.1109/TAC.1975.1101019
![]() |
[140] |
G. W. Swan, T. L. Vincent, Optimal control analysis in the chemotherapy of IGG multiple myeloma, Bull. Math. Biol., 39 (1977), 317–337. https://doi.org/10.1016/S0092-8240(77)80070-0 doi: 10.1016/S0092-8240(77)80070-0
![]() |
[141] |
G. W. Swan, Optimal control applications in the chemotherapy of multiple myeloma, Math. Med. Biol., 2 (1985), 139–160. https://doi.org/10.1093/imammb/2.3.139 doi: 10.1093/imammb/2.3.139
![]() |
[142] |
A. Ergun, K. Camphausen, L. Wein, Optimal scheduling of radiotherapy and angiogenic inhibitors, Bull. Math. Biol., 65 (2003), 407–424. https://doi.org/10.1016/S0092-8240(03)00006-5 doi: 10.1016/S0092-8240(03)00006-5
![]() |
[143] |
D. Lee, A. de Los Reyes V, Y. Kim, Optimal strategies of oncolytic virus-bortezomib therapy via the apoptotic, necroptotic, and oncolysis signaling network, Math. Biosci. Eng., 21 (2024), 3876–3909. https://doi.org/10.3934/mbe.2024173 doi: 10.3934/mbe.2024173
![]() |
[144] |
E. Ratajczyk, U. Ledzewicz, H. Schattler, Optimal control for a mathematical model of glioma treatment with oncolytic therapy and TNF-α inhibitors, J. Optim. Theory Appl., 176 (2018), 456–477. https://doi.org/10.1007/s10957-018-1218-4 doi: 10.1007/s10957-018-1218-4
![]() |
[145] | H. Schattler, Y. Kim, U. Ledzewicz, A. los Reyes V, E. Jung, On the control of cell migration and proliferation in glioblastoma, in Proceeding of the IEEE Conference on Decision and Control, (2013), 1810–1815. https://doi.org/10.1109/CDC.2013.6760145 |
[146] |
E. Jung, A. los Reyes, K. Pumares, Y. Kim, Strategies in regulating glioblastoma signaling pathways and anti-invasion therapy, PLoS One, 14 (2019), e0215547. https://doi.org/10.1371/journal.pone.0215547 doi: 10.1371/journal.pone.0215547
![]() |
[147] |
A. L. Reyes, E. Jung, Y. Kim, Optimal control strategies of eradicating invisible glioblastoma cells after conventional surgery, J. Roy. Soc. Interface, 12 (2015), 20141392. https://doi.org/10.1098/rsif.2014.1392 doi: 10.1098/rsif.2014.1392
![]() |
[148] |
A. Reyes, Y. Kim, Optimal regulation of tumour-associated neutrophils in cancer progression, R. Soc. Open Sci., 9 (2022), 210705. https://doi.org/10.1098/rsos.210705 doi: 10.1098/rsos.210705
![]() |
[149] |
G. Boivin, P. Ancey, R. de Silly, P. Kalambaden, C. Contat, B. Petit, et al., Anti-Ly6G binding and trafficking mediate positive neutrophil selection to unleash the anti-tumor efficacy of radiation therapy, Oncoimmunology, 10 (2021), 1876597. https://doi.org/10.1080/2162402X.2021.1876597 doi: 10.1080/2162402X.2021.1876597
![]() |
[150] |
Z. Zhang, X. Liu, D. Chen, J. Yu, Radiotherapy combined with immunotherapy: the dawn of cancer treatment, Signal Transduction Targeted Ther., 7 (2022), 258. https://doi.org/10.1038/s41392-022-01102-y doi: 10.1038/s41392-022-01102-y
![]() |
[151] |
M. Grinde, J. Vik, K. Camilio, I. Martinez-Zubiaurre, T. Hellevik, Ionizing radiation abrogates the pro-tumorigenic capacity of cancer-associated fibroblasts co-implanted in xenografts, Sci. Rep., 7 (2017), 46714. https://doi.org/10.1038/srep46714 doi: 10.1038/srep46714
![]() |
[152] |
O. Al-Assar, F. Demiciorglu, S. Lunardi, M. Gaspar-Carvalho, W. McKenna, R. Muschel, et al., Contextual regulation of pancreatic cancer stem cell phenotype and radioresistance by pancreatic stellate cells, Radiother. Oncol., 111 (2014), 243–251. https://doi.org/10.1016/j.radonc.2014.03.014 doi: 10.1016/j.radonc.2014.03.014
![]() |
[153] |
C. Arteaga, J. Engelman, ERBB receptors: from oncogene discovery to basic science to mechanism-based cancer therapeutics, Cancer Cell, 25 (2014), 282–303. https://doi.org/10.1016/j.ccr.2014.02.025 doi: 10.1016/j.ccr.2014.02.025
![]() |
[154] |
S. Du, S. Bouquet, C. Lo, I. Pellicciotta, S. Bolourchi, R. Parry, et al., Attenuation of the dna damage response by transforming growth factor-beta inhibitors enhances radiation sensitivity of non-small-cell lung cancer cells in vitro and in vivo, Int. J. Radiat. Oncol. Biol. Phys., 91 (2015), 91–99. https://doi.org/10.1016/j.ijrobp.2014.09.026 doi: 10.1016/j.ijrobp.2014.09.026
![]() |
[155] |
E. Dieleman, A. Uitterhoeve, M. van Hoek, R. van Os, J. Wiersma, M. Koolen, et al., Concurrent daily cisplatin and high-dose radiation therapy in patients with stage iii non-small cell lung cancer, Int. J. Radiat. Oncol. Biol. Phys., 102 (2018), 543–551. https://doi.org/10.1016/j.ijrobp.2018.07.188 doi: 10.1016/j.ijrobp.2018.07.188
![]() |
[156] |
S. Vora, B. Daly, L. Blaszkowsky, J. McGrath, M. Bankoff, S. Supran, et al., High dose radiation therapy and chemotherapy as induction treatment for stage iii nonsmall cell lung carcinoma, Cancer, 89 (2000), 1946–1952. https://doi.org/10.1002/1097-0142(20001101)89:9<1946::AID-CNCR10>3.0.CO;2-1 doi: 10.1002/1097-0142(20001101)89:9<1946::AID-CNCR10>3.0.CO;2-1
![]() |
[157] |
