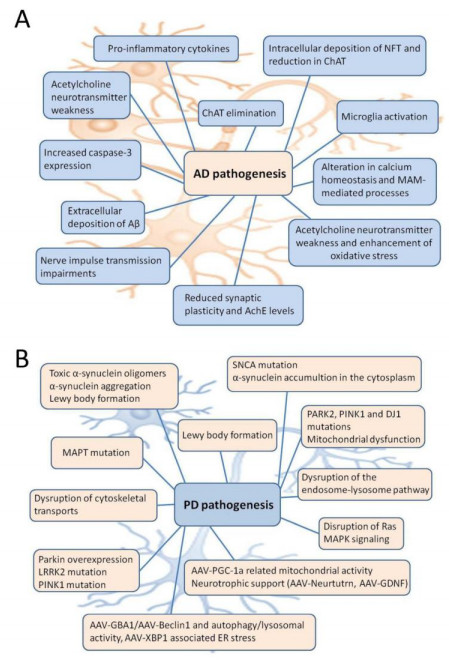
The complexity of biological systems suggests that current definitions of molecular dysfunctions are essential distinctions of a complex phenotype. This is well seen in neurodegenerative diseases (ND), such as Alzheimer's disease (AD) and Parkinson's disease (PD), multi-factorial pathologies characterized by high heterogeneity. These challenges make it necessary to understand the effectiveness of candidate biomarkers for early diagnosis, as well as to obtain a comprehensive mapping of how selective treatment alters the progression of the disorder. A large number of computational methods have been developed to explain network-based approaches by integrating individual components for modeling a complex system. In this review, high-throughput omics methodologies are presented for the identification of potent biomarkers associated with AD and PD pathogenesis as well as for monitoring the response of dysfunctional molecular pathways incorporating multilevel clinical information. In addition, principles for efficient data analysis pipelines are being discussed that can help address current limitations during the experimental process by increasing the reproducibility of benchmarking studies.
Citation: Marios G. Krokidis, Themis P. Exarchos, Panagiotis Vlamos. Data-driven biomarker analysis using computational omics approaches to assess neurodegenerative disease progression[J]. Mathematical Biosciences and Engineering, 2021, 18(2): 1813-1832. doi: 10.3934/mbe.2021094
[1] | Annalisa Vitale, Rossella Villa, Lorenzo Ugga, Valeria Romeo, Arnaldo Stanzione, Renato Cuocolo . Artificial intelligence applied to neuroimaging data in Parkinsonian syndromes: Actuality and expectations. Mathematical Biosciences and Engineering, 2021, 18(2): 1753-1773. doi: 10.3934/mbe.2021091 |
[2] | Xuewei Wang, Xiaohu Zhao, Fei Li, Qiang Lin, Zhenghui Hu . Sample entropy and surrogate data analysis for Alzheimer’s disease. Mathematical Biosciences and Engineering, 2019, 16(6): 6892-6906. doi: 10.3934/mbe.2019345 |
[3] | Leandro Donisi, Giuseppe Cesarelli, Pietro Balbi, Vincenzo Provitera, Bernardo Lanzillo, Armando Coccia, Giovanni D'Addio . Positive impact of short-term gait rehabilitation in Parkinson patients: a combined approach based on statistics and machine learning. Mathematical Biosciences and Engineering, 2021, 18(5): 6995-7009. doi: 10.3934/mbe.2021348 |
[4] | Avner Friedman, Wenrui Hao . Mathematical modeling of liver fibrosis. Mathematical Biosciences and Engineering, 2017, 14(1): 143-164. doi: 10.3934/mbe.2017010 |
[5] | Hongwei Sun, Qian Gao, Guiming Zhu, Chunlei Han, Haosen Yan, Tong Wang . Identification of influential observations in high-dimensional survival data through robust penalized Cox regression based on trimming. Mathematical Biosciences and Engineering, 2023, 20(3): 5352-5378. doi: 10.3934/mbe.2023248 |
[6] | Wei Yin, Tao Yang, GuangYu Wan, Xiong Zhou . Identification of image genetic biomarkers of Alzheimer's disease by orthogonal structured sparse canonical correlation analysis based on a diagnostic information fusion. Mathematical Biosciences and Engineering, 2023, 20(9): 16648-16662. doi: 10.3934/mbe.2023741 |
[7] | Huiqing Wang, Xiao Han, Jianxue Ren, Hao Cheng, Haolin Li, Ying Li, Xue Li . A prognostic prediction model for ovarian cancer using a cross-modal view correlation discovery network. Mathematical Biosciences and Engineering, 2024, 21(1): 736-764. doi: 10.3934/mbe.2024031 |
[8] | Shuwei Zhu, Wenping Wang, Wei Fang, Meiji Cui . Autoencoder-assisted latent representation learning for survival prediction and multi-view clustering on multi-omics cancer subtyping. Mathematical Biosciences and Engineering, 2023, 20(12): 21098-21119. doi: 10.3934/mbe.2023933 |
[9] | Yu Jin, Zhe Ren, Wenjie Wang, Yulei Zhang, Liang Zhou, Xufeng Yao, Tao Wu . Classification of Alzheimer's disease using robust TabNet neural networks on genetic data. Mathematical Biosciences and Engineering, 2023, 20(5): 8358-8374. doi: 10.3934/mbe.2023366 |
[10] | Natalya Shakhovska, Vitaliy Yakovyna, Valentyna Chopyak . A new hybrid ensemble machine-learning model for severity risk assessment and post-COVID prediction system. Mathematical Biosciences and Engineering, 2022, 19(6): 6102-6123. doi: 10.3934/mbe.2022285 |
The complexity of biological systems suggests that current definitions of molecular dysfunctions are essential distinctions of a complex phenotype. This is well seen in neurodegenerative diseases (ND), such as Alzheimer's disease (AD) and Parkinson's disease (PD), multi-factorial pathologies characterized by high heterogeneity. These challenges make it necessary to understand the effectiveness of candidate biomarkers for early diagnosis, as well as to obtain a comprehensive mapping of how selective treatment alters the progression of the disorder. A large number of computational methods have been developed to explain network-based approaches by integrating individual components for modeling a complex system. In this review, high-throughput omics methodologies are presented for the identification of potent biomarkers associated with AD and PD pathogenesis as well as for monitoring the response of dysfunctional molecular pathways incorporating multilevel clinical information. In addition, principles for efficient data analysis pipelines are being discussed that can help address current limitations during the experimental process by increasing the reproducibility of benchmarking studies.
Neurodegenerative diseases such as Alzheimer's disease (AD) and Parkinson disease (PD), involve the loss of structure or function of neurons in the brain or peripheral nervous system, including neuronal death. The topological complexity of the neuropathological vulnerability and transcriptional regulation in AD and PD in the brain suggest a need to analyze in more detail the molecular mechanisms that govern disease susceptibility and progression [1]. On the other hand, accurate and reliable biomarkers for these disorders are urgently needed to help both diagnose, especially in the early stages, and monitor the progression of symptoms. A comprehensive overview of expression profiles specific for each disease cell type specific, distinct biomarker profiles and topological composition of cell types provides the opportunity to shed light on the diversity in brain deterioration and spread of neuropathological symptoms observed between patients. Unfortunately, limited candidate biomolecules have preceded the discovery phase of the development pipeline for an objective diagnostic biomarker. Typical signs of neurodegeneration are the activation of microglia and the inflammatory response of astrocytes (neurotoxic and neuroprotective; M1- or M2-phenotype microglia and A1- or A2- phenotype astrocytes) accompanied by increased expression of cytokines as well as mediators of the immune system in the brain and cerebrospinal fluid [2]. Neuroinflammation can be characterized as contradictory, covering many distinct responses to the neurodegenerative process. Inflammation triggered by the central nervous system (CNS) innate immune response, as defined by microglial activation and accompanied by astrocyte responses along with increased expression of cytokines and immune system mediators in the brain and cerebrospinal fluid (CSF), is constant in neurodegeneration [3]. Although anti-inflammatory therapeutic strategies have not been successful in the past, the development of a strategy that potentiates protective microglia functions could be a promising model for ND treatment, suggesting that novel approaches against inflammation may be useful and could reduce the progression of the disease [4].
