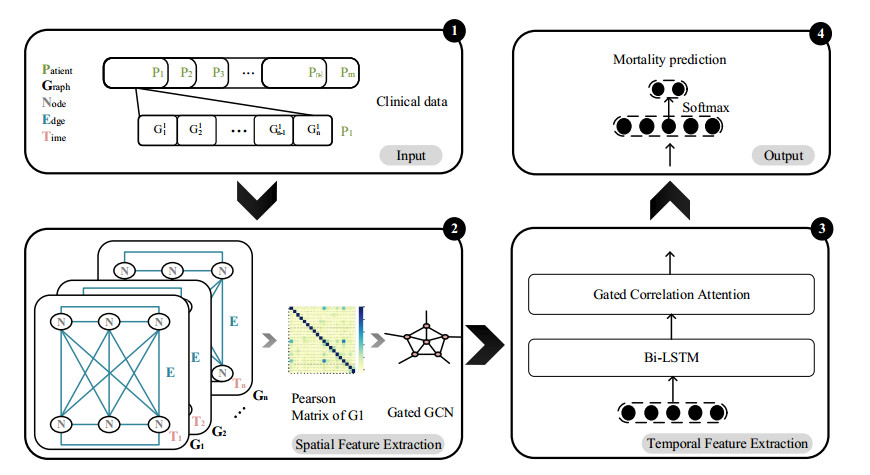
Stroke continues to be the most common cause of death in China. It has great significance for mortality prediction for stroke patients, especially in terms of analyzing the complex interactions between non-negligible factors. In this paper, we present a gated spatio-temporal correlation network (GSTCNet) to predict the one-year post-stroke mortality. Based on the four categories of risk factors: vascular event, chronic disease, medical usage and surgery, we designed a gated correlation graph convolution kernel to capture spatial features and enhance the spatial correlation between feature categories. Bi-LSTM represents the temporal features of five timestamps. The novel gated correlation attention mechanism is then connected to the Bi-LSTM to realize the comprehensive mining of spatio-temporal correlations. Using the data on 2275 patients obtained from the neurology department of a local hospital, we constructed a series of sequential experiments. The experimental results show that the proposed model achieves competitive results on each evaluation metric, reaching an AUC of 89.17%, a precision of 97.75%, a recall of 95.33% and an F1-score of 95.19%. The interpretability analysis of the feature categories and timestamps also verified the potential application value of the model for stroke.
Citation: Shuo Zhang, Yonghao Ren, Jing Wang, Bo Song, Runzhi Li, Yuming Xu. GSTCNet: Gated spatio-temporal correlation network for stroke mortality prediction[J]. Mathematical Biosciences and Engineering, 2022, 19(10): 9966-9982. doi: 10.3934/mbe.2022465
[1] | Zhenglong Tang, Chao Chen . Spatio-temporal information enhance graph convolutional networks: A deep learning framework for ride-hailing demand prediction. Mathematical Biosciences and Engineering, 2024, 21(2): 2542-2567. doi: 10.3934/mbe.2024112 |
[2] | Chunxiang Fan, Zouqin Huang, Binbin Chen, Baojin Chen, Qi Wang, Weidong Liu, Donghai Yu . Comprehensive analysis of key lncRNAs in ischemic stroke. Mathematical Biosciences and Engineering, 2020, 17(2): 1318-1328. doi: 10.3934/mbe.2020066 |
[3] | Suqi Zhang, Wenfeng Wang, Ningning Li, Ningjing Zhang . Multi-behavioral recommendation model based on dual neural networks and contrast learning. Mathematical Biosciences and Engineering, 2023, 20(11): 19209-19231. doi: 10.3934/mbe.2023849 |
[4] | Shi Liu, Kaiyang Li, Yaoying Wang, Tianyou Zhu, Jiwei Li, Zhenyu Chen . Knowledge graph embedding by fusing multimodal content via cross-modal learning. Mathematical Biosciences and Engineering, 2023, 20(8): 14180-14200. doi: 10.3934/mbe.2023634 |
[5] | Zhijun Yang, Wenjie Huang, Hongwei Ding, Zheng Guan, Zongshan Wang . Performance analysis of a two-level polling control system based on LSTM and attention mechanism for wireless sensor networks. Mathematical Biosciences and Engineering, 2023, 20(11): 20155-20187. doi: 10.3934/mbe.2023893 |
[6] | Wenzhu Song, Lixia Qiu, Jianbo Qing, Wenqiang Zhi, Zhijian Zha, Xueli Hu, Zhiqi Qin, Hao Gong, Yafeng Li . Using Bayesian network model with MMHC algorithm to detect risk factors for stroke. Mathematical Biosciences and Engineering, 2022, 19(12): 13660-13674. doi: 10.3934/mbe.2022637 |
[7] | Chongyi Tian, Longlong Lin, Yi Yan, Ruiqi Wang, Fan Wang, Qingqing Chi . Photovoltaic power prediction based on dilated causal convolutional network and stacked LSTM. Mathematical Biosciences and Engineering, 2024, 21(1): 1167-1185. doi: 10.3934/mbe.2024049 |
[8] | Xiaotong Ji, Dan Liu, Ping Xiong . Multi-model fusion short-term power load forecasting based on improved WOA optimization. Mathematical Biosciences and Engineering, 2022, 19(12): 13399-13420. doi: 10.3934/mbe.2022627 |
[9] | Jiang Xie, Junfu Xu, Celine Nie, Qing Nie . Machine learning of swimming data via wisdom of crowd and regression analysis. Mathematical Biosciences and Engineering, 2017, 14(2): 511-527. doi: 10.3934/mbe.2017031 |
[10] | Xingyu Tang, Peijie Zheng, Yuewu Liu, Yuhua Yao, Guohua Huang . LangMoDHS: A deep learning language model for predicting DNase I hypersensitive sites in mouse genome. Mathematical Biosciences and Engineering, 2023, 20(1): 1037-1057. doi: 10.3934/mbe.2023048 |
Stroke continues to be the most common cause of death in China. It has great significance for mortality prediction for stroke patients, especially in terms of analyzing the complex interactions between non-negligible factors. In this paper, we present a gated spatio-temporal correlation network (GSTCNet) to predict the one-year post-stroke mortality. Based on the four categories of risk factors: vascular event, chronic disease, medical usage and surgery, we designed a gated correlation graph convolution kernel to capture spatial features and enhance the spatial correlation between feature categories. Bi-LSTM represents the temporal features of five timestamps. The novel gated correlation attention mechanism is then connected to the Bi-LSTM to realize the comprehensive mining of spatio-temporal correlations. Using the data on 2275 patients obtained from the neurology department of a local hospital, we constructed a series of sequential experiments. The experimental results show that the proposed model achieves competitive results on each evaluation metric, reaching an AUC of 89.17%, a precision of 97.75%, a recall of 95.33% and an F1-score of 95.19%. The interpretability analysis of the feature categories and timestamps also verified the potential application value of the model for stroke.