F. Kong, R. T. Haken, M. Schipper, M. Sullivan, M. Chen, C. Lopez, et al., High-dose radiation improved local tumor control and overall survival in patients with inoperable/unresectable non-small-cell lung cancer: long-term results of a radiation dose escalation study, Int. J. Radiat. Oncol. Biol. Phys., 63 (2005), 324–333. https://doi.org/10.1016/j.ijrobp.2005.02.010 doi: 10.1016/j.ijrobp.2005.02.010
![]() |
[158] |
M. Manus, K. Lamborn, W. Khan, A. Varghese, L. Graef, S. Knox, Radiotherapy-associated neutropenia and thrombocytopenia: analysis of risk factors and development of a predictive model, Blood, 89 (1997), 2303–2310. https://doi.org/10.1182/blood.V89.7.2303 doi: 10.1182/blood.V89.7.2303
![]() |
[159] |
R. Prabhu, R. Cassidy, J. Landry, Radiation therapy and neutropenia, Curr. Probl. Cancer, 39 (2015), 292–296. https://doi.org/10.1016/j.currproblcancer.2015.07.010 doi: 10.1016/j.currproblcancer.2015.07.010
![]() |
[160] |
T. Smith, J. Khatcheressian, G. Lyman, H. Ozer, J. Armitage, L. Balducci, et al., 2006 update of recommendations for the use of white blood cell growth factors: an evidence-based clinical practice guideline, J. Clin. Oncol., 24 (2006), 3187–3205. https://doi.org/10.1200/JCO.2006.06.4451 doi: 10.1200/JCO.2006.06.4451
![]() |
[161] |
J. Crawford, C. Caserta, F. Roila, Hematopoietic growth factors: ESMO clinical practice guidelines for the applications, Ann. Oncol., 21 (2010), 248–251. https://doi.org/10.1093/annonc/mdq195 doi: 10.1093/annonc/mdq195
![]() |
[162] |
P. Mauch, L. Constine, J. Greenberger, W. Knospe, J. Sullivan, J. Liesveld, et al., Hematopoietic stem cell compartment: acute and late effects of radiation therapy and chemotherapy, Int. J. Radiat. Oncol. Biol. Phys., 31 (1995), 1319–1339. https://doi.org/10.1016/0360-3016(94)00430-S doi: 10.1016/0360-3016(94)00430-S
![]() |
[163] |
A. Farese, T. MacVittie, Filgrastim for the treatment of hematopoietic acute radiation syndrome, Drugs Today (Barc), 51 (2015), 537–548. https://doi.org/10.1358/dot.2015.51.9.2386730 doi: 10.1358/dot.2015.51.9.2386730
![]() |
[164] |
P. Cornes, P. Gascon, S. Chan, K. Hameed, C. Mitchell, P. Field, et al., Systematic review and meta-analysis of short- versus long-acting granulocyte colony-stimulating factors for reduction of chemotherapy-induced febrile neutropenia, Adv. Ther., 35 (2018), 1816–1829. https://doi.org/10.1007/s12325-018-0798-6 doi: 10.1007/s12325-018-0798-6
![]() |
[165] |
Y. Zeng, X. Lv, J. Du, Natural killer cell-based immunotherapy for lung cancer: Challenges and perspectives (review), Oncol. Rep., 46 (2021), 232. https://doi.org/10.3892/or.2021.8183 doi: 10.3892/or.2021.8183
![]() |
[166] |
P. Carrega, B. Morandi, R. Costa, G. Frumento, G. Forte, G. Altavilla, et al., Natural killer cells infiltrating human nonsmall-cell lung cancer are enriched in CD56 bright CD16(-) cells and display an impaired capability to kill tumor cells, Cancer, 112 (2008), 863–875. https://doi.org/10.1002/cncr.23239 doi: 10.1002/cncr.23239
![]() |
[167] |
S. Platonova, J. Cherfils-Vicini, D. Damotte, L. Crozet, V. Vieillard, P. Validire, et al., Profound coordinated alterations of intratumoral NK cell phenotype and function in lung carcinoma, Cancer Res., 71 (2011), 5412–5422. https://doi.org/10.1158/0008-5472.CAN-10-4179 doi: 10.1158/0008-5472.CAN-10-4179
![]() |
[168] |
Y. Laouar, F. Sutterwala, L. Gorelik, R. Flavell, Transforming growth factor-β controls T helper type 1 cell development through regulation of natural killer cell interferon-γ, Nat. Immunol., 6 (2005), 600–607. https://doi.org/10.1038/ni1197 doi: 10.1038/ni1197
![]() |
[169] |
B. Bassani, D. Baci, M. Gallazzi, A. Poggi, A. Bruno, L. Mortara, Natural killer cells as key players of tumor progression and angiogenesis: Old and novel tools to divert their pro-tumor activities into potent anti-tumor effects, Cancers (Basel), 11 (2019), 461. https://doi.org/10.3390/cancers11040461 doi: 10.3390/cancers11040461
![]() |
[170] |
M. Balsamo, W. Vermi, M. Parodi, G. Pietra, C. Manzini, P. Queirolo, et al., Melanoma cells become resistant to NK-cell-mediated killing when exposed to NK-cell numbers compatible with NK-cell infiltration in the tumor, Eur. J. Immunol., 42 (2012), 1833–1842. https://doi.org/10.1002/eji.201142179 doi: 10.1002/eji.201142179
![]() |
[171] |
T. Li, Y. Yang, X. Hua, G. Wang, W. Liu, C. Jia, et al., Hepatocellular carcinoma-associated fibroblasts trigger NK cell dysfunction via PGE2 and IDO, Cancer Lett., 318 (2012), 154–161. https://doi.org/10.1016/j.canlet.2011.12.020 doi: 10.1016/j.canlet.2011.12.020
![]() |
[172] |
T. Li, S. Yi, W. Liu, C. Jia, G. Wang, X. Hua, et al., Colorectal carcinoma-derived fibroblasts modulate natural killer cell phenotype and antitumor cytotoxicity, Med. Oncol., 30 (2013), 663. https://doi.org/10.1007/s12032-013-0663-z doi: 10.1007/s12032-013-0663-z
![]() |
[173] |
