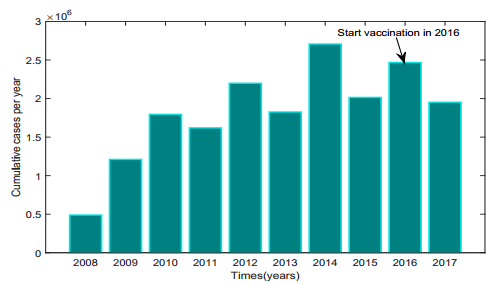
Citation: Lei Shi, Hongyong Zhao, Daiyong Wu. Modelling and analysis of HFMD with the effects of vaccination, contaminated environments and quarantine in mainland China[J]. Mathematical Biosciences and Engineering, 2019, 16(1): 474-500. doi: 10.3934/mbe.2019022
[1] | Yangjun Ma, Maoxing Liu, Qiang Hou, Jinqing Zhao . Modelling seasonal HFMD with the recessive infection in Shandong, China. Mathematical Biosciences and Engineering, 2013, 10(4): 1159-1171. doi: 10.3934/mbe.2013.10.1159 |
[2] | Yong Li, Meng Huang, Li Peng . A multi-group model for estimating the transmission rate of hand, foot and mouth disease in mainland China. Mathematical Biosciences and Engineering, 2019, 16(4): 2305-2321. doi: 10.3934/mbe.2019115 |
[3] | Chenxi Dai, ZhiWang, Weiming Wang, Yongqin Li, Kaifa Wang . Epidemics and underlying factors of multiple-peak pattern on hand, foot and mouth disease inWenzhou, China. Mathematical Biosciences and Engineering, 2019, 16(4): 2168-2188. doi: 10.3934/mbe.2019106 |
[4] | Fang Wang, Lianying Cao, Xiaoji Song . Mathematical modeling of mutated COVID-19 transmission with quarantine, isolation and vaccination. Mathematical Biosciences and Engineering, 2022, 19(8): 8035-8056. doi: 10.3934/mbe.2022376 |
[5] | Chenwei Song, Rui Xu, Ning Bai, Xiaohong Tian, Jiazhe Lin . Global dynamics and optimal control of a cholera transmission model with vaccination strategy and multiple pathways. Mathematical Biosciences and Engineering, 2020, 17(4): 4210-4224. doi: 10.3934/mbe.2020233 |
[6] | Shina D. Oloniiju, Olumuyiwa Otegbeye, Absalom E. Ezugwu . Investigating the impact of vaccination and non-pharmaceutical measures in curbing COVID-19 spread: A South Africa perspective. Mathematical Biosciences and Engineering, 2022, 19(1): 1058-1077. doi: 10.3934/mbe.2022049 |
[7] | Siyu Liu, Yong Li, Yingjie Bi, Qingdao Huang . Mixed vaccination strategy for the control of tuberculosis: A case study in China. Mathematical Biosciences and Engineering, 2017, 14(3): 695-708. doi: 10.3934/mbe.2017039 |
[8] | C. K. Mahadhika, Dipo Aldila . A deterministic transmission model for analytics-driven optimization of COVID-19 post-pandemic vaccination and quarantine strategies. Mathematical Biosciences and Engineering, 2024, 21(4): 4956-4988. doi: 10.3934/mbe.2024219 |
[9] | Hao Wang, Di Zhu, Shiqi Li, Robert A. Cheke, Sanyi Tang, Weike Zhou . Home quarantine or centralized quarantine? A mathematical modelling study on the COVID-19 epidemic in Guangzhou in 2021. Mathematical Biosciences and Engineering, 2022, 19(9): 9060-9078. doi: 10.3934/mbe.2022421 |
[10] | Tao-Li Kang, Hai-Feng Huo, Hong Xiang . Dynamics and optimal control of tuberculosis model with the combined effects of vaccination, treatment and contaminated environments. Mathematical Biosciences and Engineering, 2024, 21(4): 5308-5334. doi: 10.3934/mbe.2024234 |
Hand, foot, and mouth disease (HFMD) is a common childhood illness, mainly caused by coxsackievirus A16 (CVA16) and enterovirus 71 (EV71), as well as certain enteroviruses, including coxsackievirus A4, 5, 9, 10, B2 and 5 [1,2]. HFMD usually occurs in children under six years old, although it may also occur in older children and adults [3]. The usual incubation period is 2 to 7 days, and an infected individual will fully recover after 2 to 10 days. HFMD was first reported in New Zealand in 1957 and first named in America in 1959 [4], then it has widely spread in America [5], Europe [6,7] and Asia [8,9,10,11,12,13,14,15,16,17].
Recently, HFMD has seriously threatened the health of infants and young children in mainland China. It has been classified as a class III infectious disease in the National Stationary Notifiable Communicable Diseases, and the annual data of HFMD have been archived by the Chinese Center for Disease Control and Prevention (CCDC) [3] and the National Health and Family Planning Commission of the People's Republic of China (NHFPC) [18] since 2008. Figure 1 shows the reported annual cumulative cases of more than 1.5 million since 2010 and more than 2 million since 2014. In analyses of analyze the epidemiological characteristics and pathogenic spectrum of FHMD in mainland China, EV71 and CVA16 have been considered major pathogens in the past decade, and the positive rates of EV71 and CVA16 were about 35%∼55% and 20%∼35%, respectively [19,20,21,22,23]. As described in [22,23], the EV71 vaccine was developed and has been used in mainland China since 2016, which have protected many young children protect away from the EV71 virus infections. And, from the data shown in Figure 1, we conclude that vaccination measures may have led to a lower number of cases in 2016 or 2017 than in 2014 or 2015, in contrast to the diseases trend in the past. The clinical trial results [22,23] showed that the vaccine provides immunity only for the EV71 virus; it provides no form of protection against infection for other viruses. Furthermore, there are few studies on the effects of EV71 vaccination on the spread of HFMD. It should be noted that epidemiological modelling is an important tool helpful to understand infectious disease spread and control [24,25,26].
A number of epidemiological models that do not consider EV71 vaccination have been established to investigate the transmission dynamics and to predict infections of HFMD. For instance, Wang and Sung (2006) [27] used a susceptible-infectious-recovered (SIR) model to analyse epidemic situations of FHMD in Taiwan from 1999 to 2003; Tiing and Labadin (2008) [28] studied SIR model to predict the number of the infected and the duration of an outbreak in Sarawak Malaysia; Roy and Halder (2012) [29] proposed a susceptible-exposed-infectious-quarantine-recovered (SEIQR) model of HFMD; Ma and Liu (2013) [30] extended the SEIQR model, considered asymptomatic infectious, to formulate a more realistic SEIIeR model, which has been fitted to data of HFMD in Shandong Province, China; Wang and Xiao (2016) [31,32] further considered indirect transmission coming from the contaminated environment to establish an SEIIeRW model. Moreover, some studies on the dynamical behaviors or application of the above models are shown in [33,34,35,36,37,38,39,40]. However, most of these models have considered either quarantine for infectious individuals [30,35,37] or contaminated environment caused by infectious individuals [31] alone. Moreover, no previous studies have considered the shared effects of vaccination, quarantine measures, and maintaining sanitation on disease. From the point view of practical application, the lack of key factors may affect the modeling.
To quantify these issues, we propose a new mathematical model, which extends the existing ones such as SEIQR [30] and SEIIeRW [31], to analyse the effects of EV71 vaccination, clean-up of contaminated environments, and quarantine on HFMD in mainland China simultaneously. In order to analyse the effects of EV71 vaccination, we take the individuals withEV71 vaccination as an independent compartment in modelling, which is a well deal with the case that EV71 vaccine against the EV71 virus but not against others. A combination of analytical and numerical techniques are used to analyze the proposed model. Finally, we use our model to individually fit the monthly reported data of HFMD in mainland China from 2015 to 2017. This paper is organized as follows: In Section 2, a novel epidemiological model of HFMD is proposed. In Section 3, the dynamic behaviors of this model are analyzed. In Section 4, the model is used to fit the real reported data, and some control strategies are discussed. In Section 5, we discuss and summarize our conclusions. In Appendix A, a new estimating algorithm based on BP neural network is designed.
In this section, we propose an eight-compartment HFMD model, named SVEIIeQRW. The underlying structure of the model comprises classes of individuals are susceptible S(t), vaccinated V(t) (individuals with EV71 vaccine), exposed but not yet infectious E(t), infectious with symptoms (symptomatic infectious) I(t), infectious but not yet symptomatic (asymptomatic infectious) Ie(t), infectious and hospitalized or quarantined or isolated Q(t), and recovered R(t). Using the same description in [31], W(t) is the density of pathogen of the contaminated environment including door handles, towels, handkerchiefs, toys, utensils, bedding, underclothes, etc. at time t. Note that the individuals V(t) vaccinated with EV71 vaccine can also be infected with FHMD by FHMD viruses except EV71.
A flow diagram describing the model is shown in Figure 2, and the model can be represented by the following ordinary differential equations:
{dSdt=Λ−β1SI−β2SIe−σSW−kS−dS+η1R,dVdt=kS−ˉβ1VI−ˉβ2VIe−ˉσVW−dV+η2R,dEdt=β1SI+β2SIe+σSW+ˉβ1VI+ˉβ2VIe+ˉσVW−αE−dE,dIdt=αρE−pI−γ1I−dI−mI,dIedt=α(1−ρ)E−γ3Ie−dIe,dQdt=pI−γ2Q−dQ−mQ,dRdt=γ1I+γ2Q+γ3Ie−η1R−η2R−dR,dWdt=λ1I+λ2Ie−δW, | (2.1) |
where k,p are nonnegative, other parameters are positive and its biological meanings are listed in Table 1.
