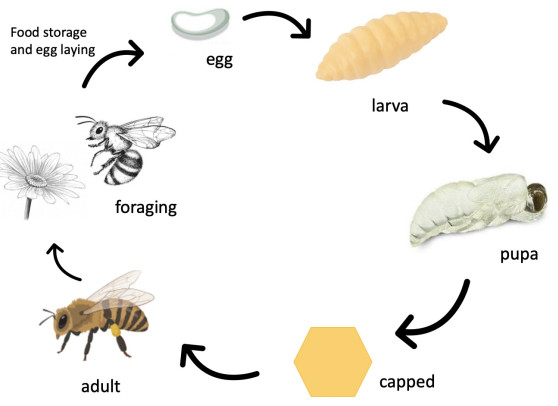
Honeybees have an irreplaceable position in agricultural production and the stabilization of natural ecosystems. Unfortunately, honeybee populations have been declining globally. Parasites, diseases, poor nutrition, pesticides, and climate changes contribute greatly to the global crisis of honeybee colony losses. Mathematical models have been used to provide useful insights on potential factors and important processes for improving the survival rate of colonies. In this review, we present various mathematical tractable models from different aspects: 1) simple bee-only models with features such as age segmentation, food collection, and nutrient absorption; 2) models of bees with other species such as parasites and/or pathogens; and 3) models of bees affected by pesticide exposure. We aim to review those mathematical models to emphasize the power of mathematical modeling in helping us understand honeybee population dynamics and its related ecological communities. We also provide a review of computational models such as VARROAPOP and BEEHAVE that describe the bee population dynamics in environments that include factors such as temperature, rainfall, light, distance and quality of food, and their effects on colony growth and survival. In addition, we propose a future outlook on important directions regarding mathematical modeling of honeybees. We particularly encourage collaborations between mathematicians and biologists so that mathematical models could be more useful through validation with experimental data.
Citation: Jun Chen, Gloria DeGrandi-Hoffman, Vardayani Ratti, Yun Kang. Review on mathematical modeling of honeybee population dynamics[J]. Mathematical Biosciences and Engineering, 2021, 18(6): 9606-9650. doi: 10.3934/mbe.2021471
[1] | Christopher M. Kribs-Zaleta, Christopher Mitchell . Modeling colony collapse disorder in honeybees as a contagion. Mathematical Biosciences and Engineering, 2014, 11(6): 1275-1294. doi: 10.3934/mbe.2014.11.1275 |
[2] | Dennis L. Chao, Dobromir T. Dimitrov . Seasonality and the effectiveness of mass vaccination. Mathematical Biosciences and Engineering, 2016, 13(2): 249-259. doi: 10.3934/mbe.2015001 |
[3] | Hao Wang, Yang Kuang . Alternative models for cyclic lemming dynamics. Mathematical Biosciences and Engineering, 2007, 4(1): 85-99. doi: 10.3934/mbe.2007.4.85 |
[4] | Adam Sullivan, Folashade Agusto, Sharon Bewick, Chunlei Su, Suzanne Lenhart, Xiaopeng Zhao . A mathematical model for within-host Toxoplasma gondii invasion dynamics. Mathematical Biosciences and Engineering, 2012, 9(3): 647-662. doi: 10.3934/mbe.2012.9.647 |
[5] | Rafael Bravo de la Parra, Ezio Venturino . A discrete two time scales model of a size-structured population of parasitized trees. Mathematical Biosciences and Engineering, 2024, 21(9): 7040-7066. doi: 10.3934/mbe.2024309 |
[6] | Lale Asik, Jackson Kulik, Kevin Long, Angela Peace . Dynamics of a stoichiometric producer-grazer system with seasonal effects on light level. Mathematical Biosciences and Engineering, 2019, 16(1): 501-515. doi: 10.3934/mbe.2019023 |
[7] | Chayu Yang, Jin Wang . A mathematical model for frogeye leaf spot epidemics in soybean. Mathematical Biosciences and Engineering, 2024, 21(1): 1144-1166. doi: 10.3934/mbe.2024048 |
[8] | Hongyong Zhao, Yangyang Shi, Xuebing Zhang . Dynamic analysis of a malaria reaction-diffusion model with periodic delays and vector bias. Mathematical Biosciences and Engineering, 2022, 19(3): 2538-2574. doi: 10.3934/mbe.2022117 |
[9] | Bruce Pell, Amy E. Kendig, Elizabeth T. Borer, Yang Kuang . Modeling nutrient and disease dynamics in a plant-pathogen system. Mathematical Biosciences and Engineering, 2019, 16(1): 234-264. doi: 10.3934/mbe.2019013 |
[10] | Lan Zou, Jing Chen, Shigui Ruan . Modeling and analyzing the transmission dynamics of visceral leishmaniasis. Mathematical Biosciences and Engineering, 2017, 14(5&6): 1585-1604. doi: 10.3934/mbe.2017082 |
Honeybees have an irreplaceable position in agricultural production and the stabilization of natural ecosystems. Unfortunately, honeybee populations have been declining globally. Parasites, diseases, poor nutrition, pesticides, and climate changes contribute greatly to the global crisis of honeybee colony losses. Mathematical models have been used to provide useful insights on potential factors and important processes for improving the survival rate of colonies. In this review, we present various mathematical tractable models from different aspects: 1) simple bee-only models with features such as age segmentation, food collection, and nutrient absorption; 2) models of bees with other species such as parasites and/or pathogens; and 3) models of bees affected by pesticide exposure. We aim to review those mathematical models to emphasize the power of mathematical modeling in helping us understand honeybee population dynamics and its related ecological communities. We also provide a review of computational models such as VARROAPOP and BEEHAVE that describe the bee population dynamics in environments that include factors such as temperature, rainfall, light, distance and quality of food, and their effects on colony growth and survival. In addition, we propose a future outlook on important directions regarding mathematical modeling of honeybees. We particularly encourage collaborations between mathematicians and biologists so that mathematical models could be more useful through validation with experimental data.
Honeybees, Apis mellifera, are essential for maintaining biodiversity in undisturbed ecosystems, and for the production of economically valuable agricultural crops worldwide [1]. One third of the food that we consume relies on pollination by bees for its production. Pollination by honeybees is valued between $15 and $20 billion annually in the US [2]. If higher-order industrial sectors that rely on the production of honey bee pollinated crops are included, the value of honey bees is an additional US$10.3 to $21.1 billion [3]. The work of Dr. vanEngelsdorp and Dr. Meixner [4] suggests that 1) although the global number of bees has been increased in the past 50 years, the geographical differences are huge even within the same region. And 2) the number of colonies in Europe and North America are both decreased. For the past 10 years, beekeepers have lost between 30–45% of their colonies annually with the most recent losses in 2019–2020 exceeding 40% (https://beeinformed.org/wp-content/uploads/2020/06/BIP_2019_2020_Losses_Abstract.pdf). Although there are more and more lost colonies, the reason why the number has not actually been decreased is that the beekeepers rapidly replace the lost colonies [4]. And beekeepers may adopt cold storage to help the colony survive over the winter smoothly [5]. These treatment methods are accompanied by relatively large costs in the context of apiculture. Therefore, the loss of the colony is a global economic, agricultural, and ecological crisis.
There is no single factor responsible for colony losses. Colonies die at different times of year due to a combination of stressors such as pesticides, lack of food sources and malnutrition, parasites such as Varroa destructor, Nosema ceranae, and viruses/pathogens including bacterial diseases. Varroa mites are considered to be one of the primary causes of colony losses worldwide [6-8]. Varroa can cause colonies to perish because parasitism compromises the bee's immune system and reduces the longevity of adult bees parasitized during development. Varroa also transmits and activates several viruses during feeding including Acute Bee Paralysis Virus (ABPV), Deformed Wing Virus (DWV) and Israeli Acute Paralysis Virus (IAPV). These viruses differ in their symptoms and virulence, but all reduce brood and adult bee survival and suppress colony growth. Colonies that are heavily infested eventually collapse from the combination of parasitism and disease especially overwintering in the U. S., Canada and Europe [4,7,9]. To prevent colony losses, beekeepers currently are applying miticides 5–7 times each year. Even with these measures, overwintering colony losses can exceed 30% [10]. Colonies also can be lost from Nosema infections. Nosema is a microsporidium and because of reduced metabolic capacities, relies heavily on its host to furnish energy for growth and reproduction [11,12]. Nosema infects adult bees and damages the midgut causing reduced nutrient absorption. Infected adult bees show symptoms of nutritional stress such as reduced larval feeding, earlier on set of foraging and reduced longevity of adult bees [13,14].
In addition to stress from pathogens and poor nutrition, colonies also can be lost from the impacts of pesticides. Pesticides can be toxic to honey bees due to their effects on the nervous system. However, pesticides also can have sublethal effects that exert stress that eventually lead to colony losses. For example, ingredients in pesticides can suppress the immune system, thus opening the way for parasitic infections and viral diseases [15-18].
The first mathematical model that incorporated colony population dynamics driven by biological and environmental factors was the BEEPOP model developed by DeGrandi-Hoffman et al. (1989) [19]. Other honeybee models followed (see review paper [20]) and can be classified as colony 'models' addressing within-hive colony dynamics (e.g., see [19,21-24]), 'Varroa models' exploring interaction between honeybees and Varroa mites (e.g., see [25-29]), also were developed as were 'foraging models' simulating the dynamics of honeybees collecting nectar and pollen to sustain colony growth (e.g., see [30-34]).
There is an increasing recognition of the importance of developing mathematically tractable models to obtain useful insights on ecological processes and factors that lead to colony losses. In this paper, we provide a review of recent progress in mathematical modeling of honeybee colony population dynamics since the review by [20]. We summarize existing models, and discuss their potential to identify combinations of factors leading to colony losses. Our review will focus on 1) honeybee only models (Section 2) with age and/or food/nutrient components; 2) honeybee-parasites and/or virus interactions models (Section 3); 3) models with effects from pesticides (Section 4); and 4) computational models (Section 5). We end by suggesting future directions for mathematical model development and applications. For convenience, in the Appendix, we also provide the equations of all models and tables that provide features of mathematical models that we review.
Honeybees are social insects that live in colonies consisting of a single egg laying queen, zero to several thousand reproductive males, and tens of thousands of reproductively sterile female workers, and 10,000–30,000 eggs, larvae and pupae (brood) [35-37]. Figure 1 provides a typical life cycle of a worker honeybee. Colonies rear brood and expand their populations in the spring and summer. Brood rearing declines in the fall and colony populations decrease in preparation for overwintering [38]. The population of workers in a colony is divided into two major temporal/behavioral subcastes: hive bees that remain in the colony and foragers that collect resources such as nectar and pollen from flowering plants, water to cool the hive, and plant resins (propolis) that has antibiotic properties and serves as part of the colony-level immune system) [39]. In general, tasks that worker bees perform are based on their age. The youngest adult workers (0–3 days old) clean cells in the brood nest, and usually by day-three begin feeding larvae. When workers are 10–14 days old, they participate in nest construction, food processing, and guarding the entrance of the nest. In the third or fourth week of life, workers transition to foraging outside the nest.
Though the dynamics of a colony population are complex, models that incorporate simple rules can reveal biologically relevant mechanics that drive population growth. For example, Dennis and Kemp (2016) [23] proposed and studied a deterministic, one-state-variable model that formulates the recruitment and loss rates of colony members in terms of density dependent social components as follows:
dAdt=λAθ+A⏟new workers with cooperative efforts−(αββ+A+μ)A⏟mortality with cooperative efforts, | (2.1) |
where A is the number of adult bees, λ is the upper maximum as adult population grows, and θ is the number of bees at which new worker population is half of maximum. The mortality function (αββ+A+μ) sets a minimum of per-individual mortality rate (μ) and a maximum of per-individual mortality rate (μ+α) for small adult population values; and β represents social effects on bees mortality. The model (2.1) incorporates cooperative effects in both birth and mortality terms that lead to strong Allee effects where there exists a critical size in adult bee numbers, below which mortality is greater than recruitment, with ensuing colony loss. The model suggests that the strong Allee effect may be the ultimate cause of colony death.
Kang et al. (2016) [40] developed a simple model that includes the cooperative effects in the honeybee colony as follows:
dHdt=rH2K+H2⏟new bees with cooperative efforts in colony−dH⏟mortality, | (2.2) |
where H is the honeybee population, r indicates daily egg-laying rate of queen, √K indicates colony size where brood survival rate is half maximum, d indicates mortality rate of bees. The model (2.2) used a Holling-Type III functional response (H2K+H2) to model collaborative efforts of hive and foraging bees to guarantee the survival of newborns. This approach is motivated by the work of Kang et al. (2011) [41] and Real (1977) [42]. We would like to point out that Dr. Eberl in his 2010 work [43] introduced a sigmoidal Hill function (HnKn+Hn) to model eclosion. Both work of Kang et al. (2016) [40] and Eberl et al. (2010) [43] have been applied in new research on honeybee population dynamics (e.g, see [22,29,44-48,50]). The nonlinear term H2K+H2 in model (2.2) can generate a saddle-node bifurcation in the system such that it induces an Allee threshold above which the colony can survive and below which the colony collapses. The model (2.2) has been extended to include age-structure [22], and the interactions with mites [29,48,49] and viruses [40].
The models (2.1) and (2.2) focus on colony populations without age-structure [23,40]. Honeybees go through different stages, thus it seems to be more realistic to propose and study population models with age-structure. In the next subsection, we will review honeybee models with age-structure, and the related models with the food resources and/or nutrition factors.
In this subsection, we will review two types of compartmental models with age structure: the models with delay terms that include varied brood stages and the compartment models without delay.
Motivated by biological stages of honeybee illustrated in Figure 1 and the honeybee-only model (2.2) from Kang et al. (2016) [40], Sweeney et al. (2019) [49] proposed the model (2.3) that includes brood larvae Bl, brood pupae Bp, and hive bees H as below:
dBldt=rH2K+H2+αBl⏟new larvae−dlBl⏟natural death−e−dlτlrH(t−τl)2K+H(t−τl)2+αBl(t−τl)⏟maturation into pupae stage,dBpdt=e−dlτlrH(t−τl)2K+H(t−τl)2+αBl(t−τl)⏟maturation from larvae stage−dpBp⏟natural death−e−dpτprH(t−τl−τp)2K+H(t−τl−τp)2+αBl(t−τl−τp)⏟maturation to adult,dHdt=e−dpτprH(t−τl−τp)2K+H(t−τl−τp)2+αBl(t−τl−τp)⏟maturation to adult−dhH⏟natural death, | (2.3) |
whose dynamics are similar to the model (2.4) by Chen et al. (2020) [22]:
dBdt=rH2K+H2+αB⏟new brood production−e−dbτrH(t−τ)2K+H(t−τ)2+αB(t−τ)⏟maturation from brood to adult−dbB,dHdt=−e−dbτrH(t−τ)2K+H(t−τ)2+αB(t−τ)⏟maturation from brood to adult−dhH⏟mortality, | (2.4) |
where B indicates brood stages, H indicates adult stage, r indicates daily egg-laying rate of queen, √K indicates colony size at which brood survival rate is half maximum, α is the regulation effects of brood, and db and dh indicate mortality rate of brood and adults, respectively. Both models assume that the new brood rH2K+H2+αB or rH2K+H2+αBl is positively regulated by adults and negatively regulated by brood B or Bl.
Both the three component model (2.3) and the two component model (2.4) exhibit strong Allee effects generated from collaborative effects of colony members, and colony survival when the egg-laying rate is above certain threshold. The dynamics comparison suggests that the additional age structure may not affect dynamics much. To further explore the effects of age structure, both age structure models are compared to the adult bee only model (2.2). It seems that the model with or without age structure has equilibrium dynamics, and additional age structure components in models may not contribute much in population dynamics. However, age structure may play an important role when additional mortality factors such as parasitism are included in the model. We will come back to this topic in Section 3.
Egg laying by the queen varies with season and colony conditions [29]. Chen et al. (2020) [22] included a variable egg laying to Messan's data-based model [29,50], and used the following periodic function (r(t)) (2.5) for queen's egg-laying rate:
r(t)=r0∗(1+cos(2π(t−ψ)γ)), | (2.5) |
where τ indicates the time in the juvenile period; ψ is the time of the maximum laying rate; and r0 is the baseline egg-laying rate. The study in Chen et al. (2020) [22] shows that seasonality may inhibit the survival of bee colonies, and at times prevent it from collapse.
Khoury et al. (2011) [21] developed a compartmental ordinary differential equation (ODE) model to determine the impact of forager death rates on colony growth and survival. The model includes age polyethism and division of labor where the adult worker population is divided into hive bees H and foragers F:
dHdt=L∗H+FH+F+ω⏟eclosion−H∗[α−σ(FF+H)]⏟recruitment to foraging,dFdt=H∗[α−σ(FF+H)]⏟foraging recruitment−dfF⏟mortality. | (2.6) |
This model assumes that the eclosion term L∗H+FH+F+ω (i.e., adult emergence from pupal state [51]) approaches the maximum egg laying rate L as colony size gets large; and α−σ(FF+H) is the recruitment function, α indicates the maximum rate that hive bees will become foragers when there are no foragers existent in the colony. Foragers can revert back to workers through social inhibition which is modelled by σ(FF+H) indicating that too many foragers can reduce the rate of hive bees becoming foragers. There is a critical threshold forager death rate beneath which colonies regulate a stable population size, and above which population decline and colony failure is predicted. The model includes recruitment of hive bees into the forager population when forager death rates are high. The recruitment of hive bees simulates precocious foraging that ultimately shortens the lifespan of adult bees, and if sustained for a long enough period, causes the colony to die. Their model provides more detailed observation of honeybee population dynamics, that the model shows that the adults switch between hives and foragers due to supply and demand, and provides a theoretical framework for experimental investigation of the colony failure. Therefore, much of the studies after this are based on the theories of Khoury et al. (2011) [21] and expanding their models to further study the reasons for the failure of honeybee colony caused by more factors [30,32,52,88,89,98,107,108,110].