Advancements in the field of omics approaches allow the monitoring of abnormalities in multiple interconnected networks necessary to detect transcriptional, translational and metabolic changes whereas they demonstrate the complexity of the dynamic alterations related to AD and PD progression, highlighting the challenges associated with designing and evaluating effective therapeutic interventions. The two neurodegenerative diseases share many clinical and pathological features and many similar cascades of neuronal reactions leading to progressive neurodegeneration occur in Alzheimer's disease and Parkinson's disease. Cognitive decline is common in both AD and PD (cortical and subcortical dementia, respectively), as well as behavioral symptoms like depression, apathy, sleep disturbances and anxiety. The toxicity of specific proteins, due to the accumulation of abundant extracellular Αβ plaques and intraneuronal neurofibrillary tau tangles present in AD as well as abnormal filaments of α-synuclein leading to progressive degeneration of dopaminergic neurons in PD, results in overlapping common pathological alterations such as the activation of glycogen synthase kinase-3 beta, mitogen-activated protein kinases, mitochondrial dysfunctions, oxidative stress and neuroinflammation [5]. Moreover, high levels of α-synuclein agammaegation into Lewy body have been reported in patients with AD, with nicotinic receptors and loss of locus coeruleus may occurring in the progression of both diseases [6]. Together with high-throughput bioinformatics methodologies and integrated omics and pharmacological data, specific biomarker panels can be studied simultaneously, allowing these highly promising technologies to recognize variable changes across multiple interconnection networks and understand the pathophysiology of complex diseases [7]. Moreover, pathway analysis along with distinct molecular profile provide important neuropathological information as well as increased disclosure of underlying disease mechanisms and guidance in the design and development of new therapeutic strategies to monitor disease efficacy. The contribution of functional imaging techniques in medicine such as the positron emission tomography or the magnetic resonance imaging also helps to enhance the accuracy of the diagnosis. These neurobiological and neuroimaging efforts can illuminate the heterogeneity of prognostic biomarkers and molecularly targeted drugs for neurodegenerative diseases that are important in selecting appropriate patients for specific treatments as a one-size-fits-all medicine to patient-specific medicine shift strategy [8].
Integrated omics methods eliminate speculation arising from the discrepancy between discovery and validation associated with biomarker-driven research process. The analysis of biomedical data allows the exploratory of important molecular entities, biological molecules and features of the interactions that lead to a mechanistic understanding of the observed clinical symptoms, while the familiarity of software packages allows the handling of genotype and phenotype data with large data integration. In attempting to depict cellular malfunctions during neuronal loss, an initial model of the system should be provided based on prior genetic information along with insights on proteome and metabolite changes as well as epigenetic abnormalities [9]. Parallel targeted omics approaches are proposed instead of a single approach to promote the exploratory of candidate biomarkers with ND cases. A large number of computational approaches have been developed to generate co-expression networks from genomics to transcriptomics and protein expression data, along with functional annotations and regulatory networks.
Alzheimer's disease (AD) is a genetically complex, multifactorial disorder that leads to memory loss, difficulty thinking, and changes in behavior. AD preferentially affects individuals over 60 years of age with a steadily increasing risk at older ages [10]. There is no treatment for AD yet and the prevalence of the disorder rises from about 1% in the under-65 population to about 40% in nonagenarians. Due to its high prevalence and unreservedly high occurrence, it poses a serious problem to both personal health and the healthcare system. The majority of AD cases are sporadic (>95% prevalence) without a specific genetic basis and the symptoms are progressive with age being the strongest risk factor [11]. The disease is preceded by a long prodromal phase and most patients have late-onset AD. The progressive hippocampal and cortical atrophy are among the neuropathological alterations in the AD brain that are visible upon neuroimaging and macroscopic examination. Moreover, among the most representative microscopic features are extracellular depositions of amyloid-β (Aβ1–42) peptide and intraneuronal tangles of hyperphosphorylated forms of microtubule associated protein tau, in combination with neuronal and synapse elimination and reactive gliosis. Microglia responses to β-amyloid are the focus of many studies that depend on the significant contribution of β-amyloid cascade to AD progression (Figure 1A). Undoubtedly, the inflammatory response and the activation of microglia associated with β-amyloid behavior are under investigation [12].
Causal mutations in specific genes have been identified in early-onset forms, including amyloid precursor protein (APP), presenilin-1 (PSEN1) and presenilin-2 (PSEN2). The apolipoprotein E (APOE) ε4 allele is highly associated with an increased risk of AD developing considering that approximately 25% of AD cases carry one or more copies of the allele. Genome-wide studies, molecular genetic investigation and pathway-based exploration can help define AD subetaoups, identify diagnostic indicators of the disease and ultimately shed light on improved and adapted treatments. Many studies that have either focused on detecting new genetic risk factors or investigated the relationship between single nucleotide polymorphisms (SNPs) and Alzheimer's disease have shown specific genes/loci associated with late-onset disease progression, among them NME8, FERMT2, PICALM, PTK2B, CD2AP, CD33, CELF1SLC24A4/RIN3, FERMT2, CASS4 and DGS2 [13]. Moreover, different analytical methodologies with an emphasis on sliding window haplotype-based approach and gene-wide analysis have shown that FRMD4A as well as TP53INP1 and IGHV1-67 are potential risk loci [14]. Furthermore, according to the GWAS investigation for single nucleotide polymorphisms related to late-onset, a significant number of variations in genes such as ATP-binding cassette subfamily A member 7 (ABCA7), CD2 associated protein (CD2AP), CD3 molecule (CD33), complement component 3b/4b receptor 1 (CRI), EPH receptor 1 (EPHA1), inositol polyphosphate-5-phosphatase D (INPP5D) and phospholipase D family member 3 (PLD3) has been reported [15].
The search for genetic risk factors for polygenic AD was initially dominated by studies querying common genetic variation, most successfully through GWAS. Specific loci including BIN1, CD2AP, PICALM and PLD3 have been associated with late-onset AD at genome-wide significance [16]. The regulatory role of ATP-binding cassette, sub-family A, member 7 (ABCA7), has been originally determined through genomics, transcriptomics and methylomics analysis as a novel risk gene and potent pharmacological target for Alzheimer's disease [17]. It should be noted here the impact of CLU, BCA7, CD33, CD2AP, EPHA1 and CRI serving as regulatory molecules on the complement response along with the strong involvement of EPHA1 and INPP5D in inflammatory cascade compared to the limited knowledge of genes such as CLU, CR1, SORL1, PLD3, and PICALM even if the latter is associated with the APOE genotypes as provided by extensive next-generation sequencing assessment of entire exomes and genomes [18]. Specific databases, including Reactome, KEGG and ALIGATOR were used to analyze the vast number of GWAS datasets that involved ADNI, central regulator genes such as SPI1 and TYROBP, complex measure of memory as phenotype, GERAD/EADI and combined TGen1 [8]. Combined techniques such as the whole genome and whole exome sequencing of a rare non-synonymous mutation have also been utilized, shedding light on a number of different genetic risk factors that contribute to AD and suggesting potential targets for the development of treatment. Characteristic indications can be identified such as ABCA7 for heterozygous PTC mutations, SORL1 in patients with early-onset AD (EOAD) and R4H7 in TREM2 with increased risk in AD with limited exome-wide significance regarding PLD3, AKAP9 and UNC5C [19]. In addition to GWAS, transcriptome-wide association studies have supported the exploration of specific loci related to transcriptional regulation as well as in parallel with GWAS data as a valuable combinatory analysis. CLU, PTK2B, and CR1 are characteristic genes provided by this approach, while postmortem brain transcriptome studies in AD and healthy controls from RNA-Seq and microarrays detected a variety of genes that were differentially expressed [20]. Moreover, functional annotation leads to differentially expressed pathways that are affected such as accumulation of abnormal neuritic plaques, synaptic transmission, endocytic APP trafficking, immune response and apoptosis.
