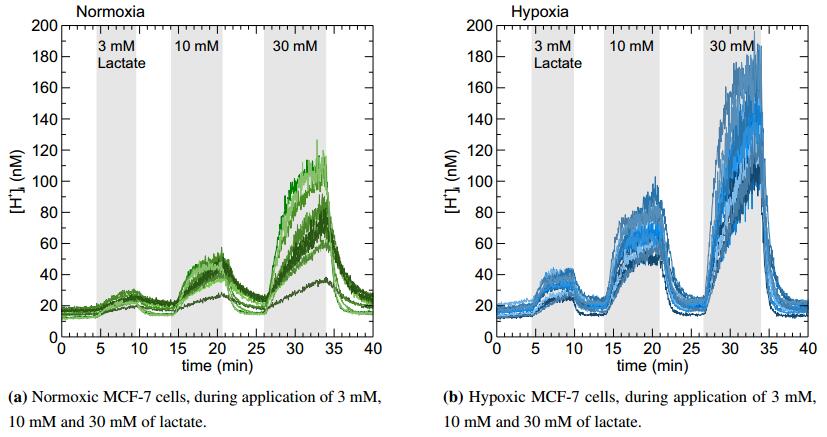
Citation: Ardak Kashkynbayev, Yerlan Amanbek, Bibinur Shupeyeva, Yang Kuang. Existence of traveling wave solutions to data-driven glioblastoma multiforme growth models with density-dependent diffusion[J]. Mathematical Biosciences and Engineering, 2020, 17(6): 7234-7247. doi: 10.3934/mbe.2020371
[1] | Benjamin Steinberg, Yuqing Wang, Huaxiong Huang, Robert M. Miura . Spatial Buffering Mechanism: Mathematical Model and Computer Simulations. Mathematical Biosciences and Engineering, 2005, 2(4): 675-702. doi: 10.3934/mbe.2005.2.675 |
[2] | H. J. Alsakaji, F. A. Rihan, K. Udhayakumar, F. El Ktaibi . Stochastic tumor-immune interaction model with external treatments and time delays: An optimal control problem. Mathematical Biosciences and Engineering, 2023, 20(11): 19270-19299. doi: 10.3934/mbe.2023852 |
[3] | Erin N. Bodine, K. Lars Monia . A proton therapy model using discrete difference equations with an example of treating hepatocellular carcinoma. Mathematical Biosciences and Engineering, 2017, 14(4): 881-899. doi: 10.3934/mbe.2017047 |
[4] | Samantha L Elliott, Emek Kose, Allison L Lewis, Anna E Steinfeld, Elizabeth A Zollinger . Modeling the stem cell hypothesis: Investigating the effects of cancer stem cells and TGF−β on tumor growth. Mathematical Biosciences and Engineering, 2019, 16(6): 7177-7194. doi: 10.3934/mbe.2019360 |
[5] | Katarzyna A. Rejniak . A Single-Cell Approach in Modeling the Dynamics of Tumor Microregions. Mathematical Biosciences and Engineering, 2005, 2(3): 643-655. doi: 10.3934/mbe.2005.2.643 |
[6] | Urszula Foryś, Jan Poleszczuk . A delay-differential equation model of HIV related cancer--immune system dynamics. Mathematical Biosciences and Engineering, 2011, 8(2): 627-641. doi: 10.3934/mbe.2011.8.627 |
[7] | Thanh Nam Nguyen, Jean Clairambault, Thierry Jaffredo, Benoît Perthame, Delphine Salort . Adaptive dynamics of hematopoietic stem cells and their supporting stroma: a model and mathematical analysis. Mathematical Biosciences and Engineering, 2019, 16(5): 4818-4845. doi: 10.3934/mbe.2019243 |
[8] | Margherita Carletti, Matteo Montani, Valentina Meschini, Marzia Bianchi, Lucia Radici . Stochastic modelling of PTEN regulation in brain tumors: A model for glioblastoma multiforme. Mathematical Biosciences and Engineering, 2015, 12(5): 965-981. doi: 10.3934/mbe.2015.12.965 |
[9] | Dan Liu, Shigui Ruan, Deming Zhu . Stable periodic oscillations in a two-stage cancer model of tumor and immune system interactions. Mathematical Biosciences and Engineering, 2012, 9(2): 347-368. doi: 10.3934/mbe.2012.9.347 |
