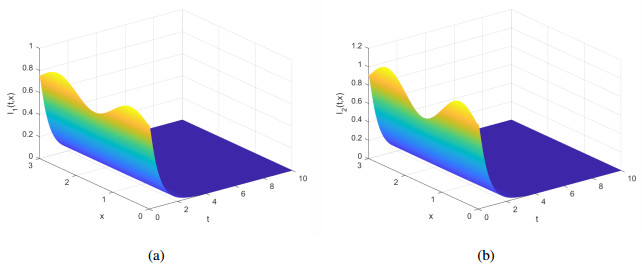
Citation: Pengyan Liu, Hong-Xu Li. Global behavior of a multi-group SEIR epidemic model with age structure and spatial diffusion[J]. Mathematical Biosciences and Engineering, 2020, 17(6): 7248-7273. doi: 10.3934/mbe.2020372
[1] | Zhisheng Shuai, P. van den Driessche . Impact of heterogeneity on the dynamics of an SEIR epidemic model. Mathematical Biosciences and Engineering, 2012, 9(2): 393-411. doi: 10.3934/mbe.2012.9.393 |
[2] | Junyuan Yang, Rui Xu, Xiaofeng Luo . Dynamical analysis of an age-structured multi-group SIVS epidemic model. Mathematical Biosciences and Engineering, 2019, 16(2): 636-666. doi: 10.3934/mbe.2019031 |
[3] | Cheng-Cheng Zhu, Jiang Zhu . Spread trend of COVID-19 epidemic outbreak in China: using exponential attractor method in a spatial heterogeneous SEIQR model. Mathematical Biosciences and Engineering, 2020, 17(4): 3062-3087. doi: 10.3934/mbe.2020174 |
[4] | Qiuyi Su, Jianhong Wu . Impact of variability of reproductive ageing and rate on childhood infectious disease prevention and control: insights from stage-structured population models. Mathematical Biosciences and Engineering, 2020, 17(6): 7671-7691. doi: 10.3934/mbe.2020390 |
[5] | Jinhu Xu, Yicang Zhou . Global stability of a multi-group model with vaccination age, distributed delay and random perturbation. Mathematical Biosciences and Engineering, 2015, 12(5): 1083-1106. doi: 10.3934/mbe.2015.12.1083 |
[6] | Cheng-Cheng Zhu, Jiang Zhu, Xiao-Lan Liu . Influence of spatial heterogeneous environment on long-term dynamics of a reaction-diffusion SVIR epidemic model with relaps. Mathematical Biosciences and Engineering, 2019, 16(5): 5897-5922. doi: 10.3934/mbe.2019295 |
[7] | Abdennasser Chekroun, Mohammed Nor Frioui, Toshikazu Kuniya, Tarik Mohammed Touaoula . Global stability of an age-structured epidemic model with general Lyapunov functional. Mathematical Biosciences and Engineering, 2019, 16(3): 1525-1553. doi: 10.3934/mbe.2019073 |
[8] | Gang Huang, Edoardo Beretta, Yasuhiro Takeuchi . Global stability for epidemic model with constant latency and infectious periods. Mathematical Biosciences and Engineering, 2012, 9(2): 297-312. doi: 10.3934/mbe.2012.9.297 |
[9] | Xichao Duan, Sanling Yuan, Kaifa Wang . Dynamics of a diffusive age-structured HBV model with saturating incidence. Mathematical Biosciences and Engineering, 2016, 13(5): 935-968. doi: 10.3934/mbe.2016024 |
[10] | Jinliang Wang, Hongying Shu . Global analysis on a class of multi-group SEIR model with latency and relapse. Mathematical Biosciences and Engineering, 2016, 13(1): 209-225. doi: 10.3934/mbe.2016.13.209 |
Since the pioneering work of Kermack and McKendrick [1], many mathematical models have been proposed attempting to gain a better understanding of disease transmission, especially for the control strategy and dynamical behavior of infectious diseases [2,3,4,5,6,7,8]. Simple models with assumption that individuals are well mixed, which implies each individual has the same probability to be infected, are beneficial in that one can obtain analytical results easily but may be lack of realism. Epidemic models with population structures, like age, sex and patch (such as communities, cities, or counties), may be a more realistic way to describe complex disease dynamics. As a matter of fact, the total population should be classified into different groups and the vital epidemic parameters should vary among different population groups. In addition, at different age stages, the effects of infectious transmission are various, which is another important and key factor that needs necessarily to be included in modeling this infectious transmission process. Thus, considering multi-group and age structure in epidemic models is very necessary and reasonable. Some recent developments on the transmission dynamics of multi-group and age structured epidemic models have been discussed in [8,9,10,11,12,13].
Since that the population distribute heterogeneously in different spatial location in the real life and they will move or diffuse for many reasons, in epidemiology, there is increasing evidence that environmental heterogeneity and individual motility have significant impact on the spread of infectious diseases [14,15]. In recent years, global behavior of spatial diffusion systems, which are suitable for diseases such as the rabies and the Black Death, has been attracted extensive attention of researchers and has been one of the hot topics [16,17,18,19,20,21,22,23,24,25]. Among these works, few take age structure or multi-group into consideration. Yang et al. [24] proposed a novel model incorporated with both age-since-infection and spacial diffusion of brucellosis infection, and the basic reproduction number and global behaviors of this system were completely investigated. Fitzgibbon et al. [19] considered a diffusive epidemic model with age structure where the disease spreads between vector and host populations. Then, the existence of solutions of the model was studied based on semigroup theory and the asymptotic behavior of the solution was analyzed. Luo et al. [21] incorporated spatial heterogeneity in n-group reaction-diffusion SIR model with nonlinear incidence rate to investigate the global dynamics of the disease-free and endemic steady states for this model. Zhao et al. [25] modeled host heterogeneity by introducing multi-group structure in a time delay SIR epidemic model and showed that basic reproduction number determines the existence of traveling waves of this system. To determine how age structure, multi-group population and diffusion of individuals affect the consequences of epidemiological processes, Ducrot et al. [18] formulated a multi-group age-structured epidemic model with the classical Fickian diffusion and studied the existence of travelling wave solutions for this model.
To the best of our knowledge, epidemic models established by researchers except for [18] only include one or two characteristics of multi-group, age structure and spatial diffusion. All the three characteristics are incorporated into epidemic model in [18], however, this model does not include the class of latent individuals. For some epidemic diseases like malaria, HIV/AIDS and West Nile virus, latent individuals may take days, months, or even years to become infectious. Moreover, the travel of latent individuals showing no symptoms can spread the disease geographically which makes disease harder to control. Motivated by the above discussion, in this paper, we investigate a diffusive version of multi-group epidemic system with age structure which is generalization of the model studied in [26] for the first time to allow for individuals moving around on the spatial habitat x∈Ω⊂Rn with smooth boundary ∂Ω. The organization of this paper is as follows. Firstly, we present our model in the next section. In section 3, some preliminaries including the positivity, boundedness, existence and uniqueness of solution, and the existence of compact global attractor of the associated solution semiflow, are established. In section 4, the sufficient conditions on the existence and global stability of disease-free and endemic steady states are stated and proved. In section 5, we conduct numerical simulations to illustrate the validity of our theoretical results. In section 6, a brief conclusion is given.
In 2015, Liu et al. [26] introduced age-of-latent and age-of-relapse into epidemic model which is appropriate for diseases such as tuberculosis and herpes virus infection. For these diseases, latent individuals may take days, months, or even years to become infectious and the treatment efficacy may decline with time for recovered individuals then cause recurrence of disease. In order to study the global dynamics o for these diseases, they formulated the following SEIR epidemic system with continuous age-dependent latency and relapse
{dS(t)dt=Λ−μS(t)−βS(t)I(t),∂e(t,a)∂t+∂e(t,a)∂a=−σ(a)e(t,a)−(μ+δ1)e(t,a),dI(t)dt=∫∞0σ(a)e(t,a)da−(μ+δ2+c)I(t)+∫∞0γ(b)r(t,b)db,∂r(t,b)∂t+∂r(t,b)∂b=−γ(b)r(t,b)−μr(t,b),e(t,0)=βS(t)I(t),r(t,0)=cI(t), | (2.1) |
for t≥0 and with initial conditions
{S(0)=S0>0,e(0,a)=e0(a)∈L1+(0,+∞),I(0)=I0>0,r(0,b)=r0(b)∈L1+(0,+∞), |
for a,b≥0, where L1+(0,+∞) is the space of functions on (0,+∞) that are nonnegative and Lebesgue integrable. At time t, the densities of susceptible individuals, latent individuals with latent age a, infectious individuals and removed individuals with relapse age b are denoted by S(t), e(t,a), I(t), r(t,b), respectively. σ(a) and γ(b) denote the conversional rate from the latent class and the relapse rate in the removed class, which depend on age a and age b, respectively. Furthermore, β is the transmission rate of the disease between susceptible and infectious individuals, Λ is the density of the recruitment into the susceptible class (including the births and immigration), μ is the natural death rate of all individuals, δ1 and δ2 are the additional death rate induced by the infectious diseases, and c is the recovery rate from the infectious class. All parameters are assumed to be positive.