Parkinson's disease is a common neurodegenerative disease characterized by progressive degeneration of dopaminergic neurons of the substantia nigra, a significant diminution in the neurotransmitter dopamine in the nigrostriatal region of the brain as well as the formation of Lewy dystrophic neuritis and Lewy body insertions [21]. One of the hallmarks of the disease is the depletion of dopamine, as the pathological evaluation of the postmortem brain indicates the degeneration of substantia nigra in the pars compacta, resulting in dopamine deficiency [22]. Although the majority of PD apparently is sporadic, 5–10% is inherited. There is currently no therapy for termination or delaying the neurodegenerative process, as the exact mechanisms governing the pathogenesis of PD require further investigation. However, a number of key genes play an important role in the etiology of PD and the elaboration of the α-synuclein pathology, whereas lysosomal and mitochondrial behavioral disruptions seem to play a crucial role in PD pathogenesis [23]. According to the histopathological and molecular profile of PD patients, the involvement of oxidative stress in the pathogenesis of the disorder has increased due to recent advances in the genetics of PD. The accumulated presence of iron in substantia nigra is perfectly consistent with iron-catalyzed oxidative stress capable of stimulating strong interaction between alpha-synuclein agammaegation and mitochondrial dysfunction. Furthermore, mitochondrial efficiencies and protein processing affect each another and is the subject of intense research, including the ubiquitin-proteasome system that regulates mitochondrial fission and fusion. According to numerous studies, reactive oxygen species can play an important role as signaling molecules that can initiate the activation of transcription of proteins or protein misfolding in the mitochondria, induction of cell-mediated immunity or sirtuin-mediated mitochondrial stress response [24]. The endoplasmic reticulum (ER) and the subsequent unfolded protein response (UPR) may be involved in the pathogenic mechanisms of the disease affecting neuronal regulation, as proteins lose their acquisition upon post-translation modification process including characteristic folded confirmation. The UPR intervenes in cellular adaptation to reinstate ER homeostasis under acute ER stress conditions while apoptosis can be triggered upon chronic exposure to reduce damaged cells. Based on this mechanism, three transmembrane sensor proteins are activated, the IRE1 (inositol-requiring enzyme-1), ATF6 (activating transcription factor 6) and PERK (protein kinase RNA-like endoplasmic reticulum kinase), along with the transcription factors, XBP1s (X box protein 1), ATF6f and ATF4. This cascade leads to regulation of the expression of important molecules associated with protein folding, protein apoptosis and autophagy [25]. Among the variety of genes related to rare monogenic forms of PD are leucine-rich repeat kinase 2 (LRRK2), parkin, DJ-1 and PTEN-induced kinase 1 (PINK1) (Figure 1B). Evidence accumulation indicates that Parkin (PARK2) is suspected of an autosomal-recessive trait of the disease, while several familial PD-linked mutations, including ATP13A2 (PARK9) have been observed. Exonic deletions or duplications in PARK2 are frequently associated with Parkinsonism, while genomic replications in the SNCA (the gene encoding α-synuclein) or predominant Ala30Pro and Ala53Thr substitutions lead to nigral neuronal loss. Similarly, exon-deletion mutations in Parkinsonism-associated PINK1 are gradually evolving into limited doses of L-DOPA [26]. Moreover, delayed progressive Parkinsonism or the expression of sporadic symptoms can be caused by mutations in DJ1 related to recovery of progressive symptom expression. The deubiquitylating enzyme, UCH‑L1, has also been found to be linked to a genetic predisposition. To this must be added the identification of an E46K mutation in a family member with Lewy bodies dementia along with selective mutations in GCH1 (GTP cyclohydrolase 1), ataxin 2 (ATXN2), VPS35 retromer complex component, DNAJC6 and DJ1 (Leu166Pro substitution), factors leading to Parkinsonism with dementia or amyotrophy [27,28].
A few susceptibility loci, including a-synuclein, tau and LRRK2 have been demonstrated in PD genome-wide association studies. These indications in combination with the already known data that a-synuclein accumulation, in the form of Lewy bodies, is also detected in other neuronal cell types in various neurodegenerative diseases may lead to a possible common mechanism of disease onset and be responsible for familial autosomal dominant PD [29]. Activation of ROS-regulated signaling molecules and related pathways may also be stimulated by reduced proteasomal activity or problematic mitochondrial efficiency that causes the detection of mitochondrial protein oxidation and degradation. Furthermore, this scenario can cause significant damage to the synaptic regions of dopaminergic cells and their intracellular environment, as this type of cells is rich in mitochondria as well as key molecules such as dopamine and α-synuclein. The important role of these signaling molecular signatures should be emphasized here, as they are able to attenuate proteasomal activity, interact with cellular and mitochondrial calcium flux and mitochondrial dynamics using NADH/FADH2 as well as electron activation and initiate intracellular mechanisms for depolarized or inefficient regulation of mitochondria in the cell compartment for macroautophagy [30]. The protective effect of a significant number of proteins involved in pathways implicated in PD, including Parkin and G2019S LRRK2, has been reported through proteomics. However, other model studies have shown a relationship between α-synuclein and the RAB1 ER-to-Golgi modulator, leading to neuronal loss [31]. Protein agammaegation has apparently been reported to be of great importance for the detection of factors that prevent the accumulation or distribution of inclusions as well as the involvement of protein quality control and clearance systems.
A promising therapeutic approach for PD can be considered gene therapy though viral vector methodologies. By performing this strategy, genes can be transferred to nuclei and genetically alter the neuronal environment of the basal ganglia or modify specific neurotrophic factors such as CDNF, VEGF-A and neurturin. Moreover, this technology can determine the limited level of dopamine release and the capacity of the circuit controlling movement, enriching the effectiveness of dopamine replacement [32]. The incorporation of specific neuroprotective genes has proven to be a sensible therapeutic approach that includes both Adeno-associated virus (AAV) and lentiviral vectors for the transport of genetic material to both the substantia nigra and the striatum. Alterations in α-synuclein levels are a demanding factor due to their significant contribution to neurotransmitter release, while silencing through RNA interference has shown nigral degeneration in animal models [33]. Parkin mutations in patients are characterized by an atypical form of Parkinsonism and delay disease progression, as the substantia nigra does not include Lewy bodies with elevated a-synuclein expression. For these cases, the PINK1-Parkin pathway as a potential pharmacological target is highly uncertain. The bacterial clustered regularly interspaced short palindromic repeats (CRISPR)-associated protein-9 nuclease (Cas9) could also provide important therapeutic indications including a variety of targeted genes. Genome-wide association data of PD patients' characteristics can be found in the PDGene database, while according to the International Parkinson's Disease and the Movement Disorders Genetic Mutation Database (MDSGene), a large international project encompassing genes for PD as well as for several other inherited movement disorders, a systematic summary of clinical cases has been classified and causative mutations in SNCA, Parkin, PINK1, VPS35, SYNJ1, DNAJC6 and FBXO7 have been described [34]. NADH ubiquinone oxidoreductase core subunit S1 and Cytochrome C oxidase subunit 4I1 are involved in PD regulatory cascade, while cross-platform datasets have shown significant monitoring between new therapeutic strategies and prognostic indicators, among them MAPK8, CDC42 and COX4I1 as well as miR-126-5p, miR-19-3p and miR-29a-3p [35]. Large-scale transcriptomic datasets obtained from a transcriptome-wide association study in dorsolateral prefrontal cortex and peripheral monocytes cells highlighted sixty-six important molecules related to predicted expression or splicing levels [36].
The use of high-throughput omics technologies is widely used in biomedical research to enable personalized medicine leading to the rapid discovery of many candidate biomarkers and approaches to enhance their validation. Genomics, transcriptomics and metabolomics contribute to the identification of important diagnostic and prognostic biomarkers that help to reveal molecular mechanisms of specific disorders whereas integration of such multiple technologies provide a more comprehensive view of disease as well as significant benefits for patient well-being [37]. Overlaying omics datasets across pathways along with extensive bioinformatic analyses enabled outcome and phenotypic distinctions to be defined, making these platforms highly important for interpreting and supporting clinical decisions (Figure 2A). Omics strategies have figured a window into the diagnostics of devastating brain disorders such as neurodegenerative diseases. Hybridization-based approaches such as microarrays have been widely used as technology over the past decade to explore the gene expression profiling that represents an efficient approach to quantifying thousands of transcripts. The method starts with reverse transcription of complementary DNA (cDNA) from RNA substrates and afterwards the samples are inserted into the array prior the analysis, such as differential gene expression. More recently, RNA-Seq and next-generation sequencing platforms have emerged as alternative approaches for gene expression profiling, providing powerful hybridization-free approaches that allow massive parallel mapping sequenced fragments of cDNA. Undoubtedly, microarrays have been overshadowed by high-throughput sequencing with RNA. The main difference among the two methods is that the latter allows for full sequencing of the whole transcriptome compared to the predefined transcripts through hybridization performed by microarrays, providing higher specificity and sensitivity to detect novel transcripts. Moreover, RNA-Seq, as a state-of-the-art technique, is accomplished through a selection of subsets of RNAs from a total RNA pool and secondly reverse transcription is performed to obtain a cDNA library, contributing to significant challenges on full-length transcript structure identification [38]. Moreover, the inability to distinguish between distinct transcript isoforms is a weakness of this approach. Surely, microarray data analysis for transcriptome profiling can be confused with adaptations to probe hybridization sensitivity between array platforms, while the development of probes for unknown sequences is not applicable and the detection and accurate quantification of limited expressed transcripts proves a difficult issue. In order to accomplish reliable meta-analyses for the interpretation of multiple independent datasets, updates to the human reference genomes should also be considered [11]. cDNA sequencing can also be executed using the directional RNA-Seq technique that provides massive knowledge of reads. The latter is useful for revealing new splicing events involved in dissecting the complexity of the disorders. Knowledge of descriptive data between these approaches is extremely important to uncover the very complex scenario caused by neurodegeneration in the context of these highly progressive disorders. A better understanding of the aspects of each methodology will help identify specific targets at the appropriate stages of particular diseases for effective and highly selective therapeutic interventions.