[10] | Maria Vittoria Barbarossa, Christina Kuttler, Jonathan Zinsl . Delay equations modeling the effects of phase-specific drugs and immunotherapy on proliferating tumor cells. Mathematical Biosciences and Engineering, 2012, 9(2): 241-257. doi: 10.3934/mbe.2012.9.241 |
The most agammaessive and invasive tumor cells usually rely on extensive glycolysis to meet their large demand for energy and biosynthetic precursors [7,12,29,33]. The increase in glycolytic activity is often triggered by hypoxia, which derives from high cell density and insufficient vascularization [8,10,28]. However, up-regulation of glycolysis can also be observed in cancer cells under aerobic conditions, a phenomenon termed 'Warburg effect' [41,42]. The increase in glycolysis leads to vast production of lactate and protons, which have to be removed from the cell to prevent acidosis, which, among other effects, would result in inhibition of glycolysis and lead to cytostasis. Cancer cells are able to extrude the excess acidic byproducts via various pH sensitive membrane transporters.
Efflux of lactate from cancer cells is primarily mediated by the monocarboxylate transporters MCT1 and MCT4 [28,30,31,40]. The SLC16 gene family of MCTs comprises 14 isoforms, the first four of which (MCT1-4) carry lactate, but also other high-energy metabolites like pyruvate and ketone bodies, in cotransport with protons in a 1:1 stoichiometry across the cell membrane [11]. Lactate transport via the MCTs has been described by different kinetic models. By measuring influx of
Imaging experiments on human MCF-7 breast cancer cells have been described in detail previously [19]. In brief MCF-7 cells, purchased from the German Collection of Microorganisms and Cell Cultures DSMZ (DSMZ-No. ACC-115), were cultured in RPMI-1640 medium (Sigma Aldrich, R1383), supplemented with
In this section we describe the mathematical model characterizing the dynamics of MCT1 as observed in the experiment. Figure 1 depicts the experimentally observed values of intracellular proton concentrations (
Since the dynamics of
To model the transport activity of MCT1 we rely on the reaction kinetics model presented in [1], where the dynamics of the MCT1 is described as the transition between six states corresponding to:
1. state S1- binding of protons on the intracellular (IC) side,
2. state S2- binding of lactate on the IC side,
3. state S3- translocation of the loaded carrier from IC side to the extracellular (EC) side,
4. state S4- release of lactate on the EC side,
5. state S5- release of protons on the EC side,
6. state S6- translocation of the empty carrier from EC side to the IC side.