Stroke is the leading cause of death in China. In 2018, stroke caused 1.57 million deaths, and the number of patients with cerebrovascular diseases has been increasing at an annual rate of 8.7% [1,2]. Meanwhile, stroke is closely related to other chronic diseases, especially hypertension, hyperlipidemia and diabetes [3]. In 2018, the age-standardized prevalence rate of hypertension in people aged 18 years and above was 25.2%, the prevalence of hypercholesterolemia was 5.8% and that of diabetes was 10.9% [1]. Because they are risk factors for stroke, the prevention and treatment of chronic diseases have become one of the most urgent tasks in China. In addition, China faces growing challenges in reducing stroke mortality, along with the increase of population and the acceleration of aging. The elderly generally suffers from arteriosclerosis, hypertension, heart disease, diabetes and other chronic diseases. It is difficult to avoid these inducements of stroke. Therefore, aging increases the susceptibility to stroke, which would further increase the risk and burden of existing disease. More importantly, stroke has a variety of vascular events, complications and etiological subtypes, which complicate the interactions among these risk factors. The mortality of different stroke subtypes and the recurrence rate of different vascular events are different [4,5]. Moreover, the choice of medication for stroke-related chronic diseases will lead to vascular complications [6,7]. Meanwhile, the option of surgery depends on the stroke subtype and vascular complications. Consequently, it is of great clinical value and guiding significance to focus on the prediction of stroke mortality and explore the interactive relationship with chronic diseases, vascular events, surgery and medication usage.
In recent years, artificial intelligence technologies have been widely applied in the field of medicine [8]. However, clinical data usually contain a large number of complex interactive patient information, such as their medication, surgery and complications. How to effectively represent the complex interactive patient information has become the key challenge. Coding technology [9] could not capture the semantic and temporal information between features. Convolutional neural networks (CNNs) [10,11] can obtain local feature information, but the ability to learn temporal information is limited. In contrast, Recurrent neural networks (RNNs) [12,13] can model temporal data correctly, but they are not skilled in processing the interaction between information.
To reasonably predict one-year stroke mortality, we propose a hybrid deep learning model, named the gated spatio-temporal correlation neural network (GSTCNet) model, to handle the various risk factors of stroke, the complex etiology, the changeable symptoms and complications, and the diverse means of diagnosis and treatment. Figure 1 displays the architecture of the GSTCNet. We regard each patient as P, each feature as a node N and the interaction between them as the edge E. N and E naturally form a graph G, where G = (N, E). T represents different timestamps. A patient could be considered as P = (G, T). We devised a gated graph convolution kernel evolved from the a priori correlation matrix to extract the spatial relations between features. It is connected to a Bi-LSTM network to encode the temporal representation. The gated correlation attention module is introduced here to combine features among different layers to further capture the temporal correlation and ensure efficient calculation. We validated the model on a clinical dataset and analyzed the ability of the clinical value of each feature and timestamp to predict one-year mortality with reference to the clinical guidelines.
The main contributions could be summarized as follows:
● It provides the GSTCNet for the interpretable prediction of stroke mortality. We validate the model interpretability by analyzing the significance of each feature category and timestamp with reference to clinical guidelines.
● We have designed a correlation gated graph convolution kernel to capture the patient spatial information. It realizes spatial correlation mining based on the pre-admission status of a patient.
● A novel gated correlation attention mechanism has been updated to evolve the simple concatenation into gated combination. It retains valuable information and captures temporal correlations.
The rest of this paper is organized as follows. Section 2 introduces the fundamental methodology. The comparison experiment results are stated in Section 3, while Section 4 is a discussion. Section 5 concludes this paper.
The dataset originated from the stroke research cohort in the neurology department of a local comprehensive hospital with data on 2275 patients over three years. Table 1 displays the inclusion and exclusion criteria. The detailed baseline and follow-up data were registered prospectively using the case report form designed specifically for this study. The registry forms were strictly designed in accordance with the clinical trial protocol, and they were reviewed by experts from various fields such as clinical and statistics. Neurologists with similar levels of training and experience completed the registration and follow-up of patient information. According to the recommendations of neurologists, we focused on four categories of features: vascular events, chronic disease, surgery and medication usage. The timestamps involved included pre-admission, in-hospital, the 3-month follow-up, the 6-month follow-up and the 1-year follow-up. Table 2 presents an overview of the features in the dataset.
Inclusion criteria | Exclusion criteria |
Age of patient ≥ 18 years | Hemorrhagic stroke |
Sign informed consent | |
Time of onset and admission ≤ 7days | Non-cerebrovascular disease events |
Cerebral infarction and Transient Ischemic Attacks |
Category | Specific features | Category | Specific features |
Vascular event | TIA | Chronic disease | Angiocardiopathy |
Angina pectoris | Thrombotic diseases | ||
Cerebral infarction | Hypertension | ||
Cerebral hemorrhage | Diabetes | ||
Myocardial infarction | Dyslipidemia | ||
Peripheral arterial events | Medication usage | Anticoagulation | |
Gastrointestinal bleeding | Antiplatelet aggregation | ||
Mucosa and skin bleeding | Hypotensive | ||
Subarachnoid hemorrhage | Lipid-lowering | ||
Surgery | Surgery | Hypoglycemic |
In this section, we ignore the timestamp and represent the information of patients according to the correlative relationship, as shown in Figure 2. Based on G1 of pre-admission, we calculate the kernel of the Graph Convolutional Network (GCN) after a series of transformations so as to extract the spatial information from G.
We adopt the Pearson coefficient to measure the inner correlations among features:
Px,y=E((x−μx)(y−μy))σxσy | (1) |
where x and y are two different features, Px,y is the correlation, σ is the standard deviation and μ is the expectation.
We obtain the Pearson matrix P and calculate the weighted adjacency matrix W as
W=|P|−In | (2) |
where |P| is the absolute of the Pearson matrix P, and In is the identity matrix. In addition, the degree matrix D of the graph is computed as
Dii=∑Nj=1Wij | (3) |
Then, the normalized graph Laplacian is computed as
L=In−D−12WD−12=UΛUT∈Rn×n | (4) |
where the graph Fourier basis U∈Rn×n is the matrix of eigenvectors of the normalized graph Laplacian. Λ is the diagonal matrix of the eigenvalues of L.
The graph convolution operator is regard as
ΦωG=Φ(L)G=Φ(UΛUT)G=UΦ(Λ)UTG | (5) |
where Φ is a non-parametric kernel. ω is the graph convolution operator and G is the input.
To reduce the cost of the graph convolution calculations, we apply Chebyshev polynomial approximation to approximate the kernel Φ with a polynomial Λ:
Φ=Φ(Λ)≈∑K−1k=0θkTk(˜Λ) | (6) |
where θ∈Rk denotes a set of coefficients, and ˜Λ=2Λλmax−In. λmax denotes the largest eigenvalue of L, and In∈[−1,1] is a diagonal matrix of the scaled eigenvalues. The graph convolution could be rewritten as
ΦωG=Φ(L)G≈∑K−1k=0θkTk(˜L)G | (7) |
where Tk(˜L) is the kth order Chebyshev polynomial at the scaled Laplacian ˜L.
Meanwhile, we upgrade the graph convolution operator ω with a sigmoid function, as shown in red in Figure 2. The sigmoid function is used as the activation function of the neural network to map variables between 0 and 1. It can realize the control of information flow and simplify calculations.
In this section, we introduce the gated CA-Bi-LSTM model, which is used to capture the temporal features, as shown in Figure 3. The model consists of a Bi-LSTM network and gated correlation attention module. The Bi-LSTM network can make full use of the feature information before and after the current state by applying forward LSTM (→LSTM) and backward LSTM (′LSTM).