Parameter | Definition |
Λ | Recruitment rate |
β1,β2, and σ | Transmission rate from I(t), Ie(t), and W(t) to S(t) respectively |
ˉβ1, ˉβ2, and ˉσ | Transmission rate from I(t), Ie(t), and W(t) to V(t) respectively |
m | Disease-related death of HFMD |
d | Progression rate leaving the children group below the age of six |
k | Vaccinated rate of S(t) |
η1 and η2 | Rate from R(t) to S(t) and V(t) respectively |
1/α | Average incubation period |
ρ | Proportion of symptomatic infectious individuals |
γ1, γ2, and γ3 | Recovery rate of I(t), Ie(t), and Q(t) respectively |
p | Quarantine rate at which symptomatic infectious individuals I(t) enters into quarantine individuals Q(t) |
λ1 and λ2 | Virus shedding rate from symptomatic and asymptomatic infected individuals respectively |
δ | Clearance rate of pathogens W(t) |
Remark 1. Only the authors in [32] have considered the vaccination measure for HFMD, but it assumed that vaccinees against all HFMD viruses, in which the individuals being vaccinated in the susceptible class straight enter to the recovered class in modelling. However, it should be noted that the current vaccine is only resistant to the EV71 virus [22,23], which is well modeled in our model by adding a vaccinated class V(t). In addition, the previous studies did not have taken into account the effects of both contaminated environment and quarantine on the spread of disease.
In this section, we discuss the dynamic behaviors for system (2.1). We first introduce some notations which will be used throughout this paper. Rn denotes the n-dimensional Euclidean space, and Rn+≜{(x1,x2,⋯,xn)∈Rn:xi≥0,i=1,⋯,n}. A=(aij)n×n denotes a matrix of n×n real matrices. The superscript T denotes matrix or vector transposition. For a continuous and bounded function f:[0,+∞)→R, denote f∞=lim inft→+∞f(t) and f∞=lim supt→+∞f(t). Let
φ(0)=(S(0),V(0),E(0),I(0),Ie(0),Q(0),R(0),W(0))∈R8+ |
be the initial condition of system (2.1). Clearly, any solution of system (2.1) with non-negative initial conditions is non-negative, and the following lemma shows that the solutions are uniformly ultimately bounded.
Lemma 3.1. The solutions of system (2.1) eventually enter
Γ={(S(t),V(t),E(t),I(t),Ie(t),Q(t),R(t),W(t))∈R8+:0≤S(t)+V(t)+E(t)+I(t)+Ie(t)+Q(t)+R(t)≤Λd,0≤W(t)≤(λ1+λ2)Λdδ}. |
Proof. It is obvious that the population size N(t)=S(t)+V(t)+E(t)+I(t)+Ie(t)+Q(t)+R(t)≥0 and W(t)≥0. From system (2.1), the population size satisfies the following equation
dNdt=Λ−dS−dV−dE−dI−dIe−(d+m)Q−(d+μ)R=Λ−dN−m(I+Q)≤Λ−dN. | (3.1) |
According to the comparison theorem, there exists t1>0 such that N(t)≤Λd, for t≥t1.
It follows from the last equation of (2.1) that for t≥t1,
dWdt=λ1I+λ2Ie−δW≤(λ1+λ2)N−δW≤(λ1+λ2)Λd−δW. | (3.2) |
Similarly, there exists t2>t1 such that W(t)≤(λ1+λ2)Λdδ, for t≥t2. Therefore, the solutions of system (2.1) are uniformly ultimately bounded. This completes the proof.
It is clear that system (2.1) has a disease-free equilibrium
E0(S0,V0,E0,I0,I0e,Q0,R0,W0)=(Λk+d,kΛd(k+d),0,0,0,0,0,0). |
Using the next generation matrix method developed by Van den and Watmough [41], we obtain the basic reproduction number
R0=R1+R2+R3, | (3.3) |
where
R1=ρα(β1S0+ˉβ1V0)(α+d)(p+γ1+d+m)≜ρRI,R2=(1−ρ)α(β2S0+ˉβ2V0)(α+d)(γ3+d)≜(1−ρ)RIe,R3=(αρλ1(α+d)(p+γ1+d+m)δ+α(1−ρ)λ2(α+d)(γ3+d)δ)(σS0+ˉσV0), |
and
RI=α(β1S0+ˉβ1V0)(α+d)(p+γ1+d+m),RIe=α(β2S0+ˉβ2V0)(α+d)(γ3+d). |
RI is the average number of secondary infected individuals generated by a symptomatic infected individual; RIe is the average number of secondary infected individuals generated by an asymptomatic infected individual; R3 is the average number of secondary infected individuals generated by free-living viruses in the environment shed by the infected individuals. R0 is a central concept in measuring the transmission of infectious diseases.
In this following, we discuss the stability of the disease-free equilibrium and the persistence for system (2.1).
Theorem 3.2. The disease-free equilibrium E0 is locally asymptotically stable if R0<1 and unstable if R0>1.
Proof. The Jacobian matrix J(E0) of system (2.1) at E0 is given by
[−(k+d)00−β1S0−β2S00η1−σS0k−d0−ˉβ1V0−ˉβ2V00η2−ˉσV000−(α+d)β1S0+ˉβ1V0β2S0+ˉβ2V000σS0+ˉσV000αρ−(p+γ1+d+m)000000α(1−ρ)0−(γ3+d)000000p0−(γ2+d+m)00000γ1γ3γ2−(η1+η2+d)0000λ1λ200−δ]. | (3.4) |
We can obtain the eigenvalues for (3.4) as −(k+d), −d, −(γ2+d+m), −(η1+η2+d), and roots of
λ4+a1λ3+a2λ2+a3λ+a4=0, | (3.5) |
where
a1=(α+d)+(p+γ1+d+m)+(γ3+d),a2=(α+d)δ+(p+γ1+d+m)(γ3+d)+(γ3+d)δ+(α+d)(p+γ1+d+m)(1−R1)+(α+d)(γ3+d)(1−R2),a3=(p+γ1+d+m)(γ3+d)δ+(α+d)(p+γ1+d+m)[1−(R1+R2)]+(α+d)(p+γ1+d+m)(γ3+d)δ(1−R3),a4=(α+d)(p+γ1+d+m)(γ3+d)δ(1−R0). |
Note that if R0<1, then R1<1, R2<1, R3<1. Hence, it can get that ai>0,i=1,⋯,4. Further, one has
|a11a3a2|>0, |a110a3a2a10a4a3|>0,|a1100a3a2a110a4a3a2000a4|>0. |
According to Hurwitz criterion, all the roots of (3.5) have negative real part. Therefore, E0 is locally asymptotically stable. If R0>1, then a4<0. This means that (3.5) has at least one positive real part root. Then, E0 is unstable. This completes the proof.
In order to analyse the global asymptotic stability of the disease-free equilibrium E0 for system (2.1), denote
ˆR0={ραˆβ1(α+d)(p+γ1+d+m)+(1−ρ)αˆβ2(α+d)(γ3+d)+(αρλ1(α+d)(p+γ1+d+m)δ+α(1−ρ)λ2(α+d)(γ3+d)δ)ˆσ}Λd, |
where ˆβ1=max(β1,ˉβ1),ˆβ2=max(β2,ˉβ2),ˆσ=max(σ,ˉσ). It is obvious that R0≤ˆR0. Moreover, it follows that R0=ˆR0 if and only if β1=ˉβ1,β2=ˉβ2,σ=ˉσ.
Theorem 3.3. If ˆR0<1, then the disease-free equilibrium E0 is globally asymptotically stable.
Proof. From Theorem 3.2, we have that E0 is locally asymptotically stable. Then, it only needs to prove the global attraction of the disease-free equilibrium E0.