It is clear that the variations of different epidemic parameters between or within different groups can be well realized according to the description of multi-group epidemic models. Hence, Liu and Feng [27] extended model (2.1) to the situation in which the population is divided into n groups according to different contact patterns and derived the following multi-group SEIR epidemic model
{dSk(t)dt=Λk−μkSk(t)−n∑j=1βkjSk(t)Ij(t),∂ek(t,a)∂t+∂ek(t,a)∂a=−σk(a)ek(t,a)−(μk+δ1k)ek(t,a),dIk(t)dt=∫∞0σk(a)ek(t,a)da−(μk+δ2k+ck)Ik(t)+∫∞0γk(b)rk(t,b)db,∂rk(t,b)∂t+∂rk(t,b)∂b=−γk(b)rk(t,b)−μkrk(t,b),ek(t,0)=n∑j=1βkjSk(t)Ij(t),rk(t,0)=ckIk(t), | (2.2) |
for t≥0 and with initial conditions
{Sk(0)=S0k>0,ek(0,a)=e0k(a)∈L1+(0,+∞),Ik(0)=I0k>0,rk(0,b)=r0k(b)∈L1+(0,+∞), |
for a,b>0. Λk, μk and ck denote the recruitment rate of the susceptible class, the per-capita natural death rate and the recovery rate from the infectious class in group k, respectively. βkj denotes the transmission rate of the disease between susceptible individuals in group k and infectious individuals in group j. δ1k and δ2k denote the additional death rates of exposed and infectious individuals induced by the infectious diseases in group k, respectively. σk(a) denotes the conversional rate from the latent class in group k, which depends on age a and γk(b) denotes the relapse rate from the removed class into the infectious class in group k, which depends on age b.
Spatial diffusion is an intrinsic characteristic for investigating the roles of spatial heterogeneity on diseases mechanisms and transmission routes and can lead to rich dynamics. Based on this fact, we generalize (2.2) by taking account of the case that individuals move or diffuse around on the spatial habitat x∈Ω⊂Rn with smooth boundary ∂Ω. Let Sk(t,x) and Ik(t,x) be the densities of susceptible individuals and infectious individuals at time t and location x∈Ω in group k, respectively, where the habitat Ω is bounded and connected. And let ek(t,a,x) and rk(t,b,x) denote the densities of individuals in the latent class with age a and the removed class with age b at time t and location x in group k, respectively. Hence, the n-group diffusive SEIR epidemic model with age-dependent latent and relapse has the following form
{∂Sk(t,x)∂t=d1k△Sk(t,x)+Λk−μkSk(t,x)−n∑j=1βkjSk(t,x)Ij(t,x),∂ek(t,a,x)∂t+∂ek(t,a,x)∂a=d2k△ek(t,a,x)−σk(a)ek(t,a,x)−(μk+δ1k)×ek(t,a,x),∂Ik(t,x)∂t=d3k△Ik(t,x)+∫∞0σk(a)ek(t,a,x)da−(μk+δ2k+ck)Ik(t,x)+∫∞0γk(b)rk(t,b,x)db,∂rk(t,b,x)∂t+∂rk(t,b,x)∂b=d4k△rk(t,b,x)−γk(b)rk(t,b,x)−μkrk(t,b,x),ek(t,0,x)=n∑j=1βkjSk(t,x)Ij(t,x),rk(t,0,x)=ckIk(t,x), | (2.3) |
for x∈Ω, a,b∈R+=(0,+∞), with the homogeneous Neumann boundary conditions
∂Sk(t,x)∂ν=∂ek(t,a,x)∂ν=∂Ik(t,x)∂ν=∂rk(t,b,x)∂ν=0,x∈∂Ω, |
and initial functions
Sk(0,x)=S0k(x),ek(0,a,x)=e0k(a,x),Ik(0,x)=I0k(x),rk(0,b,x)=r0k(b,x). |
d1k, d2k, d3k, d4k denote the diffusion coefficients of susceptible individuals, exposed individuals, infectious individuals and removed individuals in group k, respectively. And the other parameters have the same biological meanings as in (2.2). The homogeneous Neumann boundary conditions imply that there is no population flux across the boundary ∂Ω.
We define the functional spaces X=C(ˉΩ,R) and Y=L1(R+,X) for model (2.3) equipped, respectively, with the norms
|ϕ|X=supx∈ˉΩ|ϕ(x)|,‖φ‖Y=∫∞0|φ(a,⋅)|Xda, |
for ϕ∈X, φ∈Y. The positive cones are denoted by X+ and Y+. In addition, we define a vector space Z=(C([0,T],X))2n with the norm
‖ψ‖Z=maxisup0≤t≤T|ψi(t,⋅)|X,ψ=(ψ1,ψ2,...,ψ2n)∈Z. |
Throughout this paper, for convenience, we always denote S=(S1,S2,...,Sn), e=(e1,e2,...,en), I=(I1,I2,...,In), r=(r1,r2,...,rn), and S0=(S01,S02,...,S0n), e0=(e01,e02,...,e0n), I0=(I01,I02,...,I0n), r0=(r01,r02,...,r0n). We also denote (y1,y2,...,yn)T>(z1,z2,...,zn)T as yi>zi for all i=1,2,...,n. For each i=1,2,3,4, we suppose that Tik:C(ˉΩ,R)→C(ˉΩ,R) is the C0 semigroup generated by dikΔ subjects to the Neumann boundary condition in group k. From subsection 2.1 in [28], we have
(Tik(t)[ϕ])(x)=∫ΩΓik(t,x,y)ϕ(y)dy, |
for all t>0 and ϕ∈C(ˉΩ,R), where Γik(t,x,y) is the Green function. We have that Tik, i=1,2,3,4, k=1,2,...,n are compact and strongly positive for each t>0 by the Corollary 7.2.3 in [29]. Integrating the second equation in model (2.3) along the characteristic line t−a=c, where c is a constant, we obtain
ek(t,a,x)={∫ΩΓ2k(a,x,y)ek(t−a,0,y)dyπ1k(a),t≥a,∫ΩΓ2k(a,x,y)e0k(a−t,y)dyπ1k(a)π1k(a−t),t<a, | (2.4) |
where π1k(a)=e−∫a0[μk+δ1k+σk(s)]ds. Similarly,
rk(t,b,x)={∫ΩΓ4k(b,x,y)rk(t−b,0,y)dyπ2k(b),t≥b,∫ΩΓ4k(b,x,y)r0k(b−t,y)dyπ2k(b)π2k(b−t),t<b, | (2.5) |
where π2k(b)=e−∫b0[μk+γk(s)]ds. To study the asymptotic behaviors of the dynamics of model (2.3), we require the following assumptions on the model parameters.
Assumption 2.1. For each k,j=1,2,...,n,
(H1)d1k,d2k,d3k,d4k,Λk,μk,δ1k,δ2k,ck>0.
(H2)βkj∈R+, and the n-dimensional square matrix (βkj)n×n is irreducible.
(H3)σk(⋅),γk(⋅)∈L∞(R+,R+), ˉσk>0 and ˉγk>0, where ˉσk:=esssupa∈R+σk(a), ˉγk:=esssupb∈R+γk(b).
We define Ak(t,x)=ek(t,0,x), Bk(t,x)=rk(t,0,x) for (t,x)∈R+×Ω, and let A=(A1,A2,...,An), B=(B1,B2,...,Bn) and C=(A,B). Positiveness of the solutions of model (2.3) is given below.
Theorem 3.1. Suppose that there exists a solution (S(t,⋅),e(t,⋅,⋅),I(t,⋅),r(t,⋅,⋅))∈Xn×Yn×Xn×Yn of (2.3) corresponding to (S0,e0,I0,r0)∈Xn+×Yn+×Xn+×Yn+ with an interval of existence [0,T], T>0. Then
(S(t,⋅),e(t,⋅,⋅),I(t,⋅),r(t,⋅,⋅))∈Xn+×Yn+×Xn+×Yn+, |
for all t∈[0,T].
Proof. From the first equation of (2.3), we have
Sk(t,x)=ˆFSk(t,x)+∫t0e−∫ta[μk+n∑j=1βkjIj(τ,x)]dτΛk∫ΩΓ1k(t−a,x,y)dyda, |
where ˆFSk(t,x)=e−∫t0[μk+n∑j=1βkjIj(τ,x)]dτ∫ΩΓ1k(t,x,y)S0k(y)dy. The positivity of Λk and S0k ensures Sk(t,x)>0 for each (t,x)∈[0,T]×Ω. The positivity of C which means the positivity for Ak and Bk, k=1,2,...,n is established by constructing Picard sequences as follows.