Based on the model above (2.6), Booton et al. (2017) [52] proposed a hive-forager model with external stress to investigate the impact on the regulatory processes of recruitment to the forager class, social inhibition and the laying rate of the queen.
dHdt=L∗H+FH+F+ω⏟eclosion−H∗[α−σ(FF+H)]⏟recruitment−H∗μH+F+ϕ⏟stress−H∗γ(H+F)⏟limiting function at high densities,dFdt=H∗[α−σ(FF+H)]⏟recruitment−dfF⏟mortality−F∗μH+F+ϕ⏟stress, | (2.7) |
where H is the in-hive worker population and F is forager population. The model (2.7) predicts that constant density-dependent stress acting through an Allee effect on the hive can result in sudden catastrophic switches in dynamical behaviour and the eventual collapse of the hive. In addition, the model suggests that increased stress levels can be counteracted by a higher egg laying rate, lower levels of forager recruitment or lower levels of natural mortality of foragers. Increasing social inhibition cannot maintain the colony under high levels of stress.
The interactions between food and population dynamics in honeybee colonies are very complex. Food collection is affected by the forager population size and food availability in the colony and the environment outside the hive. If food resources are limited in the colony, hive bees (H) will be recruited to become foragers (F) (precocious foragers). Precocious foraging shortens the lifespan of adult workers and affects the age structure of the colony and the amount of brood it can rear. These dynamics were captured in a model by Khoury et al. (2013) [30] when they extended their model (2.6) in Khoury et al. (2011) [21] by including food availability (f) and brood population (B) to explore how food availability interacts with the intrinsic demographic processes, and used time delay (τ) in maturation term to present the days of adult bees emerging from pupation:
dBdt=Lf2f2+b2HH+v⏟new brood−ϕB(t)⏟maturation from brood to hives,dHdt=ϕB(t−τ)⏟maturation from brood to hives−H(αmin+αmaxb2b2+f2−σFF+H)⏟recruitment,dFdt=H(αmin+αmaxb2b2+f2−σFF+H)⏟recruitment−dfF⏟death,dfdt=cF⏟food collected−γbB⏟brood consumption−γhH⏟hives consumption−γfF⏟foragers consumption. | (2.8) |
The study of the model (2.8) above suggests that both food availability and forager death rate have very strong influences on colony growth and development. It predicts that colony population eventually stabilizes at an equilibrium size determined by the forager death rate, and is no longer affected by increasing food availability, whereas food stores continued to increase. The dynamics of the model suggest that different forager death rates result in qualitatively and quantitatively different colony outcomes, which range from a stable population with an excess of food stores, to a stable population with limited food stores, to zero population with residual food stores. Their new model adds the influence of food to the original recruitment term, it is used to explore the dynamic of colony by interaction between the food storage and foragers mortality, and also provides a mathematical foundation for more complex performance of the honeybee colony [32,89].
Precocious foraging occurs under chronic stress from lack of food, disease, or loss of foragers. Precocious foraging reduces effective foraging and forager longevity thus leading to low food reserves and high mortality rates. In order to identify the most effective strategies to improve colony resilience, Perry et al. (2015) [32] extended the model in Khoury et al. (2013) [30] to the following one, the population of uncapped brood is denoted by B, hive bees by H, foragers by F, and the weight of stored food by f:
dBdt=Lf2f2+b2HH+v⏟new brood−ϕB(t)⏟maturation from brood to hives,dHdt=ϕB(t−τ)⏟maturation from brood to hives−H(αmin+αmaxb2b2+f2−σFF+H)⏟recruitment,dFdt=T(a)H(αmin+αmaxb2b2+f2−σFF+H)⏟Recruitment with survival rate−dfM(a)F⏟death,dfdt=cTN(a)F⏟food collected−γbB⏟brood consumption−γa(H+F)⏟adults consumption, | (2.9) |
which assumes that the amount of trips taken and transitional survival (T(a)) are a function of the age at onset of foraging. The death rate of foragers is a function of the age at onset of foraging. The model (2.9) by Perry et al. (2015) [32] assumes that food storage depends on foraging trips which in turn depends on the age of onset of foraging. This assumption resulted in a breakdown in the division of labor and loss of the adult population, leaving only brood, food, and few adults in the hive. This work explains social processes that may drive rapid depopulation of a colony, and explores possible strategies to prevent colony failure.
In addition to the above-discussed food and the dynamics system of honeybee population, there are also regulatory mechanisms for task allocation via the "common stomach" (sometimes called "social crop"). The concept of a "common stomach", where all bees in a colony have similar amounts of food in their crops, has been incorporated in varied models for honeybees [53-56]. Honeybees communicate substance/information through a common stomach to store or retrieve pollen or nectar [53]. To study the economic benefits of pollen and nectar foraging strategies to both plants and honeybees under different weather conditions, Schmickl and Karsai (2016) [55] proposed and studied a detailed model that includes the nectar flows and nectar qualities offered by plants under distinct raining conditions. This model [55] simulates three strategies (adaptive, fixed and proactive) under different rains periods that affect protein stores and consumption rates in the colony. The adaptive strategy is similar to the principles that honeybees follow in that there is relatively a low-level of pollen foraging in good weather. During periods of rain when foraging activity stops, there is decreased pollen consumption and brood cannibalism. Vigorous pollen foraging occurs after the rainy period. Fixed strategy is that bees forage on pollen at a fixed recruitment rate and will not conserve protein when pollen is scarce. The proactive strategy is based on the fixed strategy, except that foraging rates enable an excess of pollen to be stored for periods of inclement weather. The model indicates that the adaptive strategy benefits bees and plants in that increased pollen foraging can compensate for lost pollen collection during the rain, while increasing pollination rates. Brood survival also was greater in colonies employing the adaptive strategy, but this depended upon the rain pattern with tropical patterns having less impact than continental. The model also indicates that the proactive strategy may cause colonies to optimize growth, but because it is at the expense of nectar collection the colony will be low on nectar stores causing starvation over the winter.
Schmickl and Karsai (2017) [56] extended the model of Schmickl and Karsai (2016) [55] to study the resilience and homeostatic regulation of honeybee colonies by including two "common stomachs" and the related foraging behavior. The first common stomach is the saturation of workers and brood with proteins that affect both the influx and the consumption of pollen. The second common stomach is the saturation of nectar stores, which affects the ratio of loaded to unloaded foraging bees returning to the hive, which in turn regulates the recruitment of nectar foraging bees. This model has a longer time scale than the previous study [55]. The study of Schmickl and Karsai (2017) [56] combined the simulation results with actual experimental data to explore the dynamics of adult forager populations, brood population, and protein and sugar stocks in the hive under varied environmental conditions.
The growth, development, productivity, and health of a honeybee colony is dependent upon fulfilling the nutritional demands of larvae and adult workers [57]. Vitellogenin (VG) is an egg yolk protein which is the primary source of amino acids [58], and it appears to be one of the most important regulators of immunity and longevity of honeybees [59-61]. The recent modeling work on the effect of honeybee nutrition on colony survival by Messan et al. (2018) [50] investigated how VG may affect colony dynamics (please see the detailed model in Appendix B model (B.1)). The non-linear differential equation system describes how population dynamics of colonies are influenced pollen stores and VG. Their study [50] focused on the effects of VG content per nurse bee and how it interacts with the essential demographic and allocation processes within the colony to influence colony growth. They showed that the size of both the brood and worker populations are directly dependent upon the increase of levels of VG titers in nurse bees; and the coexistence of both brood and worker populations is also dependent upon the quantity of food fed to the brood' (e.g., pollen collected and converted to VG and available foragers). In addition, the study in Messan et al. (2018) [50] explored how seasonal changes in pollen collection improves the prediction of long term colony dynamics.
The model proposed and studied in Messan et al. (2018) [50] is unique and different than most models in the literature that study the population dynamics of honeybees and nutrient stores. For example, Schmickl and Crailsheim (2007) [62] created a simulation model to study the population and resource dynamics of a honeybee colony and included the effect of division of labor in the hive. Their modeling approach is too complicated to be mathematical trackable. The model presented in Khoury et al. (2013) [30] has similar assumptions in the model of Messan et al. (2018) [50] with one of the main differences being that the assumption of the transition from nurse bee to forager. The model of Messan et al. (2018) [50] assumes that the transition from nurse bee to foragers increases by a transition rate that depends on the levels of VG in the nurse bee and not only by the absence of stored food and reduced social inhibition. In BEEHAVE by Becher et al. (2014) [33], they assume that the level of pollen and nectar stores in the colony affects the age at which workers initiate foraging activities while they do not take into account mechanisms changing at the molecular [60,63-65] and physiological level [60,66] as the model of Messan et al. (2018) [50].
The study by Messan et al. (2018) [50] presented basic but important assumptions that can help us understand and have greater insights in the complexity of honeybee population dynamics given their nutritional status. Their model can be extended by limiting the influx of pollen in the late summer to determine the effects on adult lifespan and colony survival. The effects that brood cannibalism or breaks in brood rearing at different times of year can have on colony survival also can be studied through simulations. Other mechanism to be considered is the transition back from forager to nurse bee under certain environmental conditions [67], and the interplay of diseases or infections, such as Nosema ceranae which is known to alter VG levels, and therefore alter normal age polyethism causing colony imbalance [68,69].
Social insect groups have evolved a collective immune defense against parasites. These "social immune systems" are built on the cooperation of individual members in the colony to fight the spread of disease. Collective defense can prevent the entry and spread of parasites. In the study of the social immune system, it is necessary to carry out a complex integration of information about the parasites and the internal state of the colony [70]. Laomettachit et al. (2021) [71] proposed a system (please see the detailed model in Appendix B model (B.2)) that describes the spread of infection within the colony when honeybee members engage in the trophallactic activity to distribute nectar to study the social immunity in honeybee society. Their model considered the social segregation of worker bees and a hygienic response. The segregation limits the forgers that exposed to pathogens interacting with bees in the colony, and hygienic response is the healthy nurses exterminate infected bees to control the infections spreading. Their study suggested that the segregation is the first line of defense in pathogens into the colony, and the hygienic response is the second line of defense. Their study also found the egg-laying rate is a critical factor in maintain healthy colonies against infection. In summary, they suggested the egg-laying rate, that is decreasing or pause combining the infections in the early spring, can damage the social immunity, and even cause colony collapse.
Honeybee colonies provide a favorable environment for parasites and pathogens because of the warm temperature and the high concentration of hosts, which may contribute to honeybees disappearing in large numbers across the globe [72]. Studies showed that most of the losses could be generally attributed to Varroa mites who have been implicated as the main culprit in dying colonies [73-75]. In the following subsections, we will provide review of mathematical modeling that includes Varroa alone and with virus transmission. We also discuss a model of the effects of Nosema on colony dynamics.
Varroa mites are a parasite of both adult bees and the developing brood and can shorten the life span of their hosts [79]. Adult female mites undergo two phases in their life cycle, the phoretic and reproductive phases. During the phoretic phase, female Varroa feed on adult bees and move from bee to bee as they pass one another in the colony. Mite reproduction can occur only if honeybee brood is available [24]. The reproductive phase begins when a mature female leaves her adult host, enters a brood cell containing a larva shortly before it is capped, and sequesters herself in the bottom of the cell. Egg-laying commences about 60 hours after a cell is capped, and both mother and offspring feed on the host's fat body [80]. Mature offspring mate within the cell, but only mature females survive outside the cell. In the average temperate climate, mite populations can increase 12-fold in colonies which have brood half of the year and 800-fold in colonies which have brood year-round. The period of mite population increase continues through the spring and summer and peaks in the fall when brood rearing is nearly done. This makes the mites very difficult to control, especially in warmer climates where colonies maintain brood year-round [28].
Sweeney et al. (2019) in his undergraduate honor thesis [49] developed a realistic five-dimensional honeybee-mite interaction model with age structure in both honeybee and mite (see Figure 2, and the detailed math model in Appendix B model (2.3)). This model includes three delay terms: one delay term e−dlτlrH(t−τl)2K+H(t−τl)2 describes the transition from larvae to pupae, and the second one e−∫tt−τp[dp+αpMp(s)a+Bp(s)]dsrH(t−τp−τl)2K+H(t−τp−τl)2 describes the transition from pupae to adult; and the third delay term cαpBp(t−τp)a+Bp(t−τp)Mp(t−τp) describes the newborn mites coming from the parasitism of honeybee brood B. The modeling approach of those delay terms is adapted from Chen et al. (2020) [22].
The advantages of the honeybee-mite interaction model proposed and studied by Sweeney et al. (2019) [49] are that it includes 1) three stages in honeybees: larvae, pupae, and adult bees; 2) two stages in mites: phoretic stage and reproductive stage; 3) mites at the phoretic stage are parasitizing adult bees H which contributes to the additional mortality rate; and 4) mites at the reproductive stage are parasitizing pupae Bp to reproduce. The simple analysis and the simulations suggest that parasitism combined with the stage structures can destabilize the system causing fluctuating dynamics.
The disadvantage of the proposed model by Sweeney et al. (2019) [49] is the difficulty of rigorous mathematical analysis. To explore the mechanisms that generate instability of the honeybee-mite interaction dynamics, Messan et al. (2021) [29] simplified the model of Sweeney et al. (2019) [49] to the following three-dimensional honeybee-mite interaction model:
dBdt=rH2K+H2⏟egg production−αbprobability of Mattaching to B⏞Ba+BM⏟parasitism on brood−dbB⏟natural death−e−∫tt−τ[db+αbM(s)a+B(s)]dsrH(t−τ)2K+H(t−τ)2⏟maturation from egg to adult,dHdt=e−∫tt−τ[db+αbM(s)a+B(s)]dsrH(t−τ)2K+H(t−τ)2⏟transition from brood−αhprobability of Mattaching to H⏞Ha+HM⏟parasitism on adult bee−dhH⏟natural death,dMdt=cαbBa+BM⏟newborns from brood parasitism−dmM⏟natural death, | (3.1) |
where the brood B consists of both larvae Bl and pupa Bp in model (B.4), and no stage structure for the mites. When there is no mite in the system, i.e., M=0, the model (3.1) reduces to the two-stage honeybee model (2.4) studied by Chen et al. (2020) [22]. Both the work by Chen et al. (2020) [22] and the model (B.4) without mites by Sweeney et al. (2019) [49] suggest that time delay does not affect dynamics, while the study of models (3.1) and (B.4) shows that the large time delay in the presence of parasite mites (i.e., M>0) could destabilize population dynamics and drive the colony to collapse. In addition, both models [22,29] use real data to show the effects of seasonality Eq (2.5), and their study suggested seasonality is a key factor for colony's collapse or survival.
Both age-structured honeybee models without mites in models (3.1) and (B.4) suggest that the delay or age-structure terms may not play significant roles in population dynamics. Kang et al. (2016) [40] proposed a simple honeybee only model (2.2) that has been extend to the honeybee-mite interaction model without age-structure.
dShdt=rS2hK+S2h⏟newborns−dhSh⏟ death−αShSm⏟negative effects of parasitism,dSmdt=cαShSm⏟newborns of mites−dmSm⏟ death, | (3.2) |
where Sh indicates the population of honeybees (includes all brood and adults) and Sm indicates the population of mites. Their model can have bistability and go through the subcritical Hopf-bifurcation that leads to the extinction of colony. The study shows that parasitism could destabilize population dynamics of honeybees.
Messan et al. (2017) [48] extends the model (3.2) to the following simple two-patch honeybee-mite interaction model (B.5), the detailed math model in Appendix B (B.5), to explore the mite migration effects on the population dynamics of honeybees and mites. This study suggests that migration between two patches can generate extremely complicated dynamics including multiple attractors, and plays the key role in determining whether honeybee colony survives or collapses. For example, the study shows that the median migration rate can potentially enable mites and honeybees to coexist, while a high migration rate can rescue one colony in two patches where both were going to collapse.
Viruses are one of the main threats to the health and survival of honeybees. More than 20 viruses have been identified to infect honey bees worldwide [33]. The viruses most commonly detected are: Acute Bee Paralysis Virus (ABPV), Black Queen Cell Virus (BQCV), Deformed Wing Virus (DWV), Israel Acute Paralysis Virus (IAPV), Kashmir Bee Virus (KBV), Sacbrood Virus (SBV) and Chronic Bee Paralysis Virus (CBPV) [81]. For a complete discussion of symptoms associated with each virus and the mode of transmission see [82]. Though several different viruses can infect worker bees throughout the year [83,84], by the fall, DWV often is the predominant virus detected [85]. This is because of differences in the quantitative dynamics among the viruses transmitted by Varroa. Those dynamics can generate a shifting succession of virus infections that ultimately leaves DWV as the predominant infection [86]. High levels of DWV in the fall are a harbinger of colony loss over the winter [87].
Kang et al. (2016) [40] extended their honeybee only model (Eq (2.2)) to the honeybee-virus model (3.2) shown below:
dShdt=r(Sh+ρIh)2K+(Sh+ρIh)2⏟Newborns−dhSh⏟natural mortality−βhShIhSh+Ih⏟infectious effect,dIhdt=βhShIhSh+Ih⏟infectious effect−(dh+μh)Ih⏟death, | (3.3) |
which assumes that infections only spread horizontally with additional mortality μh in infected honeybees, new adults bees are all healthy, and the virus-infected bees have reduced performance that is denoted by ρ. The dynamics of model (3.3) seem to be relatively simple: i.e., no limit cycle. The study of Kang et al. (2016) [40] shows that 1) honeybees can persist with proper initial conditions when the virus transmission rate among honeybees βh is not large, 2) both honeybees and the virus can coexist if βh is in the medium range, and 3) the large virus transmission rate βh among honeybees can drive the colony to extinction.