Metabolomics is the latest systems biology approach that offers great potential for diagnosing and predicting neurodegenerative diseases by measuring the levels of individual metabolites simultaneously in clinical and biological samples, discovering reliable biomarkers as well as developing effective therapeutic interventions. The advantage of this powerful methodology is based on its ability to demonstrate qualitative and quantitative changes of a defined set of metabolites which are also dynamic with each other, reflecting changes in multiple functional and regulatory networks [39]. Quantitative measurements of the human metabolites can be performed either by mass spectrometry (MS) or nuclear magnetic resonance spectroscopy, two powerful analytical platforms utilized also for detection and structural characterization of molecules. MS is a destructive technique that provides high sensitivity, selectivity, flexibility and the ability to analyze a wide range of metabolites providing quantitative data. Combination with chromatography techniques such as liquid chromatography, gas chromatography or capillary electrophoresis is essential for sample separation prior to mass spectrometer injection. This step is used as multiple executions to obtain a sufficient number of samples and increases the analysis time along with the selection of the ionization approach such as electrospray ionization (ESI) or matrix-assisted laser desorption ionization (MALDI) [40].
Nuclear magnetic resonance spectroscopy-based metabolomics, on the other hand, is a non-destructive, low-cost approach that provides detailed information on molecular structure. Cryogenic probes and high-field magnets are used during sample quantification, however, this methodology is no less sensitive to low molecular weight metabolites [41]. As the brain is highly dependent on glucose, which consumes about 20% of the total energy derived from glucose, cerebral hypometabolism and abnormalities associated with reduced glucose utilization can be observed with this approach. Plasma and CSF metabolomics studies in patients with MCI and AD showed an effect on ketone bodies to maintain energy homeostasis, while analysis of the metabolic network of the first group showed changes in lipid metabolism, mitochondrial ketone bodies and tricarboxylic acid cycle. On the other hand, AD individuals indicated metabolic alterations associated with neurotransmission and inflammation as well as in TCA cycle and lysine metabolism [42]. The glycerophospholipid pattern predominately altered in AD cortex with increased NAA levels while mitochondrial dysfunction and aspartate metabolism correlated with dementia and AD pathology have been observed in the frontal cortex from 21 AD and 19 CN in a metabolomic study using UPLC-HILIC-MS and ionKey/MS [43]. Cerebellum, middle frontal gyrus and inferior temporal gyrus analysis from 14 AD, 14 CN, and 15 asymptomatic patients revealed global brain UFA perturbations and region-specific alterations in patients with AD. Moreover, reduced linoleic acid, linolenic acid and arachidonic acid within the middle frontal gyrus and elevated levels of docosahexaenoic acid were observed, evidence that may be indicated as peripheral threshold fingerprints associated to Aβ plaques, tau tangles, and cognitive decline [44]. Using ultra performance liquid chromatography-tandem mass spectrometry alterations in 17 metabolites in the methionine cycle were depicted in AD plasma and CSF samples [45]. Moreover, the levels of sphingomyelins and ether-containing phosphatidylcholines were found altered in a cohort consisting of 732 fasting plasma samples from an ADNI-1 pool [46]. In another lipidomic study using a high-resolution mass spectrometer, polyamine metabolism, sphingolipid transport and saturated fatty acid biosynthesis were found altered in AD plasma samples with parallel diminution of ceramides and phosphocholines [47]. Lastly, 3-hydroxypalmitoleylcarnitine, lysoPC and specific amino acids such as histamine, citrulline, and nitrotyrosine were indicated as a well-defined metabolic framework in analysis of aMCI/AD plasma samples by UPLC-MS [48].
The starting point for implementing an integrated analytical approach is the development of the appropriate protocols that includes a sequence of steps through which biological samples are processed and analyzed measurements (Figure 2B). These biological protocols derive key features from existing methods found in conventional testing procedures performed in clinical practice. All the steps that will be able to support the process are the ones that will determine its specifications in terms of design and functionality [49]. Through the detailed modeling of the individual experimental phases, it is possible to implement an efficient computational tool that achieves complete automation of the experimental process with high accuracy of the produced results. Initially, an important step is to normalize the data values to control all samples if the expression values from different experiments are comparable. The results cannot be taken into account using incorrect normalization or inflated false positives. Further steps are the supplementary values for the absent expression values, the adoption of a common nomenclature and the total number of reads, along with the deletion of samples having similar identifiers and the finding of differentially expressed genes between the control and the test state [50]. Statistical approaches such as signal-to-noise ratio, fold-change, and correlation coefficient are usually followed to unravel the differentially expressed molecules.
Focusing on pathway analysis methodologies, over-representation analysis can derive a model which is based on genomics data and describes process complexity. The basic premise of a Functional Class Scoring (FCS) analysis is that in addition to large changes in gene expression, smaller changes are important whose component significantly affects the condition of the pathway [51]. Three steps comprise this approach, starting with the calculation of statistic at the gene level as well as in the general methodology. Compilation of individual statistics from the genes of each pathway into a pathway-level statistic is following, a process which is equivalent to selecting statistics at the gene-level set. Lastly, a statistical evaluation of each pathway should be performed based on the null hypothesis adopted. Furthermore, the standard hypothesis of a Pathway Topology Based Analysis is that the interactions contained in the pathway topology are important in studying the correlation of changes that occur between parts of a pathway, addressing some of the limitations of previous methods. These interactions follow the same general methodology except for the calculation of statistics at the genome level where the topology of the whole pathway is used [52]. These approaches provide information on the nature of their members' interaction, which allows different weights to be assigned to each gene depending on the alterations that occur in each individual's the expression and the effect it has on the condition of the entire pathway. In addition, different biological states may correspond to different interactions between the same genes. Knowledge of the interactions allows the approach to be able to distinguish the two states which by considering only the genes and their expression would be observed identical. Among the key limitations can be pointed out that these methodologies can determine whether there is an enrichment of the pathway without addressing the exact time point of this specific enrichment as well as they do not take into account pathway correlations to detect possible interactions among them (Table 1). The Subpathway-GM method is one approach of indentifying biologically interesting metabolic pathways by integrating information from genes and metabolites, as well as specifying their location within the topology of a pathway. The method incorporates data sets from different omics related to the disease's condition and studies in depth the respective enzymatic networks within the metabolic pathway [53]. In the case of irreversible reactions, a substrate points to the corresponding enzyme while an enzyme points to the corresponding product. In the case of reversible reactions, the opposite directions may occur. The Topology Enrichment Analysis detects correlations between genes within enriched biologically important pathways. The method extracts linear and non-linear sub-paths which are ultimately scored using specific criteria for specialized data as well as for case-control studies [54].
Method type | Disease | Highlights | Reference |
Genomics and transcriptomics analysis | AD | new genes/loci associated with late-onset disease progression, (NME8, FERMT2, PTK2B, CASS4, DGS2) | Yan et al. [13] |
genome-wide association study | AD | new risk loci (FRMD4A, TP53INP1, IGHV1-67) | Escott-Price et al. [14] |
genome-wide association study | AD | late-onset AD associated loci (BIN1, CD2AP, PICALM, PLD3) | Van Acker et al. [16] |
genome-wide association study | AD | single nucleotide polymorphisms related to late-onset disease progression (ABCA7, CD2AP, CD33, PLD3 | De Roeck et al. [17] |
whole genome and whole exome sequencing | AD | complement response (CLU, BCA7, CD33, CD2AP, EPHA1), inflammatory cascade (EPHA1, INPP5D) | Reitz et al. [18] |
whole genome and whole exome sequencing | AD | new risk loci related to early-onset progression (SORL1, R4H7) | Bellenguez et al. [19] |
transcriptome-wide association studies | AD | accumulation of abnormal neuritic plaques, synaptic transmission, endocytic APP trafficking, immune response and apoptosis (CLU, PTK2B, and CR1) | Ciryam et al. [20] |
genome-wide association study | AD | PARK-SYNJ1, PARK-DNAJC6, PARK-FBXO7, CHCHD2, GBA | Klein et al. [34] |
meta-analysis of multiple gene expression arrays datasets | AD | MAPK8, CDC42, NDUFS1, COX4I1, SDHC and miR-126-5p, miR-19-3p, miR-29a-3p, lipid metabolism and mitochondrial dysregulation | Chi et al. [35] |
transcriptome-wide association study | AD | 66 risk genes with predicted expression or splicing levels (SNCA, CLASP2, TMEM175, GPNMB, CTSB, CAMLG, and NUDT14) | Li et al. [36] |
genome-wide association study | PD | familial autosomal dominant PD (a-synuclein, tau and LRRK2) | O'Hara et al. [29] |
transcriptomics/meta analysis brain samples | PD | signaling pathways and protein-protein interaction networks (REST as an upstream regulator) | Kelly et al. [62] |
transcriptomics/meta analysis brain samples | PD | changes in dopaminergic neuronal transcription, NPTX2, DEFA3, DEFA1 | Mariani et al. [63] |
frontal cortex metabolic profiling | AD | mitochondrial dysfunction, aspartate metabolism variation | Paglia et al. [43] |
brain tissue metabolic profiling | AD | dysregulation in the metabolism of unsaturated fatty acids | Snowden et al. [44] |
plasma and CSF metabolic profiling | AD | changes in the methionine cycle (homocysteine, S-adenosylmethionine, 5-methyltetrahydrofolic acid) | Guiraud et al. [45] |
plasma metabolic profiling | AD | alterations in sphingomyelins and ether-containing phosphatidylcholines | Toledo et al. [46] |
plasma metabolomics | AD | alterations in 3-hydroxypalmitoleylcarnitine and lyso phosphatidylcholines | Mapstone et al. [48] |
Computational biology and bioinformatics provide important assistance in biomedical research for the analysis, clustering and validation of molecular and clinical indications, with the aim of prognostic evidence, diagnostic markers identification and efficient treatment. The development of high-throughput methodologies in accordance with state-of-the-art infrastructure and powerful tools is highly important, whereas the establishment of bioinformatics platforms for data pre-processing can support the storage and integration of clinical data, combination of up-to-date biomedical knowledge with analytical pipelines and therapeutic development [55,56]. A computational workflow for biomarker extraction is depicted in Figure 3. The proposed workflow consists of three stages, namely the: (ⅰ) data curation stage, (ⅱ) biomarker extraction stage, and (ⅲ) validation stage. The data curation stage involves automated functionalities for the detection of similarities among the input medical data using covariance and correlation-based scores, as well as, functionalities for outlier detection using both univariate (e.g., the interquartile range) and multivariate (e.g., the isolation forests and the elliptic envelopes) methods and data standardization. In addition, the data curation stage eliminates any data incompatibilities that are present within the data structure, as well as, resolves data format issues that obscure data management and analysis [57]. The biomarker extraction stage is then applied on the curated data and involves functionalities for: (ⅰ) the definition of the target feature (i.e., the feature of interest), (ⅱ) the application of class imbalance handling methods by randomly down sampling the majority class (i.e., the group of controls) with replacement, given a specific downsampling ratio, and (ⅲ) the application of feature selection methods, such as, the False Correlation Based Feature (FCBF) selection algorithm or feature ranking methods, such as false detection rate (FDR) methods based on ANOVA scores, and the Information Gain (IG), among others, to identify prominent features (biomarkers) that are highly associated with the pre-defined target feature and less associated among them. The prediction performance of the extracted biomarkers is validated against the performance of existing biomarkers in the validation stage, where supervised machine learning algorithms are applied, to yield explainable and robust AI models for disease prediction and patient risk stratification, among others [58].