Solving equation Ik for system (2.3), we have
Ik(t,x)=FIk(t,x)+∫t0e−(μk+δ2k+ck)(t−a)∫ΩΓ3k(t−a,x,y)×[∫∞0σk(b)ek(a,b,y)db+∫∞0γk(b)rk(a,b,y)db]dyda, | (3.1) |
where FIk(t,x)=e−(μk+δ2k+ck)t∫ΩΓ3k(t,x,y)I0k(y)dy. For (t,x)∈[0,T]×Ω, by (2.4) and (2.5), we obtain
∫∞0σk(b)ek(t,b,y)db=FAk(t,y)+∫t0σk(b)π1k(b)∫ΩΓ2k(b,y,z)Ak(t−b,z)dzdb, | (3.2) |
where FAk(t,y)=∫∞0σk(b+t)π1k(b+t)π1k(b)∫ΩΓ2k(b+t,y,z)e0k(b,z)dzdb, and
∫∞0γk(b)rk(t,b,y)db=FBk(t,y)+∫t0γk(b)π2k(b)∫ΩΓ4k(b,y,z)Bk(t−b,z)dzdb, | (3.3) |
where FBk(t,y)=∫∞0γk(b+t)π2k(b+t)π2k(b)∫ΩΓ4k(b+t,y,z)r0k(b,z)dzdb. From (3.1)–(3.3) and the definitions of Ak and Bk, we have
Ak(t,x)=n∑j=1βkjSk(t,x){FIj(t,x)+∫t0e−(μj+δ2j+cj)(t−a)∫ΩΓ3j(t−a,x,y)[FAj(a,y)+∫a0σj(b)π1j(b)∫ΩΓ2j(b,y,z)Aj(a−b,z)dzdb+FBj(a,y)+∫a0γj(b)π2j(b)×∫ΩΓ4j(b,y,z)Bj(a−b,z)dzdb]dyda}, |
and
Bk(t,x)=ck{FIk(t,x)+∫t0e−(μk+δ2k+ck)(t−a)∫ΩΓ3k(t−a,x,y)[FAk(a,y)+∫a0σk(b)×π1k(b)∫ΩΓ2k(b,y,z)Ak(a−b,z)dzdb+FBk(a,y)+∫a0γk(b)π2k(b)×∫ΩΓ4k(b,y,z)Bk(a−b,z)dzdb]dyda}. |
Let
A(0)k(t,x)=n∑j=1βkjSk(t,x){FIj(t,x)+∫t0e−(μj+δ2j+cj)(t−a)∫ΩΓ3j(t−a,x,y)×[FAj(a,y)+FBj(a,y)]dyda}, |
and
B(0)k(t,x)=ck{FIk(t,x)+∫t0e−(μk+δ2k+ck)(t−a)∫ΩΓ3k(t−a,x,y)×[FAk(a,y)+FBk(a,y)]dyda}. |
Then it is obvious that A(0)k(t,x)>0, B(0)k(t,x)>0. Now we assume that A(m)k(t,x)>0, B(m)k(t,x)>0 (m∈N) for e0k>0, r0k>0 and (t,x)∈[0,T]×Ω. Then
A(m+1)k(t,x)=A(0)k(t,x)+n∑j=1βkjSk(t,x){∫t0e−(μj+δ2j+cj)(t−a)∫ΩΓ3j(t−a,x,y)[∫a0σj(b)×π1j(b)∫ΩΓ2j(b,y,z)A(m)j(a−b,z)dzdb+∫a0γj(b)π2j(b)∫ΩΓ4j(b,y,z)×B(m)j(a−b,z)dzdb]dyda}, |
and
B(m+1)k(t,x)=B(0)k(t,x)+ck{∫t0e−(μk+δ2k+ck)(t−a)∫ΩΓ3k(t−a,x,y)[∫a0σk(b)π1k(b)×∫ΩΓ2k(b,y,z)A(m)k(a−b,z)dzdb+∫a0γk(b)π2k(b)∫ΩΓ4k(b,y,z)×B(m)k(a−b,z)dzdb]dyda}. |
From the positivity of βkj, σk and γk, together with the positivity of Γ2k, Γ3k and Γ4k, it follows that
A(1)k(t,x)−A(0)k(t,x)=n∑j=1βkjSk(t,x){∫t0e−(μj+δ2j+cj)(t−a)∫ΩΓ3j(t−a,x,y)[∫a0σj(b)×π1j(b)∫ΩΓ2j(b,y,z)A(0)j(a−b,z)dzdb+∫a0γj(b)π2j(b)∫ΩΓ4j(b,y,z)×B(0)j(a−b,z)dzdb]dyda}>0, |
and
B(1)k(t,x)−B(0)k(t,x)=ck{∫t0e−(μk+δ2k+ck)(t−a)∫ΩΓ3k(t−a,x,y)[∫a0σk(b)π1k(b)∫ΩΓ2k(b,y,z)×A(0)k(a−b,z)dzdb+∫a0γk(b)π2k(b)∫ΩΓ4k(b,y,z)B(0)k(a−b,z)dzdb]dyda}>0, |
which lead to C(1)(t,x)−C(0)(t,x)>0 for (t,x)∈[0,T]×Ω. We assume that C(m)(t,x)−C(m−1)(t,x)>0 for all m≥2, that is, A(m)k(t,x)−A(m−1)k(t,x)>0 and B(m)k(t,x)−B(m−1)k(t,x)>0, k=1,2,...,n. Then,
A(m+1)k(t,x)−A(m)k(t,x)=n∑j=1βkjSk(t,x){∫t0e−(μj+δ2j+cj)(t−a)∫ΩΓ3j(t−a,x,y)(∫a0σj(b)×π1j(b)∫ΩΓ2j(b,y,z)[A(m)j(a−b,z)−A(m−1)j(a−b,z)]dzdb+∫a0γj(b)×π2j(b)∫ΩΓ4j(b,y,z)[B(m)j(a−b,z)−B(m−1)j(a−b,z)]dzdb)dyda}>0, |
and
B(m+1)k(t,x)−B(m)k(t,x)=ck{∫t0e−(μk+δ2k+ck)(t−a)∫ΩΓ3k(t−a,x,y)(∫a0σk(b)π1k(b)∫ΩΓ2k(b,y,z)×[A(m)k(a−b,z)−A(m−1)k(a−b,z)]dzdb+∫a0γk(b)π2k(b)∫ΩΓ4k(b,y,z)×[B(m)k(a−b,z)−B(m−1)k(a−b,z)]dzdb)dyda}>0. |
Hence applying mathematical induction, we show that the sequence {C(m)}∞0 is monotonically increasing.
Next, applying the contraction mapping principle, we show the sequence {C(m)}∞0 converges to C(t,x) for any (t,x)∈[0,T]×Ω as m approaches infinity. To this end, we define a variable
ˆC(m)(t,x)=e−λtC(m)(t,x),for someλ∈R+. |
By the definitions of A(m)k and B(m)k, we have
ˆA(m+1)k(t,x)=e−λtA(0)k(t,x)+n∑j=1βkjSk(t,x){∫t0e−(μj+δ2j+cj)a∫ΩΓ3j(a,x,y)[∫t−a0σj(b)×π1j(b)∫ΩΓ2j(b,y,z)e−λ(a+b)ˆA(m)j(t−a−b,z)dzdb+∫t−a0γj(b)π2j(b)×∫ΩΓ4j(b,y,z)e−λ(a+b)ˆB(m)j(t−a−b,z)dzdb]dyda}, |
and
ˆB(m+1)k(t,x)=e−λtB(0)k(t,x)+ck{∫t0e−(μk+δ2k+ck)a∫ΩΓ3k(a,x,y)[∫t−a0σk(b)π1k(b)×∫ΩΓ2k(b,y,z)e−λ(a+b)ˆA(m)k(t−a−b,z)dzdb+∫t−a0γk(b)π2k(b)×∫ΩΓ4k(b,y,z)e−λ(a+b)ˆB(m)k(t−a−b,z)dzdb]dyda}. |
For any m∈N,
ˆA(m+1)k−ˆA(m)k≤n∑j=1βkjˆSk{∫t0e−(μj+δ2j+cj)(a)∫ΩΓ3j(a,x,y)[∫t−a0σj(b)π1j(b)∫ΩΓ2j(b,y,z)×e−λ(a+b)dzdb+∫t−a0γj(b)π2j(b)∫ΩΓ4j(b,y,z)e−λ(a+b)dzdb]dyda}×‖ˆC(m)−ˆC(m−1)‖Z≤n∑j=1βkjˆSk(ˉσj+ˉγj)λ2‖ˆC(m)−ˆC(m−1)‖Z, |
where ˆSk=maxt∈[0,T]|Sk(t,⋅)|X, and
ˆB(m+1)k−ˆB(m)k≤ck{∫t0e−(μk+δ2k+ck)a∫ΩΓ3k(a,x,y)[∫t−a0σk(b)π1k(b)∫ΩΓ2k(b,y,z)×e−λ(a+b)dzdb+∫t−a0γk(b)π2k(b)∫ΩΓ4k(b,y,z)e−λ(a+b)dzdb]dyda}×‖ˆC(m)−ˆC(m−1)‖Z≤ck(ˉσk+ˉγk)λ2‖ˆC(m)−ˆC(m−1)‖Z. |
Hence,
‖ˆC(m+1)−ˆC(m)‖Z≤Kλ‖ˆC(m)−ˆC(m−1)‖Z≤Kmλ‖ˆC(1)−ˆC(0)‖Z, |
where Kλ=max{Mλ,Nλ}, Mλ=maxk{n∑j=1βkjˆSk(ˉσj+ˉγj)λ2}, Nλ=maxk{ck(ˉσk+ˉγk)λ2}. Therefore, for any m1>m2, m1,m2∈N,
‖ˆC(m1)−ˆC(m2)‖Z≤Km1λ1−Kλ‖ˆC(1)−ˆC(0)‖Z. |
We choose λ sufficiently large such that n∑j=1βkjˆSk(ˉσj+ˉγj)λ2<1 and ck(ˉσk+ˉγk)λ2<1 for all k=1,2,...,n, then Kλ<1. Hence, ‖ˆC(m1)−ˆC(m2)‖Z→0 as m2→∞ which implies that ˆC(m)→ˆC and thus C(m)→C as m→∞. Furthermore, we have A(m)k→Ak and B(m)k→Bk for k=1,2,...,n as m→∞. Since sequence {C(m)}∞0 is monotonically increasing, we obtain Ak and Bk are positive for k=1,2,...,n.