Note that any solution of system (2.1) with nonnegative initial is nonnegative and uniformly ultimataly bounded. By the fluctuation lemma [42], , there exists a sequence {tn} such that tn→+∞,S(tn)→S∞ and dS(tn)dt→0 as n→+∞. It follows from the first equation of (2.1) that
dS(tn)dt+β1S(tn)I(tn)+β2S(tn)Ie(tn)+σS(tn)W(tn)+(k+d)S(tn)=Λ+η1R(tn). | (3.6) |
Letting n→+∞, one has from (3.6) that
(k+d)S∞≤[(k+d)+I∞+Ie∞+W∞]S∞≤Λ+η1R∞. | (3.7) |
Similarly, it follows from the other equations of (2.1) that
dV∞≤(d+I∞+Ie∞+W∞)V∞≤kS∞+η2R∞, | (3.8) |
(α+d)E∞≤(β1I∞+β2I∞e+σW∞)S∞+(ˉβ1I∞+ˉβ2I∞e+ˉσW∞)V∞, | (3.9) |
(p+γ1+d+m)I∞≤αρE∞, | (3.10) |
(γ3+d)I∞e≤α(1−ρ)E∞, | (3.11) |
(γ2+d+m)Q∞≤pI∞, | (3.12) |
(η1+η2+d)R∞≤γ1I∞+γ2Q∞+γ3I∞e, | (3.13) |
δW∞≤λ1I∞+λ2I∞e. | (3.14) |
By (3.7) and (3.8), one has
S∞≤Λk+d+η1k+dR∞, | (3.15) |
V∞≤kΛd(k+d)+kη1d(k+d)R∞+η2dR∞. | (3.16) |
We claim that E∞=0. Suppose not, it follows from (3.10), (3.11) and (3.14) that
I∞≤αρp+γ1+d+mE∞, | (3.17) |
I∞e≤α(1−ρ)γ3+dE∞, | (3.18) |
W∞≤λ1αρδ(p+γ1+d+m)E∞+λ2α(1−ρ)δ(γ3+d)E∞. | (3.19) |
Substituting inequalities (3.17), (3.18), (3.19) into (3.9), after some simplification, we derive that
ρα(β1S∞+ˉβ1V∞)(α+d)(p+γ1+d+m)+(1−ρ)α(β2S∞+ˉβ2V∞)(α+d)(γ3+d)+(αρλ1(α+d)(p+γ1+d+m)δ+α(1−ρ)λ2(α+d)(γ3+d)δ)(σS∞+ˉσV∞)≥1. | (3.20) |
By (3.20), one has that
{ραˆβ1(α+d)(p+γ1+d+m)+(1−ρ)αˆβ2(α+d)(γ3+d)+(αρλ1(α+d)(p+γ1+d+m)δ+α(1−ρ)λ2(α+d)(γ3+d)δ)ˆσ}(S∞+V∞)≥ρα(β1S∞+ˉβ1V∞)(α+d)(p+γ1+d+m)+(1−ρ)α(β2S∞+ˉβ2V∞)(α+d)(γ3+d)+(αρλ1(α+d)(p+γ1+d+m)δ+α(1−ρ)λ2(α+d)(γ3+d)δ)(σS∞+ˉσV∞)≥1. | (3.21) |
It follows from (3.21) that
S∞+V∞≥ΛdˆR0, |
which is a contradiction since S∞+V∞≤Λd and ˆR0<1. This proves the claim. Since E∞=0, then one has from (3.10)-(3.14) that I∞=0,I∞e=0,Q∞=0,R∞=0,W∞=0. Therefore,
limt→+∞E(t)=limt→+∞I(t)=limt→+∞Ie(t)=limt→+∞Q(t)=limt→+∞R(t)=limt→+∞W(t)=0. | (3.22) |
By (3.15) and (3.16), one has
S∞≤Λk+d,V∞≤kΛd(k+d). | (3.23) |
Moreover, using the fluctuation lemma again, there exists a sequence {sn} such that sn→+∞,S(sn)→+∞, and dS(sn)dt→0 as n→+∞. By the first and second equations of (2.1), one has
dS(sn)dt=Λ−β1S(sn)I(sn)−β2S(sn)Ie(sn)−σS(sn)W(sn)−(k+d)S(sn)+η1R(sn),dV(sn)dt=kS(sn)−ˉβ1V(sn)I(sn)−ˉβ2V(sn)Ie(sn)−ˉσV(sn)W(sn)−dV(sn)+η2R(sn). |
Letting n→+∞ and by using (3.22), we obtain that
S∞=Λk+d,V∞=kΛd(k+d). | (3.24) |
It follows from (3.23) and (3.24) that
limt→+∞S(t)=Λk+d,limt→+∞V(t)=kΛd(k+d). |
Thus,
limt→+∞(S(t),V(t),E(t),I(t),Ie(t),Q(t),R(t),W(t))=E0. |
This completes the proof.
Theorem 3.4. If R0>1, then system (2.1) is uniformly persistent. That is, if R0>1, there exists a small positive constant ε>0 such that I∞>ε, Ie∞>ε for system (2.1) with initial value φ(0) and I(0)>0, Ie(0)>0.
Proof. In order to prove this result, the uniform persistence theorem in [44] is used. Define
X={(S,V,E,I,Ie,Q,R,W)∈Γ},X0={(S,V,E,I,Ie,Q,R,W)∈X:E>0,I>0,Ie>0,Q>0,R>0,W>0},∂X0=X∖X0. |
Now we prove that system (2.1) is uniformly persistent with respect to (X,X0).
First, it is easy to verify that both X and X0 are positively invariant for system (2.1), and X0 is relatively closed in X. Moreover, by Theorem 3.1, the system (2.1) is point dissipative. Thus, the system (2.1) must exist a globally attractor. Set
M∂={(S(0),V(0),E(0),I(0),Ie(0),Q(0),R(0),W(0))∈∂X0:(S(t),V(t),E(t),I(t),Ie(t),Q(t),R(t),W(t))∈∂X0,∀t≥0}. |
Now we prove that
M∂={(S,V,0,0,0,0,0,0)∈∂X:S≥0,V≥0}≜M′∂. |
It is obvious that M′∂⊆M∂, then we only need to prove M∂⊆M′∂. Suppose not, let φ(t) be a solution of system (2.1) with the initial condition φ(0). Then, for any
φ(t)=(S(t),V(t),E(t),I(t),Ie(t),Q(t),R(t),W(t))∈M∂ |
and φ(t)≠M′∂, there must exist at least one of E(t), I(t), Ie(t), Q(t), R(t), W(t) which is not zero. Without loss of generality, assume that E(t)=0, I(t)=0, Ie(t)=0, Q(t)=0, R(t)=0, but W(t)>0. From system (2.1), one has the following equations, for ∀t>0,
E(t)=e−(α+d)t[E(0)+∫t0[(β1I(u)+β2Ie(u)+σW(u))S(u)+(ˉβ1I(u)+ˉβ2Ie(u)+ˉσW(u))V(u)]du]>0,I(t)=e−(p+γ1+d+m)t[I(0)+∫t0αρE(u)du]>0,Ie(t)=e−(γ3+d)t[Ie(0)+∫t0α(1−ρ)E(u)du]>0,Q(t)=e−(γ2+d+m)t[Q(0)+∫t0pI(u)du]>0,R(t)=e−(η1+η2+d)t[R(0)+∫t0(γ1I(u)+γ2Q(u)+γ3Ie(u))du]>0,W(t)=e−δt[W(0)+∫t0(λ1I(u)+λ2Ie(u))du]>0. |
This means that φ(t)∉∂X0 for t>0, which contradicts the assumption that φ(t)∈M∂. Hence, we obtain that M∂⊆M′∂. Since M∂=M′∂, we obtain that M∂ only has the disease-free equilibrium E0(S0,V0,0,0,0,0,0,0) and E0 is compact and isolate invariant for φ(0)∈M∂.
Next, we only need to prove that Ws(E0)∩X0=∅, where Ws(E0) denotes the stable manifold of E0. That is, there exists a positive constant ε such that for any solution Φt(φ(0)) of system (2.1) with the initial condition φ(0)∈X0, one has
D(Φt(φ(0)),E0)∞≥ε, |
where D is a distance function in X0. Suppose not, then D(Φt(φ(0)),E0)∞<ˉε, for ∀ˉε>0. Hence, there must exists T>0 such that Λk+d−ˉε≤S(t)≤Λk+d+ˉε, kΛd(k+d)−ˉε≤V(t)≤kΛd(k+d)+ˉε, 0≤E(t)≤ˉε, 0≤I(t)≤ˉε, 0≤Q(t)≤ˉε and 0≤W(t)≤ˉε, for t>T. For t>T, we have
{dEdt≥L1(ˉε)I+L2(ˉε)Ie+L3(ˉε)W−(α+d)E,dIdt=αρE−(p+γ1+d+m)I,dIedt=α(1−ρ)E−(γ3+d)Ie,dWdt=λ1I+λ2Ie−δW, | (3.25) |
where
L1(ˉε)=β1(Λk+d−ˉε)+ˉβ1(kΛd(k+d)−ˉε),L2(ˉε)=β2(Λk+d−ˉε)+ˉβ2(kΛd(k+d)−ˉε),L3(ˉε)=σ(Λk+d−ˉε)+ˉσ(kΛd(k+d)−ˉε). |
Consider an auxiliary system as follows
dudt=ˉA(ˉε)u, | (3.26) |
where u=(u1,u2,u3,u4)T and
ˉA(ˉε)=(−(α+d)L1(ˉε)L2(ˉε)L3(ˉε)αρ−(p+γ1+d+m)00α(1−ρ)0−(γ3+d)00λ1λ2−δ). | (3.27) |
Recall that stability modulus of matrix ˉA(ˉε), denoted by S(ˉA(ˉε)), is defined by
S(ˉA(ˉε))=max{Reλ:λis an eigenvalue ofˉA(ˉε)}. |
Note that ˉA(ˉε) is irreducible and has non-negative off-diagonal elements, then S(ˉA(ˉε)) is a simple eigenvalue of ˉA(ˉε) with a positive eigenvector (see Theorem A. 5 in [43]). By Lemma 2.1 in [44] and the proof of Theorem 2 in [41], the following equivalent inequations are hold:
R0<1⟺S(ˉA(0))<0, and R0>1⟺S(ˉA(0))>0. | (3.28) |
Since S(ˉA(ˉε)) is continous in ˉε, choose ˉε>0 small enough such that S(ˉA(ˉε))>0 as R0>1. Hence, let u(t)=(u1(t),u2(t),u3(t),u4(t))T be a positive solution of the auxiliary system (3.26), which is strictly increasing with ui(t)→+∞ as t→+∞, i=1,…,4. By the comparison principle, one has
limt→+∞E(t)=+∞,limt→+∞I(t)=+∞,limt→+∞Ie(t)=+∞,limt→+∞W(t)=+∞. | (3.29) |
By system (2.1), since
limt→+∞I(t)=+∞,limt→+∞Ie(t)=+∞, |
it is also gained that
limt→+∞Q(t)=+∞,limt→+∞R(t)=+∞. |
This contracts with our assumption. Hence, E0 is an isolated invariant set in X and Ws(E0)∩X0=∅.
Therefore, the system (2.1) is uniformly persistent if R0>1. This completes the proof.
Remark 2. As far as the authors' knowledge, all the existing results of autonomous epidemiological models for HFMD such as [28,29,31,34,35,40] have obtained the similar result that the basic reproduction number R0 is the only criterion to determine whether the disease is eliminated, i.e., the model only has a disease-free equilibrium if R0<1 and it is uniformly persistent if R0>1. Because we consider vaccination and the vaccinated individuals can also be infected by HFMD viruses except EV71 in modeling, different from the existing results, the disease is extinct as ˆR0<1 (see Theorem 3.4). In addition, our model may have positive equilibriums even if the basic reproduction number R0 is less than 1 (see the analyses in Section 5).