→hn=→LSTM(Tn),t∈[1,N] | (8) |
′hn=′LSTM(Tn),t∈[1,N] | (9) |
Hn=→hn+′hn | (10) |
where Tn is the calculated result of the patient information G in the above section. Hn is the final state of the hidden layer, which merges the hidden layer feature information learned by two unidirectional LSTM networks.
Next, we incorporate a gated correlation attention module to pay more attention to the timestamp and capture potential temporal correlations. We first compute the hidden representation of Hn, denoted as uni:
uni=tanh[Hn∗w+b] | (11) |
where w is the weight matrix, and b is the bias vector.
Then, we obtain the attention score αn of the corresponding timestamp, as follows:
αni=uni∗u | (12) |
αn=softmax([αn1,αn2,αn3,⋯,αni]) | (13) |
where u is the trainable parameter.
Then, the context vector Cn is computed as
Cn=αn∗Hn | (14) |
Finally, we concatenate the hidden layers state vector Hn and context vectors Cn as the final vector Fn, as shown in green in Figure 3:
Fn=sigmoid(Hn)+Cn | (15) |
where the gating calculation is also realized via the sigmoid function to avoid information redundancy caused by concatenation, as shown in red in Figure 3.
The binary classifier p for mortality prediction is given as
p=softmax(Fn) | (16) |
We continue the choice of focal loss from our previous work [14], defined as
FL(pt)=−(1−pt)γlog(pt) | (17) |
where
pt={p1−pify=1otherwise | (18) |
Among them, γ is an adjustable concentration parameter, γ > 0.
All experiments were implemented on a Linux server (CPU: Intel(R) Core (TM) i7-8700 K CPU@3.70 GHz, GPU: GeForce GTX 1080Ti, 12 GB). We adopted 10-fold cross validation to evaluate these models. The epoch and batch sizes were respectively set to 100 and 10.
First, we constructed two sets of experiments, each containing seven functions, to verify the choice of optimizer and loss function.
Second, we implemented 10 machine learning models: XGBoost (XGB), AdaBoost (Ada), random forest (RF), support vector machine (SVM), decision tree (DT), k-nearest neighbor (KNN), linear discriminant analysis (LDA), naive Bayes (NB), gradient boosting DT (GBDT) and classification and regression tree (CART) on the Scikit-learn 0.22.1 platform. The parameters were the default parameters in the library.
Third, we compared some related and advanced deep learning algorithms: GCN, Bi-RNN, Bi-GRU, Bi-LSTM, Att-Bi-LSTM, CA-Bi-LSTM, GCN + Bi-LSTM, GCN + Att-Bi-LSTM and GSTCNet. Keras 2.2.4, with TensorFlow 1.12.0 as the backend, was used to implement them. The parameter lstm_dim of LSTM cell was 25.
Fourth, we verified the value of the gate mechanism equipped in this model by using different combination strategies.
Finally, we analyzed the clinical values of different feature categories and timestamps with reference to the clinical guidelines to improve the interpretability of the model results.
In the experiments, we adopted four common evaluation metrics: the AUC, precision, recall and F1-score.
AUC=AreaunderROCcurve(19) | (19) |
PrecisionTPTP+FP | (20) |
RecallTPTP+FN | (21) |
F1−score2∗Precision∗RecallPrecision+Recall | (22) |
In the formulas TP, TN, FP and FN denote true positive, true negative, false positive and false negative, respectively.
Table 3 shows the comparison of seven different optimizers: SGD, RMSprop, Nadam, Adagrad, Adamax, Adadelta and Adam. The Adam optimizer achieved the best performance, with an AUC of 0.8917, a precision of 0.9775, a recall of 0.9533 and an F1-score of 0.9519 based on the model. Table 4 presents the comparison of seven different loss functions: mean squared error, mean absolute error, squared hinge, hinge, logcosh, cross entropy and focal loss. It appears that focal loss achieved the best performance. A total of 108 of the 2275 patients whose data were used in the dataset died within one year, accounting for 5% of the total. This indicates that the dataset was seriously imbalanced, which is consistent with the original intention of the focal loss design. Most of the loss functions achieved close levels of performance on the three evaluation metrics of precision, recall and F1-score. There were great differences in the AUC results. The worst performance was hinge, which was below 0.5.
Optimizer | AUC | Precision | Recall | F1-score |
SGD | 0.8799 | 0.9606 | 0.9512 | 0.9401 |
RMSprop | 0.8178 | 0.9635 | 0.9318 | 0.9311 |
Nadam | 0.8367 | 0.9626 | 0.9393 | 0.9346 |
Adagrad | 0.8694 | 0.9632 | 0.9468 | 0.9403 |
Adamax | 0.8471 | 0.9645 | 0.9441 | 0.9390 |
Adadelta | 0.8606 | 0.9655 | 0.9389 | 0.9369 |
Adam | 0.8917 | 0.9775 | 0.9533 | 0.9519 |
Loss function | AUC | Precision | Recall | F1-score |
Mean squared error | 0.8310 | 0.9636 | 0.9446 | 0.9393 |
Mean absolute error | 0.5094 | 0.9523 | 0.9521 | 0.9291 |
Squared hinge | 0.8017 | 0.9619 | 0.9424 | 0.9360 |
Hinge | 0.4993 | 0.9523 | 0.9525 | 0.9294 |
Logcosh | 0.7609 | 0.9612 | 0.9446 | 0.9357 |
Cross entropy | 0.8460 | 0.9617 | 0.9402 | 0.9345 |
Focal loss | 0.8917 | 0.9775 | 0.9533 | 0.9519 |
Table 5 presents the comparison of GSTCNet with other machine learning models. GSTCNet achieved the optimum performance, with an AUC of 0.8917, a precision of 0.9775, a recall of 0.9533 and an F1-score of 0.9519. Among the machine learning models in Table 5, XGB achieved the best AUC (0.8851) and F1-score (0.9425), and NB achieved the best precision (0.9464), but yielded the worst recall (0.6624). The SVM achieved the best recall of 0.9525. Although the DT and KNN models achieved levels of performance that were close to our model in terms of precision, recall and F1-score, their AUC values of 0.6495 and 0.5926, respectively, were much lower than that achieved by the GSTCNet.