Another method for the identification of biomarkers, based on bioinformatics approaches is through the estimation of the protein-protein interaction affinity (PPIA) from gene expression data and further identification of a set of protein-protein interactions and single proteins as network biomarkers for diagnosing/prognosticating diseases. Firstly, PPIA can be approximated by the law of mass action. Then a model can be developed to indicate a set of PPIAs and single proteins as network biomarkers, where theoretically each class or cluster of samples is depicted by an ellipsoid. The selection of minimal numbers of PPIs and proteins can be extracted according to this process to maximize the distance between different ellipsoids [59]. The "Expression Data UpStream Analysis" (EDUSA), an innovative bioinformatics approach, has been developed for the compelling analysis and categorization of the genomic pattern to assess a coherent explanation of the PD mechanism, revealing the different levels of disease progress by summarizing data within a single or multiple annotation [60]. As an example of EDUSA disease-oriented genomic expression profiling data analysis, the methodology begins with sample collection, the analysis of differentially expressed genes is the second step, followed by the categorization of over-represented biological families using the Expression Analysis Systematic Explorer software as well as the depletion of repeated groups applied to conjugated gene clusters and terminates by defining a hierarchy of interactions between processes.
The advantages of this highly promising process may include the rapid identification of pathways affected by disorder through tissue samples taken from both patient and non-PD groups. Transcriptomic data related to neurological disorders have been also provided though a web portal tool that included annotations to each study for in-depth investigation [61]. Moreover, a meta-analysis approach was proposed to observe altered gene expression in PD brain microarrays datasets as well as to compare these profiles with AD datasets, noting the similarities and cross-talk between PD and AD differentially expressed genes in the same direction and pathways [62]. Another software called Transcriptome Mapper (TRAM) has been used in brain PD and microarray data from healthy individuals, and meta-analysis has also been performed to determine changes in dopaminergic neuronal transcription by integrating multiple datasets from independent trials. Deregulation of characteristic genomic loci and regions involving neurodegenerative functional pathways as well as genes and non-coding RNA transcripts can be ascertained by this methodology, while genetic signatures with effective overexpression in substantia nigra like NPTX2, DEFA3 and DEFA1 were reported [63]. Modifying known cellular pathways and investigating molecular processes could verify system-level variations in omics datasets, and to address this issue, a variety of pathways and approaches can be extracted from known databases such as KEGG (Kyoto Encyclopedia of Genes and Genomes), Gene Ontology Resource, Reactome and BioCarta [64]. PANTHER and NCI-PID are also common pathway databases, while other complementary methods follow network and GO analysis. Omics-based pathways were clustered into different groups, while an over-representation analysis related to abundance evidence derived from omics datasets were depicted and GSEA (Gene set Enrichment Analysis) limited the need to describe a significant threshold. Network Module-based Pathway Analysis (NMPA) uses algorithms to explore prior knowledge from intracellular networks as well as determine sub-network regions and correlate them with common pathways. In addition, Network Topology-based Pathway Analysis (NTPA) methodology utilizes molecular network mapping to achieve direct quantification of pathway mappings and multiplicity of interconnections [65]. For each of these groups of pathway analysis a variety of software applications can be provided and substantial and visible results can be extracted among them DAVID and GOToolBox over-representation analysis tools. Non-topology-based approaches, known as gene set analysis techniques, should be highlighted including either the overpresentation analysis followed by EASE, Onto-Express and WebGestalt or the functional class scoring methods such as GlobalTest, GSA, PADOG, GSEA and FunCluster [66]. Additionally, PWEA, PathNet and ToPASeq, along with FunMOD, have been developed and can be used for network topology- and network module-based pathway analysis in an attempt to include all this important knowledge in the analysis. A crucial parameter could be also the selection of the appropriate pathway process that is highly related to the prior computation of the modified expression pattern for the individual molecular index within each omics dataset [67]. Furthermore, a detailed pathway analysis of genome wide association studies and the bias of sequencing results related to the related imbalance and amount of gene sets should be noted. In an effort to distinguish a PD-related modified molecular network, targeted software tools can be included either for network disruption analysis or for causal reasoning clarification, while these strategies are able to identify the regulatory network involved at a relevant biological level as well as regulatory linkages between genes or protein cascades. These tools are Whistle and SiGNet for causal reasoning and data analysis or GenePEN, BioNet/HEINZ and ClustEx for network disturbances study [23]. However, for the categorization or clustering of diseased subfamilies, machine learning for multilevel predictive modeling and high-dimensional data are extremely necessary. Computational approaches, following machine learning models, may give the opportunity to delineate the power of correlations between variables of interest or responses to intervention. In order to predict specific clinical outcomes or diagnostics phenotypes model-based and model-free approaches may be assessed. The application of model-based methods is highly dependent on the a priori statistical statements, such as determining the relationship between variables and model-specific assumptions associated with the process probability distributions while model-free techniques have the capacity to construct non-parametric representations using machine learning algorithms with fewer assumptions [68]. In addition, a variety of known software tools can be included for machine learning analyses of omics data that provide pathway categorization and visualization. Typical examples are Limma and RankProd, which are oriented towards ranking and feature selection, ArrayMining for multipurpose machine learning analysis and GGobi, which helps with visualization of low-dimensional data. WebGestalt and ToppGene can also examine the regulatory characteristics of PD-related genes, can dissect the global landscape mapping of molecular interactions, and unravel specific key genes with a highly regulatory role in PD for further analytical investigation [69].
The continued progression of high-throughput approaches helps to increase the reproducibility and the interpretation of biomedical data and results. In neurodegenerative disorders such as AD and PD, integrated omics methods and network-based analyses play a significant role in the discovery of new biomarkers, the analysis of disease states and the validation of potential therapeutics. Biological pathways analysis, including a set of computational tools, is used to extract knowledge from data resulting from multiple sequencing techniques, creating important information that attempts to describe the underlying biological processes. Through these approaches, the structure of the interactions that take place in neuronal environments and the response to possible disturbances can emerge, strengthening the data agreement with the experimental findings.
This research has been co-financed by the European Union and Greek national funds through the Operational Program Competitiveness, Entrepreneurship and Innovation, under the call RESEARCH–CREATE–INNOVATE (project code: T1EDK-05029).
The authors declare no conflicts of interest regarding the publication of this paper.