Transitions between these states (S1-S6) are modeled via simple first order reaction kinetics. Due to the difference in the relative speeds of each state transition, fast transitions are assumed to be in steady state. Using such quasi-steady state constraints, in [1] an algebraic expression for the net efflux (
T(Hi,He,Li,Le)=MT(k12k56KiKl)HiLi−(k21k65KoKe)HeLePMolsecP=(AHi+BHe+CLi+DLe)1sec A=k12(KiKlLi+KoLe+KiKo(Kl+1)LiLe)1sec MolB=k65(KiKeKlLi+KoKeLe+KiKoKeLiLe)1sec Mol, |
where
k12=.88521sec Molk56=10.141seck21=1.0451seck65=0.57211sec MolKo=k54k45=151MolKe=k16k61=45Ki=k23k32=KoKl=k34k43=KeMT=105MolD=k21Ko(Ke+1)1sec MolC=k56KiKl(Ke+1)1sec Mol. |
The buffer capacity
β=−d[H]+d(pH). |
The minus sign is due to the fact that increase of proton concentration results in decrease of pH, while conventionally the buffering power is a positive quantity. From this we deduce
β=−d[H]+d(pH)=−d[H]+dtd(pH)dt⇒d(pH)dtβ=−d[H]+dt. |
The right hand side (RHS) in the last equation above represents the applied change in protons with respect to time, while the left hand side (LHS) represents the observed change in pH. In our case the applied change in protons is due to the MCT1 transporter, thus we get that
βd(pH)dt=−T⇒d[H]+dt=ln(10)[H]+βT⇒d[H]+dt=2.3[H]+βT. |
The experimental data in Figure 1 only feature time dependence without any spatial component. Therefore, a naive modeling approach would be to formulate a system of ordinary stochastic differential equations, thus not involving any partial derivatives, but accounting (by way of the noise terms) for randomness. Considering the key biological processes described above, we formulate the following SDE system (1) which we shall refer to as the one-point model. Figure 2a illustrates the corresponding proton exchange.
dHidt=(2.3Hiβi)(−T(Hi,He,Li,Le))+r1Li+σ1Hiξ1t | (1a) |
dHedt=(2.3Heβe)(T(Hi,He,Li,Le))+σ2Heξ2t | (1b) |
dLidt=−T(Hi,He,Li,Le)+r1Li+σ3Liξ3t | (1c) |
dLedt=T(Hi,He,Li,Le)+c(t) | (1d) |
with initial conditions
Hi(0)=20nM,He(0)=40nM(≈7.4 pH),Li(0)=35μM,Le(0)=0. | (1e) |
Thereby,
The simulation results for the one-point model (1) are shown in Figure 3 for the normoxic regime. The red curve depicts the mean value of the intracellular proton concentration averaged over 100 simulated cells (sample paths). It suggests that the time dynamics of
Since a cell is a 3D structure with non-zero volume, its constituents are subjected to spatial effects, like e.g., diffusion, spatial distribution of the MCT1 on the cell membrane, etc. It is of particular importance to note that no membrane transporter can have access to the full concentration of the required constituents at any time point. Instead, only a fraction of the total concentration in the vicinity of the transporters is actively involved. Following this line of argument, we consider the concentration of intracellular protons to be divided into
Spatial movement (presumably diffusion) enables the conversion from unavailable to available protons and vice versa. This interconversion is modeled in this ODE-SDE setting via simple first order reaction kinetics between the respective compartments (or components):
![]() |
The model constructed this way will be refered to as two-compartment model and its dynamics are illustrated in Figure 2b. The concrete mathematical setting will be described below in the equations (2)-(3) supplemented with the corresponding prescriptions of the coefficient functions on the right hand sides and the initial conditions. The model involves the major unknowns
Modeling of CAII and CAIX
In the MCF-7 cell line used in this study, intracellular CAII is constantly expressed, both under normoxic and hypoxic conditions, while the expression of extracellular CAIX is strongly upregulated under hypoxia [19]. Both CAII and CAIX have been shown to facilitate transport activity of MCT1 via a non-catalytic mechanism, presumably by functioning as a proton collector and distributor for the transporter [1,2,6,19,26]. For extracellular CAIX the implications of this 'proton antenna' on the proton dynamics are as follows:
1. During
2. During
Since CAIX is only expressed under hypoxic conditions, these enhancing effects are only applied for hypoxic, but not for normoxic cells. CAII plays an analogous role at the IC side of the MCT1, both in normoxic and hypoxic cells. Figure 4 illustrates these influences in the hypoxic case.