Kribs and Mitchell (2014) [31] investigated the scenario that CCD is provoked by a transmissible pathogen or contaminant introduced by foraging activities to understand how colony collapse might occur as a perturbation of normal hive dynamics. In the model, H indicates hive bees, F indicates forager bees, and I indicates infected bees.
dHdt=LHH+Ω⏟eclosion−(γH+αHΦF+I+Φ)⏟recruitment,dFdt=(γH+αHΦF+I+Φ)⏟recruitment−μ1F⏟natural death−βFII+K⏟infectious effect,dIdt=βFII+K⏟infectious effect−μ2I⏟death due to disease, | (3.4) |
where virus infection brought into the colony is by the foragers and spreads in the hive via both direct bee-to-bee transmission and indirect (via contaminated plant vectors) transmission. The disease dynamics assumed in this paper are very general, and is not particularly discussed in relation to any vector or virus. But this model still helps us to understand the dynamics of healthy colonies and colonies collapsing because of CCD, especially the impact of accelerated forager recruitment on the collapse of the colony, and the balance between recruitment rate and laying egg rate.
Betti et al. (2016) [88] proposed a PDE model that takes into account age structure and seasonal effects on the dynamics of a colony infected with a pathogen.
∂HS∂t+∂HS∂a=−u(a)HS⏟recruitment−βˉNHS⏟infectious effect,∂HI∂t+∂HI∂a=βˉNHS⏟infectious effect−u(a)HI⏟recruitment−dH(a)HI⏟death due to disease,∂FS∂t+∂FS∂a=u(a)HS⏟recruitment−μ(a)FS⏟natural death−βˉNFS⏟infectious effect,∂FI∂t+∂FI∂a=u(a)HI⏟recruitment+βˉNFS⏟infectious effect−(μ(a)+dF(a))FI⏟death, | (3.5) |
where a is age and t is time, for example, HS(a,t) means susceptible hive bees of age a at time t and μ(a) is natural death rate. The model assumes that the hive bees and foragers spread the infection at a rate (β) between or within classes. Figure 1 shows the processes between hive bees and foragers are social inhibition and transition. The term u(a) represents similarly in the recruitment of Khoury et al. (2011) [21]: u(a)=α(aa+k)2(1−σN∫(Fs+FI)da)HV(a−aR) with N being the total number of bees, α being the maximum rate of recruitment, k being that recruitment will be half the maximum rate of recruitment and 1σ being the maximum proportion of bees that are foraging at any given time. The term HV(a−aR) is the Heaviside function to reflect that recruitment cannot begin before age aR. When determining the initial value, the food factor is introduced, HS(0,t)=LS, where L indicates the daily egg-laying rate of the queen, and S is the survivability function that includes food to determine how many eggs can survive.
The PDE model (3.5) shows that the age-dependent recruitment rate affects the dynamic of disease within the colony. The natural age-structure has a certain control effect on disease transmission. In other words, if there is an interference with the age-structure, it may also increase the extent of infection. The modeling work by Chen et al. (2020) [22] and Messan et al. (2021) [29] show that time delay alone may not have huge impacts on the dynamic of bee-only model, while delay combined with mites may lead to colony collapsing. The model by Betti et al. (2016) [88] showed that the maturation time from hive bees to foragers affects both colony dynamics and infection dynamics if a pathogen is present in the colony. The age dependent model in Betti et al. (2016) [88] captures the dynamics of 'spring dwindling' which occurs due to the fact that worker bees emerged from the previous fall are dying faster than the emergence of new workers in the spring. In addition, the model of Betti et al. (2016) [88] also includes seasonality by adding a transition model after end-of-winter to expose the process of colony aging from winter months to active young bees. The work of Betti et al. (2016) [88] indicates that colonies are particularly vulnerable to collapse during spring dwindling especially if disease or other stress factors are present; and the declining population of bee colony in the early spring is the natural consequence due to seasonal vulnerability.
The host-parasite relationship between honeybees and Varroa mites has been complicated by the mite's close association with a wide range of honeybee viral pathogens. While Varroa parasitism can weaken honey bee immunity and deplete nutrient reserves in their host, the most serious threat is transmission of viruses [82,90,91]. Varroa transmit numerous viruses during feeding on developing and adult bees. Mathematical models are powerful tools that could help us obtain insights on how mites and/or viruses contribute to the collapse of honeybee colonies.
Several models have captured the mechanics of virus infections in colonies in the presence of mites [40,92]. Kang et al. (2016) [40] proposed and studied a honeybee-mite-virus model (B.6) shown in Appendix B model (B.6)) that incorporates parasitic interactions between honeybees and Varroa mites with terms describing virus transmission between bees and mites at different lifestages. The honeybee-mite-virus model (B.6) incorporated colony's internal organization (i.e., division of labor) that can generate Allee effects, and five virus transmission terms between honeybees and mites at different stages of Varroa mites. The routes of transmission include from: honeybees to honeybees, adult honeybees to phoretic mites, honeybee brood to reproductive mites, reproductive mites to honeybee brood, and honeybees to phoretic mites.
The model (B.6) captures the synergistic effects of parasitism and virus infections on honeybee population dynamics and persistence of virus in the colony. The components included in the honeybee-mite-virus model (B.6) allow us to explore the dynamics of the: honeybee only model (2.1), honeybee-mite model (3.2), honeybee-virus model (3.3), and honeybee population with both mites and viruses. The model revealed that colony survival is strongly affected by initial conditions (i.e., colony size) due to Allee effects experienced by the honeybee population. High adult to brood ratios enable coexistence of Varroa and virus in the population. However, low adult to brood ratios have destabilizing effects on the system which generate fluctuating dynamics that lead to a catastrophic event where both honeybees and mites become extinct. This model outcome is similar to colony losses over the winter that are commonly seen under conditions of high Varroa and virus (particularly DWV) titers in the fall [87]. Additionally, persistent viral infection in late winter and early spring can lead to high mortality of worker bees, and an inability to rear sufficient amounts of brood (Allee effects) to enable the colony to build in the spring.
The model (B.6) by Kang et al. (2016) [40] applies to disease/virus in the general fashion. Dénes and A. Ibrahim (2019) [93] studied a honeybee-mites-virus system with susceptible bees, bees infected with mites and bees infected with viruses (please see the detailed math model in Appendix B model (B.7)). Their results suggest that in order to eradicate disease/mites, the related transmission rates needs to be decreased. In the following subsections, we will provide reviews on literature work that have been applied to specific viruses such as ABPV and DWV.
Acute Bee Paralysis Virus (ABPV) and Deformed Wing Virus (DWV) are transmitted by Varroa[82,94]. ABPV is deadly in the sense that brood infected with it do not metamorphose to the adult stage. DWV causes death of the pupae and reduced longevity of adult bees [90,91]. And American Foulbrood (AFB) is a fatal bacterial disease of honey bee brood, and AFB is considered a very destructive and lethal brood disease [95].
Sumpter et al. (2004) [92] proposed a model that used SIR-like framework to study the effect of a honeybee colony dynamics with Varroa mites and virus. They studied ABPV and DWV separately. The model assumed the total number of mites is constant, therefore the death and birth rates of mites are not important in their model. Since viruses can exist in different stages of the life cycle of bees, seasonal effects are included and reflected in the values of different parameters for each season. A critical mite load that a colony can tolerate was estimated, and it was determined that as the number of mites exceeds the critical mite load, the disease free equilibrium becomes unstable, which means an epidemic occurs. Recommendations for reducing colony losses generated from the model were that mite and virus levels need to be controlled in the summer, and delaying control until fall increases the likelihood of colony loss.
The process of bee eclosion depends on how well the brood is cared for during development. For that purpose, a sufficient number of healthy adult worker bees are required. The relationship between healthy adult workers and eclosion was modeled by Eberl et al. (2010) [43] by extending the ABPV model by Sumpter and Martin (2004) [92]. They formulated brood maintenance as a Sigmoidal Hill function which introduced Allee effects and led to the collapse equilibrium being locally stable which was not present in Sumpter and Martin (2004) [92]. The model predicted colony death at points where the uninfected population drops below a certain threshold that is dependent on the brood maintenance terms. We would like to point out that Eberl et al. (2010) [43] also includes the seasonality through simulating different parameters values in four seasons. Their simulations suggested that an infected colony may collapse after several years in the spring. This result is in line with the work by Betti et al. (2016) [88].
Since the transmission of ABPV depends on the Varroa mites, it is important to track the mite population. Therefore, Ratti et al. (2012) [44] (see also Devillers (2014) [97]) adopted the framework of Eberl et al. (2010) [43] and introduced logistic growth for mites (please see the detailed model in Appendix B model (B.8)). As a result, mite-induced death rates have also been considered. Ratti et al. (2012) [44] assumed that the carrying capacity (α(HS+HI)) is dependent on the total bee population which is a proxy for the brood size. For a model with logistic growth of mites, infestations may lead to a die-off of the colony or to bee-mite coexistence. The bee colony that otherwise coexists with mites might start dying when the virus is introduced. This die-off may take several years. Whether or not the bee population can fight off a virus epidemic depends on model parameters describing disease transmission and how fast infected bees die, as well as on the size of the mite population in the stable bee-mite coexistence equilibrium. They studied the model in constant-coefficients analytically and then investigated in computer simulations whether or not these findings carry over to the more general transient case in which the parameters are taken as seasonal averages. The model did not take into account seasonal or yearly fluctuations in conditions.
Research has shown that seasonality has the potential to change the dynamics of infectious diseases since it creates complex fluctuations in the host and vector populations. Most infectious diseases change with temperature, rainfall or other seasonal effects most of which are periodic and predictable. The effects of seasonality on honeybee and thus mite populations have been reported in the literature. Ratti et al. (2015) [45] adopted the framework of Ratti et al. (2012) [44] to study the seasonal effects on the mite and virus-infested colony by considering the coefficients to be periodic functions of time with a period of 1 year. They used Floquet theory to analyze the stability of virus-free periodic solutions. For controlling vector-borne disease, it is required to control the vector. Therefore, Ratti et al. (2015) [45] studied the effect of varroacide application by introducing additional death rates (δi) in the bees and mite equations. In addition to the existence of periodic endemic solution in the absence of virus, it was found that the success of varroacide treatment depends on the cumulative efficacy over the year, rather than a specific time course. They also found that in order to keep disease under control, it is not necessary to eradicate mites completely.
Forager bees from diseased colonies experience reduced homing and flight capabilities which makes it important to track forager populations in models as in Khoury et al. (2011) [21]. Ratti et al. (2017) [47] constructed a model combining their ABPV model (from Ratti et al. (2015) [45]) with a division of labor component from Khoury et al. (2011) [21] to study forager loss. In their model (B.9) (please see the detailed model in Appendix B model (B.9)), they assumed that the total bee population was divided into hive bees and foragers, all newly emerged bees are uninfected, and only horizontal virus transmission occurs vectored by Varroa. Ratti et al. (2017) [47] categorized the population into uninfected hive bees (H), uninfected forager bees (F), infected hive bees (HI), virus-free mites (MS) and virus-carrying mites (MI) (see Eq (B.9)). Ratti et al. (2017) [47] The model predicted that mite and bees can co-exist without treatment in virus-free conditions. If virus exists and a varroacide is applied causing a decline in virus-carrying mites that exceeds the mite birth rate, the disease-free equilibrium can be stable. Simulation indicates that infected colonies eventually collapse in the absence of Varroa treatment.
By following the work of Henry et al. (2012) [98], Ratti et al. (2017) [47] incorporated the increased forager mortality due to exposure to pesticides as a result of which the bees fail to return to the hive. The idea was to study the dynamics of the colony under multiple stressors (mites, virus, and pesticides). Though they investigated the effect of increased forager loss particularly due to pesticides, the framework can be easily adapted to forager loss due to other factors. They also suggested that a honeybee colony that experiences periods of increased forager loss early in the season can withstand these losses and recover to (almost) normal strength, if the losses remain below a defined threshold. If the losses exceed this threshold the colony fails rapidly. The level of external forager loss that can be tolerated is only slightly higher for healthy colonies compared to colonies with Varroa and ABPV.
Britton et al. (2021) [99] proposed the first model to study the dynamics of DWV that classified bees as covertly infected (HX), chronically overtly infected (HY), and acutely overtly infected (HZ), and mites as covert infected (MU) and overt infected (MV) based on the infection levels. The model equations for spring, summer, and fall are given by
dHXdt=αe−cMVh(HX+κHY)−μHX−γMHXN−ρHX−τHXHYN−τ′HXHZN−ϕHXNMV,dHYdt=ρHX+τHXHYN+τ′HXHZN−νHY−γMHYN+ϕHXNMV,dHZdt=αp(1−e−cMV)h(HX+κHY)−ηHZ−γMHZN,dMUdt=rMU+r(1−θ)MV−rMUMkN−σMU−ψMUHYN−ψ′MUHZN,dMVdt=rθMV−rMVMkN+σMU+ψMUHYN+ψ′MUHZN. | (3.6) |
The mite model for winter is given by
dMUdt=−sMU,dMVdt=−sMV. | (3.7) |
Moreover, they modeled the spontaneous transition from one infected class to the other, and included logistic growth for mites for all seasons except winter [44,45,47]. Since there is no brood in winter, the mite population dynamics is much simpler and hence the mite equations are reduced to having only negative linear terms (see Eq (3.7)). Mites are no longer considered to be a mechanical vector; the virus actually replicates in the body of the mites. For the bee dynamics, they factored in the vertical as well as horizontal transmission. When possible, they calculated the R0 using next generation matrix. The model did not incorporate seasonal variations analytically or computationally. The focus of this paper was on gaining the insight into the disease dynamics by computing the reproduction number using weighted directed graphs with the next generation method. They found that in the absence of mites, the condition for overt infection leads to unrealistic results. They also found that the overt infection can be maintained either by the transmission of overt virus between adult bees (for unrealistic parameters) or by vertical transmission in mites coupled with their interactions with acutely overtly infected bees. This demonstrates the importance of considering transmission involving mites and chronically overtly infected bees.
Datta et al. (2013) [100] studied the spreading of American foulbrood (AFB) by using an SIR model and estimated parameter values by using Markov chain Monte Carlo (MCMC) scheme based on data of AFB epidemic in the island of Jersey bee colony during the summer of 2010. They found that both distance-based and owner-based transmissions contribute to the spread of AFB. And the simulations of the stochastic SIR model, they showed that the earlier inspection, the better control of the epidemic, and the higher possibility of AFB extinction.
Jatulan et al. (2015) [101] build a compartmental ordinary differential equations system (please see the detailed model in Appendix B model (B.10)) to study American Foulbrood (AFB) infection within a honeybee colony. Their model is different from others in the sense that it includes how inside environment such as contaminated cells and outside environment infect bees. Their study suggested that AFB infection eventually leads to colony collapse; and infection thresholds were predicted based on the stability of the equilibrium states.
Nosema ceranae is an intracellular parasite of honeybees. However, its effects on colony mortality remains poorly understood. However, Nosema will cause precocious foraging and learning and memory deficits [105]. Infected bees defecate and deposit spores in the hive on the wax comb and hive bees can ingest them while cleaning comb cells. During colony confinement in the winter, bees will not defecate and can have millions of spores their digestive tract. There is also evidence that Nosema can be spread via oral-oral transmission, through trophallaxis [106]. Hive bees mouth parts get infected with Nosema while cleaning the hive and it gets transmitted to the uninfected hive bees during trophallaxis.
Betti et al. (2014) [89] presented a generic model with a working example of Nosema to study the dynamics of the spread of disease within a bee colony and the effects on colony demographics. The model for active season (spring, summer and fall) is following:
dHSdt=LS⏟new hive bees−HSR⏟recruitment−(βHHHI+βHFFI)HS⏟susceptible hive bees become infected,dFSdt=HSR⏟recruitment−mFS⏟natural death−(βHFHI+βFFFI)FS⏟susceptible foragers become infected,dHIdt=(βHHHI+βHFFI)HS⏟infected hive bees−HIR⏟recruitment−dHHI⏟death due to disease,dFIdt=HIR⏟recruitment+(βHFHI+βFFFI)FS⏟infected foragers−(m+dF)FI⏟death,dfdt=c(FS+FI)⏟collected food−γAN⏟consumed by foragers and hive bees−γLLS⏟consumed by the larvae, | (3.8) |
where HS and FS indicate the susceptible hive bees and foragers respectively, HI and FI are the infected hive bees and foragers respectively, and f is food. Parameter L is the egg-laying rate, S is the proportion of eggs that survive to adult bees, R is the proportion of recruitment from maturing hive bees to foraging duties. And all βi,j is the contact between stage i bees and stage j bees. Parameter m indicates natural death rate, c os the food collecting rate, γA and γL are the food consumption by adults and larvae, and N=FI+HI+FS+HS. The age structure part of this model is based on Khoury et al. (2011 & 2013) [21,30]. The study considered a direct transmission from bee to bee (e.g., by feeding). Adult bees will contract Nosema either from eating food contaminated by infected bees, or while removing fecal matter from the colony. The model also considers seasonality; winter is one season and the remaining (spring, summer and fall) are combined as another season, that is the model we showed above. The parameters are taken to be constant in the two seasons. And in the winter season, they proposed a corresponding model which is no newborn term (L=0) because Queen stop laying egg in the winter, no recruitment term (R=0) because all bees stay at the colony, and no new food (c=0) because no foragers go outside to collect food. The model indicated that transmission rate and death rate due to disease are the key factors that determine colony survival. In comparison, the transmission rate has a greater impact on survival than the mortality. The model also predicted that a time interval of approximately 20 days before the onset of winter is when the colony is most affected by the onset of infection. From this point of view, the transition between winter and non-winter seems to be an important aspect of seasonality and colony survival. Therefore, the transition model between the winter and non-winter is also worth learning, that Betti et al. (2016) [88] proposed 21-day transition equations we discussed before.