By (2.4) and (2.5), together with the positivity of e0k, r0k, Ak and Bk, we conclude that ek(t,a,x) and rk(t,a,x) are positive. For the positivity of Ik, we prove this by contradiction. Suppose that there exist x0∈Ω and t0=inf{t∈R+|Ik(t,x0)=0} such that
Ik(t0,x0)=0,Ik(t,x0)>0,∂Ik(t0,x0)∂t≤0,t∈[0,t0). |
By the third equation of system (2.3), we can easily obtain
∂Ik(t0,x0)∂t=FAk(t0,x0)+FBk(t0,x0)+∫t00σk(a)π1k(a)∫ΩΓ2k(a,x0,y)Ak(t0−a,y)dyda+∫t00γk(a)π2k(a)∫ΩΓ4k(a,x0,y)Bk(t0−a,y)dyda>0. |
This leads to a contradiction. Hence, for any t∈[0,T], we have (S(t,⋅),e(t,⋅,⋅),I(t,⋅),r(t,⋅,⋅))∈Xn+×Yn+×Xn+×Yn+.
Let Nk(t)=∫Ω[Sk(t,x)+∫∞0ek(t,a,x)da+Ik(t,x)+∫∞0rk(t,b,x)db]dx denotes the total population at time t in group k and region Ω.
Theorem 3.2. If
lima→+∞ek(t,a,x)=0,limb→+∞rk(t,b,x)=0, | (3.4) |
for all t>0, x∈Ω, the region Π defined by
Π={(Sk,ek,Ik,rk)|Nk≤Λkμk|Ω|}, |
is positively invariant for system (2.3).
Proof. Following condition (3.4) and the equations of system (2.3), we have
∂Sk(t,x)∂t+∫∞0∂ek(t,a,x)∂tda+∂Ik(t,x)∂t+∫∞0∂rk(t,b,x)∂tdb=d1k△Sk(t,x)+d2k∫∞0△ek(t,a,x)da+d3k△Ik(t,x)+d4k∫∞0△rk(t,b,x)da+Λk−μkSk(t,x)−n∑j=1βkjSk(t,x)Ij(t,x)−∫∞0∂ek(t,a,x)∂ada−∫∞0σk(a)×ek(t,a,x)da−∫∞0(μk+δ1k)ek(t,a,x)da+∫∞0σk(a)ek(t,a,x)da−(μk+δ2k+ck)Ik(t,x)+∫∞0γk(b)rk(t,b,x)db−∫∞0∂rk(t,b,x)∂bdb−∫∞0γk(b)rk(t,b,x)db−∫∞0μkrk(t,b,x)db=d1k△Sk(t,x)+d2k∫∞0△ek(t,a,x)da+d3k△Ik(t,x)+d4k∫∞0△rk(t,b,x)da+Λk−μkSk(t,x)−∫∞0(μk+δ1k)ek(t,a,x)da−(μk+δ2k)Ik(t,x)−∫∞0μkrk(t,b,x)db<d1k△Sk(t,x)+d2k∫∞0△ek(t,a,x)da+d3k△Ik(t,x)+d4k∫∞0△rk(t,b,x)da+Λk−μkSk(t,x)−μk∫∞0ek(t,a,x)da−μkIk(t,x)−μk∫∞0rk(t,b,x)db. |
Noting the Neumann boundary conditions of system (2.3) and using the Gauss formula, we derive
∫Ωd1k△Sk(t,x)dx=∫Ω∫∞0d2k△ek(t,a,x)dadx=∫Ωd3k△Ik(t,x)dx=∫Ω∫∞0d4k△rk(t,b,x)dadx=0. |
It follows that
dNk(t)dt=∫Ω[∂Sk(t,x)∂t+∫∞0∂ek(t,a,x)∂tda+∂Ik(t,x)∂t+∫∞0∂rk(t,b,x)∂tdb]dx<∫Ω{Λk−μk[Sk(t,x)+∫∞0ek(t,a,x)da+Ik(t,x)+∫∞0rk(t,b,x)db]}dx=Λk|Ω|−μkNk(t). |
Thus if Nk(t)>Λkμk|Ω|, then dNk(t)dt<0. Moreover, we observe the ordinary differential equation
dNk(t)dt=Λk|Ω|−μkNk(t), |
with general solution
Nk(t)=Λkμk|Ω|+[Nk(0)−Λkμk|Ω|]e−μkt, |
where Nk(0) means the initial value of total population in group k and region Ω. By applying the standard comparison theorem, we have for all t≥0,
Nk(t)≤Λkμk|Ω|,ifNk(0)≤Λkμk|Ω|. |
Hence, Π is positive invariant for system (2.3).
The existence and uniqueness of the solution of model (2.3) follow from Banach-Picard fixed point theorem.
Theorem 3.3. Let initial functions satisfy (S0,e0,I0,r0)∈Xn+×Yn+×Xn+×Yn+. Then the system (2.3) has a unique solution (S(t,⋅),e(t,⋅,⋅),I(t,⋅),r(t,⋅,⋅))∈Xn+×Yn+×Xn+×Yn+ for t∈[0,T].
Proof. Solving equation Sk for system (2.3), we have
Sk(t,x)=FSk(t,x)+∫t0e−μk(t−a)∫ΩΓ1k(t−a,x,y)[Λk−Ak(a,y)]dyda, | (3.5) |
for (t,x)∈[0,T]×Ω, where FSk(t,x)=e−μkt∫ΩΓ1k(t,x,y)S0k(y)dy. From (3.1)–(3.5) and the definitions of Ak and Bk, we have
Ak(t,x)=n∑j=1βkj{FSk(t,x)+∫t0e−μk(t−a)∫ΩΓ1k(t−a,x,y)[Λk−Ak(a,y)]dyda}×{FIj(t,x)+∫t0e−(μj+δ2j+cj)(t−a)∫ΩΓ3j(t−a,x,y)[FAj(a,y)+∫a0σj(b)×π1j(b)∫ΩΓ2j(b,y,z)Aj(a−b,z)dzdb+FBj(a,y)+∫a0γj(b)π2j(b)×∫ΩΓ4j(b,y,z)Bj(a−b,z)dzdb]dyda}:=F1k[C](t,x), | (3.6) |
and
Bk(t,x)=ck{FIk(t,x)+∫t0e−(μk+δ2k+ck)(t−a)∫ΩΓ3k(t−a,x,y)[FAk(a,y)+∫a0σk(b)×π1k(b)∫ΩΓ2k(b,y,z)Ak(a−b,z)dzdb+FBk(a,y)+∫a0γk(b)π2k(b)×∫ΩΓ4k(b,y,z)Bk(a−b,z)dzdb]dyda}:=F2k[C](t,x), | (3.7) |
where F1k, F2k:Z→C([0,T],X) are nonlinear operators for each k=1,2,...,n. For the sake of convenience, we define for each (t,x)∈[0,T]×Ω,
FCk(t,x)=FSk+∫t0e−μk(t−a)∫ΩΓ1k(t−a,x,y)Λkdyda,FDk(t,x)=FIk(t,x)+∫t0e−(μk+δ2k+ck)(t−a)∫ΩΓ3k(t−a,x,y)[FAk(a,y)+FBk(a,y)]dyda,Θ1(Ak)=∫t0e−μk(t−a)∫ΩΓ1k(t−a,x,y)Ak(a,y)dyda,Θ2(Ak)=∫t0e−(μk+δ2k+ck)(t−a)∫ΩΓ3k(t−a,x,y)∫a0σk(b)π1k(b)×∫ΩΓ2k(b,y,z)Ak(a−b,z)dzdbdyda,Θ3(Bk)=∫t0e−(μk+δ2k+ck)(t−a)∫ΩΓ3k(t−a,x,y)∫a0γk(b)π2k(b)×∫ΩΓ4k(b,y,z)Bk(a−b,z)dzdbdyda. |
Then
F1k[C]=n∑j=1βkj[FCk−Θ1(Ak)][FDj+Θ2(Aj)+Θ3(Bj)],F2k[C]=ck[FDk+Θ2(Ak)+Θ3(Bk)]. |
For any C, ˉC∈Z, we set ˜C=C−ˉC. Then, from the positivity of Ak and Bk proved in Theorem 3.1, we have
F1k[C]−F1k[ˉC]=n∑j=1βkj{FCk[Θ2(˜Aj)+Θ3(˜Bj)]−FDjΘ1(˜Ak)−Θ1(˜Ak)×[Θ2(Aj)+Θ3(Bj)]−Θ1(ˉAk)[Θ2(˜Aj)+Θ3(˜Bj)]}≤n∑j=1βkjFCk[Θ2(˜Aj)+Θ3(˜Bj)]≤n∑j=1βkj|FCk(ˆΘ2+ˆΘ3)|‖˜C‖Z, |
and
F2k[C]−F2k[C]=ck[Θ2(˜Ak)+Θ3(˜Bk)]≤ck|ˆΘ2+ˆΘ3|‖˜C‖Z, |
where
ˆΘ2=∫t0e−(μk+δ2k+ck)(t−a)∫ΩΓ3k(t−a,x,y)∫a0σk(b)π1k(b)×∫ΩΓ2k(b,y,z)dzdbdyda,ˆΘ3=∫t0e−(μk+δ2k+ck)(t−a)∫ΩΓ3k(t−a,x,y)∫a0γk(b)π2k(b)×∫ΩΓ4k(b,y,z)Bk(a−b,z)dzdbdyda. |
Denote
m1k(T)=n∑j=1βkj|FCk(T,⋅)[ˆΘ2(T,⋅)+ˆΘ3(T,⋅)]|X,m2k(T)=ck|ˆΘ2(T,⋅)+ˆΘ3(T,⋅)|X,m(T)=max{m11(T),m12(T),...,m1n(T),m21(T),m22(T),...,m2n(T)}, |
and
F[C]=(F11,F12,...,F1n,F21,F22,...,F2n)[C]:Z→Z. |
Clearly, we can choose T small enough such that m1k(T)<1 and m2k(T)<1 for all k=1,2,...,n. Consequently, we have m(T)<1. Then
‖FC−FˉC‖Z≤m(T)‖C−ˉC‖Z. |
Hence, applying contraction operator theorem [30], we conclude that F has a unique fixed point C=(A1,A2,...,An,B1,B2,...,Bn). From Theorem 3.1, (2.4) and (2.5), together with Ak(t,x)=n∑j=1βkjSk(t,x)Ij(t,x) and Bk(t,x)=ckIk(t,x), we derive the existence and uniqueness of the solution (S(t,⋅),e(t,⋅,⋅),I(t,⋅),r(t,⋅,⋅))∈Xn+×Yn+×Xn+×Yn+ for system (2.3).