The roles of CAIX and CAII are modeled in the following way:
1. The values of the parameters
2. Comparatively, the values of the parameters
Apart from this we make
To properly capture the dynamic behavior of CAIX we need to adequately incorporate the time dependent behavior of the exchange coefficients
We also introduce two buffer modulators
βe=ΔpHΔ[HCl] (mM), |
with
The mathematical description: Equations for proton and lactate dynamics
dHiudt=Hia<−>Hiu⏞k4Hia−k3Hiu+production by gylcolysis⏞r1Hiu+noise⏞σ4Hiuξt | (2a) |
dLeudt=applied Le⏞C1(t)−Le used by MCT1⏞C2(t) | (2b) |
dk1dt=rate of change of flow from Hea to Heu⏞kkC3(t) | (2c) |
dk2dt=rate of change of flow from Heu to Hea⏞kkC3(t) | (2d) |
k6(t)=−0.056(Hea(t)−Hea(0))2+20 | (2e) |
dLidt=−MCT1 transport activity⏞T(Hia,Hea,Li,Lea)+glycolytic lactate production⏞r1Li+noise⏞σ3Liξt | (3a) |
dLeadt=MCT1 transport activity⏞T(Hia,Hea,Li,Lea)+effectively applied Le⏞C2(t) | (3b) |
dHiadt=(buffer⏞k52.3Hiβi)(−MCT1 transport activity⏞T(Hia,Hea,Li,Lea))+Hia<−>Hiu⏞k3Hiu−k4Hia+noise⏞σ1Hiaξt | (3c) |
dHeadt=(buffer⏞2.3Heβek6)(MCT1 transport activity⏞T(Hia,Hea,Li,Lea))+Hea<−>Heu⏞k1(t)Heu−k2(t)Hea+noise⏞σ2Heaξt | (3d) |
where
C1(t)=m1S401(t)+m2S402(t)+m3S403(t)C2(t)=m1S61(t)+m2S62(t)+m3S63(t)C3(t)=m4S61(t)+m5S62(t)+m6S63(t) |
with
Sp1(t):=exp(−s1(.004t−t1)p)(−s1(p(.004t−t1)p−1).004)Sp2(t):=exp(−s2(.004t−t2)p)(−s2(p(.004t−t2)p−1).004)Sp3(t):=exp(−s3(.004t−t3)p)(−s3(p(.004t−t3)p−1).004). |
Thereby
Initial conditions:
Hiu(0)=1.998⋅10−8, Heu(0)=.65⋅10−7.4, Leu(0)=0, k1(0)=k10, k2(0)=k20Hia(0)=2⋅10−11,Hea(0)=.35⋅10−7.4,Lea(0)=0,Li(0)=5⋅10−6. |
Observe that
Numerical simulations
In order to validate the model (2) - (3) we perform numerical simulations and show that the model prediction fits the experimentally observed data in an appropriate manner. The choice of model parameters such as buffer capacity, pool exchange rates, MCT1 transporter rates etc. is crucial for reducing the error between the simulated result and the experimental data. Statistical methods should be used in order to estimate the involved parameters, however, of the 20 model parameters only at most 10 can be assigned fixed values directly based on experimental information. The rest are free variables that need to be statistically estimated. This is, however, a nontrivial problem, mainly due to the following challenges:
1. the model (3) is a coupled system of nonlinear stochastic differential equations involving several major unknowns:
2. since the parameters are present on the RHS of the equations and since there is no quantitative data for the derivatives, even if (3) consisted of only one equation (say for
3. finally, the nonlinear terms may render the error minimization task non-convex.
Taking these facts into consideration we tried the following error minimization approaches:
1. We first tried a maximum log-likelihood method. As
2. This led us to use Monte-Carlo based local search techniques. Since we already had a resonably good hand-picked guess of the parameters, we decided to randomly search, with
σ1=.00521sec,σ2=.00521sec,σ3=.00281sec,σ4=.00441sec,m1:=.003Molsec,m2:=.01Molsec,m3:=.03Molsec,m4:=.0002Molsec,m5:=.00075Molsec,m6:=.0009Molsec,t1:=1.75,r1:=10−51sec,s1:=15,s2:=5,s3:=5,βi=.04Mol,βe=.006Mol. |
Apart from the above, the parameter values for the environment type (i.e. normoxic and hypoxic) are given below.