C. Granville, R. Memmott, A. Balogh, J. Mariotti, S. Kawabata, W. Han, et al., A central role for Foxp3+ regulatory T cells in K-Ras-driven lung tumorigenesis, PLoS One, 4 (2009), e5061. https://doi.org/10.1371/journal.pone.0005061 doi: 10.1371/journal.pone.0005061
![]() |
[174] |
M. J. Smyth, M. W. Teng, J. Swann, K. Kyparissoudis, D. I. Godfrey, Y. Hayakawa, CD4+ CD25+T regulatory cells suppress NK cell-mediated immunotherapy of cancer, J. Immunol., 176 (2006), 1582–1587. https://doi.org/10.4049/jimmunol.176.3.1582 doi: 10.4049/jimmunol.176.3.1582
![]() |
[175] |
F. Ghiringhelli, C. Menard, M. Terme, C. Flament, J. Taieb, N. Chaput, et al., CD4+CD25+ regulatory T cells inhibit natural killer cell functions in a transforming growth factor-beta-dependent manner, J. Exp. Med., 202 (2005), 1075–1085. https://doi.org/10.1084/jem.20051511 doi: 10.1084/jem.20051511
![]() |
[176] |
A. Page, N. Chuvin, J. Valladeau-Guilemond, S. Depil, Development of NK cell-based cancer immunotherapies through receptor engineering, Cell Mol. Immunol., 21 (2024), 315–331. https://doi.org/10.1038/s41423-024-01145-x doi: 10.1038/s41423-024-01145-x
![]() |
[177] |
J. Markert, P. Liechty, W. Wang, S. Gaston, E. Braz, M. Karrasch, et al., Phase Ib trial of mutant herpes simplex virus G207 inoculated pre-and post-tumor resection for recurrent GBM, Mol. Ther., 17 (2009), 199–207. https://doi.org/10.1038/mt.2008.228 doi: 10.1038/mt.2008.228
![]() |
[178] |
M. Grimes, B. Hall, L. Foltz, T. Levy, K. Rikova, J. Gaiser, et al., Integration of protein phosphorylation, acetylation, and methylation data sets to outline lung cancer signaling networks, Sci. Signal., 11 (2018), eaaq1087. https://doi.org/10.1126/scisignal.aaq1087 doi: 10.1126/scisignal.aaq1087
![]() |
[179] |
J. Qu, Y. Zhang, X. Chen, H. Yang, C. Zhou, N. Yang, Newly developed anti-angiogenic therapy in non-small cell lung cancer, Oncotarget, 9 (2017), 10147–10163. https://doi.org/10.18632/oncotarget.23755 doi: 10.18632/oncotarget.23755
![]() |
[180] |
S. Revels, J. Lee, Anti-angiogenic therapy in nonsquamous non-small cell lung cancer (NSCLC) with tyrosine kinase inhibition (TKI) that targets the VEGF receptor (VEGFR): perspective on phase Ⅲ clinical trials, J. Thorac. Dis., 10 (2018), 617–620. https://doi.org/10.21037/jtd.2018.01.105 doi: 10.21037/jtd.2018.01.105
![]() |
[181] |
M. Stratigos, A. Matikas, A. Voutsina, D. Mavroudis, V. Georgoulias, Targeting angiogenesis in small cell lung cancer, Transl. Lung Cancer Res., 5 (2016), 389–400. https://doi.org/10.21037/tlcr.2016.08.04 doi: 10.21037/tlcr.2016.08.04
![]() |
[182] |
S. Lee, H. Kwak, M. Kang, Y. Park, G. Jeong, Fibroblast-associated tumour microenvironment induces vascular structure-networked tumouroid, Sci. Rep., 8 (2018), 2365. https://doi.org/10.1038/s41598-018-20886-0 doi: 10.1038/s41598-018-20886-0
![]() |
[183] |
B. Lee, J. Konen, S. Wilkinson, A. Marcus, Y. Jiang, Local alignment vectors reveal cancer cell-induced ECM fiber remodeling dynamics, Sci. Rep., 7 (2017), 39498. https://doi.org/10.1038/srep39498 doi: 10.1038/srep39498
![]() |
[184] |
S. Pyonteck, L. Akkari, A. Schuhmacher, R. Bowman, L. Sevenich, D. Quail, et al., CSF-1R inhibition alters macrophage polarization and blocks glioma progression, Nat. Med., 19 (2013), 1264–1272. https://doi.org/10.1038/nm.3337 doi: 10.1038/nm.3337
![]() |
[185] |
S. Arelaki, A. Arampatzioglou, K. Kambas, C. Papagoras, P. Miltiades, I. Angelidou, et al., Gradient infiltration of neutrophil extracellular traps in colon cancer and evidence for their involvement in tumour growth, PLoS One, 11 (2016), e0154484. https://doi.org/10.1371/journal.pone.0154484 doi: 10.1371/journal.pone.0154484
![]() |
[186] |
A. Houghton, D. Rzymkiewicz, H. Ji, A. Gregory, E. Egea, H. Metz, et al., Neutrophil elastase-mediated degradation of IRS-1 accelerates lung tumor growth, Nat. Med., 16 (2010), 219–223. https://doi.org/10.1038/nm.2084 doi: 10.1038/nm.2084
![]() |
[187] |
J. Park, R. Wysocki, Z. Amoozgar, L. Maiorino, M. Fein, J. Jorns, et al., Cancer cells induce metastasis-supporting neutrophil extracellular DNA traps, Sci. Transl. Med., 8 (2016), 361ra138. https://doi.org/10.1126/scitranslmed.aag1711 doi: 10.1126/scitranslmed.aag1711
![]() |
[188] |
A. Chapgier, S. Boisson-Dupuis, E. Jouanguy, G. Vogt, J. Feinberg, A. Prochnicka-Chalufour, et al., Novel STAT1 alleles in otherwise healthy patients with mycobacterial disease, PLoS Genet., 2 (2006), e131. https://doi.org/10.1371/journal.pgen.0020131 doi: 10.1371/journal.pgen.0020131
![]() |
[189] |
P. Klover, W. Muller, G. Robinson, R. Pfeiffer, D. Yamaji, L. Hennighausen, Loss of STAT1 from mouse mammary epithelium results in an increased Neu-induced tumor burden, Neoplasia, 12 (2010), 899–905. https://doi.org/10.1593/neo.10716 doi: 10.1593/neo.10716
![]() |
[190] |