Model | AUC | Precision | Recall | F1-score |
XGB | 0.8851 | 0.9400 | 0.9521 | 0.9425 |
Ada | 0.8521 | 0.9213 | 0.9252 | 0.9217 |
RF | 0.8369 | 0.9274 | 0.9495 | 0.9340 |
SVM | 0.7859 | 0.9073 | 0.9525 | 0.9294 |
DT | 0.6495 | 0.9355 | 0.9288 | 0.9335 |
KNN | 0.5926 | 0.9133 | 0.9345 | 0.9233 |
LDA | 0.8527 | 0.9292 | 0.9411 | 0.9338 |
NB | 0.7876 | 0.9464 | 0.6624 | 0.7570 |
GBDT | 0.8697 | 0.9253 | 0.9317 | 0.9246 |
CART | 0.8680 | 0.9239 | 0.9277 | 0.9217 |
GSTCNet | 0.8917 | 0.9775 | 0.9533 | 0.9519 |
Table 6 shows the comparison of the GSTCNet with other deep learning models. The GCN achieved an AUC of 0.7284, a precision of 0.9526, a recall of 0.9455 and an F1-score of 0.9272. Bi-RNN based models without a GCN achieved similar experimental results. The Bi-LSTM model achieved the best AUC (0.8398). CA-Bi-LSTM, as an independent model, achieved the a level of performance that was close to the best, i.e., an AUC of 0.7994, a precision of 0.9600, a recall of 0.9252 and an F1-score of 0.9249. The performances of the models combining a GCN and Bi-LSTM were better than those of the independent models. The precision of the GCN + Bi-LSTM model increased from 0.9606 to 0.9624, the recall increased from 0.9420 to 0.9433 and the F1-score increased from 0.9350 to 0.9368. Because of the considerations of correlation, the GCN + CA-Bi-LSTM model showed a more balanced performance improvement. Finally, the GSTCNet further improved the AUC from 0.8829 to 0.8917, the precision from 0.9611 to 0.9775, the recall from 0.9436 to 0.9533 and the F1-score from 0.9417 to 0.9519. Compared with some of the machine learning models in Table 5, the performance of some of the RNN-based deep learning models did not indicate obvious advantages. Due to the total number of features of each timestamp being 20, which means that there were five timestamps, the learning advantages of these models when applied to time-series data are not prominent. This also validates that our work could fully consider the potential spatial and temporal correlations. The spatial correlations were captured by the gated GCN kernel derived from Pearson matrix of the patients' baseline states. The gated correlation attention mechanism more comprehensively focuses on the potential temporal correlations.
Model | AUC | Precision | Recall | F1-score |
GCN | 0.7284 | 0.9526 | 0.9455 | 0.9272 |
Bi-RNN | 0.8341 | 0.9628 | 0.9450 | 0.9388 |
Bi-GRU | 0.8165 | 0.9627 | 0.9424 | 0.9371 |
Bi-LSTM | 0.8398 | 0.9606 | 0.9420 | 0.9350 |
Att-Bi-LSTM | 0.7945 | 0.9619 | 0.9327 | 0.9303 |
CA-Bi-LSTM | 0.7994 | 0.9600 | 0.9252 | 0.9249 |
GCN+Bi-LSTM | 0.8375 | 0.9624 | 0.9433 | 0.9368 |
GCN+Att-Bi-LSTM | 0.7844 | 0.9605 | 0.9389 | 0.9328 |
GCN+CA-Bi-LSTM | 0.8829 | 0.9611 | 0.9436 | 0.9417 |
GSTCNet | 0.8917 | 0.9775 | 0.9533 | 0.9519 |
"+" denotes the combination of different basic network structures. |
Table 7 demonstrates the influence of the gate mechanism for different combination strategies. The performances of the GCN and CA-Bi-LSTM models were improved by the addition of a gate mechanism. The average improvement in the four metrics was about 0.01. After the fusion of the two models, the performance greatly improved. The effect of the gate mechanism on different models varied accordingly. The most obvious metric is the AUC. The gated GCN + CA-Bi-LSTM model achieved an AUC of 0.8878, while the GCN + gated CA-Bi-LSTM model directly achieved an AUC higher than 0.9, making it the best AUC among all of the models. In general, the model in this paper still has advantages in terms of other metrics, and the addition of the gate mechanism ensures the reasonable utilization of computing resources and prevents redundant computing.
Model | AUC | Precision | Recall | F1-score |
GCN | 0.7284 | 0.9526 | 0.9455 | 0.9272 |
Gated GCN | 0.7336 | 0.9642 | 0.9529 | 0.9307 |
CA-Bi-LSTM | 0.7994 | 0.9600 | 0.9252 | 0.9249 |
Gated CA-Bi-LSTM | 0.8057 | 0.9637 | 0.9345 | 0.9332 |
GCN+CA-Bi-LSTM | 0.8829 | 0.9611 | 0.9436 | 0.9417 |
Gated GCN+ CA-Bi-LSTM | 0.8875 | 0.9614 | 0.9498 | 0.9431 |
GCN+Gated CA-Bi-LSTM | 0.9015 | 0.9621 | 0.9487 | 0.9445 |
GSTNet | 0.8917 | 0.9775 | 0.9533 | 0.9519 |
"+" denotes the combination of different basic network structures. |
Figure 4 details the comparison of the combinations with different category features to explain the results with reference to the clinical guidelines. In general, when the number of feature categories was maximized, the performance of the model was improved most obviously. The values for the precision, recall and F1-score were similar. We took the AUC as the representative metric to analyze the interpretability of the model results for different combinations of feature categories.
Comparing V + M and V + C, the AUC of V + C was 0.8387, and the AUC of V + M was 0.7979. Similarly, when comparing S + M and S + C and V + S + M and V + S + C, the AUC values of S + C and V + S + C were higher. Because chronic diseases and medication usage are synchronous, the situation of medication depends on the occurrence of chronic diseases. However, the correlation between chronic diseases and other attribute features is more direct, and the impact on the final results is also more important. We continue to set V + S in contrast; it shows the worst AUC of 0.7075. On the one hand, the feature is binary, meaning that it contains less information. On the other hand, the clinical meaning of this feature is mostly carotid artery stenting and other surgical operations. Most patients undergoing this kind of operation are placed in the neurosurgery or neuro intervention department. That is to say, most patients choose the no option, so its correlation with other attribute features is greatly weakened.
Comparing S + C and V + C, the AUC of S + C was 0.8707, and the AUC of V+ C was 0.8387. Similarly, when comparing S + M and V + M, the AUC of S + M was 0.8073, and the AUC of V + M was 0.7979. When considering the correlation with chronic diseases and medication usage, surgery is more important than vascular events. However, most of the patients in this cohort were treated in the neurology department, and surgical operation requires a transfer to the neurosurgery department. However, surgery still shows significant predictive value and interactive effects in risk factor mining. This is because, in the actual clinical process, patients with a critical stroke will be transferred to neurology department for follow-up treatment after surgery in the neurosurgery department if they present stable vital signs. Therefore, there were such patients in the cohort study wherein the condition of surgery actually reflected the severity of stroke; thus, more significant performance changes and interactive effects could be observed. This also provides evidence to support the attention to postoperative critical patients.
Comparing V + C and M + C, the AUC of V + C was 0.8387, and the AUC of M + C was 0.8167. Similarly, when comparing V + S + C and S + M + C, the AUC of V + S + C was 0.8658, and the AUC of S + M + C was 0.8373. The results show that vascular events have a greater impact on the outcome. In the dataset, vascular events included cerebral infarction, TIA, etc., which directly reflect the health of the patients. When they are combined with chronic diseases, they could more comprehensively reflect the health status of patients.
Figure 5 visually shows the importance of timetamps. It is based on the averages of the attention weights for all patients, the living patients and the dead patients. For all patients and the living patients, the importance of each timestamp was basically equal. For the dead patients, the importance was found to be quite different. The importance of pre-admission was found to be significantly higher than that of the other two groups, and the 1-year follow-up was significantly lower than them. The importance of the in-hospital and 3-month follow-up timestamps was lower, but increased at 6 months follow-up. The research results based on CNSR-Ⅲ show that the history of a stroke is a risk factor for an adverse functional outcome after 3 months follow-up, recurrence by the 1-year follow-up, cerebrovascular disease-related death and an adverse functional outcome. The research suggests that, in terms of clinical treatment. more active treatment and disease management may be needed for patients with a history of stroke [15]. Regarding the pre-admission timestamp, vascular events related to stroke history accounted for nearly 50%. Meanwhile, according to clinical experience, patients with a history of stroke will receive more attention from medical personnel after the second admission, which could effectively reduce the risk of death in hospital. In the wider population, stroke history continues to be one of the increased risk factors for stroke recurrence 90 days after stroke [16,17]. Therefore, patients with a history of stroke have an increased risk of recurrence within 3 months, so the importance of the 3-month follow-up for the final death of patients also increases.