In particular, if we ignore the influence of vaccination, i.e., let k=0 for system (2.1) and define the model as SEIIeQRW, then the reproduction number for SEIIeQRW is defined as
ˇR0={ραβ1(α+d)(p+γ1+d+m)+(1−ρ)αβ2(α+d)(γ3+d)+(αρλ1(α+d)(p+γ1+d+m)δ+α(1−ρ)λ2(α+d)(γ3+d)δ)σ}Λd. |
By using the similar analysis as that in the proof of 3.3 and Theorem 3.4, one can easily derive the following corollary. Its proof is omitted.
Corollary 1. If ˇR0<1, then system SEIIeQRW has an unique disease-free equilibrium ˇE0(S0,E0,I0,I0e,Q0,R0,W0)=(Λd,0,0,0,0,0,0) and ˇE0 is globally asymptotically stable; If ˇR0>1, then system SEIIeQRW is uniformly persistent.
From Corollary 1, it is clear that ˇR0 is the only threshold to decide whether the disease is extinct or not.
In this section, by using system (2.1), we simulate the reported data of HFMD in mainland China. From the website of CCDC [3], and NHFPC [18], we have obtained the monthly numbers of newly reported HFMD cases in mainland China from January 2015 to December 2017. We only consider the population of young children under six years old, a high risk group for HFMD. Note that EV71 virus has been vaccinated in mainland China starting in 2016, then we assume that the vaccinated rate k of system (2.1) is zero before 2016.
First, based on BP neural network, we design an algorithm to estimate unknown parameters of system (2.1) (see Appendix A) to. Then, we estimate parameters of system (2.1) by minimizing the following error function
ER=12∑i=1(Ii−ˆIi)2/N, | (4.1) |
where ˆIi is the reported data of HFMD in the i month. Ii is numerically computed solutions of I(t) of system (2.1) in the i month. N is the annual total population of less than 6 years old.
All the estimated parameters are shown in Table 2, and the error ER for every year is computed and included in Table 3. It is obvious that ER<0.0001 in every year. Therefore, our model fits well with the reported data of HFMD for each year from 2015 to 2017 in mainland China. Taking the year of 2017 as an example, the numerical simulation of the number of symptomatic infectious individuals I(t) of HFMD by system (2.1) is shown in Figure 3.
Para. | 2015 | 2016 | 2017 | References |
Λ | 1382638 | 1488333 | 1518641 | [45] |
m | 6.46×10−5 | 3.42×10−4 | 1.27×10−4 | [3,18] |
β1 | 1.08×10−7 | 1.11×10−7 | 1.08×10−7 | Fitting |
β2 | 2.44×10−8 | 2.50×10−8 | 2.38×10−7 | Fitting |
σ | 7.61×10−11 | 7.51×10−11 | 7.6×10−11 | Fitting |
ˉβ1 | 0 | 6.76×10−8 | 6.58×10−8 | Fitting |
ˉβ2 | 0 | 1.38×10−8 | 1.38×10−8 | Fitting |
ˉσ | 0 | 5.65×10−11 | 4.47×10−11 | Fitting |
d | 1.42×10−2 | 1.28×10−2 | 1.31×10−2 | [45] |
k | 0 | 1.21×10−3 | 1.31×10−3 | Fitting |
η1 | 0.035 | 0.035 | 0.035 | [31] |
η2 | 0 | 1.5×10−6 | 1.5×10−6 | Assumption |
1/α | 4/30 | 4/30 | 4/30 | [28] |
ρ | 0.2294 | 0.2299 | 0.2316 | Fitting |
γ1 | 3.5293 | 3.5293 | 3.5293 | [28] |
γ2 | 3.5293 | 3.5293 | 3.5293 | [28] |
γ3 | 3.5293 | 3.5293 | 3.5293 | Assumption |
p | 0.0025 | 0.0025 | 0.0025 | [30] |
λ1 | 93.8 | 93.8 | 93.8 | [31] |
λ2 | 78.9 | 78.9 | 78.9 | [31] |
δ | 2.7 | 2.7 | 2.7 | [31] |
S(0) | 95020000 | 96288000 | 95820000 | [45] |
V(0) | 0 | 0 | 5×105 | Assumption |
E(0) | 300000 | 300000 | 300000 | Assumption |
I(0) | 57763 | 79499 | 77412 | [3,18] |
Ie(0) | 150000 | 150000 | 150000 | Assumption |
Q(0) | 0 | 0 | 0 | Assumption |
R(0) | 28000 | 28000 | 28000 | Assumption |
W(0) | 0 | 0 | 0 | Assumption |
Year | 2015 | 2016 | 2017 |
ER | 3.47×10−6 | 8.54×10−6 | 3.86×10−6 |
We all know that the basic reproduction number R0 is an important quantity in characterizing the spread of disease.
Based on the basis of our parameter values, the basic reproduction number R0 and basic components of R0 include R1,R2,R3 are calculated in every year in Table 4. Note that the basic reproduction R0=R1+R2+R3 for each year is greater than 1, where R1,R2, and R3 with respect to symptomatic infected, asymptomatic infected individual, and free-living viruses in the environment, respectively. Moreover, in Table 4, the values of R1 and R2 are far greater than R3, which means that the new patient has a high probability of being infected by symptomatic infectious individuals and asymptomatic infectious individuals, rather than the contaminated environment caused by infectious individuals.
Year | 2015 | 2016 | 2017 |
R1 | 0.6651 | 0.6535 | 0.6377 |
R2 | 0.4011 | 0.4418 | 0.4066 |
R3 | 0.0515 | 0.0546 | 0.0532 |
R0 | 1.1177 | 1.1499 | 1.0975 |
Next, by using Latin hypercube sampling (LHS) and partial rank correlation coefficients (PRCCS) [46] (Marino et al., 2008), we investigate the effects of parameters on R0. It notes that PRCCS, showing which parameters have the largest influence on model outcomes, is calculated using the rank transformed LHS matrix and output matrix [46]. Take 2000 simulations per round, choose a uniform distribution for all the parameters with ranges listed in Table 5, and test for significant PRCCs for all parameters of system (2.1).
Parameter | Distribution | PRCC | p-Value |
Λ | U(1.38×106,1.53×106) | 0.2692 | 2.49×10−5 |
β1 | U(1.05×10−7,1.25×10−7) | 0.9705 | 0 |
β2 | U(2.2×10−8,2.6×10−8) | 0.8901 | 0 |
σ | U(7.0×10−11,8.0×10−11) | 0.4059 | 0 |
ˉβ1 | U(6.5×10−8,7.0×10−8) | 0.5397 | 0 |
ˉβ2 | U(1.1×10−8,1.5×10−8) | 0.4250 | 0 |
ˉσ | U(4.0×10−11,6.0×10−11) | 0.0729 | 1.04×10−11 |
k | U(1.1×10−3,1.5×10−3) | -0.7439 | 0 |
d | U(1.2×10−2,1.5×10−2) | -0.1280 | 0.0013 |
α | U(7.3, 7.7) | 0.00173 | 0.0943 |
ρ | U(0.22, 0.25) | 0.1351 | 0 |
γ1 | U(3.4, 3.6) | -0.8908 | 0 |
γ3 | U(3.4, 3.6) | -0.8101 | 0 |
λ1 | U(85, 95) | 0.1833 | 3.44×10−15 |
λ2 | U(75, 85) | 0.6495 | 0 |
δ | U(2.5, 3.0) | -0.7123 | 0 |
m | U(5.0×10−5,4.0×10−4) | -0.00215 | 0.0029 |
p | U(2.0×10−3,3.0×10−3) | -0.5388 | 0 |
Figure 4 shows that the PRCC values illustrate the dependence of R0 on each input parameter. Assume that absolute values of PRCC>0.4 is highly negatively correlated between input parameters and R0, then it is easy to know that β1,β2,σ,ˉβ1,ˉβ2,λ2 are highly positively correlated to R0, while k,γ1,γ3,δ,p are highly negatively correlated to R0. That is, reducing positively correlated parameters and increasing negatively correlated parameters can reduce value of R0, in which lower value of R0 means lower spread of the epidemics.
According to sensitivity analysis of R0 in Subsection 4.2, we have known the key factors affecting the spread of HFMD. Then, some detailed numerical experiments provide effective measures for the prevention and control of the spread of FHMD in mainland China. Moreover, we pay more attention to that the varieties of vaccinated individuals, quarantined individuals, and environmental health impact HFMD epidemic in mainland China, which can be shown by adjusting the related parameters k,p,δ for system (2.1). Taking the year of 2017 as an example, the prevention and control strategies are proposed as follows:
Strategy 1: Increasing the vaccinated rate k for the susceptible individuals S(t) can reduce the spread of HFMD. Though the vaccinated individuals V(t) can also be infected, but they have a lower infected probability than the unvaccinated individuals S(t). In Table 2, all transmission rates of V(t) of system (2.1) such as ˉβ1,ˉβ2 and ˉσ are smaller than the transmission rates of S(t). Figure 5(a) shows that the simulation of I(t) of system (2.1) decreases with increasing the vaccinated rate k, while the solution curve of symptomatic infected individuals I(t) of system (2.1) is at the top in the figure as k=0. Moreover, Figure 5(b) and Figure 5(c) show that the peak values and annual cumulative cases of symptomatic infected individuals I(t) decreases with the increase of k. Except for parameter k, Assuming that all the parameter of system (2.1) are shown in Table 1, and comparing Figure 5(d) with Figure 5(a), Figure 5(b) and Figure 5(c), we obtain the following results: (ⅰ) The size of R0 determines the degree of the disease outbreaks, which are the same as the analyses in Subsection 4.2; (ⅱ) If the vaccinated rate k<0.07, i.e., R0<1, then the disease persists (see Theorem 3.3); (ⅲ) If the vaccinated rate k>0.07, which means that R0<1 and ˆR0>1, then we can not be sure whether the disease disappears or persists (see Theorem 3.3 and Theorem 3.4, and the reasons are analyzed in detail in Section 5). Furthermore, In order to investigate the effects of current EV71 vaccination strategy for HFMD in mainland China, we compare the annual simulated cumulative cases by system (2.1) without vaccination with the report cases. In Figure 6, the annual reported cumulative cases are lower than the annual simulation cumulative cases, and we also obtain that the current vaccine measures have reduced the total number of patients in 2016 and 2017 by 17% and 22%, respectively.