1. Parameters for the simulation in the case of normoxic cells: %with
k10=.00021sec,k20=.00021sec,k3=.000151sec,k4=.000151sec,k5=.251sec,kk=2.6,t2:=4.4,t3:=7.25. |
2. Parameters for the simulation in the case of hypoxic cells. %in
k10=.000321sec,k20=.000321sec,k3=.0001751sec,k4=.0001751sec,k5=.51sec,kk=1.8,t2:=4.2,t3:=7.35. |
Using the above parameters we numerically simulated the model (2) - (3) by a finite difference scheme combined with an Euler-Maruyama time discretization. The 500 Monte-Carlo iterations were done in order to estimate the averages. Based on this we obtained the results in Figure 5 for intra- and extracellular proton concentrations in both the normoxic and the hypoxic regimes. Figure 6 shows the computed transport dynamics of MCT1. The numerical simulations indicate that the model is able to correctly predict the MCT1-induced dynamics of intracellular protons. In particular, the undersaturation of
Our model suggests that extracellular and intracellular carbonic anhydrases can facilitate MCT1-mediated proton/lactate cotransport by accelerating the exchange of protons between the immediate surrounding of the transporter and the bulk solution. It has been shown that
This project has been supported by the BioComp Research Unit at the University of Kaiserslautern (TUK).
The authors declare there is no conflict of interest.
[1] | Cancer, Fact Sheets, World Health Organization, 2018. Available from: https://www.who.int/en/news-room/fact-sheets/detail/cancer. |
[2] | Brain Cancer, Fact Sheets, National Cancer Institute. Available from: https://www.cancer.gov/types/brain/patient/adult-brain-treatment-pdq. |
[3] | International Agency for Research on Cancer, Report of World Health Organization, 2018. Available from: http://gco.iarc.fr/today/data/factsheets/populations/900-world-fact-sheets.pdf. |
[4] | Brain Tumor: Statistics, eJournal Cancer, Net of American Society of Clinical Oncology, January 2020. Available from: https://www.cancer.net/cancer-types/brain-tumor/statistics. |
[5] | Glioblastoma Multiforme: a giving smarter guide to accelerating research progress, Faster Cures Glioblastoma Report, Center of Milken Institute, 2014. Available from: https://www.fastercures.org/assets/Uploads. |
[6] |
T. Alarcón, H. M. Byrne, P. K. Maini, A multiple scale model for tumor growth, Multiscale Model. Simul., 3 (2005), 440-475. doi: 10.1137/040603760
![]() |
[7] | K. R. Swanson, E. Alvord, J. Murray, A quantative model for differential motility of gliomas in grey and white matter, Cell Proliferation, 33, (2000), 317-330. |
[8] | K. R. Swanson, C. Bridge, J. Murray, E. Alvord, Virtual and real brain tumors: using mathematical modeling to quantify glioma growth and invasion, J. Neurol. Sci., 216, (2003), 1-10. |
[9] | S. E. Eikenberry, T. Sankar, M. Preul, E. Kostelich, C. Thalhauser and Y. Kuang, Virtual glioblastoma: Growth, migration and treatment in a three-dimensional mathematical model, Cell Proliferation, 42 (2009), 115-134. |
[10] |
G. Powathil, M. Kohandel, S. Sivaloganathan, A. Oza, M. Milosevic, Mathematical modeling of brain tumors: effects of radiotherapy and chemotherapy, Phys. Med. Biol., 52 (2007), 3291-3306. doi: 10.1088/0031-9155/52/11/023