[1] | C. A. Lane, J. Hardy, J. M Schott, Alzheimer's disease, Eur. J. Neurol., 25 (2017), 59-70. |
[2] | H. S. Kwon, S. H. Koh, Neuroinflammation in neurodegenerative disorders: the roles of microglia and astrocytes, Transl. Neurodegener., 9 (2020), 42. |
[3] | C. Marogianni, M. Sokratous, E. Dardiotis, G. M. Hadjigeorgiou, D. Bogdanos, G. Xiromerisiou, Neurodegeneration and inflammation—an interesting interplay in Parkinson's disease, Int. J. Mol. Sci., 21 (2020), 8421. |
[4] |
S. H. Kim, M. Y. Noh, H. J. Kim, K. W. Oh, J. Park, S. Lee, et al., A therapeutic strategy for Alzheimer's disease focused on immune-inflammatory modulation, Dement Neurocogn. Disord., 18 (2019), 33-46. doi: 10.12779/dnd.2019.18.2.33
![]() |
[5] | Z. Han, R. Tian, P. Ren, W. Zhou, P. Wang, M. Luo, et al., Parkinson's disease and Alzheimer's disease: a Mendelian randomization study, BMC Med. Genet., 19 (2018), 215. |
[6] | A. Xie, J. Gao, L. Xu, D. Meng, Shared mechanisms of neurodegeneration in Alzheimer's disease and Parkinson's disease, Biomed. Res. Int., 2014 (2014), 648740. |
[7] | J. Montaner, L. Ramiro, A. Simats, S. Tiedt, K. Makris, G. C. Jickling, et al., Multilevel omics for the discovery of biomarkers and therapeutic targets for stroke, Nat. Rev. Neurol., 16 (2020), 247-264. |
[8] | W. J. Lukiw, A. Vergallo, S. Lista, H. Hampel, Y. Zhao, Biomarkers for Alzheimer's disease (AD) and the application of precision medicine, J. Pers. Med., 10 (2020), 138. |
[9] |
E. M. Cilento, L. Jin, T. Stewart, M. Shi, L. Sheng, J. Zhang, Mass spectrometry: A platform for biomarker discovery and validation for Alzheimer's and Parkinson's diseases, J. Neurochem., 151 (2019), 397-416. doi: 10.1111/jnc.14635
![]() |
[10] |
J. Verheijen, K. Sleegers, Understanding Alzheimer disease at the interface between genetics and transcriptomics, Trends Genet., 34 (2018), 434-447. doi: 10.1016/j.tig.2018.02.007
![]() |
[11] |
E. Cuyvers, K. Sleegers, Genetic variations underlying Alzheimer's disease: evidence from genome-wide association studies and beyond, Lancet Neurol., 15 (2016), 857-868. doi: 10.1016/S1474-4422(16)00127-7
![]() |
[12] | F. L. Heppner, R. M. Ransohoff, B. Becher, Immune attack: The role of inflammation in Alzheimer disease, Nature, 16 (2015), 358-372. |
[13] | Y. Yan, A. Zhao, Y. Qui, Y. Li, R. Yan, Y. Wang, et al., Genetic association of FERMT2, HLA-DRB1, CD2AP, and PTK2B polymorphisms with Alzheimer's disease risk in the southern Chinese population, Front. Aging Neurosci., 12 (2020), 16. |
[14] | V. Escott-Price, C. Bellenguez, L. S. Wang, S. H. Choi, D. Harold, L. Jones, et al., Gene-wide analysis detects two new susceptibility genes for Alzheimer's disease. PLoS One, 9 (2014), e94661. |
[15] | I. López González, P. Garcia-Esparcia, F. Llorens, I. Ferrer, Genetic and transcriptomic profiles of inflammation in neurodegenerative diseases: Alzheimer, Parkinson, Creutzfeldt-Jakob and Tauopathies, Int. J. Mol. Sci., 17 (2016), 206. |
[16] | Z. P. Van Acker, M. Bretou, Wim Annaert, Endo-lysosomal dysregulations and late-onset Alzheimer's disease: impact of genetic risk factors, Mol. Neurodegener., 14 (2019), 20. |
[17] | A. De Roeck, C. Van Broeckhoven, K. Sleegers, The role of ABCA7 in Alzheimer's disease: evidence from genomics, transcriptomics and methylomics, Acta Neuropathol., 138 (2019), 201-220. |
[18] |
C. Reitz, Novel susceptibility loci for Alzheimer's disease, Future Neurol., 10 (2015), 547-558. doi: 10.2217/fnl.15.42
![]() |
[19] | C. Bellenguez, C. Charbonnier, B. Grenier-Boley, O. Quenez, K. Le Guennec, G. Nicolas, et al., Contribution to Alzheimer's disease risk of rare variants in TREM2, SORL1, and ABCA7 in 1779 cases and 1273 controls, Neurobiol. Aging, 59 (2017), 220.e1-220.e9. |
[20] |
P. Ciryam, R. Kundra, R. Freer, R. I. Morimoto, C. M. Dobson, M. Vendruscolo, A transcriptional signature of Alzheimer's disease is associated with a metastable subproteome at risk for aggregation, Proc. Natl. Acad. Sci. USA, 113 (2016), 4753-4758. doi: 10.1073/pnas.1516604113
![]() |
[21] | L. V. Kalia, A. E. Lang, Parkinson's disease, Lancet, 386 (2015), 896-912. |
[22] | A. Masato, N. Plotegher, D. Boassa, L. Bubacco, Impaired dopamine metabolism in Parkinson's disease pathogenesis, Mol. Neurodegener., 14 (2019), 35. |
[23] |
M. G. Krokidis, Identification of biomarkers associated with Parkinson's disease by gene expression profiling studies and bioinformatics analysis, AIMS Neurosci., 6 (2019), 333-345. doi: 10.3934/Neuroscience.2019.4.333
![]() |
[24] | A. Grünewald, K. R. Kumar, C. M. Sue, New insights into the complex role of mitochondria in Parkinson's disease, Prog. Neurobiol., 177 (2019), 79-93. |
[25] | C. A. D. Costa, W. E. Manaa, E. Duplan, F. Checler, The endoplasmic reticulum stress/unfolded protein response and their contributions to Parkinson's disease physiopathology, Cells, 9 (2020), 2495. |
[26] | M. Deffains, M. H. Canron, M. Teil, Q. Li, B. Dehay, E. Bezard, et al., L-DOPA regulates α-synuclein accumulation in experimental parkinsonism, Neuropathol. Appl. Neurobiol., 2020. |
[27] |
L. P. Dolgacheva, A. V. Berezhnov, E. I. Fedotova, V. P. Zinchenko, A. Y. Abramov, Role of DJ-1 in the mechanism of pathogenesis of Parkinson's disease, J. Bioenerg. Biomembr., 51 (2019), 175-188. doi: 10.1007/s10863-019-09798-4
![]() |
[28] | A. Urbizu, K. Beyer, Epigenetics in Lewy body diseases: Impact on gene expression, utility as a biomarker, and possibilities for therapy, Int. J. Mol. Sci., 21 (2020), 4718. |
[29] | D. M. O'Hara, G. Pawar, S. K. Kalia, L. V. Kalia, LRRK2 and α-Synuclein: Distinct or synergistic players in Parkinson's disease?, Front. Neurosci., 14 (2020), 577. |
[30] | T. K. Lin, K. J. Lin, K. L. Lin, C. W. Liou, S. D. Chen, Y. C. Chuang, et al., When friendship turns sour: effective communication between mitochondria and intracellular organelles in Parkinson's disease, Front. Cell. Dev. Biol., 8 (2020), 607392. |
[31] | B. Wang, K. R. Stanford, M. Kundu, ER-to-Golgi trafficking and its implication in neurological diseases, Cells, 9 (2020), 408. |
[32] | T. M. Axelsen, D. P. D. Woldbye, Gene therapy for Parkinson's disease, an update, J. Parkinsons Dis., 8 (2018), 195-215. |
[33] | T. J. Collier, D. E. Jr. Redmond, K. Steece-Collier, J. W. Lipton, F. P. Manfredsson, Is a-synuclein loss-of-function a contributor to parkinsonian pathology? Evidence from non-human primates, Front. Neurosci., 10 (2016), 12. |
[34] | C. Klein, N. Hattori, C. Marras, MDSGene: Closing data gaps in genotype-phenotype correlations of monogenic Parkinson's disease, J. Parkinsons Dis., 8 (2018), S25-S30. |
[35] | J. Chi, Q. Xie, J. Jia, X. Liu, J. Sun, Y. Deng, L. Yi, Integrated analysis and identification of novel biomarkers in Parkinson's disease, Front. Aging Neurosci., 10 (2018), 178. |
[36] | Y. I. Li, G. Wong, J. Humphrey, T. Raj, Prioritizing Parkinson's disease genes using population-scale transcriptomic data, Nat. Commun., 10 (2019), 994. |
[37] |
K. J. Karczewski, M. P. Snyder, Integrative omics for health and disease, Nat. Rev. Genet., 19 (2018), 299-310. doi: 10.1038/nrg.2018.4
![]() |
[38] | B. Wang, V. Kumar, A. Olson, D. Ware, Reviving the transcriptome studies: an insight into the emergence of single-molecule transcriptome sequencing, Front. Genet., 10 (2019), 384. |