R. Noy, J. Pollard, Tumor-associated macrophages: from mechanisms to therapy, Immunity, 41 (2014), 49–61. https://doi.org/10.1016/j.immuni.2014.06.010 doi: 10.1016/j.immuni.2014.06.010
![]() |
[191] |
A. Mantovani, S. Biswas, M. Galdiero, A. Sica, M. Locati, Macrophage plasticity and polarization in tissue repair and remodelling, J. Pathol., 229 (2013), 176–185. https://doi.org/10.1002/path.4133 doi: 10.1002/path.4133
![]() |
[192] |
B. Yan, J. Wei, Y. Yuan, R. Sun, D. Li, J. Luo, et al., IL-6 cooperates with G-CSF to induce protumor function of neutrophils in bone marrow by enhancing STAT3 activation, J. Immunol., 190 (2013), 5882–5893. https://doi.org/10.4049/jimmunol.1201881 doi: 10.4049/jimmunol.1201881
![]() |
[193] |
K. Steinbach, P. Schick, F. Trepel, H. Raffler, J. Dohrmann, G. Heilgeist, et al., Estimation of kinetic parameters of neutrophilic, eosinophilic, and basophilic granulocytes in human blood, Blut, 39 (1979), 27–38. https://doi.org/10.1007/BF01008072 doi: 10.1007/BF01008072
![]() |
[194] |
D. Dale, W. C. Liles, C. Llewellyn, E. Rodger, T. H. Price, Neutrophil transfusions: kinetics and functions of neutrophils mobilized with granulocyte-colony-stimulating factor and dexamethasone, Transfusion, 38 (1998), 713–721. https://doi.org/10.1046/j.1537-2995.1998.38898375509.x doi: 10.1046/j.1537-2995.1998.38898375509.x
![]() |
[195] |
Y. Kim, H. Othmer, A hybrid model of tumor-stromal interactions in breast cancer, Bull. Math. Biol., 75 (2013), 1304–1350. https://doi.org/10.1007/s11538-012-9787-0 doi: 10.1007/s11538-012-9787-0
![]() |
[196] |
J. Diao, E. Winter, C. Cantin, W. Chen, L. Xu, D. Kelvin, et al., In situ replication of immediate dendritic cell (DC) precursors contributes to conventional DC homeostasis in lymphoid tissue, J. Immunol., 176 (2006), 7196–7206. https://doi.org/10.4049/jimmunol.176.12.7196 doi: 10.4049/jimmunol.176.12.7196
![]() |
[197] |
P. Salmon, J. L. Cotonnec, A. Galazka, A. Abdul-Ahad, A. Darragh, Pharmacokinetics and pharmacodynamics of recombinant human interferon-beta in healthy male volunteers, J. Interferon Cytokine Res., 16 (1996), 759–964. https://doi.org/10.1089/jir.1996.16.759 doi: 10.1089/jir.1996.16.759
![]() |
[198] |
Y. Zhang, D. Wallace, C. de Lara, H. Ghattas, B. Asquith, A. Worth, et al., In vivo kinetics of human natural killer cells: the effects of ageing and acute and chronic viral infection, Immunology, 121 (2007), 258–265. https://doi.org/10.1111/j.1365-2567.2007.02573.x doi: 10.1111/j.1365-2567.2007.02573.x
![]() |
[199] |
K. Stringaris, Orphan NKs! the mystery of the self-renewing NK cells, Blood, 129 (2017), 1890–1891. https://doi.org/10.1182/blood-2016-12-755546 doi: 10.1182/blood-2016-12-755546
![]() |
[200] |
N. Stute, V. Santana, J. Rodman, M. Schell, J. Ihle, W. Evans, Pharmacokinetics of subcutaneous recombinant human granulocyte colony-stimulating factor in children, Blood, 79 (1992), 2849–2854. https://doi.org/10.1182/blood.V79.11.2849.2849 doi: 10.1182/blood.V79.11.2849.2849
![]() |
[201] |
E. Shochat, V. Rom-Kedar, L. Segel, G-CSF control of neutrophils dynamics in the blood, Bull. Math. Biol., 69 (2007), 2299–2338. https://doi.org/10.1007/s11538-007-9221-1 doi: 10.1007/s11538-007-9221-1
![]() |
[202] |
L. Deng, H. Liang, M. Xu, X. Yang, B. Burnette, A. Arina, et al., Sting-dependent cytosolic dna sensing promotes radiation-induced type Ⅰ interferon-dependent antitumor immunity in immunogenic tumors, Immunity, 41 (2014), 843–52. https://doi.org/10.1016/j.immuni.2014.10.019 doi: 10.1016/j.immuni.2014.10.019
![]() |
[203] |
J. Andrejeva, D. Young, S. Goodbourn, R. Randall, Degradation of STAT1 and STAT2 by the Ⅴ proteins of simian virus 5 and human parainfluenza virus type 2, respectively: consequences for virus replication in the presence of alpha/beta and gamma interferons, J. Virol., 76 (2002), 2159–2167. https://doi.org/10.1128/jvi.76.5.2159-2167.2002 doi: 10.1128/jvi.76.5.2159-2167.2002
![]() |
[204] | L. Yang, R. Wang, Z. Ma, Y. Xiao, Y. Nan, Y. Wang, et al., Porcine reproductive and respiratory syndrome virus antagonizes JAK/STAT3 signaling via nsp5 by inducing STAT3 degradation, J. Virol., 91 (2017), e02087–16. |
[205] |
R. Rooswinkel, B. van de Kooij, E. de Vries, M. Paauwe, R. Braster, et al., Antiapoptotic potency of Bcl-2 proteins primarily relies on their stability, not binding selectivity, Blood, 123 (2014), 2806–2815. https://doi.org/10.1182/blood-2013-08-519470 doi: 10.1182/blood-2013-08-519470
![]() |
[206] |
M. Blagosklonny, M. Alvarez, A. Fojo, L. Neckers, bcl-2 protein downregulation is not required for differentiation of multidrug resistant HL60 leukemia cells, Leuk. Res., 20 (1996), 101–107. https://doi.org/10.1016/0145-2126(95)00103-4 doi: 10.1016/0145-2126(95)00103-4
![]() |
[207] |