![]() |
[11] | H. Hatzikirou, A. Deutsch, C. Schaller, M. Simon, K. Swanson, Mathematical modelling of Glioblastoma tumour development: a review, Math. Models Methods Appl. Sci., 24 (2005), 1779-1794. |
[12] |
N. L. Martirosyan, E. M. Rutter, W. L. Ramey, E. J. Kostelich, Y. Kuang, M. C. Preul, Mathematically modeling the biological properties of Gliomas: a review, Math. Biosci. Eng., 12 (2015), 879-905. doi: 10.3934/mbe.2015.12.879
![]() |
[13] |
J. Murray, Glioblastoma brain tumours: Estimating the time from brain tumour initiation and resolution of a patient survival anomaly after similar treatment protocols, J. Biol. Dyn., 6 (2012), 118-127. doi: 10.1080/17513758.2012.678392
![]() |
[14] | L. Curtin, A. Hawkins-Daarud, K. G. van der Zee, K. R. Swanson, M. R. Owen, Speed switch in glioblastoma growth rate due to enhanced hypoxia-induced migration, Bull. Math. Biol., 82 (2020), 43. |
[15] | P. Gerlee, S. Nelander, The impact of phenotypic switching on glioblastoma growth and invasion, PLoS Comput. Biol., 8, (2012), e1002556. |
[16] | T. L. Stepien, E. M. Rutter, Y. Kuang, Traveling waves of a go-or-grow model of glioma growth, SIAM J. Appl. Math., 78, (2018), 1178-1801. |
[17] | T. L. Stepien, E. M. Rutter, Y. Kuang, A data-motivated density-dependent diffusion model of in vitro glioblastoma growth, Math. Biosci. Eng., 12, (2015), 1157-1172. |
[18] | A. M. Stein, T. Demuth, D. Mobley, M. Berens, L.M. Sander, A mathematical model of glioblastoma tumor spheroid invasion in a three-dimensional in vitro experiment, Biophys. J., 92, (2007), 356-365. |
[19] |
H. Enderling, M. A. J. Chaplain, Mathematical modeling of tumor growth and treatment, Curr. Pharm. Des., 20 (2014), 4934-4940. doi: 10.2174/1381612819666131125150434
![]() |
[20] | Y. Kuang, J. D. Nagy, S. E. Eikenberry, Introduction to mathematical oncology, CRC Press, 2015. |
[21] |
L. Han, S. Eikenberry, C. He, L. Johnson, M. C. Preul, E. J. Kostelich, Y. Kuang, Patient-specific parameter estimates of glioblastoma multiforme growth dynamics from a model with explicit birth and death rates, Math. Biosci. Eng., 16, (2019), 5307-5323. doi: 10.3934/mbe.2019265
![]() |
[22] | Z. Wen, M. Fan, A. M. Asiri, E. O. Alzahrani, M. M. El-Dessoky, Y. Kuang, Global existence and uniqueness of classical solutions for a generalized quasilinear parabolic equation with application to a glioblastoma growth model, Math. Biosci. Eng., 14, (2017), 407-420. |
[23] | E. M. Rutter, T. L. Stepien, B. J. Anderies, J. D. Plasencia, E. C. Woolf, A. C. Scheck, et al., Mathematical Analysis of Glioma Growth in a Murine Model, Sci. Rep., 7, (2017), 2508. |
[24] | R. A. Fisher, The wave of advance of advantageous genes, Ann. Genet., 7 (1937), 355—369. |
[25] | A. N. Kolmogorov, I. G. Petrovsky, N. S. Piskunov, Investigation of the equation of diffusion combined with increasing of the substance and its application to a biology problem, Bull. Moscow State Univ. Ser. A: Math. Mech., 1 (1937), 1—25. |
[26] | M. Bramson, Convergence of solutions of the Kolmogorov equation to travelling wave, Memoirs Amer. Math. Soc., 285 (1983), 8—31. |
[27] |