Clinically, plenty of studies have focused on the cohort data of stroke patients, such as the Stroke Prognostic Instrument-Ⅰ, Ⅱ [18], Essen Stroke Risk Score [19] and Recurrence Risk Estimator-90 [20]. Although they have important clinical significance, strict scoring criteria and a professional medical background still cause limitations. Recently, machine learning has become an important auxiliary tool in clinical medicine, includinge naïve Bayes [21], SVM [22], RF [23] and XGBoost methods [24]. These methods effectively improve the utilization of clinical data. However, the representation of complex clinical data still needs further exploration.
Deep learning methods continue to gain more attention in the medical field [25,26,27]. Specific to stroke, CNN-based methods have outstanding performance on medical tasks such as medical image processing [28], etiology classification [29] and lesion prediction [30]. RNN-based methods adopt the modeling ability of temporal data to improve the clinical value for tasks of disease prediction [31], rehabilitation assistance [32] and prognosis prediction [33]. Although existing studies greatly improve the accuracy of risk prediction, most previous studies mainly considered the sequential features, not analyzing the spatial features [34,35]. GCN-based methods could be easily embedded into network architecture to capture graph structure information via message transmission between graph nodes; this helps to maintain high interpretability [36]. Recently, they have demonstrated convincing performance when applied for biomedical network analysis, such as disease prediction [37,38] and drug prediction [39,40].
Our work may further advance the efforts of these previous works by proposing a gated spatio-temporal correlation neural network for one-year mortality risk prediction of stroke patients. The relationships among different diseases, medications and operations are complex and interactive. Meanwhile, the relationships change over time. The individual features of patients are not independent, as they are related to each other through a correlative relationship. Therefore, we reconstructed the patient information to consider correlation and pay attention to temporal relations. This work fully considered the relationship between different features throughout the whole course of stroke, demonstrated accurate predictions and interpreted and analyzed the model results based on clinical guidelines.
Nonetheless, our study has several limitations. First, the data on the stroke patients were multimodal, as they included medical images, biological signals and so on. To more comprehensively reflect the patient status, the fusion of multimodal data will become the focus of our follow-up research. Second, although our data came from a clinic, they were extracted from a single-center data source. Multicenter research will further improve the potential application value of our work. Finally, the size of the dataset is still an obstacle to our progress. Our data came from the statistics of patients over three years, which means a lot of human, material and financial resources. How to obtain patient information more efficiently, reduce the workload of doctors and expand the scale of the dataset will be the basis for promoting the development of artificial intelligence in medicine.
In this paper, we explored the risk factors for stroke mortality and proposed a gated spatio-temporal correlation neural network to accurately predict the one-year mortality of stroke patients. First, the spatial features are extracted from the clinical data by using the GCN derived from a Pearson matrix. The gating function of GCN avoids redundant calculation and ensures efficient mining of internal correlation of data. The succeeding Bi-LSTM network serves to enhance the expression of temporal information. Through the gated correlation attention mechanism, the correlation of different timestamps are fused to comprehensively express the description of patients. The experiment provided convincing proof that our model achieves the best results. In addition, we followed clinical norms, verified the importance of mortality in each feature category and timestamp and explained them according to the clinical guidelines.
This work was supported in part by the Science and Technology Innovation 2030-New Generation of Artificial Intelligence Major Project under Grant No. 2021ZD0111000, the Key Science and Research Program of Henan Province under Grant 21A520044 and the Non-Profit Central Research Institute Fund of the Chinese Academy of Medical Sciences under Grant 2020-PT310-01.
The authors declare that there is no conflict of interest.
[1] |
Y. Wang, Z. Li, H. Gu, Y. Zhai, Y. Jiang, X. Zhao, et al., China stroke statistics 2019: A report from the national center for healthcare quality management in neurological diseases, China national clinical research center for neurological diseases, the Chinese stroke association, national center for chronic and non-communicable disease control and prevention, Chinese center for disease control and prevention and institute for global neuroscience and stroke collaborations, Stroke Vasc. Neurol., 5 (2020), 211-369.https://doi.org/10.1136/svn-2020-000457 doi: 10.1136/svn-2020-000457
![]() |
[2] |
S. Wu, B. Wu, M. Liu, Z. Chen, W. Wang, C. S. Anderson, et al., Stroke in China: advances and challenges in epidemiology, prevention, and management, Lancet Neurol., 18 (2019), 394-405.https://doi.org/10.1016/S1474-4422(18)30500-3 doi: 10.1016/S1474-4422(18)30500-3
![]() |
[3] |