S. Magal, A. Jackman, S. Ish-Shalom, L. Botzer, P. Gonen, R. Schlegel, et al., Downregulation of Bax mRNA expression and protein stability by the E6 protein of human papillomavirus 16, J. Gen. Virol., 86 (2005), 611–621. https://doi.org/10.1099/vir.0.80453-0 doi: 10.1099/vir.0.80453-0
![]() |
[208] |
M. Xin, X. Deng, Nicotine inactivation of the proapoptotic function of Bax through phosphorylation, J. Biol. Chem., 280 (2005), 10781–10789. https://doi.org/10.1074/jbc.M500084200 doi: 10.1074/jbc.M500084200
![]() |
[209] |
E. Gaffney, K. Pugh, P. Maini, F. Arnold, Investigating a simple model of cutaneous wound healing angiogenesis, J. Math. Biol., 45 (2002), 337–374. https://doi.org/10.1007/s002850200161 doi: 10.1007/s002850200161
![]() |
[210] |
G. Pettet, H. Byrne, D. McElwain, J. Norbury, A model of wound-healing angiogenesis in soft tissue, Math. Biosci., 136 (1996), 35–63. https://doi.org/10.1016/0025-5564(96)00044-2 doi: 10.1016/0025-5564(96)00044-2
![]() |
[211] |
P. Moghe, R. Nelson, R. Tranquillo, Cytokine-stimulated chemotaxis of human neutrophils in a 3-D conjoined fibrin gel assay, J. Immunol. Methods, 180 (1995), 193–211. https://doi.org/10.1016/0022-1759(94)00314-M doi: 10.1016/0022-1759(94)00314-M
![]() |
[212] |
D. Brown, Dependence of neurones on astrocytes in a coculture system renders neurones sensitive to transforming growth factor beta1-induced glutamate toxicity, J. Neurochem., 72 (1999), 943–953. https://doi.org/10.1046/j.1471-4159.1999.0720943.x doi: 10.1046/j.1471-4159.1999.0720943.x
![]() |
[213] | S. Koka, J. Vance, G. Maze, Bone growth factors: potential for use as an osseointegration enhancement technique (OET), J. West Soc. Periodontol. Periodontal. Abstr., 43 (1995), 97–104. |
[214] |
E. Woodcock, S. Land, R. Andrews, A low affinity, low molecular weight endothelin-a receptor present in neonatal rat heart, Clin. Exp. Pharmacol. Physiol., 20 (1993), 331–334. https://doi.org/10.1111/j.1440-1681.1993.tb01697.x doi: 10.1111/j.1440-1681.1993.tb01697.x
![]() |
[215] |
M. Serizawa, T. Takahashi, N. Yamamoto, Y. Koh, Combined treatment with erlotinib and a transforming growth factor-β type Ⅰ receptor inhibitor effectively suppresses the enhanced motility of erlotinib-resistant non-small-cell lung cancer cells, J. Thorac. Oncol., 8 (2013), 259–269. https://doi.org/10.1097/JTO.0b013e318279e942 doi: 10.1097/JTO.0b013e318279e942
![]() |
[216] |
J. Maher, R. Zhang, G. Palanisamy, K. Perkins, L. Liu, P. Brassil, et al., Lung-restricted ALK5 inhibition avoids systemic toxicities associated with TGFβ pathway inhibition, Toxicol. Appl. Pharmacol., 438 (2022), 115905. https://doi.org/10.1016/j.taap.2022.115905 doi: 10.1016/j.taap.2022.115905
![]() |
[217] |
L. Spender, G. J. Ferguson, G. Hughes, B. Davies, F. Goldberg, B. Herrera, et al., Preclinical evaluation of AZ12601011 and AZ12799734, inhibitors of transforming growth factor β superfamily type 1 receptors, Mol. Pharmacol., 95 (2019), 222–234. https://doi.org/10.1124/mol.118.112946 doi: 10.1124/mol.118.112946
![]() |
[218] |
A. Friedman, J. P. Tian, G. Fulci, E. A. Chiocca, J. Wang, Glioma virotherapy: effects of innate immune suppression and increased viral replication capacity, Cancer Res., 66 (2006), 2314–2319. https://doi.org/10.1158/0008-5472.CAN-05-2661 doi: 10.1158/0008-5472.CAN-05-2661
![]() |
[219] |
X. Liu, Z. Wang, Y. Yang, L. Wang, R. Sun, Y. Zhao, et al., Active components with inhibitory activities on IFN-γ/STAT1 and IL-6/ATAT3 signaling pathways from caulis trachelospermi, Molecules, 19 (2014), 11560–11571. https://doi.org/10.3390/molecules190811560 doi: 10.3390/molecules190811560
![]() |
[220] |
L. Chen, S. Willis, A. Wei, B. Smith, J. Fletcher, M. Hinds, et al., Differential targeting of prosurvival Bcl-2 proteins by their BH3-only ligands allows complementary apoptotic function, Mol. Cell, 17 (2005), 393–403. https://doi.org/10.1016/j.molcel.2004.12.030 doi: 10.1016/j.molcel.2004.12.030
![]() |
[221] |
M. Shanmugam, H. Shen, F. Tang, F. Arfuso, M. Rajesh, L. Wang, et al., Potential role of genipin in cancer therapy, Pharmacol. Res., 133 (2018), 195–200. https://doi.org/10.1016/j.phrs.2018.05.007 doi: 10.1016/j.phrs.2018.05.007
![]() |
[222] |
P. Gascon, U. Fuhr, F. Sorgel, M. Kinzig-Schippers, A. Makhson, S. Balser, et al., Development of a new G-CSF product based on biosimilarity assessment, Ann. Oncol., 21 (2010), 1419–1429. https://doi.org/10.1093/annonc/mdp574 doi: 10.1093/annonc/mdp574
![]() |
[223] |
Z. Tekdemir, A. Seckin, T. Kacar, E. Yilmaz, S. Bekiroglu, Evaluation of structural, biological, and functional similarity of biosimilar granulocyte colony stimulating factor to its reference product, Pharm. Res., 37 (2020), 215. https://doi.org/10.1007/s11095-020-02932-7 doi: 10.1007/s11095-020-02932-7
![]() |
Par | Description | Dimensionless Value | Refs |
(Dimensional Value) | |||
Cancer module | |||
r | Tumor growth rate | 0.015 (0.015h−1) | [60], Est |