Petric et al. (2016) [107] specifically developed a model for Nosema ceranae (please see the detailed model in Appendix B model (B.11)) based on the assumption that infected bees deposit spores to an environmental reservoir in the hive created by defecation and hive bees ingest those spores while cleaning comb cells. The model incorporate two stressors: 1) infection of a honeybee colony with Nosema ceranae following Khoury et al. (2011) [21] and 2) increased forager loss. Seasonality was incorporated in the same way as in Ratti et al. (2015 & 2017) [45,47]. Due to lack of data at that time, they performed sensitivity analysis of the model instead of the complete parameterization. The model indicates that the colony can survive if each stressor alone is applied but the combination of infection and increased forager loss causes the colony to fail.
Muhammad and Eberl (2020) [108] extended the model of Petric et al. (2016) [107] by including direct transmission (e.g., by oral-oral route) following Betti et al. (2014) [89] (please see the detailed model in Appendix B model (B.12)). The model indicated that including direct transmission of a disease in a time-periodic model of a discrete structured population can induce what appears to be a chaotic transition from an endemic state to colony failure in a small range of the transmission rate. They also concluded that the observed chaotic transition is heavily influenced by the Holling-Type III functional form of the eclosion terms.
Not only Nosema can spread within a colony, but also between colonies, therefore there is a competitive relationship between different species of Nosema. Natsopoulou et al. (2015) [109] presented an ODE model that focused on Nosema by investigating the within-host competition between two different Nosema species (Nosema apis and Nosema ceranae) as below:
dAdt=(1−A)(1−C)AOA⏟N. Apis transmission+(1−A)ACmA⏟Mixed transmission−νA⏟Natural death,dCdt=(1−C)(1−A)COC⏟N. ceranae transmission+(1−C)CAmC⏟Mixed transmission−νC⏟Natural death, | (3.9) |
where A indicates Nosema apis and C indicates Nosema ceranae. They found that the competition is asymmetric, and the order of infection determines the outcome of the interspecific competition. It was also found that the prevalence of Nosema ceranae was higher causing the growth of Nosema apis to be inhibited.
Since Nosema has adverse affects on the food consumption and foraging ability, Comper and Eberl (2020) [110] constructed a model (B.13) (please see the detailed model in Appendix B) to study the effect of food in a colony infested with Nosema. They categorized food as harvested food i.e., honey and externally supplied food i.e., sugar syrup. They considered sugar syrup to be given to bees before winter as a way to make up for the honey that was harvested. They used the framework for division of labor from Khoury et al. (2011) [21] and indirect Nosema transmission from Petric et al. (2016) [107]. Seasonality was taken into consideration and was adapted in the same manner as in Petric et al. (2016) [107], Ratti et al. (2015) [45], and Ratti et al. (2017) [47]. Comper and Eberl (2020) [110] found the same three possible outcomes as in Petric et al. (2016) [107]. The honey yield was found to be directly proportional to the Nosema strength in the colony. One of the main parameters was found to be the supplementary sugar syrup. They noticed that if the supply of sugar syrup falls below a certain level, the colony does not survive. Honey loss cannot be made up by using supplementary sugar syrup. Honey loss due to disease can be repaired by applying a Nosema treatment if the level of infection in the colony is moderate. However, treatment does not have any effect if the infection levels are high.
Pesticides are an environmental stressor causing population reduction. Honeybees are exposed to pesticides while foraging pesticide-treated crops. Foragers bring back the pesticides to the hive which stays in the wax comb and in the pollen and nectar stores. Thus, honeybees do not get exposed only during the flowering period but much longer [111]. The effects of pesticide exposure include significant decrease in survival of foragers, reduced flight performance, compromised immunity, difficulty in protein digestion, and bee feeding [18].
Pesticides also have adverse effects on the memory, learning ability and navigational skills of the foragers. Henry et al. (2012) [98] study the foragers homing failure due to pesticides exposure. In addition to real experiments, they also combined model simulations by Khoury et al. (2011) [21] with their experimental data to predict the dynamics of colonies. They assumed the forager mortality rate includes daily natural mortality and additional death rate because of post-exposure homing failure. Henry et al. (2012) [98] found regardless of the queen's egg-laying rate, the population exposed to the treated nectar will decline during pollination, and will hardly recover afterwards. This paper is an excellent application of mathematical models and helps to give parameter values closer to reality for the mathematical model.
As mentioned in Section 3.3.1, Ratti et al. (2017) [47] following the work of Henry et al. (2012) [98] studied the effect of pesticides via loss of foragers in a colony infested with mites and ABPV. The objective was to investigate how the colony dynamics would change if a colony, that is already under stress (due to mites and ABPV), experiences increased forager loss (e.g., due to pesticides). They studied the first 3 months of a beekeeping season (i.e., the spring season) encompassing the blooming period of crops that are treated with pesticides and visited by bees. Increased forager loss was formulated in the model by using a linear death term (see model (B.9)). The study concluded that a honeybee colony that experiences periods of increased forager loss early in the season can withstand these losses and recover to (almost) normal strength, if the losses remain below a threshold. If the losses exceed this threshold the colony fails.
Magal et al. (2019) [112] developed a honeybee difference equation model that tracks the foragers leaving and entering the hive each day to study the effect of pesticides leading to homing failure. The number of uncontaminated (U) and contaminated (C) forager bees each day is given by
U(n+1)=F(U(n),C(n)),C(n+1)=G(U(n),C(n)), | (4.1) |
where F(U,C) and G(U,C) represents the daily change in uncontaminated (U) and contaminated (C) foragers respectively and are given by
F(U,C)=βV(U,C)2K2+V(U,C)2⏟daily production of newuncontaminated foragers+e−μe−αU⏟daily uncontaminated foragersthat survive and return home,G(U,C)=pe−μC⏟daily contaminated foragersthat survive and return home +pe−μ(1−e−α)U⏟daily new contaminated foragers from contamination ofuncontaminated foragers that survive and return home. | (4.2) |
The function V(U,C) indicates daily total uncontaminated and contaminated foragers returning home and is given by
V(U,C)=pe−μC⏟daily existing contaminatedforagers that surviveand return home +pe−μ(1−e−α)U⏟daily new contaminatedforagers that survive and return home+e−μe−αU⏟daily uncontaminated foragers that survive andreturn home, |
where p is the fraction of contaminated forager bees that succeeded in returning to the hive at the end the day, β is the rate of new foragers per day, μ is the natural morality rate of forager bees and α is the rate of contamination. One of the key factors of colony survive is the critical forager population viability threshold. If the population is below the threshold, the colony dies off rapidly, otherwise the population stabilizes. This dynamic of their model is incorporated as an Allee effect, that a sufficient number of young hive bees is necessary to offset the forager loss. Magal et al. (2019) [112] discussed three equilibria (one trivial and two interior) by varying the parameter p. By varying this parameter, the model captured sublethal effects of pesticides leading to colony loss due to the reduction of homing ability by foragers.
Magal et al. (2020) [113] extended their previous model in Magal et al. (2019) [112] (see model (4.2)) by including spatial components to study colony collapse due to pesticides. The model consists of differential and difference equations for the spatial distribution of uncontaminated and contaminated foraging bees. In addition to considering whether contaminated foragers can successfully return to the colony, they also considered a new condition of whether foragers will return to the previously visited foraging site. They assumed that foragers revisit the locations based on their memory and not on the waggle dance. Furthermore, they assumed that a proportion of foragers will return to the hive and start over the next day without the memory from the previous day. Included was a proportion of foragers that use their memory from the previous day. Their model focused on the density of uncontaminated (u(t,x,y)) and contaminated forager bees (c(t,x,y)) at time t and location (x,y)∈R2:
∂tu(t,x,y)=εΔu(t,x,y)⏟diffusion−μ(x,y)u(t,x,y)⏟mortality−α(x,y)u(t,x,y)⏟daily new contaminated foragers,∂tc(t,x,y)=εΔc(t,x,y)⏟diffusion−μ(x,y)c(t,x,y)⏟mortality+α(x,y)c(t,x,y)⏟daily new contaminated foragers, | (4.3) |
where μ(x,y) indicates the mortality rate of the bees, and α(x,y) indicate the rate of contamination of the forager bees by pesticides. The parameter ε represents the diffusion rate of uncontaminated foragers. They found that a high fraction of contaminated foragers that fail to return home brings the population below the thresh old and leads to colony collapse. Also, if the fraction of all foragers that return to previous foraging sites is high and spatial variation is present in the contaminated environment, foragers who visit contaminated sites multiple times have a higher probability of becoming contaminated, and colony collapse ensues.
Rumkee et al. (2015) [114] used BEEHAVE model to study the effects of pesticides on different life stages of honeybees. They explored the dynamics of bees through five stresses: increasing the mortality of larvae, hive bees and foragers, and reducing the egg laying rate. They set control mortality by multiplied and daily background mortalities by different percentages. Included was a proposed LIS50 to indicate the level of stress leading to 50% colony mortality. Small LIS50 means this pressure exerts high. Their results suggested that the mortality of adults has a greater impact on the colony than the mortality of larvae or the egg-laying rate. Different stresses also have corresponding levels of influence on the colony dynamics under the seasonality, such as forager mortality in winter and in-hive mortality in summer.
The earliest computational model, DeGrandi-Hoffman et al. (1989) BEEPOP [19], included fundamental factors for colony growth including egg laying, brood development, worker aging from house bees to foragers, and number of foraging days before the worker was removed from the colony. The BEEPOP model incorporates weather conditions (temperature, photoperiod, wind speed and rainfall) into colony population size predictions. The model tracks numbers of workers and drones in all life stages, by treating the number of eggs a queen lays each day as a cohort. The cohort is aged from egg to adult forager and then removed from the colony population after a defined number of days of foraging. The daily number of eggs the queen lays is determined by temperature, daylength and colony size. More eggs are laid in the spring and summer than fall and winter in comparably sized colonies because temperatures are warmer and daylength is longer. All other conditions being equal, more eggs are laid by queens in large colonies compared with small colonies. The number of bees in all cohorts are summed daily and reported as total numbers of eggs, larvae, pupae and adult bees.
Factors affecting the survival of bees in the various lifestages were not included in the original BEEPOP model, with the exception of forager longevity. The number of days that an adult worker could forage was initialized at the start of a simulation. If foragers had a short lifespan (e.g., 4–6 days), this would simulate stress from factors such as poor nutrition, disease, or distant nectar and pollen sources. Though the BEEPOP model is simple in its structure, it did generate realistic predictions on colony population dynamics. For example, the incorporation of weather conditions into predictions of daily egg laying rates enabled the model to capture colony population dynamics in different latitudes and climates. Sensitivity analysis revealed that the queen's egg laying potential and worker longevity exert significant effects on colony population growth, and this has been supported through field studies by others [115].
The effects of Varroa mite parasitism were included in a later version of the BEEPOP model [28]. VARROAPOP considered the growth of the Varroa population in a colony and its impact on colony dynamics due to reduction in worker longevity. The model assumes that adult workers parasitized during development have reduced longevity as adults [94,116,117]. The reduction in longevity is a function of the number of foundress mites (i.e., reproductive female mites) that enter a worker cell. Workers that are parasitized by multiple foundresses during development have shorter lifespans than those that are singly infested. During times of colony growth in the spring and summer, there often are more brood cells to parasitize than mites to infest them, and so most cells are infested by a single foundress. However in the fall, brood rearing declines. Multiple infestations of brood cells can occur because the mite population has increased throughout the spring and summer, and there are fewer brood cells in the fall to infest. The multiple infestations of brood cells in the fall and the resulting reduction in worker longevity causes the colony age structure to be skewed to short-lived adult bees. If the population of short-lived adults is sufficiently large, the model predicts that the colony will perish in the spring when flight weather resumes. The death of colonies over the winter and in the spring due to mite infestations in the fall is a common occurrence [87] that is captured in VARROAPOP simulations.
The VARROAPOP model has been useful in the development of control strategies for Varroa. The model can simulate the application of miticides to reduce the population of phoretic mites. Specifics of a miticide application such as the date when the application occurs, period of effectiveness, and the percentage of phoretic mites that will die from exposure (this value if reduced over the period of effectiveness for the miticide) can be entered into a simulation. Conducting simulations where miticides were applied at different times of year under weather conditions occurring in different geographic locations generated recommendations on the optimum time and number of miticide applications required to control Varroa populations and limit their impact on colony survival. VARROAPOP predicted that a single well-timed miticide treatment in mid-August to mid-September could adequately control Varroa and avoid mite populations that could cause a colony's demise. The model also was used to develop a commercial miticide by determining the minimum period when the active ingredient needed to be effective and the percentage of phoretic mites that had to be removed from the population.
VARROAPOP was constructed to include the migration of mites into colonies on foragers. However, the impact that mite migration could have on actual Varroa population growth in colonies was not fully realized until comparisons were made between Varroa population growth occurring from reproduction alone, and actual mite population growth especially in the fall [28,48,118]. Actual mite populations beginning in September were significantly higher than those predicted from reproduction even though mite numbers were small when the colonies were established and accurately predicted by VARROAPOP throughout the summer. The disparity between actual mite numbers and those predicted by the model occurred when the frequency of foragers with mites on their bodies collected at colony entrances increased.
Most recently, VARROAPOP was modified to track nectar and pollen collection and use by the colony based on larval and adult populations.Other modifications enable users to assign mortality rates for any life stage for a defined period. The age of first foraging for worker bees also can be changed for defined intervals during a simulation. Multiple intervals can be described for a single simulation so that the effects of increased mortality due to various stress factors can evaluated. The feature enables simulations to include effects of nutritional stress (altered brood mortality and age of first foraging of adult workers), swarming [119] and pesticide exposure (increased mortality at any lifestage and reduced forager lifespan) at [120]. Conducting simulations using Monte Carlo and sensitivity analysis techniques to determine the impact of foliar, seed or soil pesticide applications indicated that queen egg laying potential and forager lifespan determined the survival of colonies exposed to pesticides. The model also indicated that contact toxicity to foragers that resulted in reduced longevity, pesticides application method and rate, and nectar contamination (increase mortality of all lifestages) were critical parameters for colony growth and survival. The model predicted that foliar applications had the greatest effect on colony dynamics as they reduced forager lifespan and that of colony members through nectar contamination. However, simulations also revealed that the relative impact of the parameters fluctuated throughout the simulation period in relation to the status of other inputs. The time-conditional results from the simulations were expected as colony size, brood production and age structure change throughout a colony's yearly cycle.
Building on the VARROAPOP model, Becher et al. (2014) [33] constructed a model that includes effects of Varroa mites, viruses and related impaired foraging behavior, changes in landscape structure, and pesticide exposure on colony growth and survival (the BEEHAVE model). Colony structure and brood production components of BEEHAVE are similar to BEEPOP and VARROAPOP in that they are generated from difference equations that create cohorts of individuals that are aged from egg to adult. Varroa populations are initialized as phoretic mites (i.e., mites on adult bees) and reproduce in brood cells. Mites can infect developing pupae with DWV and ABPV, or be virus-free and not infect their pupal host. The foraging model is an agent-based system simulating foraging on actual and hypothetical landscapes. Cohorts of workers that reach the age of first foraging join the foraging population. The bees' foraging decisions are dependent on the energetic efficiency of a food source (for nectar collection) or by the total trip duration (for pollen collection). Energy gains and losses of each foraging trip are calculated. Forager mortality occurs after predetermined distances or maximum age is achieved.
BEEHAVE was used to simulate the effects that combinations of stress factors such as Varroa, low forage availability, and increased forager mortality, as a proxy for pesticide exposure, might have on colony survival at different times of year. Using data from large-scale colony feeding studies for model calibration and validation, Abi-Akar et al. (2020) [121] identified factors that are critical to overwintering success for a range of landscape compositions, weather conditions, and supplemental feeding schedules. The model predicted that factors affecting over wintering survival were weather conditions, and the effects that supplemental feeding in the fall have on colony size and food stores.
The BEEHAVE model also was used to simulate the sublethal effects of pesticides, and determine when those effects would manifest in colony dynamics, and if they could be mitigated [123], when those effects would manifest in colony dynamics, and if the effects could be mitigated. The sublethal effects included impaired forager orientation (simulated by doubling the distance to forage during period of exposure), impaired floral handling time (doubling handling time during a foraging trip), effects on brood care (halving the brood to in-hive bee ratio) and increased forager mortality. The greatest colony-level impact occurred due to sublethal effects of brood care. However, timing of the event was critical and most pronounced in the spring when colonies were building. Handling time effects were most pronounced in late summer and had long-lasting colony level impacts. The effects could be related to the importance of collecting sufficient pollen and nectar to meet seasonal requirements. In the spring, pollen collection drives brood production and colony size. In late summer, nectar collection is needed to insure sufficient stores for overwintering. Simulations indicated that increasing the availability of non-contaminated forage could improve colony recovery following pesticide exposure by increasing brood production (spring) or nectar stores (late summer). BEEHAVE also was used to evaluate the effects of the neonicotinoid, clothianidin, on colony growth and survival. Simulations with mortality due to pollen contamination indicated adverse effects only at high levels of exposure not seen in field measurements. No colony effects were predicted when clothianidin concentrations similar to field measurements were used.