Strategy 2: Increasing the quarantined rate p for the symptomatic infectious individuals I(t) can reduce the spread of HFMD. From system (2.1), the quarantined individuals Q(t) can not spread diseases and the symptomatic infectious individuals I(t) are highly infectious, then transforming the symptomatic infectious into the quarantined is an efficient control strategy. From Figure 7(d), we obtain that the disease persists if the quarantined rate p<0.73, while the long-term behaviors of the disease may not be ensure if p>0.73. The other analyses for Figure 7 are the same as strategy 1.
Strategy 3: Increasing the clearance rate δ of the pathogens caused by the infectious individuals containing I(t) and Ie(t) can reduce the spread of HFMD (see Figure 8). For instance, we can improve health-care education such as washing hands after using the toilet and before meals, and making air fresh indoors and so on. It should popularize health knowledge and advocate good personal hygiene habits in kindergardens, schools, hospitals and other places. Kindergardens should clean and disinfect toys and appliances every day. Moreover, hospitals should strengthen infection control practices to avoid nosocomial cross infection. In addition, from Figure 8(d), the disease persists.
In short, if we use the above prevention and control measures, the HFMD would be effectively controlled and the number of infections would decrease rapidly in short time. Those measures can effectively prevent and control the large-scale diffusion of HFMD in mainland China.
In this paper, we have proposed an eight-compartment dynamic model for HFMD in mainland China. The proposed model extends the existing models by considering vaccination, contaminated environment and quarantine simultaneously, where the vaccinated individuals with EV71 vaccine can also be infected by other FHMD viruses and the transmission rates with respect to the vaccinated individuals may be different from the unvaccinated individuals. Hence, unlike the previous results, we take the vaccinated individuals as an independent compartment in modeling. The main purpose of this study is to examine the effect of vaccination, contaminated environment and quarantine on the spread of HFMD in mainland China.
By investigating the dynamic behaviors of our model, we have identified two critical parameters as the basic reproduction number R0 and the threshold parameter ˆR0 (R0≤ˆR0). Then, the dynamic behaviors of system (2.1) could be divided into three cases (see Section 3):
(ⅰ) If R0≤ˆR0<1, then system (2.1) has only one disease-free equilibrium E0 and E0 is globally asymptotically stable, which means that the disease is extinct (see Theorem 3.3);
(ⅱ) If 1<R0≤ˆR0, then system (2.1) is uniformly persistent (see Theorem 3.4);
(ⅲ) If R0<1<ˆR0, then dynamic behaviors of system (2.1) are difficult to determine.
Unlike the previous results of epidemiological models for HFMD [28,29,31,34,35,40], in which the disease-free equilibrium is globally asymptotically stable if the basic reproduction number R0<1, in case (iii) that E0 of system (2.1) may not be globally asymptotically stable and system (2.1) may have positive equilibriums. For example, if take Λ=1.3×104,β1=2.29×10−7,β2=1.5×10−7,σ=1×10−7,ˉβ1=0.1×10−7,ˉβ2=1×10−7,ˉσ=0.7×10−7,α=0.4,ρ=0.5,η1=2.29,η2=1.5,γ1=0.9,γ2=2,γ3=0.9,λ1=0.04,λ2=0.04,δ=0.4,d=1×10−3,p=0.1,k=0.1,m=1×10−4 for system (2.1), then we obtain a disease-free equilibrium
E0(S0,V0,E0,I0,I0e,Q0,R0,W0)=(1.2871×105,1.2871×107,0,0,0,0,0,0) |
and a positive equilibrium (see Figure 9)
E∗(S∗,V∗,E∗,I∗,I∗e,Q∗,R∗,W∗)=(3.7283×106,3.3361×106,4.0799×106,8.1508×105,9.0564×105,4.0732×104,1.6302×104,1.7207×105). |
Based on the above parameter values, we calculate R0=0.8981 and ˆR0=2.6993. Then, in this case, when R0<1<ˆR0, it is obvious that system (2.1) has a disease-free equilibrium and a positive equilibrium, and none of them is globally asymptotically stable. The reason our model has dynamic behaviors different from those seen in the existing research is that our model considers vaccination, and the vaccinated individuals can also be infected by HFMD viruses except EV71; thus, the reproduction number R0 can not be the only threshold to judge whether the disease is extinct or not.
The proposed model has been used to individually fit the annual reported cases of HFMD in mainland China from 2015 to 2017. Moreover, the basic reproduction number R0 of each year is greater than 1 (see Table 4), which means system (1) is uniformly persistent. Using Latin hypercube sampling (LHS) and partial rank correlation coefficients (PRCCS) for sensitive analysis of R0, we find the key factors affecting transmission of HFMD, where the highly positively correlated parameters are β1,β2,σ,ˉβ1,ˉβ2,λ2 and the highly negatively correlated parameters are k,γ1,γ3,δ,p (see Subsection 4.2). We analyse effect of the varieties of the rates k,δ and p, with respect to vaccinated individuals, quarantined individuals and environmental health respectively, on the spread of HFMD in mainland China. As a result, we deduce that increasing the rates k,δ,p can reduce the number of infectious individuals in detail in Subsection 4.3. Moreover, it also easily verifies that for any k>0 or δ>0 or p>0, the threshold parameter ˆR0>1. This means that our control strategies can reduce the spread of FHMD in mainland China, but the disease may not go extinct in mainland China. To eliminate the disease, it is necessary to reduce the rates β1,β2,σ,ˉβ1,ˉβ2 and γ1,γ3, but this is usually very difficult, in that the average contact rate of children may be hard to control and because in mainland China, medical conditions are difficult to improve over a short period.
It should be mentioned that we fitted our proposed model to the reported data and estimated some unknown parameters in each year, so that it is not necessary to consider seasonal infection variations of this disease. Note that HFMD is a typical seasonal disease, which is usually affected by temperature and humidity [19,30,33,36]. In the future, we will model periodic infection of the disease together with vaccination, contaminated environment, and quarantine. The authors thank the anonymous referees, whose careful reading, insights, valuable comments, and suggestions significantly enabled us to improve the quality of the paper.
H. Zhao is supported by the National Natural Science Foundation of China (No 11571170). D. Wu is supported by the Natural Science Foundation of Anhui Province of China (No 1608085MA14), and the Programs of Educational Commission of Anhui Province of China (No KJ2015A152). The authors thank anonymous reviewers for their valuable comments. Their comments have enhanced the clarity and the quality.
All authors declare no conflicts of interest in this paper.
The BP neural network algorithm for estimating parameters
The parameter estimation methods for nonlinear problem that are most often used in practical applications are the nonlinear least squares estimator, Metropolis-Hastings algorithm, and so on [47]. The nonlinear least squares estimator is a parameter estimation method of nonlinear model parameters based on the criterion of error squared and minimum [48]. The Metropolis-Hastings algorithm estimates the posterior distributions of the parameters and the latent variables by using Markov chain Monte Carlo methods, which usually assumes that the sample satisfies certain probability distribution such as normal distribution, Gaussian distribution, chi-square distribution and so on [49]. Possibly due to limited by sample size, prior distribution assumptions or other constraints, the above two algorithms are used to estimate the unknown parameters of system (2.1) are not well, such as between the reported HFMD cases in mainland China and the simulation of I(t) of system (2.1) in 2017, the fitting errors ER with respect to the nonlinear least squares estimator (use the Fmincon function in Matlab) and metropolis-Hastings algorithm (use the source code in [47]) are 4.03×10−4 and 7.46×10−5, respectively, which are much larger than that of our estimated algorithm based on BP neural network (see Table 3). Because the neural network is not limited by the hypothesis of sample size and prior probability distribution, we design an algorithm based on BP neural network [50], which is one of the most widely neural network used and highly efficient [50], to estimate unknown parameters of system (2.1).
The topology of the BP neural network is shown in Figure 10. The BP neural network consists of three layers: input layer, hidden layer and output layer. The number of nodes in the input layer, the hidden layer and the output layer are 12, 93 and 8, respectively. For simplicity, we assume that θ=(θ1,θ2,...,θm)T denotes the vector of unknown parameters of system (2.1), where each element value must be set in reasonable interval. For example, set θ=(β1,β2,σ,ˉβ1,ˉβ2,ˉσ,ρ,k) in 2017 seen in Table 2. Let I=(I1,I2,...,I12)T and ˆI=(ˆI1,ˆI2,...,ˆI12)T. Taking the year of 2017 as an example, the estimation algorithm is described as follows:
Step1 Generate sample data: Generate n group sample data θi=(θi1,θi2,...,θim)T, i=1,2,...,n by Latin hypercube sampling. Then, taking θi into system (2.1), we can also obtain n group numerically computed data Ii=(Ii1,Ii2,...,Ii12)T, i=1,2,...,n seen in Table 2;
Step2 Use BP neural network to train data: Set ˉP=(I1,I2,...,In) and ˉT=(θ1,θ2,...,θn), and each element of ˉP and ˉT is normalized into interval [−1,1]. Then, taking ˉP as input vector and ˉT as output vector, a three-layers BP neural network is used to train dataset, and let Net be the training completed network, which the training error is less than the setting value. The detailed process of BP neural network can be seen in [50], and its specific settings are described below;
Step3 : Estimate parameters: First, taking reported data ˆI=(ˆI1,ˆI2,...,ˆI12)T of HFMD as the input vector into the training completed network Net, we can gain a output forecast vector θ∗=(θ∗1,θ∗2,...,θ∗m)T. Then, taking θ∗ into system (2.1), it get the simulation of Ii. Finally, we set a small positive content ε=0.0001 to estimate the error function ER (4.1). if ER<ε, the estimated parameters θ∗ are reasonable, otherwise it goes to step 1 and reset the settings of BP neural network.