To further establish the global existence of the solution of system (2.3), we need the following lemma.
Lemma 3.1. [31]. The following problem
{∂ω(t,x)∂t=dω△ω(t,x)+Λ−μω(t,x),x∈Ω,∂ω(t,x)∂ν=0,x∈∂Ω, |
admits a unique positive steady state ω∗=Λμ, which is globally attractive in X.
Theorem 3.4. Let initial functions satisfy (S0,e0,I0,r0)∈Xn+×Yn+×Xn+×Yn+. Then the system (2.3) has a unique solution (S(t,⋅),e(t,⋅,⋅),I(t,⋅),r(t,⋅,⋅))∈Xn+×Yn+×Xn+×Yn+ for t∈R+.
Proof. To extend the domain of existence from t∈[0,T] to t∈R+, it suffices to show that the solution does not blow up in finite time. In fact, by Theorem 3.1, we have that
∂Sk(t,x)∂t≤d1k△Sk(t,x)+Λk−μkSk(t,x), |
for all t>0 and x∈Ω. From Lemma 3.1 and the comparison principle, we get that Sk(t,x) is bounded above by the upper solution Λkμk.
We now claim that ek(t,a,x)<+∞ for all t>0, a>0, x∈Ω and k=1,2,...,n. From (2.4), it is sufficient to show that ek(t,0,x)<+∞ for all t>0 and x∈Ω. Suppose on the contrary that there exist te>0 and xe∈Ω such that
limt→te−0ek(t,0,xe)=+∞. |
We then have from (3.5) that
limt→te−0Sk(t,xe)=−∞, |
which implies that Sk(t,xe) is negative in the neighborhood of te. This contradicts to the positivity of Sk, which has been proved in Theorem 3.1. Furthermore, from ek(t,0,x)=n∑j=1βkjSk(t,x)Ij(t,x) and rk(t,0,x)=ckIk(t,x) in (2.4), we obtain Ik(t,x)<+∞ and rk(t,0,x)<+∞. And from (2.5), we get rk(t,a,x)<+∞ for all t>0, a>0, x∈Ω and k=1,2,...,n. Thus, blow up never occurs, and we obtain a solution (S(t,⋅),e(t,⋅,⋅),I(t,⋅),r(t,⋅,⋅))∈Xn+×Yn+×Xn+×Yn+ for t∈R+.
Theorem 3.5. The solution semiflow Φ(t)=(S(t,⋅),e(t,⋅,⋅),I(t,⋅),r(t,⋅,⋅)):Xn+×Yn+×Xn+×Yn+∖(0,0,0,0)→Xn+×Yn+×Xn+×Yn+∖(0,0,0,0) of model (2.3) has a compact and global attractor as condition (3.4) holds.
Proof. According to Theorem 3.2, we know that system (2.3) is ultimately bounded, which implies that solution semiflow Φ(t) is point dissipative on Xn+×Yn+×Xn+×Yn+∖(0,0,0,0). By Theorem 2.6 in [15], we can get that Φ(t) is compact for any t>0. Thus, from Theorem 3.4.8 in [32], we further obtain that Φ(t) has a compact and global attractor in Xn+×Yn+×Xn+×Yn+∖(0,0,0,0).
It is easy to see that model (2.3) has a unique disease-free steady state ˉE=(ˉS,0,0,0)T where ˉS=(ˉS1,ˉS2,...,ˉSn)T and ˉSk=Λkμk, k=1,2,...,n.
We denote E∗=(S∗,e∗(⋅),I∗,r∗(⋅))T as the space-independent endemic steady state of (2.3), where S∗=(S∗1,S∗2,...,S∗n)T, e∗(⋅)=(e∗1(⋅),e∗2(⋅),...,e∗n(⋅))T, I∗=(I∗1,I∗2,...,I∗n)T and r∗(⋅)=(r∗1(⋅),r∗2(⋅),...,r∗n(⋅))T. Then, E∗ satisfies
{Λk−μkS∗k−n∑j=1βkjS∗kI∗j=0,de∗k(a)dt=−[σk(a)+μk+δ1k]e∗k(a),∫∞0σk(a)e∗k(a)da−(μk+δ2k+ck)I∗k+∫∞0γk(b)r∗k(b)db=0,dr∗k(b)dt=−[γk(b)+μk]r∗k(b),e∗k(0)=n∑j=1βkjS∗kI∗j,r∗k(0)=ckI∗k. | (4.1) |
We denote fk(I∗)=n∑j=1βkjI∗j:Rn+→R+, hk(I∗)=Λkμk+fk(I∗):Rn+→R+, k=1,2,...,n for brevity. By solving the Eq (4.1), we get
S∗k=hk(I∗),e∗k(a)=S∗kπ1k(a)fk(I∗),r∗k(b)=ckπ2k(b)I∗k, | (4.2) |
where π1k(⋅) and π2k(⋅) are given in (2.4) and (2.5), respectively. Substituting (4.2) into the third equation in (4.1) and rearranging it, we have
I∗k=Lkhk(I∗)fk(I∗)(μk+δ2k+ck)−ckPk, | (4.3) |
where Lk=∫∞0σk(a)π1k(a)da and Pk=∫∞0γk(b)π2k(b)db. Let us define a matrix-valued function M(x) on Rn to Rn×n, where M(x)ij=Liβijhi(x)(μi+δ2i+ci)−ciPi, x=(x1,x2,...,xn)T∈Rn. Then, (4.3) is equivalent to
I∗=M(I∗)I∗. | (4.4) |
On the existence of the endemic equilibrium E∗ of system (2.3), we prove the following theorem.
Theorem 4.1. Let M0=(ˉSiLiβij(μi+δ2i+ci)−ciPi)n×n. If ρ(M0)>1, where ρ(M0) represents the spectral radius of M0, then system (2.3) has a space-independent steady state E∗.
Proof. From (4.4), we only need to show that the nonlinear operator ˜M(x):=M(x)x, x∈Rn+, has at least one positive fixed point x∗∈Rn+. We define ‖˜M(x)‖:=max1≤k≤n|˜M(x)k|, where ˜M(x)k denotes the k-th entry of vector ˜M(x). Then, ˜M(x) is monotone increasing with respect to x∈Rn+ and uniformly bounded above by max1≤k≤n|ΛkLk(μk+δ2k+ck)−ckPk|.
It is obvious that ˜M(0)=0 and M(0) is the strong Fréchet derivative of ˜M(⋅) at the origin. Since M(0)=M0, we have ρ(M(0))>1. Thus, it follows from the Perron-Frobenius theorem (see [33]) that ρ(M(0)) is a simple eigenvalue of M(0) corresponding to a strictly positive eigenvector, and there exists no nonnegative eigenvector of M(0) corresponding to eigenvalue 1. Hence, we apply Theorem 4.11 of [34], to conclude that operator ˜M(⋅) has at least one positive fixed point x∗∈Rn+.