Furthering investigations into the relationship between forage availability and colony growth, Becher et al. (2016) [34] developed a software tool to examine how scout bees explore the floral landscape. BEESCOUT uses image files imported into the program to serve as a "forage map". The location of floral (i.e., food) patches relative to the colony are calculated and used to generate probabilities of scouts finding the food patches. Different scouting strategies can be used for food detection, and probabilities of scouts finding food sources can compared. Detection probabilities of forage patches are used as input for the BEEHAVE model, to explore realistic scenarios of colony growth and death in response to different stressors. The nectar and pollen resources than can be collected are incorporated into the BEEHAVE model by linking information on patch size, location food availability, and detection probability (BEESCOUT) with daily amounts of nectar and pollen provided by the patch, sugar concentration, and handling times to collect full nectar and pollen loads as initialized in BEEHAVE. The total amount on nectar and pollen provided on each day during the flowering period is then calculated based on the patch size. Predictions from the simulations where the food source was close to the colonies fit empirical data with reasonable accuracy. However, no empirical data were available for food sources further away. Still, predictions from the model show that landscape structure and connectivity of food sources can have a strong impact on colony growth. The model can be a valuable tool for designing landscape configurations that incorporate searching behavior of bees, detection probabilities, food availability, and foraging decisions that increase patch visitation rates and enhance colony development and survival.
The BEEHAVE model was designed to simulate honey bee colony dynamics, but Becher et al. (2018) [124] modified the model to simulate the growth, behavior and survival of six bumblebee species native to the UK. The model uses an agent-based approach to generate predictions based in a mapped landscape where nectar and pollen resources can be approximated based on the model of Becher et al. (2016) [34] and can include the effects of multiple stressors on colony growth and survival. The model captures colony dynamics using individual behaviors that are driven by spatio-temporal resource availability in various landscape compositions. The model incorporates energy budgets and depletion of resources in mapped landscapes so that interspecific and intraspecific competition can emerge. Simulations on the effects of pesticide exposure indicate the impact on colony survival depends on the population size at the time of exposure.
Computational models have been developed to simulate the interactions between Varroa and the viruses they transmit, and predict the effects on colony survival. The first Varroa-virus model was developed by Martin (1998) [26] and Martin (2001) [38] and simulated the dynamics of a colony infested with Varroa that transmitted either DWV or ABPV to the parasitized bees. The model indicated that DWV would remain at low levels through much of the spring and summer with little effect on colony growth. However, DWV infections increased in the fall as there were more mites carrying the virus. This led to high levels of DWV-infected bees in the winter cluster and eventual colony death due to the reduced lifespan of infected bees and the effects on the colony age structure. In contrast, ABPV causes rapid death of infected bees, The model predicted that ABPV was difficult to establish in a colony especially at low mite populations. Colony death occurred only when Varroa populations were large with high levels of mites carrying the virus. The model accurately predicted that DWV would become more widely established in bee populations than ABPV and be the predominant cause of death in Varroa infested colonies.
Wilkinson and Smith (2002) [27] presented a difference equation model (with a time step of one day) for adult worker bees, worker bee brood, drone bee brood, phoretic mites, and non-phoretic mites. Unlike Martin (1998) [26], a simple but detailed sensitivity analysis was performed in which input variables were ranked in terms of relative importance. They found that viable reproduction in worker cells, percentage of drone brood, mite invasion of drone brood and mite reproduction in drone brood are the most important factors determining Varroa population growth rate.
Ratti et al. (2016) [46] studied the effect of swarming on a colony infested with mites and ABPV using discrete-continuous framework based on Ratti et al. (2015) [45]. They studied swarming due to overcrowding and at fixed time intervals. This study was done mainly computationally. The model predicted that in a mite-virus infested colony, the proportion of mites leaving the parent colony with the swarm may determine the fate of the colony. A colony, that otherwise dies off due to virus, can survive if the percentage of mites leaving the parent colony is above a critical value.
In addition to applying mathematical and computational models for simulation, machine learning also is being used to predict colony survival. Calovi et al. (2021) [125] used random forest (RF) matching learning method to evaluate the importance of weather, topography, land use, and management factors on the apiary and colony-level overwintering mortality. Predictions on overwintering survival from the model were more accurate at the colony than the apiary level. In colonies where Varroa was controlled, temperature (expressed in growing degree days) and precipitation the previous year were the most important predictors of colony survival over the winter. Topographic factors and landscape quality were not important predictors of overwintering survival. Beekeepers that provided data for the model may have fed their colonies protein and carbohydrate supplements during periods when flowering plants were unavailable making it difficult to quantify landscape effects. Since the important variables for predicting overwintering success were weather-related, a simpler predictive RF model was created without landscape or topographic variables. Predictions from the second model were as accurate as the full model. Because the model requires only weather conditions to generate predictions, it could be used to predict overwintering survival under projected climate change scenarios, and serve as a basis for developing decision support tools to effectively manage colonies in a changing climate.
Addressing the global crisis of honeybee losses will require an integrated approach to evaluate the combination of factors that culminate in the collapse of a colony population. Mathematical models can be powerful tools to provide insights on how various stresses contribute to colony losses. These theoretical tools are needed to identify mechanisms that can be difficult or costly to quantify in field and will be an essential component in developing strategies to improve colony health.
The most common cause of colony losses is Varroa mites and the viruses they transmit [7,87]. An effective method of control is the selection of Varroa tolerant bee stock. Modelling interactions between honeybees and Varroa could point to key combinations of traits that could enhance the ability of selected lines to reduce the growth of Varroa populations and viral transmission among bees. Increases in Varroa populations and virus levels are connected, and it might be assumed that the virus, especially DWV, is the cause of colony loss [94,126] due to effects on adult longevity and the age structure of the colony. Modeling the connection between virus transmission rates and longevity of infected workers could provide targets for the selection of bee stocks with traits that reduce viral transmission or replication. Models that incorporate spatial scales can provide landscape level information on the spread of mite and viruses among colonies in an apiary. This information could be valuable in generating apiary-level recommendations for the arrangement of hives and application of miticide treatments.
Climate change is an important factor contributing to the ongoing decline of pollinators [130]. Models that describe the effects of environmental factors on plant growth and flowering characteristics (i.e., phenology, nutrients in pollen and nectar) under current and future weather scenarios could be instrumental in predicting where crops and pollinator plantings could be established, and the traits they would require to survive. Climate change also could affect the yearly cycle of honeybee colonies especially if fall and winter temperatures are conducive for flight. Models that incorporate future weather conditions generated by climate projection models could determine the effects of late season foraging activity on the age structure of colonies, and predict the impact on overwinter survival with and without additional stress factors such as pathogens or poor nutrition.
Pesticides (fungicides and insecticides) are widely considered to be a key factor in the reduction of insect pollinators. The effects of pesticides vary depending upon modes of action. Responses to pesticides that are neurotoxins differ from those that are metabolic inhibitors or that disrupt development. As more information becomes available on sublethal effects of pesticides, the impact on colony survival during and after exposure could be predicted by incorporating the information into colony population dynamics models. Analyses to determine periods when colonies are most vulnerable to loss from sublethal effects also could be defined so that beekeepers could avoid areas where pesticides are applied during those times. Models that include effects of pathogens or poor nutrition that reduce brood rearing and adult bee longevity also could capture the interactions with pesticide exposure that culminate in colony loss. Recommendations for rescuing colonies exposed to pesticides by improving nutrition [134] or controlling pests such as Varroa might also be generated within the framework of colony dynamics.
Colony losses are economic liabilities to beekeepers that jeopardize the solvency of their businesses. Economic models are needed to support decision making in implementing management protocols, estimating returns on investment at a colony level, setting prices for colony rental for pollination and assessing the cost of colony losses at different times of year. An economic model of a commercial beekeeping operation has been reported [5], but others are needed that include different management strategies and revenue streams that could increase the profitability of beekeeping operations. Economic models also could be a tool to continually update the value of honey bee pollination in crop production and the agricultural economy as acreages of bee pollinated crops increase.
One goal of our paper is to review what we have done in mathematical modeling of honeybees and suggest future studies that could occur. We found that with few exceptions [22,29,50,56,129], the validation of theoretical models with field data is lacking. Model validation with experimental data is needed to provide confidence in model predictions. The complexities of factors that determine the survivorship of colonies will require the construction of models, but they must be based on field data that include determinants of colony health (e.g., colony size, mite loads, presence of disease). Collaborations between mathematicians and biologist are needed to construct models that put field data into a broader context and make use of their predictive power. These collaborations could be instrumental in reducing colony losses because the models would provide a deeper understanding of the shifting vulnerabilities of colonies throughout their seasonal cycle, and serve as a basis for management tools to preserve colony health.
This research is partially supported by NSF-DMS (Award Number 1716802 & 2052820); NSF- IOS/DMS (Award Number 1558127) and The James S. McDonnell Foundation 21st Century Science Initiative in Studying Complex Systems Scholar Award (UHC Scholar Award 220020472).
The authors declare there is no conflict of interest.
Division of labor | Transmission Mode | Seasonality/Weather factor | Other | ||
Dennis and Kemp (2016) | |||||
Chen et al. (2020) | ✘ | ✘ | |||
Khoury et al. (2011) | ✘ | ||||
Booton et al. (2017) | ✘ | Stress | |||
Khoury et al. (2013) | ✘ | Food | |||
Perry et al. (2015) | ✘ | Food | |||
Schmickl and Karsai (2016) | ✘ | ✘ | Common Stomach | ||
Schmickl and Karsai (2017) | ✘ | ✘ | Common Stomach | ||
Messan et al. (2018) | ✘ | ✘ | Nutrition | ||
Laomettachit et al. (2021) | ✘ | Infection within a colony | social immunity | ||
Sweeney et al. (2019) | ✘ | Varroa | |||
Messan et al. (2021) | ✘ | Varroa | ✘ | ||
Kang et al. (2016) | ✘ | Varroa & Virus | |||
Messan et al. (2017) | Varroa | Migration | |||
Kribs and Mitchell (2014) | ✘ | Virus (Direct and Indirect) | |||
Betti et al. (2016) | ✘ | Virus (Direct) | ✘ | Food | |
D | #233;nes and A. Ibrahim (2019) | ✘ | Varroa & Virus | ||
Sumpter and Martin (2004) | ABPV & Varroa | ||||
Eberl et al. (2010) | ABPV & Varroa | ✘ | |||
Ratti et al. (2012) | ABPV & Varroa | ||||
Ratti et al. (2015) | ABPV & Varroa | ✘ | |||
Ratti et al. (2017) | ✘ | ABPV & Varroa | ✘ | Pesticide & Varroacide | |
Britton et al. (2021) | DWV | ✘ | |||
Datta et al. (2013) | AFB | ✘ | |||
Jatulan et al. (2015) | ✘ | AFB | ✘ | cell comb | |
Betti et al. (2014) | ✘ | Nosema Direct and Indirect | ✘ | ||
Petric et al. (2016) | ✘ | Nosema Indirect | ✘ | ||
Muhammad and Eberl (2020) | ✘ | Nosema Indirect and Direct | ✘ | ||
Natsopoulou et al. (2015) | ✘ | Nosema ceranae and Nosema apis | ✘ | Competition | |
Comper and Eberl (2020) | ✘ | Nosema Indirect | ✘ | Food | |
Magal et al. (2019) | ✘ | Pesticide | |||
Magal et al. (2020) | ✘ | Pesticide & Space |
Messan et al. (2018): [50]
dBdt=cqαqVeg⏟egg production−βbhB⏟maturation from brood to nurses,dNdt=βbhB⏟maturation from brood to nurses−N∗βhf((VN)−1)⏟maturation to foragers−dhN⏟mortality,dFdt=N∗βhf((VN)−1)⏟maturation from nurses−dfF⏟mortality,dPdt=F∗SB1+aB+bP⏟regulated pollen collection−αNP⏟removing rate of pollen by nurse bee for VG production−dpP⏟deterioration,dVdt=cαNP⏟VG production by nurse bee with available pollen−αbBV⏟brood consumption−(1−cq)αqV⏟Queen metabolic costs−αhNV⏟nurses metabolic costs −V∗βhf((VN)−1)⏟maturation to forager−dhV⏟removal rate due to nurses death, | (B.1) |
where B indicates brood, N indicates nurses, F indicates foragers, P indicates pollen storage and V indicates the rate of change of VG in nurse bees.
Laomettachit et al. (2021): [71] Core model
dBsdt=l0−BsnB−pt0⋅kNB⋅Ni⋅Bs,dBidt=−BinB+pt0⋅kNB⋅Ni⋅Bs−krem⋅Bi⋅Ns−kd⋅Bi,dNsdt=BsnB−NsnN−pt1⋅kRN⋅Ri1⋅Ns−pt,rem⋅krem⋅Bi⋅Ns,dNidt=BinB−NinN+pt1⋅kRN⋅Ri1⋅Ns−krem⋅Ni⋅Ns+pt,rem⋅krem⋅Bi⋅Ns−kd⋅Ni, | (B.2) |
where Bs represents the number of healthy brood, Bi represents the number of infected brood, Ns represents the number of healthy nurses and Ni represents the number of infected nurses.
Extended model
dRs0dt=NsnN−Rs0nR−kFR⋅(Fs1+Fi1)⋅Rs0+Rs1ts,dRs1dt=−Rs1nR+kFR⋅(Fs1+(1−pt2)⋅Fi1)⋅Rs0−Rs1ts,dRi0dt=NinN−Ri0nR−kFR⋅(Fs1+Fi1)⋅Ri0+Ri1ts,dRi1dt=−Ri1nR+kFR⋅(Fs1+Fi1)⋅Ri0+pt2⋅kFR⋅Fi⋅Rs0−Ri1ts,dFs0dt=Rs0nR−Fs0nF+kFR⋅(Rs0+Ri0)⋅Fs1−kr⋅Fs0,dFs1dt=Rs1nR−Fs1nF−kFR⋅(Rs0+Ri0)⋅Fs1+kr⋅Fs0,dFi0dt=Ri0nR−Fi0nF+kFR⋅(Rs0+Ri0)⋅Fi1−kr⋅Fi0,dFi1dt=Ri1nR−Fi1nF−kFR⋅(Rs0+Ri0)⋅Fi1+psurv⋅kr⋅Fi0, | (B.3) |
where Rs, Ri, Fs and Fi represent the number of nectar-receivers, infected nectar-receivers, healthy foragers, and infected foragers, respectively. Nectar-receivers and foragers can also change their nectar-loaded state between unloaded (subscript 0) and loaded (subscript 1).
Sweeney et al. (2019): [49]
dBldt=rH2K+H2⏟egg production−dlBl⏟natural death−e−dlτlrH(t−τl)2K+H(t−τl)2⏟maturation from larvae to pupae,dBpdt=e−dlτlrH(t−τl)2K+H(t−τl)2⏟maturation from larvae to pupae−αbprobability of Mpattaching to Bp⏞Bpa+BpMp⏟parasitism on brood−dpBp⏟natural death−e−∫tt−τp[dp+αpMp(s)a+Bp(s)]dsrH(t−τp−τl)2K+H(t−τp−τl)2⏟maturation to adult,dHdt=e−∫tt−τp[dp+αpMp(s)a+Bp(s)]dsrH(t−τp−τl)2K+H(t−τp−τl)2⏟maturation to adult−αhprobability of Mhattaching to H⏞Ha+HMh⏟parasitism on adult bee−dhH⏟natural death,dMhdt=cαpBp(t−τp)a+Bp(t−τp)Mp(t−τp)⏟new mites from brood parasitism−dmhMh⏟natural death−βMh⏟transition to reproductive mites Mp,dMpdt=βMh⏟transition from phoretic mites−dmpMp⏟natural death. | (B.4) |
Messan et al. (2017): [48]
dH1dt=r1H21K1+H21⏟successful reproduction at colony 1−dh1H1⏟natural death−α1H1M1⏟parasitism effects,dM1dt=c1α1H1M1⏟newborns−dm1M1⏟natural death+ρ21H2a2+H2M2−ρ12H1a1+H1M1⏟Net dispersal effects at colony 1,dH2dt=r2H22K2+H22⏟successful reproduction at colony 2−dh2H2⏟natural death−α2H2M2⏟parasitism effects,dM2dt=c2α2H2M2⏟newborns−dm2M2⏟natural death+ρ12H1a1+H1M1−ρ21H2a2+H2M2⏟Net dispersal effects at colony 2, | (B.5) |
where ρij represents the migration rate of mites from Patch j to Patch i.
Kang et al. (2016): [40]
dShdt=r(Sh+ρIh)2K+(Sh+ρIh)2⏟newborns−dhSh⏟natural death−βhShIhSh+Ih⏟honeybee infected, by nestmates−βmhShImSh+Ih⏟adult honeybees infected by the phoretic mites −βmhShIm⏟brood infected by the reproductive mites−αSh(Sm+Im)⏟parasitism by mites,dIhdt=Sh(βhIhSh+Ih+βmhImSh+Ih+βmhIm)−αIh(Sm+Im)⏟consumed by mites−(dh+μh)Ih⏟death,dSmdt=Sm(cαSh−βhmIhSh+Ih⏟the phoretic mites infected by adult honeybees−dm),dImdt=cα(Ih(Sm+Im)+ShIm)⏟mites born with virus infections+βhmIhSmSh+Ih−(dm+μm)Im. | (B.6) |
Dénes and A. Ibrahim (2019): [93]
dSdt=A−β1SHm−β2SHi+αHm+αHi−dS,dHmdt=β1SHm−β2HmHi−αHm−dHm,dHidt=β2SHi+β2HmHi−αHi−dHi−δHi, | (B.7) |
where Hs indicates susceptible hive bees, Fs indicates susceptible forager bees, Hm indicates hive bees infested by non-infectious vectors and Hi indicates hive bees infested by infectious vectors.
Ratti et al. (2012): [44]
dMIdt=β1(MS−MI)HIHS+HI⏟uninfected mites to infected mites−β2MIHSHS+HI⏟infected mites lose virus to uninfected host,dHSdt=μHnSKn+HnSe−MIk⏟new healthy bees−β3MIHSHS+HI⏟infected bees−d1HS⏟death−γ1MSHS⏟death because of mites,dHIdt=β3MIHSHS+HI⏟new infected bees−d2HI⏟death−γ2MSHI⏟death due to mites,dMdt=rM(1−Mα(HS+HI))⏟logistic growth for total mites, | (B.8) |
where MI indicates the number of mites that carry the virus, HS and HI indicate the number of honeybees that are virus free and the number of honeybees that are infected with the virus, respectively.