Figure 11 shows n group numerically computed symptomatic data Ii of system (2.1), i=1,2,...,n, which are generated in step 1. In step 2, by using Matlab 2014b toolbox, the main settings of BP neural network are described as: (1) The transfer functions of hidden layer and output layer are tansig and purelin, respectively; (2) The network training function is trainlm; (3) The weight/bias learning function is learngdm; (4) The training error function is mse (mean squared error), and its value is 0.01; (5) The maximum iterations is 5000. Figure 12 shows the iteration process of the BP neural network, which the network complete training data before the setting maximum iterations 5000.
[1] | F. C. Tseng, H. C. Huang, C. Y. Chi, T. L. Lin, C. C. Liu, J. W. Jian, L. C. Hsu, H. S. Wu, J. Y. Yang and Y. W. Chang, Epidemiological survey of enterovirus infections occurring in Taiwan between 2000 and 2005: analysis of sentinel physician surveillance data, J. Med. Virol., 79 (2007), 1850–1860. |
[2] | Q. Zhu, Y. Hao, J. Ma, S. Yu and Y. Wang, Surveillance of hand, foot, and mouth disease in mainland China (2008-2009), Biomed. Environ. Sci., 24 (2011), 349–356. |
[3] | Chinese Center for Disease Control and Prevention (CCDC), National Public Health Statistical Data. 2018 Available from: http://www.chinacdc.cn/tjsj/fdcrbbg/index.html. |
[4] | N. J. Schmidt, E. H. Lennette and H. H. Ho, An apparently new enterovirus isolated from patients with disease of the central nervous system, J. Infect. Dis., 129 (1974), 304–309. |
[5] | G. L. Repass, W. C. Palmer and F. F. Stancampiano, Hand, foot and mouth disease: identifying and managing an acute viral syndrome, Clev. Clin. J. Med., 81 (2014), 537–543. |
[6] | M. Cabrerizo, D. Tarragó, C. Muñoz-Almagro, A. E. Del, M. Domínguez-Gil, J. M. Eiros, I. López-Miragaya, C. P´erez, J. Reina and A. Otero, Molecular epidemiology of enterovirus 71, coxsackievirus A16 and A6 associated with hand, foot and mouth disease in Spain, Clin. Microbiol. Infec., 20 (2014), O150–O156. |
[7] | S. Ljubin-Sternak, V. Slavic-Vrzic, T. Vilibić-Č avlek and I. Gjenero-Margan, Outbreak of hand, foot and mouth disease caused by coxsackie A16 virus in a childcare center in croatia, European Communicable Disease Bulletin, 21 (2011), 9–11. |
[8] | N. Sarma, A. Sarkar, A. Mukherjee, A. Ghosh, S. Dhar and R. Malakar, Epidemic of hand, foot and mouth disease in west bengal, india in august, 2007: a multicentric study, Indian J. Dermatol., 54 (2009), 26–30. |
[9] | O. M. How,W. S. Chang, M. Anand, P. Yuwana, P. David, C. Daniella, D. S. Sylvia, C. C. Hee, T. P. Hooi and C. M. Jane, Identification and validation of clinical predictors for the risk of neurological involvement in children with hand, foot, and mouth disease in Sarawak, Bmc Infect. Dis., 9 (2009), 3–3. |
[10] | T. Fujimoto, M. Chikahira, S. Yoshida, H. Ebira, A. Hasegawa, A. Totsuka and O. Nishio, Outbreak of central nervous system disease associated with hand, foot, and mouth disease in Japan during the summer of 2000: detection and molecular epidemiology of enterovirus 71, Microbiol. Immunol., 46 (2002), 621–627. |
[11] | J.Wang, Z. Cao, D. D. Zeng, Q.Wang, X.Wang and H. Qian, Epidemiological analysis, detection, and comparison of space-time patterns of Beijing hand-foot-mouth disease (2008-2012), PLoS One, 9 (2014). Article ID: e92745. |
[12] | W. S. Ryu, B. Kang, J. Hong, S. Hwang, J. Kim and D. S. Cheon, Clinical and etiological characteristics of enterovirus 71-related diseases during a recent 2-year period in Korea, J. Clin. Microbiol., 48 (2010), 2490–2494. |
[13] | J. Cheng, J.Wu, Z. Xu, R. Zhu, X.Wang, K. Li, L.Wen, H. Yang and H. Su, Associations between extreme precipitation and childhood hand, foot and mouth disease in urban and rural areas in Hefei, China, Sci. Total Environ., 497 (2014), 484–490. |
[14] | H. X. Nguyen, C. Chu, H. L. T. Nguyen, H. T. Nguyen, C. M. Do, S. Rutherford and D. Phung, Temporal and spatial analysis of hand, foot, and mouth disease in relation to climate factors: A study in the Mekong Delta region, Vietnam, Sci. Total Environ., 581 (2017), 766–772. |
[15] | F. Gou, X. Liu, X. Ren, D. Liu, H. Liu, K. Wei, X. Yang, Y. Cheng, Y. Zheng and X. Jiang, Socioecological factors and hand, foot and mouth disease in dry climate regions: a Bayesian spatial approach in Gansu, China, Int. J. Biometeorol., 61 (2017), 137–147. |
[16] | W. Jing, T. Hu, D. Sun, S. Ding, M. J. Carr, W. Xing, S. Li, X. Wang and W. Shi, Epidemiological characteristics of hand, foot, and mouth disease in Shandong, China, 2009-2016, Sci. Rep., 7 (2017). Article ID: 8900. |
[17] | Y. Weng, W. Chen, W. He, M. Huang, Y. Zhu and Y. Yan, Serotyping and genetic characterization of hand, foot, and mouth disease (HFMD)-associated enteroviruses of no-ev71 and non-cva16 circulating in fujian, china, 2011-2015, Med. Sci. Monitor, 23 (2017), 2508–2518. |
[18] | National Health and Family Planning Commission of the Peoples Republic of China (NHFPC), China Health Statistical Yearbook. 2018 Available from: http://www.moh.gov.cn/zwgk/yqbb3/ejlist.shtml. |
[19] | Z. Chang, F. Liu, L. Bin, Z. Wang and L. Zeng, Analysis on surveillance data of hand, foot and mouth disease in China, January - May 2017, Disease Surveillance, 32 (2017), 447–452. |
[20] | Y. Cao, Z. Hong, L. Jin, O. U. Jian-Ming and R. Hong, Surveillance of hand, foot and mouth disease in China, 2011-2012, Disease Surveillance, 28 (2013), 975–980. |
[21] | J. Sun, Z. Chang, L. Wang and L. Wang, Analysis on the epidemic situation of hand, foot and mouth disease in China in January - March, 2013, Practical Prev. Med., 21 (2014), 183–186. |
[22] | J. T. Wu, M. Jit, Y. Zheng, K. Leung and W. Xing, Routine pediatric enterovirus 71 vaccination in China: a cost-effectiveness analysis, Plos Med., 13 (2016). Article ID: e1002013. |
[23] | S. Takahashi, Q. Liao, T. P. Van Boeckel, W. Xing, J. Sun, V. Y. Hsiao, C. J. Metcalf, Z. Chang, F. Liu and J. Zhang, Hand, foot, and mouth disease in China: modeling epidemic dynamics of enterovirus serotypes and implications for vaccination, Plos Med., 13 (2016). Article ID: e1001958. |
[24] | N. Ziyadi, A male-female mathematical model of human papillomavirus (HPV) in African American population, Math. Biosci. Eng., 14 (2017), 339–358. |
[25] | S. Liu, Y. Li, Y. Bi, and Q. Huang, Mixed vaccination strategy for the control of tuberculosis: A case study in China, Math. Biosci. Eng., 14 (2017), 695–708. |
[26] | M. L. Manyombe, J. Mbang, J. Lubuma and B. Tsanou, Global dynamics of a vaccination model for infectious diseases with asymptomatic carriers, Math. Biosci., 13 (2016), 813–840. |
[27] | Y. C. Wang and F. C. Sung, Modeling the infections for Enteroviruses in Taiwan, Taipei: Institute of Environmental Health (2006). Article ID: 228559790. |
[28] | F. C. S. Tiing and J. Labadin, A simple deterministic model for the spread of hand, foot and mouth disease (HFMD) in Sarawak, 2008 Second Asia International Conference on Modelling & Simulation, (2008), 947–952. |
[29] | N. Roy, Mathematical modeling of hand-foot-mouth disease: quarantine as a control measure, Int. J. Adv. Sci. Eng. Technol. Res., 1 (2012), 1–11. |