Theorem 4.2. For all k=1,2,...,n, if
∫∞0αkσk(θ)π1k(θ)dθ<1,∫∞0γk(θ)π2k(θ)dθ<1, | (4.5) |
where αk=n∑j=1ˉSjβjkμk+δ2k, π1k(⋅) and π2k(⋅) are given in (2.4) and (2.5), respectively, then the disease-free steady state ˉE is globally asymptotically stable.
Proof. We construct a Lyapunov function
V(t)=n∑k=1Vk(t), |
where
Vk(t)=∫Ω[V1k(t,x)+V2k(t,x)+V3k(t,x)+V4k(t,x)]dx, |
V1k(t,x)=ˉSk[Sk(t,x)ˉSk−1−lnSk(t,x)ˉSk],V2k(t,x)=∫∞0χk(a)ek(t,a,x)da,V3k(t,x)=αkIk(t,x),V4k(t,x)=∫∞0ψk(b)rk(t,b,x)db, |
and
χk(a)=∫∞aαkσk(θ)π1k(θ)π1k(a)dθ,ψk(b)=∫∞bαkγk(θ)π2k(θ)π2k(b)dθ. | (4.6) |
Taking the derivation of V1k(t,x) along the trajectory of (2.3) with respect to t, we have
∂V1k∂t=Sk(t,x)−ˉSkSk(t,x)∂Sk(t,x)∂t=d1k[Sk(t,x)−ˉSk]△Sk(t,x)Sk(t,x)−μk[Sk(t,x)−ˉSk]2Sk(t,x)+ˉSkn∑j=1βkjIj(t,x)−Sk(t,x)n∑j=1βkjIj(t,x). | (4.7) |
Recalling (2.4), we can rewrite V2k(t,x) as follows
V2k(t,x)=∫t0χk(t−a)∫ΩΓ2k(t−a,x,y)Ak(a,y)dyπ1k(t−a)da+∫∞0χk(a+t)∫ΩΓ2k(a+t,x,y)e0k(a,y)dyπ1k(a+t)π1k(a)da. |
Thus, we calculate ∂V2k∂t along the solution of system (2.3) and get
∂V2k∂t=χk(0)Ak(t,x)+∫∞0{χ′k(a)−[μk+δ1k+σk(a)−d2k△]χk(a)}×ek(t,a,x)da. | (4.8) |
Similarly,
∂V3k∂t=αkd3k△Ik(t,x)+αk∫∞0σk(a)ek(t,a,x)da+αk∫∞0γk(b)rk(t,b,x)db−n∑j=1ˉSjβjkIk(t,x)−αkckIk(t,x). | (4.9) |
From (2.5), we get
V4k(t,x)=∫t0ψk(t−b)∫ΩΓ4k(t−b,x,y)Bk(b,y)dyπ2k(t−b)db+∫∞0ψk(b+t)∫ΩΓ4k(b+t,x,y)r0k(b,y)dyπ2k(b+t)π2k(b)db. |
Thus,
∂V4k∂t=ψk(0)Bk(t,x)+∫∞0{ψ′k(b)−[μk+γk(b)−d4k△]ψk(b)}rk(t,b,x)db. | (4.10) |
Hence, combining (4.7)–(4.10), we calculate the derivative of Vk(t) along the solution trajectory of (2.3) as
dVkdt=−d1kˉSk∫Ω|△Sk(t,x)|2S2k(t,x)dx−∫Ωμk[Sk(t,x)−ˉSk]2Sk(t,x)dx+∫Ω[χk(0)−1]×Ak(t,x)dx+∫Ω[ψk(0)−αk]Bk(t,x)dx+∫Ω∫∞0{αkσk(a)−[μk+δ1k+σk(a)−d2k△]χk(a)+χ′k(a)}ek(t,a,x)da+∫Ω∫∞0{αkγk(b)−[μk+γk(b)−d4k△]ψk(b)+ψ′k(b)}×rk(t,b,x)db+∫ΩˉSkn∑j=1βkjIj(t,x)dx−∫Ωn∑j=1ˉSjβjkIk(t,x)dx. |
Using (4.6), we yield that
dVdt=−n∑k=1d1kˉSk∫Ω|△Sk(t,x)|2S2kdx−n∑k=1∫Ωμk[Sk(t,x)−ˉSk]2Sk(t,x)dx+n∑k=1∫Ω[χk(0)−1]Ak(t,x)dx+n∑k=1∫Ω[ψk(0)−αk]Bk(t,x)dx. |
Thus, from (4.5), we have
χk(0)<1,ψk(0)<αk, |
which implies the global asymptotic stability of disease-free steady state ˉE.
Theorem 4.3. If ρ(M0)>1, lima→+∞ek(t,a,x)=0 and limb→+∞rk(t,b,x)=0 for all t>0, x∈Ω, then the space-independent steady state E∗ is globally asymptotically stable.
Proof. We define g(x)=x−1−lnx, clearly, g(x) is always positive for x>0 and g′(x)=1−1x. Consider a matrix D=(ˉβkj)n×n with entry ˉβkj=βkjLkS∗kI∗j and a digraph G=(U,H) which contains a set U={1,2,...,n} of vertices and a set H of arcs (k,j) leading from initial vertex k to terminal vertex j, then, we denote a weighted digraph as (G,D) for which each arc (j,k) is assigned a positive weight ˉβkj. Furthermore, we denote ˉD as the Laplacian matrix of matrix (G,D). Then, the irreducibility of matrix (βkj)n×n implies that ˉD is also irreducible. Let qk denote the cofactor of k-th diagonal element of ˉD. And we construct a Lyapunov function as the following form
W(t)=n∑k=1qkWk(t), |
where
Wk(t)=∫Ω[W1k(t,x)+W2k(t,x)+W3k(t,x)+W4k(t,x)]dx, |
W1k(t,x)=LkS∗kg[Sk(t,x)S∗k],W2k(t,x)=∫∞0Ψ1k(a)e∗k(a)g[ek(t,a,x)e∗k(a)]da,W3k(t,x)=I∗kg[Ik(t,x)I∗k],W4k(t,x)=∫∞0Ψ2k(b)r∗k(b)g[rk(t,b,x)r∗k(b)]db, |
and
Ψ1k(a)=∫∞aσk(s)π1k(s)π1k(a)ds,Ψ2k(b)=∫∞bγk(s)π2k(s)π2k(b)ds. | (4.11) |
For convenience, we denote Jk(t,x)=n∑j=1βkjIj(t,x) and J∗k=n∑j=1βkjI∗j. Taking the derivation of W1k along the trajectory of (2.3) with respect to t, we have
∂W1k∂t=LkS∗k[1S∗k−1Sk(t,x)]∂Sk(t,x)∂t=Lk[1−S∗kSk(t,x)]d1k△Sk(t,x)−LkμkSk(t,x)[Sk(t,x)−S∗k]2+LkS∗kJ∗k×[1+Jk(t,x)J∗k−S∗kSk(t,x)−Sk(t,x)Jk(t,x)S∗kJ∗k]. | (4.12) |
Calculating the derivative of W2k along the solution of system (2.3) yields
∂W2k∂t=∫∞0Ψ1k(a)e∗k(a)∂∂tg[ek(t,a,x)e∗k(a)]da=∫∞0Ψ1k(a)[1−e∗k(a)ek(t,a,x)]{d2kΔek(t,a,x)−∂∂aek(t,a,x)−[μk+δ1k+σk(a)]ek(t,a,x)}da=∫∞0Ψ1k(a)[1−e∗k(a)ek(t,a,x)]d2kΔek(t,a,x)da−∫∞0Ψ1k(a)e∗k(a)×[ek(t,a,x)e∗k(a)−1][1ek(t,a,x)∂∂aek(t,a,x)+μk+δ1k+σk(a)]da=∫∞0Ψ1k(a)[1−e∗k(a)ek(t,a,x)]d2kΔek(t,a,x)da−∫∞0Ψ1k(a)e∗k(a)×∂∂ag[ek(t,a,x)e∗k(a)]da=∫∞0Ψ1k(a)[1−e∗k(a)ek(t,a,x)]d2kΔek(t,a,x)da+Ψ1k(0)e∗k(0)×g[ek(t,0,x)e∗k(0)]+∫∞0g[ek(t,a,x)e∗k(a)]dda[Ψ1k(a)e∗k(a)]da=∫∞0Ψ1k(a)[1−e∗k(a)ek(t,a,x)]d2kΔek(t,a,x)da+LkS∗kJ∗kg[Sk(t,x)Jk(t,x)S∗kJ∗k]+∫∞0σk(a)e∗k(a)[1−ek(t,a,x)e∗k(a)+lnek(t,a,x)e∗k(a)]da. | (4.13) |
Similarly,
∂W3k∂t=[1−I∗kIk(t,x)][d3k△Ik(t,x)+∫∞0σk(a)ek(t,a,x)da−(μk+δ2k+ck)×Ik(t,x)+∫∞0γk(b)rk(t,b,x)db]=[1−I∗kIk(t,x)]{d3k△Ik(t,x)+∫∞0σk(a)e∗k(a)[ek(t,a,x)e∗k(a)−Ik(t,x)I∗k]da+∫∞0γk(b)r∗k(b)[rk(t,b,x)r∗k(b)−Ik(t,x)I∗k]db}=[1−I∗kIk(t,x)]d3k△Ik(t,x)+∫∞0σk(a)e∗k(a)[1−Ik(t,x)I∗k+ek(t,a,x)e∗k(a)−I∗kek(t,a,x)Ik(t,x)e∗k(a)]da+∫∞0γk(b)r∗k(b)[1−Ik(t,x)I∗k+rk(t,b,x)r∗k(b)−I∗krk(t,b,x)Ik(t,x)r∗k(b)]db, | (4.14) |
and
∂W4k∂t=∫∞0Ψ2k(b)[1−r∗k(b)rk(t,b,x)]d4kΔrk(t,b,x)db+PkckI∗kg[Ik(t,x)I∗k]+∫∞0γk(b)r∗k(b)[1−rk(t,b,x)r∗k(b)+lnrk(t,b,x)r∗k(b)]db. | (4.15) |
Hence, combining (4.12)–(4.15), we calculate the derivative of Wk(t) along the solution trajectory of (2.3) as