Ratti et al. (2017): [47]
dHdt=μ(H+F)iKi+(H+F)ih(MI)⏟new hive bees−β1MIHH+HI+F⏟infected hives−(d1+δ1)H⏟death−γ1(MI+MS)H⏟death due to mites−H(σ1−σ2(FH+F))⏟recruitment,dFdt=H(σ1−σ2(FH+F))⏟recruitment−β1MIFH+HI+F⏟infected foragers−(p+d2+δ2)F⏟death−γ2(MI+MS)F⏟death due to mites,dHIdt=β1MIH+FH+HI+F⏟infected bees−(d3+δ3)HI⏟death−γ3(MI+MS)HI⏟death due to mites,dMIdt=rMI(1−MI+MSα(H+HI+F))⏟logistic growth of mites+β2MSHIH+HI+F⏟virus-free mites tovirus-carrying mites−β3MIH+FH+HI+F⏟virus-carrying mites lose virus to uninfected host−δ4MI⏟death due to virus,dMSdt=rMS(1−MI+MSα(H+HI+F))⏟logistic growth of mites−β2MSHIH+HI+F⏟virus-free mites to virus-carrying mites+β3MIH+FH+HI+F⏟virus-carrying mites losevirus to uninfected host−δ5MS⏟death dueto virus. | (B.9) |
Jatulan et al. (2015) : [101]
dBsdt=M−α1PBs−CvM−β1Bs,dBodt=β1Bs−β2Bo,dBidt=α1PBs+CvM−ϕ1ϕ2Bi,dCdt=ϕ1ϕ2Bi[σ(v−C)],dAsdt=β2(1−α1P)Bo−α2CvAs−α3PAs−α4As−μAs,dAidt=β2α1PBo+α2CvAs+α3PAs+α4As−μAi, | (B.10) |
where
P=Ai1+As+AiandM=LAs+Aiω+As+Ai. |
The parameter Bs indicates the number of healthy brood in the hive before n hours old, Bo indicates healthy brood in the hive older than n hours old, Bi indicates infected brood in the hive, C indicates infected cell combs, As indicates adult bees without AFB spores and Ai indicates adult bees with AFB spores.
Petric et al. (2016): [107]
dHdt=βZnk(t)n+Zn⏟bee eclosion −σ1H+σ2F+FIZF⏟recruitment−η0H⏟Natural death−αHEλ+E⏟acquisition of disease,dHIdt=−σ1HI+σ2F+FIZFI⏟recruitment−η1HI⏟Natural death+αHEλ+E⏟acquisition of disease,dFdt=σ1H−σ2F+FIZF⏟recruitment−ϕ0F⏟Natural death,dFIdt=σ1HI−σ2F+FIZFI⏟recruitment−ϕ1FI⏟Natural death,dEdt=γHI⏟spore deposition−δE⏟spores lose viability−˜αHEλ+E⏟spore uptake, | (B.11) |
where H,HI,F, and FI represents the number of uninfected hive bees, infected hive bees, uninfected foragers, and infected foragers respectively.
Muhammad and Eberl (2020): [108]
dHdt=βZnkn+Zn⏟bee eclosion −σ1H⏟recruitment+σ2F+FIZF⏟social inhibition−η0H⏟Natural death−αHEλ+E⏟acquisition of disease−μ(HI+FI)H⏟direct transmission,dHIdt=−σ1HI⏟recruitment+σ2F+FIZFI⏟social inhibition−η1HI⏟Natural death+αHEλ+E⏟acquisition of disease+μ(HI+FI)H⏟direct transmission,dFdt=σ1H⏟recruitment−σ2F+FIZF⏟social inhibition−ϕ0F⏟Natural death−μ(HI+FI)F⏟direct transmission,dFIdt=σ1HI⏟recruitment−σ2F+FIZFI⏟social inhibition−ϕ1FI⏟Natural death+μ(HI+FI)F⏟direct transmission,dEdt=γHI⏟spore deposition−δE⏟spores lose viability−˜αHEλ+E⏟spore uptake. | (B.12) |
Comper and Eberl (2020): [110]
dHdt=βZnkn+Zn⏟bee eclosion −σ1H⏟recruitment+σ2F+FIZF⏟social inhibition−η0H⏟Natural death−(˜η0a2a2+(f+sZ)2)H⏟starvation induced death−αHEλ+E⏟acquisition of disease−μ(HI+FI)H⏟direct transmission,dHIdt=−σ1HI⏟recruitment+σ2F+FIZFI⏟social inhibition−η1HI⏟Natural death−(˜η1b2b2+(f+sZ)2)HI⏟starvation induced death+αHEλ+E⏟acquisition of disease+μ(HI+FI)H⏟direct transmission,dFdt=σ1H⏟recruitment−σ2F+FIZF⏟social inhibition−ϕ0F⏟Natural death−(˜ϕ0a2a2+(f+sZ)2)F⏟starvation induced death−μ(HI+FI)F⏟direct transmission,dFIdt=σ1HI⏟recruitment−σ2F+FIZFI⏟social inhibition−ϕ1FI⏟Natural death−(˜ϕ1b2b2+(f+sZ)2)FI⏟starvation induced death+μ(HI+FI)F⏟direct transmission,dEdt=γHI⏟spore deposition−δE⏟spores lose viability−˜αHEλ+E⏟spore uptake,dfdt=c0F+c1FI⏟Food collection−(θ0fh+f+νs)(H+F)−(θ1fh+f+νs)(HI+FI)⏟Food consumption (workers)−θbβZ2k2+Z2⏟Food consumption (brood)−−ln(f(th)f(t0))th−t0⏟Harvest,dsdt=μ⏟Sugar addition−(θ0sh+s)(H+F)−(θ1sh+s)(HI+FI)⏟Sugar consumption. | (B.13) |
[1] |
K. M. Smith, E. H. Loh, M. K. Rostal, C. M. Zambrana-Torrelio, L. Mendiola, P. Daszak, Pathogens, pests, and economics: drivers of honey bee colony declines and losses, EcoHealth, 10 (2013), 434–445. doi: 10.1007/s10393-013-0870-2
![]() |
[2] | R. Johnson, Honey Bee Colony Collapse Disorder, CRS Report for Congressional Research Service Washington, Washington, D. C., 2010. |
[3] |
S. S. Chopra, B. R. Bakshi, V. Khanna, Economic dependence of us industrial sectors on animal-mediated pollination service, Environ. Sci. Technol., 49 (2015), 14 441–14 451. doi: 10.1021/es505828n
![]() |
[4] |
D. vanEngelsdorp, M. D. Meixner, A historical review of managed honey bee populations in europe and the united states and the factors that may affect them, J. Invertebr. Pathol., 103 (2010), S80–S95. doi: 10.1016/j.jip.2009.06.011
![]() |
[5] |
G. DeGrandi-Hoffman, H. Graham, F. Ahumada, M. Smart, N. Ziolkowski, The economics of honey bee (hymenoptera: Apidae) management and overwintering strategies for colonies used to pollinate almonds, J. Econ. Entomol., 112 (2019), 2524–2533. doi: 10.1093/jee/toz213
![]() |
[6] |
E. Genersch, American foulbrood in honeybees and its causative agent, paenibacillus larvae, J. Invertebr. Pathol., 103 (2010), S10–S19. doi: 10.1016/j.jip.2009.06.015
![]() |
[7] |
E. Guzmán-Novoa, L. Eccles, Y. Calvete, J. Mcgowan, P. G. Kelly, A. Correa-Benítez, Varroa destructor is the main culprit for the death and reduced populations of overwintered honey bee (apis mellifera) colonies in Ontario, Canada, Apidologie, 41 (2010), 443–450. doi: 10.1051/apido/2009076
![]() |
[8] |
C. Van Dooremalen, L. Gerritsen, B. Cornelissen, J. J. van der Steen, F. van Langevelde, T. Blacquiere, Winter survival of individual honey bees and honey bee colonies depends on level of varroa destructor infestation, PloS One, 7 (2012), e36285. doi: 10.1371/journal.pone.0036285
![]() |
[9] |
A. C. Highfield, A. El Nagar, L. C. Mackinder, L. M. L. Noël, M. J. Hall, S. J. Martin, et al., Deformed wing virus implicated in overwintering honeybee colony losses, Appl. Environ. Microbiol., 75 (2009), 7212–7220. doi: 10.1128/AEM.02227-09
![]() |
[10] |
M. A. Doeke, M. Frazier, C. M. Grozinger, Overwintering honey bees: biology and management, Curr. Opin. Insect Sci., 10 (2015), 185–193. doi: 10.1016/j.cois.2015.05.014
![]() |
[11] |
B. A. Williams, Unique physiology of host-parasite interactions in microsporidia infections, Cell. Microbiol., 11 (2009), 1551–1560. doi: 10.1111/j.1462-5822.2009.01362.x
![]() |
[12] |
R. Martín-Hernández, C. Botías, L. Barrios, A. Martínez-Salvador, A. Meana, C. Mayack, et al., Comparison of the energetic stress associated with experimental nosema ceranae and nosema apis infection of honeybees (apis mellifera), Parasitol. Res., 109 (2011), 605–612. doi: 10.1007/s00436-011-2292-9
![]() |
[13] |
C. Mayack, D. Naug, Energetic stress in the honeybee apis mellifera from nosema ceranae infection, J. Invertebr. Pathol., 100 (2009), 185–188. doi: 10.1016/j.jip.2008.12.001
![]() |
[14] |
L. Paris, H. El Alaoui, F. Delbac, M. Diogon, Effects of the gut parasite nosema ceranae on honey bee physiology and behavior, Curr. Opin. Insect Sci., 26 (2018), 149–154. doi: 10.1016/j.cois.2018.02.017
![]() |
[15] | F. Sánchez-Bayo, D. Goulson, F. Pennacchio, F. Nazzi, K. Goka, N. Desneux, Are bee diseases linked to pesticides? A brief review, Environ. Int., 89 (2016), 7–11. |
[16] | E. D. Pilling, P. C. Jepson, Synergism between ebi fungicides and a pyrethroid insecticide in the honeybee (apis mellifera), Pestic. Sci., 9 (1993), 293–297. |
[17] |
J. S. Pettis, E. M. Lichtenberg, M. Andree, J. Stitzinger, R. Rose, D. vanEngelsdorp, Crop pollination exposes honey bees to pesticides which alters their susceptibility to the gut pathogen nosema ceranae, PloS One, 8 (2013), e70182. doi: 10.1371/journal.pone.0070182
![]() |
[18] |
A. Fisher II, G. DeGrandi-Hoffman, B. H. Smith, M. Johnson, O. Kaftanoglu, T. Cogley, et al., Colony field test reveals dramatically higher toxicity of a widely-used mito-toxic fungicide on honey bees (apis mellifera), Environ. Pollut., 269 (2021), 115964. doi: 10.1016/j.envpol.2020.115964
![]() |
[19] |
G. DeGrandi-Hoffman, S. A. Roth, G. Loper, E. Erickson Jr, Beepop: a honeybee population dynamics simulation model, Ecol. Modell., 45 (1989), 133–150. doi: 10.1016/0304-3800(89)90088-4
![]() |
[20] |
M. A. Becher, J. L. Osborne, P. Thorbek, P. J. Kennedy, V. Grimm, Towards a systems approach for understanding honeybee decline: a stocktaking and synthesis of existing models, J. Appl. Ecol., 50 (2013), 868–880. doi: 10.1111/1365-2664.12112
![]() |
[21] |
D. S. Khoury, M. R. Myerscough, A. B. Barron, A quantitative model of honey bee colony population dynamics, PloS One, 6 (2011), e18491. doi: 10.1371/journal.pone.0018491
![]() |
[22] | J. Chen, K. Messan, M. R. Messan, G. DeGrandi-Hoffman, D. Bai, Y. Kang, How to model honeybee population dynamics: stage structure and seasonality, Math. Appl. Sci. Eng., 1 (2020), 91–125. |
[23] |
B. Dennis, W. P. Kemp, How hives collapse: allee effects, ecological resilience, and the honey bee, PloS One, 11 (2016), e0150055. doi: 10.1371/journal.pone.0150055
![]() |
[24] | K. Vetharaniam, N. D. Barlow, Modelling biocontrol of Varroa destructor using a benign haplotype as a competitive antagonist, N. Z. J. Ecol., 30 (2006), 87–102. |
[25] |
S. J. Martin, Ontogenesis of the mite varroa jacobsoni oud. in worker brood of the honeybee Apis mellifera L. under natural conditions, Exp. Appl. Acarol., 18 (1994), 87–100. doi: 10.1007/BF00055033
![]() |
[26] |
S. Martin, A population model for the ectoparasitic mite varroa jacobsoni in honey bee (Apis mellifera) colonies, Ecol. Modell., 109 (1998), 267–281. doi: 10.1016/S0304-3800(98)00059-3
![]() |
[27] |
D. Wilkinson, G. C. Smith, A model of the mite parasite, varroa destructor, on honeybees (Apis mellifera) to investigate parameters important to mite population growth, Ecol. Modell., 148 (2002), 263–275. doi: 10.1016/S0304-3800(01)00440-9
![]() |
[28] |
G. DeGrandi-Hoffman, R. Curry, A mathematical model of varroa mite (varroa destructor anderson and trueman) and honeybee (Apis mellifera L.) population dynamics, Int. J. Acarol., 30 (2004), 259–274. doi: 10.1080/01647950408684393
![]() |
[29] |
K. Messan, M. Rodriguez Messan, J. Chen, G. DeGrandi-Hoffman, Y. Kang, Population dynamics of varroa mite and honeybee: effects of parasitism with age structure and seasonality, Ecol. Modell., 440 (2021), 109359. doi: 10.1016/j.ecolmodel.2020.109359
![]() |
[30] |
D. S. Khoury, A. B. Barron, M. R. Myerscough, Modelling food and population dynamics in honey bee colonies, PloS One, 8 (2013), e59084. doi: 10.1371/journal.pone.0059084
![]() |
[31] |
C. M. Kribs-Zaleta, C. Mitchell, Modeling colony collapse disorder in honeybees as a contagion. Math. Biosci. Eng., 11 (2014), 1275–1294. doi: 10.3934/mbe.2014.11.1275
![]() |
[32] |
C. J. Perry, E. Søvik, M. R. Myerscough, A. B. Barron, Rapid behavioral maturation accelerates failure of stressed honey bee colonies, Proc. Natl. Acad. Sci., 112 (2015), 3427–3432. doi: 10.1073/pnas.1422089112
![]() |
[33] |
M. A. Becher, V. Grimm, P. Thorbek, J. Horn, P. J. Kennedy, J. L. Osborne, Beehave: a systems model of honeybee colony dynamics and foraging to explore multifactorial causes of colony failure, J. Appl. Ecol., 51 (2014), 470–482. doi: 10.1111/1365-2664.12222
![]() |
[34] |
M. Becher, V. Grimm, J. Knapp, J. Horn, G. Twiston-Davies, J. Osborne, Beescout: A model of bee scouting behaviour and a software tool for characterizing nectar/pollen landscapes for beehave, Ecol. Modell., 340 (2016), 126–133. doi: 10.1016/j.ecolmodel.2016.09.013
![]() |
[35] |
S. Allan, K. Slessor, M. Winston, G. King, The influence of age and task specialization on the production and perception of honey bee pheromones, J. Insect Physiol., 33, (1987), 917–922. doi: 10.1016/0022-1910(87)90003-5
![]() |
[36] |
R. E. Page, C. Y. Peng, Aging and development in social insects with emphasis on the honey bee, Apis mellifera L. Exp. Gerontol., 36 (2001), 695–711. doi: 10.1016/S0531-5565(00)00236-9
![]() |
[37] | M. L. Winston, The Biology of the Honey Bee, Harvard University Press, 1991. |
[38] |
S. J. Martin, The role of varroa and viral pathogens in the collapse of honeybee colonies: a modelling approach, J. Appl. Ecol., 38 (2001), 1082–1093. doi: 10.1046/j.1365-2664.2001.00662.x
![]() |
[39] |
J. D. Evans, M. Spivak, Socialized medicine: individual and communal disease barriers in honey bees, J. Invertebr. Pathol., 103 (2010), S62–S72. doi: 10.1016/j.jip.2009.06.019
![]() |
[40] |
Y. Kang, K. Blanco, T. Davis, Y. Wang, G. DeGrandi-Hoffman, Disease dynamics of honeybees with varroa destructor as parasite and virus vector, Math. Biosci., 275 (2016), 71–92. doi: 10.1016/j.mbs.2016.02.012
![]() |
[41] |
Y. Kang, R. Clark, M. Makiyama, J. Fewell, Mathematical modeling on obligate mutualism: Interactions between leaf-cutter ants and their fungus garden, J. Theor. Biol., 289 (2011), 116–127. doi: 10.1016/j.jtbi.2011.08.027
![]() |
[42] |
L. A. Real, The kinetics of functional response, Am. Nat., 111 (1977), 289–300. doi: 10.1086/283161
![]() |
[43] | H. J. Eberl, M. R. Frederick, P. G. Kevan, Importance of brood maintenance terms in simple models of the honeybee-varroa destructor-acute bee paralysis virus complex, Electron. J. Differ. Equations, 19 (2010), 85–98. |
[44] | V. Ratti, P. G. Kevan, H. J. Eberl, A mathematical model for population dynamics in honeybee colonies infested with varroa destructor and the acute bee paralysis virus, Can. Appl. Math. Q., 21 (2012), 63–93. |
[45] |
V. Ratti, P. G. Kevan, H. J. Eberl, A mathematical model of the honeybee-varroa destructor-acute bee paralysis virus system with seasonal effects, Bull. Math. Biol., 77 (2015), 1493–1520. doi: 10.1007/s11538-015-0093-5
![]() |
[46] | V. Ratti, P. G. Kevan, H. J. Eberl, A discrete-continuous modeling framework to study the role of swarming in a honeybee-varroa destrutor-virus system, in Mathematical and Computational Approaches in Advancing Modern Science and Engineering, Springer, (2016), 299–308. |
[47] |
V. Ratti, P. G. Kevan, H. J. Eberl, A mathematical model of forager loss in honeybee colonies infested with varroa destructor and the acute bee paralysis virus, Bull. Math. Biol., 79 (2017), 1218–1253. doi: 10.1007/s11538-017-0281-6