T. Guan, J. Ma, M. Li, T. Xue, Z. Lan, J. Guo, et al., Rapid transitions in the epidemiology of stroke and its risk factors in China from 2002 to 2013, Neurology, 89 (2017), 53-61. httpss://doi.org/10.1212/WNL.0000000000004056 doi: 10.1212/WNL.0000000000004056
![]() |
[4] |
P. Zhou, J. Liu, L. Wang, W. Feng, Z. Cao, P. Wang, et al., Association of small dense low-density lipoprotein cholesterol with stroke risk, severity and prognosis, J. Atheroscler. Thromb., 27 (2020), 1310-1324.https://doi.org/10.5551/jat.53132 doi: 10.5551/jat.53132
![]() |
[5] |
S. Schönenberger, P. L. Hendén, C. Z. Simonsen, L. Uhlmann, C. Klose, J. A. R. Pfaff, et al., Association of general anesthesia vs procedural sedation with functional outcome among patients with acute ischemic stroke undergoing thrombectomy: a systematic review and meta-analysis, JAMA-J. Am. Med. Assoc., 322 (2019), 1283-1293.https://doi.org/10.1001/jama.2019.11455 doi: 10.1001/jama.2019.11455
![]() |
[6] |
C. C. Hu, A. Low, E. O'Connor, P. Siriratnam, C. Hair, T. Kraemer, et al., Diabetes in ischaemic stroke in a regional Australian hospital - uncharted territory, Intern. Med. J., 52 (2020), 574-580.https://doi.org/10.1111/imj.15073 doi: 10.1111/imj.15073
![]() |
[7] |
J. Xiang, H. Li, J. Xiong, F. Hua, S. Huang, Y. Jiang, et al., Acupuncture for post-stroke insomnia: A protocol for systematic review and meta-analysis, Medicine, 99 (2020), e21381.https://doi.org/10.1097/MD.0000000000021381 doi: 10.1097/MD.0000000000021381
![]() |
[8] |
Y. Ou, S. Sun, H. Gan, R. Zhou, Z. Yang. An improved self-supervised learning for EEG classification, Math. Biosci. Eng., 19 (2022), 6907-6922.https://doi.org/10.3934/mbe.2022325 doi: 10.3934/mbe.2022325
![]() |
[9] |
R. Elham, A. Hesham, A bag-of-words feature engineering approach for assessing health conditions using accelerometer data, Smart Health, 16 (2020), 100116.https://doi.org/10.1016/j.smhl.2020.100116 doi: 10.1016/j.smhl.2020.100116
![]() |
[10] |
Z. Zhang, Z. Ji, Q. Chen, S. Yuan, W. Fan, Joint optimization of cycleGAN and CNN classifier for detection and localization of retinal pathologies on color fundus photographs, IEEE J. Biomed. Health, 26 (2022), 115-126.https://doi.org/10.1109/JBHI.2021.3092339. doi: 10.1109/JBHI.2021.3092339
![]() |
[11] |
X. Zhang, Y. Hu, Z. Xiao, J. Fang, R. Higashita, J. Liu, Machine learning for cataract classification/grading on ophthalmic imaging modalities: A survey. Mach. Intell. Res., 19 (2022), 184-208.https://doi.org/10.1007/s11633-022-1329-0 doi: 10.1007/s11633-022-1329-0
![]() |
[12] |
Y. S. Baek, S. C. Lee, W. I. Choi, H. H. Kim, Prediction of atrial fibrillation from normal ECG using artificial intelligence in patients with unexplained stroke, Eur. Heart J., 41 (2020), ehaa946.0348.https://doi.org/10.1093/ehjci/ehaa946.0348 doi: 10.1093/ehjci/ehaa946.0348
![]() |
[13] |
S. Liu, X. Wang, Y. Xiang, H. Xu, H. Wang, B. Tang, Multi-channel fusion LSTM for medical event prediction using EHRs, J. Biomed. Inf., 127 (2022), 104011.https://doi.org/10.1016/j.jbi.2022.104011 doi: 10.1016/j.jbi.2022.104011
![]() |
[14] |
S. Zhang, J. Wang, L. Pei, K. Liu, Y. Gao, H. Fang, et al., Interpretability analysis of one-Year mortality prediction for stroke patients based on deep neural network, IEEE J. Biomed. Health, 26 (2022), 1903-1910.https://doi.org/10.1109/JBHI.2021.3123657 doi: 10.1109/JBHI.2021.3123657
![]() |
[15] |
S. Cheng, Q Xu, Z. Xu, Y. Shi, Y. Liu, Z. Li, et al., Effect of prior stroke on stroke outcomes in patients with Ischemic cerebrovascular disease, Chin. J. Stroke, 16 (2021), 1242-1247.https://doi.org/10.3969/j.issn.1673-5765.2021.12.008 doi: 10.3969/j.issn.1673-5765.2021.12.008
![]() |
[16] |
E. C. Leira, K. C. Chang, P. H. Davis, W. R. Clarke, R. F. Woolson, M. D. Hansen, et al., Can we predict early recurrence in acute stroke?, Cerebrovasc. Dis., 18 (2014), 139-144.https://doi.org/10.1159/000079267 doi: 10.1159/000079267
![]() |
[17] |
H. Ay, L. Gungor, E. M. Arsavae, J. Rosand, M. Vangel, T. Benner, et al., A score to predict early risk of recurrence after ischemic stroke, Neurology, 74 (2010), 128.https://doi.org/10.1212/WNL.0b013e3181ca9cff doi: 10.1212/WNL.0b013e3181ca9cff
![]() |
[18] |
W. N. Kernan, C. M. Viscoli, L. M. Brass, R. W. Makuch, P. M. Sarrel, R. S. Roberts, et al., The stroke prognosis instrument ii (spi-ii): A clinical pre-diction instrument for patients with transient ischemia and nondisabling ischemic stroke, Stroke, 31 (2000), 456-462.https://doi.org/10.1161/01.STR.31.2.456 doi: 10.1161/01.STR.31.2.456
![]() |
[19] |
CAPRIE Steering Committee, A randomised, blinded, trial of clopidogrel versus aspirin in patients at risk of ischaemic events (CAPRIE), Lancet, 348 (1996), 1329-1339.https://doi.org/10.1016/s0140-6736(96)09457-3 doi: 10.1016/s0140-6736(96)09457-3
![]() |
[20] |
E. M. Arsava, G. Kim, J. Oliveira-Filho, L. Gungor, H. J. Noh, M. Lordelo, et al., Prediction of early recurrence after acute ischemic stroke, JAMA Neurol., 73 (2016), 396-401.https://doi.org/10.1001/jamaneurol.2015.4949 doi: 10.1001/jamaneurol.2015.4949
![]() |
[21] |
L. C. Hung, S. F. Sung, Y. H. Hu, A machine learning approach to predicting readmission or mortality in patients hospitalized for stroke or transient ischemic attack, Appl. Sci., 10 (2020), 6337.https://doi.org/10.3390/app10186337 doi: 10.3390/app10186337
![]() |
[22] |
A. D. Jamthikar, D. Gupta, L. E. Mantella, L. Saba, J. R. Laird, A. M. Johri, et al., Multiclass machine learning vs. conventional calculators for stroke/CVD risk assessment using carotid plaque predictors with coronary angiography scores as gold standard: A 500 participants study, Int. J. Cardiovas. Imag., 37 (2021), 1171-1187.https://doi.org/10.1007/s10554-020-02099-7 doi: 10.1007/s10554-020-02099-7
![]() |
[23] |