dWkdt=−Lkd1kS∗k∫Ω|∇Sk(t,x)|2S2k(t,x)dx−∫Ω∫∞0d2ke∗k(a)Ψ1k(a)|∇ek(t,a,x)|2e2k(t,a,x)dadx−d3kI∗k∫Ω|∇Ik(t,x)|2I2k(t,x)dx−∫Ω∫∞0d4kr∗k(b)Ψ2k(b)|∇rk(t,b,x)|2r2k(t,b,x)dbdx−Lkμk∫Ω[Sk(t,x)−S∗k]2Sk(t,x)dx+PkckI∗k∫Ωg[Ik(t,x)I∗k]dx+LkS∗kn∑j=1βkj×I∗j∫Ω[Jk(t,x)J∗k−S∗kSk(t,x)−lnSk(t,x)Jk(t,x)S∗kJ∗k]dx+∫Ω∫∞0σk(a)e∗k(a)×[2−Ik(t,x)I∗k−I∗kek(t,a,x)Ik(t,x)e∗k(a)+lnek(t,a,x)e∗k(a)]dadx+∫Ω∫∞0γk(b)r∗k(b)×[2−Ik(t,x)I∗k−I∗krk(t,b,x)Ik(t,x)r∗k(b)+lnrk(t,b,x)r∗k(b)]dbdx=−Lkd1kS∗k∫Ω|∇Sk(t,x)|2S2k(t,x)dx−∫Ω∫∞0d2ke∗k(a)Ψ1k(a)|∇ek(t,a,x)|2e2k(t,a,x)dadx−d3kI∗k∫Ω|∇Ik(t,x)|2I2k(t,x)dx−∫Ω∫∞0d4kr∗k(b)Ψ2k(b)|∇rk(t,b,x)|2r2k(t,b,x)dbdx−Lkμk∫Ω[Sk(t,x)−S∗k]2Sk(t,x)dx−LkS∗kJ∗k∫Ωg[S∗kSk(t,x)]dx−∫Ω∫∞0σk(a)×e∗k(a)g[I∗kek(t,a,x)Ik(t,x)e∗k(a)]dadx−∫Ω∫∞0γk(b)r∗k(b)g[I∗krk(t,b,x)Ik(t,x)r∗k(b)]dbdx−LkS∗kJ∗k∫Ω{g[Ik(t,x)I∗k]−g[Jk(t,x)J∗k]}dx. |
By Theorem 2.3 in [35], the following identity holds
n∑k=1qk{LkS∗kJ∗k∫Ω[g(Ik(t,x)I∗k)−g(Jk(t,x)J∗k)]dx}=0. |
Hence, together with the property of g, we have dWdt≤0. Furthermore, the strict equality holds only if Sk(t,x)=S∗k, ek(t,a,x)=e∗k(a), Ik(t,x)=I∗k, and rk(t,b,x)=r∗k(b). Thus, T∗={E∗}⊂Ω is the largest invariant subset of {(S,e,I,r):dWdt=0}, and the Lyapunov-LaSalle invariance principle implies that the endemic equilibrium E∗ is globally asymptotically stable when ρ(M0)>1.
In this section, we present numerical examples to demonstrate the validity and applicability of our main results, Theorems 4.2 and 4.3. For simplicity, we consider the case of two groups, a normalized 1-dimensional space (Ω = [0, 3]) and a normalized maximum age (a = 2). Firstly, we fix some parameter values as follows,
d11=0.2,d21=0.5,d31=0.4,d41=0.1,μ1=0.6,c1=0.006,d12=0.4,d22=0.1,d32=0.3,d42=0.2,μ2=0.7,c2=0.006. |
Then, we take the initial functions as
S01(x)=0.8×(1+0.3×sinπx),e01(a,x)=1.2×e−a,I01(x)=0.5×(1.5+0.2×sinπx),r01(a,x)=1.5×e−a,S02(x)=0.7×(1+0.2×sinπx),e02(a,x)=1.3×e−a,I02(x)=0.6×(1.5+0.3×sinπx),r02(a,x)=1.4×e−a. |
Example 5.1. If we choose other parameters as
Λ1=3,β11=0.04,β12=0.07,δ11=0.2,δ21=0.9,Λ2=2,β21=0.06,β22=0.05,δ12=0.5,δ22=0.8,σ1(a)=0.003×(1+sin(a−5)π2),γ1(a)=0.1×(1+sin(a−5)π2),σ2(a)=0.002×(1+sin(a−5)π2),γ2(a)=0.2×(1+sin(a−5)π2), | (5.1) |
then we have
∫∞0α1σ1(θ)π11(θ)dθ≈0.00051<1,∫∞0γ1(θ)π21(θ)dθ≈0.0817<1,∫∞0α2σ2(θ)π12(θ)dθ≈0.00027<1,∫∞0γ2(θ)π22(θ)dθ≈0.13319<1. |
In this case, from Theorem 4.2, we expect the disease-free steady state ˉE to be globally asymptotically stable. In fact, in Figure 1, the infective population I1(t,x) and I2(t,x) converge to zero over time.
Example 5.2. If we choose other parameters as
Λ1=5,β11=0.99,β12=0.97,δ11=0.002,δ12=0.005,Λ2=7,β21=0.96,β22=0.98,δ21=0.009,δ22=0.008,σ1(a)=35×(1+sin(a−5)π2),γ1(a)=0.1×(1+sin(a−5)π2),σ2(a)=30×(1+sin(a−5)π2),γ2(a)=0.2×(1+sin(a−5)π2), | (5.2) |
then we have ρ(M0)≈3.89217>1. In this case, from Theorem 4.3, we expect the space-independent steady state E∗ to be globally asymptotically stable. In fact, in Figure 2, the infective population I1(t,x) and I2(t,x) converge to the positive distribution over time.
In this paper, as an additional structure of the system, we focus on the spatial diffusion of the population. Models with spatial diffusion allow individuals to move to adjacent positions through a random walk process, this is a key factor in considering the geographical spread of infectious diseases. Firstly, we propose the n-group diffusive SEIR epidemic model with age-dependent latent and relapse, it is a generalization of the model in [27] to a spatially diffusive system. Then, we investigate the positivity, boundedness, existence and uniqueness of solution and the existence of compact global attractor of the associated solution semiflow for this system. For these results, we use the method of constructing Picard sequences, Banach-Picard fixed point theorem and theories of partial functional differential equations. Thereafter, we establish the existence of disease-free and endemic steady states based on Perron-Frobenius theorem. we utilize appropriate Lyapunov functionals, graph-theoretical results and the LaSalle's invariance principle to prove the global stability of disease-free and endemic steady states. Thus, we presented the results of numerical simulations to verify the validity of our main theorems. This is important from the viewpoint of applications.
In this epidemic model, we are concerned with two kinds of spatial heterogeneity: the patch structure and spatial diffusion. Furthermore, age-of-latent and age-of-relapse are included into the epidemic model which is appropriate for diseases such as tuberculosis and herpes virus infection. Dynamical results obtained in this paper provide theoretical foundation for seeking effective measures to prevent, control and study disease transmission.
The expressions of basic reproduction number and endemic steady state depends on space are not analyzed in this paper owing to the complexity of model. In addition, how to improve the sufficient conditions that ensure the stabilities of steady states and make them be depended on basic reproduction number is also need to investigate. We leave these issues for future research.
The authors are very grateful to the editor and the anonymous referees for their valuable comments and suggestions, which helped us to improve the presentation of this work significantly.
This work was supported by the National Natural Science Foundation of China (Grant Number: 11971329).
The authors declare there is no conflict of interest.