![]() |
[48] |
K. Messan, G. DeGrandi-Hoffman, C. Castillo-Chavez, Y. Kang, Migration effects on population dynamics of the honeybee-mite interactions, Math. Modell. Nat. Phenom., 12 (2017), 84–115. doi: 10.1051/mmnp/201712206
![]() |
[49] | B. F. Sweeney, Mathematical modeling of honeybee population dynamics, 2019. |
[50] |
M. R. Messan, R. E. Page Jr, Y. Kang, Effects of vitellogenin in age polyethism and population dynamics of honeybees, Ecol. Modell., 388 (2018), 88–107. doi: 10.1016/j.ecolmodel.2018.09.011
![]() |
[51] |
K. Tomioka, A. Matsumoto, The circadian system in insects: cellular, molecular, and functional organization, Adv. Insect Physiol., 56 (2019), 73–115. doi: 10.1016/bs.aiip.2019.01.001
![]() |
[52] |
R. D. Booton, Y. Iwasa, J. A. Marshall, D. Z. Childs, Stress-mediated allee effects can cause the sudden collapse of honey bee colonies, J. Theor. Biol., 420 (2017), 213–219. doi: 10.1016/j.jtbi.2017.03.009
![]() |
[53] |
T. D. Seeley, Adaptive significance of the age polyethism schedule in honeybee colonies, Behav. Ecol. Sociobiol., 11 (1982), 287–293. doi: 10.1007/BF00299306
![]() |
[54] |
I. Karsai, T. Schmickl, Regulation of task partitioning by a "common stomach" : a model of nest construction in social wasps, Behav. Ecol., 22 (2011), 819–830. doi: 10.1093/beheco/arr060
![]() |
[55] |
T. Schmickl, I. Karsai, How regulation based on a common stomach leads to economic optimization of honeybee foraging, J. Theor. Biol., 389 (2016), 274–286. doi: 10.1016/j.jtbi.2015.10.036
![]() |
[56] |
T. Schmickl, K. Istvan, Resilience of honeybee colonies via common stomach: a model of self-regulation of foraging, PloS One, 12 (2017), e0188004. doi: 10.1371/journal.pone.0188004
![]() |
[57] |
R. Brodschneider, K. Crailsheim, Nutrition and health in honey bees, Apidologie, 41 (2010), 278–294. doi: 10.1051/apido/2010012
![]() |
[58] |
A. L. Hughes, Life-history evolution at the molecular level: adaptive amino acid composition of avian vitellogenins, Proc. R. Soc. B., 282 (2015), 20151105. doi: 10.1098/rspb.2015.1105
![]() |
[59] |
G. V. Amdam, O. Rueppell, M. K. Fondrk, R. E. Page, C. M. Nelson, The nurse's load: early-life exposure to brood-rearing affects behavior and lifespan in honey bees (Apis mellifera), Exp. Gerontol, 44 (2009), 467–471. doi: 10.1016/j.exger.2009.02.013
![]() |
[60] |
G. V. Amdam, S. W. Omholt, The regulatory anatomy of honeybee lifespan, J. Theor. Biol., 216 (2002), 209–228. doi: 10.1006/jtbi.2002.2545
![]() |
[61] |
U. Glavinic, B. Stankovic, V. Draskovic, J. Stevanovic, T. Petrovic, N. Lakic, et al., Dietary amino acid and vitamin complex protects honey bee from immunosuppression caused by nosema ceranae, PLoS One, 12 (2017), e0187726. doi: 10.1371/journal.pone.0187726
![]() |
[62] |
T. Schmickl, K. Crailsheim, Hopomo: A model of honeybee intracolonial population dynamics and resource management, Ecol. Modell., 204 (2007), 219–245. doi: 10.1016/j.ecolmodel.2007.01.001
![]() |
[63] |
G. V. Amdam, S. W. Omholt, The hive bee to forager transition in honeybee colonies: the double repressor hypothesis, J. Theor. Biol., 223 (2003), 451–464. doi: 10.1016/S0022-5193(03)00121-8
![]() |
[64] |
K. R. Guidugli, A. M. Nascimento, G. V. Amdam, A. R. Barchuk, S. Omholt, Z. L. Simões, et al., Vitellogenin regulates hormonal dynamics in the worker caste of a eusocial insect, FEBS Lett., 579 (2005), 4961–4965. doi: 10.1016/j.febslet.2005.07.085
![]() |
[65] | G. Amdam, K. Ihle, R. Page, Regulation of honeybee worker (Apis mellifera) life histories by vitellogenin, in Hormones, Brain and Behavior, (2009), 1003–1027. |
[66] |
B. R. Johnson, Limited flexibility in the temporal caste system of the honey bee, Behav. Ecol. Sociobiol., 58 (2005), 219–226. doi: 10.1007/s00265-005-0949-z
![]() |
[67] |
J. Oettler, A. L. Nachtigal, L. Schrader, Expression of the foraging gene is associated with age polyethism, not task preference, in the ant cardiocondyla obscurior, PLoS One, 10 (2015), e0144699. doi: 10.1371/journal.pone.0144699
![]() |
[68] |
M. Goblirsch, Z. Y. Huang, M. Spivak, Physiological and behavioral changes in honey bees (Apis mellifera) induced by nosema ceranae infection, PLoS One, 8 (2013), e58165. doi: 10.1371/journal.pone.0058165
![]() |
[69] |
L. R. BenVau, J. C. Nieh, Larval honey bees infected with nosema ceranae have increased vitellogenin titers as young adults, Sci. Rep., 7 (2017), 1–8. doi: 10.1038/s41598-016-0028-x
![]() |
[70] |
S. Cremer, S. A. Armitage, P. Schmid-Hempel, Social immunity, Curr. Biol., 17 (2007), R693–R702. doi: 10.1016/j.cub.2007.06.008
![]() |
[71] |
T. Laomettachit, M. Liangruksa, T. Termsaithong, A. Tangthanawatsakul, O. Duangphakdee, A model of infection in honeybee colonies with social immunity, PloS One, 16 (2021), e0247294. doi: 10.1371/journal.pone.0247294
![]() |
[72] |
D. R. Tarpy, T. D. Seeley, Lower disease infections in honeybee (Apis mellifera) colonies headed by polyandrous vs monandrous queens, Naturwiss., 93 (2006), 195–199. doi: 10.1007/s00114-006-0091-4
![]() |
[73] |
D. Sammataro, U. Gerson, G. Needham, Parasitic mites of honey bees: life history, implications, and impact, Annu. Rev. Entomol., 45 (2000), 519–548. doi: 10.1146/annurev.ento.45.1.519
![]() |
[74] |
M. Shen, L. Cui, N. Ostiguy, D. L. Cox-Foster, Intricate transmission routes and interactions between picorna-like viruses (Kashmir bee virus and sacbrood virus) with the honeybee host and the parasitic varroa mite, J. Gen. Virol., 86 (2005), 2281–2289. doi: 10.1099/vir.0.80824-0
![]() |
[75] |
S. J. Martin, The role of Varroa and viral pathogens in the collapse of honeybee colonies: a modelling approach, J. Appl. Ecol., 38 (2001), 1082–1093. doi: 10.1046/j.1365-2664.2001.00662.x
![]() |
[76] |
D. vanEngelsdorp, M. D. Meixner, A historical review of managed honey bee populations in europe and the united states and the factors that may affect them, J. Invertebr. Pathol., 103 (2010), S80–S95. doi: 10.1016/j.jip.2009.06.011
![]() |
[77] |
I. Fries, Nosema apis—a parasite in the honey bee colony, Bee World, 74 (1993), 5–19. doi: 10.1080/0005772X.1993.11099149
![]() |
[78] |
M. Higes, R. Martín, A. Meana, Nosema ceranae, a new microsporidian parasite in honeybees in europe, J. Invertebr. Pathol., 92 (2006), 93–95. doi: 10.1016/j.jip.2006.02.005
![]() |
[79] |
Y. S. Peng, Y. Fang, S. Xu, L. Ge, The resistance mechanism of the Asian honey bee, Apis cerana Fabr., to an ectoparasitic mite, Varroa jacobsoni Oudemans, J. Invertebr. Pathol., 49 (1987), 54–60. doi: 10.1016/0022-2011(87)90125-X
![]() |
[80] |
S. D. Ramsey, R. Ochoa, G. Bauchan, C. Gulbronson, J. D. Mowery, A. Cohen, et al., Varroa destructor feeds primarily on honey bee fat body tissue and not hemolymph, Proc. Natl. Acad. Sci., 116 (2019), 1792–1801. doi: 10.1073/pnas.1818371116
![]() |
[81] | G. Tantillo, M. Bottaro, A. Di Pinto, V. Martella, P. Di Pinto, V. Terio, Virus infections of honeybees Apis mellifera, Ital. J. Food Saf., 4 (2015), 5364. |
[82] |
G. DeGrandi-Hoffman, Y. Chen, Nutrition, immunity and viral infections in honey bees, Curr. Opin. Insect Sci., 10 (2015), 170–176. doi: 10.1016/j.cois.2015.05.007
![]() |
[83] |
J. Carrillo-Tripp, B. C. Bonning, W. A. Miller, Challenges associated with research on rna viruses of insects, Curr. Opin. Insect Sci., 8 (2015), 62–68. doi: 10.1016/j.cois.2014.11.002
![]() |
[84] |
J. R. De Miranda, E. Genersch, Deformed wing virus, J. Invertebr. Pathol., 103 (2010), S48–S61. doi: 10.1016/j.jip.2009.06.012
![]() |
[85] |
G. DeGrandi-Hoffman, V. Corby-Harris, Y. Chen, H. Graham, M. Chambers, E. W. DeJong, et al., Can supplementary pollen feeding reduce varroa mite and virus levels and improve honey bee colony survival? Exp. Appl. Acarol., 82 (2020), 455–473. doi: 10.1007/s10493-020-00562-7
![]() |
[86] |
F. Mondet, J. R. de Miranda, A. Kretzschmar, Y. Le Conte, A. R. Mercer, On the front line: quantitative virus dynamics in honeybee (Apis mellifera L.) colonies along a new expansion front of the parasite varroa destructor, PLoS Pathog., 10 (2014), e1004323. doi: 10.1371/journal.ppat.1004323
![]() |
[87] |
E. Genersch, W. Von Der Ohe, H. Kaatz, A. Schroeder, C. Otten, R. Büchler, et al., The german bee monitoring project: a long term study to understand periodically high winter losses of honey bee colonies, Apidologie, 41 (2010), 332–352. doi: 10.1051/apido/2010014
![]() |
[88] |
M. Betti, L. Wahl, M. Zamir, Age structure is critical to the population dynamics and survival of honeybee colonies, R. Soc. Open Sci., 3 (2016), 160444. doi: 10.1098/rsos.160444
![]() |
[89] |
M. I. Betti, L. M. Wahl, M. Zamir, Effects of infection on honey bee population dynamics: a model, PloS One, 9 (2014), e110237. doi: 10.1371/journal.pone.0110237
![]() |
[90] | Y. P. Chen, R. Siede, Honey bee viruses, Adv. Virus Res., 70 (2007), 33–80. |
[91] | P. A. Moore, M. E. Wilson, J. A. Skinner, Honey bee viruses, the deadly varroa mite associates, Bee Health, 19 (2015), 2015. |
[92] |
D. J. Sumpter, S. J. Martin, The dynamics of virus epidemics in varroa-infested honey bee colonies, J. Anim. Ecol., 73 (2004), 51–63. doi: 10.1111/j.1365-2656.2004.00776.x
![]() |
[93] |
A. Dénes, M. A. Ibrahim, Global dynamics of a mathematical model for a honeybee colony infested by virus-carrying varroa mites, J. Appl. Math. Comput., 61 (2019), 349–371. doi: 10.1007/s12190-019-01250-5
![]() |
[94] |
B. Dainat, J. D. Evans, Y. P. Chen, L. Gauthier, P. Neumann, Dead or alive: deformed wing virus and varroa destructor reduce the life span of winter honeybees, Appl. Environ. Microbiol., 78 (2012), 981–987. doi: 10.1128/AEM.06537-11
![]() |
[95] |
H. Hansen, C. J. Brødsgaard, American foulbrood: a review of its biology, diagnosis and control, Bee World, 80 (1999), 5–23. doi: 10.1080/0005772X.1999.11099415
![]() |
[96] |
S. J. Martin, A. C. Highfield, L. Brettell, E. M. Villalobos, G. E. Budge, M. Powell, et al., Global honey bee viral landscape altered by a parasitic mite, Science, 336 (2012), 1304–1306. doi: 10.1126/science.1220941
![]() |
[97] | J. Devillers, In Silico Bees, CRC Press, 2014. |
[98] |
M. Henry, M. Beguin, F. Requier, O. Rollin, J. F. Odoux, P. Aupinel, et al., A common pesticide decreases foraging success and survival in honey bees, Science, 336 (2012), 348–350. doi: 10.1126/science.1215039
![]() |
[99] | N. F. Britton, K. J. White, The effect of covert and overt infections on disease dynamics in honey-bee colonies, Bull. Math. Biol., 83 (2021), 1–23. |
[100] |
S. Datta, J. C. Bull, G. E. Budge, M. J. Keeling, Modelling the spread of american foulbrood in honeybees, J. R. Soc. Interface, 10 (2013), 20130650. doi: 10.1098/rsif.2013.0650
![]() |
[101] | E. O. Jatulan, J. F. Rabajante, C. G. B. Banaay, A. C. Fajardo Jr, E. C. Jose, A mathematical model of intra-colony spread of american foulbrood in european honeybees (Apis mellifera L.), PLoS One, 10 (2017), e0143805. |
[102] |
Y. Chen, J. D. Evans, I. B. Smith, J. S. Pettis, Nosema ceranae is a long-present and wide-spread microsporidian infection of the european honey bee (Apis mellifera) in the united states, J. Invertebr. Pathol., 97 (2008), 186–188. doi: 10.1016/j.jip.2007.07.010
![]() |
[103] |
L. Bailey, Honey bee pathology, Annu. Rev. Entomol., 13 (1968), 191–212. doi: 10.1146/annurev.en.13.010168.001203
![]() |
[104] |
M. Goblirsch, Nosema ceranae disease of the honey bee (Apis mellifera), Apidologie, 49 (2018), 131–150. doi: 10.1007/s13592-017-0535-1
![]() |
[105] | S. L. Gage, C. Kramer, S. Calle, M. Carroll, M. Heien, G. DeGrandi-Hoffman, Nosema ceranae parasitism impacts olfactory learning and memory and neurochemistry in honey bees (apis mellifera), J. Exp. Biol., 221 (2018). |
[106] |
W. F. Huang, L. F. Solter, Comparative development and tissue tropism of nosema apis and nosema ceranae, J. Invertebr. Pathol., 113 (2013), 35–41. doi: 10.1016/j.jip.2013.01.001
![]() |
[107] | A. Petric, E. Guzman-Novoa, H. J. Eberl, A mathematical model for the interplay of nosema infection and forager losses in honey bee colonies, J. Biol. Dyn., (2016), 1–31. |
[108] |
N. Muhammad, H. J. Eberl, Two routes of transmission for nosema infections in a honeybee population model with polyethism and time-periodic parameters can lead to drastically different qualitative model behavior, Commun. Nonlinear Sci. Numer. Simul., 84 (2020), 105207. doi: 10.1016/j.cnsns.2020.105207
![]() |
[109] |
M. E. Natsopoulou, D. P. McMahon, V. Doublet, J. Bryden, R. J. Paxton, Interspecific competition in honeybee intracellular gut parasites is asymmetric and favours the spread of an emerging infectious disease, Proc. R. Soc. B, 282 (2015), 20141896. doi: 10.1098/rspb.2014.1896
![]() |
[110] |
J. R. Comper, H. J. Eberl, Mathematical modelling of population and food storage dynamics in a honey bee colony infected with nosema ceranae, Heliyon, 6 (2020), e04599. doi: 10.1016/j.heliyon.2020.e04599
![]() |
[111] |
C. A. Mullin, M. Frazier, J. L. Frazier, S. Ashcraft, R. Simonds, J. S. Pettis, et al., High levels of miticides and agrochemicals in north american apiaries: implications for honey bee health, PloS One, 5 (2010), e9754. doi: 10.1371/journal.pone.0009754
![]() |
[112] |
P. Magal, G. Webb, Y. Wu, An environmental model of honey bee colony collapse due to pesticide contamination, Bull. Math. Biol., 81 (2019), 4908–4931. doi: 10.1007/s11538-019-00662-5
![]() |
[113] |
P. Magal, G. F. Webb, Y. Wu, A spatial model of honey bee colony collapse due to pesticide contamination of foraging bees, J. Math. Biol., 80 (2020), 2363–2393. doi: 10.1007/s00285-020-01498-7
![]() |
[114] |