J. N. Heo, J. G. Yoon, H. Park, Y. D. Kim, H. S. Nam, J. H. Heo, Machine learning based model for prediction of outcomes in acute stroke, Stroke, 50 (2019), 1263-1265.https://doi.org/10.1161/STROKEAHA.118.024293 doi: 10.1161/STROKEAHA.118.024293
![]() |
[24] |
C. Jiang, T. Chen, X. Du, X. Li, L. He, Y. Lai, et al., A simple and easily implemented risk model to predict 1-year ischemic stroke and systemic embolism in Chinese patients with atrial fibrillation, Chin. Med. J. -Peking, 134 (2021), 6.https://doi.org/10.1097/CM9.0000000000001515 doi: 10.1097/CM9.0000000000001515
![]() |
[25] |
X. Zhang, Z. Xiao, H. Fu, Y. Hu, J. Yuan, Y. Xu, et al., Attention to region: Region-based integration-and-recalibration networks for nuclear cataract classification using AS-OCT images, Med. Image Anal., 80 (2022), 102499.https://doi.org/10.1016/j.media.2022.102499 doi: 10.1016/j.media.2022.102499
![]() |
[26] |
Y. Su, Y. Shi, W. Lee, L. Cheng, H. Guo, TAHDNet: Time-aware hierarchical dependency network for medication recommendation, J. Biomed. Inf., 129 (2022), 104069.https://doi.org/10.1016/j.jbi.2022.104069 doi: 10.1016/j.jbi.2022.104069
![]() |
[27] |
X. Zhang, Z. Xiao, L. Hu, G. Xu, R. Higashita, W. Chen, et al., CCA-Net: Clinical-awareness attention network for nuclear cataract classification in AS-OCT, Knowl. -Based Syst., 250 (2022), 109109.https://doi.org/10.1016/j.knosys.2022.109109 doi: 10.1016/j.knosys.2022.109109
![]() |
[28] |
S. Zhang, S. Xu, L. Tan, H. Wang, J. Meng, Stroke lesion detection and analysis in MRI images based on deep learning, J. Healthc. Eng., 5 (2021), 1-9.https://doi.org/10.1155/2021/5524769 doi: 10.1155/2021/5524769
![]() |
[29] |
S. Zhang, J. Wang, L. Pei, K. Liu, Y. Gao, H. Fang, et al., Interpretable CNN for ischemic stroke subtype classification with active model adaptation, BMC Med. Inf. Decis., 22 (2022), 3.https://doi.org/10.1186/s12911-021-01721-5 doi: 10.1186/s12911-021-01721-5
![]() |
[30] |
L. Hokkinen, T. Mkel, S. Savolainen, M. Kangasniemi, Evaluation of a CTA-based convolutional neural network for infarct volume prediction in anterior cerebral circulation ischaemic stroke, Eur. Radiol. Exp., 5 (2021), 25.https://doi.org/10.1186/s41747-021-00225-1 doi: 10.1186/s41747-021-00225-1
![]() |
[31] | P. Chantamit-O-Pas, M. Goyal. Long short-term memory recurrent neural network for stroke prediction, in Proceeding of the Machine Learning and Data Mining in Pattern Recognition (MLDM), 2018,312-323.https://doi.org/10.1007/978-3-319-96136-1_25 |
[32] |
L. Chen, B. Gu, Z. Wang, L. Zhang, M. Xu, S. Liu, et al., EEG-controlled functional electrical stimulation rehabilitation for chronic stroke: system design and clinical application, Front. Med. -PRC, 15 (2021), https://doi.org/10.1007/s11684-020-0794-5 doi: 10.1007/s11684-020-0794-5
![]() |
[33] |
Q. Li, X. Chai, C. Zhang, X. Wang, W. Ma, Prediction model of ischemic stroke recurrence using PSO-LSTM in mobile medical monitoring system, Comput. Intel. Neurosci., 2022 (2022), 8936103.https://doi.org/10.1155/2022/8936103 doi: 10.1155/2022/8936103
![]() |
[34] |
M. Jian, J. Wang, H. Yu, G. Wang, Integrating object proposal with attention networks for video saliency detection, Inf. Sci., 576 (2021), 819-830.https://doi.org/10.1016/j.ins.2021.08.069 doi: 10.1016/j.ins.2021.08.069
![]() |
[35] |
M. Jian, J. Wang, H. Yu, G. Wang, X. Meng, L. Yang, et al., Visual saliency detection by integrating spatial position prior of object with background cues, Exp. Syst. Appl., 168 (2021), 114219.https://doi.org/10.1016/j.eswa.2020.114219 doi: 10.1016/j.eswa.2020.114219
![]() |
[36] | T. N. Kipf, M. Welling, Semi-supervised classification with graph convolutional networks, in Proceeding of 2017 International Conference on Learning Representations (ICLR), 2017.https://doi.org/10.1145/3097983.3097997 |
[37] |
B. Chen, J. Li, G. Lu, H. Yu, D. Zhang, Label co-occurrence learning with graph convolutional networks for multi-label chest X-Ray image classification, IEEE J. Biomed. Health, 24 (2020), 2292-2302.https://doi.org/10.1109/JBHI.2020.2967084 doi: 10.1109/JBHI.2020.2967084
![]() |
[38] |
E. El-allaly, M. Sarrouti, N. En-Nahnahi, S. O. E. Alaoui, An attentive joint model with transformer-based weighted graph convolutional network for extracting adverse drug event relation, J. Biomed. Inf., 125 (2022), 103968.https://doi.org/10.1016/j.jbi.2021.103968 doi: 10.1016/j.jbi.2021.103968
![]() |
[39] |
W. Peng, T. Chen, W. Dai, Predicting drug response based on multi-omics fusion and graph convolution, IEEE J. Biomed. Health, 26 (2022), 1384-1393.https://doi.org/10.1109/JBHI.2021.3102186 doi: 10.1109/JBHI.2021.3102186
![]() |
[40] |
C. Mao, L. Yao, Y. Luo, MedGCN: Medication recommendation and lab test imputation via graph convolutional networks, J. Biomed. Inf., 127 (2022), 104000.https://doi.org/10.1016/j.jbi.2022.104000 doi: 10.1016/j.jbi.2022.104000
![]() |
1. | Noemi Scarpato, Patrizia Ferroni, Fiorella Guadagni, XAI Unveiled: Revealing the Potential of Explainable AI in Medicine - A Systematic Review, 2024, 2169-3536, 1, 10.1109/ACCESS.2024.3514197 |
Inclusion criteria | Exclusion criteria |
Age of patient ≥ 18 years | Hemorrhagic stroke |
Sign informed consent | |
Time of onset and admission ≤ 7days | Non-cerebrovascular disease events |
Cerebral infarction and Transient Ischemic Attacks |
Category | Specific features | Category | Specific features |
Vascular event | TIA | Chronic disease | Angiocardiopathy |
Angina pectoris | Thrombotic diseases | ||
Cerebral infarction | Hypertension | ||
Cerebral hemorrhage | Diabetes | ||
Myocardial infarction | Dyslipidemia | ||
Peripheral arterial events | Medication usage | Anticoagulation | |
Gastrointestinal bleeding | Antiplatelet aggregation | ||