D. G. Kendall, A form of wave propagation associated with the equation of heat conduction, Proc. Camb. Phil. Soc., 44 (1948), 591-593. doi: 10.1017/S0305004100024609
![]() |
[28] | D. G. Aronson, H. F. Weinberger, Nonlinear diffusion in population genetics, combustion and nerve pulse propagation, in Partial Differential Equations and Related Topics (ed. J.A. Goldstein), Springer, (1975), 5-49. |
[29] |
D. G. Aronson, H. F. Weinberger, Multidimensional nonlinear diffusion arising in population genetics, Adv. Math., 30 (1978), 33-76. doi: 10.1016/0001-8708(78)90130-5
![]() |
[30] | O. Dieckman, Dynamics in Bio-Mathematical perspective, Math. Comput. Sci. II, 4 (1986), 23-50. |
[31] | J. Murray, Mathematical Biology I: An Introduction, 3dedition, Springer, 2002. |
[32] | J. Murray, Mathematical Biology II: Spatial models and biomedical applications, 3dedition, Springer, 2003. |
[33] | P. Tracqui, G. Cruywagen, D. Woodward, G. Bartoo, J. Murray, E. Alvord, A mathematical model of glioma growth: the effect of extent of surgical resection, Cell Proliferation, 29, (1996), 269-288. |
[34] | M. Kot, Elements of mathematical ecology, Cambridge University Press, Cambridge, 2001. |
[35] | J. Doke, GRABIT, MATLAB Central File Exchange, 2005. Available from: https: //www.mathworks.com/matlabcentral/fileexchange/7173-grabit. |
[36] |
J. C. Lagarias, J. A. Reeds, M. H. Wright, E. P. Wright, Convergence properties of the Nelder-Mead simplex method in low dimensions, SIAM J. Optim., 9 (1998), 112-147. doi: 10.1137/S1052623496303470
![]() |
[37] |
N. J. Armstrong, K. J. Painter, J. A. Sherratt, A continuum approach to modelling cell-cell adhesion, J. Theor. Biol., 243 (2006), 98-113. doi: 10.1016/j.jtbi.2006.05.030
![]() |
1. | Samantha Ames, Jacob T. Andring, Robert McKenna, Holger M. Becker, CAIX forms a transport metabolon with monocarboxylate transporters in human breast cancer cells, 2020, 39, 0950-9232, 1710, 10.1038/s41388-019-1098-6 | |
2. | Holger M. Becker, Carbonic anhydrase IX and acid transport in cancer, 2020, 122, 0007-0920, 157, 10.1038/s41416-019-0642-z | |
3. | Holger M. Becker, Joachim W. Deitmer, Transport Metabolons and Acid/Base Balance in Tumor Cells, 2020, 12, 2072-6694, 899, 10.3390/cancers12040899 | |
4. | Nicola Piasentin, Edoardo Milotti, Roberto Chignola, The control of acidity in tumor cells: a biophysical model, 2020, 10, 2045-2322, 10.1038/s41598-020-70396-1 | |
5. | Manh Tien Tran, Effects of Tumor Microenvironment Acidification on Progression of Pancreatic Ductal Adenocarcinoma: A Review, 2021, 2708-7999, 17, 10.32948/ajo.2021.08.14 | |
6. | Juan Deng, Shaofei Yuan, Wenjie Pan, Qimeng Li, Zhonglin Chen, Nanotherapy to Reshape the Tumor Microenvironment: A New Strategy for Prostate Cancer Treatment, 2024, 9, 2470-1343, 26878, 10.1021/acsomega.4c03055 | |
7. | Jacob E. Combs, Akilah B. Murray, Carrie L. Lomelino, Mam Y. Mboge, Mario Mietzsch, Nicole A. Horenstein, Susan C. Frost, Robert McKenna, Holger M. Becker, Disruption of the Physical Interaction Between Carbonic Anhydrase IX and the Monocarboxylate Transporter 4 Impacts Lactate Transport in Breast Cancer Cells, 2024, 25, 1422-0067, 11994, 10.3390/ijms252211994 |