J. C. Rumkee, M. A. Becher, P. Thorbek, P. J. Kennedy, J. L. Osborne, Predicting honeybee colony failure: using the beehave model to simulate colony responses to pesticides, Environ. Sci. Technol., 49 (2015), 12 879–12 887. doi: 10.1021/acs.est.5b03593
![]() |
[115] |
O. Rueppell, R. Linford, P. Gardner, J. Coleman, K. Fine, Aging and demographic plasticity in response to experimental age structures in honeybees (Apis mellifera L.), Behav. Ecol. Sociobiol., 62 (2008), 1621–1631. doi: 10.1007/s00265-008-0591-7
![]() |
[116] |
D. De Jong, P. H. De Jong, Longevity of africanized honey bees (hymenoptera: Apidae) infested by varroa jacobsoni (parasitiformes: Varroidae), J. Econ. Entomol., 76 (1983), 766–768. doi: 10.1093/jee/76.4.766
![]() |
[117] |
H. Kovac, K. Crailsheim, Lifespan of apis mellifera carnica pollm. infested by varroa jacobsoni oud. in relation to season and extent of infestation, J. Apic. Res., 27 (1988), 230–238. doi: 10.1080/00218839.1988.11100808
![]() |
[118] |
G. DeGrandi-Hoffman, F. Ahumada, V. Zazueta, M. Chambers, G. Hidalgo, E. W. Dejong, Population growth of varroa destructor (acari: Varroidae) in honey bee colonies is affected by the number of foragers with mites, Exp. Appl. Acarol., 69 (2016), 21–34. doi: 10.1007/s10493-016-0022-9
![]() |
[119] |
G. DeGrandi-Hoffman, F. Ahumada, H. Graham, Are dispersal mechanisms changing the host-parasite relationship and increasing the virulence of varroa destructor (mesostigmata: Varroidae) in managed honey bee (hymenoptera: Apidae) colonies? Environ. Entomol., 46 (2017), 737–746. doi: 10.1093/ee/nvx077
![]() |
[120] |
A. C. Kuan, G. DeGrandi-Hoffman, R. J. Curry, K. V. Garber, A. R. Kanarek, M. N. Snyder, et al., Sensitivity analyses for simulating pesticide impacts on honey bee colonies, Ecol. Modell, 376 (2018), 15–27. doi: 10.1016/j.ecolmodel.2018.02.008
![]() |
[121] |
F. Abi-Akar, A. Schmolke, C. Roy, N. Galic, S. Hinarejos, Simulating honey bee large-scale colony feeding studies using the beehave model? Part ii: analysis of overwintering outcomes, Environ. Toxicol. Chem., 39 (2020), 2286–2297. doi: 10.1002/etc.4844
![]() |
[122] |
M. Switanek, K. Crailsheim, H. Truhetz, R. Brodschneider, Modelling seasonal effects of temperature and precipitation on honey bee winter mortality in a temperate climate, Sci. Total Environ., 579 (2017), 1581–1587. doi: 10.1016/j.scitotenv.2016.11.178
![]() |
[123] |
P. Thorbek, P. J. Campbell, P. J. Sweeney, H. M. Thompson, Using beehave to explore pesticide protection goals for european honeybee (Apis melifera L.) worker losses at different forage qualities, Environ. Toxicol. Chem., 36 (2017), 254–264. doi: 10.1002/etc.3504
![]() |
[124] |
M. A. Becher, G. Twiston-Davies, T. D. Penny, D. Goulson, E. L. Rotheray, J. L. Osborne, Bumble-beehave: a systems model for exploring multifactorial causes of bumblebee decline at individual, colony, population and community level, J. Appl. Ecol., 55 (2018), 2790–2801. doi: 10.1111/1365-2664.13165
![]() |
[125] |
M. Calovi, C. M. Grozinger, D. A. Miller, S. C. Goslee, Summer weather conditions influence winter survival of honey bees (Apis mellifera) in the northeastern united states, Sci. Rep., 11 (2021), 1–12. doi: 10.1038/s41598-020-79139-8
![]() |
[126] |
B. Dainat, P. Neumann, Clinical signs of deformed wing virus infection are predictive markers for honey bee colony losses, J. Invertebr. Pathol., 112 (2013), 278–280. doi: 10.1016/j.jip.2012.12.009
![]() |
[127] |
J. D. Evans, C. Saegerman, C. Mullin, E. Haubruge, B. K. Nguyen, M. Frazier, et al., Colony collapse disorder: a descriptive study, PloS One, 4 (2009), e6481. doi: 10.1371/journal.pone.0006481
![]() |
[128] |
G. DeGrandi-Hoffman, F. Ahumada, R. Curry, G. Probasco, L. Schantz, Population growth of varroa destructor (acari: Varroidae) in commercial honey bee colonies treated with beta plant acids, Exp. Appl. Acarol., 64 (2014), 171–186. doi: 10.1007/s10493-014-9821-z
![]() |
[129] | J. L. Harris, A population model and its application to the study of honey bee colonies, IRE Trans. Circuit Theory, 2 (1980), 44–49. |
[130] |
C. A. Halsch, A. M. Shapiro, J. A. Fordyce, C. C. Nice, J. H. Thorne, D. P. Waetjen, et al., Insects and recent climate change, Proc. Natl. Acad. Sci., 118 (2021), e2002543117. doi: 10.1073/pnas.2002543117
![]() |
[131] |
F. Bodenheimer, Studies in animal populations. ii. seasonal population-trends of the honey-bee, Q. Rev. Biol., 12 (1937), 406–425. doi: 10.1086/394540
![]() |
[132] | B. Castellanos-Potenciano, F. Gallardo-López, G. Diaz-Padilla, A. Pérez-Vázquez, C. Landeros-Sánchez, et al., Spatio-temporal mobility of apiculture affected by the climate change in the beekeeping of the gulf of Mexico, Appl. Ecol. Environ. Res., 15 (2017), 163–175. |
[133] | Y. Le Conte, M. Navajas, Climate change: impact on honey bee populations and diseases, Rev. Sci. Tech., 27 (2008), 499–510. |
[134] |
M. K. Crone, C. M. Grozinger, Pollen protein and lipid content influence resilience to insecticides in honey bees (apis mellifera), J. Exp. Biol., 224 (2021), jeb242040. doi: 10.1242/jeb.242040
![]() |
[135] |
A. C. Velásquez, C. D. M. Castroverde, S. Y. He, Plant-pathogen warfare under changing climate conditions, Curr. Biol., 28 (2018), R619–R634. doi: 10.1016/j.cub.2018.03.054
![]() |
[136] |
P. D. Noyes, M. K. McElwee, H. D. Miller, B. W. Clark, L. A. Van Tiem, K. C. Walcott, et al., The toxicology of climate change: environmental contaminants in a warming world, Environ. Int., 35 (2009), 971–986, 2009. doi: 10.1016/j.envint.2009.02.006
![]() |
1. | Kristina Garber, Gloria DeGrandi-Hoffman, Robert Curry, Jeffrey M. Minucci, Daniel E. Dawson, Cameron Douglass, Joseph P. Milone, S. Thomas Purucker, Simulating the Effects of Pesticides on Honey Bee (Apis mellifera L.) Colonies with BeePop+, 2022, 3, 2673-4133, 275, 10.3390/ecologies3030022 | |
2. | Alec C. Nichols, Sean Phillips, Alexander A. Soderlund, 2023, Resilience-based Optimization of Investigative Satellite Swarm via an Artificial Honeybee Colony Algorithm, 978-1-62410-699-6, 10.2514/6.2023-2358 | |
3. | Atanas Z. Atanasov, Miglena N. Koleva, Lubin Vulkov, 2023, Chapter 19, 978-3-031-27033-8, 279, 10.1007/978-3-031-27034-5_19 | |
4. | Silvia Pătruică, Roxana Nicoleta Lazăr, Genoveva Buzamăt, Marius Boldea, Economic Benefits of Using Essential Oils in Food Stimulation Administrated to Bee Colonies, 2023, 13, 2077-0472, 594, 10.3390/agriculture13030594 | |
5. | Atanas Z. Atanasov, Miglena N. Koleva, Lubin G. Vulkov, 2023, Chapter 24, 978-3-031-21483-7, 269, 10.1007/978-3-031-21484-4_24 | |
6. | Slavi Georgiev, Lubin Vulkov, Parameters Identification and Numerical Simulation for a Fractional Model of Honeybee Population Dynamics, 2023, 7, 2504-3110, 311, 10.3390/fractalfract7040311 | |
7. | Jürgen Groeneveld, Tomas Martinovic, Tuomas Rossi, Ondrej Salamon, Kata Sara-aho, Volker Grimm, Prototype Biodiversity Digital Twin: honey bees in agricultural landscapes, 2024, 10, 2367-7163, 10.3897/rio.10.e125167 | |
8. | K. Bhagya laxmi, K. Shiva Reddy, Dynamical behavior of obligatory mutualistic-cheater interactions under the influence of white noise, 2024, 11, 26668181, 100860, 10.1016/j.padiff.2024.100860 | |
9. | Atanas Z. Atanasov, Miglena N. Koleva, Lubin G. Vulkov, Inverse Problem Numerical Analysis of Forager Bee Losses in Spatial Environment without Contamination, 2023, 15, 2073-8994, 2099, 10.3390/sym15122099 | |
10. | Slavi Georgiev, Lubin Vulkov, 2024, Chapter 35, 978-3-031-53211-5, 395, 10.1007/978-3-031-53212-2_35 | |
11. | David C. Elzinga, W. Christopher Strickland, Generalized Stressors on Hive and Forager Bee Colonies, 2023, 85, 0092-8240, 10.1007/s11538-023-01219-3 | |
12. | Germán Zapata‐Hernández, Martina Gajardo‐Rojas, Matías Calderón‐Seguel, Ariel A. Muñoz, Karen P. Yáñez, Fabrice Requier, Francisco E. Fontúrbel, Pablo I. Ormeño‐Arriagada, Héctor Arrieta, Advances and knowledge gaps on climate change impacts on honey bees and beekeeping: A systematic review, 2024, 30, 1354-1013, 10.1111/gcb.17219 | |
13. | Abdelhak Rouabah, Chantal Rabolin‐Meinrad, Camille Gay, Olivier Therond, Models of bee responses to land use and land cover changes in agricultural landscapes – a review and research agenda, 2024, 99, 1464-7931, 2003, 10.1111/brv.13109 | |
14. | Gloria DeGrandi-Hoffman, Vanessa Corby-Harris, Henry Graham, Mona Chambers, Emily Watkins deJong, Lucy Snyder, David Tarpy, Do diet and Fumagillin treatment impact Vairimorpha (Nosema) spp. (Microspora: Nosematidae) infections in honey bees (Hymenoptera: Apidae) and improve survival and growth of colonies overwintered in cold storage?, 2024, 0022-0493, 10.1093/jee/toae187 | |
15. | John Purdy, 2024, 9780323917933, 1, 10.1016/B978-0-323-91793-3.00005-5 | |
16. | Miglena N. Koleva, Lubin G. Vulkov, Reconstruction coefficient analysis of honeybee collapse due to pesticide contamination, 2023, 2675, 1742-6588, 012024, 10.1088/1742-6596/2675/1/012024 | |
17. | Atanas Z. Atanasov, Slavi G. Georgiev, Lubin G. Vulkov, Analysis of the Influence of Brood Deaths on Honeybee Population, 2024, 14, 2076-3417, 11412, 10.3390/app142311412 | |
18. | Piotr Grabowski, Comment on “An approach to the modeling of honey bee colonies” by Romero-Leiton et al. (2022), 2025, 25, 1399-1183, 1, 10.5194/we-25-1-2025 | |
19. | Manhong Ye, Yinhong Jiang, Qiannan Han, Xiaoyuan Li, Chuang Meng, Chao Ji, Feng Ji, Bin Zhou, Probiotic Potential of Enterococcus lactis GL3 Strain Isolated from Honeybee (Apis mellifera L.) Larvae: Insights into Its Antimicrobial Activity Against Paenibacillus larvae, 2025, 12, 2306-7381, 165, 10.3390/vetsci12020165 | |
20. | Igor Kurdin, Aleksandra Kurdina, Internet of Things Smart Beehive Network: Homogeneous Data, Modeling, and Forecasting the Honey Robbing Phenomenon, 2025, 10, 2411-5134, 23, 10.3390/inventions10020023 | |
21. | Tao Feng, Hao Wang, Complex dynamics in plant-pollinator-parasite interactions: facultative versus obligate behaviors and novel bifurcations, 2025, 90, 0303-6812, 10.1007/s00285-025-02210-3 | |
22. | Atanas Atanasov, Slavi Georgiev, Lubin Vulkov, 2025, Chapter 2, 978-3-031-76785-2, 15, 10.1007/978-3-031-76786-9_2 | |
23. | Jun Chen, Adrian Fisher, Jon F. Harrison, Gloria DeGrandi-Hoffman, Jennifer H. Fewell, Yun Kang, Modeling pesticide impacts on honey bee dynamics from mathematical and experimental integration, 2025, 611, 00225193, 112167, 10.1016/j.jtbi.2025.112167 |
Division of labor | Transmission Mode | Seasonality/Weather factor | Other | ||
Dennis and Kemp (2016) | |||||
Chen et al. (2020) | ✘ | ✘ | |||
Khoury et al. (2011) | ✘ | ||||
Booton et al. (2017) | ✘ | Stress | |||
Khoury et al. (2013) | ✘ | Food | |||
Perry et al. (2015) | ✘ | Food | |||
Schmickl and Karsai (2016) | ✘ | ✘ | Common Stomach | ||
Schmickl and Karsai (2017) | ✘ | ✘ | Common Stomach | ||
Messan et al. (2018) | ✘ | ✘ | Nutrition | ||
Laomettachit et al. (2021) | ✘ | Infection within a colony | social immunity | ||
Sweeney et al. (2019) | ✘ | Varroa | |||
Messan et al. (2021) | ✘ | Varroa | ✘ | ||
Kang et al. (2016) | ✘ | Varroa & Virus | |||
Messan et al. (2017) | Varroa | Migration | |||
Kribs and Mitchell (2014) | ✘ | Virus (Direct and Indirect) | |||
Betti et al. (2016) | ✘ | Virus (Direct) | ✘ | Food | |
D | #233;nes and A. Ibrahim (2019) | ✘ | Varroa & Virus | ||
Sumpter and Martin (2004) | ABPV & Varroa | ||||
Eberl et al. (2010) | ABPV & Varroa | ✘ | |||
Ratti et al. (2012) | ABPV & Varroa | ||||
Ratti et al. (2015) | ABPV & Varroa | ✘ | |||
Ratti et al. (2017) | ✘ | ABPV & Varroa | ✘ | Pesticide & Varroacide | |
Britton et al. (2021) | DWV | ✘ | |||
Datta et al. (2013) | AFB | ✘ | |||
Jatulan et al. (2015) | ✘ | AFB | ✘ | cell comb | |
Betti et al. (2014) | ✘ | Nosema Direct and Indirect | ✘ | ||
Petric et al. (2016) | ✘ | Nosema Indirect | ✘ | ||
Muhammad and Eberl (2020) | ✘ | Nosema Indirect and Direct | ✘ | ||
Natsopoulou et al. (2015) | ✘ | Nosema ceranae and Nosema apis | ✘ | Competition | |
Comper and Eberl (2020) | ✘ | Nosema Indirect | ✘ | Food | |
Magal et al. (2019) | ✘ | Pesticide | |||
Magal et al. (2020) | ✘ | Pesticide & Space |
Division of labor | Transmission Mode | Seasonality/Weather factor | Other | ||
Dennis and Kemp (2016) | |||||
Chen et al. (2020) | ✘ | ✘ | |||
Khoury et al. (2011) | ✘ | ||||
Booton et al. (2017) | ✘ | Stress | |||
Khoury et al. (2013) | ✘ | Food | |||
Perry et al. (2015) | ✘ | Food | |||
Schmickl and Karsai (2016) | ✘ | ✘ | Common Stomach | ||
Schmickl and Karsai (2017) | ✘ | ✘ | Common Stomach | ||
Messan et al. (2018) | ✘ | ✘ | Nutrition | ||
Laomettachit et al. (2021) | ✘ | Infection within a colony | social immunity | ||
Sweeney et al. (2019) | ✘ | Varroa | |||
Messan et al. (2021) | ✘ | Varroa | ✘ | ||
Kang et al. (2016) | ✘ | Varroa & Virus | |||
Messan et al. (2017) | Varroa | Migration | |||
Kribs and Mitchell (2014) | ✘ | Virus (Direct and Indirect) | |||
Betti et al. (2016) | ✘ | Virus (Direct) | ✘ | Food | |
D | #233;nes and A. Ibrahim (2019) | ✘ | Varroa & Virus | ||
Sumpter and Martin (2004) | ABPV & Varroa | ||||
Eberl et al. (2010) | ABPV & Varroa | ✘ | |||
Ratti et al. (2012) | ABPV & Varroa | ||||
Ratti et al. (2015) | ABPV & Varroa | ✘ | |||
Ratti et al. (2017) | ✘ | ABPV & Varroa | ✘ | Pesticide & Varroacide | |
Britton et al. (2021) | DWV | ✘ | |||
Datta et al. (2013) | AFB | ✘ | |||
Jatulan et al. (2015) | ✘ | AFB | ✘ | cell comb | |
Betti et al. (2014) | ✘ | Nosema Direct and Indirect | ✘ | ||
Petric et al. (2016) | ✘ | Nosema Indirect | ✘ | ||
Muhammad and Eberl (2020) | ✘ | Nosema Indirect and Direct | ✘ | ||
Natsopoulou et al. (2015) | ✘ | Nosema ceranae and Nosema apis | ✘ | Competition | |
Comper and Eberl (2020) | ✘ | Nosema Indirect | ✘ | Food | |
Magal et al. (2019) | ✘ | Pesticide | |||
Magal et al. (2020) | ✘ | Pesticide & Space |