C0 | Carrying capacity of a tumor | 1 (10−3g/cm3) | [60], Est |
δ1 | Killing rate of tumor cells by N1 TANs | 0.002 (20cm3/g⋅h) | [85], Est |
δ2 | Killing rate of tumor cells by apoptosis | 0.002 (0.002h−1) | Est |
δ3 | Killing rate of tumor cells by NK cells | 0.002 (0.002cm3/g⋅h) | Est |
N2/N1 modules | |||
λ6 | IL-6-induced signaling source of the N2 TANs | 3.5×10−4 (3.5×10−8g/cm2⋅h) | [85,192], Est |
λG | TGF-β signaling rate | 3.5×10−2 (3.5×103h−1) | [85], Est |
k1 | Autocatalytic production rate of N2 TANs | 0.14 (1.4×10−5g/cm3⋅h) | [85], Est |
k2 | Hill-type coefficient | 1 (1.0) | [85], Est |
α | Inhibition strength of N2 TANs by N1 TANs | 1.5 (1.5×108cm6/g2) | [85], Est |
μN2 | Decay rate of N2 TANs | 3.5×10−2 (0.035h−1) | [193,194] |
λN | neupogen signaling rate | 3.5×10−2 (70h−1) | Est |
λS1 | IFN-γ signaling rate | 3.5×10−2 (3.5×102h−1) | [85], Est |
k3 | Autocatalytic production rate of N1 TANs | 0.14 (1.4×10−5g/cm3⋅h) | [85], Est |
k4 | Hill-type coefficient | 1 (1.0) | [85], Est |
β | Inhibition strength of N1 TANs by N2 TANs | 1 (1×108cm6/g2) | [85], Est |
μN1 | Decay rate of N1 TANs | 3.5×10−2 (0.035h−1) | [193,194] |
Therapeutics | |||
λC | Secretion rate of TGF-β by cancer cells | 2.89×10−2 (2.89×10−8h−1) | [85], Est |
γL | Degradation rate of TGF-β by TGF-β inhibitor | 2.0 (1.4247×104cm3/g⋅h) | [85], Est |
μG | Decay rate of TGF-β | 2.89×10−2 (0.0289h−1) | [8,91,100,195] |
uL | TGF-β inhibitor injection rate | 0–0.5 (7.0192×10−8g/cm3⋅h) | Est |
μL | Decay rate of TGF-β inhibitor | 0.231 (0.231h−1) | [196] |
λK | Secretion rate of IFN-γ by NK cells | 4.62×10−2 (2.77×10−10h−1) | Est |
uS | IFN-γ injection rate | 0–0.2 (2×10−9g/cm3⋅h) | Est |
μS | Decay rate of IFN-β | 0.1386 (0.1386h−1) | [197] |
λNK | Source of endogenous NK cells | 4.1×10−3 (2×10−3g/cm3⋅h) | Est |
uK | NK cells injection rate | 0–0.01 (10−3g/cm3⋅h) | Est |
k5 | Reduced rate of NK cells by TGF-β | 0.02 (2×10−3g/cm3⋅h) | Est |
k6 | Hill-type coefficient | 1 (1.0) | Est |
θ | Inhibition strength of NK cells by TGF-β | 4 (4×1018cm6/g2) | Est |
μK | Decay rate of NK cells | 4.1×10−3 (0.0041h−1) | [198,199] |
μN | Decay rate of neupogen | 0.1733 (0.1733h−1) | [200,201] |
Par | Description | Dimensionless Value/(Dimensional Value) | Refs |
Intracellular modules | |||
λS2 | IFN-γ signaling rate | 2.89×10−2 (7.0227h−1) | [107,202], Est |
a1 | autocatalytic production rate (STAT1 module) | 0.1156 (2.8091×10−7g/cm3⋅h) | [107], Est |
a2 | Hill-type coefficient (STAT1 module) | 1.0 (1.0) | [107], Est |
γ | Inhibition strength of STAT1 by STAT3 | 1.5 (7.8765×1011cm6/g2) | [107], Est |
μS1 | Decay rate of STAT1 | 2.89×10−2 (0.0289h−1) | [203,204] |
λ1 | Signaling source of STAT3 | 2.89×10−2 (3.9882×10−8g/cm3⋅h) | [107], Est |
K1 | Inhibition parameter | 5.0 (5.0) | [107], Est |
λS3 | IFN-γ signaling rate | 1.0 (108cm3/g) | [107], Est |
a3 | autocatalytic production rate (STAT3 module) | 0.1156 (1.5953×10−7g/cm3⋅h) | [107], Est |
a4 | Hill-type coefficient (STAT3 module) | 1.0 (1.0) | [107], Est |
δ | Inhibition strength of STAT3 by STAT1 | 1.0 (4.1152×105cm6/g2) | [107], Est |
μS3 | Decay rate of STAT3 | 2.89×10−2 (0.0289h−1) | [203,204] |
λ2 | Signaling source of Bcl-2 | 5.8×10−3 (1.508×10−9g/cm3⋅h) | [107], Est |
a5 | autocatalytic production rate (Bcl-2 module) | 2.89×10−2 (7.514×10−9g/cm3⋅h) | [107], Est |
a6 | Hill-type coefficient (Bcl-2 module) | 1.0 (1.0) | [107], Est |
ω | Inhibition strength of Bcl-2 by STAT1 | 1.0 (1.6935×1011cm6/g2) | [107], Est |
λ3 | Signaling from STAT3 | 3.47×10−2 (0.0065h−1) | [107], Est |
μB | Relative decay rate of Bcl-2 | 3.47×10−2 (0.0347h−1) | [205,206] |
λ4 | Signaling source of BAX | 5.8×10−3 (4.3×10−5g/cm3⋅h) | [107], Est |
a7 | autocatalytic production rate (BAX module) | 0.1156 (8.52×10−4g/cm3⋅h) | [107], Est |
a8 | Hill-type coefficient (BAX module) | 1.0 (1.0) | [107], Est |
ζ | Inhibition strength of BAX by Bcl-2 | 1.0 (1.4793×1013cm6/g2) | [107], Est |
μX | Relative decay rate of BAX | 0.1445 (0.1445h−1) | [207,208] |
Threshold | |||
thN1 | Threshold of N1 TANs | 1.3 | [85], Est |
thN2 | Threshold of N2 TANs | 1.8 | [85], Est |
thS1 | Threshold of STAT1 | 1.8 | [107], Est |
thS3 | Threshold of STAT3 | 1.3 | [107], Est |
thB | Threshold of Bcl-2 | 1.44 | [107], Est |
thX | Threshold of BAX | 0.3 | [107], Est |
Parameter | λ6 | λG | k1 | k2 | α | μN2 |
Minimum | 3.5×10−5 | 0.0035 | 0.014 | 0.1 | 0.15 | 0.0035 |
Baseline | 3.5×10−4 | 0.035 | 0.14 | 1 | 1.5 | 0.035 |
Maximum | 3.5×10−3 | 0.35 | 1.4 | 5 | 5 | 0.35 |
Parameter | λS1 | k3 | k4 | β | μN1 | λS2 |
Minimum | 0.0035 | 0.014 | 0.1 | 0.1 | 0.0035 | 2.89×10−3 |
Baseline | 0.035 | 0.14 | 1 | 1 | 0.035 | 0.0289 |
Maximum | 0.35 | 1.4 | 5 | 5 | 0.35 | 0.289 |
Parameter | a1 | a2 | γ | μS1 | λ1 | K1 |
Minimum | 1.156×10−2 | 0.1 | 0.15 | 2.89×10−3 | 2.89×10−3 | 0.5 |
Baseline | 0.1156 | 1 | 1 | 0.0289 | 0.0289 | 5 |