[30] | Y. Ma, M. Liu, Q. Hou and J. Zhao, Modelling seasonal HFMD with the recessive infection in Shandong, China, Math. Biosci. Eng., 10 (2013), 1159–1171. |
[31] | J.Wang, Y. Xiao and R. A. Cheke, Modelling the effects of contaminated environments on HFMD infections in mainland China, Marriage Fam Rev, 35 (2016), 77–97. |
[32] | J. Wang, Y. Xiao and Z. Peng, Modelling seasonal HFMD infections with the effects of contaminated environments in mainland China, Appl. Math. Comput., 271 (2016), 615–627. |
[33] | Y. Zhu, B. Xu, X. Lian, W. Lin, Z. Zhou and W. Wang, A hand-foot-and-mouth disease model with periodic transmission rate in Wenzhou, China, Abstr. Appl. Anal., 15 (2014), 1–11. |
[34] | Y. Li, J. Zhang and X. Zhang, Modelling and preventive measures of hand, foot and mouth disease (HFMD) in China, Int. J. Environ. Res. Pub. Heal., 11 (2014), 3108–3117. |
[35] | Y. Li, L. Wang, L. Pang and S. Liu, The data fitting and optimal control of a hand, foot and mouth disease (HFMD) model with stage structure, Appl. Math. Comput., 276 (2016), 61–74. |
[36] | J. Liu, Threshold dynamics for a HFMD epidemic model with periodic transmission rate, Nonlinear Dynam., 64 (2011), 89–95. |
[37] | J. Y. Yang, Y. Chen and F. Q. Zhang, Stability analysis and optimal control of a hand-foot-mouth disease (HFMD) model, J. Appl. Math. Comput., 41 (2013), 99–117. |
[38] | G. P. Samanta, Analysis of a delayed hand-foot-mouth disease epidemic model with pulse vaccination, Syst. Sci. Control Eng., 2 (2014), 61–73. |
[39] | R. Viriyapong and S. Wichaino, Mathematical modeling of hand, foot and mouth disease in the Northern Thailand, Far East J. Math. Sci., 100 (2016), 805–820. |
[40] | S. Sharma and G. P. Samanta, Analysis of a hand-foot-mouth disease model, Int. J. Biomath., 10 (2017). Article ID: 1750016. |
[41] | P. V. D. Driessche and J. Watmough, Reproduction numbers and sub-threshold endemic equilibria for compartmental models of disease, Math. Biosci., 180 (2002), 29–48. |
[42] | W. M. Hirsch, H. Hanisch and J. P. Gabriel, Differential equation models of some parasitic infections: methods for the study of asymptotic behavior, Commun. Pure. Appl. Math., 38 (1985), 733-753. |
[43] | X. Zhao, Uniform persistence and periodic coexistence states in infinite-dimensional periodic semiflows with applications, Can. Appl. Math. Q., 3 (1995), 473–495. |
[44] | W. Wang and X. Zhao, An epidemic model in a patchy environment, Math. Biosci., 190 (2004), 97–112. |
[45] | National Bureau of Statistics of China (NBSC), China Demographic Yearbook of 2013, 2014, 2015, 2016, 2017. 2018 Available from: http://www.stats.gov.cn/tjsj/ndsj/. |
[46] | S. Marino, I. B. Hogue, C. J. Ray and D. E. Kirschner, A methodology for performing global uncertainty and sensitivity analysis in systems biology, J. Theor. Biol., 254 (2008), 178–196. |
[47] | Y. Xiao, Y. Zhou and S. Tang, Principles of biological mathematics, Xi'an Jiaotong University Press, 2012. |
[48] | H. O. Hartley, A. Booker, Nonlinear Least Squares Estimation, Ann. Math. Stat., 36 (1965), 638– 650. |
[49] | G. Arminger and B. O. Muth´en, A Bayesian approach to nonlinear latent variable models using the Gibbs sampler and the Metropolis-Hastings algorithm, Psychometrika, 63 (1998), 271–300. |
[50] | S. S. Haykin, Neural networks and learning machines, China Machine Press, 2009. |
1. | David M. Brown, Yun Zhang, Richard H. Scheuermann, Epidemiology and Sequence-Based Evolutionary Analysis of Circulating Non-Polio Enteroviruses, 2020, 8, 2076-2607, 1856, 10.3390/microorganisms8121856 | |
2. | Lei Shi, Hongyong Zhao, Daiyong Wu, Dynamical analysis for a reaction-diffusion HFMD model with nonsmooth saturation treatment function, 2021, 95, 10075704, 105593, 10.1016/j.cnsns.2020.105593 | |
3. | Lei Shi, Hongyong Zhao, Daiyong Wu, Modeling Periodic HFMD with the Effect of Vaccination in Mainland China, 2020, 2020, 1076-2787, 1, 10.1155/2020/8763126 | |
4. | Hongyong Zhao, Lei Shi, Jing Wang, Kai Wang, A stage structure HFMD model with temperature-dependent latent period, 2021, 93, 0307904X, 745, 10.1016/j.apm.2021.01.010 | |
5. | Lei Shi, Hongyong Zhao, Daiyong Wu, A reaction-diffusion HFMD model with nonsmooth treatment function, 2021, 2021, 1687-1847, 10.1186/s13662-021-03294-z | |
6. | Jia Rui, Kaiwei Luo, Qiuping Chen, Dexing Zhang, Qinglong Zhao, Yanhong Zhang, Xiongjie Zhai, Zeyu Zhao, Siyu Zhang, Yuxue Liao, Shixiong Hu, Lidong Gao, Zhao Lei, Mingzhai Wang, Yao Wang, Xingchun Liu, Shanshan Yu, Fang Xie, Jia Li, Ruoyun Liu, Yi-Chen Chiang, Benhua Zhao, Yanhua Su, Xu-Sheng Zhang, Tianmu Chen, Wen-Ping Guo, Early warning of hand, foot, and mouth disease transmission: A modeling study in mainland, China, 2021, 15, 1935-2735, e0009233, 10.1371/journal.pntd.0009233 | |
7. | Jiaxu Le, Jie Hong, Zheng Zhao, Yue Chen, Yi Hu, Zhaorui Chang, Zhijie Zhang, Age-specific transmission for different virus serotypes of hand, foot and mouth disease and the impact of interventions in East China, 2009–2015, 2022, 8, 24058440, e12042, 10.1016/j.heliyon.2022.e12042 | |
8. | Yi Xu, Jinmin Ma, Wenjie Ouyang, Rosary Sin Yu Yao, Wei Cao, Jiandong Li, Rongrong Zou, Chunxiao Fang, Fansen Zeng, Fengxia Yang, Xinfa Wang, Jing Yuan, Huimin Xia, Hui Wang, Sitang Gong, Yingxia Liu, Suppression of innate and acquired immunity in severe hand foot and mouth disease caused by EV71 infections in children, 2023, 248, 15216616, 109260, 10.1016/j.clim.2023.109260 | |
9. | Suvankar Majee, Soovoojeet Jana, Dhiraj Kumar Das, T.K. Kar, Global dynamics of a fractional-order HFMD model incorporating optimal treatment and stochastic stability, 2022, 161, 09600779, 112291, 10.1016/j.chaos.2022.112291 | |
10. | Beibei Zhang, Lai Zhang, Zhi Ling, The asymptotic behavior of bacterial and viral diseases model on a growing domain, 2021, 0003-6811, 1, 10.1080/00036811.2021.1999421 | |
11. | Siti Nurleena Abu Mansor, Majid Khan Majahar Ali, Farah Aini Abdullah, 2024, 3150, 0094-243X, 030019, 10.1063/5.0228542 | |
12. | You Zhou, Beibei Zhang, Zhi Ling, DYNAMICAL BEHAVIOR OF THE FECAL-ORAL TRANSMISSION DISEASES MODEL ON A T-PERIODIC EVOLUTION DOMAIN, 2024, 14, 2156-907X, 717, 10.11948/20230025 | |
13. | Changlei Tan, Shuang Li, Yong Li, Zhihang Peng, Dynamic modeling and data fitting of climatic and environmental factors and people's behavior factors on hand, foot, and mouth disease (HFMD) in Shanghai, China, 2023, 9, 24058440, e18212, 10.1016/j.heliyon.2023.e18212 | |
14. | Li Jia, Hongwu Tan, Hui Cao, Analysis of Dynamics of a Recurrent Infectious Disease SIRS Model with Age Structure and Two Delays, 2024, 34, 0218-1274, 10.1142/S0218127424501281 | |
15. | Quanman Hu, Yaqi Xie, Fucang Ji, Fei Zhao, Xiaoru Song, Saiwei Lu, Zijie Li, Juan Geng, Haiyan Yang, Jinzhao Long, Yuefei Jin, Shuaiyin Chen, Guangcai Duan, Effectiveness of EV-A71 Vaccine and Its Impact on the Incidence of Hand, Foot and Mouth Disease: A Systematic Review, 2024, 12, 2076-393X, 1028, 10.3390/vaccines12091028 | |
16. | Tongrui Zhang, Zhijie Zhang, Zhiyuan Yu, Qimin Huang, Daozhou Gao, Effects of behaviour change on HFMD transmission, 2023, 17, 1751-3758, 10.1080/17513758.2023.2244968 | |