[39] | F. R. Pinu, S. A. Goldansaz, J. Jaine, Translational metabolomics: current challenges and future opportunities, Metabolites, 9 (2019), 108. |
[40] | J. L. Ren, A. H. Zhang, L. Kong, X. J. Wang, Advances in mass spectrometry-based metabolomics for investigation of metabolites, RSC Adv., 8 (2018), 22335. |
[41] | J. M. Wilkins, E. Trushina, Application of metabolomics in Alzheimer's disease, Front. Neurol., 8 (2018), 719. |
[42] | E. Trushina, T. Dutta, X. M. Persson, M. M. Mielke, R. C. Petersen, Identification of altered metabolic pathways in plasma and CSF in mild cognitive impairment and Alzheimer's disease using metabolomics, PLoS One, 8 (2013), e63644. |
[43] |
G. Paglia, M. Stocchero, S. Cacciatore, S. Lai, P. Angel, M. T. Alam, et al., Unbiased metabolomic investigation of Alzheimer's disease brain points to dysregulation of mitochondrial aspartate metabolism, J. Proteome Res., 15 (2016), 608-618. doi: 10.1021/acs.jproteome.5b01020
![]() |
[44] | S. G. Snowden, A. A. Ebshiana, A. Hye, Y. An, O. Pletnikova, R. O'Brien, et al., Association between fatty acid metabolism in the brain and Alzheimer disease neuropathology and cognitive performance: a nontargeted metabolomic study, PLoS Med., 14 (2017), e1002266. |
[45] |
S. P. Guiraud, I. Montoliu, L. Da Silva, L. Dayon, A. N. Galindo, J. Corthesy, et al., High-throughput and simultaneous quantitative analysis of homo-cysteine-methionine cycle metabolites and co-factors in blood plasma and cerebrospinal fluid by isotope dilution LC-MS/MS, Anal. Bioanal. Chem., 409 (2017), 295-305. doi: 10.1007/s00216-016-0003-1
![]() |
[46] |
J. B. Toledo, M. Arnold, G. Kastenmuller, R. Chang, R. A. Baillie, X. Han, et al., Metabolic network failures in Alzheimer's disease-a biochemical road map, Alzheimers Dement., 13 (2017), 965-84. doi: 10.1016/j.jalz.2017.01.020
![]() |
[47] | S. F. Graham, O. P. Chevallier, C. T. Elliott, C. Holscher, J. Johnston, B. McGuinness, et al., Untargeted metabolomic analysis of human plasma indicates differentially affected polyamine and l-arginine metabolism in mild cognitive impairment subjects converting to Alzheimer's disease, PLoS One, 10 (2015), e0119452. |
[48] |
M. Mapstone, F. Lin, M. A. Nalls, A. K. Cheema, A. B. Singleton, M. S. Fiandaca, et al., What success can teach us about failure: the plasma metabolome of older adults with superior memory and lessons for Alzheimer's disease, Neurobiol. Aging, 51 (2017), 148-55. doi: 10.1016/j.neurobiolaging.2016.11.007
![]() |
[49] |
M. Benson, Clinical implications of omics and systems medicine: focus on predictive and individualized treatment, J. Intern. Med., 279 (2016), 229-240. doi: 10.1111/joim.12412
![]() |
[50] |
C. Evans, J. Hardin, D. M. Stoebel, Selecting between-sample RNA-Seq normalization methods from the perspective of their assumptions, Brief Bioinform., 19 (2018), 776-792. doi: 10.1093/bib/bbx008
![]() |
[51] | F. Maleki, K. Ovens, D. J. Hogan, A. J. Kusalik, Gene set analysis: challenges, opportunities, and future research, Front. Genet., 11 (2020), 654. |
[52] | I. Ihnatova, V. Popovici, E. Budinska, A critical comparison of topology-based pathway analysis methods, PLoS One, 13 (2018), e0191154. |
[53] | N. Xie, L. Zhang, W. Gao, C. Huang, P. E. Huber, X. Zhou, et al., Subpathway-GM: identification of metabolic subpathways via joint power of interesting genes and metabolites and their topologies within pathways, Signal. Transduct. Target. Ther., 5 (2020), 227. |
[54] |
Q. Yang, S. Wang, E. Dai, S. Zhou, D. Liu, H. Liu, et al., Pathway enrichment analysis approach based on topological structure and updated annotation of pathway, Brief Bioinform., 20 (2019), 168-177. doi: 10.1093/bib/bbx091
![]() |
[55] |
P. Kong, P. Lei, S. Zhang, D. Li, J. Zhao, B. Zhang, Integrated microarray analysis provided a new insight of the pathogenesis of Parkinson's disease, Neurosci. Lett., 662 (2018), 51-58. doi: 10.1016/j.neulet.2017.09.051
![]() |
[56] | M. G. Krokidis, P. Vlamos, Transcriptomics in amyotrophic lateral sclerosis, Front. Biosci. (Elite Ed), 10 (2018), 103-121. |
[57] | L. Bravo-Merodio, L. A. Williams, G. V. Gkoutos, A. Acharjee, Omics biomarker identification pipeline for translational medicine. J. Transl. Med., 17 (2019), 155. |
[58] |
M. A. Myszczynska, P. N. Ojamies, A. M. B. Lacoste, D. Neil, A. Saffari, R. Mead, et al., Applications of machine learning to diagnosis and treatment of neurodegenerative diseases, Nat. Rev. Neurol., 16 (2020), 440-456. doi: 10.1038/s41582-020-0377-8
![]() |
[59] | J. Xin, X. Ren, L. Chen, Y. Wang, Identifying network biomarkers based on protein-protein interactions and expression data, BMC Med. Genomics, 8 (2015), S11. |
[60] |
L. Fu, K. Fu, Analysis of Parkinson's disease pathophysiology using an integrated genomics-bioinformatics approach, Pathophysiology, 22 (2015), 15-29. doi: 10.1016/j.pathophys.2014.10.002
![]() |
[61] | R. Al-Ouran, Y. W. Wan, C. G. Mangleburg, T. V. Lee, K. Allison, J. M. Shulman, et al., A portal to visualize transcriptome profiles in mouse models of neurological disorders, Genes (Basel), 10 (2019), E759. |
[62] | J. Kelly, R. Moyeed, C. Carroll, D. Albani, X. Li, Gene expression meta-analysis of Parkinson's disease and its relationship with Alzheimer's disease, Mol. Brain., 12 (2019), 16. |
[63] | E. Mariani, F. Frabetti, A. Tarozzi, M. C. Pelleri, F. Pizzetti, R. Casadei, Meta-analysis of Parkinson's disease transcriptome data using TRAM software: whole substantia nigra tissue and single dopamine neuron differential gene expression, PLoS One, 11 (2016), e0161567. |
[64] |
P. Kong, P. Lei, S. Zhang, D. Li, J. Zhao, B. Zhang, Integrated microarray analysis provided a new insight of the pathogenesis of Parkinson's disease, Neurosci. Lett., 662 (2018), 51-58. doi: 10.1016/j.neulet.2017.09.051
![]() |
[65] |
E. Glaab, Computational systems biology approaches for Parkinson's disease, Cell Tissue Res., 373 (2018), 91-109. doi: 10.1007/s00441-017-2734-5
![]() |
[66] | T. M. Nguyen, A. Shafi, T. Nguyen, S. Draghici, Identifying significantly impacted pathways: a comprehensive review and assessment, Genome Biol., 20 (2019), 203. |
[67] | J. Ma, A. Shojaie, G. Michailidis, A comparative study of topology-based pathway enrichment analysis methods, BMC Bioinform., 20 (2019), 546. |
[68] | C. Gao, H. Sun, T. Wang, M. Tang, N. I. Bohnen, M. L. T. M. Müller, et al., Model-based and model-free machine learning techniques for diagnostic prediction and classification of clinical outcomes in Parkinson's disease, Sci. Rep., 8 (2018), 7129. |
[69] |
Y. Hu, Z. Pan, Y. Hu, L. Zhang, J. Wang, Network and pathway-based analyses of genes associated with Parkinson's disease, Mol. Neurobiol., 54 (2017), 4452-4465. doi: 10.1007/s12035-016-9998-8
![]() |
1. | Marios G. Krokidis, Georgios N. Dimitrakopoulos, Aristidis G. Vrahatis, Christos Tzouvelekis, Dimitrios Drakoulis, Foteini Papavassileiou, Themis P. Exarchos, Panayiotis Vlamos, A Sensor-Based Perspective in Early-Stage Parkinson’s Disease: Current State and the Need for Machine Learning Processes, 2022, 22, 1424-8220, 409, 10.3390/s22020409 | |
2. | Marios G. Krokidis, Themis P. Exarchos, Panayiotis Vlamos, 2021, Chapter 57-1, 978-3-319-75479-6, 1, 10.1007/978-3-319-75479-6_57-1 | |