Maximum | 1.156 | 5 | 5 | 0.289 | 0.289 | 10 |
Parameter | λS3 | a3 | a4 | δ | μS3 | λ2 |
Minimum | 0.1 | 1.156×10−2 | 0.1 | 0.1 | 2.89×10−3 | 5.8×10−4 |
Baseline | 1 | 0.1156 | 1 | 1 | 0.0289 | 5.8×10−3 |
Maximum | 5 | 1.156 | 5 | 5 | 0.289 | 0.058 |
Parameter | a5 | a6 | ω | λ3 | μB | λ4 |
Minimum | 2.89×10−3 | 0.1 | 0.1 | 3.47×10−3 | 3.47×10−3 | 5.8×10−4 |
Baseline | 0.0289 | 1 | 1 | 0.0347 | 0.0347 | 5.8×10−3 |
Maximum | 0.289 | 5 | 5 | 0.347 | 0.347 | 0.058 |
Parameter | a7 | a8 | ζ | μX | λK | μS |
Minimum | 1.156×10−2 | 0.1 | 0.1 | 1.445×10−2 | 2.77×10−3 | 1.386×10−2 |
Baseline | 0.1156 | 1 | 1 | 0.1445 | 0.0277 | 0.1386 |
Maximum | 1.156 | 5 | 5 | 1.445 | 0.277 | 1.386 |
Parameter | λC | μG | λNK | k5 | k6 | θ |
Minimum | 2.89×10−3 | 2.89×10−3 | 2×10−4 | 2×10−4 | 0.1 | 0.4 |
Baseline | 0.0289 | 0.0289 | 2×10−3 | 2×10−3 | 1 | 4 |
Maximum | 0.289 | 0.289 | 2×10−2 | 2×10−2 | 5 | 8 |
Parameter | μK | D | αRT | βRT | ϵN2 | ϵN1 |
Minimum | 4.1×10−4 | 0 | 0.003 | 0.0003 | 0.05 | 0.05 |
Baseline | 4.1×10−3 | 0−5 | 0.03 | 0.003 | 0.5 | 0.5 |
Maximum | 0.041 | 5 | 0.3 | 0.03 | 1 | 1 |
Parameter | λRK | λRG | r | δ1 | δ2 | δ3 |
Minimum | 0.075 | 0.015 | 0.0015 | 0.0002 | 0.0002 | 0.0002 |
Baseline | 0.75 | 0.15 | 0.015 | 0.002 | 0.002 | 0.002 |
Maximum | 1.5 | 1.5 | 0.15 | 0.02 | 0.02 | 0.02 |
Par | Description | Value | Refs |
Random motility/Diffusion coefficient | |||
DC | Cancer cells | 3.6×10−6cm2/h | [8,91,100,209,210] |
DN2 | N2 TANs | 3.6×10−6cm2/h | [91,211] |
DN1 | N1 TANs | 3.6×10−6cm2/h | [91,211] |
DK | NK cells | 3.6×10−6cm2/h | [74] |
DG | TGF-β | 3.5×10−3cm2/h | [212,213,214] |
DS | IFN-γ | 3.5×10−3cm2/h | Est |
Par | Description | Value | Refs |
C∗ | Concentration of cancer cells | 1.0×10−3g/cm3 | [91,187] |
N∗1 | Concentration of N1 TANs | 1.0×10−4g/cm3 | [187,211] |
N∗2 | Concentration of N2 TANs | =N∗1 | [187,211] |
G∗ | Concentration of TGF-β | 1ng/mL | [215] |
L∗ | Concentration of TGF-β inhibitor | 380nM | [216,217] |
S∗ | Concentration of IFN-γ | 10ng/mL | [202] |
K∗ | Concentration of NK cells | 1.0×10−3g/mm3 | [74,218] |
S∗1 | Concentration of STAT1 | 2.43μg/mL | [219] |
S∗3 | Concentration of STAT3 | 1.38μg/mL | [219] |
B∗ | Concentration of Bcl-2 | 1.0×10−2μM | [220] |
X∗ | Concentration of BAX | 351μM | [221] |
N∗ | Concentration of Neupogen | 50ng/mL | [222,223] |
Par | Description | Dimensionless Value | Refs |
(Dimensional Value) | |||
Cancer module | |||
r | Tumor growth rate | 0.015 (0.015h−1) | [60], Est |
C0 | Carrying capacity of a tumor | 1 (10−3g/cm3) | [60], Est |
δ1 | Killing rate of tumor cells by N1 TANs | 0.002 (20cm3/g⋅h) | [85], Est |
δ2 | Killing rate of tumor cells by apoptosis | 0.002 (0.002h−1) | Est |
δ3 | Killing rate of tumor cells by NK cells | 0.002 (0.002cm3/g⋅h) | Est |
N2/N1 modules | |||
λ6 | IL-6-induced signaling source of the N2 TANs | 3.5×10−4 (3.5×10−8g/cm2⋅h) | [85,192], Est |
λG | TGF-β signaling rate | 3.5×10−2 (3.5×103h−1) | [85], Est |
k1 | Autocatalytic production rate of N2 TANs | 0.14 (1.4×10−5g/cm3⋅h) | [85], Est |
k2 | Hill-type coefficient | 1 (1.0) | [85], Est |
α | Inhibition strength of N2 TANs by N1 TANs | 1.5 (1.5×108cm6/g2) | [85], Est |
μN2 | Decay rate of N2 TANs | 3.5×10−2 (0.035h−1) | [193,194] |
λN | neupogen signaling rate | 3.5×10−2 (70h−1) | Est |
λS1 | IFN-γ signaling rate | 3.5×10−2 (3.5×102h−1) | [85], Est |
k3 | Autocatalytic production rate of N1 TANs | 0.14 (1.4×10−5g/cm3⋅h) | [85], Est |
k4 | Hill-type coefficient | 1 (1.0) | [85], Est |
β | Inhibition strength of N1 TANs by N2 TANs | 1 (1×108cm6/g2) | [85], Est |
μN1 | Decay rate of N1 TANs | 3.5×10−2 (0.035h−1) | [193,194] |
Therapeutics | |||
λC | Secretion rate of TGF-β by cancer cells | 2.89×10−2 (2.89×10−8h−1) | [85], Est |
γL | Degradation rate of TGF-β by TGF-β inhibitor | 2.0 (1.4247×104cm3/g⋅h) | [85], Est |
μG | Decay rate of TGF-β | 2.89×10−2 (0.0289h−1) | [8,91,100,195] |
uL | TGF-β inhibitor injection rate | 0–0.5 (7.0192×10−8g/cm3⋅h) | Est |
μL | Decay rate of TGF-β inhibitor | 0.231 (0.231h−1) | [196] |
λK | Secretion rate of IFN-γ by NK cells | 4.62×10−2 (2.77×10−10h−1) | Est |
uS | IFN-γ injection rate | 0–0.2 (2×10−9g/cm3⋅h) | Est |
μS | Decay rate of IFN-β | 0.1386 (0.1386h−1) | [197] |
λNK | Source of endogenous NK cells | 4.1×10−3 (2×10−3g/cm3⋅h) | Est |
uK | NK cells injection rate | 0–0.01 (10−3g/cm3⋅h) | Est |
k5 | Reduced rate of NK cells by TGF-β | 0.02 (2×10−3g/cm3⋅h) | Est |
k6 | Hill-type coefficient | 1 (1.0) | Est |
θ | Inhibition strength of NK cells by TGF-β | 4 (4×1018cm6/g2) | Est |
μK | Decay rate of NK cells | 4.1×10−3 (0.0041h−1) | [198,199] |
μN | Decay rate of neupogen | 0.1733 (0.1733h−1) | [200,201] |
Par | Description | Dimensionless Value/(Dimensional Value) | Refs |
Intracellular modules | |||
λS2 | IFN-γ signaling rate | 2.89×10−2 (7.0227h−1) | [107,202], Est |