17. | Hui Wang, Weihua Li, Lei Shi, Gaofang Chen, Zhengwen Tu, Modeling and analysis of the effect of optimal virus control on the spread of HFMD, 2024, 14, 2045-2322, 10.1038/s41598-024-56839-z | |
18. | Yiming Tang, Hairong Lian, Shiwen Jing, Zhaohai Ma, Hopf Bifurcation of an Age-Structured Hand–Foot–Mouth Disease Model in a Contaminated Environment, 2024, 34, 0218-1274, 10.1142/S0218127424501967 | |
19. | Dongxue Yan, Yongxian Jin, Hui Cao, Yu Cao, Stability and Hopf Bifurcation for a delayed hand–foot–mouth disease model with continuous age-structure in the exposed class, 2025, 84, 14681218, 104310, 10.1016/j.nonrwa.2024.104310 | |
20. | Zuhur Alqahtani, Mahmoud H. DarAssi, Yousef AbuHour, Areej Almuneef, Assessing the Role of Vaccination in the Control of Hand, Foot, and Mouth Disease Transmission, 2025, 13, 2227-7390, 268, 10.3390/math13020268 | |
21. | Jiraporn Lamwong, Puntani Pongsumpun, Modeling the spread of hand, foot, and mouth disease using ABC fractional derivatives: a focus on environmental and vaccination impacts in children, 2025, 11, 2363-6203, 10.1007/s40808-024-02232-8 |
Parameter | Definition |
Λ | Recruitment rate |
β1,β2, and σ | Transmission rate from I(t), Ie(t), and W(t) to S(t) respectively |
ˉβ1, ˉβ2, and ˉσ | Transmission rate from I(t), Ie(t), and W(t) to V(t) respectively |
m | Disease-related death of HFMD |
d | Progression rate leaving the children group below the age of six |
k | Vaccinated rate of S(t) |
η1 and η2 | Rate from R(t) to S(t) and V(t) respectively |
1/α | Average incubation period |
ρ | Proportion of symptomatic infectious individuals |
γ1, γ2, and γ3 | Recovery rate of I(t), Ie(t), and Q(t) respectively |
p | Quarantine rate at which symptomatic infectious individuals I(t) enters into quarantine individuals Q(t) |
λ1 and λ2 | Virus shedding rate from symptomatic and asymptomatic infected individuals respectively |
δ | Clearance rate of pathogens W(t) |
Para. | 2015 | 2016 | 2017 | References |
Λ | 1382638 | 1488333 | 1518641 | [45] |
m | 6.46×10−5 | 3.42×10−4 | 1.27×10−4 | [3,18] |
β1 | 1.08×10−7 | 1.11×10−7 | 1.08×10−7 | Fitting |
β2 | 2.44×10−8 | 2.50×10−8 | 2.38×10−7 | Fitting |
σ | 7.61×10−11 | 7.51×10−11 | 7.6×10−11 | Fitting |
ˉβ1 | 0 | 6.76×10−8 | 6.58×10−8 | Fitting |
ˉβ2 | 0 | 1.38×10−8 | 1.38×10−8 | Fitting |
ˉσ | 0 | 5.65×10−11 | 4.47×10−11 | Fitting |
d | 1.42×10−2 | 1.28×10−2 | 1.31×10−2 | [45] |
k | 0 | 1.21×10−3 | 1.31×10−3 | Fitting |
η1 | 0.035 | 0.035 | 0.035 | [31] |
η2 | 0 | 1.5×10−6 | 1.5×10−6 | Assumption |
1/α | 4/30 | 4/30 | 4/30 | [28] |
ρ | 0.2294 | 0.2299 | 0.2316 | Fitting |
γ1 | 3.5293 | 3.5293 | 3.5293 | [28] |
γ2 | 3.5293 | 3.5293 | 3.5293 | [28] |
γ3 | 3.5293 | 3.5293 | 3.5293 | Assumption |
p | 0.0025 | 0.0025 | 0.0025 | [30] |
λ1 | 93.8 | 93.8 | 93.8 | [31] |
λ2 | 78.9 | 78.9 | 78.9 | [31] |
δ | 2.7 | 2.7 | 2.7 | [31] |
S(0) | 95020000 | 96288000 | 95820000 | [45] |
V(0) | 0 | 0 | 5×105 | Assumption |
E(0) | 300000 | 300000 | 300000 | Assumption |
I(0) | 57763 | 79499 | 77412 | [3,18] |
Ie(0) | 150000 | 150000 | 150000 | Assumption |
Q(0) | 0 | 0 | 0 | Assumption |
R(0) | 28000 | 28000 | 28000 | Assumption |
W(0) | 0 | 0 | 0 | Assumption |
Year | 2015 | 2016 | 2017 |
ER | 3.47×10−6 | 8.54×10−6 | 3.86×10−6 |
Year | 2015 | 2016 | 2017 |
R1 | 0.6651 | 0.6535 | 0.6377 |
R2 | 0.4011 | 0.4418 | 0.4066 |
R3 | 0.0515 | 0.0546 | 0.0532 |
R0 | 1.1177 | 1.1499 | 1.0975 |
Parameter | Distribution | PRCC | p-Value |
Λ | U(1.38×106,1.53×106) | 0.2692 | 2.49×10−5 |
β1 | U(1.05×10−7,1.25×10−7) | 0.9705 | 0 |
β2 | U(2.2×10−8,2.6×10−8) | 0.8901 | 0 |
σ | U(7.0×10−11,8.0×10−11) | 0.4059 | 0 |
ˉβ1 | U(6.5×10−8,7.0×10−8) | 0.5397 | 0 |
ˉβ2 | U(1.1×10−8,1.5×10−8) | 0.4250 | 0 |
ˉσ | U(4.0×10−11,6.0×10−11) | 0.0729 | 1.04×10−11 |
k | U(1.1×10−3,1.5×10−3) | -0.7439 | 0 |
d | U(1.2×10−2,1.5×10−2) | -0.1280 | 0.0013 |
α | U(7.3, 7.7) | 0.00173 | 0.0943 |
ρ | U(0.22, 0.25) | 0.1351 | 0 |
γ1 | U(3.4, 3.6) | -0.8908 | 0 |
γ3 | U(3.4, 3.6) | -0.8101 | 0 |
λ1 | U(85, 95) | 0.1833 | 3.44×10−15 |
λ2 | U(75, 85) | 0.6495 | 0 |
δ | U(2.5, 3.0) | -0.7123 | 0 |
m | U(5.0×10−5,4.0×10−4) | -0.00215 | 0.0029 |
p | U(2.0×10−3,3.0×10−3) | -0.5388 | 0 |
Parameter | Definition |
Λ | Recruitment rate |
β1,β2, and σ | Transmission rate from I(t), Ie(t), and W(t) to S(t) respectively |
ˉβ1, ˉβ2, and ˉσ | Transmission rate from I(t), Ie(t), and W(t) to V(t) respectively |
m | Disease-related death of HFMD |
d | Progression rate leaving the children group below the age of six |
k | Vaccinated rate of S(t) |
η1 and η2 | Rate from R(t) to S(t) and V(t) respectively |
1/α | Average incubation period |
ρ | Proportion of symptomatic infectious individuals |
γ1, γ2, and γ3 | Recovery rate of I(t), Ie(t), and Q(t) respectively |
p | Quarantine rate at which symptomatic infectious individuals I(t) enters into quarantine individuals Q(t) |
λ1 and λ2 | Virus shedding rate from symptomatic and asymptomatic infected individuals respectively |
δ | Clearance rate of pathogens W(t) |
Para. | 2015 | 2016 | 2017 | References |
Λ | 1382638 | 1488333 | 1518641 | [45] |
m | 6.46×10−5 | 3.42×10−4 | 1.27×10−4 | [3,18] |
β1 | 1.08×10−7 | 1.11×10−7 | 1.08×10−7 | Fitting |
β2 | 2.44×10−8 | 2.50×10−8 | 2.38×10−7 | Fitting |
σ | 7.61×10−11 | 7.51×10−11 | 7.6×10−11 | Fitting |
ˉβ1 | 0 | 6.76×10−8 | 6.58×10−8 | Fitting |
ˉβ2 | 0 | 1.38×10−8 | 1.38×10−8 | Fitting |
ˉσ | 0 | 5.65×10−11 | 4.47×10−11 | Fitting |
d | 1.42×10−2 | 1.28×10−2 | 1.31×10−2 | [45] |
k | 0 | 1.21×10−3 | 1.31×10−3 | Fitting |
η1 | 0.035 | 0.035 | 0.035 | [31] |
η2 | 0 | 1.5×10−6 | 1.5×10−6 | Assumption |
1/α | 4/30 | 4/30 | 4/30 | [28] |
ρ | 0.2294 | 0.2299 | 0.2316 | Fitting |
γ1 | 3.5293 | 3.5293 | 3.5293 | [28] |
γ2 | 3.5293 | 3.5293 | 3.5293 | [28] |
γ3 | 3.5293 | 3.5293 | 3.5293 | Assumption |
p | 0.0025 | 0.0025 | 0.0025 | [30] |
λ1 | 93.8 | 93.8 | 93.8 | [31] |
λ2 | 78.9 | 78.9 | 78.9 | [31] |
δ | 2.7 | 2.7 | 2.7 | [31] |
S(0) | 95020000 | 96288000 | 95820000 | [45] |
V(0) | 0 | 0 | 5×105 | Assumption |
E(0) | 300000 | 300000 | 300000 | Assumption |
I(0) | 57763 | 79499 | 77412 | [3,18] |
Ie(0) | 150000 | 150000 | 150000 | Assumption |
Q(0) | 0 | 0 | 0 | Assumption |
R(0) | 28000 | 28000 | 28000 | Assumption |
W(0) | 0 | 0 | 0 | Assumption |
Year | 2015 | 2016 | 2017 |
ER | 3.47×10−6 | 8.54×10−6 | 3.86×10−6 |
Year | 2015 | 2016 | 2017 |
R1 | 0.6651 | 0.6535 | 0.6377 |
R2 | 0.4011 | 0.4418 | 0.4066 |
R3 | 0.0515 | 0.0546 | 0.0532 |
R0 | 1.1177 | 1.1499 | 1.0975 |
Parameter | Distribution | PRCC | p-Value |
Λ | U(1.38×106,1.53×106) | 0.2692 | 2.49×10−5 |
β1 | U(1.05×10−7,1.25×10−7) | 0.9705 | 0 |
β2 | U(2.2×10−8,2.6×10−8) | 0.8901 | 0 |
σ | U(7.0×10−11,8.0×10−11) | 0.4059 | 0 |
ˉβ1 | U(6.5×10−8,7.0×10−8) | 0.5397 | 0 |
ˉβ2 | U(1.1×10−8,1.5×10−8) | 0.4250 | 0 |
ˉσ | U(4.0×10−11,6.0×10−11) | 0.0729 | 1.04×10−11 |
k | U(1.1×10−3,1.5×10−3) | -0.7439 | 0 |
d | U(1.2×10−2,1.5×10−2) | -0.1280 | 0.0013 |
α | U(7.3, 7.7) | 0.00173 | 0.0943 |
ρ | U(0.22, 0.25) | 0.1351 | 0 |
γ1 | U(3.4, 3.6) | -0.8908 | 0 |
γ3 | U(3.4, 3.6) | -0.8101 | 0 |
λ1 | U(85, 95) | 0.1833 | 3.44×10−15 |
λ2 | U(75, 85) | 0.6495 | 0 |
δ | U(2.5, 3.0) | -0.7123 | 0 |
m | U(5.0×10−5,4.0×10−4) | -0.00215 | 0.0029 |
p | U(2.0×10−3,3.0×10−3) | -0.5388 | 0 |