3. | Katerina Pierouli, Eleni Papakonstantinou, Louis Papageorgiou, Io Diakou, Thanasis Mitsis, Konstantina Dragoumani, Demetrios Spandidos, Flora Bacopoulou, George Chrousos, George Goulielmos, Elias Eliopoulos, Dimitrios Vlachakis, Role of non‑coding RNAs as biomarkers and the application of omics technologies in Alzheimer's disease (Review), 2022, 51, 1107-3756, 10.3892/ijmm.2022.5208 | |
4. | Marios G. Krokidis, Georgios Dimitrakopoulos, Aristidis G. Vrahatis, Themis P. Exarchos, Panagiotis Vlamos, 2021, Recent Dimensionality Reduction Techniques for Visualizing High-Dimensional Parkinson’s Disease Omics Data, 978-1-6654-3902-2, 4460, 10.1109/BigData52589.2021.9671736 | |
5. | Oscar Salvador Barrera-Vázquez, Elizabeth Sulvaran-Guel, Gibrán Pedraza-Vázquez, Juan Carlos Gomez-Verjan, 2022, Chapter 17, 978-3-030-89600-3, 189, 10.1007/978-3-030-89601-0_17 | |
6. | Marios G. Krokidis, Themis P. Exarchos, Panagiotis Vlamos, 2023, Chapter 57, 978-3-319-75921-0, 967, 10.1007/978-3-319-75922-7_57 | |
7. | Felipe G. Ravagnani, Hellen P. Valerio, Jersey H.S. Maués, Arthur N. de Oliveira, Renato D. Puga, Karina Griesi-Oliveira, Fabíola R. Picosse, Henrique B. Ferraz, Rodrigo R. Catharino, Graziella E. Ronsein, Patrícia de Carvalho Aguiar, Omics profile of iPSC-derived astrocytes from Progressive Supranuclear Palsy (PSP) patients, 2023, 116, 13538020, 105847, 10.1016/j.parkreldis.2023.105847 | |
8. | Sanchit Dhankhar, Somdutt Mujwar, Nitika Garg, Samrat Chauhan, Monika Saini, Prerna Sharma, Suresh Kumar, Satish Kumar Sharma, Mohammad Amjad Kamal, Nidhi Rani, Artificial Intelligence in The Management of Neurodegenerative Disorders, 2024, 23, 18715273, 931, 10.2174/0118715273266095231009092603 | |
9. | Beatriz Barros-Santos, Carlos Campos-Marques, Andreia Filipa Salvador, Joana Margarida Silva, Integration of omics to explore novel disease pathways in Down syndrome neurodegeneration – focusing on integrated stress response, 2025, 29499216, 10.1016/j.bosn.2025.04.006 |
Method type | Disease | Highlights | Reference |
Genomics and transcriptomics analysis | AD | new genes/loci associated with late-onset disease progression, (NME8, FERMT2, PTK2B, CASS4, DGS2) | Yan et al. [13] |
genome-wide association study | AD | new risk loci (FRMD4A, TP53INP1, IGHV1-67) | Escott-Price et al. [14] |
genome-wide association study | AD | late-onset AD associated loci (BIN1, CD2AP, PICALM, PLD3) | Van Acker et al. [16] |
genome-wide association study | AD | single nucleotide polymorphisms related to late-onset disease progression (ABCA7, CD2AP, CD33, PLD3 | De Roeck et al. [17] |
whole genome and whole exome sequencing | AD | complement response (CLU, BCA7, CD33, CD2AP, EPHA1), inflammatory cascade (EPHA1, INPP5D) | Reitz et al. [18] |
whole genome and whole exome sequencing | AD | new risk loci related to early-onset progression (SORL1, R4H7) | Bellenguez et al. [19] |
transcriptome-wide association studies | AD | accumulation of abnormal neuritic plaques, synaptic transmission, endocytic APP trafficking, immune response and apoptosis (CLU, PTK2B, and CR1) | Ciryam et al. [20] |
genome-wide association study | AD | PARK-SYNJ1, PARK-DNAJC6, PARK-FBXO7, CHCHD2, GBA | Klein et al. [34] |
meta-analysis of multiple gene expression arrays datasets | AD | MAPK8, CDC42, NDUFS1, COX4I1, SDHC and miR-126-5p, miR-19-3p, miR-29a-3p, lipid metabolism and mitochondrial dysregulation | Chi et al. [35] |
transcriptome-wide association study | AD | 66 risk genes with predicted expression or splicing levels (SNCA, CLASP2, TMEM175, GPNMB, CTSB, CAMLG, and NUDT14) | Li et al. [36] |
genome-wide association study | PD | familial autosomal dominant PD (a-synuclein, tau and LRRK2) | O'Hara et al. [29] |
transcriptomics/meta analysis brain samples | PD | signaling pathways and protein-protein interaction networks (REST as an upstream regulator) | Kelly et al. [62] |
transcriptomics/meta analysis brain samples | PD | changes in dopaminergic neuronal transcription, NPTX2, DEFA3, DEFA1 | Mariani et al. [63] |
frontal cortex metabolic profiling | AD | mitochondrial dysfunction, aspartate metabolism variation | Paglia et al. [43] |
brain tissue metabolic profiling | AD | dysregulation in the metabolism of unsaturated fatty acids | Snowden et al. [44] |
plasma and CSF metabolic profiling | AD | changes in the methionine cycle (homocysteine, S-adenosylmethionine, 5-methyltetrahydrofolic acid) | Guiraud et al. [45] |
plasma metabolic profiling | AD | alterations in sphingomyelins and ether-containing phosphatidylcholines | Toledo et al. [46] |
plasma metabolomics | AD | alterations in 3-hydroxypalmitoleylcarnitine and lyso phosphatidylcholines | Mapstone et al. [48] |
Method type | Disease | Highlights | Reference |
Genomics and transcriptomics analysis | AD | new genes/loci associated with late-onset disease progression, (NME8, FERMT2, PTK2B, CASS4, DGS2) | Yan et al. [13] |
genome-wide association study | AD | new risk loci (FRMD4A, TP53INP1, IGHV1-67) | Escott-Price et al. [14] |
genome-wide association study | AD | late-onset AD associated loci (BIN1, CD2AP, PICALM, PLD3) | Van Acker et al. [16] |
genome-wide association study | AD | single nucleotide polymorphisms related to late-onset disease progression (ABCA7, CD2AP, CD33, PLD3 | De Roeck et al. [17] |
whole genome and whole exome sequencing | AD | complement response (CLU, BCA7, CD33, CD2AP, EPHA1), inflammatory cascade (EPHA1, INPP5D) | Reitz et al. [18] |
whole genome and whole exome sequencing | AD | new risk loci related to early-onset progression (SORL1, R4H7) | Bellenguez et al. [19] |
transcriptome-wide association studies | AD | accumulation of abnormal neuritic plaques, synaptic transmission, endocytic APP trafficking, immune response and apoptosis (CLU, PTK2B, and CR1) | Ciryam et al. [20] |
genome-wide association study | AD | PARK-SYNJ1, PARK-DNAJC6, PARK-FBXO7, CHCHD2, GBA | Klein et al. [34] |
meta-analysis of multiple gene expression arrays datasets | AD | MAPK8, CDC42, NDUFS1, COX4I1, SDHC and miR-126-5p, miR-19-3p, miR-29a-3p, lipid metabolism and mitochondrial dysregulation | Chi et al. [35] |
transcriptome-wide association study | AD | 66 risk genes with predicted expression or splicing levels (SNCA, CLASP2, TMEM175, GPNMB, CTSB, CAMLG, and NUDT14) | Li et al. [36] |
genome-wide association study | PD | familial autosomal dominant PD (a-synuclein, tau and LRRK2) | O'Hara et al. [29] |
transcriptomics/meta analysis brain samples | PD | signaling pathways and protein-protein interaction networks (REST as an upstream regulator) | Kelly et al. [62] |
transcriptomics/meta analysis brain samples | PD | changes in dopaminergic neuronal transcription, NPTX2, DEFA3, DEFA1 | Mariani et al. [63] |
frontal cortex metabolic profiling | AD | mitochondrial dysfunction, aspartate metabolism variation | Paglia et al. [43] |
brain tissue metabolic profiling | AD | dysregulation in the metabolism of unsaturated fatty acids | Snowden et al. [44] |
plasma and CSF metabolic profiling | AD | changes in the methionine cycle (homocysteine, S-adenosylmethionine, 5-methyltetrahydrofolic acid) | Guiraud et al. [45] |
plasma metabolic profiling | AD | alterations in sphingomyelins and ether-containing phosphatidylcholines | Toledo et al. [46] |
plasma metabolomics | AD | alterations in 3-hydroxypalmitoleylcarnitine and lyso phosphatidylcholines | Mapstone et al. [48] |