a1 | autocatalytic production rate (STAT1 module) | 0.1156 (2.8091×10−7g/cm3⋅h) | [107], Est |
a2 | Hill-type coefficient (STAT1 module) | 1.0 (1.0) | [107], Est |
γ | Inhibition strength of STAT1 by STAT3 | 1.5 (7.8765×1011cm6/g2) | [107], Est |
μS1 | Decay rate of STAT1 | 2.89×10−2 (0.0289h−1) | [203,204] |
λ1 | Signaling source of STAT3 | 2.89×10−2 (3.9882×10−8g/cm3⋅h) | [107], Est |
K1 | Inhibition parameter | 5.0 (5.0) | [107], Est |
λS3 | IFN-γ signaling rate | 1.0 (108cm3/g) | [107], Est |
a3 | autocatalytic production rate (STAT3 module) | 0.1156 (1.5953×10−7g/cm3⋅h) | [107], Est |
a4 | Hill-type coefficient (STAT3 module) | 1.0 (1.0) | [107], Est |
δ | Inhibition strength of STAT3 by STAT1 | 1.0 (4.1152×105cm6/g2) | [107], Est |
μS3 | Decay rate of STAT3 | 2.89×10−2 (0.0289h−1) | [203,204] |
λ2 | Signaling source of Bcl-2 | 5.8×10−3 (1.508×10−9g/cm3⋅h) | [107], Est |
a5 | autocatalytic production rate (Bcl-2 module) | 2.89×10−2 (7.514×10−9g/cm3⋅h) | [107], Est |
a6 | Hill-type coefficient (Bcl-2 module) | 1.0 (1.0) | [107], Est |
ω | Inhibition strength of Bcl-2 by STAT1 | 1.0 (1.6935×1011cm6/g2) | [107], Est |
λ3 | Signaling from STAT3 | 3.47×10−2 (0.0065h−1) | [107], Est |
μB | Relative decay rate of Bcl-2 | 3.47×10−2 (0.0347h−1) | [205,206] |
λ4 | Signaling source of BAX | 5.8×10−3 (4.3×10−5g/cm3⋅h) | [107], Est |
a7 | autocatalytic production rate (BAX module) | 0.1156 (8.52×10−4g/cm3⋅h) | [107], Est |
a8 | Hill-type coefficient (BAX module) | 1.0 (1.0) | [107], Est |
ζ | Inhibition strength of BAX by Bcl-2 | 1.0 (1.4793×1013cm6/g2) | [107], Est |
μX | Relative decay rate of BAX | 0.1445 (0.1445h−1) | [207,208] |
Threshold | |||
thN1 | Threshold of N1 TANs | 1.3 | [85], Est |
thN2 | Threshold of N2 TANs | 1.8 | [85], Est |
thS1 | Threshold of STAT1 | 1.8 | [107], Est |
thS3 | Threshold of STAT3 | 1.3 | [107], Est |
thB | Threshold of Bcl-2 | 1.44 | [107], Est |
thX | Threshold of BAX | 0.3 | [107], Est |
Parameter | λ6 | λG | k1 | k2 | α | μN2 |
Minimum | 3.5×10−5 | 0.0035 | 0.014 | 0.1 | 0.15 | 0.0035 |
Baseline | 3.5×10−4 | 0.035 | 0.14 | 1 | 1.5 | 0.035 |
Maximum | 3.5×10−3 | 0.35 | 1.4 | 5 | 5 | 0.35 |
Parameter | λS1 | k3 | k4 | β | μN1 | λS2 |
Minimum | 0.0035 | 0.014 | 0.1 | 0.1 | 0.0035 | 2.89×10−3 |
Baseline | 0.035 | 0.14 | 1 | 1 | 0.035 | 0.0289 |
Maximum | 0.35 | 1.4 | 5 | 5 | 0.35 | 0.289 |
Parameter | a1 | a2 | γ | μS1 | λ1 | K1 |
Minimum | 1.156×10−2 | 0.1 | 0.15 | 2.89×10−3 | 2.89×10−3 | 0.5 |
Baseline | 0.1156 | 1 | 1 | 0.0289 | 0.0289 | 5 |
Maximum | 1.156 | 5 | 5 | 0.289 | 0.289 | 10 |
Parameter | λS3 | a3 | a4 | δ | μS3 | λ2 |
Minimum | 0.1 | 1.156×10−2 | 0.1 | 0.1 | 2.89×10−3 | 5.8×10−4 |
Baseline | 1 | 0.1156 | 1 | 1 | 0.0289 | 5.8×10−3 |
Maximum | 5 | 1.156 | 5 | 5 | 0.289 | 0.058 |
Parameter | a5 | a6 | ω | λ3 | μB | λ4 |
Minimum | 2.89×10−3 | 0.1 | 0.1 | 3.47×10−3 | 3.47×10−3 | 5.8×10−4 |
Baseline | 0.0289 | 1 | 1 | 0.0347 | 0.0347 | 5.8×10−3 |
Maximum | 0.289 | 5 | 5 | 0.347 | 0.347 | 0.058 |
Parameter | a7 | a8 | ζ | μX | λK | μS |
Minimum | 1.156×10−2 | 0.1 | 0.1 | 1.445×10−2 | 2.77×10−3 | 1.386×10−2 |
Baseline | 0.1156 | 1 | 1 | 0.1445 | 0.0277 | 0.1386 |
Maximum | 1.156 | 5 | 5 | 1.445 | 0.277 | 1.386 |
Parameter | λC | μG | λNK | k5 | k6 | θ |
Minimum | 2.89×10−3 | 2.89×10−3 | 2×10−4 | 2×10−4 | 0.1 | 0.4 |
Baseline | 0.0289 | 0.0289 | 2×10−3 | 2×10−3 | 1 | 4 |
Maximum | 0.289 | 0.289 | 2×10−2 | 2×10−2 | 5 | 8 |
Parameter | μK | D | αRT | βRT | ϵN2 | ϵN1 |
Minimum | 4.1×10−4 | 0 | 0.003 | 0.0003 | 0.05 | 0.05 |
Baseline | 4.1×10−3 | 0−5 | 0.03 | 0.003 | 0.5 | 0.5 |
Maximum | 0.041 | 5 | 0.3 | 0.03 | 1 | 1 |
Parameter | λRK | λRG | r | δ1 | δ2 | δ3 |
Minimum | 0.075 | 0.015 | 0.0015 | 0.0002 | 0.0002 | 0.0002 |
Baseline | 0.75 | 0.15 | 0.015 | 0.002 | 0.002 | 0.002 |
Maximum | 1.5 | 1.5 | 0.15 | 0.02 | 0.02 | 0.02 |
Par | Description | Value | Refs |
Random motility/Diffusion coefficient | |||
DC | Cancer cells | 3.6×10−6cm2/h | [8,91,100,209,210] |
DN2 | N2 TANs | 3.6×10−6cm2/h | [91,211] |
DN1 | N1 TANs | 3.6×10−6cm2/h | [91,211] |
DK | NK cells | 3.6×10−6cm2/h | [74] |
DG | TGF-β | 3.5×10−3cm2/h | [212,213,214] |
DS | IFN-γ | 3.5×10−3cm2/h | Est |
Par | Description | Value | Refs |
C∗ | Concentration of cancer cells | 1.0×10−3g/cm3 | [91,187] |
N∗1 | Concentration of N1 TANs | 1.0×10−4g/cm3 | [187,211] |
N∗2 | Concentration of N2 TANs | =N∗1 | [187,211] |
G∗ | Concentration of TGF-β | 1ng/mL | [215] |
L∗ | Concentration of TGF-β inhibitor | 380nM | [216,217] |
S∗ | Concentration of IFN-γ | 10ng/mL | [202] |
K∗ | Concentration of NK cells | 1.0×10−3g/mm3 | [74,218] |
S∗1 | Concentration of STAT1 | 2.43μg/mL | [219] |
S∗3 | Concentration of STAT3 | 1.38μg/mL | [219] |
B∗ | Concentration of Bcl-2 | 1.0×10−2μM | [220] |
X∗ | Concentration of BAX | 351μM | [221] |
N∗ | Concentration of Neupogen | 50ng/mL | [222,223] |