[1] |
W. O. Kermack, A. G. McKendrick, A contribution to the mathematical theory of epidemics, Proc. R. Soc. London, Ser. A, 115 (1927), 700-721. doi: 10.1098/rspa.1927.0118
![]() |
[2] | P. A. Naik, Global dynamics of a fractional-order SIR epidemic model with memory, Int. J. Biomath., 13 (2020), 1-24. |
[3] | P. A. Naik, J. Zu, M. Ghoreishi, Stability analysis and approximate solution of SIR epidemic model with Crowley-Martin type functional response and holling type-II treatment rate by using homotopy analysis method, J. Appl. Anal. Comput., 10 (2020), 1482-1515. |
[4] | P. A. Naik, J. Zu, K. M. Owolabi, Global dynamics of a fractional order model for the transmission of HIV epidemic with optimal control, Chaos Solitons Fractals, 138 (2020), 109826. |
[5] | P. A. Naik, J. Zu, K. M. Owolabi, Modeling the mechanics of viral kinetics under immune control during primary infection of HIV-1 with treatment in fractional order, Phys. A, 545 (2020), 123816. |
[6] | J. Zu, M. Li, Y. Gu, S. Fu, Modelling the evolutionary dynamics of host resistance-related traits in a susceptible-infected community with density-dependent mortality, Discrete Contin. Dyn. Syst. Ser. B, 25 (2020), 3049-3086. |
[7] | J. Zu, G. Zhuang, P. Liang, F. Cui, F. Wang, H. Zheng, et al., Estimating age-related incidence of HBsAg seroclearance in chronic hepatitis B virus infections of China by using a dynamic compartmental model, Sci. Rep., 7 (2017), 2912. |
[8] | J. Zu, M. Li, G. Zhuang, P. Liang, F. Cui, F. Wang, et al., Estimating the impact of test-and-treat strategies on hepatitis B virus infection in China by using an age-structured mathematical model, Medicine, 97 (2018), e0484. |
[9] |
A. Chekroun, M. N. Frioui, T. Kuniya, T. M. Touaoula, Global stability of an age-structured epidemic model with general Lyapunov functional, Math. Biosci. Eng., 16 (2019), 1525-1553. doi: 10.3934/mbe.2019073
![]() |
[10] |
Z. Feng, W. Huang, C. Castillo-Chavez, Global behavior of a multi-group SIS epidemic model with age structure, J. Differ. Equations, 218 (2005), 292-324. doi: 10.1016/j.jde.2004.10.009
![]() |
[11] |
T. Kuniya, Global stability analysis with a discretization approach for an age-structured multigroup SIR epidemic model, Nonlinear Anal. Real World Appl., 12 (2011), 2640-2655. doi: 10.1016/j.nonrwa.2011.03.011
![]() |
[12] |
J. Wang, H. Shu, Global analysis on a class of multi-group SEIR model with latency and relapse, Math. Biosci. Eng., 13 (2016), 209-225. doi: 10.3934/mbe.2016.13.209
![]() |
[13] |
J. Yang, R. Xu, X. Luo, Dynamical analysis of an age-structured multi-group SIVS epidemic model, Math. Biosci. Eng., 16 (2019), 636-666. doi: 10.3934/mbe.2019031
![]() |
[14] | R. S. Cantrell, C. Cosner, Spatial ecology via reaction-diffusion equations, Wiley Series in Mathematical and Computational Biology, John Wiley and Sons Ltd, Chichester, 2004. |
[15] | J. Wu, Theory and applications of partial functional differential equations, Springer-Verlag, New York, 1996. |
[16] | A. Chekroun, T. Kuniya, An infection age-space structured SIR epidemic model with Neumann boundary condition, Appl. Anal., 1-14. |
[17] |
R. Cui, Y. Lou, A spatial SIS model in advective heterogeneous environments, J. Differ. Equations, 261 (2016), 3305-3343. doi: 10.1016/j.jde.2016.05.025
![]() |
[18] |
A. Ducrot, P. Magal, S. Ruan, Travelling wave solutions in multigroup age-structured epidemic models, Arch. Ration. Mech. Anal., 195 (2010), 311-331. doi: 10.1007/s00205-008-0203-8
![]() |
[19] |
W. E. Fitzgibbon, J. J. Morgan, G. F. Webb, Y. Wu, A vector-host epidemic model with spatial structure and age of infection, Nonlinear Anal. Real World Appl., 41 (2018), 692-705. doi: 10.1016/j.nonrwa.2017.11.005
![]() |
[20] |
T. Kuniya, R. Oizumi, Existence result for an age-structured SIS epidemic model with spatial diffusion, Nonlinear Anal. Real World Appl., 23 (2015), 196-208. doi: 10.1016/j.nonrwa.2014.10.006
![]() |
[21] |
Y. Luo, S. Tang, Z. Teng, Global dynamics in a reaction-diffusion multi-group SIR epidemic model with nonlinear incidence, Nonlinear Anal. Real World Appl., 50 (2019), 365-385. doi: 10.1016/j.nonrwa.2019.05.008
![]() |
[22] |
X. Wang, X. Q. Zhao, J. Wang, A cholera epidemic model in a spatiotemporally heterogeneous environment, J. Math. Anal. Appl., 468 (2018), 893-912. doi: 10.1016/j.jmaa.2018.08.039
![]() |
[23] |
J. Yang, Z. Jin, F. Xu, Threshold dynamics of an age-space structured SIR model on heterogeneous environment, Appl. Math. Lett., 96 (2019), 69-74. doi: 10.1016/j.aml.2019.03.009
![]() |
[24] |
J. Yang, R. Xu, J. Li, Threshold dynamics of an age-space structured brucellosis disease model with Neumann boundary condition, Nonlinear Anal. Real World Appl., 50 (2019), 192-217. doi: 10.1016/j.nonrwa.2019.04.013
![]() |
[25] |
L. Zhao, Z. C. Wang, S. Ruan, Traveling wave solutions in a two-group SIR epidemic model with constant recruitment, J. Math. Biol., 77 (2018), 1871-1915. doi: 10.1007/s00285-018-1227-9
![]() |
[26] |
L. Liu, J. Wang, X. Liu, Global stability of an SEIR epidemic model with age-dependent latency and relapse, Nonlinear Anal. Real World Appl., 24 (2015), 18-35. doi: 10.1016/j.nonrwa.2015.01.001
![]() |
[27] |
L. Liu, X. Feng, A multigroup SEIR epidemic model with age-dependent latency and relapse, Math. Methods Appl. Sci., 41 (2018), 6814-6833. doi: 10.1002/mma.5193
![]() |
[28] |
X. Ren, Y. Tian, L. Liu, X. Liu, A reaction-diffusion within-host HIV model with cell-to-cell transmission, J. Math. Biol., 76 (2018), 1831-1872. doi: 10.1007/s00285-017-1202-x
![]() |
[29] | H. L. Smith, Monotone dynamical systems: an introduction to the theory of competitive and cooperative systems, vol. 41 of Mathematical Surveys and Monographs, American Mathematical Society, Providence, RI, 1995. |
[30] | K. Yosida, Functional analysis, Springer, New York, 1978. |
[31] |
Y. Lou, X. Q. Zhao, A reaction-diffusion malaria model with incubation period in the vector population, J. Math. Biol., 62 (2011), 543-568. doi: 10.1007/s00285-010-0346-8
![]() |
[32] | J. K. Hale, Asymptotic behavior of dissipative systems, American Mathematical Society, Providence, RI, 1988. |
[33] | A. Berman, R. J. Plemmons, Nonnegative matrices in mathematical sciences, Academic Press, New York, 1979. |
[34] | M. A. Krasnosel'skiǐ, Positive solutions of operator equations, Noordhoff, Groningen, 1964. |
[35] |
M. Y. Li, Z. Shuai, Global-stability problem for coupled systems of differential equations on networks, J. Differ. Equations, 248 (2010), 1-20. doi: 10.1016/j.jde.2009.09.003
![]() |
1. | Yi Li, Mandy Chen, Joshy George, Edison T. Liu, R. Krishna Murthy Karuturi, Adaptive Sentinel Testing in Workplace for COVID-19 Pandemic, 2022, 1557-8666, 10.1089/cmb.2022.0291 | |
2. | Peng Wu, Zhaosheng Feng, Xuebing Zhang, Global dynamics of an age–space structured HIV/AIDS model with viral load-dependent infection and conversion rates, 2022, 412, 03770427, 114309, 10.1016/j.cam.2022.114309 | |
3. | Yifan Xing, Hong-Xu Li, Almost periodic solutions for a SVIR epidemic model with relapse, 2021, 18, 1551-0018, 7191, 10.3934/mbe.2021356 | |
4. | Yanlin Ding, Jianjun Jiao, Global analysis and control for a vector-borne epidemic model with multi-edge infection on complex networks, 2023, 21, 2391-5455, 10.1515/math-2022-0580 | |
5. | Victoria Chebotaeva, Paula A. Vasquez, Erlang-Distributed SEIR Epidemic Models with Cross-Diffusion, 2023, 11, 2227-7390, 2167, 10.3390/math11092167 | |
6. | Peng Wu, Ran Zhang, Anwarud Din, Mathematical analysis of an age-since infection and diffusion HIV/AIDS model with treatment adherence and Dirichlet boundary condition, 2023, 214, 03784754, 1, 10.1016/j.matcom.2023.06.018 | |
7. | Zeyan Zhang, Chunmei Zhang, 2024, Exponential stability of stochastic multi-group models with regime-switching diffusion under the local conditions, 978-9-8875-8158-1, 1171, 10.23919/CCC63176.2024.10662306 |