Mucosa and skin bleeding | Hypotensive | ||
Subarachnoid hemorrhage | Lipid-lowering | ||
Surgery | Surgery | Hypoglycemic |
Optimizer | AUC | Precision | Recall | F1-score |
SGD | 0.8799 | 0.9606 | 0.9512 | 0.9401 |
RMSprop | 0.8178 | 0.9635 | 0.9318 | 0.9311 |
Nadam | 0.8367 | 0.9626 | 0.9393 | 0.9346 |
Adagrad | 0.8694 | 0.9632 | 0.9468 | 0.9403 |
Adamax | 0.8471 | 0.9645 | 0.9441 | 0.9390 |
Adadelta | 0.8606 | 0.9655 | 0.9389 | 0.9369 |
Adam | 0.8917 | 0.9775 | 0.9533 | 0.9519 |
Loss function | AUC | Precision | Recall | F1-score |
Mean squared error | 0.8310 | 0.9636 | 0.9446 | 0.9393 |
Mean absolute error | 0.5094 | 0.9523 | 0.9521 | 0.9291 |
Squared hinge | 0.8017 | 0.9619 | 0.9424 | 0.9360 |
Hinge | 0.4993 | 0.9523 | 0.9525 | 0.9294 |
Logcosh | 0.7609 | 0.9612 | 0.9446 | 0.9357 |
Cross entropy | 0.8460 | 0.9617 | 0.9402 | 0.9345 |
Focal loss | 0.8917 | 0.9775 | 0.9533 | 0.9519 |
Model | AUC | Precision | Recall | F1-score |
XGB | 0.8851 | 0.9400 | 0.9521 | 0.9425 |
Ada | 0.8521 | 0.9213 | 0.9252 | 0.9217 |
RF | 0.8369 | 0.9274 | 0.9495 | 0.9340 |
SVM | 0.7859 | 0.9073 | 0.9525 | 0.9294 |
DT | 0.6495 | 0.9355 | 0.9288 | 0.9335 |
KNN | 0.5926 | 0.9133 | 0.9345 | 0.9233 |
LDA | 0.8527 | 0.9292 | 0.9411 | 0.9338 |
NB | 0.7876 | 0.9464 | 0.6624 | 0.7570 |
GBDT | 0.8697 | 0.9253 | 0.9317 | 0.9246 |
CART | 0.8680 | 0.9239 | 0.9277 | 0.9217 |
GSTCNet | 0.8917 | 0.9775 | 0.9533 | 0.9519 |
Model | AUC | Precision | Recall | F1-score |
GCN | 0.7284 | 0.9526 | 0.9455 | 0.9272 |
Bi-RNN | 0.8341 | 0.9628 | 0.9450 | 0.9388 |
Bi-GRU | 0.8165 | 0.9627 | 0.9424 | 0.9371 |
Bi-LSTM | 0.8398 | 0.9606 | 0.9420 | 0.9350 |
Att-Bi-LSTM | 0.7945 | 0.9619 | 0.9327 | 0.9303 |
CA-Bi-LSTM | 0.7994 | 0.9600 | 0.9252 | 0.9249 |
GCN+Bi-LSTM | 0.8375 | 0.9624 | 0.9433 | 0.9368 |
GCN+Att-Bi-LSTM | 0.7844 | 0.9605 | 0.9389 | 0.9328 |
GCN+CA-Bi-LSTM | 0.8829 | 0.9611 | 0.9436 | 0.9417 |
GSTCNet | 0.8917 | 0.9775 | 0.9533 | 0.9519 |
"+" denotes the combination of different basic network structures. |
Model | AUC | Precision | Recall | F1-score |
GCN | 0.7284 | 0.9526 | 0.9455 | 0.9272 |
Gated GCN | 0.7336 | 0.9642 | 0.9529 | 0.9307 |
CA-Bi-LSTM | 0.7994 | 0.9600 | 0.9252 | 0.9249 |
Gated CA-Bi-LSTM | 0.8057 | 0.9637 | 0.9345 | 0.9332 |
GCN+CA-Bi-LSTM | 0.8829 | 0.9611 | 0.9436 | 0.9417 |
Gated GCN+ CA-Bi-LSTM | 0.8875 | 0.9614 | 0.9498 | 0.9431 |
GCN+Gated CA-Bi-LSTM | 0.9015 | 0.9621 | 0.9487 | 0.9445 |
GSTNet | 0.8917 | 0.9775 | 0.9533 | 0.9519 |
"+" denotes the combination of different basic network structures. |
Inclusion criteria | Exclusion criteria |
Age of patient ≥ 18 years | Hemorrhagic stroke |
Sign informed consent | |
Time of onset and admission ≤ 7days | Non-cerebrovascular disease events |
Cerebral infarction and Transient Ischemic Attacks |
Category | Specific features | Category | Specific features |
Vascular event | TIA | Chronic disease | Angiocardiopathy |
Angina pectoris | Thrombotic diseases | ||
Cerebral infarction | Hypertension | ||
Cerebral hemorrhage | Diabetes | ||
Myocardial infarction | Dyslipidemia | ||
Peripheral arterial events | Medication usage | Anticoagulation | |
Gastrointestinal bleeding | Antiplatelet aggregation | ||
Mucosa and skin bleeding | Hypotensive | ||
Subarachnoid hemorrhage | Lipid-lowering | ||
Surgery | Surgery | Hypoglycemic |
Optimizer | AUC | Precision | Recall | F1-score |
SGD | 0.8799 | 0.9606 | 0.9512 | 0.9401 |
RMSprop | 0.8178 | 0.9635 | 0.9318 | 0.9311 |
Nadam | 0.8367 | 0.9626 | 0.9393 | 0.9346 |
Adagrad | 0.8694 | 0.9632 | 0.9468 | 0.9403 |
Adamax | 0.8471 | 0.9645 | 0.9441 | 0.9390 |
Adadelta | 0.8606 | 0.9655 | 0.9389 | 0.9369 |
Adam | 0.8917 | 0.9775 | 0.9533 | 0.9519 |
Loss function | AUC | Precision | Recall | F1-score |
Mean squared error | 0.8310 | 0.9636 | 0.9446 | 0.9393 |
Mean absolute error | 0.5094 | 0.9523 | 0.9521 | 0.9291 |
Squared hinge | 0.8017 | 0.9619 | 0.9424 | 0.9360 |
Hinge | 0.4993 | 0.9523 | 0.9525 | 0.9294 |
Logcosh | 0.7609 | 0.9612 | 0.9446 | 0.9357 |
Cross entropy | 0.8460 | 0.9617 | 0.9402 | 0.9345 |
Focal loss | 0.8917 | 0.9775 | 0.9533 | 0.9519 |
Model | AUC | Precision | Recall | F1-score |
XGB | 0.8851 | 0.9400 | 0.9521 | 0.9425 |
Ada | 0.8521 | 0.9213 | 0.9252 | 0.9217 |
RF | 0.8369 | 0.9274 | 0.9495 | 0.9340 |
SVM | 0.7859 | 0.9073 | 0.9525 | 0.9294 |
DT | 0.6495 | 0.9355 | 0.9288 | 0.9335 |
KNN | 0.5926 | 0.9133 | 0.9345 | 0.9233 |
LDA | 0.8527 | 0.9292 | 0.9411 | 0.9338 |
NB | 0.7876 | 0.9464 | 0.6624 | 0.7570 |
GBDT | 0.8697 | 0.9253 | 0.9317 | 0.9246 |
CART | 0.8680 | 0.9239 | 0.9277 | 0.9217 |
GSTCNet | 0.8917 | 0.9775 | 0.9533 | 0.9519 |
Model | AUC | Precision | Recall | F1-score |
GCN | 0.7284 | 0.9526 | 0.9455 | 0.9272 |
Bi-RNN | 0.8341 | 0.9628 | 0.9450 | 0.9388 |
Bi-GRU | 0.8165 | 0.9627 | 0.9424 | 0.9371 |
Bi-LSTM | 0.8398 | 0.9606 | 0.9420 | 0.9350 |
Att-Bi-LSTM | 0.7945 | 0.9619 | 0.9327 | 0.9303 |
CA-Bi-LSTM | 0.7994 | 0.9600 | 0.9252 | 0.9249 |
GCN+Bi-LSTM | 0.8375 | 0.9624 | 0.9433 | 0.9368 |
GCN+Att-Bi-LSTM | 0.7844 | 0.9605 | 0.9389 | 0.9328 |
GCN+CA-Bi-LSTM | 0.8829 | 0.9611 | 0.9436 | 0.9417 |
GSTCNet | 0.8917 | 0.9775 | 0.9533 | 0.9519 |
"+" denotes the combination of different basic network structures. |
Model | AUC | Precision | Recall | F1-score |
GCN | 0.7284 | 0.9526 | 0.9455 | 0.9272 |
Gated GCN | 0.7336 | 0.9642 | 0.9529 | 0.9307 |
CA-Bi-LSTM | 0.7994 | 0.9600 | 0.9252 | 0.9249 |
Gated CA-Bi-LSTM | 0.8057 | 0.9637 | 0.9345 | 0.9332 |
GCN+CA-Bi-LSTM | 0.8829 | 0.9611 | 0.9436 | 0.9417 |
Gated GCN+ CA-Bi-LSTM | 0.8875 | 0.9614 | 0.9498 | 0.9431 |
GCN+Gated CA-Bi-LSTM | 0.9015 | 0.9621 | 0.9487 | 0.9445 |
GSTNet | 0.8917 | 0.9775 | 0.9533 | 0.9519 |
"+" denotes the combination of different basic network structures. |